
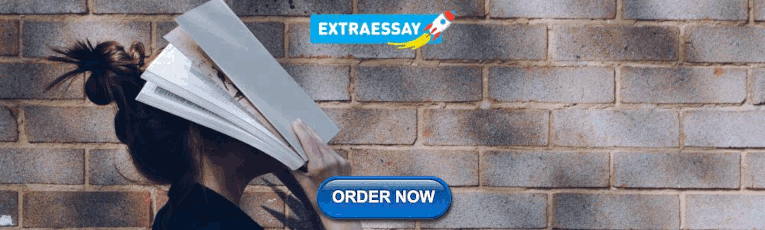
1.9: Data Collection by Experiments
Chapter 1: understanding statistics, chapter 2: summarizing and visualizing data, chapter 3: measure of central tendency, chapter 4: measures of variation, chapter 5: measures of relative standing, chapter 6: probability distributions, chapter 7: estimates, chapter 8: distributions, chapter 9: hypothesis testing, chapter 10: analysis of variance, chapter 11: correlation and regression, chapter 12: statistics in practice.
The JoVE video player is compatible with HTML5 and Adobe Flash. Older browsers that do not support HTML5 and the H.264 video codec will still use a Flash-based video player. We recommend downloading the newest version of Flash here, but we support all versions 10 and above.

There are several ways to collect data from a sample and extrapolate the data to the entire population.
The experimental study is a common method of data collection. Here, the samples are manipulated by applying some form of treatment before collecting data.
Suppose a researcher wants to know the effect of sunlight on plant growth.
In this experiment, one group of plants is exposed to sunlight, and another group is kept in the dark. After a month, the heights of the plants are recorded, and an inference– whether sunlight is required for plant growth–is drawn. Thus, in an experiment, the samples are manipulated before collecting the data.
Clinical trials are typical examples of data collection by experiments. Before a drug or treatment method is released for public use, its efficacy is tested on a small number of randomly selected groups of volunteers.
Here, one group of subjects is treated with specific doses of drugs or treatment methods, and a control group may be given a placebo. Then, the effects on disease symptoms are evaluated.
Data collection is a systematic method of obtaining, observing, measuring, and analyzing accurate information. An experimental study is a standard method of data collection that involves the manipulation of the samples by applying some form of treatment prior to data collection. It refers to manipulating one variable to determine its changes on another variable. The sample subjected to treatment is known as “experimental units.”
An example of the experimental method is a public clinical trial of a drug. For instance, to test the efficacy of a new drug effective in treating blood pressure, one needs to perform an experimental data collection. The new drug is given to a small number of randomly selected volunteers who suffer from chronic high blood pressure. One group of subjects is treated with specific doses of drugs or treatment methods, and a control group may be given a placebo. The subjects are monitored for a few weeks. The symptoms of disease treatment and after-effects of the drug are observed, and the data is collected. As this process involves modifying the subjects, it is categorized under the experimental method.
Another example is studying the effect of a particular fertilizer on the plant's growth. For this purpose, a few plants are taken and subjected to treatment with the new fertilizer. The growth of the plants is monitored daily for a few weeks, and the data is collected.
Get cutting-edge science videos from J o VE sent straight to your inbox every month.
mktb-description
We use cookies to enhance your experience on our website.
By continuing to use our website or clicking “Continue”, you are agreeing to accept our cookies.
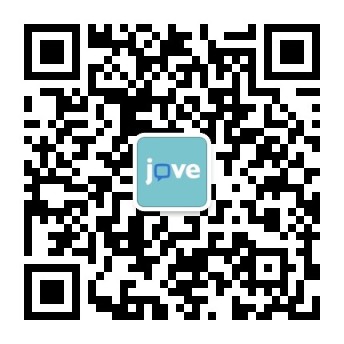
Have a language expert improve your writing
Run a free plagiarism check in 10 minutes, automatically generate references for free.
- Knowledge Base
- Methodology
- Data Collection Methods | Step-by-Step Guide & Examples
Data Collection Methods | Step-by-Step Guide & Examples
Published on 4 May 2022 by Pritha Bhandari .
Data collection is a systematic process of gathering observations or measurements. Whether you are performing research for business, governmental, or academic purposes, data collection allows you to gain first-hand knowledge and original insights into your research problem .
While methods and aims may differ between fields, the overall process of data collection remains largely the same. Before you begin collecting data, you need to consider:
- The aim of the research
- The type of data that you will collect
- The methods and procedures you will use to collect, store, and process the data
To collect high-quality data that is relevant to your purposes, follow these four steps.
Table of contents
Step 1: define the aim of your research, step 2: choose your data collection method, step 3: plan your data collection procedures, step 4: collect the data, frequently asked questions about data collection.
Before you start the process of data collection, you need to identify exactly what you want to achieve. You can start by writing a problem statement : what is the practical or scientific issue that you want to address, and why does it matter?
Next, formulate one or more research questions that precisely define what you want to find out. Depending on your research questions, you might need to collect quantitative or qualitative data :
- Quantitative data is expressed in numbers and graphs and is analysed through statistical methods .
- Qualitative data is expressed in words and analysed through interpretations and categorisations.
If your aim is to test a hypothesis , measure something precisely, or gain large-scale statistical insights, collect quantitative data. If your aim is to explore ideas, understand experiences, or gain detailed insights into a specific context, collect qualitative data.
If you have several aims, you can use a mixed methods approach that collects both types of data.
- Your first aim is to assess whether there are significant differences in perceptions of managers across different departments and office locations.
- Your second aim is to gather meaningful feedback from employees to explore new ideas for how managers can improve.
Prevent plagiarism, run a free check.
Based on the data you want to collect, decide which method is best suited for your research.
- Experimental research is primarily a quantitative method.
- Interviews , focus groups , and ethnographies are qualitative methods.
- Surveys , observations, archival research, and secondary data collection can be quantitative or qualitative methods.
Carefully consider what method you will use to gather data that helps you directly answer your research questions.
Method | When to use | How to collect data |
---|---|---|
Experiment | To test a causal relationship. | Manipulate variables and measure their effects on others. |
Survey | To understand the general characteristics or opinions of a group of people. | Distribute a list of questions to a sample online, in person, or over the phone. |
Interview/focus group | To gain an in-depth understanding of perceptions or opinions on a topic. | Verbally ask participants open-ended questions in individual interviews or focus group discussions. |
Observation | To understand something in its natural setting. | Measure or survey a sample without trying to affect them. |
Ethnography | To study the culture of a community or organisation first-hand. | Join and participate in a community and record your observations and reflections. |
Archival research | To understand current or historical events, conditions, or practices. | Access manuscripts, documents, or records from libraries, depositories, or the internet. |
Secondary data collection | To analyse data from populations that you can’t access first-hand. | Find existing datasets that have already been collected, from sources such as government agencies or research organisations. |
When you know which method(s) you are using, you need to plan exactly how you will implement them. What procedures will you follow to make accurate observations or measurements of the variables you are interested in?
For instance, if you’re conducting surveys or interviews, decide what form the questions will take; if you’re conducting an experiment, make decisions about your experimental design .
Operationalisation
Sometimes your variables can be measured directly: for example, you can collect data on the average age of employees simply by asking for dates of birth. However, often you’ll be interested in collecting data on more abstract concepts or variables that can’t be directly observed.
Operationalisation means turning abstract conceptual ideas into measurable observations. When planning how you will collect data, you need to translate the conceptual definition of what you want to study into the operational definition of what you will actually measure.
- You ask managers to rate their own leadership skills on 5-point scales assessing the ability to delegate, decisiveness, and dependability.
- You ask their direct employees to provide anonymous feedback on the managers regarding the same topics.
You may need to develop a sampling plan to obtain data systematically. This involves defining a population , the group you want to draw conclusions about, and a sample, the group you will actually collect data from.
Your sampling method will determine how you recruit participants or obtain measurements for your study. To decide on a sampling method you will need to consider factors like the required sample size, accessibility of the sample, and time frame of the data collection.
Standardising procedures
If multiple researchers are involved, write a detailed manual to standardise data collection procedures in your study.
This means laying out specific step-by-step instructions so that everyone in your research team collects data in a consistent way – for example, by conducting experiments under the same conditions and using objective criteria to record and categorise observations.
This helps ensure the reliability of your data, and you can also use it to replicate the study in the future.
Creating a data management plan
Before beginning data collection, you should also decide how you will organise and store your data.
- If you are collecting data from people, you will likely need to anonymise and safeguard the data to prevent leaks of sensitive information (e.g. names or identity numbers).
- If you are collecting data via interviews or pencil-and-paper formats, you will need to perform transcriptions or data entry in systematic ways to minimise distortion.
- You can prevent loss of data by having an organisation system that is routinely backed up.
Finally, you can implement your chosen methods to measure or observe the variables you are interested in.
The closed-ended questions ask participants to rate their manager’s leadership skills on scales from 1 to 5. The data produced is numerical and can be statistically analysed for averages and patterns.
To ensure that high-quality data is recorded in a systematic way, here are some best practices:
- Record all relevant information as and when you obtain data. For example, note down whether or how lab equipment is recalibrated during an experimental study.
- Double-check manual data entry for errors.
- If you collect quantitative data, you can assess the reliability and validity to get an indication of your data quality.
Data collection is the systematic process by which observations or measurements are gathered in research. It is used in many different contexts by academics, governments, businesses, and other organisations.
When conducting research, collecting original data has significant advantages:
- You can tailor data collection to your specific research aims (e.g., understanding the needs of your consumers or user testing your website).
- You can control and standardise the process for high reliability and validity (e.g., choosing appropriate measurements and sampling methods ).
However, there are also some drawbacks: data collection can be time-consuming, labour-intensive, and expensive. In some cases, it’s more efficient to use secondary data that has already been collected by someone else, but the data might be less reliable.
Quantitative research deals with numbers and statistics, while qualitative research deals with words and meanings.
Quantitative methods allow you to test a hypothesis by systematically collecting and analysing data, while qualitative methods allow you to explore ideas and experiences in depth.
Reliability and validity are both about how well a method measures something:
- Reliability refers to the consistency of a measure (whether the results can be reproduced under the same conditions).
- Validity refers to the accuracy of a measure (whether the results really do represent what they are supposed to measure).
If you are doing experimental research , you also have to consider the internal and external validity of your experiment.
In mixed methods research , you use both qualitative and quantitative data collection and analysis methods to answer your research question .
Operationalisation means turning abstract conceptual ideas into measurable observations.
For example, the concept of social anxiety isn’t directly observable, but it can be operationally defined in terms of self-rating scores, behavioural avoidance of crowded places, or physical anxiety symptoms in social situations.
Before collecting data , it’s important to consider how you will operationalise the variables that you want to measure.
Cite this Scribbr article
If you want to cite this source, you can copy and paste the citation or click the ‘Cite this Scribbr article’ button to automatically add the citation to our free Reference Generator.
Bhandari, P. (2022, May 04). Data Collection Methods | Step-by-Step Guide & Examples. Scribbr. Retrieved 1 July 2024, from https://www.scribbr.co.uk/research-methods/data-collection-guide/
Is this article helpful?
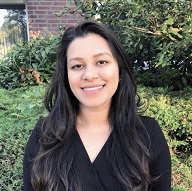
Pritha Bhandari
Other students also liked, qualitative vs quantitative research | examples & methods, triangulation in research | guide, types, examples, what is a conceptual framework | tips & examples.
- Skip to secondary menu
- Skip to main content
- Skip to primary sidebar
Statistics By Jim
Making statistics intuitive
Experimental Design: Definition and Types
By Jim Frost 3 Comments
What is Experimental Design?
An experimental design is a detailed plan for collecting and using data to identify causal relationships. Through careful planning, the design of experiments allows your data collection efforts to have a reasonable chance of detecting effects and testing hypotheses that answer your research questions.
An experiment is a data collection procedure that occurs in controlled conditions to identify and understand causal relationships between variables. Researchers can use many potential designs. The ultimate choice depends on their research question, resources, goals, and constraints. In some fields of study, researchers refer to experimental design as the design of experiments (DOE). Both terms are synonymous.

Ultimately, the design of experiments helps ensure that your procedures and data will evaluate your research question effectively. Without an experimental design, you might waste your efforts in a process that, for many potential reasons, can’t answer your research question. In short, it helps you trust your results.
Learn more about Independent and Dependent Variables .
Design of Experiments: Goals & Settings
Experiments occur in many settings, ranging from psychology, social sciences, medicine, physics, engineering, and industrial and service sectors. Typically, experimental goals are to discover a previously unknown effect , confirm a known effect, or test a hypothesis.
Effects represent causal relationships between variables. For example, in a medical experiment, does the new medicine cause an improvement in health outcomes? If so, the medicine has a causal effect on the outcome.
An experimental design’s focus depends on the subject area and can include the following goals:
- Understanding the relationships between variables.
- Identifying the variables that have the largest impact on the outcomes.
- Finding the input variable settings that produce an optimal result.
For example, psychologists have conducted experiments to understand how conformity affects decision-making. Sociologists have performed experiments to determine whether ethnicity affects the public reaction to staged bike thefts. These experiments map out the causal relationships between variables, and their primary goal is to understand the role of various factors.
Conversely, in a manufacturing environment, the researchers might use an experimental design to find the factors that most effectively improve their product’s strength, identify the optimal manufacturing settings, and do all that while accounting for various constraints. In short, a manufacturer’s goal is often to use experiments to improve their products cost-effectively.
In a medical experiment, the goal might be to quantify the medicine’s effect and find the optimum dosage.
Developing an Experimental Design
Developing an experimental design involves planning that maximizes the potential to collect data that is both trustworthy and able to detect causal relationships. Specifically, these studies aim to see effects when they exist in the population the researchers are studying, preferentially favor causal effects, isolate each factor’s true effect from potential confounders, and produce conclusions that you can generalize to the real world.
To accomplish these goals, experimental designs carefully manage data validity and reliability , and internal and external experimental validity. When your experiment is valid and reliable, you can expect your procedures and data to produce trustworthy results.
An excellent experimental design involves the following:
- Lots of preplanning.
- Developing experimental treatments.
- Determining how to assign subjects to treatment groups.
The remainder of this article focuses on how experimental designs incorporate these essential items to accomplish their research goals.
Learn more about Data Reliability vs. Validity and Internal and External Experimental Validity .
Preplanning, Defining, and Operationalizing for Design of Experiments

This phase of the design of experiments helps you identify critical variables, know how to measure them while ensuring reliability and validity, and understand the relationships between them. The review can also help you find ways to reduce sources of variability, which increases your ability to detect treatment effects. Notably, the literature review allows you to learn how similar studies designed their experiments and the challenges they faced.
Operationalizing a study involves taking your research question, using the background information you gathered, and formulating an actionable plan.
This process should produce a specific and testable hypothesis using data that you can reasonably collect given the resources available to the experiment.
- Null hypothesis : The jumping exercise intervention does not affect bone density.
- Alternative hypothesis : The jumping exercise intervention affects bone density.
To learn more about this early phase, read Five Steps for Conducting Scientific Studies with Statistical Analyses .
Formulating Treatments in Experimental Designs
In an experimental design, treatments are variables that the researchers control. They are the primary independent variables of interest. Researchers administer the treatment to the subjects or items in the experiment and want to know whether it causes changes in the outcome.
As the name implies, a treatment can be medical in nature, such as a new medicine or vaccine. But it’s a general term that applies to other things such as training programs, manufacturing settings, teaching methods, and types of fertilizers. I helped run an experiment where the treatment was a jumping exercise intervention that we hoped would increase bone density. All these treatment examples are things that potentially influence a measurable outcome.
Even when you know your treatment generally, you must carefully consider the amount. How large of a dose? If you’re comparing three different temperatures in a manufacturing process, how far apart are they? For my bone mineral density study, we had to determine how frequently the exercise sessions would occur and how long each lasted.
How you define the treatments in the design of experiments can affect your findings and the generalizability of your results.
Assigning Subjects to Experimental Groups
A crucial decision for all experimental designs is determining how researchers assign subjects to the experimental conditions—the treatment and control groups. The control group is often, but not always, the lack of a treatment. It serves as a basis for comparison by showing outcomes for subjects who don’t receive a treatment. Learn more about Control Groups .
How your experimental design assigns subjects to the groups affects how confident you can be that the findings represent true causal effects rather than mere correlation caused by confounders. Indeed, the assignment method influences how you control for confounding variables. This is the difference between correlation and causation .
Imagine a study finds that vitamin consumption correlates with better health outcomes. As a researcher, you want to be able to say that vitamin consumption causes the improvements. However, with the wrong experimental design, you might only be able to say there is an association. A confounder, and not the vitamins, might actually cause the health benefits.
Let’s explore some of the ways to assign subjects in design of experiments.
Completely Randomized Designs
A completely randomized experimental design randomly assigns all subjects to the treatment and control groups. You simply take each participant and use a random process to determine their group assignment. You can flip coins, roll a die, or use a computer. Randomized experiments must be prospective studies because they need to be able to control group assignment.
Random assignment in the design of experiments helps ensure that the groups are roughly equivalent at the beginning of the study. This equivalence at the start increases your confidence that any differences you see at the end were caused by the treatments. The randomization tends to equalize confounders between the experimental groups and, thereby, cancels out their effects, leaving only the treatment effects.
For example, in a vitamin study, the researchers can randomly assign participants to either the control or vitamin group. Because the groups are approximately equal when the experiment starts, if the health outcomes are different at the end of the study, the researchers can be confident that the vitamins caused those improvements.
Statisticians consider randomized experimental designs to be the best for identifying causal relationships.
If you can’t randomly assign subjects but want to draw causal conclusions about an intervention, consider using a quasi-experimental design .
Learn more about Randomized Controlled Trials and Random Assignment in Experiments .
Randomized Block Designs
Nuisance factors are variables that can affect the outcome, but they are not the researcher’s primary interest. Unfortunately, they can hide or distort the treatment results. When experimenters know about specific nuisance factors, they can use a randomized block design to minimize their impact.
This experimental design takes subjects with a shared “nuisance” characteristic and groups them into blocks. The participants in each block are then randomly assigned to the experimental groups. This process allows the experiment to control for known nuisance factors.
Blocking in the design of experiments reduces the impact of nuisance factors on experimental error. The analysis assesses the effects of the treatment within each block, which removes the variability between blocks. The result is that blocked experimental designs can reduce the impact of nuisance variables, increasing the ability to detect treatment effects accurately.
Suppose you’re testing various teaching methods. Because grade level likely affects educational outcomes, you might use grade level as a blocking factor. To use a randomized block design for this scenario, divide the participants by grade level and then randomly assign the members of each grade level to the experimental groups.
A standard guideline for an experimental design is to “Block what you can, randomize what you cannot.” Use blocking for a few primary nuisance factors. Then use random assignment to distribute the unblocked nuisance factors equally between the experimental conditions.
You can also use covariates to control nuisance factors. Learn about Covariates: Definition and Uses .
Observational Studies
In some experimental designs, randomly assigning subjects to the experimental conditions is impossible or unethical. The researchers simply can’t assign participants to the experimental groups. However, they can observe them in their natural groupings, measure the essential variables, and look for correlations. These observational studies are also known as quasi-experimental designs. Retrospective studies must be observational in nature because they look back at past events.
Imagine you’re studying the effects of depression on an activity. Clearly, you can’t randomly assign participants to the depression and control groups. But you can observe participants with and without depression and see how their task performance differs.
Observational studies let you perform research when you can’t control the treatment. However, quasi-experimental designs increase the problem of confounding variables. For this design of experiments, correlation does not necessarily imply causation. While special procedures can help control confounders in an observational study, you’re ultimately less confident that the results represent causal findings.
Learn more about Observational Studies .
For a good comparison, learn about the differences and tradeoffs between Observational Studies and Randomized Experiments .
Between-Subjects vs. Within-Subjects Experimental Designs
When you think of the design of experiments, you probably picture a treatment and control group. Researchers assign participants to only one of these groups, so each group contains entirely different subjects than the other groups. Analysts compare the groups at the end of the experiment. Statisticians refer to this method as a between-subjects, or independent measures, experimental design.
In a between-subjects design , you can have more than one treatment group, but each subject is exposed to only one condition, the control group or one of the treatment groups.
A potential downside to this approach is that differences between groups at the beginning can affect the results at the end. As you’ve read earlier, random assignment can reduce those differences, but it is imperfect. There will always be some variability between the groups.
In a within-subjects experimental design , also known as repeated measures, subjects experience all treatment conditions and are measured for each. Each subject acts as their own control, which reduces variability and increases the statistical power to detect effects.
In this experimental design, you minimize pre-existing differences between the experimental conditions because they all contain the same subjects. However, the order of treatments can affect the results. Beware of practice and fatigue effects. Learn more about Repeated Measures Designs .
Assigned to one experimental condition | Participates in all experimental conditions |
Requires more subjects | Fewer subjects |
Differences between subjects in the groups can affect the results | Uses same subjects in all conditions. |
No order of treatment effects. | Order of treatments can affect results. |
Design of Experiments Examples
For example, a bone density study has three experimental groups—a control group, a stretching exercise group, and a jumping exercise group.
In a between-subjects experimental design, scientists randomly assign each participant to one of the three groups.
In a within-subjects design, all subjects experience the three conditions sequentially while the researchers measure bone density repeatedly. The procedure can switch the order of treatments for the participants to help reduce order effects.
Matched Pairs Experimental Design
A matched pairs experimental design is a between-subjects study that uses pairs of similar subjects. Researchers use this approach to reduce pre-existing differences between experimental groups. It’s yet another design of experiments method for reducing sources of variability.
Researchers identify variables likely to affect the outcome, such as demographics. When they pick a subject with a set of characteristics, they try to locate another participant with similar attributes to create a matched pair. Scientists randomly assign one member of a pair to the treatment group and the other to the control group.
On the plus side, this process creates two similar groups, and it doesn’t create treatment order effects. While matched pairs do not produce the perfectly matched groups of a within-subjects design (which uses the same subjects in all conditions), it aims to reduce variability between groups relative to a between-subjects study.
On the downside, finding matched pairs is very time-consuming. Additionally, if one member of a matched pair drops out, the other subject must leave the study too.
Learn more about Matched Pairs Design: Uses & Examples .
Another consideration is whether you’ll use a cross-sectional design (one point in time) or use a longitudinal study to track changes over time .
A case study is a research method that often serves as a precursor to a more rigorous experimental design by identifying research questions, variables, and hypotheses to test. Learn more about What is a Case Study? Definition & Examples .
In conclusion, the design of experiments is extremely sensitive to subject area concerns and the time and resources available to the researchers. Developing a suitable experimental design requires balancing a multitude of considerations. A successful design is necessary to obtain trustworthy answers to your research question and to have a reasonable chance of detecting treatment effects when they exist.
Share this:

