- COVID-19 Tracker
- Biochemistry
- Anatomy & Physiology
- Microbiology
- Neuroscience
- Animal Kingdom
- NGSS High School
- Latest News
- Editors’ Picks
- Weekly Digest
- Quotes about Biology
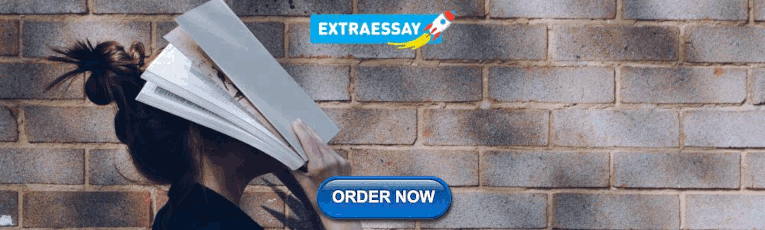
Controlled Experiment
Reviewed by: BD Editors
Controlled Experiment Definition
A controlled experiment is a scientific test that is directly manipulated by a scientist, in order to test a single variable at a time. The variable being tested is the independent variable , and is adjusted to see the effects on the system being studied. The controlled variables are held constant to minimize or stabilize their effects on the subject. In biology, a controlled experiment often includes restricting the environment of the organism being studied. This is necessary to minimize the random effects of the environment and the many variables that exist in the wild.
In a controlled experiment, the study population is often divided into two groups. One group receives a change in a certain variable, while the other group receives a standard environment and conditions. This group is referred to as the control group , and allows for comparison with the other group, known as the experimental group . Many types of controls exist in various experiments, which are designed to ensure that the experiment worked, and to have a basis for comparison. In science, results are only accepted if it can be shown that they are statistically significant . Statisticians can use the difference between the control group and experimental group and the expected difference to determine if the experiment supports the hypothesis , or if the data was simply created by chance.
Examples of Controlled Experiment
Music preference in dogs.
Do dogs have a taste in music? You might have considered this, and science has too. Believe it or not, researchers have actually tested dog’s reactions to various music genres. To set up a controlled experiment like this, scientists had to consider the many variables that affect each dog during testing. The environment the dog is in when listening to music, the volume of the music, the presence of humans, and even the temperature were all variables that the researches had to consider.
In this case, the genre of the music was the independent variable. In other words, to see if dog’s change their behavior in response to different kinds of music, a controlled experiment had to limit the interaction of the other variables on the dogs. Usually, an experiment like this is carried out in the same location, with the same lighting, furniture, and conditions every time. This ensures that the dogs are not changing their behavior in response to the room. To make sure the dogs don’t react to humans or simply the noise of the music, no one else can be in the room and the music must be played at the same volume for each genre. Scientist will develop protocols for their experiment, which will ensure that many other variables are controlled.
This experiment could also split the dogs into two groups, only testing music on one group. The control group would be used to set a baseline behavior, and see how dogs behaved without music. The other group could then be observed and the differences in the group’s behavior could be analyzed. By rating behaviors on a quantitative scale, statistics can be used to analyze the difference in behavior, and see if it was large enough to be considered significant. This basic experiment was carried out on a large number of dogs, analyzing their behavior with a variety of different music genres. It was found that dogs do show more relaxed and calm behaviors when a specific type of music plays. Come to find out, dogs enjoy reggae the most.
Scurvy in Sailors
In the early 1700s, the world was a rapidly expanding place. Ships were being built and sent all over the world, carrying thousands and thousands of sailors. These sailors were mostly fed the cheapest diets possible, not only because it decreased the costs of goods, but also because fresh food is very hard to keep at sea. Today, we understand that lack of essential vitamins and nutrients can lead to severe deficiencies that manifest as disease. One of these diseases is scurvy.
Scurvy is caused by a simple vitamin C deficiency, but the effects can be brutal. Although early symptoms just include general feeling of weakness, the continued lack of vitamin C will lead to a breakdown of the blood cells and vessels that carry the blood. This results in blood leaking from the vessels. Eventually, people bleed to death internally and die. Before controlled experiments were commonplace, a simple physician decided to tackle the problem of scurvy. James Lind, of the Royal Navy, came up with a simple controlled experiment to find the best cure for scurvy.
He separated sailors with scurvy into various groups. He subjected them to the same controlled condition and gave them the same diet, except one item. Each group was subjected to a different treatment or remedy, taken with their food. Some of these remedies included barley water, cider and a regiment of oranges and lemons. This created the first clinical trial , or test of the effectiveness of certain treatments in a controlled experiment. Lind found that the oranges and lemons helped the sailors recover fast, and within a few years the Royal Navy had developed protocols for growing small leafy greens that contained high amounts of vitamin C to feed their sailors.
Related Biology Terms
- Field Experiment – An experiment conducted in nature, outside the bounds of total control.
- Independent Variable – The thing in an experiment being changed or manipulated by the experimenter to see effects on the subject.
- Controlled Variable – A thing that is normalized or standardized across an experiment, to remove it from having an effect on the subject being studied.
- Control Group – A group of subjects in an experiment that receive no independent variable, or a normalized amount, to provide comparison.
1. Why is it necessary for scientist to conduct controlled experiments? A. They allow for more definite relationships of cause and effect to be established B. Without control, anything could happen in the experiment C. Safety first! Controls are just silly safety precautions
2. A population of birds is being studied. Which of the following describes a controlled experiment on the birds? A. The birds are subjected to a lighting strike, and the results observed. B. The birds are split in two groups, one left in the wild and one kept in captivity. After time, the effects are measured and compared. C. The birds are observed as they migrate from North to South America.
3. A scientist is interested in the effects of a pesticide on the eggs of certain birds. The scientist takes some eggs and coats them with pesticide, and does not coat a second group of eggs. He places them in an incubator for several days, then measures several chemical and physical properties of the eggs. Is this a good controlled experiment? A. Yes B. No C. Maybe, but there may be more to the picture
Cite This Article
Subscribe to our newsletter, privacy policy, terms of service, scholarship, latest posts, white blood cell, t cell immunity, satellite cells, embryonic stem cells, popular topics, natural selection, adenosine triphosphate (atp), pituitary gland, mitochondria, water cycle.
Have a language expert improve your writing
Run a free plagiarism check in 10 minutes, automatically generate references for free.
- Knowledge Base
- Methodology
- Controlled Experiments | Methods & Examples of Control
Controlled Experiments | Methods & Examples of Control
Published on 19 April 2022 by Pritha Bhandari . Revised on 10 October 2022.
In experiments , researchers manipulate independent variables to test their effects on dependent variables. In a controlled experiment , all variables other than the independent variable are controlled or held constant so they don’t influence the dependent variable.
Controlling variables can involve:
- Holding variables at a constant or restricted level (e.g., keeping room temperature fixed)
- Measuring variables to statistically control for them in your analyses
- Balancing variables across your experiment through randomisation (e.g., using a random order of tasks)
Table of contents
Why does control matter in experiments, methods of control, problems with controlled experiments, frequently asked questions about controlled experiments.
Control in experiments is critical for internal validity , which allows you to establish a cause-and-effect relationship between variables.
- Your independent variable is the colour used in advertising.
- Your dependent variable is the price that participants are willing to pay for a standard fast food meal.
Extraneous variables are factors that you’re not interested in studying, but that can still influence the dependent variable. For strong internal validity, you need to remove their effects from your experiment.
- Design and description of the meal
- Study environment (e.g., temperature or lighting)
- Participant’s frequency of buying fast food
- Participant’s familiarity with the specific fast food brand
- Participant’s socioeconomic status
Prevent plagiarism, run a free check.
You can control some variables by standardising your data collection procedures. All participants should be tested in the same environment with identical materials. Only the independent variable (e.g., advert colour) should be systematically changed between groups.
Other extraneous variables can be controlled through your sampling procedures . Ideally, you’ll select a sample that’s representative of your target population by using relevant inclusion and exclusion criteria (e.g., including participants from a specific income bracket, and not including participants with colour blindness).
By measuring extraneous participant variables (e.g., age or gender) that may affect your experimental results, you can also include them in later analyses.
After gathering your participants, you’ll need to place them into groups to test different independent variable treatments. The types of groups and method of assigning participants to groups will help you implement control in your experiment.
Control groups
Controlled experiments require control groups . Control groups allow you to test a comparable treatment, no treatment, or a fake treatment, and compare the outcome with your experimental treatment.
You can assess whether it’s your treatment specifically that caused the outcomes, or whether time or any other treatment might have resulted in the same effects.
- A control group that’s presented with red advertisements for a fast food meal
- An experimental group that’s presented with green advertisements for the same fast food meal
Random assignment
To avoid systematic differences between the participants in your control and treatment groups, you should use random assignment .
This helps ensure that any extraneous participant variables are evenly distributed, allowing for a valid comparison between groups .
Random assignment is a hallmark of a ‘true experiment’ – it differentiates true experiments from quasi-experiments .
Masking (blinding)
Masking in experiments means hiding condition assignment from participants or researchers – or, in a double-blind study , from both. It’s often used in clinical studies that test new treatments or drugs.
Sometimes, researchers may unintentionally encourage participants to behave in ways that support their hypotheses. In other cases, cues in the study environment may signal the goal of the experiment to participants and influence their responses.
Using masking means that participants don’t know whether they’re in the control group or the experimental group. This helps you control biases from participants or researchers that could influence your study results.
Although controlled experiments are the strongest way to test causal relationships, they also involve some challenges.
Difficult to control all variables
Especially in research with human participants, it’s impossible to hold all extraneous variables constant, because every individual has different experiences that may influence their perception, attitudes, or behaviors.
But measuring or restricting extraneous variables allows you to limit their influence or statistically control for them in your study.
Risk of low external validity
Controlled experiments have disadvantages when it comes to external validity – the extent to which your results can be generalised to broad populations and settings.
The more controlled your experiment is, the less it resembles real world contexts. That makes it harder to apply your findings outside of a controlled setting.
There’s always a tradeoff between internal and external validity . It’s important to consider your research aims when deciding whether to prioritise control or generalisability in your experiment.
Experimental designs are a set of procedures that you plan in order to examine the relationship between variables that interest you.
To design a successful experiment, first identify:
- A testable hypothesis
- One or more independent variables that you will manipulate
- One or more dependent variables that you will measure
When designing the experiment, first decide:
- How your variable(s) will be manipulated
- How you will control for any potential confounding or lurking variables
- How many subjects you will include
- How you will assign treatments to your subjects
Cite this Scribbr article
If you want to cite this source, you can copy and paste the citation or click the ‘Cite this Scribbr article’ button to automatically add the citation to our free Reference Generator.
Bhandari, P. (2022, October 10). Controlled Experiments | Methods & Examples of Control. Scribbr. Retrieved 18 June 2024, from https://www.scribbr.co.uk/research-methods/controlled-experiments/
Is this article helpful?
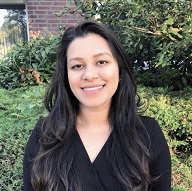
Pritha Bhandari

In order to continue enjoying our site, we ask that you confirm your identity as a human. Thank you very much for your cooperation.
Controlled Experiment
A Controlled Experiment is a type of research where one element is adjusted to identify its impact on the outcome.

A controlled experiment is a comprehensive methodology employed in scientific research to reduce the effect of variables other than the independent variable. It involves segregating the subjects into two or more groups, including the control group that does not undergo any modifications in the independent variables, and the test group(s) that do. After the treatment, researchers compare the results across these groups to deduce how adjustments in variables influence outcomes.
This procedure promotes a high level of control, thereby increasing the validity and reliability of results. It serves as an objective mechanism to dissect cause-and-effect relationships, which drives data-driven insights.
To illustrate, a digital marketing company intends to identify the best timings for sending emails to their subscribers. They could conduct a controlled experiment, sending emails at different times to the control and test groups and then comparing their open rates. If the open rates significantly differ, this provides evidence that the timing impacts email interaction.
Why is Controlled Experiment important?
Controlled experiments play a key role by presenting empirical evidence in determining the effectiveness of a marketing strategy. They allow marketers to optimize for specific metrics by isolating variables one at a time to understand how each influences the outcome. This can hugely aid decision-making processes, ensuring marketing plans are built and tweaked relying on data rather than intuition.
Which factors impact Controlled Experiment ?
Recruiting larger and diverse samples can enhance the generalizability of results. Moreover, ensuring the experiment is as double-blinded as possible reduces biases. Employing rigorous statistical techniques, repetitions and incorporating potential confounders in the experimental design can improve reliability, validity, and the overall quality of a controlled experiment.
How can Controlled Experiment be improved?
Several factors can impact the results of controlled experiments. This includes statistical noise, uncontrolled variables, measurement errors and selection bias. It’s of utmost importance that these factors are carefully considered during the experiment design process to ensure accurate and applicable results.
What is Controlled Experiment 's relationship with other metrics?
Controlled experimentation sits at the center of highlighting correlations between different ecommerce metrics. For example, you can understand the relationship between webpage load speed (independent variable) and user engagement metrics (dependent variable) by adjusting the speed for the test group. The results can potentially highlight correlations between these ecommerce metrics, helping to optimize your digital platform for maximum engagement.

Explore more Glossary terms
Cost of goods sold.
Cost of Goods Sold (COGS) is an accounting metric used to calculate the cost of any goods sold in an ecommerce business.
Churn rate is an important metric that helps measure the attrition or customer loss rate within the ecommerce industry o...
Customer Engagement Score
Customer Engagement Score (CES) quantifies customer interaction with a service or product, aiding e-commerce in churning...
Click-through rate (CTR)
Click-through rate (CTR) is a metric used in online marketing to measure how many times a user clicks on a specific link...
Cross-Device Tracking
Cross-Device Tracking is the process of collecting user-level data as users interact with an application across multiple...
Cost per Follower
Cost per Follower is a metric that measures the effectiveness of social media marketing, allowing businesses to track th...
What Is A Controlled Experiment? Aren’t All Experiments Controlled?
Why should you experiment, how should you experiment, key parameters of a controlled experiment, is there such a thing as an uncontrolled experiment.
A procedure that helps you understand the influence of various factors that affect a result and the extent of their effect in a controlled environment.
Have you ever done science experiments that have numerous parameters you need to take care of to get an accurate result?
If so, I know exactly how that feels!
Most of the time, you won’t get a perfect value, but rather a value that is nearly correct. It can be so frustrating at times, as you need to take care of the amount of catalyst, the temperature, pressure and a million other things!
I wonder who found out that you need precisely ‘this’ thing in exactly ‘this’ amount to get ‘that’ thing! Well, over time, I’ve realized just how much important these parameters are. These values help us set up a controlled environment where the experiment can occur.
And while many people loathe doing lengthy experiments, scientists have performed these exact same experiments a million times to find the perfect mix of parameters that give a predictable result! Now that’s perseverance!!

Recommended Video for you:
There was a time when scientists speculated about plants being alive in the same way as humans. Jagdish Chandra Bose was the scientist who was able to prove that plants are indeed living things by noting their response to different stimuli. He used an experiment wherein the roots of a plant’s stem were dipped in a solution of Bromine Chloride, a poison . He observed the pulse of the plant as a white spot on the crescograph, a device that could magnify the motion of plant tissues up to 10,000 times.