Reader Interactions
March 23, 2024 at 2:35 pm
Dear Jim You wrote a superb document, I will use it in my Buistatistics course, along with your three books. Thank you very much! Miguel
March 23, 2024 at 5:43 pm
Thanks so much, Miguel! Glad this post was helpful and I trust the books will be as well.
April 10, 2023 at 4:36 am
What are the purpose and uses of experimental research design?
Comments and Questions Cancel reply

AP® Statistics
Data collection methods: what to know for ap® statistics.
- The Albert Team
- Last Updated On: March 1, 2022
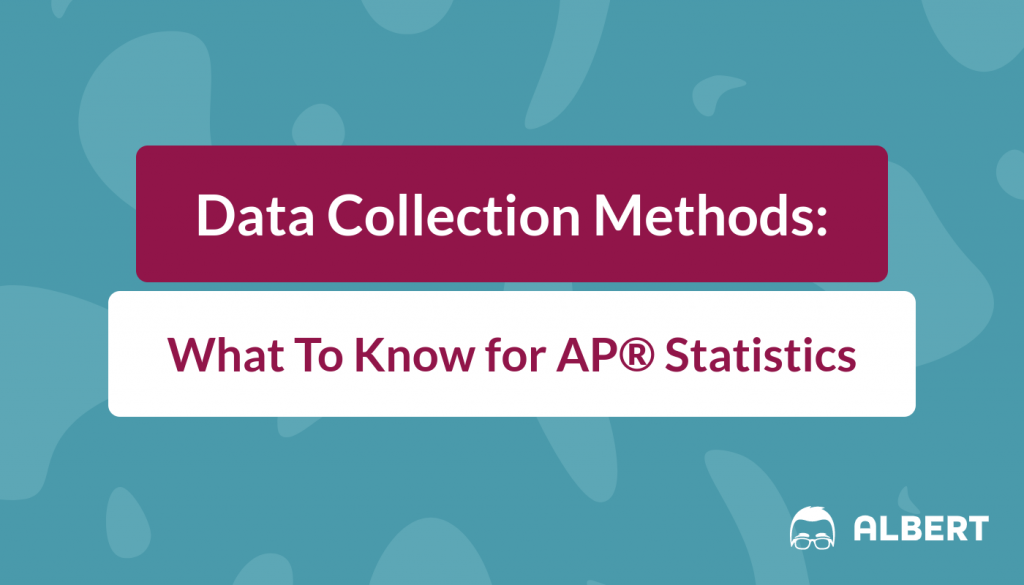
Introduction
When faced with a research problem, you need to collect, analyze and interpret data to answer your research questions. Examples of research questions that could require you to gather data include how many people will vote for a candidate, what is the best product mix to use and how useful is a drug in curing a disease. The research problem you explore informs the type of data you’ll collect and the data collection method you’ll use. In this article, we will explore various types of data, methods of data collection and advantages and disadvantages of each. After reading our review, you will have an excellent understanding of when to use each of the data collection methods we discuss.
Types of Data
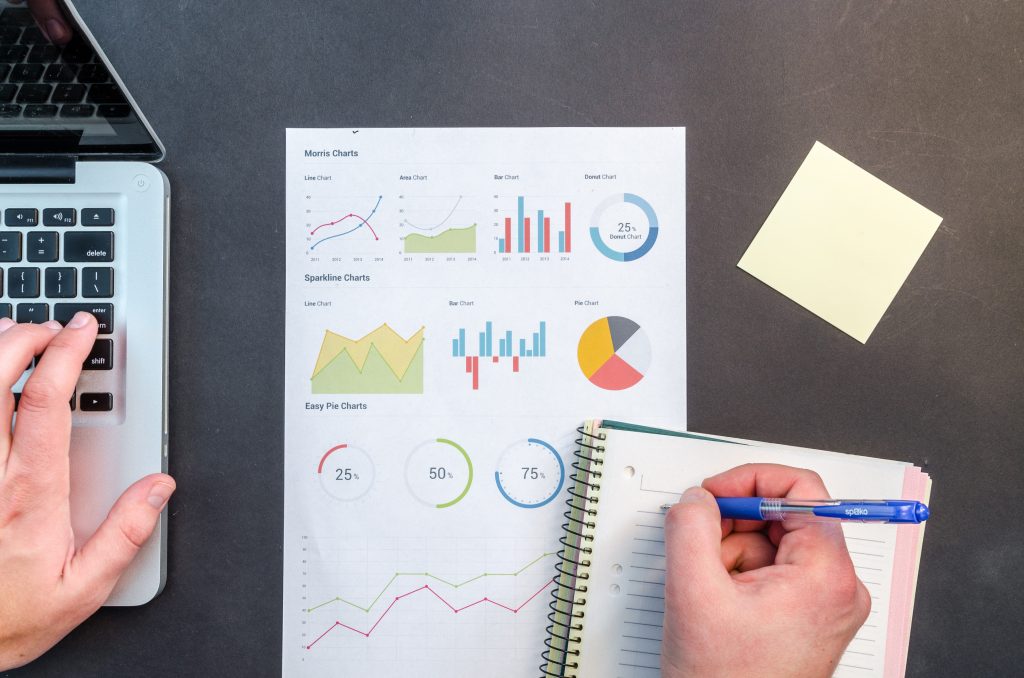
Quantitative Data
Data that is expressed in numbers and summarized using statistics to give meaningful information is referred to as quantitative data . Examples of quantitative data we could collect are heights, weights, or ages of students. If we obtain the mean of each set of measurements, we have meaningful information about the average value for each of those student characteristics.
Qualitative Data
When we use data for description without measurement, we call it qualitative data . Examples of qualitative data are student attitudes towards school, attitudes towards exam cheating and friendliness of students to teachers. Such data cannot be easily summarized using statistics.
Primary Data
When we obtain data directly from individuals, objects or processes, we refer to it as primary data . Quantitative or qualitative data can be collected using this approach. Such data is usually collected solely for the research problem to you will study. Primary data has several advantages. First, we tailor it to our specific research question, so there are no customizations needed to make the data usable. Second, primary data is reliable because you control how the data is collected and can monitor its quality. Third, by collecting primary data, you spend your resources in collecting only required data. Finally, primary data is proprietary, so you enjoy advantages over those who cannot access the data.
Despite its advantages, primary data also has disadvantages of which you need to be aware. The first problem with primary data is that it is costlier to acquire as compared to secondary data. Obtaining primary data also requires more time as compared to gathering secondary data.
Secondary Data
When you collect data after another researcher or agency that initially gathered it makes it available, you are gathering secondary data . Examples of secondary data are census data published by the US Census Bureau, stock prices data published by CNN and salaries data published by the Bureau of Labor Statistics.
One advantage to using secondary data is that it will save you time and money, although some data sets require you to pay for access. A second advantage is the relative ease with which you can obtain it. You can easily access secondary data from publications, government agencies, data aggregation websites and blogs. A third advantage is that it eliminates effort duplication since you can identify existing data that matches your needs instead of gather new data.
Despite the benefits it offers, secondary data has its shortcomings. One limitation is that secondary data may not be complete. For it to meet your research needs, you may need to enrich it with data from other sources. A second shortcoming is that you cannot verify the accuracy of secondary data, or the data may be outdated. A third challenge you face when using secondary data is that documentation may be incomplete or missing. Therefore, you may not be aware of any problems that happened in data collection which would otherwise influence its interpretation. Another challenge you may face when you decide to use secondary data is that there may be copyright restrictions.
Now that we’ve explained the various types of data you can collect when conducting research, we will proceed to look at methods used to collect primary and secondary data.
Methods Employed in Primary Data Collection
When you decide to conduct original research, the data you gather can be quantitative or qualitative. Generally, you collect quantitative data through sample surveys, experiments and observational studies. You obtain qualitative data through focus groups, in-depth interviews and case studies. We will discuss each of these data collection methods below and examine their advantages and disadvantages.
Sample Surveys
A survey is a data collection method where you select a sample of respondents from a large population in order to gather information about that population. The process of identifying individuals from the population who you will interview is known as sampling .
To gather data through a survey, you construct a questionnaire to prompt information from selected respondents. When creating a questionnaire, you should keep in mind several key considerations. First, make sure the questions and choices are unambiguous. Second, make sure the questionnaire will be completed within a reasonable amount of time. Finally, make sure there are no typographical errors. To check if there are any problems with your questionnaire, use it to interview a few people before administering it to all respondents in your sample. We refer to this process as pretesting.
Using a survey to collect data offers you several advantages. The main benefit is time and cost savings because you only interview a sample, not the large population. Another benefit is that when you select your sample correctly, you will obtain information of acceptable accuracy. Additionally, surveys are adaptable and can be used to collect data for governments, health care institutions, businesses and any other environment where data is needed.
A major shortcoming of surveys occurs when you fail to select a sample correctly; without an appropriate sample, the results will not accurately generalize the population.
Ways of Interviewing Respondents
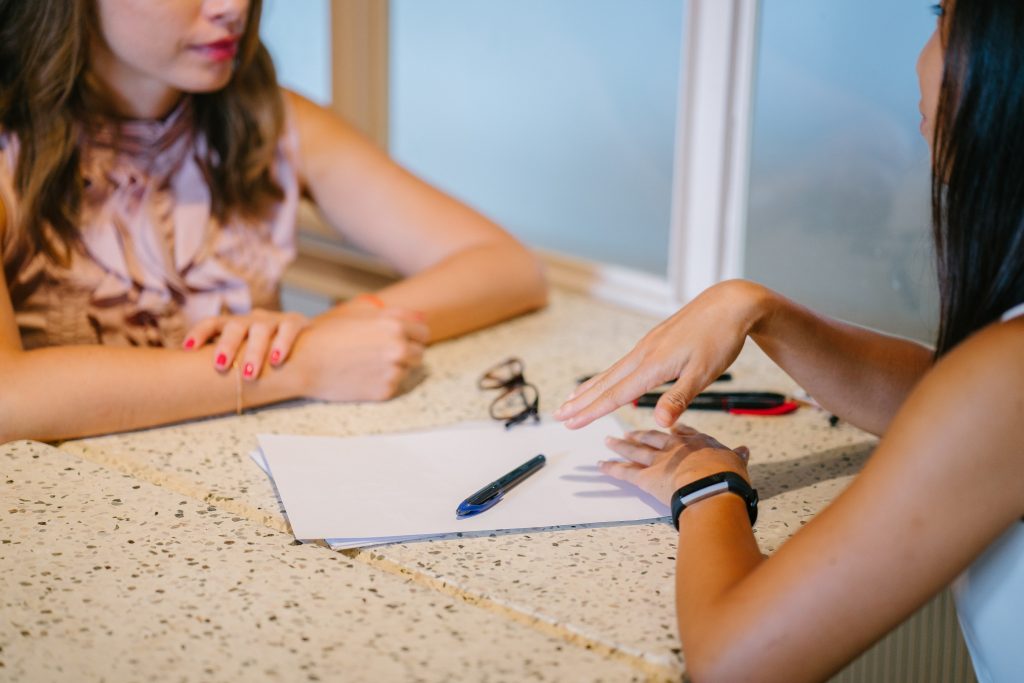
Once you have selected your sample and developed your questionnaire, there are several ways you can interview participants. Each approach has its advantages and disadvantages.
In-person Interviewing
When you use this method, you meet with the respondents face to face and ask questions. In-person interviewing offers several advantages. This technique has excellent response rates and enables you to conduct interviews that take a longer amount of time. Another benefit is you can ask follow-up questions to responses that are not clear.
In-person interviews do have disadvantages of which you need to be aware. First, this method is expensive and takes more time because of interviewer training, transport, and remuneration. A second disadvantage is that some areas of a population, such as neighborhoods prone to crime, cannot be accessed which may result in bias.
Telephone Interviewing
Using this technique, you call respondents over the phone and interview them. This method offers the advantage of quickly collecting data, especially when used with computer-assisted telephone interviewing. Another advantage is that collecting data via telephone is cheaper than in-person interviewing.
One of the main limitations with telephone interviewing it’s hard to gain the trust of respondents. Due to this reason, you may not get responses or may introduce bias. Since phone interviews are generally kept short to reduce the possibility of upsetting respondents, this method may also limit the amount of data you can collect.
Online Interviewing
With online interviewing, you send an email inviting respondents to participate in an online survey. This technique is used widely because it is a low-cost way of interviewing many respondents. Another benefit is anonymity; you can get sensitive responses that participants would not feel comfortable providing with in-person interviewing.
When you use online interviewing, you face the disadvantage of not getting a representative sample. You also cannot seek clarification on responses that are unclear.
Mailed Questionnaire
When you use this interviewing method, you send a printed questionnaire to the postal address of the respondent. The participants fill in the questionnaire and mail it back. This interviewing method gives you the advantage of obtaining information that respondents may be unwilling to give when interviewing in person.
The main limitation with mailed questionnaires is you are likely to get a low response rate. Keep in mind that inaccuracy in mailing address, delays or loss of mail could also affect the response rate. Additionally, mailed questionnaires cannot be used to interview respondents with low literacy, and you cannot seek clarifications on responses.
Focus Groups
When you use a focus group as a data collection method, you identify a group of 6 to 10 people with similar characteristics. A moderator then guides a discussion to identify attitudes and experiences of the group. The responses are captured by video recording, voice recording or writing—this is the data you will analyze to answer your research questions. Focus groups have the advantage of requiring fewer resources and time as compared to interviewing individuals. Another advantage is that you can request clarifications to unclear responses.
One disadvantage you face when using focus groups is that the sample selected may not represent the population accurately. Furthermore, dominant participants can influence the responses of others.
Observational Data Collection Methods
In an observational data collection method, you acquire data by observing any relationships that may be present in the phenomenon you are studying. There are four types of observational methods that are available to you as a researcher: cross-sectional, case-control, cohort and ecological.
In a cross-sectional study, you only collect data on observed relationships once. This method has the advantage of being cheaper and taking less time as compared to case-control and cohort. However, cross-sectional studies can miss relationships that may arise over time.
Using a case-control method, you create cases and controls and then observe them. A case has been exposed to a phenomenon of interest while a control has not. After identifying the cases and controls, you move back in time to observe how your event of interest occurs in the two groups. This is why case-control studies are referred to as retrospective. For example, suppose a medical researcher suspects a certain type of cosmetic is causing skin cancer. You recruit people who have used a cosmetic, the cases, and those who have not used the cosmetic, the controls. You request participants to remember the type of cosmetic and the frequency of its use. This method is cheaper and requires less time as compared to the cohort method. However, this approach has limitations when individuals you are observing cannot accurately recall information. We refer to this as recall bias because you rely on the ability of participants to remember information. In the cosmetic example, recall bias would occur if participants cannot accurately remember the type of cosmetic and number of times used.
In a cohort method, you follow people with similar characteristics over a period. This method is advantageous when you are collecting data on occurrences that happen over a long period. It has the disadvantage of being costly and requiring more time. It is also not suitable for occurrences that happen rarely.
The three methods we have discussed previously collect data on individuals. When you are interested in studying a population instead of individuals, you use an ecological method. For example, say you are interested in lung cancer rates in Iowa and North Dakota. You obtain number of cancer cases per 1000 people for each state from the National Cancer Institute and compare them. You can then hypothesize possible causes of differences between the two states. When you use the ecological method, you save time and money because data is already available. However the data collected may lead you to infer population relationships that do not exist.
Experiments
An experiment is a data collection method where you as a researcher change some variables and observe their effect on other variables. The variables that you manipulate are referred to as independent while the variables that change as a result of manipulation are dependent variables. Imagine a manufacturer is testing the effect of drug strength on number of bacteria in the body. The company decides to test drug strength at 10mg, 20mg and 40mg. In this example, drug strength is the independent variable while number of bacteria is the dependent variable. The drug administered is the treatment, while 10mg, 20mg and 40mg are the levels of the treatment.
The greatest advantage of using an experiment is that you can explore causal relationships that an observational study cannot. Additionally, experimental research can be adapted to different fields like medical research, agriculture, sociology, and psychology. Nevertheless, experiments have the disadvantage of being expensive and requiring a lot of time.
This article introduced you to the various types of data you can collect for research purposes. We discussed quantitative, qualitative, primary and secondary data and identified the advantages and disadvantages of each data type. We also reviewed various data collection methods and examined their benefits and drawbacks. Having read this article, you should be able to select the data collection method most appropriate for your research question. Data is the evidence that you use to solve your research problem. When you use the correct data collection method, you get the right data to solve your problem.
Looking for Statistics practice?
You can find thousands of practice questions on Albert.io. Albert.io lets you customize your learning experience to target practice where you need the most help. We’ll give you challenging practice questions to help you achieve mastery in Statistics.
Start practicing here .
Are you a teacher or administrator interested in boosting Statistics student outcomes?
Learn more about our school licenses here .
Interested in a school license?
12 thoughts on “data collection methods: what to know for ap® statistics”.
Great article.
Thanks for the great article
Happy to help!
Thank you for this article. Mostly I appreciate that part about methods of data collection and ways of interviewing respondents
Sure thing.
Thanks for elaborate explanation. Osiet agabo busitema university uganda
This sight is indeed fruitful in knowledge.
This article helps me to learn several data collection methods. Thank you!
You’re very welcome! Glad the article helped.
I mean what a great article
Thanks, John!
Comments are closed.
Popular Posts

AP® Score Calculators
Simulate how different MCQ and FRQ scores translate into AP® scores

AP® Review Guides
The ultimate review guides for AP® subjects to help you plan and structure your prep.

Core Subject Review Guides
Review the most important topics in Physics and Algebra 1 .
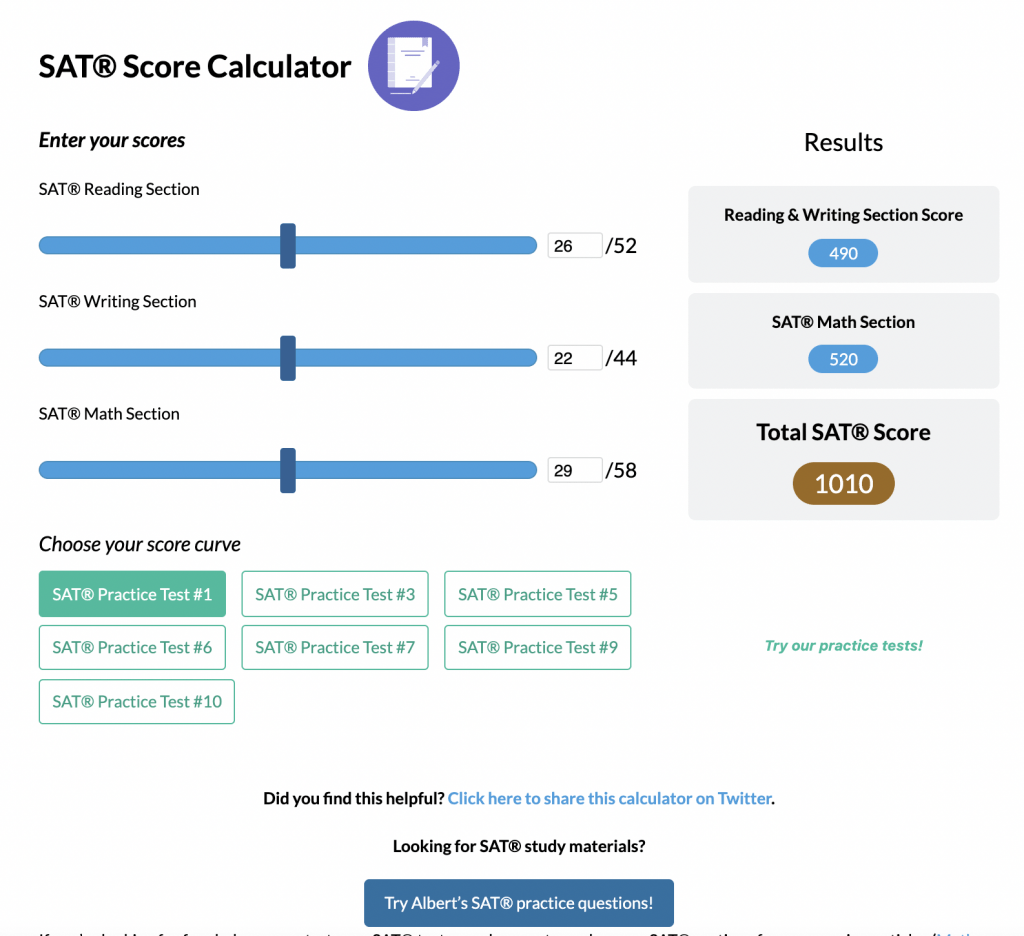
SAT® Score Calculator
See how scores on each section impacts your overall SAT® score

ACT® Score Calculator
See how scores on each section impacts your overall ACT® score
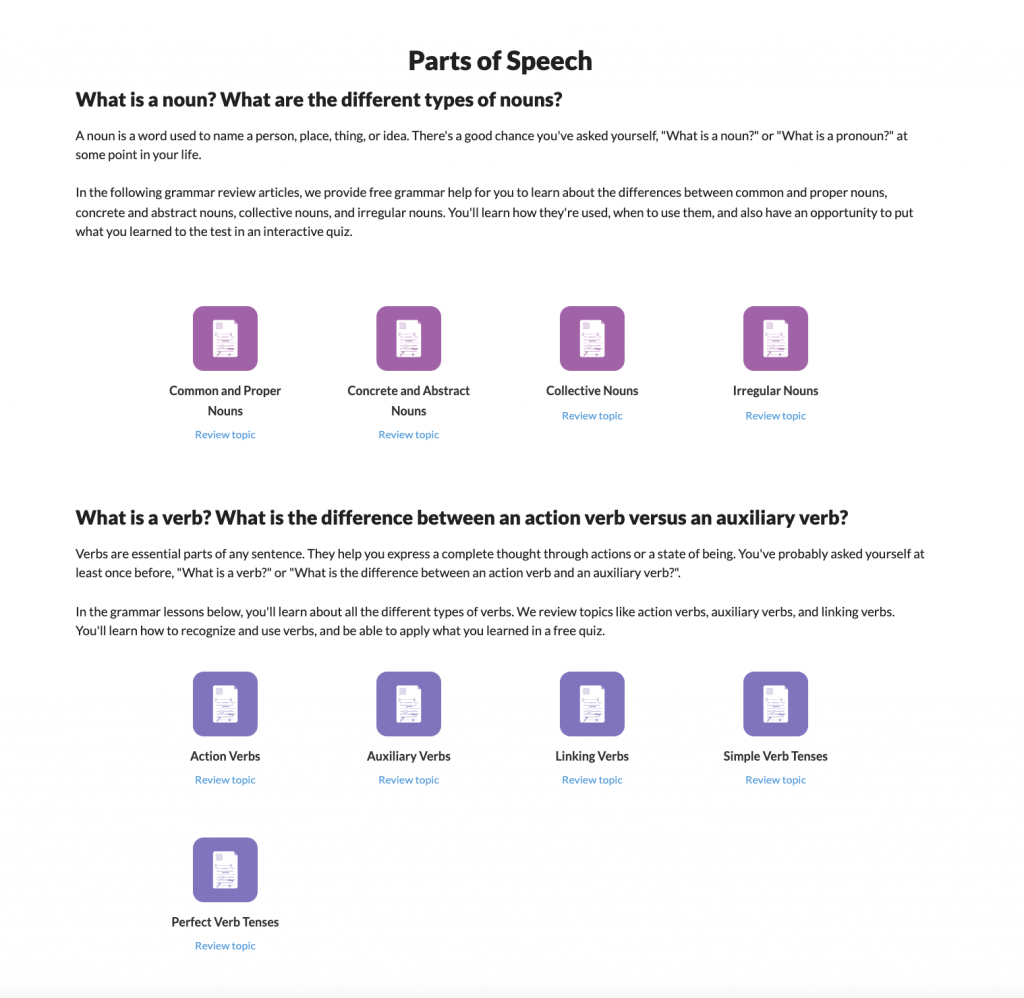
Grammar Review Hub
Comprehensive review of grammar skills