This experiment may have been groundbreaking at that time, but his result was derived because of the three steps that every scientist follows to arrive at a conclusion.
- Scientists observe a certain phenomenon that interests them or sparks their curiosity.
- They form a hypothesis, i.e., they try to establish a ‘cause-effect’ relationship for the phenomenon. There are multiple hypotheses for a single occurrence that may or may not be correct.
Example: the atomic model was proposed by many scientists before the most recent Quantum model was accepted. Simply put, a hypothesis is the possible cause of the effect that one wishes to study.
- Now, the hypothesis is often based on mathematical calculations or general observations, but until they are disproved, the theory is not accepted.
- This is where experiments come into the picture. Various experiments are done that can support the hypothesis. If a particular theory is supported by experimental backing, the hypothesis becomes a “scientific theory/discovery”.
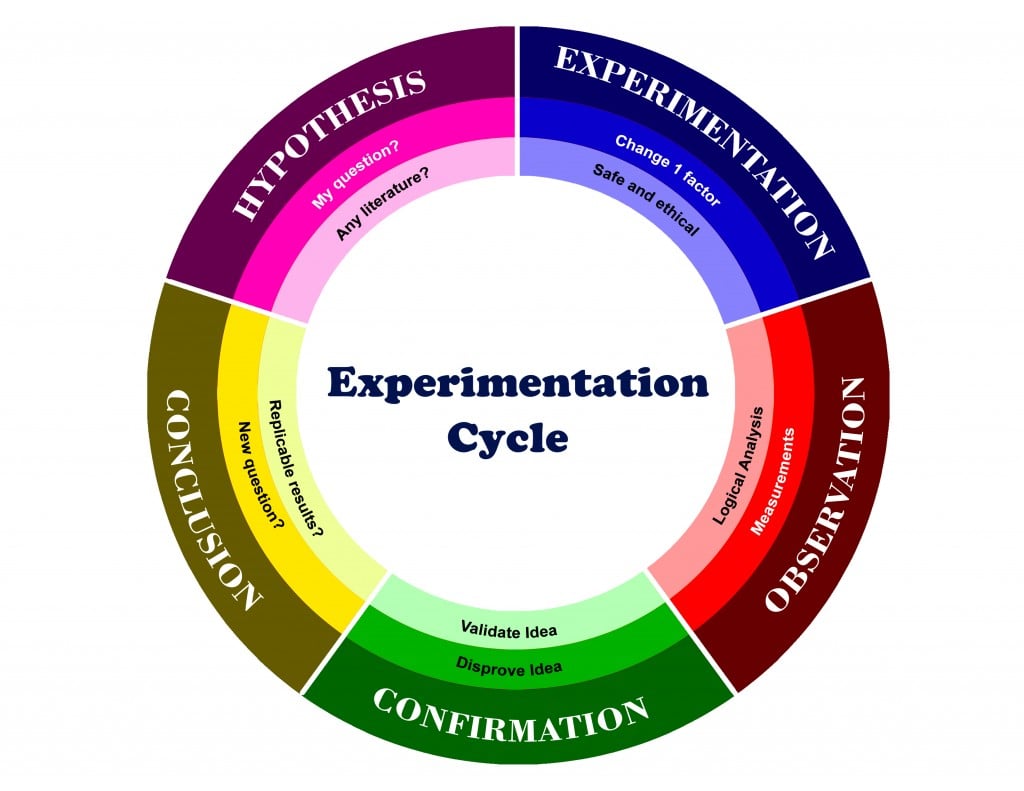
Also Read: What Is Endogeneity? What Is An Exogenous Variable?
To reach effective results, you need to test your hypothesis by performing an experiment, but it’s not as if any random experiment can give you results. A controlled experiment allows you to isolate and study the clear result that will eventually allow you to draw conclusions.
A single phenomenon is the result of multiple factors, but how do you know the independent effect of each factor? A controlled experiment basically limits the scope of the result because only one or two factors affecting the result are allowed to vary. All the other factors are kept constant.
Also Read: What Is An Independent Variable?
Now, when you perform an experiment, you’re basically looking for two things
- The factors that affect the final result.
- The extent to which each factor contributes to the result.
We can identify the elements that affect the result by keeping all the other elements constant. These variables/factors that are constant are known as control variables/constant variables .
If we want to test the effect of a certain (factor) fertilizer on plants, we take two plants, both identical in all respects, such that all the other factors affecting its growth remain constant. Now, to one plant we add the fertilizer, and to the other, we add no fertilizer. Thus, after the allotted time period, if the fertilizer was actually useful, you will see that the growth in one plant is greater than the other. Here, the plant that got the fertilizer is the experimental group and the one without the fertilizer is the control group .
If you’re wondering what the use of the control group is, it basically provides you with a minimal value to start with. It allows you to compare the effect of the fertilizer with respect to the normal growth factor and the extent to which the fertilizer enhanced the growth of the plant. A controlled experiment tries to form a link between the cause and the effect. If we are to study the effect of fertilizers on plant growth, the cause will be the ‘fertilizer’ and its effect would be the ‘growth of the plant’. In other words:
- The fertilizer would be the independent variable — a variable that is changed and modified to study its effect.
- The growth of the plant will be the dependent variable— a variable that is being tested and whose value depends on the independent variable.

Well, after reading all of this, it’s pretty obvious that controlled experiments are often set up that way and don’t occur naturally. They also give results that are reliable and spot on!
Clearly, experiments that don’t have any control variables are uncontrolled in every way. In fact, the entire natural phenomenon that gave rise to a scientist’s hypothesis is an uncontrolled experiment. This implies that, without control, you can still get results, but those results are unclear. You can draw conclusions from uncontrolled experiments, but it’s a lot harder to determine the true influence of individual factors when all of them are acting at the same time.
Some experiments, however, are impossible to control! Experiments that require testing on humans are influenced by genetic makeup, metabolism and psychology, among other factors, all of which are beyond human control. Thus, there is often a result that is simply averaged and used because no particular result can reflect the whole effect.
Uncontrolled experiments may not give perfect results, but they often help scientists observe patterns. A task that was performed better by more females than males helps to identify that there is possibly an element of female psychology, a hormone or temperament that influenced the result.
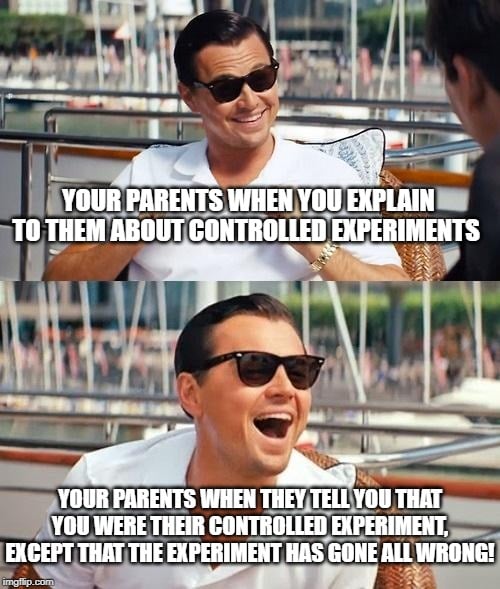
Controlled experimentation is the most widely preferred method used to study and prove a hypothesis. Nature is an intelligent experimenter and designs phenomena that are intricate and detailed, and we humans are still trying to understand those details, so we need to break things into parts before we can understand the whole picture. This is where controlled experimentation helps us. All in all, controlled experimentation aids us in understanding things at a pace we are comfortable with, while giving us time to explore the depths to which we want to study a given occurrence.