AP® Posters
Download updated posters summarizing the main topics and structure for each AP® exam.
Methods of data collection: experiments and focus groups
Cite this chapter.
- Sotirios Sarantakos 2
355 Accesses
The successful completion of a sampling procedure connects the research with the respondents and specifies the kind and number of respondents who will be involved. The investigator knows at this stage not only what be studied, but also who to approach to collect the required information. The information will be available, provided that the right ‘connection’ between the researcher and the respondents is made. This connection is made through the methods of data collection.
This is a preview of subscription content, log in via an institution to check access.
Access this chapter
Institutional subscriptions
Unable to display preview. Download preview PDF.
Author information
Authors and affiliations.
Charles Sturt University, Australia
Sotirios Sarantakos
You can also search for this author in PubMed Google Scholar
Copyright information
© 1998 Sotirios Sarantakos
About this chapter
Sarantakos, S. (1998). Methods of data collection: experiments and focus groups. In: Social Research. Palgrave, London. https://doi.org/10.1007/978-1-349-14884-4_7
Download citation
DOI : https://doi.org/10.1007/978-1-349-14884-4_7
Publisher Name : Palgrave, London
Print ISBN : 978-0-333-73868-9
Online ISBN : 978-1-349-14884-4
eBook Packages : Palgrave Social & Cultural Studies Collection Social Sciences (R0)
Share this chapter
Anyone you share the following link with will be able to read this content:
Sorry, a shareable link is not currently available for this article.
Provided by the Springer Nature SharedIt content-sharing initiative
- Publish with us
Policies and ethics
- Find a journal
- Track your research
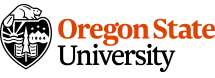
Chapter 10. Introduction to Data Collection Techniques
Introduction.
Now that we have discussed various aspects of qualitative research, we can begin to collect data. This chapter serves as a bridge between the first half and second half of this textbook (and perhaps your course) by introducing techniques of data collection. You’ve already been introduced to some of this because qualitative research is often characterized by the form of data collection; for example, an ethnographic study is one that employs primarily observational data collection for the purpose of documenting and presenting a particular culture or ethnos. Thus, some of this chapter will operate as a review of material already covered, but we will be approaching it from the data-collection side rather than the tradition-of-inquiry side we explored in chapters 2 and 4.
Revisiting Approaches
There are four primary techniques of data collection used in qualitative research: interviews, focus groups, observations, and document review. [1] There are other available techniques, such as visual analysis (e.g., photo elicitation) and biography (e.g., autoethnography) that are sometimes used independently or supplementarily to one of the main forms. Not to confuse you unduly, but these various data collection techniques are employed differently by different qualitative research traditions so that sometimes the technique and the tradition become inextricably entwined. This is largely the case with observations and ethnography. The ethnographic tradition is fundamentally based on observational techniques. At the same time, traditions other than ethnography also employ observational techniques, so it is worthwhile thinking of “tradition” and “technique” separately (see figure 10.1).
TYPE | As in... | Approaches where you commonly see this technique... | Guidelines |
---|---|---|---|
Interview-based studies | ; Ethnography (along with Observations); Mixed Methods; Grounded Theory; Narrative Inquiry; Feminist Approaches | Semi-structured or unstructured interviews with one to 100 participants, depending on tradition | |
Case Study; Feminist Approaches; Mixed Methods; often used as a supplementary technique | SIngle or comparative focused discussions with 5-12 persons | ||
Participant-observation studies; ethnographic studies | ; Grounded Theory; Symbolic Interactionism; Case Study | Multiple observations in "field," with written fieldnotes serving as the data | |
Historical or archival research or content analysis | ; Content Analysis; Narrative Inquiry; Mixed Methods | Systematic and rigorous analyses of documents employing coding techniques | |
Photo/drawing elicitations; photovoice | Phenomenology; Grounded Theory; Ethnography | Supplemental technique asking participants to draw/explain or view/explain visual material | |
Autoethnography; Oral Histories | Narrative Inquiry; Case Study; Oral History | Largely chronologically-structured collection of a person's life history; can be a single illustrative case |
Figure 10.1. Data Collection Techniques
Each of these data collection techniques will be the subject of its own chapter in the second half of this textbook. This chapter serves as an orienting overview and as the bridge between the conceptual/design portion of qualitative research and the actual practice of conducting qualitative research.
Overview of the Four Primary Approaches
Interviews are at the heart of qualitative research. Returning to epistemological foundations, it is during the interview that the researcher truly opens herself to hearing what others have to say, encouraging her interview subjects to reflect deeply on the meanings and values they hold. Interviews are used in almost every qualitative tradition but are particularly salient in phenomenological studies, studies seeking to understand the meaning of people’s lived experiences.
Focus groups can be seen as a type of interview, one in which a group of persons (ideally between five and twelve) is asked a series of questions focused on a particular topic or subject. They are sometimes used as the primary form of data collection, especially outside academic research. For example, businesses often employ focus groups to determine if a particular product is likely to sell. Among qualitative researchers, it is often used in conjunction with any other primary data collection technique as a form of “triangulation,” or a way of increasing the reliability of the study by getting at the object of study from multiple directions. [2] Some traditions, such as feminist approaches, also see the focus group as an important “consciousness-raising” tool.
If interviews are at the heart of qualitative research, observations are its lifeblood. Researchers who are more interested in the practices and behaviors of people than what they think or who are trying to understand the parameters of an organizational culture rely on observations as their primary form of data collection. The notes they make “in the field” (either during observations or afterward) form the “data” that will be analyzed. Ethnographers, those seeking to describe a particular ethnos, or culture, believe that observations are more reliable guides to that culture than what people have to say about it. Observations are thus the primary form of data collection for ethnographers, albeit often supplemented with in-depth interviews.
Some would say that these three—interviews, focus groups, and observations—are really the foundational techniques of data collection. They are far and away the three techniques most frequently used separately, in conjunction with one another, and even sometimes in mixed methods qualitative/quantitative studies. Document review, either as a form of content analysis or separately, however, is an important addition to the qualitative researcher’s toolkit and should not be overlooked (figure 10.1). Although it is rare for a qualitative researcher to make document review their primary or sole form of data collection, including documents in the research design can help expand the reach and the reliability of a study. Document review can take many forms, from historical and archival research, in which the researcher pieces together a narrative of the past by finding and analyzing a variety of “documents” and records (including photographs and physical artifacts), to analyses of contemporary media content, as in the case of compiling and coding blog posts or other online commentaries, and content analysis that identifies and describes communicative aspects of media or documents.
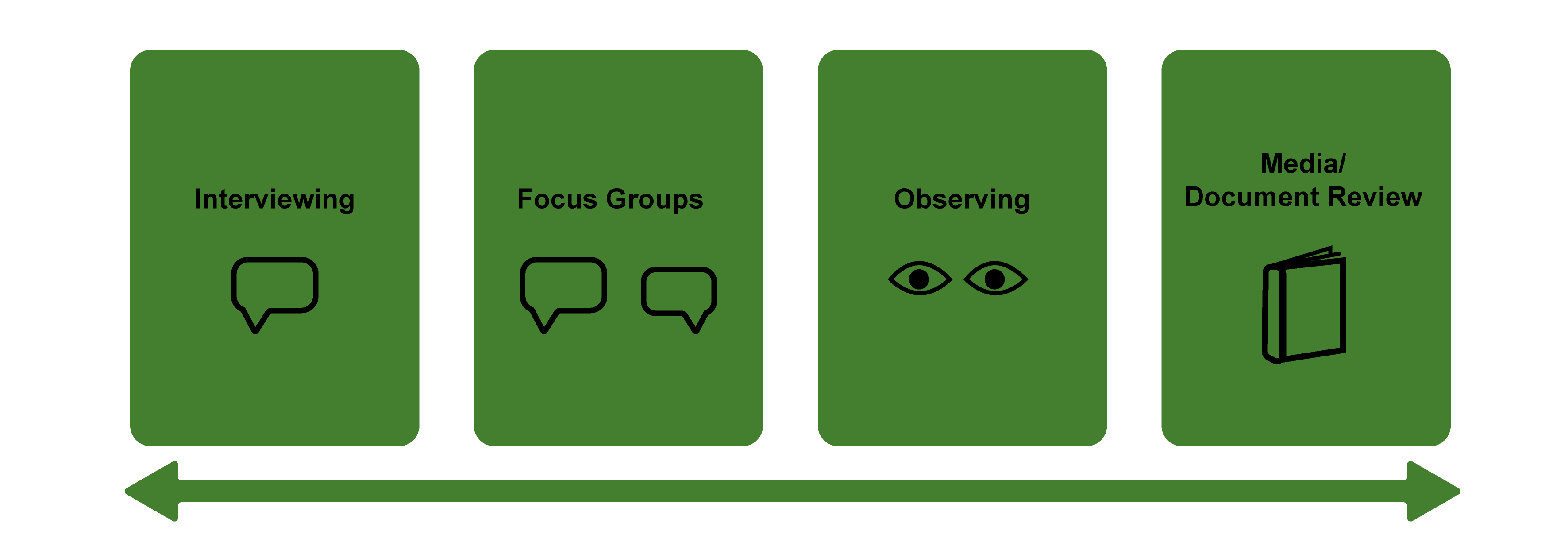
In addition to these four major techniques, there are a host of emerging and incidental data collection techniques, from photo elicitation or photo voice, in which respondents are asked to comment upon a photograph or image (particularly useful as a supplement to interviews when the respondents are hesitant or unable to answer direct questions), to autoethnographies, in which the researcher uses his own position and life to increase our understanding about a phenomenon and its historical and social context.
Taken together, these techniques provide a wide range of practices and tools with which to discover the world. They are particularly suited to addressing the questions that qualitative researchers ask—questions about how things happen and why people act the way they do, given particular social contexts and shared meanings about the world (chapter 4).
Triangulation and Mixed Methods
Because the researcher plays such a large and nonneutral role in qualitative research, one that requires constant reflectivity and awareness (chapter 6), there is a constant need to reassure her audience that the results she finds are reliable. Quantitative researchers can point to any number of measures of statistical significance to reassure their audiences, but qualitative researchers do not have math to hide behind. And she will also want to reassure herself that what she is hearing in her interviews or observing in the field is a true reflection of what is going on (or as “true” as possible, given the problem that the world is as large and varied as the elephant; see chapter 3). For those reasons, it is common for researchers to employ more than one data collection technique or to include multiple and comparative populations, settings, and samples in the research design (chapter 2). A single set of interviews or initial comparison of focus groups might be conceived as a “pilot study” from which to launch the actual study. Undergraduate students working on a research project might be advised to think about their projects in this way as well. You are simply not going to have enough time or resources as an undergraduate to construct and complete a successful qualitative research project, but you may be able to tackle a pilot study. Graduate students also need to think about the amount of time and resources they have for completing a full study. Masters-level students, or students who have one year or less in which to complete a program, should probably consider their study as an initial exploratory pilot. PhD candidates might have the time and resources to devote to the type of triangulated, multifaceted research design called for by the research question.
We call the use of multiple qualitative methods of data collection and the inclusion of multiple and comparative populations and settings “triangulation.” Using different data collection methods allows us to check the consistency of our findings. For example, a study of the vaccine hesitant might include a set of interviews with vaccine-hesitant people and a focus group of the same and a content analysis of online comments about a vaccine mandate. By employing all three methods, we can be more confident of our interpretations from the interviews alone (especially if we are hearing the same thing throughout; if we are not, then this is a good sign that we need to push a little further to find out what is really going on). [3] Methodological triangulation is an important tool for increasing the reliability of our findings and the overall success of our research.
Methodological triangulation should not be confused with mixed methods techniques, which refer instead to the combining of qualitative and quantitative research methods. Mixed methods studies can increase reliability, but that is not their primary purpose. Mixed methods address multiple research questions, both the “how many” and “why” kind, or the causal and explanatory kind. Mixed methods will be discussed in more detail in chapter 15.
Let us return to the three examples of qualitative research described in chapter 1: Cory Abramson’s study of aging ( The End Game) , Jennifer Pierce’s study of lawyers and discrimination ( Racing for Innocence ), and my own study of liberal arts college students ( Amplified Advantage ). Each of these studies uses triangulation.
Abramson’s book is primarily based on three years of observations in four distinct neighborhoods. He chose the neighborhoods in such a way to maximize his ability to make comparisons: two were primarily middle class and two were primarily poor; further, within each set, one was predominantly White, while the other was either racially diverse or primarily African American. In each neighborhood, he was present in senior centers, doctors’ offices, public transportation, and other public spots where the elderly congregated. [4] The observations are the core of the book, and they are richly written and described in very moving passages. But it wasn’t enough for him to watch the seniors. He also engaged with them in casual conversation. That, too, is part of fieldwork. He sometimes even helped them make it to the doctor’s office or get around town. Going beyond these interactions, he also interviewed sixty seniors, an equal amount from each of the four neighborhoods. It was in the interviews that he could ask more detailed questions about their lives, what they thought about aging, what it meant to them to be considered old, and what their hopes and frustrations were. He could see that those living in the poor neighborhoods had a more difficult time accessing care and resources than those living in the more affluent neighborhoods, but he couldn’t know how the seniors understood these difficulties without interviewing them. Both forms of data collection supported each other and helped make the study richer and more insightful. Interviews alone would have failed to demonstrate the very real differences he observed (and that some seniors would not even have known about). This is the value of methodological triangulation.
Pierce’s book relies on two separate forms of data collection—interviews with lawyers at a firm that has experienced a history of racial discrimination and content analyses of news stories and popular films that screened during the same years of the alleged racial discrimination. I’ve used this book when teaching methods and have often found students struggle with understanding why these two forms of data collection were used. I think this is because we don’t teach students to appreciate or recognize “popular films” as a legitimate form of data. But what Pierce does is interesting and insightful in the best tradition of qualitative research. Here is a description of the content analyses from a review of her book:
In the chapter on the news media, Professor Pierce uses content analysis to argue that the media not only helped shape the meaning of affirmative action, but also helped create white males as a class of victims. The overall narrative that emerged from these media accounts was one of white male innocence and victimization. She also maintains that this narrative was used to support “neoconservative and neoliberal political agendas” (p. 21). The focus of these articles tended to be that affirmative action hurt white working-class and middle-class men particularly during the recession in the 1980s (despite statistical evidence that people of color were hurt far more than white males by the recession). In these stories fairness and innocence were seen in purely individual terms. Although there were stories that supported affirmative action and developed a broader understanding of fairness, the total number of stories slanted against affirmative action from 1990 to 1999. During that time period negative stories always outnumbered those supporting the policy, usually by a ratio of 3:1 or 3:2. Headlines, the presentation of polling data, and an emphasis in stories on racial division, Pierce argues, reinforced the story of white male victimization. Interestingly, the news media did very few stories on gender and affirmative action. The chapter on the film industry from 1989 to 1999 reinforces Pierce’s argument and adds another layer to her interpretation of affirmative action during this time period. She sampled almost 60 Hollywood films with receipts ranging from four million to 184 million dollars. In this chapter she argues that the dominant theme of these films was racial progress and the redemption of white Americans from past racism. These movies usually portrayed white, elite, and male experiences. People of color were background figures who supported the protagonist and “anointed” him as a savior (p. 45). Over the course of the film the protagonists move from “innocence to consciousness” concerning racism. The antagonists in these films most often were racist working-class white men. A Time to Kill , Mississippi Burning , Amistad , Ghosts of Mississippi , The Long Walk Home , To Kill a Mockingbird , and Dances with Wolves receive particular analysis in this chapter, and her examination of them leads Pierce to conclude that they infused a myth of racial progress into America’s cultural memory. White experiences of race are the focus and contemporary forms of racism are underplayed or omitted. Further, these films stereotype both working-class and elite white males, and underscore the neoliberal emphasis on individualism. ( Hrezo 2012 )
With that context in place, Pierce then turned to interviews with attorneys. She finds that White male attorneys often misremembered facts about the period in which the law firm was accused of racial discrimination and that they often portrayed their firms as having made substantial racial progress. This was in contrast to many of the lawyers of color and female lawyers who remembered the history differently and who saw continuing examples of racial (and gender) discrimination at the law firm. In most of the interviews, people talked about individuals, not structure (and these are attorneys, who really should know better!). By including both content analyses and interviews in her study, Pierce is better able to situate the attorney narratives and explain the larger context for the shared meanings of individual innocence and racial progress. Had this been a study only of films during this period, we would not know how actual people who lived during this period understood the decisions they made; had we had only the interviews, we would have missed the historical context and seen a lot of these interviewees as, well, not very nice people at all. Together, we have a study that is original, inventive, and insightful.
My own study of how class background affects the experiences and outcomes of students at small liberal arts colleges relies on mixed methods and triangulation. At the core of the book is an original survey of college students across the US. From analyses of this survey, I can present findings on “how many” questions and descriptive statistics comparing students of different social class backgrounds. For example, I know and can demonstrate that working-class college students are less likely to go to graduate school after college than upper-class college students are. I can even give you some estimates of the class gap. But what I can’t tell you from the survey is exactly why this is so or how it came to be so . For that, I employ interviews, focus groups, document reviews, and observations. Basically, I threw the kitchen sink at the “problem” of class reproduction and higher education (i.e., Does college reduce class inequalities or make them worse?). A review of historical documents provides a picture of the place of the small liberal arts college in the broader social and historical context. Who had access to these colleges and for what purpose have always been in contest, with some groups attempting to exclude others from opportunities for advancement. What it means to choose a small liberal arts college in the early twenty-first century is thus different for those whose parents are college professors, for those whose parents have a great deal of money, and for those who are the first in their family to attend college. I was able to get at these different understandings through interviews and focus groups and to further delineate the culture of these colleges by careful observation (and my own participation in them, as both former student and current professor). Putting together individual meanings, student dispositions, organizational culture, and historical context allowed me to present a story of how exactly colleges can both help advance first-generation, low-income, working-class college students and simultaneously amplify the preexisting advantages of their peers. Mixed methods addressed multiple research questions, while triangulation allowed for this deeper, more complex story to emerge.
In the next few chapters, we will explore each of the primary data collection techniques in much more detail. As we do so, think about how these techniques may be productively joined for more reliable and deeper studies of the social world.
Advanced Reading: Triangulation
Denzin ( 1978 ) identified four basic types of triangulation: data, investigator, theory, and methodological. Properly speaking, if we use the Denzin typology, the use of multiple methods of data collection and analysis to strengthen one’s study is really a form of methodological triangulation. It may be helpful to understand how this differs from the other types.
Data triangulation occurs when the researcher uses a variety of sources in a single study. Perhaps they are interviewing multiple samples of college students. Obviously, this overlaps with sample selection (see chapter 5). It is helpful for the researcher to understand that these multiple data sources add strength and reliability to the study. After all, it is not just “these students here” but also “those students over there” that are experiencing this phenomenon in a particular way.
Investigator triangulation occurs when different researchers or evaluators are part of the research team. Intercoding reliability is a form of investigator triangulation (or at least a way of leveraging the power of multiple researchers to raise the reliability of the study).
Theory triangulation is the use of multiple perspectives to interpret a single set of data, as in the case of competing theoretical paradigms (e.g., a human capital approach vs. a Bourdieusian multiple capital approach).
Methodological triangulation , as explained in this chapter, is the use of multiple methods to study a single phenomenon, issue, or problem.
Further Readings
Carter, Nancy, Denise Bryant-Lukosius, Alba DiCenso, Jennifer Blythe, Alan J. Neville. 2014. “The Use of Triangulation in Qualitative Research.” Oncology Nursing Forum 41(5):545–547. Discusses the four types of triangulation identified by Denzin with an example of the use of focus groups and in-depth individuals.
Mathison, Sandra. 1988. “Why Triangulate?” Educational Researcher 17(2):13–17. Presents three particular ways of assessing validity through the use of triangulated data collection: convergence, inconsistency, and contradiction.
Tracy, Sarah J. 2010. “Qualitative Quality: Eight ‘Big-Tent’ Criteria for Excellent Qualitative Research.” Qualitative Inquiry 16(10):837–851. Focuses on triangulation as a criterion for conducting valid qualitative research.
- Marshall and Rossman ( 2016 ) state this slightly differently. They list four primary methods for gathering information: (1) participating in the setting, (2) observing directly, (3) interviewing in depth, and (4) analyzing documents and material culture (141). An astute reader will note that I have collapsed participation into observation and that I have distinguished focus groups from interviews. I suspect that this distinction marks me as more of an interview-based researcher, while Marshall and Rossman prioritize ethnographic approaches. The main point of this footnote is to show you, the reader, that there is no single agreed-upon number of approaches to collecting qualitative data. ↵
- See “ Advanced Reading: Triangulation ” at end of this chapter. ↵
- We can also think about triangulating the sources, as when we include comparison groups in our sample (e.g., if we include those receiving vaccines, we might find out a bit more about where the real differences lie between them and the vaccine hesitant); triangulating the analysts (building a research team so that your interpretations can be checked against those of others on the team); and even triangulating the theoretical perspective (as when we “try on,” say, different conceptualizations of social capital in our analyses). ↵
Introduction to Qualitative Research Methods Copyright © 2023 by Allison Hurst is licensed under a Creative Commons Attribution-ShareAlike 4.0 International License , except where otherwise noted.
Have a language expert improve your writing
Run a free plagiarism check in 10 minutes, generate accurate citations for free.
- Knowledge Base
Methodology
- Data Collection | Definition, Methods & Examples
Data Collection | Definition, Methods & Examples
Published on June 5, 2020 by Pritha Bhandari . Revised on June 21, 2023.
Data collection is a systematic process of gathering observations or measurements. Whether you are performing research for business, governmental or academic purposes, data collection allows you to gain first-hand knowledge and original insights into your research problem .
While methods and aims may differ between fields, the overall process of data collection remains largely the same. Before you begin collecting data, you need to consider:
- The aim of the research
- The type of data that you will collect
- The methods and procedures you will use to collect, store, and process the data
To collect high-quality data that is relevant to your purposes, follow these four steps.
Table of contents
Step 1: define the aim of your research, step 2: choose your data collection method, step 3: plan your data collection procedures, step 4: collect the data, other interesting articles, frequently asked questions about data collection.
Before you start the process of data collection, you need to identify exactly what you want to achieve. You can start by writing a problem statement : what is the practical or scientific issue that you want to address and why does it matter?
Next, formulate one or more research questions that precisely define what you want to find out. Depending on your research questions, you might need to collect quantitative or qualitative data :
- Quantitative data is expressed in numbers and graphs and is analyzed through statistical methods .
- Qualitative data is expressed in words and analyzed through interpretations and categorizations.
If your aim is to test a hypothesis , measure something precisely, or gain large-scale statistical insights, collect quantitative data. If your aim is to explore ideas, understand experiences, or gain detailed insights into a specific context, collect qualitative data. If you have several aims, you can use a mixed methods approach that collects both types of data.
- Your first aim is to assess whether there are significant differences in perceptions of managers across different departments and office locations.
- Your second aim is to gather meaningful feedback from employees to explore new ideas for how managers can improve.
Here's why students love Scribbr's proofreading services
Discover proofreading & editing
Based on the data you want to collect, decide which method is best suited for your research.
- Experimental research is primarily a quantitative method.
- Interviews , focus groups , and ethnographies are qualitative methods.
- Surveys , observations, archival research and secondary data collection can be quantitative or qualitative methods.
Carefully consider what method you will use to gather data that helps you directly answer your research questions.
Method | When to use | How to collect data |
---|---|---|
Experiment | To test a causal relationship. | Manipulate variables and measure their effects on others. |
Survey | To understand the general characteristics or opinions of a group of people. | Distribute a list of questions to a sample online, in person or over-the-phone. |
Interview/focus group | To gain an in-depth understanding of perceptions or opinions on a topic. | Verbally ask participants open-ended questions in individual interviews or focus group discussions. |
Observation | To understand something in its natural setting. | Measure or survey a sample without trying to affect them. |
Ethnography | To study the culture of a community or organization first-hand. | Join and participate in a community and record your observations and reflections. |
Archival research | To understand current or historical events, conditions or practices. | Access manuscripts, documents or records from libraries, depositories or the internet. |
Secondary data collection | To analyze data from populations that you can’t access first-hand. | Find existing datasets that have already been collected, from sources such as government agencies or research organizations. |
When you know which method(s) you are using, you need to plan exactly how you will implement them. What procedures will you follow to make accurate observations or measurements of the variables you are interested in?
For instance, if you’re conducting surveys or interviews, decide what form the questions will take; if you’re conducting an experiment, make decisions about your experimental design (e.g., determine inclusion and exclusion criteria ).
Operationalization
Sometimes your variables can be measured directly: for example, you can collect data on the average age of employees simply by asking for dates of birth. However, often you’ll be interested in collecting data on more abstract concepts or variables that can’t be directly observed.
Operationalization means turning abstract conceptual ideas into measurable observations. When planning how you will collect data, you need to translate the conceptual definition of what you want to study into the operational definition of what you will actually measure.
- You ask managers to rate their own leadership skills on 5-point scales assessing the ability to delegate, decisiveness and dependability.
- You ask their direct employees to provide anonymous feedback on the managers regarding the same topics.
You may need to develop a sampling plan to obtain data systematically. This involves defining a population , the group you want to draw conclusions about, and a sample, the group you will actually collect data from.
Your sampling method will determine how you recruit participants or obtain measurements for your study. To decide on a sampling method you will need to consider factors like the required sample size, accessibility of the sample, and timeframe of the data collection.
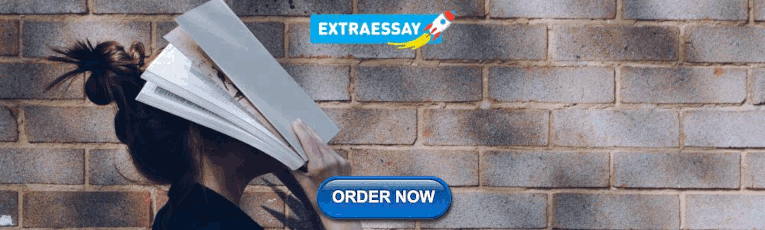
Standardizing procedures
If multiple researchers are involved, write a detailed manual to standardize data collection procedures in your study.
This means laying out specific step-by-step instructions so that everyone in your research team collects data in a consistent way – for example, by conducting experiments under the same conditions and using objective criteria to record and categorize observations. This helps you avoid common research biases like omitted variable bias or information bias .
This helps ensure the reliability of your data, and you can also use it to replicate the study in the future.
Creating a data management plan
Before beginning data collection, you should also decide how you will organize and store your data.
- If you are collecting data from people, you will likely need to anonymize and safeguard the data to prevent leaks of sensitive information (e.g. names or identity numbers).
- If you are collecting data via interviews or pencil-and-paper formats, you will need to perform transcriptions or data entry in systematic ways to minimize distortion.
- You can prevent loss of data by having an organization system that is routinely backed up.
Finally, you can implement your chosen methods to measure or observe the variables you are interested in.
The closed-ended questions ask participants to rate their manager’s leadership skills on scales from 1–5. The data produced is numerical and can be statistically analyzed for averages and patterns.
To ensure that high quality data is recorded in a systematic way, here are some best practices:
- Record all relevant information as and when you obtain data. For example, note down whether or how lab equipment is recalibrated during an experimental study.
- Double-check manual data entry for errors.
- If you collect quantitative data, you can assess the reliability and validity to get an indication of your data quality.
If you want to know more about statistics , methodology , or research bias , make sure to check out some of our other articles with explanations and examples.
- Student’s t -distribution
- Normal distribution
- Null and Alternative Hypotheses
- Chi square tests
- Confidence interval
- Cluster sampling
- Stratified sampling
- Data cleansing
- Reproducibility vs Replicability
- Peer review
- Likert scale
Research bias
- Implicit bias
- Framing effect
- Cognitive bias
- Placebo effect
- Hawthorne effect
- Hindsight bias
- Affect heuristic
Data collection is the systematic process by which observations or measurements are gathered in research. It is used in many different contexts by academics, governments, businesses, and other organizations.
When conducting research, collecting original data has significant advantages:
- You can tailor data collection to your specific research aims (e.g. understanding the needs of your consumers or user testing your website)
- You can control and standardize the process for high reliability and validity (e.g. choosing appropriate measurements and sampling methods )
However, there are also some drawbacks: data collection can be time-consuming, labor-intensive and expensive. In some cases, it’s more efficient to use secondary data that has already been collected by someone else, but the data might be less reliable.
Quantitative research deals with numbers and statistics, while qualitative research deals with words and meanings.
Quantitative methods allow you to systematically measure variables and test hypotheses . Qualitative methods allow you to explore concepts and experiences in more detail.
Reliability and validity are both about how well a method measures something:
- Reliability refers to the consistency of a measure (whether the results can be reproduced under the same conditions).
- Validity refers to the accuracy of a measure (whether the results really do represent what they are supposed to measure).
If you are doing experimental research, you also have to consider the internal and external validity of your experiment.
Operationalization means turning abstract conceptual ideas into measurable observations.
For example, the concept of social anxiety isn’t directly observable, but it can be operationally defined in terms of self-rating scores, behavioral avoidance of crowded places, or physical anxiety symptoms in social situations.
Before collecting data , it’s important to consider how you will operationalize the variables that you want to measure.
In mixed methods research , you use both qualitative and quantitative data collection and analysis methods to answer your research question .
Cite this Scribbr article
If you want to cite this source, you can copy and paste the citation or click the “Cite this Scribbr article” button to automatically add the citation to our free Citation Generator.
Bhandari, P. (2023, June 21). Data Collection | Definition, Methods & Examples. Scribbr. Retrieved July 1, 2024, from https://www.scribbr.com/methodology/data-collection/
Is this article helpful?
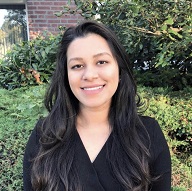
Pritha Bhandari
Other students also liked, qualitative vs. quantitative research | differences, examples & methods, sampling methods | types, techniques & examples, what is your plagiarism score.
Teach yourself statistics
Data Collection Methods
Before we can derive conclusions from data, we need to know how the data were collected; that is, we need to know the method(s) of data collection.
Note: Your browser does not support HTML5 video. If you view this web page on a different browser (e.g., a recent version of Edge, Chrome, Firefox, or Opera), you can watch a video treatment of this lesson.
Methods of Data Collection
In this lesson, we will cover four methods of data collection.
- Census . A census is a study that obtains data from every member of a population . In most studies, a census is not practical, because of the cost and/or time required.
- Sample survey . A sample survey is a study that obtains data from a subset of a population, in order to estimate population attributes.
In the analysis phase, the researcher compares group scores on some dependent variable . Based on the analysis, the researcher draws a conclusion about whether a treatment ( independent variable ) had a causal effect on the dependent variable.
- Observational study . Like experiments, observational studies attempt to understand cause-and-effect relationships. However, unlike experiments, the researcher is not able to control (1) how subjects are assigned to groups and/or (2) which treatments each group receives.
Data Collection Methods: Pros and Cons
Each method of data collection has advantages and disadvantages.
- Resources . When the population is large, a sample survey has a big resource advantage over a census. A well-designed sample survey can provide very precise estimates of population parameters - quicker, cheaper, and with less manpower than a census.
Observational studies do not feature random selection; so generalizing from the results of an observational study to a larger population can be a problem.
- Causal inference . Cause-and-effect relationships can be teased out when subjects are randomly assigned to groups. Therefore, experiments, which allow the researcher to control assignment of subjects to treatment groups, are the best method for investigating causal relationships.
Test Your Understanding
Which of the following statements are true?
I. A sample survey is a type of experiment. II. An observational study requires fewer resources than an experiment. III. The best method for investigating causal relationships is an observational study.
(A) I only (B) II only (C) III only (D) All of the above. (E) None of the above.
The correct answer is (E). Unlike an experiment, a sample survey does not require the researcher to assign treatments to survey respondents. Therefore, a sample survey is not necessarily an experiment. A sample survey could be an observational study, rather than an experiment. An observational study may or may not require fewer resources (time, money, manpower) than an experiment. The best method for investigating causal relationships is an experiment - not an observational study - because an experiment features randomized assignment of subjects to treatment groups.
- Skip to main content
- Skip to primary sidebar
- Skip to footer
- QuestionPro

- Solutions Industries Gaming Automotive Sports and events Education Government Travel & Hospitality Financial Services Healthcare Cannabis Technology Use Case NPS+ Communities Audience Contactless surveys Mobile LivePolls Member Experience GDPR Positive People Science 360 Feedback Surveys
- Resources Blog eBooks Survey Templates Case Studies Training Help center