- Controlled experiments (article) | Khan Academy. Khan Academy
- How Acharya Jagadish Chandra Bose proved plants have .... India Today
- What are Independent and Dependent Variables?-NCES Kids .... The National Center for Education Statistics

Zankhana has completed her Bachelors in Electronics and Telecommunications Engineering. She is an avid reader of works of mythology and history. She is trained in Hindustani Classical Singing and Kathak. She likes to travel and trusts her artsy heart and scientific mind to take her to places that she has dreamt of.
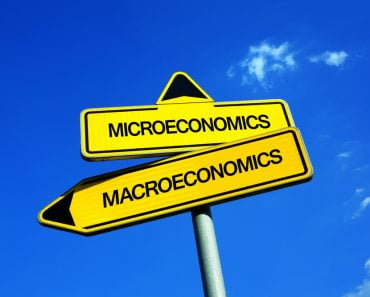
Is Economics A Science?
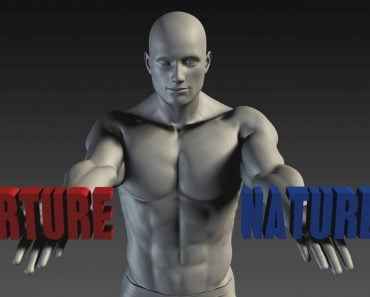
Are We Born With A Fixed Personality Or Can It Be Manipulated By Our Environment?
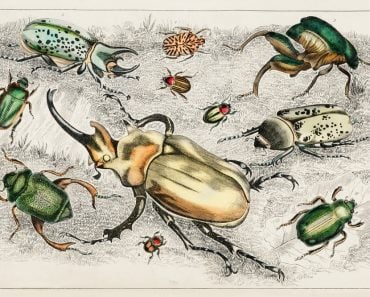
Does (Natural) History Repeat Itself?
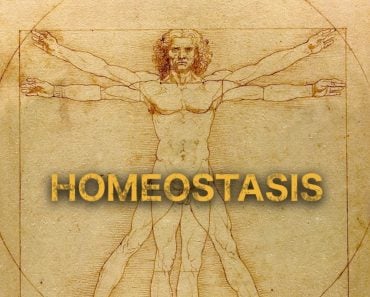
What Is Homeostasis?
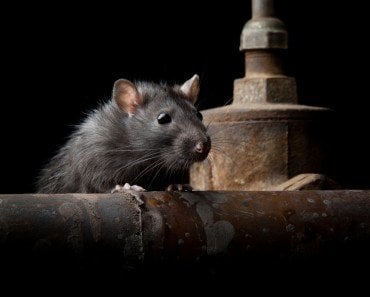
Why Are Rats The Most Preferred Animals For Experiments?
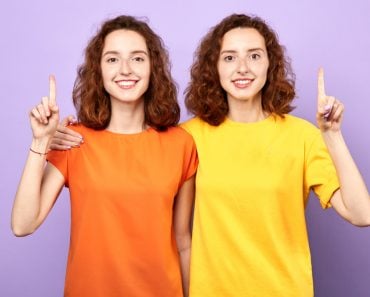
Are We Genetically Predetermined To Like What We Like?
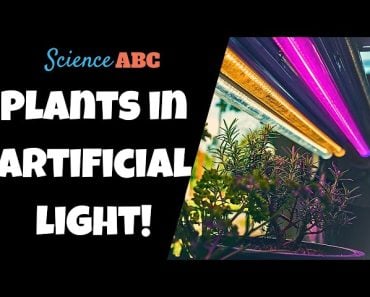
This Is What Happens When You Try To Grow A Plant In Artificial Light
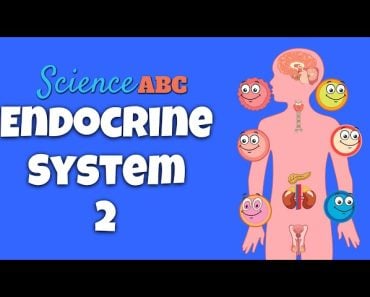
Endocrine System: Glands and Hormones
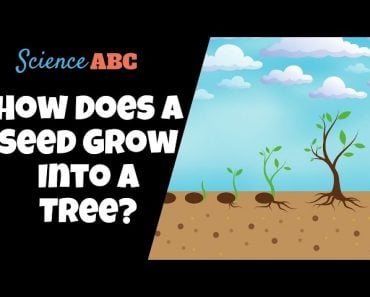
Germination: How Does A Seed Become A Plant?
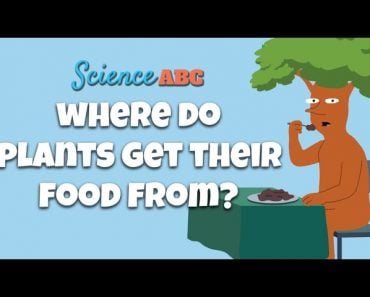
Photosynthesis: The Biochemistry Behind How Plants Make Their Food
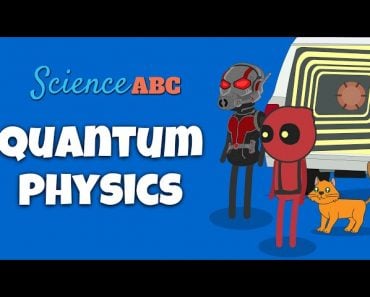
Quantum Physics: Here’s Why Movies Always Get It Wrong
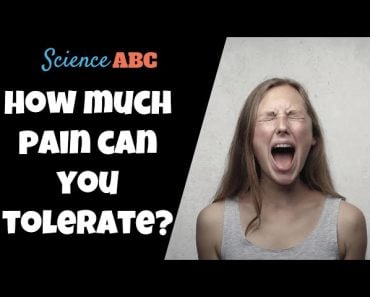
Max Pain: How Much Pain Can A Person Take?
- Controlled Experiments: Methods, Examples & Limitations

What happens in experimental research is that the researcher alters the independent variables so as to determine their impacts on the dependent variables.
Therefore, when the experiment is controlled, you can expect that the researcher will control all other variables except for the independent variables . This is done so that the other variables do not have an influence on the dependent variables.
In this article, we are going to consider controlled experiment, how important it is in a study, and how it can be designed. But before we dig deep, let us look at the definition of a controlled experiment.
What is a Controlled Experiment?
In a scientific experiment, a controlled experiment is a test that is directly altered by the researcher so that only one variable is studied at a time. The single variable being studied will then be the independent variable.
This independent variable is manipulated by the researcher so that its effect on the hypothesis or data being studied is known. While the researcher studies the single independent variable, the controlled variables are made constant to reduce or balance out their impact on the research.
To achieve a controlled experiment, the research population is mostly distributed into two groups. Then the treatment is administered to one of the two groups, while the other group gets the control conditions. This other group is referred to as the control group.
The control group gets the standard conditions and is placed in the standard environment and it also allows for comparison with the other group, which is referred to as the experimental group or the treatment group. Obtaining the difference between these two groups’ behavior is important because in any scientific experiment, being able to show the statistical significance of the results is the only criterion for the results to be accepted.
So to determine whether the experiment supports the hypothesis, or if the data is a result of chance, the researcher will check for the difference between the control group and experimental group. Then the results from the differences will be compared with the expected difference.
For example, a researcher may want to answer this question, do dogs also have a music taste? In case you’re wondering too, yes, there are existing studies by researchers on how dogs react to different music genres.
Back to the example, the researcher may develop a controlled experiment with high consideration on the variables that affect each dog. Some of these variables that may have effects on the dog are; the dog’s environment when listening to music, the temperature of the environment, the music volume, and human presence.
The independent variable to focus on in this research is the genre of the music. To determine if there is an effect on the dog while listening to different kinds of music, the dog’s environment must be controlled. A controlled experiment would limit interaction between the dog and other variables.
In this experiment, the researcher can also divide the dogs into two groups, one group will perform the music test while the other, the control group will be used as the baseline or standard behavior. The control group behavior can be observed along with the treatment group and the differences in the two group’s behavior can be analyzed.
What is an Experimental Control?
Experimental control is the technique used by the researcher in scientific research to minimize the effects of extraneous variables. Experimental control also strengthens the ability of the independent variable to change the dependent variable.
For example, the cause and effect possibilities will be examined in a well-designed and properly controlled experiment if the independent variable (Treatment Y) causes a behavioral change in the dependent variable (Subject X).
In another example, a researcher feeds 20 lab rats with an artificial sweetener and from the researcher’s observation, six of the rats died of dehydration. Now, the actual cause of death may be artificial sweeteners or an unrelated factor. Such as the water supplied to the rats being contaminated or the rats could not drink enough, or suffering a disease.
Read: Nominal, Ordinal, Interval & Ratio Variable + [Examples]
For a researcher, eliminating these potential causes one after the other will consume time, and be tedious. Hence, the researcher can make use of experimental control. This method will allow the researcher to divide the rats into two groups: one group will receive the artificial sweetener while the other one doesn’t. The two groups will be placed in similar conditions and observed in similar ways. The differences that now occur in morbidity between the two groups can be traced to the sweetener with certainty.
From the example above, the experimental control is administered as a form of a control group. The data from the control group is then said to be the standard against which every other experimental outcome is measured.
Purpose & Importance of Control in Experimentation
1. One significant purpose of experimental controls is that it allows researchers to eliminate various confounding variables or uncertainty in their research. A researcher will need to use an experimental control to ensure that only the variables that are intended to change, are changed in research.
2. Controlled experiments also allow researchers to control the specific variables they think might have an effect on the outcomes of the study. The researcher will use a control group if he/she believes some extra variables can form an effect on the results of the study. This is to ensure that the extra variable is held constant and possible influences are measured.
3. Controlled experiments establish a standard that the outcome of a study should be compared to, and allow researchers to correct for potential errors.
Read more: What are Cross-Sectional Studies: Examples, Definition, Types
Methods of Experimental Control
Here are some methods used to achieve control in experimental research
- Use of Control Groups
Control groups are required for controlled experiments. Control groups will allow the researcher to run a test on fake treatment, and comparable treatment. It will also compare the result of the comparison with the researcher’s experimental treatment. The results will allow the researcher to understand if the treatment administered caused the outcome or if other factors such as time, or others are involved and whether they would have yielded the same effects.
For an example of a control group experiment, a researcher conducting an experiment on the effects of colors in advertising, asked all the participants to come individually to a lab. In this lab, environmental conditions are kept the same all through the research.
For the researcher to determine the effect of colors in advertising, each of the participants is placed in either of the two groups: the control group or the experimental group.
In the control group, the advertisement color is yellow to represent the clothing industry while blue is given as the advertisement color to the experimental group to represent the clothing industry also. The only difference in these two groups will be the color of the advertisement, other variables will be similar.
- Use of Masking (blinding)
Masking occurs in an experiment when the researcher hides condition assignments from the participants. If it’s double-blind research, both the researcher and the participants will be in the dark. Masking or blinding is mostly used in clinical studies to test new treatments.
Masking as a control measure takes place because sometimes, researchers may unintentionally influence the participants to act in ways that support their hypotheses. In another scenario, the goal of the study might be revealed to the participants through the study environment and this may influence their responses.
Masking, however, blinds the participants from having a deeper knowledge of the research whether they’re in the control group or the experimental group. This helps to control and reduce biases from either the researcher or the participants that could influence the results of the study.
- Use of Random Assignment
Random assignment or distribution is used to avoid systematic differences between participants in the experimental group and the control group. This helps to evenly distribute extraneous participant variables, thereby making the comparison between groups valid. Another usefulness of random assignment is that it shows the difference between true experiments from quasi-experiments.
Learn About: Double-Blind Studies in Research: Types, Pros & Cons
How to Design a Controlled Experiment
For a researcher to design a controlled experiment, the researcher will need:
- A hypothesis that can be tested.
- One or more independent variables can be changed or manipulated precisely.
- One or more dependent variables can be accurately measured.
Then, when the researcher is designing the experiment, he or she must decide on:
- How will the variables be manipulated?
- How will control be set up in case of any potential confounding variables?
- How large will the samples or participants included in the study be?
- How will the participants be distributed into treatment levels?
How you design your experimental control is highly significant to your experiment’s external and internal validity.
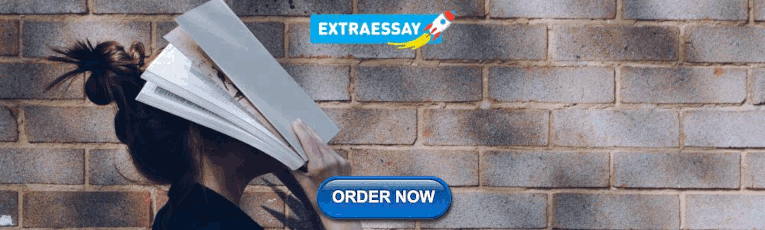
Controlled Experiment Examples
1. A good example of a controlled group would be an experiment to test the effects of a drug. The sample population would be divided into two, the group receiving the drug would be the experimental group while the group receiving the placebo would be the control group (Note that all the variables such as age, and sex, will be the same).
The only significant difference between the two groups will be the taking of medication. You can determine if the drug is effective or not if the control group and experimental group show similar results.
2. Let’s take a look at this example too. If a researcher wants to determine the impact of different soil types on the germination period of seeds, the researcher can proceed to set up four different pots. Each of the pots would be filled with a different type of soil and then seeds can be planted on the soil. After which each soil pot will be watered and exposed to sunlight.
The researcher will start to measure how long it took for the seeds to sprout in each of the different soil types. Control measures for this experiment might be to place some seeds in a pot without filling the pot with soil. The reason behind this control measure is to determine that no other factor is responsible for germination except the soil.
Here, the researcher can also control the amount of sun the seeds are exposed to, or how much water they are given. The aim is to eliminate all other variables that can affect how quickly the seeds sprouted.
Experimental controls are important, but it is also important to note that not all experiments should be controlled and It is still possible to get useful data from experiments that are not controlled.
Explore: 21 Chrome Extensions for Academic Researchers in 2021
Problems with Controlled Experiments
It is true that the best way to test for cause and effect relationships is by conducting controlled experiments. However, controlled experiments also have some challenges. Some of which are:
- Difficulties in controlling all the variables especially when the participants in your research are human participants. It can be impossible to hold all the extra variables constant because all individuals have different experiences that may influence their behaviors.
- Controlled experiments are at risk of low external validity because there’s a limit to how the results from the research can be extrapolated to a very large population .
- Your research may lack relatability to real world experience if they are too controlled and that will make it hard for you to apply your outcomes outside a controlled setting.
Control Group vs an Experimental Group
There is a thin line between the control group and the experimental group. That line is the treatment condition. As we have earlier established, the experimental group is the one that gets the treatment while the control group is the placebo group.
All controlled experiments require control groups because control groups will allow you to compare treatments, and to test if there is no treatment while you compare the result with your experimental treatment.
Therefore, both the experimental group and the control group are required to conduct a controlled experiment
FAQs about Controlled Experiments
- Is the control condition the same as the control group?
The control group is different from the control condition. However, the control condition is administered to the control group.
- What are positive and negative control in an experiment?
The negative control is the group where no change or response is expected while the positive control is the group that receives the treatment with a certainty of a positive result.
While the controlled experiment is beneficial to eliminate extraneous variables in research and focus on the independent variable only to cause an effect on the dependent variable.
Researchers should be careful so they don’t lose real-life relatability to too controlled experiments and also, not all experiments should be controlled.