Home QuestionPro QuestionPro Products
Data Collection Methods: Types & Examples
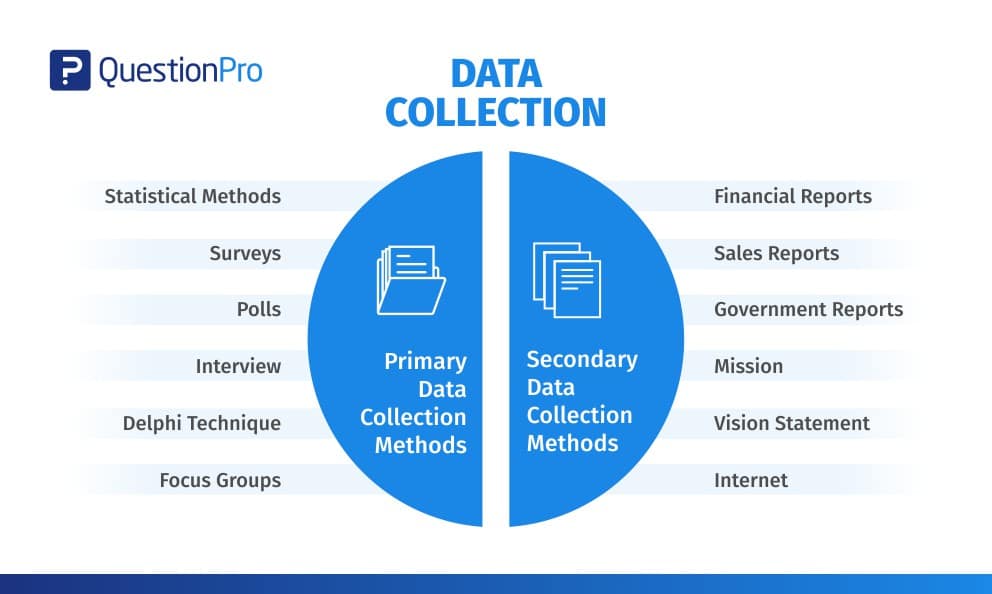
Data is a collection of facts, figures, objects, symbols, and events gathered from different sources. Organizations collect data using various methods to make better decisions. Without data, it would be difficult for organizations to make appropriate decisions, so data is collected from different audiences at various times.
For example, an organization must collect data on product demand, customer preferences, and competitors before launching a new product. If data is not collected beforehand, the organization’s newly launched product may fail for many reasons, such as less demand and inability to meet customer needs.
Although data is a valuable asset for every organization, it does not serve any purpose until it is analyzed or processed to achieve the desired results.
What are Data Collection Methods?
Data collection methods are techniques and procedures for gathering information for research purposes. They can range from simple self-reported surveys to more complex quantitative or qualitative experiments.
Some common data collection methods include surveys , interviews, observations, focus groups, experiments, and secondary data analysis . The data collected through these methods can then be analyzed and used to support or refute research hypotheses and draw conclusions about the study’s subject matter.
Understanding Data Collection Methods
Data collection methods encompass a variety of techniques and tools for gathering quantitative and qualitative data. These methods are integral to the data collection process and ensure accurate and comprehensive data acquisition.
Quantitative data collection methods involve systematic approaches to collecting data, like numerical data, such as surveys, polls, and statistical analysis, aimed at quantifying phenomena and trends.
Conversely, qualitative data collection methods focus on capturing non-numerical information, such as interviews, focus groups, and observations, to delve deeper into understanding attitudes, behaviors, and motivations.
Combining quantitative and qualitative data collection techniques can enrich organizations’ datasets and gain comprehensive insights into complex phenomena.
Effective utilization of accurate data collection tools and techniques enhances the accuracy and reliability of collected data, facilitating informed decision-making and strategic planning.
LEARN ABOUT: Self-Selection Bias
Importance of Data Collection Methods
Data collection methods play a crucial role in the research process as they determine the quality and accuracy of the data collected. Here are some major importance of data collection methods.
- Quality and Accuracy: The choice of data collection technique directly impacts the quality and accuracy of the data obtained. Properly designed methods help ensure that the data collected is error-free and relevant to the research questions.
- Relevance, Validity, and Reliability: Effective data collection methods help ensure that the data collected is relevant to the research objectives, valid (measuring what it intends to measure), and reliable (consistent and reproducible).
- Bias Reduction and Representativeness: Carefully chosen data collection methods can help minimize biases inherent in the research process, such as sampling bias or response bias. They also aid in achieving a representative sample, enhancing the findings’ generalizability.
- Informed Decision Making: Accurate and reliable data collected through appropriate methods provide a solid foundation for making informed decisions based on research findings. This is crucial for both academic research and practical applications in various fields.
- Achievement of Research Objectives: Data collection methods should align with the research objectives to ensure that the collected data effectively addresses the research questions or hypotheses. Properly collected data facilitates the attainment of these objectives.
- Support for Validity and Reliability: Validity and reliability are essential to research validity. The choice of data collection methods can either enhance or detract from the validity and reliability of research findings. Therefore, selecting appropriate methods is critical for ensuring the credibility of the research.
The importance of data collection methods cannot be overstated, as they play a key role in the research study’s overall success and internal validity .
Types of Data Collection Methods
The choice of data collection method depends on the research question being addressed, the type of data needed, and the resources and time available. Data collection methods can be categorized into primary and secondary methods.
1. Primary Data Collection Methods
Primary data is collected from first-hand experience and is not used in the past. The data gathered by primary data collection methods are highly accurate and specific to the research’s motive.
Primary data collection methods can be divided into two categories: quantitative methods and qualitative methods .
Quantitative Methods:
Quantitative techniques for market research and demand forecasting usually use statistical tools. In these techniques, demand is forecasted based on historical data. These methods of primary data collection are generally used to make long-term forecasts. Statistical analysis methods are highly reliable as subjectivity is minimal.
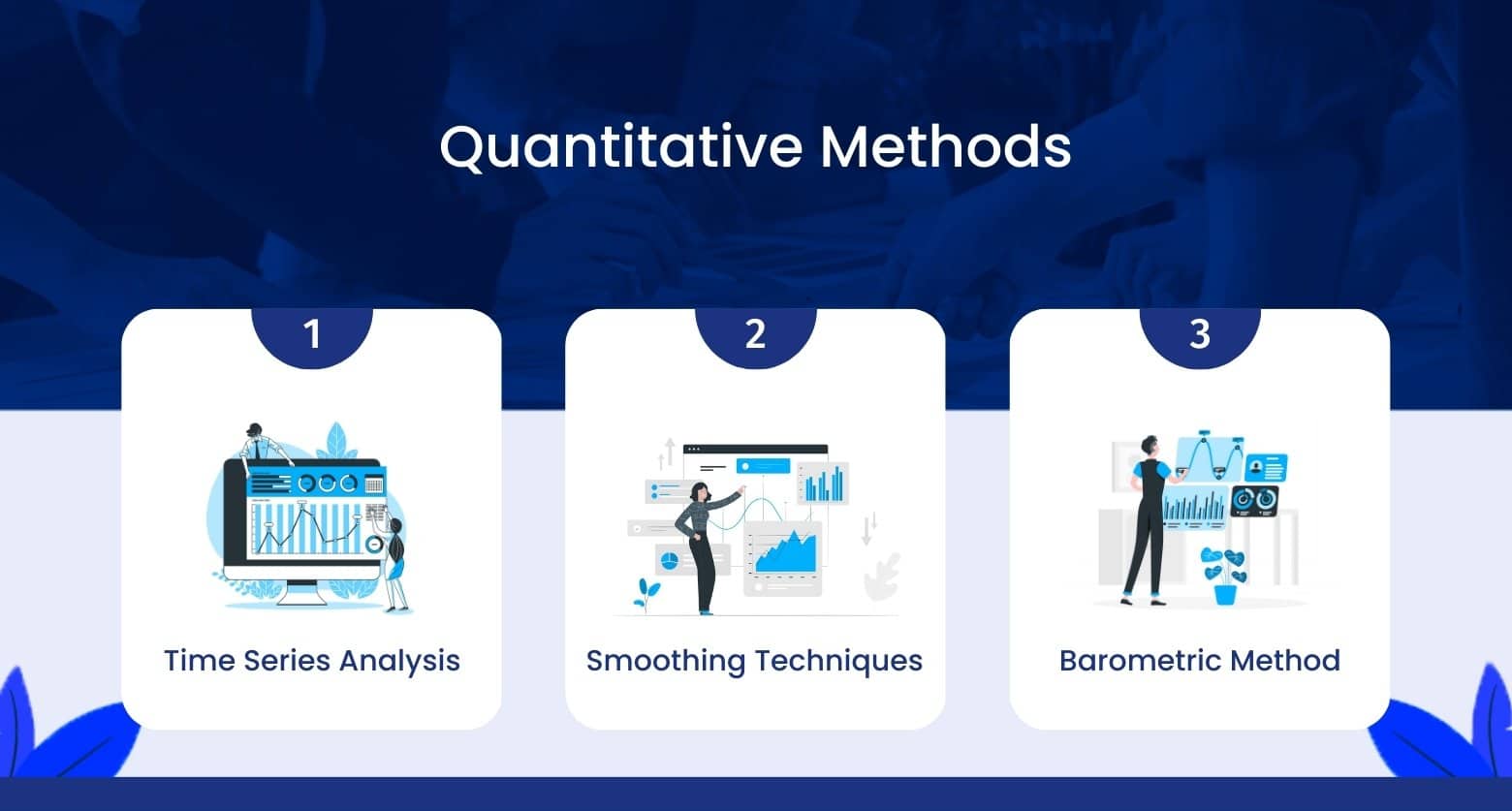
- Time Series Analysis: A time series refers to a sequential order of values of a variable, known as a trend, at equal time intervals. Using patterns, an organization can predict the demand for its products and services over a projected time period.
- Smoothing Techniques: Smoothing techniques can be used in cases where the time series lacks significant trends. They eliminate random variation from the historical demand, helping identify patterns and demand levels to estimate future demand. The most common methods used in smoothing demand forecasting are the simple moving average and weighted moving average methods.
- Barometric Method: Also known as the leading indicators approach, researchers use this method to speculate future trends based on current developments. When past events are considered to predict future events, they act as leading indicators.
Qualitative Methods:
Qualitative data collection methods are especially useful when historical data is unavailable or when numbers or mathematical calculations are unnecessary.
Qualitative research is closely associated with words, sounds, feelings, emotions, colors, and non-quantifiable elements. These techniques are based on experience, judgment, intuition, conjecture, emotion, etc.
Quantitative methods do not provide the motive behind participants’ responses, often don’t reach underrepresented populations, and require long periods of time to collect the data. Hence, it is best to combine quantitative methods with qualitative methods.
1. Surveys: Surveys collect data from the target audience and gather insights into their preferences, opinions, choices, and feedback related to their products and services. Most survey software offers a wide range of question types.
You can also use a ready-made survey template to save time and effort. Online surveys can be customized to match the business’s brand by changing the theme, logo, etc. They can be distributed through several channels, such as email, website, offline app, QR code, social media, etc.
You can select the channel based on your audience’s type and source. Once the data is collected, survey software can generate various reports and run analytics algorithms to discover hidden insights.
A survey dashboard can give you statistics related to response rate, completion rate, demographics-based filters, export and sharing options, etc. Integrating survey builders with third-party apps can maximize the effort spent on online real-time data collection .
Practical business intelligence relies on the synergy between analytics and reporting , where analytics uncovers valuable insights, and reporting communicates these findings to stakeholders.
2. Polls: Polls comprise one single or multiple-choice question . They are useful when you need to get a quick pulse of the audience’s sentiments. Because they are short, it is easier to get responses from people.
Like surveys, online polls can be embedded into various platforms. Once the respondents answer the question, they can also be shown how they compare to others’ responses.
Interviews: In this method, the interviewer asks the respondents face-to-face or by telephone.
3. Interviews: In face-to-face interviews, the interviewer asks a series of questions to the interviewee in person and notes down responses. If it is not feasible to meet the person, the interviewer can go for a telephone interview.
This form of data collection is suitable for only a few respondents. It is too time-consuming and tedious to repeat the same process if there are many participants.
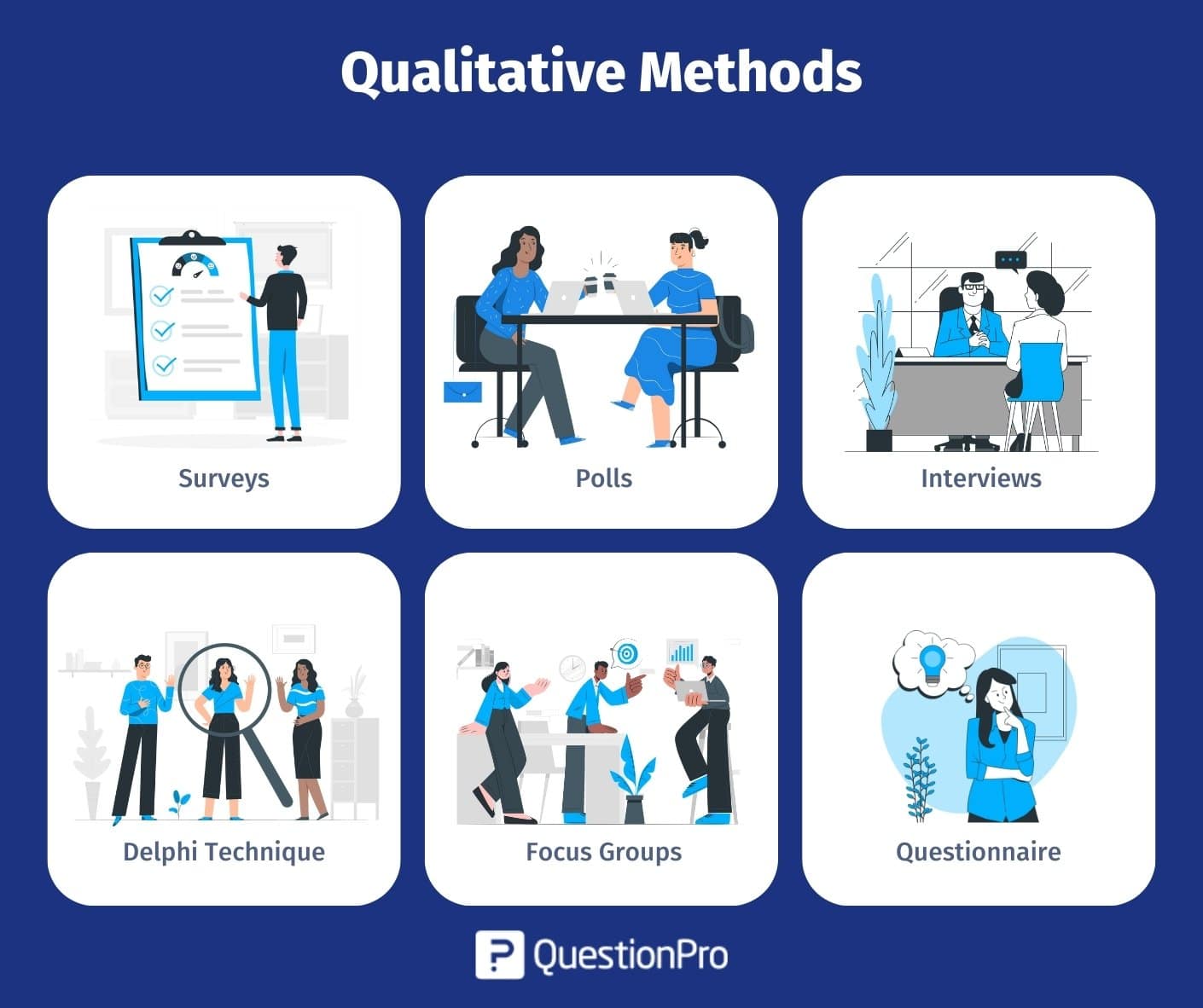
4. Delphi Technique: In the Delphi method, market experts are provided with the estimates and assumptions of other industry experts’ forecasts. Experts may reconsider and revise their estimates and assumptions based on this information. The consensus of all experts on demand forecasts constitutes the final demand forecast.
5. Focus Groups: Focus groups are one example of qualitative data in education . In a focus group, a small group of people, around 8-10 members, discuss the common areas of the research problem. Each individual provides his or her insights on the issue concerned.
A moderator regulates the discussion among the group members. At the end of the discussion, the group reaches a consensus.
6. Questionnaire: A questionnaire is a printed set of open-ended or closed-ended questions that respondents must answer based on their knowledge and experience with the issue. The questionnaire is part of the survey, whereas the questionnaire’s end goal may or may not be a survey.
2. Secondary Data Collection Methods
Secondary data is data that has been used in the past. The researcher can obtain data from the data sources , both internal and external, to the organizational data .
Internal sources of secondary data:
- Organization’s health and safety records
- Mission and vision statements
- Financial Statements
- Sales Report
- CRM Software
- Executive summaries
External sources of secondary data:
- Government reports
- Press releases
- Business journals
Secondary data collection methods can also involve quantitative and qualitative techniques. Secondary data is easily available, less time-consuming, and expensive than primary data. However, the authenticity of the data gathered cannot be verified using these methods.
Secondary data collection methods can also involve quantitative and qualitative observation techniques. Secondary data is easily available, less time-consuming, and more expensive than primary data.
However, the authenticity of the data gathered cannot be verified using these methods.
Regardless of the data collection method of your choice, there must be direct communication with decision-makers so that they understand and commit to acting according to the results.
For this reason, we must pay special attention to the analysis and presentation of the information obtained. Remember that these data must be useful and functional to us, so the data collection method has much to do with it.
LEARN ABOUT: Data Asset Management
How Can QuestionPro Help to Create Effective Data Collection?
QuestionPro is a comprehensive online survey software platform that can greatly assist in various data collection methods. Here’s how it can help:
- Survey Creation: QuestionPro offers a user-friendly interface for creating surveys with various question types, including multiple-choice, open-ended, Likert scale, and more. Researchers can customize surveys to fit their specific research needs and objectives.
- Diverse Distribution Channels: The platform provides multiple channels for distributing surveys, including email, web links, social media, and website embedding surveys. This enables researchers to reach a wide audience and collect data efficiently.
- Panel Management: QuestionPro offers panel management features, allowing researchers to create and manage panels of respondents for targeted data collection. This is particularly useful for longitudinal studies or when targeting specific demographics.
- Data Analysis Tools: The platform includes robust data analysis tools that enable researchers to analyze survey responses in real-time. Researchers can generate customizable reports, visualize data through charts and graphs, and identify trends and patterns within the data.
- Data Security and Compliance: QuestionPro prioritizes data security and compliance with regulations such as GDPR and HIPAA. The platform offers features such as SSL encryption, data masking, and secure data storage to ensure the confidentiality and integrity of collected data.
- Mobile Compatibility: With the increasing use of mobile devices, QuestionPro ensures that surveys are mobile-responsive, allowing respondents to participate in surveys conveniently from their smartphones or tablets.
- Integration Capabilities: QuestionPro integrates with various third-party tools and platforms, including CRMs, email marketing software, and analytics tools. This allows researchers to streamline their data collection processes and incorporate survey data into their existing workflows.
- Customization and Branding: Researchers can customize surveys with their branding elements, such as logos, colors, and themes, enhancing the professional appearance of surveys and increasing respondent engagement.
The conclusion you obtain from your investigation will set the course of the company’s decision-making, so present your report clearly and list the steps you followed to obtain those results.
Make sure that whoever will take the corresponding actions understands the importance of the information collected and that it gives them the solutions they expect.
QuestionPro offers a comprehensive suite of features and tools that can significantly streamline the data collection process, from survey creation to analysis, while ensuring data security and compliance. Remember that at QuestionPro, we can help you collect data easily and efficiently. Request a demo and learn about all the tools we have for you.
Frequently Asked Questions (FAQs)
A: Common methods include surveys, interviews, observations, focus groups, and experiments.
A: Data collection helps organizations make informed decisions and understand trends, customer preferences, and market demands.
A: Quantitative methods focus on numerical data and statistical analysis, while qualitative methods explore non-numerical insights like attitudes and behaviors.
A: Yes, combining methods can provide a more comprehensive understanding of the research topic.
A: Technology streamlines data collection with tools like online surveys, mobile data gathering, and integrated analytics platforms.
MORE LIKE THIS
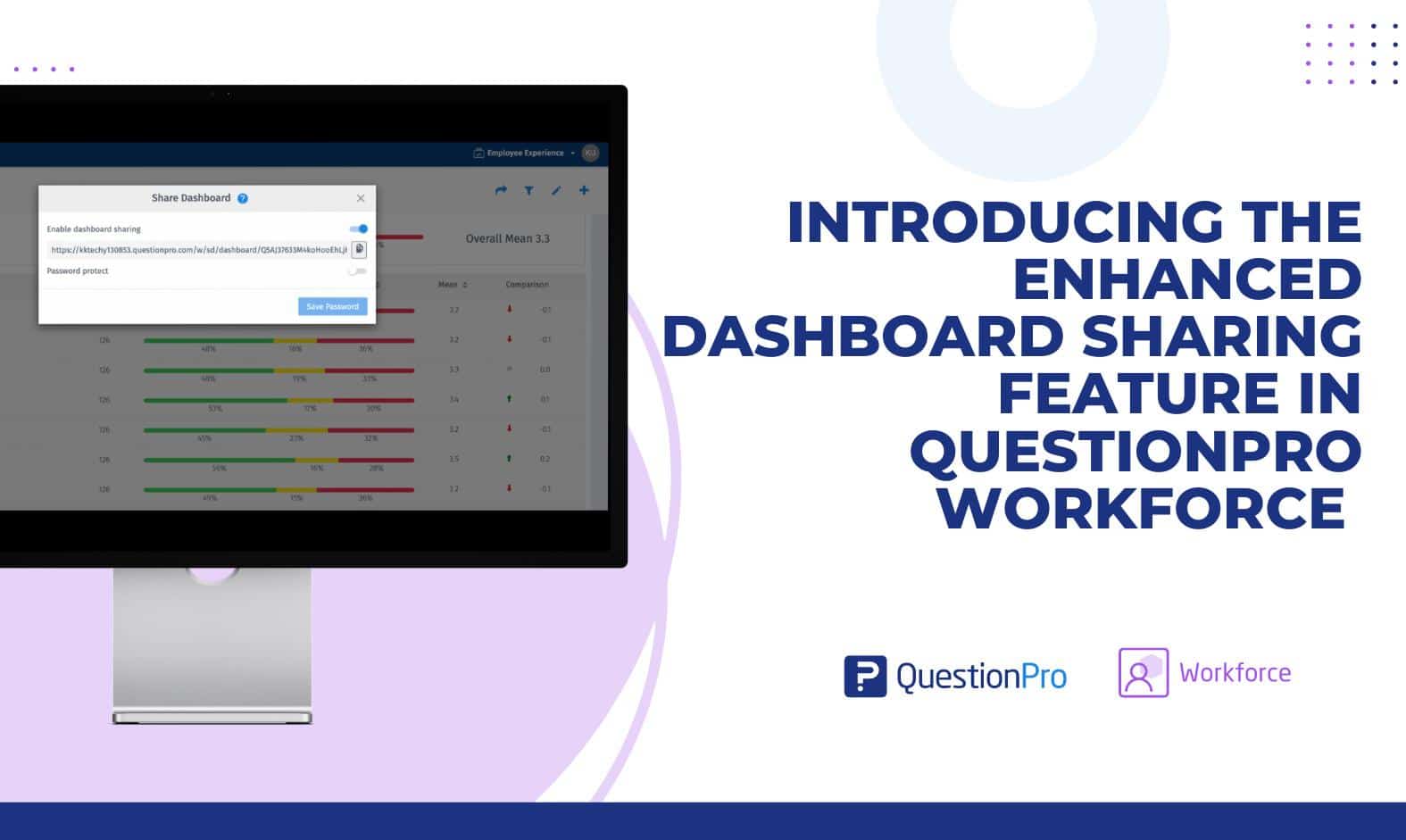
When You Have Something Important to Say, You want to Shout it From the Rooftops
Jun 28, 2024
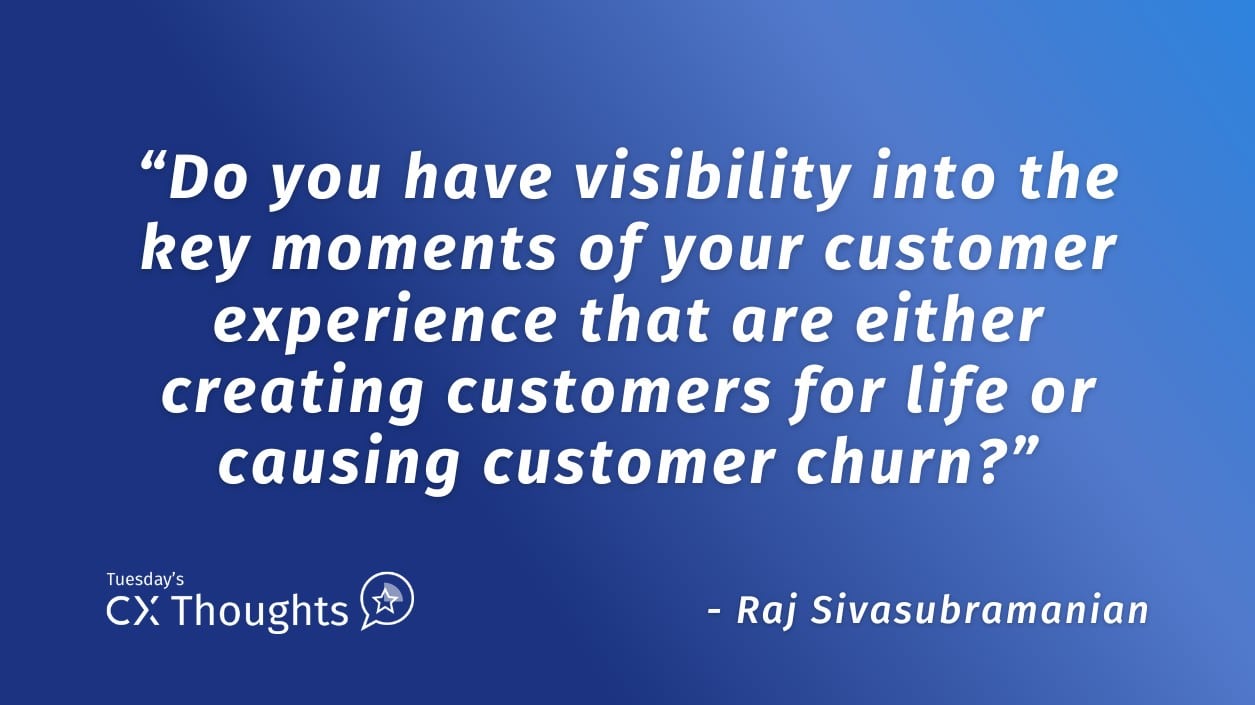
The Item I Failed to Leave Behind — Tuesday CX Thoughts
Jun 25, 2024
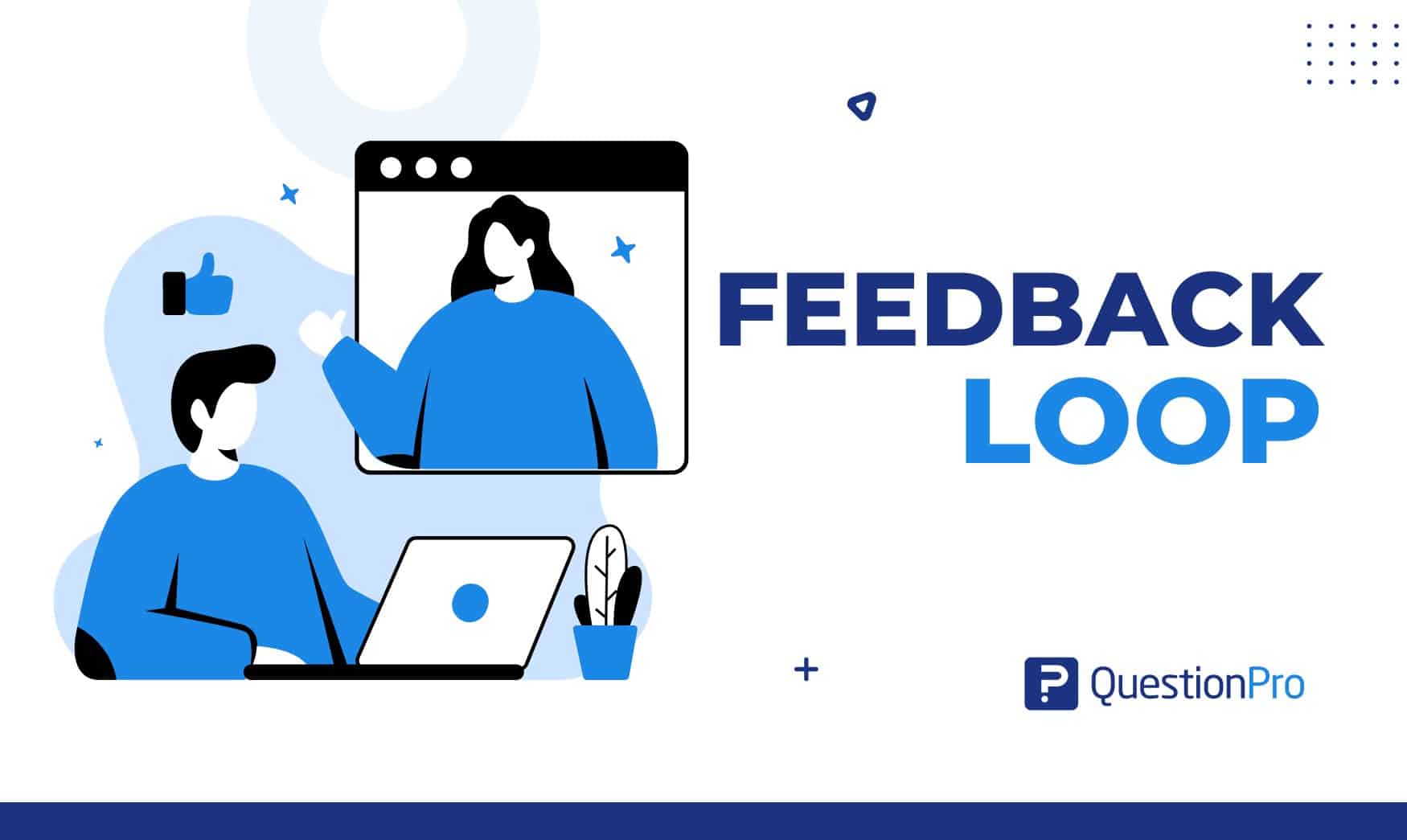
Feedback Loop: What It Is, Types & How It Works?
Jun 21, 2024
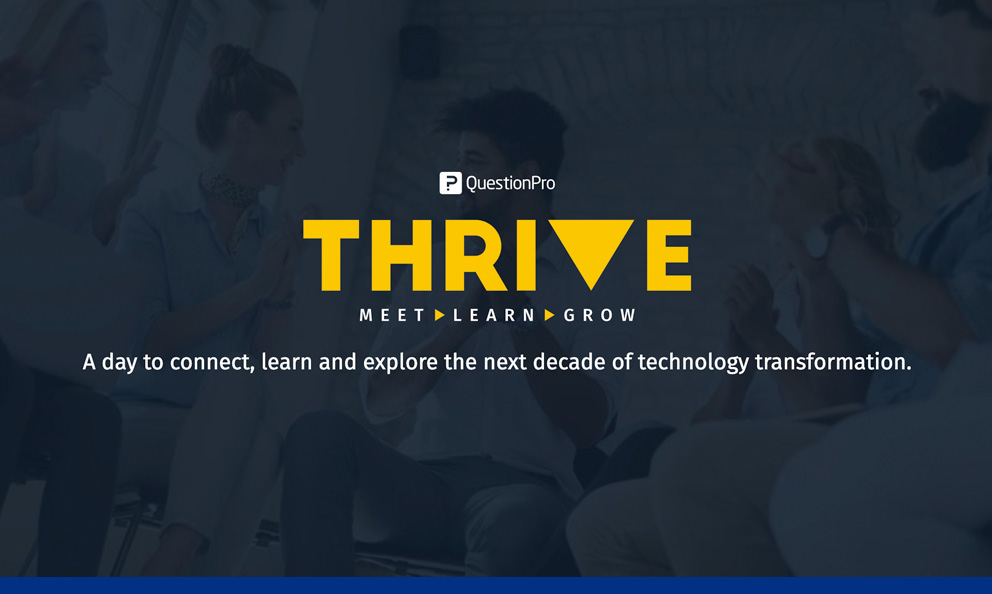
QuestionPro Thrive: A Space to Visualize & Share the Future of Technology
Jun 18, 2024
Other categories
- Academic Research
- Artificial Intelligence
- Assessments
- Brand Awareness
- Case Studies
- Communities
- Consumer Insights
- Customer effort score
- Customer Engagement
- Customer Experience
- Customer Loyalty
- Customer Research
- Customer Satisfaction
- Employee Benefits
- Employee Engagement
- Employee Retention
- Friday Five
- General Data Protection Regulation
- Insights Hub
- Life@QuestionPro
- Market Research
- Mobile diaries
- Mobile Surveys
- New Features
- Online Communities
- Question Types
- Questionnaire
- QuestionPro Products
- Release Notes
- Research Tools and Apps
- Revenue at Risk
- Survey Templates
- Training Tips
- Tuesday CX Thoughts (TCXT)
- Uncategorized
- Video Learning Series
- What’s Coming Up
- Workforce Intelligence

In order to continue enjoying our site, we ask that you confirm your identity as a human. Thank you very much for your cooperation.
- Privacy Policy

Home » Primary Data – Types, Methods and Examples
Primary Data – Types, Methods and Examples
Table of Contents

Primary Data
Definition:
Primary Data refers to data that is collected firsthand by a researcher or a team of researchers for a specific research project or purpose. It is original information that has not been previously published or analyzed, and it is gathered directly from the source or through the use of data collection methods such as surveys, interviews, observations, and experiments.
Types of Primary Data
Types of Primary Data are as follows:
Surveys are one of the most common types of primary data collection methods. They involve asking a set of standardized questions to a sample of individuals or organizations, usually through a questionnaire or an online form.
Interviews involve asking open-ended or structured questions to a sample of individuals or groups in person, over the phone, or through video conferencing. They can be conducted in a one-on-one setting or in a focus group.
Observations
Observations involve systematically recording the behavior or activities of individuals or groups in a natural or controlled setting. This type of data collection is often used in fields such as anthropology, sociology, and psychology.
Experiments
Experiments involve manipulating one or more variables and observing the effects on an outcome of interest. They are commonly used in scientific research to establish cause-and-effect relationships.
Case studies
Case studies involve in-depth analysis of a particular individual, group, or organization. They typically involve collecting a variety of data, including interviews, observations, and documents.
Action research
Action research involves collecting data to improve a specific practice or process within an organization or community. It often involves collaboration between researchers and practitioners.
Formats of Primary Data
Some common formats for primary data collection include:
- Textual data : This includes written responses to surveys or interviews, as well as written notes from observations.
- Numeric data: Numeric data includes data collected through structured surveys or experiments, such as ratings, rankings, or test scores.
- Audio data : Audio data includes recordings of interviews, focus groups, or other discussions.
- Visual data: Visual data includes photographs or videos of events, behaviors, or phenomena being studied.
- Sensor data: Sensor data includes data collected through electronic sensors, such as temperature readings, GPS data, or motion data.
- Biological data : Biological data includes data collected through biological samples, such as blood, urine, or tissue samples.
Primary Data Analysis Methods
There are several methods that can be used to analyze primary data collected from research, including:
- Descriptive statistics: Descriptive statistics involve summarizing and describing the characteristics of the data collected, such as mean, median, mode, and standard deviation.
- Inferential statistics: Inferential statistics involve making inferences about a population based on a sample of data. This can include techniques such as hypothesis testing and confidence intervals.
- Qualitative analysis: Qualitative analysis involves analyzing non-numerical data, such as textual data from interviews or observations, to identify themes, patterns, or trends.
- Content analysis: Content analysis involves analyzing textual data to identify and categorize specific words or phrases, allowing researchers to identify themes or patterns in the data.
- Coding : Coding involves categorizing data into specific categories or themes, allowing researchers to identify patterns and relationships in the data.
- Data visualization : Data visualization involves creating graphs, charts, and other visual representations of data to help researchers identify patterns and relationships in the data.
Primary Data Gathering Guide
Here are some general steps to guide you in gathering primary data:
- Define your research question or problem: Clearly define the purpose of your research and the specific questions you want to answer.
- Determine the data collection method : Decide which primary data collection method(s) will be most appropriate to answer your research question or problem.
- Develop a data collection instrument : If you are using surveys or interviews, create a structured questionnaire or interview guide to ensure that you ask the same questions of all participants.
- Identify your target population : Identify the group of individuals or organizations that will provide the data you need to answer your research question or problem.
- Recruit participants: Use various methods to recruit participants, such as email, social media, or advertising.
- Collect the data : Conduct your survey, interview, observation, or experiment, ensuring that you follow your data collection instrument.
- Verify the data : Check the data for completeness, accuracy, and consistency. Resolve any missing data or errors.
- Analyze the data: Use appropriate statistical or qualitative analysis techniques to interpret the data.
- Draw conclusions: Use the results of your analysis to answer your research question or problem.
- Communicate your findings : Share your results through a written report, presentation, or publication.
Examples of Primary Data
Some real-time examples of primary data are:
- Customer surveys: When a company collects data through surveys or questionnaires, they are gathering primary data. For example, a restaurant might ask customers to rate their dining experience.
- Market research : Companies may conduct primary research to understand consumer trends or market demand. For instance, a company might conduct interviews or focus groups to gather information about consumer preferences.
- Scientific experiments: Scientists may gather primary data through experiments, such as observing the behavior of animals or testing new drugs on human subjects.
- Traffic counts: Traffic engineers might collect primary data by monitoring the flow of cars on a particular road to determine how to improve traffic flow.
- Consumer behavior : Companies may use primary data to track consumer behavior, such as how customers use a product or interact with a website.
- Social media analytics : Companies can collect primary data by analyzing social media metrics such as likes, comments, and shares to understand how their customers are engaging with their brand.
Applications of Primary Data
Primary data is useful in a wide range of applications, including research, business, and government. Here are some specific applications of primary data:
- Research : Primary data is essential for conducting scientific research, such as in fields like psychology, sociology, and biology. Researchers collect primary data through experiments, surveys, and observations.
- Marketing : Companies use primary data to understand customer needs and preferences, track consumer behavior, and develop marketing strategies. This data is typically collected through surveys, focus groups, and other market research methods.
- Business planning : Primary data can inform business decisions such as product development, pricing strategies, and expansion plans. For example, a company may gather primary data on the buying habits of its customers to decide what products to offer and how to price them.
- Public policy: Primary data is used by government agencies to develop and evaluate public policies. For example, a city government might use primary data on traffic patterns to decide where to build new roads or improve public transportation.
- Education : Primary data is used in education to evaluate student performance, identify areas of need, and develop curriculum. Teachers may gather primary data through assessments, observations, and surveys to improve their teaching methods and help students succeed.
- Healthcare : Primary data is used by healthcare professionals to diagnose and treat illnesses, track patient outcomes, and develop new treatments. Doctors and researchers collect primary data through medical tests, clinical trials, and patient surveys.
- Environmental management: Primary data is used to monitor and manage natural resources and the environment. For example, scientists and environmental managers collect primary data on water quality, air quality, and biodiversity to develop policies and programs aimed at protecting the environment.
- Product testing: Companies use primary data to test new products before they are released to the market. This data is collected through surveys, focus groups, and product testing sessions to evaluate the effectiveness and appeal of the product.
- Crime prevention : Primary data is used by law enforcement agencies to identify crime hotspots, track criminal activity, and develop crime prevention strategies. Police departments may collect primary data through crime reports, surveys, and community meetings to better understand the needs and concerns of the community.
- Disaster response: Primary data is used by emergency responders and disaster management agencies to assess the impact of disasters and develop response plans. This data is collected through surveys, interviews, and observations to identify the needs of affected populations and allocate resources accordingly.
Purpose of Primary Data
The purpose of primary data is to gather information directly from the source, without relying on secondary sources or pre-existing data. This data is collected through research methods such as surveys, interviews, experiments, and observations. Primary data is valuable because it is tailored to the specific research question or problem at hand and is collected with a specific purpose in mind. Some of the main purposes of primary data include:
- To answer research questions: Researchers use primary data to answer specific research questions, such as understanding consumer preferences, evaluating the effectiveness of a program, or testing a hypothesis.
- To gather original information : Primary data provides new and original information that is not available from other sources. This data can be used to make informed decisions, develop new products, or design new programs.
- To tailor research methods: Primary data collection methods can be customized to fit the research question or problem. This allows researchers to gather the most relevant and accurate information possible.
- To control the quality of data: Researchers have greater control over the quality of primary data, as they can design and implement the data collection methods themselves. This reduces the risk of errors or biases that may be present in secondary data sources.
- To address specific populations : Primary data can be collected from specific populations, such as customers, patients, or students. This allows researchers to gather data that is directly relevant to their research question or problem.
When to use Primary Data
Primary data should be used when the specific information required for a research question or problem cannot be obtained from existing data sources. Here are some situations where primary data would be appropriate to use:
- When no secondary data is available: Primary data should be collected when there is no existing data available that addresses the research question or problem.
- When the available secondary data is not relevant: Existing secondary data may not be specific or relevant enough to address the research question or problem at hand.
- When the research requires specific information : Primary data collection allows researchers to gather information that is tailored to their specific research question or problem.
- When the research requires a specific population: Primary data can be collected from specific populations, such as customers, patients, or employees, to provide more targeted and relevant information.
- When the research requires control over the data collection process: Primary data allows researchers to have greater control over the data collection process, which can ensure the data is of high quality and relevant to the research question or problem.
- When the research requires current or up-to-date information: Primary data collection can provide more current and up-to-date information than existing secondary data sources.
Characteristics of Primary Data
Primary data has several characteristics that make it unique and valuable for research purposes. These characteristics include:
- Originality : Primary data is collected for a specific research question or problem and is not previously published or available in any other source.
- Relevance : Primary data is collected to directly address the research question or problem at hand and is therefore highly relevant to the research.
- Accuracy : Primary data collection methods can be designed to ensure the data is accurate and reliable, reducing the risk of errors or biases.
- Timeliness: Primary data is collected in real-time or near real-time, providing current and up-to-date information for the research.
- Specificity : Primary data can be collected from specific populations, such as customers, patients, or employees, providing targeted and relevant information.
- Control : Researchers have greater control over the data collection process, allowing them to ensure the data is collected in a way that is most relevant to the research question or problem.
- Cost : Primary data collection can be more expensive than using existing secondary data sources, as it requires resources such as personnel, equipment, and materials.
Advantages of Primary Data
There are several advantages of using primary data in research. These include:
- Specificity : Primary data collection can be tailored to the specific research question or problem, allowing researchers to gather the most relevant and targeted information possible.
- Control : Researchers have greater control over the data collection process, which can ensure the data is of high quality and relevant to the research question or problem.
- Timeliness : Primary data is collected in real-time or near real-time, providing current and up-to-date information for the research.
- Flexibility : Primary data collection methods can be adjusted or modified during the research process to ensure the most relevant and useful data is collected.
- Greater depth : Primary data collection methods, such as interviews or focus groups, can provide more in-depth and detailed information than existing secondary data sources.
- Potential for new insights : Primary data collection can provide new and unexpected insights into a research question or problem, which may not have been possible using existing secondary data sources.
Limitations of Primary Data
While primary data has several advantages, it also has some limitations that researchers need to be aware of. These limitations include:
- Time-consuming: Primary data collection can be time-consuming, especially if the research requires collecting data from a large sample or a specific population.
- Limited generalizability: Primary data is collected from a specific population, and therefore its generalizability to other populations may be limited.
- Potential bias: Primary data collection methods can be subject to biases, such as social desirability bias or interviewer bias, which can affect the accuracy and reliability of the data.
- Potential for errors: Primary data collection methods can be prone to errors, such as data entry errors or measurement errors, which can affect the accuracy and reliability of the data.
- Ethical concerns: Primary data collection methods, such as interviews or surveys, may raise ethical concerns related to confidentiality, privacy, and informed consent.
About the author
Muhammad Hassan
Researcher, Academic Writer, Web developer
You may also like