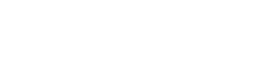
Connect to Formplus, Get Started Now - It's Free!
- cofounding variables
- control groups
- controlled experiment
- controlled experiment examples
- controlled experiments
- experimental control
- extraneous variables
- busayo.longe
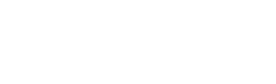
You may also like:
Descriptive Research Designs: Types, Examples & Methods
Ultimate guide to Descriptive research. Definitions, designs, types, examples and methodology.

How to do a Meta Analysis: Methodology, Pros & Cons
In this article, we’ll go through the concept of meta-analysis, what it can be used for, and how you can use it to improve how you...
Extraneous Variables Explained: Types & Examples
In this article, we are going to discuss extraneous variables and how they impact research.
Lurking Variables Explained: Types & Examples
In this article, we’ll discuss what a lurking variable means, the several types available, its effects along with some real-life examples
Formplus - For Seamless Data Collection
Collect data the right way with a versatile data collection tool. try formplus and transform your work productivity today..
Frequently asked questions
What are the requirements for a controlled experiment.
In a controlled experiment , all extraneous variables are held constant so that they can’t influence the results. Controlled experiments require:
- A control group that receives a standard treatment, a fake treatment, or no treatment.
- Random assignment of participants to ensure the groups are equivalent.
Depending on your study topic, there are various other methods of controlling variables .
Frequently asked questions: Methodology
Attrition refers to participants leaving a study. It always happens to some extent—for example, in randomized controlled trials for medical research.
Differential attrition occurs when attrition or dropout rates differ systematically between the intervention and the control group . As a result, the characteristics of the participants who drop out differ from the characteristics of those who stay in the study. Because of this, study results may be biased .
Action research is conducted in order to solve a particular issue immediately, while case studies are often conducted over a longer period of time and focus more on observing and analyzing a particular ongoing phenomenon.
Action research is focused on solving a problem or informing individual and community-based knowledge in a way that impacts teaching, learning, and other related processes. It is less focused on contributing theoretical input, instead producing actionable input.
Action research is particularly popular with educators as a form of systematic inquiry because it prioritizes reflection and bridges the gap between theory and practice. Educators are able to simultaneously investigate an issue as they solve it, and the method is very iterative and flexible.
A cycle of inquiry is another name for action research . It is usually visualized in a spiral shape following a series of steps, such as “planning → acting → observing → reflecting.”
To make quantitative observations , you need to use instruments that are capable of measuring the quantity you want to observe. For example, you might use a ruler to measure the length of an object or a thermometer to measure its temperature.
Criterion validity and construct validity are both types of measurement validity . In other words, they both show you how accurately a method measures something.
While construct validity is the degree to which a test or other measurement method measures what it claims to measure, criterion validity is the degree to which a test can predictively (in the future) or concurrently (in the present) measure something.
Construct validity is often considered the overarching type of measurement validity . You need to have face validity , content validity , and criterion validity in order to achieve construct validity.
Convergent validity and discriminant validity are both subtypes of construct validity . Together, they help you evaluate whether a test measures the concept it was designed to measure.
- Convergent validity indicates whether a test that is designed to measure a particular construct correlates with other tests that assess the same or similar construct.
- Discriminant validity indicates whether two tests that should not be highly related to each other are indeed not related. This type of validity is also called divergent validity .
You need to assess both in order to demonstrate construct validity. Neither one alone is sufficient for establishing construct validity.
- Discriminant validity indicates whether two tests that should not be highly related to each other are indeed not related
Content validity shows you how accurately a test or other measurement method taps into the various aspects of the specific construct you are researching.
In other words, it helps you answer the question: “does the test measure all aspects of the construct I want to measure?” If it does, then the test has high content validity.
The higher the content validity, the more accurate the measurement of the construct.
If the test fails to include parts of the construct, or irrelevant parts are included, the validity of the instrument is threatened, which brings your results into question.
Face validity and content validity are similar in that they both evaluate how suitable the content of a test is. The difference is that face validity is subjective, and assesses content at surface level.
When a test has strong face validity, anyone would agree that the test’s questions appear to measure what they are intended to measure.
For example, looking at a 4th grade math test consisting of problems in which students have to add and multiply, most people would agree that it has strong face validity (i.e., it looks like a math test).
On the other hand, content validity evaluates how well a test represents all the aspects of a topic. Assessing content validity is more systematic and relies on expert evaluation. of each question, analyzing whether each one covers the aspects that the test was designed to cover.
A 4th grade math test would have high content validity if it covered all the skills taught in that grade. Experts(in this case, math teachers), would have to evaluate the content validity by comparing the test to the learning objectives.
Snowball sampling is a non-probability sampling method . Unlike probability sampling (which involves some form of random selection ), the initial individuals selected to be studied are the ones who recruit new participants.
Because not every member of the target population has an equal chance of being recruited into the sample, selection in snowball sampling is non-random.
Snowball sampling is a non-probability sampling method , where there is not an equal chance for every member of the population to be included in the sample .
This means that you cannot use inferential statistics and make generalizations —often the goal of quantitative research . As such, a snowball sample is not representative of the target population and is usually a better fit for qualitative research .
Snowball sampling relies on the use of referrals. Here, the researcher recruits one or more initial participants, who then recruit the next ones.
Participants share similar characteristics and/or know each other. Because of this, not every member of the population has an equal chance of being included in the sample, giving rise to sampling bias .
Snowball sampling is best used in the following cases:
- If there is no sampling frame available (e.g., people with a rare disease)
- If the population of interest is hard to access or locate (e.g., people experiencing homelessness)
- If the research focuses on a sensitive topic (e.g., extramarital affairs)
The reproducibility and replicability of a study can be ensured by writing a transparent, detailed method section and using clear, unambiguous language.
Reproducibility and replicability are related terms.
- Reproducing research entails reanalyzing the existing data in the same manner.
- Replicating (or repeating ) the research entails reconducting the entire analysis, including the collection of new data .
- A successful reproduction shows that the data analyses were conducted in a fair and honest manner.
- A successful replication shows that the reliability of the results is high.
Stratified sampling and quota sampling both involve dividing the population into subgroups and selecting units from each subgroup. The purpose in both cases is to select a representative sample and/or to allow comparisons between subgroups.
The main difference is that in stratified sampling, you draw a random sample from each subgroup ( probability sampling ). In quota sampling you select a predetermined number or proportion of units, in a non-random manner ( non-probability sampling ).
Purposive and convenience sampling are both sampling methods that are typically used in qualitative data collection.
A convenience sample is drawn from a source that is conveniently accessible to the researcher. Convenience sampling does not distinguish characteristics among the participants. On the other hand, purposive sampling focuses on selecting participants possessing characteristics associated with the research study.
The findings of studies based on either convenience or purposive sampling can only be generalized to the (sub)population from which the sample is drawn, and not to the entire population.
Random sampling or probability sampling is based on random selection. This means that each unit has an equal chance (i.e., equal probability) of being included in the sample.
On the other hand, convenience sampling involves stopping people at random, which means that not everyone has an equal chance of being selected depending on the place, time, or day you are collecting your data.
Convenience sampling and quota sampling are both non-probability sampling methods. They both use non-random criteria like availability, geographical proximity, or expert knowledge to recruit study participants.
However, in convenience sampling, you continue to sample units or cases until you reach the required sample size.
In quota sampling, you first need to divide your population of interest into subgroups (strata) and estimate their proportions (quota) in the population. Then you can start your data collection, using convenience sampling to recruit participants, until the proportions in each subgroup coincide with the estimated proportions in the population.
A sampling frame is a list of every member in the entire population . It is important that the sampling frame is as complete as possible, so that your sample accurately reflects your population.