Information in Research – Types and Examples

Qualitative Data – Types, Methods and Examples

Research Data – Types Methods and Examples

Secondary Data – Types, Methods and Examples

Quantitative Data – Types, Methods and Examples
Statistics/Methods of Data Collection/Experiments
- What Is Statistics?
- Subjects in Modern Statistics
- What Do I Need to Know to Learn Statistics?
- Primary and Secondary Data
- Quantitative and Qualitative Data
Experiments
- Sample Surveys
- Observational Studies
- Data Cleaning
- Moving Average
- Mean, Median, and Mode
- Geometric Mean
- Harmonic Mean
- Relationships among Arithmetic, Geometric, and Harmonic Mean
- Geometric Median
- Range of the Data
- Variance and Standard Deviation
- Quartiles and Quartile Range
- Comparative Bar Charts
- Scatter Plots
- Comparative Pie Charts
- Line Graphs
- Frequency Polygon
- Combinatorics
- Bernoulli Trials
- Introductory Bayesian Analysis
- Uniform Distribution
- Bernoulli Distribution
- Binomial Distribution
- Poisson Distribution
- Geometric Distribution
- Negative Binomial Distribution
- Hypergeometric Distribution
- Exponential Distribution
- Gamma Distribution
- Normal Distribution
- Chi-Square Distribution
- Student-t Distribution
- F Distribution
- Beta Distribution
- Weibull Distribution
- Purpose of Statistical Tests
- Formalism Used
- Different Types of Tests
- z Test for a Single Mean
- z Test for Two Means
- t Test for a single mean
- t Test for Two Means
- paired t Test for comparing Means
- One-Way ANOVA F Test
- z Test for a Single Proportion
- z Test for Two Proportions
- Testing whether Proportion A Is Greater than Proportion B in Microsoft Excel
- Spearman's Rank Coefficient
- Pearson's Product Moment Correlation Coefficient
- Chi-Squared Test for Multiple Proportions
- Chi-Squared Test for Contingency
- Approximations of distributions
- Unbiasedness
- Measures of goodness
- Completeness
- Sufficiency and Minimal Sufficiency
- Ancillarity
- Summary Statistics Problems
- Data-Display Problems
- Distributions Problems
- Data-Testing Problems
- Basic Linear Algebra and Gram-Schmidt Orthogonalization
- Unconstrained Optimization
- Quantile Regression
- Numerical Comparison of Statistical Software
- Numerics in Excel
- Statistics/Numerical_Methods/Random Number Generation
- Time Series Analysis
- Principal Component Analysis
- Factor Analysis for metrical data
- Factor Analysis for ordinal data
- Canonical Correlation Analysis
- Discriminant Analysis
- Analysis of Tuberculosis
edit this box
In a experiment the experimenter applies 'treatments' to groups of subjects. For example the experimenter may give one drug to group 1 and a different drug or a placebo to group 2, to determine the effectiveness of the drug. This is what differentiates an 'experiment' from an 'observational study'.
Scientists try to identify cause-and-effect relationships because this kind of knowledge is especially powerful, for example, drug A cures disease B. Various methods exist for detecting cause-and-effect relationships. An experiment is a method that most clearly shows cause-and-effect because it isolates and manipulates a single variable, in order to clearly show its effect. Experiments almost always have two distinct variables: First, an independent variable (IV) is manipulated by an experimenter to exist in at least two levels (usually "none" and "some"). Then the experimenter measures the second variable, the dependent variable (DV).
So, the reason scientists utilize experiments is that it is the only way to determine causal relationships between variables. Experiments tend to be artificial because they try to make both groups identical with the single exception of the levels of the independent variable.
- Book:Statistics
Navigation menu
Thank you for visiting nature.com. You are using a browser version with limited support for CSS. To obtain the best experience, we recommend you use a more up to date browser (or turn off compatibility mode in Internet Explorer). In the meantime, to ensure continued support, we are displaying the site without styles and JavaScript.
- View all journals
- Explore content
- About the journal
- Publish with us
- Sign up for alerts
- Published: 01 July 2024
Time of sample collection is critical for the replicability of microbiome analyses
- Celeste Allaband ORCID: orcid.org/0000-0003-1832-4858 1 , 2 , 3 ,
- Amulya Lingaraju 2 ,
- Stephany Flores Ramos ORCID: orcid.org/0000-0002-1918-9769 1 , 2 , 3 ,
- Tanya Kumar 4 ,
- Haniyeh Javaheri 2 ,
- Maria D. Tiu 2 ,
- Ana Carolina Dantas Machado 2 ,
- R. Alexander Richter 2 ,
- Emmanuel Elijah 5 , 6 ,
- Gabriel G. Haddad 3 , 7 , 8 ,
- Vanessa A. Leone 9 ,
- Pieter C. Dorrestein ORCID: orcid.org/0000-0002-3003-1030 3 , 5 , 6 , 10 ,
- Rob Knight ORCID: orcid.org/0000-0002-0975-9019 3 , 6 , 11 , 12 , 13 &
- Amir Zarrinpar ORCID: orcid.org/0000-0001-6423-5982 2 , 6 , 13 , 14 , 15
Nature Metabolism ( 2024 ) Cite this article
Metrics details
- Animal disease models
- Circadian regulation
- Research management
As the microbiome field moves from descriptive and associative research to mechanistic and interventional studies, being able to account for all confounding variables in the experimental design, which includes the maternal effect 1 , cage effect 2 , facility differences 3 , as well as laboratory and sample handling protocols 4 , is critical for interpretability of results. Despite significant procedural and bioinformatic improvements, unexplained variability and lack of replicability still occur. One underexplored factor is that the microbiome is dynamic and exhibits diurnal oscillations that can change microbiome composition 5 , 6 , 7 . In this retrospective analysis of 16S amplicon sequencing studies in male mice, we show that sample collection time affects the conclusions drawn from microbiome studies and its effect size is larger than those of a daily experimental intervention or dietary changes. The timing of divergence of the microbiome composition between experimental and control groups is unique to each experiment. Sample collection times as short as only 4 hours apart can lead to vastly different conclusions. Lack of consistency in the time of sample collection may explain poor cross-study replicability in microbiome research. The impact of diurnal rhythms on the outcomes and study design of other fields is unknown but likely significant.
This is a preview of subscription content, access via your institution
Access options
Access Nature and 54 other Nature Portfolio journals
Get Nature+, our best-value online-access subscription
24,99 € / 30 days
cancel any time
Subscribe to this journal
Receive 12 digital issues and online access to articles
111,21 € per year
only 9,27 € per issue
Buy this article
- Purchase on Springer Link
- Instant access to full article PDF
Prices may be subject to local taxes which are calculated during checkout
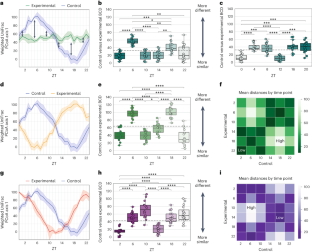
Data availability
Literature review data are at https://github.com/knightlab-analyses/dynamics/data/ . Figure 1 , mock data are at https://github.com/knightlab-analyses/dynamics/data/MockData . Figure 2 (Allaband/Zarrinpar 2021) data are under EBI accession ERP110592 . Figure 3 data (longitudinal IHC) are under EBI accession ERP110592 and (longitudinal circadian TRF) EBI accession ERP123226 . Figure 4 data (Zarrinpar/Panda 2014) are in the Supplementary Excel file attached to the source paper 13 ; (Leone/Chang 2015) figshare for the 16S amplicon sequence data are at https://doi.org/10.6084/m9.figshare.882928 (ref. 63 ). Extended Data Fig. 2 data (Caporaso/Knight 2011) are at MG-RAST project mgp93 (IDs mgm4457768.3 and mgm4459735.3). Extended Data Fig. 3 data (Wu/Chen 2018) are under ENA accession PRJEB22049 . Extended Data Fig. 4 data (Tuganbaev/Elinav 2021) are under ENA accession PRJEB38869 .
Code availability
All relevant code notebooks are on GitHub at https://github.com/knightlab-analyses/dynamics/notebooks .
Schloss, P. D. Identifying and overcoming threats to reproducibility, replicability, robustness, and generalizability in microbiome research. mBio 9 , e00525–18 (2018).
Article PubMed PubMed Central Google Scholar
Gilbert, J. A. et al. Current understanding of the human microbiome. Nat. Med. 24 , 392–400 (2018).
Article CAS PubMed PubMed Central Google Scholar
Knight, R. et al. Best practices for analysing microbiomes. Nat. Rev. Microbiol. 16 , 410–422 (2018).
Article CAS PubMed Google Scholar
Ley, R. E. et al. Obesity alters gut microbial ecology. Proc. Natl Acad. Sci. USA 102 , 11070–11075 (2005).
Deloris Alexander, A. et al. Quantitative PCR assays for mouse enteric flora reveal strain-dependent differences in composition that are influenced by the microenvironment. Mamm. Genome 17 , 1093–1104 (2006).
Friswell, M. K. et al. Site and strain-specific variation in gut microbiota profiles and metabolism in experimental mice. PLoS ONE 5 , e8584 (2010).
Sinha, R. et al. Assessment of variation in microbial community amplicon sequencing by the Microbiome Quality Control (MBQC) project consortium. Nat. Biotechnol. 35 , 1077–1086 (2017).
Alvarez, Y., Glotfelty, L. G., Blank, N., Dohnalová, L. & Thaiss, C. A. The microbiome as a circadian coordinator of metabolism. Endocrinology 161 , bqaa059 (2020).
Frazier, K. & Chang, E. B. Intersection of the gut microbiome and circadian rhythms in metabolism. Trends Endocrinol. Metab. 31 , 25–36 (2020).
Heddes, M. et al. The intestinal clock drives the microbiome to maintain gastrointestinal homeostasis. Nat. Commun. 13 , 6068 (2022).
Leone, V. et al. Effects of diurnal variation of gut microbes and high-fat feeding on host circadian clock function and metabolism. Cell Host Microbe 17 , 681–689 (2015).
Thaiss, C. A. et al. Transkingdom control of microbiota diurnal oscillations promotes metabolic homeostasis. Cell 159 , 514–529 (2014).
Zarrinpar, A., Chaix, A., Yooseph, S. & Panda, S. Diet and feeding pattern affect the diurnal dynamics of the gut microbiome. Cell Metab. 20 , 1006–1017 (2014).
Liang, X., Bushman, F. D. & FitzGerald, G. A. Rhythmicity of the intestinal microbiota is regulated by gender and the host circadian clock. Proc. Natl Acad. Sci. USA 112 , 10479–10484 (2015).
Thaiss, C. A. et al. Microbiota diurnal rhythmicity programs host transcriptome oscillations. Cell 167 , 1495–1510 (2016).
Yu, F. et al. Deficiency of intestinal Bmal1 prevents obesity induced by high-fat feeding. Nat. Commun. 12 , 5323 (2021).
Leone, V. A. et al. Atypical behavioral and thermoregulatory circadian rhythms in mice lacking a microbiome. Sci. Rep. 12 , 14491 (2022).
Thaiss, C. A., Zeevi, D., Levy, M., Segal, E. & Elinav, E. A day in the life of the meta-organism: diurnal rhythms of the intestinal microbiome and its host. Gut Microbes 6 , 137–142 (2015).
Mukherji, A., Kobiita, A., Ye, T. & Chambon, P. Homeostasis in intestinal epithelium is orchestrated by the circadian clock and microbiota cues transduced by TLRs. Cell 153 , 812–827 (2013).
Weger, B. D. et al. The mouse microbiome is required for sex-specific diurnal rhythms of gene expression and metabolism. Cell Metab. 29 , 362–382 (2019).
Kaczmarek, J. L., Musaad, S. M. & Holscher, H. D. Time of day and eating behaviors are associated with the composition and function of the human gastrointestinal microbiota. Am. J. Clin. Nutr. 106 , 1220–1231 (2017).
Skarke, C. et al. A pilot characterization of the human chronobiome. Sci. Rep. 7 , 17141 (2017).
Jones, J., Reinke, S. N., Ali, A., Palmer, D. J. & Christophersen, C. T. Fecal sample collection methods and time of day impact microbiome composition and short chain fatty acid concentrations. Sci. Rep. 11 , 13964 (2021).
Collado, M. C. et al. Timing of food intake impacts daily rhythms of human salivary microbiota: a randomized, crossover study. FASEB J. 32 , 2060–2072 (2018).
Kohn, J. N. et al. Differing salivary microbiome diversity, community and diurnal rhythmicity in association with affective state and peripheral inflammation in adults. Brain. Behav. Immun. 87 , 591–602 (2020).
Takayasu, L. et al. Circadian oscillations of microbial and functional composition in the human salivary microbiome. DNA Res. 24 , 261–270 (2017).
Reitmeier, S. et al. Arrhythmic gut microbiome signatures predict risk of type 2 diabetes. Cell Host Microbe 28 , 258–272 (2020).
Allaband, C. et al. Intermittent hypoxia and hypercapnia alter diurnal rhythms of luminal gut microbiome and metabolome. mSystems https://doi.org/10.1128/mSystems.00116-21 (2021).
Tuganbaev, T. et al. Diet diurnally regulates small intestinal microbiome-epithelial-immune homeostasis and enteritis. Cell 182 , 1441–1459 (2020).
Wu, G. et al. Light exposure influences the diurnal oscillation of gut microbiota in mice. Biochem. Biophys. Res. Commun. 501 , 16–23 (2018).
Nelson, R. J. et al. Time of day as a critical variable in biology. BMC Biol. 20 , 142 (2022).
Dantas Machado, A. C. et al. Diet and feeding pattern modulate diurnal dynamics of the ileal microbiome and transcriptome. Cell Rep. 40 , 111008 (2022).
Morton, J. T. et al. Establishing microbial composition measurement standards with reference frames. Nat. Commun. 10 , 2719 (2019).
Caporaso, J. G. et al. Moving pictures of the human microbiome. Genome Biol. 12 , R50 (2011).
Bisanz, J. E., Upadhyay, V., Turnbaugh, J. A., Ly, K. & Turnbaugh, P. J. Meta-analysis reveals reproducible gut microbiome alterations in response to a high-fat diet. Cell Host Microbe 26 , 265–272.e4 (2019).
Kohsaka, A. et al. High-fat diet disrupts behavioral and molecular circadian rhythms in mice. Cell Metab. 6 , 414–421 (2007).
Hatori, M. et al. Time-restricted feeding without reducing caloric intake prevents metabolic diseases in mice fed a high-fat diet. Cell Metab. 15 , 848–860 (2012).
Baker, F. Normal rumen microflora and microfauna of cattle. Nature 149 , 220 (1942).
Article Google Scholar
Zhang, L., Wu, W., Lee, Y.-K., Xie, J. & Zhang, H. Spatial heterogeneity and co-occurrence of mucosal and luminal microbiome across swine intestinal tract. Front. Microbiol. 9 , 48 (2018).
Klymiuk, I. et al. Characterization of the luminal and mucosa-associated microbiome along the gastrointestinal tract: results from surgically treated preterm infants and a murine model. Nutrients 13 , 1030 (2021).
Kim, D. et al. Comparison of sampling methods in assessing the microbiome from patients with ulcerative colitis. BMC Gastroenterol. 21 , 396 (2021).
Tripathi, A. et al. Intermittent hypoxia and hypercapnia reproducibly change the gut microbiome and metabolome across rodent model systems. mSystems 4 , e00058–19 (2019).
Uhr, G. T., Dohnalová, L. & Thaiss, C. A. The Dimension of Time in Host-Microbiome Interactions. mSystems 4 , e00216–e00218 (2019).
Voigt, R. M. et al. Circadian disorganization alters intestinal microbiota. PLoS ONE 9 , e97500 (2014).
McDonald, D. et al. American gut: an open platform for citizen science microbiome research. mSystems 3 , e00031–18 (2018).
Borodulin, K. et al. Cohort profile: the National FINRISK Study. Int. J. Epidemiol. 47 , 696–696i (2018).
Article PubMed Google Scholar
Ren, B. et al. Methionine restriction improves gut barrier function by reshaping diurnal rhythms of inflammation-related microbes in aged mice. Front. Nutr. 8 , 746592 (2021).
Beli, E., Prabakaran, S., Krishnan, P., Evans-Molina, C. & Grant, M. B. Loss of diurnal oscillatory rhythms in gut microbiota correlates with changes in circulating metabolites in type 2 diabetic db/db mice. Nutrients 11 , E2310 (2019).
Wang, L. et al. Methionine restriction regulates cognitive function in high-fat diet-fed mice: roles of diurnal rhythms of SCFAs producing- and inflammation-related microbes. Mol. Nutr. Food Res. 64 , e2000190 (2020).
Guo, T. et al. Oolong tea polyphenols ameliorate circadian rhythm of intestinal microbiome and liver clock genes in mouse model. J. Agric. Food Chem. 67 , 11969–11976 (2019).
Mistry, P. et al. Circadian influence on the microbiome improves heart failure outcomes. J. Mol. Cell. Cardiol. 149 , 54–72 (2020).
Shao, Y. et al. Effects of sleeve gastrectomy on the composition and diurnal oscillation of gut microbiota related to the metabolic improvements. Surg. Obes. Relat. Dis. 14 , 731–739 (2018).
Bolyen, E. et al. Reproducible, interactive, scalable and extensible microbiome data science using QIIME 2. Nat. Biotechnol. 37 , 852–857 (2019).
Amir, A. et al. Deblur rapidly resolves single-nucleotide community sequence patterns. mSystems 2 , e00191–16 (2017).
Mirarab, S., Nguyen, N. & Warnow, T. in Biocomputing 2012 , 247–258 (World Scientific, 2011).
Lozupone, C., Lladser, M. E., Knights, D., Stombaugh, J. & Knight, R. UniFrac: an effective distance metric for microbial community comparison. ISME J. 5 , 169–172 (2011).
Lauber, C. L., Zhou, N., Gordon, J. I., Knight, R. & Fierer, N. Effect of storage conditions on the assessment of bacterial community structure in soil and human-associated samples: Influence of short-term storage conditions on microbiota. FEMS Microbiol. Lett. 307 , 80–86 (2010).
Marotz, C. et al. Evaluation of the effect of storage methods on fecal, saliva, and skin microbiome composition. mSystems 6 , e01329–20 (2021).
Song, S. J. et al. Preservation methods differ in fecal microbiome stability, affecting suitability for field studies. mSystems 1 , e00021–16 (2016).
Wu, G. D. et al. Sampling and pyrosequencing methods for characterizing bacterial communities in the human gut using 16S sequence tags. BMC Microbiol. 10 , 206 (2010).
Piedrahita, J. A., Zhang, S. H., Hagaman, J. R., Oliver, P. M. & Maeda, N. Generation of mice carrying a mutant apolipoprotein E gene inactivated by gene targeting in embryonic stem cells. Proc. Natl Acad. Sci. USA 89 , 4471–4475 (1992).
Chaix, A., Zarrinpar, A., Miu, P. & Panda, S. Time-restricted feeding is a preventative and therapeutic intervention against diverse nutritional challenges. Cell Metab. 20 , 991–1005 (2014).
Gibbons, S. Diel Mouse Gut Study (HF/LF diet) . figshare https://doi.org/10.6084/m9.figshare.882928 (2015).
Download references
Acknowledgements
C.A. was supported by NIH T32 OD017863. S.F.R. is supported by the Soros Foundation. A.L. is supported by the AHA Postdoctoral Fellowship grant. T.K. is supported by NIH T32 GM719876. A.C.D.M. is supported by R01 HL148801-02S1. G.G.H. and A.Z. are supported by NIH R01 HL157445. A.Z. is further supported by the VA Merit BLR&D Award I01 BX005707 and NIH grants R01 AI163483, R01 HL148801, R01 EB030134 and U01 CA265719. All authors receive institutional support from NIH P30 DK120515, P30 DK063491, P30 CA014195, P50 AA011999 and UL1 TR001442.
Author information
Authors and affiliations.
Division of Biomedical Sciences, University of California, San Diego, La Jolla, CA, USA
Celeste Allaband & Stephany Flores Ramos
Division of Gastroenterology, University of California, San Diego, La Jolla, CA, USA
Celeste Allaband, Amulya Lingaraju, Stephany Flores Ramos, Haniyeh Javaheri, Maria D. Tiu, Ana Carolina Dantas Machado, R. Alexander Richter & Amir Zarrinpar
Department of Pediatrics, University of California, San Diego, La Jolla, CA, USA
Celeste Allaband, Stephany Flores Ramos, Gabriel G. Haddad, Pieter C. Dorrestein & Rob Knight
Medical Scientist Training Program, University of California San Diego, La Jolla, CA, USA
Tanya Kumar
Skaggs School of Pharmacy and Pharmaceutical Sciences, University of California, San Diego, La Jolla, CA, USA
Emmanuel Elijah & Pieter C. Dorrestein
Center for Microbiome Innovation, University of California, San Diego, La Jolla, CA, USA
Emmanuel Elijah, Pieter C. Dorrestein, Rob Knight & Amir Zarrinpar
Department of Neurosciences, University of California, San Diego, La Jolla, CA, USA
Gabriel G. Haddad
Rady Children’s Hospital, San Diego, CA, USA
Department of Animal and Dairy Sciences, University of Wisconsin-Madison, Madison, WI, USA
Vanessa A. Leone
Center for Computational Mass Spectrometry, University of California, San Diego, La Jolla, CA, USA
Pieter C. Dorrestein
Department of Computer Science and Engineering, University of California, San Diego, La Jolla, CA, USA
Halıcıoğlu Data Science Institute, University of California, San Diego, La Jolla, CA, USA
Shu Chien-Gene Lay Department of Bioengineering, University of California, San Diego, La Jolla, CA, USA
Rob Knight & Amir Zarrinpar
Division of Gastroenterology, Jennifer Moreno Department of Veterans Affairs Medical Center, La Jolla, CA, USA
Amir Zarrinpar
Institute of Diabetes and Metabolic Health, University of California, San Diego, La Jolla, CA, USA
You can also search for this author in PubMed Google Scholar
Contributions
C.A. and A.Z. conceptualized the work. C.A., E.E., P.C.D., R.K. and A.Z. determined the methodology. C.A., A.L., S.F.R., T.K., H.J., M.D.T., A.C.D.M. and R.A.R. were involved in data investigation. C.A., S.F.R., T.K., H.J., M.D.T., A.C.D.M. and R.A.R. created visualizations. A.Z. acquired funding and was the project administrator. R.K. and A.Z. supervised the work. G.G.H. and V.A.L. provided resources. C.A., A.L., S.F.R., T.K., H.J., M.D.T. and A.Z. wrote the first draft. All authors contributed to the review and editing of the manuscript.
Corresponding author
Correspondence to Amir Zarrinpar .
Ethics declarations
Competing interests.
A.Z. is a co-founder and a chief medical officer, and holds equity in Endure Biotherapeutics. P.C.D. is an advisor to Cybele and co-founder and advisor to Ometa and Enveda with previous approval from the University of California, San Diego. All other authors declare no competing interests.
Peer review
Peer review information.
Nature Metabolism thanks Robin Voigt-Zuwala, Jacqueline M. Kimmey, John R. Kirby and the other, anonymous, reviewer(s) for their contribution to the peer review of this work. Primary Handling Editor: Yanina-Yasmin Pesch, in collaboration with the Nature Metabolism team.
Additional information
Publisher’s note Springer Nature remains neutral with regard to jurisdictional claims in published maps and institutional affiliations.
Extended data
Extended data fig. 1 microbiome literature review..
A ) 2019 Literature Review Summary. Of the 586 articles containing microbiome (16 S or metagenomic) data, found as described in the methods section, the percentage of microbiome articles from each of the publication groups. B ) The percentage of microbiome articles belonging to each individual journal in 2019. Because the numerous individual journals from Science represented low percentages individually, they were grouped together. C ) The percentage articles where collection time was explicitly stated (yes: 8 AM, ZT4, etc.), implicitly stated (relative: ‘before surgery’, ‘in the morning’, etc.), or unstated (not provided: ‘daily’, ‘once a week’, etc.). D ) Meta-Analysis Inclusion Criteria Flow Chart. Literature review resulting in the five previously published datasets for meta-analysis 11 , 13 , 28 , 29 , 30 .
Extended Data Fig. 2 Single Time Point (Non-Circadian) Example.
A ) Weighted UniFrac PCoA Plot - modified example from Moving Pictures Qiime2 tutorial data [ https://docs.qiime2.org/2022.11/tutorials/moving-pictures/ ]. Each point is a sample. Points were coloured by body site of origin. There are 8 gut, 8 left palm, 9 right palm, and 9 tongue samples. B ) Within-Condition Distances (WCD) boxplot/stripplot for each body site (n = 8–9 mouse per group per time point). C ) Between Condition Distances (BCD) boxplot/stripplot for each unique body site comparison (n = 8–9 mouse per group per time point). D ) All pairwise grouping comparisons, both WCD and BCD, are shown in the boxplots/stripplots (n = 8–9 mouse per group per time point). Only WCD to BCD statistical differences are shown. Boxplot centre line indicates median, edges of boxes are quartiles, error bars are min and max values. Significance was determined using a paired Mann-Whitney-Wilcoxon test two-sided with Bonferroni correction. Notation: ns (not significant) = p > 0.05, * = p < 0.05; ** = p < 0.01; *** = p < 0.001, **** = p < 0.00001.
Extended Data Fig. 3 Additional Analysis of Apoe-/- Mice Exposed to IHC Conditions.
A ) Weighted UniFrac PCoA stacked view (same as Fig. 2b but different orientation). Good for assessing overall similarity not broken down by time point. Significance determined by PERMANOVA (p = 0.005). B ) Weighted UniFrac PCoA of only axis 1 over time. C ) Boxplot/scatterplot of within-group weighted UniFrac distance values for the control group (Air, n = 3–4 samples per time point). Unique non-zero values in the matrix were kept. Dotted line indicates the mean of all values presented. No significant differences (p > 0.05) found. D ) Boxplot/scatterplot of within-group weighted UniFrac distance values for the experimental group (IHC, n = 3–4 samples per time point)). Unique non-zero values in the matrix were kept. Dotted line indicates the mean of all values presented. No significant differences (p > 0.05) found. E ) Boxplot/scatterplot of within-group weighted UniFrac distance values for both control (Air) and experimental (IHC) groups [n = 3–4 samples per group per time point]. Mann-Whitney-Wilcoxon test with Bonferroni correction used to determine significant differences between groups. Boxplot centre line indicates median, edges of boxes are quartiles, error bars are min and max values. Notation: ns = not significant, p > 0.05; * = p < 0.05; ** = p < 0.01; *** = p < 0.001.
Extended Data Fig. 4 Irregular differences in diurnal rhythm patterns leads to generally minor shifts in BCD when comparing LD vs DD mice.
A ) Experimental design. Balb/c mice were fed NCD ad libitum under 0:24 L:D (24 hr darkness, DD) experimental conditions and compared to 12:12 L:D (LD) control conditions. After 2 weeks, mice from each group were euthanized every 4 hours for 24 hours (N = 4–5 mice/condition) and samples were collected from the proximal small intestine (‘jejunum’) and distal small intestine (‘ileum’) contents. B ) BCD for luminal contents of proximal small intestine samples comparing LD to DD mice (N = 4–5 mice/condition). Dotted line is the average of all shown weighted UniFrac distances. Significance was determined using a paired Mann-Whitney-Wilcoxon test two-sided with Bonferroni correction; notation: **** = p < 0.00001. C ) BCD for luminal contents of distal small intestine samples comparing LD to DD mice (N = 4–5 mice/condition). Dotted line is the average of all shown weighted UniFrac distances. Boxplot centre line indicates median, edges of boxes are quartiles, error bars are min and max values.
Extended Data Fig. 5 Localized changes in BCD between luminal and mucosal contents.
A ) Experimental design and sample collection for a local site study. Small intestinal samples were collected every 4 hours for 24 hours (N = 4–5 mice/condition, skipping ZT8). Mice were fed ad libitum on the same diet (NCD) for 4 weeks before samples were taken. B ) BCD for luminal vs mucosal conditions (N = 4–5 mice/condition). The dotted line is the average of all shown weighted UniFrac distances. Significance is determined using the Mann-Whitney-Wilcoxon test two-sided with Bonferroni correction. C ) Heatmap of mean BCD distances comparing luminal and mucosal by time point (N = 4–5 mice/condition). Highest value highlighted in navy, lowest value highlighted in gold. Boxplot centre line indicates median, edges of boxes are quartiles, error bars are min and max values. Significance was determined using a paired Mann-Whitney-Wilcoxon test two-sided with Bonferroni correction. Notation: * = p < 0.05; ** = p < 0.01; *** = p < 0.001, **** = p < 0.00001. D ) Experimentally relevant log ratio, highlighting the changes seen at ZT20 (N = 4–5 mice/condition). Boxplot center line indicates median, edges of boxes are quartiles, error bars are min and max values. Significance was determined using a paired Mann-Whitney-Wilcoxon test two-sided with Bonferroni correction. Notation: * = p < 0.05; ** = p < 0.01; *** = p < 0.001, **** = p < 0.00001.
Supplementary information
Reporting summary, rights and permissions.
Reprints and permissions
About this article
Cite this article.
Allaband, C., Lingaraju, A., Flores Ramos, S. et al. Time of sample collection is critical for the replicability of microbiome analyses. Nat Metab (2024). https://doi.org/10.1038/s42255-024-01064-1
Download citation
Received : 27 October 2022
Accepted : 08 May 2024
Published : 01 July 2024
DOI : https://doi.org/10.1038/s42255-024-01064-1
Share this article
Anyone you share the following link with will be able to read this content:
Sorry, a shareable link is not currently available for this article.
Provided by the Springer Nature SharedIt content-sharing initiative
Quick links
- Explore articles by subject
- Guide to authors
- Editorial policies
Sign up for the Nature Briefing newsletter — what matters in science, free to your inbox daily.

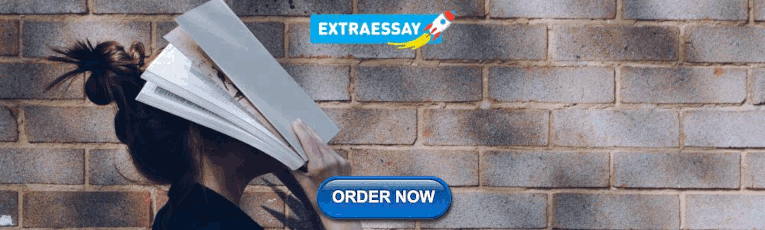
COMMENTS
Data collection is a systematic method of obtaining, observing, measuring, and analyzing accurate information. An experimental study is a standard method of data collection that involves the manipulation of the samples by applying some form of treatment prior to data collection. It refers to manipulating one variable to determine its changes on ...
Data collection methods; Method When to use How to collect data; Experiment: To test a causal relationship. Manipulate variables and measure their effects on others. Survey: To understand the general characteristics or opinions of a group of people. Distribute a list of questions to a sample online, in person, or over the phone. Interview/focus ...
An experiment is a data collection procedure that occurs in controlled conditions to identify and understand causal relationships between variables. Researchers can use many potential designs. The ultimate choice depends on their research question, resources, goals, and constraints. In some fields of study, researchers refer to experimental ...
An experiment is a data collection method where you as a researcher change some variables and observe their effect on other variables. The variables that you manipulate are referred to as independent while the variables that change as a result of manipulation are dependent variables. Imagine a manufacturer is testing the effect of drug strength ...
Experimental design is a process of planning and conducting scientific experiments to investigate a hypothesis or research question. It involves carefully designing an experiment that can test the hypothesis, and controlling for other variables that may influence the results. ... Experimental design data collection methods are techniques and ...
The frequencies expected by chance are calculated by multiplying the frequency for the row times the frequency for the column and dividing by the total number of observations (N). Kappa is calculated by using fO and fC on the diagonal where the categories match. Thus: fO - fC (50 + 20) - (42 + 12) 70 - 54 16.
the other hand, although a suitable data collection method helps to plan good research, it cannot necessarily guarantee the overall success of the research project (Olsen, 2012). II. TYPES OF DATA Before selecting a data collection method, the type of data that is required for the study should be determined (Kabir, 2016).
Data collection: experiments and focus groups 167 Flexibility of design In qualitative research, the research design is as flexible as the methods, allowing for modification in order to adjust it to the data. In quantitative research the design is developed at the beginning of the project and deviations of any kind are not
The investigator knows at this stage not only what be studied, but also who to approach to collect the required information. The information will be available, provided that the right 'connection' between the researcher and the respondents is made. This connection is made through the methods of data collection.
Data collection is the process of gathering and collecting information from various sources to analyze and make informed decisions based on the data collected. This can involve various methods, such as surveys, interviews, experiments, and observation. In order for data collection to be effective, it is important to have a clear understanding ...
common methods of experiments and its data collection techniques. 2 Experiments Experiments carried out for the purpose of collecting data to be analysed and studied are carried out in the laboratory, in the field or using computer numerical models. It also can be a combination of two or three of the said techniques [1].
Figure 10.1. Data Collection Techniques. Each of these data collection techniques will be the subject of its own chapter in the second half of this textbook. This chapter serves as an orienting overview and as the bridge between the conceptual/design portion of qualitative research and the actual practice of conducting qualitative research.
Data Collection Methods and Tools for Research; A Step-by-Step Guide to Choose Data Collection Technique for Academic and Business Research Projects ... experiments, surveys, interviews, and ...
Data collection methods; Method When to use How to collect data; Experiment: To test a causal relationship. Manipulate variables and measure their effects on others. Survey: To understand the general characteristics or opinions of a group of people. Distribute a list of questions to a sample online, in person or over-the-phone. Interview/focus ...
Designing experiments for data collection is important when the data required for analysis isn't available. The key goal is to design a way to collect the best subset of data quickly and efficiently. Data plays a central role in data science and machine learning. Most often, we assume that the data to be used for analysis or model building is ...
However, unlike experiments, the researcher is not able to control (1) how subjects are assigned to groups and/or (2) which treatments each group receives. Data Collection Methods: Pros and Cons. Each method of data collection has advantages and disadvantages. Resources. When the population is large, a sample survey has a big resource advantage ...
Data collection is the process of gathering and measuring information on variables of interest, in an. research design in the world but if you cannot collect the required data you will be not be ...
The experimental method of data collection involves conducting controlled experiments to study cause-and-effect relationships between variables. In this method, researchers manipulate one or more independent variables to observe their effects on a dependent variable. The participants or subjects are randomly assigned to different groups or conditions to ensure unbiased results.
The importance of data collection methods cannot be overstated, as they play a key role in the research study's overall success and internal validity. Types of Data Collection Methods. The choice of data collection method depends on the research question being addressed, the type of data needed, and the resources and time available.
Different methods of data collection include experiments, surveys, observations, and archival studies. In an experiment, one component of the environment or situation is manipulated to see if it ...
The purpose of primary data is to gather information directly from the source, without relying on secondary sources or pre-existing data. This data is collected through research methods such as surveys, interviews, experiments, and observations. Primary data is valuable because it is tailored to the specific research question or problem at hand ...
Scientists try to identify cause-and-effect relationships because this kind of knowledge is especially powerful, for example, drug A cures disease B. Various methods exist for detecting cause-and-effect relationships. An experiment is a method that most clearly shows cause-and-effect because it isolates and manipulates a single variable, in ...
This document discusses various data collection methods used in business research including censuses, surveys, observation, questionnaires, interviews, and case studies. It then focuses on experimental methods, defining independent, dependent, and controlled variables. An experiment aims to test one variable at a time in a fair, unbiased manner ...
Considering the widespread omission of both the time of sample collection and confirmation of simultaneous collection of experimental and control samples in most studies (Extended Data Fig. 1), it ...
ABSTRACT. Despite the observed decrease in liver fat associated with metabolic-associated fatty liver disease (MAFLD) in mice following fecal microbiota transplantation, the clinical effects and underlying mechanisms of washed microbiota transplantation (WMT), a refined method of fecal microbiota transplantation, for the treatment of MAFLD remain unclear.