Stratified and cluster sampling may look similar, but bear in mind that groups created in cluster sampling are heterogeneous , so the individual characteristics in the cluster vary. In contrast, groups created in stratified sampling are homogeneous , as units share characteristics.
Relatedly, in cluster sampling you randomly select entire groups and include all units of each group in your sample. However, in stratified sampling, you select some units of all groups and include them in your sample. In this way, both methods can ensure that your sample is representative of the target population .
A systematic review is secondary research because it uses existing research. You don’t collect new data yourself.
The key difference between observational studies and experimental designs is that a well-done observational study does not influence the responses of participants, while experiments do have some sort of treatment condition applied to at least some participants by random assignment .
An observational study is a great choice for you if your research question is based purely on observations. If there are ethical, logistical, or practical concerns that prevent you from conducting a traditional experiment , an observational study may be a good choice. In an observational study, there is no interference or manipulation of the research subjects, as well as no control or treatment groups .
It’s often best to ask a variety of people to review your measurements. You can ask experts, such as other researchers, or laypeople, such as potential participants, to judge the face validity of tests.
While experts have a deep understanding of research methods , the people you’re studying can provide you with valuable insights you may have missed otherwise.
Face validity is important because it’s a simple first step to measuring the overall validity of a test or technique. It’s a relatively intuitive, quick, and easy way to start checking whether a new measure seems useful at first glance.
Good face validity means that anyone who reviews your measure says that it seems to be measuring what it’s supposed to. With poor face validity, someone reviewing your measure may be left confused about what you’re measuring and why you’re using this method.
Face validity is about whether a test appears to measure what it’s supposed to measure. This type of validity is concerned with whether a measure seems relevant and appropriate for what it’s assessing only on the surface.
Statistical analyses are often applied to test validity with data from your measures. You test convergent validity and discriminant validity with correlations to see if results from your test are positively or negatively related to those of other established tests.
You can also use regression analyses to assess whether your measure is actually predictive of outcomes that you expect it to predict theoretically. A regression analysis that supports your expectations strengthens your claim of construct validity .
When designing or evaluating a measure, construct validity helps you ensure you’re actually measuring the construct you’re interested in. If you don’t have construct validity, you may inadvertently measure unrelated or distinct constructs and lose precision in your research.
Construct validity is often considered the overarching type of measurement validity , because it covers all of the other types. You need to have face validity , content validity , and criterion validity to achieve construct validity.
Construct validity is about how well a test measures the concept it was designed to evaluate. It’s one of four types of measurement validity , which includes construct validity, face validity , and criterion validity.
There are two subtypes of construct validity.
- Convergent validity : The extent to which your measure corresponds to measures of related constructs
- Discriminant validity : The extent to which your measure is unrelated or negatively related to measures of distinct constructs
Naturalistic observation is a valuable tool because of its flexibility, external validity , and suitability for topics that can’t be studied in a lab setting.
The downsides of naturalistic observation include its lack of scientific control , ethical considerations , and potential for bias from observers and subjects.
Naturalistic observation is a qualitative research method where you record the behaviors of your research subjects in real world settings. You avoid interfering or influencing anything in a naturalistic observation.
You can think of naturalistic observation as “people watching” with a purpose.
A dependent variable is what changes as a result of the independent variable manipulation in experiments . It’s what you’re interested in measuring, and it “depends” on your independent variable.
In statistics, dependent variables are also called:
- Response variables (they respond to a change in another variable)
- Outcome variables (they represent the outcome you want to measure)
- Left-hand-side variables (they appear on the left-hand side of a regression equation)
An independent variable is the variable you manipulate, control, or vary in an experimental study to explore its effects. It’s called “independent” because it’s not influenced by any other variables in the study.
Independent variables are also called:
- Explanatory variables (they explain an event or outcome)
- Predictor variables (they can be used to predict the value of a dependent variable)
- Right-hand-side variables (they appear on the right-hand side of a regression equation).
As a rule of thumb, questions related to thoughts, beliefs, and feelings work well in focus groups. Take your time formulating strong questions, paying special attention to phrasing. Be careful to avoid leading questions , which can bias your responses.
Overall, your focus group questions should be:
- Open-ended and flexible
- Impossible to answer with “yes” or “no” (questions that start with “why” or “how” are often best)
- Unambiguous, getting straight to the point while still stimulating discussion
- Unbiased and neutral
A structured interview is a data collection method that relies on asking questions in a set order to collect data on a topic. They are often quantitative in nature. Structured interviews are best used when:
- You already have a very clear understanding of your topic. Perhaps significant research has already been conducted, or you have done some prior research yourself, but you already possess a baseline for designing strong structured questions.
- You are constrained in terms of time or resources and need to analyze your data quickly and efficiently.
- Your research question depends on strong parity between participants, with environmental conditions held constant.
More flexible interview options include semi-structured interviews , unstructured interviews , and focus groups .
Social desirability bias is the tendency for interview participants to give responses that will be viewed favorably by the interviewer or other participants. It occurs in all types of interviews and surveys , but is most common in semi-structured interviews , unstructured interviews , and focus groups .
Social desirability bias can be mitigated by ensuring participants feel at ease and comfortable sharing their views. Make sure to pay attention to your own body language and any physical or verbal cues, such as nodding or widening your eyes.
This type of bias can also occur in observations if the participants know they’re being observed. They might alter their behavior accordingly.
The interviewer effect is a type of bias that emerges when a characteristic of an interviewer (race, age, gender identity, etc.) influences the responses given by the interviewee.
There is a risk of an interviewer effect in all types of interviews , but it can be mitigated by writing really high-quality interview questions.
A semi-structured interview is a blend of structured and unstructured types of interviews. Semi-structured interviews are best used when:
- You have prior interview experience. Spontaneous questions are deceptively challenging, and it’s easy to accidentally ask a leading question or make a participant uncomfortable.
- Your research question is exploratory in nature. Participant answers can guide future research questions and help you develop a more robust knowledge base for future research.
An unstructured interview is the most flexible type of interview, but it is not always the best fit for your research topic.
Unstructured interviews are best used when:
- You are an experienced interviewer and have a very strong background in your research topic, since it is challenging to ask spontaneous, colloquial questions.
- Your research question is exploratory in nature. While you may have developed hypotheses, you are open to discovering new or shifting viewpoints through the interview process.
- You are seeking descriptive data, and are ready to ask questions that will deepen and contextualize your initial thoughts and hypotheses.
- Your research depends on forming connections with your participants and making them feel comfortable revealing deeper emotions, lived experiences, or thoughts.
The four most common types of interviews are:
- Structured interviews : The questions are predetermined in both topic and order.
- Semi-structured interviews : A few questions are predetermined, but other questions aren’t planned.
- Unstructured interviews : None of the questions are predetermined.
- Focus group interviews : The questions are presented to a group instead of one individual.
Deductive reasoning is commonly used in scientific research, and it’s especially associated with quantitative research .
In research, you might have come across something called the hypothetico-deductive method . It’s the scientific method of testing hypotheses to check whether your predictions are substantiated by real-world data.
Deductive reasoning is a logical approach where you progress from general ideas to specific conclusions. It’s often contrasted with inductive reasoning , where you start with specific observations and form general conclusions.
Deductive reasoning is also called deductive logic.
There are many different types of inductive reasoning that people use formally or informally.
Here are a few common types:
- Inductive generalization : You use observations about a sample to come to a conclusion about the population it came from.
- Statistical generalization: You use specific numbers about samples to make statements about populations.
- Causal reasoning: You make cause-and-effect links between different things.
- Sign reasoning: You make a conclusion about a correlational relationship between different things.
- Analogical reasoning: You make a conclusion about something based on its similarities to something else.
Inductive reasoning is a bottom-up approach, while deductive reasoning is top-down.
Inductive reasoning takes you from the specific to the general, while in deductive reasoning, you make inferences by going from general premises to specific conclusions.
In inductive research , you start by making observations or gathering data. Then, you take a broad scan of your data and search for patterns. Finally, you make general conclusions that you might incorporate into theories.
Inductive reasoning is a method of drawing conclusions by going from the specific to the general. It’s usually contrasted with deductive reasoning, where you proceed from general information to specific conclusions.
Inductive reasoning is also called inductive logic or bottom-up reasoning.
A hypothesis states your predictions about what your research will find. It is a tentative answer to your research question that has not yet been tested. For some research projects, you might have to write several hypotheses that address different aspects of your research question.
A hypothesis is not just a guess — it should be based on existing theories and knowledge. It also has to be testable, which means you can support or refute it through scientific research methods (such as experiments, observations and statistical analysis of data).
Triangulation can help:
- Reduce research bias that comes from using a single method, theory, or investigator
- Enhance validity by approaching the same topic with different tools
- Establish credibility by giving you a complete picture of the research problem
But triangulation can also pose problems:
- It’s time-consuming and labor-intensive, often involving an interdisciplinary team.
- Your results may be inconsistent or even contradictory.
There are four main types of triangulation :
- Data triangulation : Using data from different times, spaces, and people
- Investigator triangulation : Involving multiple researchers in collecting or analyzing data
- Theory triangulation : Using varying theoretical perspectives in your research
- Methodological triangulation : Using different methodologies to approach the same topic
Many academic fields use peer review , largely to determine whether a manuscript is suitable for publication. Peer review enhances the credibility of the published manuscript.
However, peer review is also common in non-academic settings. The United Nations, the European Union, and many individual nations use peer review to evaluate grant applications. It is also widely used in medical and health-related fields as a teaching or quality-of-care measure.
Peer assessment is often used in the classroom as a pedagogical tool. Both receiving feedback and providing it are thought to enhance the learning process, helping students think critically and collaboratively.
Peer review can stop obviously problematic, falsified, or otherwise untrustworthy research from being published. It also represents an excellent opportunity to get feedback from renowned experts in your field. It acts as a first defense, helping you ensure your argument is clear and that there are no gaps, vague terms, or unanswered questions for readers who weren’t involved in the research process.
Peer-reviewed articles are considered a highly credible source due to this stringent process they go through before publication.
In general, the peer review process follows the following steps:
- First, the author submits the manuscript to the editor.
- Reject the manuscript and send it back to author, or
- Send it onward to the selected peer reviewer(s)
- Next, the peer review process occurs. The reviewer provides feedback, addressing any major or minor issues with the manuscript, and gives their advice regarding what edits should be made.
- Lastly, the edited manuscript is sent back to the author. They input the edits, and resubmit it to the editor for publication.
Exploratory research is often used when the issue you’re studying is new or when the data collection process is challenging for some reason.
You can use exploratory research if you have a general idea or a specific question that you want to study but there is no preexisting knowledge or paradigm with which to study it.
Exploratory research is a methodology approach that explores research questions that have not previously been studied in depth. It is often used when the issue you’re studying is new, or the data collection process is challenging in some way.
Explanatory research is used to investigate how or why a phenomenon occurs. Therefore, this type of research is often one of the first stages in the research process , serving as a jumping-off point for future research.
Exploratory research aims to explore the main aspects of an under-researched problem, while explanatory research aims to explain the causes and consequences of a well-defined problem.
Explanatory research is a research method used to investigate how or why something occurs when only a small amount of information is available pertaining to that topic. It can help you increase your understanding of a given topic.
Clean data are valid, accurate, complete, consistent, unique, and uniform. Dirty data include inconsistencies and errors.
Dirty data can come from any part of the research process, including poor research design , inappropriate measurement materials, or flawed data entry.
Data cleaning takes place between data collection and data analyses. But you can use some methods even before collecting data.
For clean data, you should start by designing measures that collect valid data. Data validation at the time of data entry or collection helps you minimize the amount of data cleaning you’ll need to do.
After data collection, you can use data standardization and data transformation to clean your data. You’ll also deal with any missing values, outliers, and duplicate values.
Every dataset requires different techniques to clean dirty data , but you need to address these issues in a systematic way. You focus on finding and resolving data points that don’t agree or fit with the rest of your dataset.
These data might be missing values, outliers, duplicate values, incorrectly formatted, or irrelevant. You’ll start with screening and diagnosing your data. Then, you’ll often standardize and accept or remove data to make your dataset consistent and valid.
Data cleaning is necessary for valid and appropriate analyses. Dirty data contain inconsistencies or errors , but cleaning your data helps you minimize or resolve these.
Without data cleaning, you could end up with a Type I or II error in your conclusion. These types of erroneous conclusions can be practically significant with important consequences, because they lead to misplaced investments or missed opportunities.
Data cleaning involves spotting and resolving potential data inconsistencies or errors to improve your data quality. An error is any value (e.g., recorded weight) that doesn’t reflect the true value (e.g., actual weight) of something that’s being measured.
In this process, you review, analyze, detect, modify, or remove “dirty” data to make your dataset “clean.” Data cleaning is also called data cleansing or data scrubbing.
Research misconduct means making up or falsifying data, manipulating data analyses, or misrepresenting results in research reports. It’s a form of academic fraud.
These actions are committed intentionally and can have serious consequences; research misconduct is not a simple mistake or a point of disagreement but a serious ethical failure.
Anonymity means you don’t know who the participants are, while confidentiality means you know who they are but remove identifying information from your research report. Both are important ethical considerations .
You can only guarantee anonymity by not collecting any personally identifying information—for example, names, phone numbers, email addresses, IP addresses, physical characteristics, photos, or videos.
You can keep data confidential by using aggregate information in your research report, so that you only refer to groups of participants rather than individuals.
Research ethics matter for scientific integrity, human rights and dignity, and collaboration between science and society. These principles make sure that participation in studies is voluntary, informed, and safe.
Ethical considerations in research are a set of principles that guide your research designs and practices. These principles include voluntary participation, informed consent, anonymity, confidentiality, potential for harm, and results communication.
Scientists and researchers must always adhere to a certain code of conduct when collecting data from others .
These considerations protect the rights of research participants, enhance research validity , and maintain scientific integrity.
In multistage sampling , you can use probability or non-probability sampling methods .
For a probability sample, you have to conduct probability sampling at every stage.
You can mix it up by using simple random sampling , systematic sampling , or stratified sampling to select units at different stages, depending on what is applicable and relevant to your study.
Multistage sampling can simplify data collection when you have large, geographically spread samples, and you can obtain a probability sample without a complete sampling frame.
But multistage sampling may not lead to a representative sample, and larger samples are needed for multistage samples to achieve the statistical properties of simple random samples .
These are four of the most common mixed methods designs :
- Convergent parallel: Quantitative and qualitative data are collected at the same time and analyzed separately. After both analyses are complete, compare your results to draw overall conclusions.
- Embedded: Quantitative and qualitative data are collected at the same time, but within a larger quantitative or qualitative design. One type of data is secondary to the other.
- Explanatory sequential: Quantitative data is collected and analyzed first, followed by qualitative data. You can use this design if you think your qualitative data will explain and contextualize your quantitative findings.
- Exploratory sequential: Qualitative data is collected and analyzed first, followed by quantitative data. You can use this design if you think the quantitative data will confirm or validate your qualitative findings.
Triangulation in research means using multiple datasets, methods, theories and/or investigators to address a research question. It’s a research strategy that can help you enhance the validity and credibility of your findings.
Triangulation is mainly used in qualitative research , but it’s also commonly applied in quantitative research . Mixed methods research always uses triangulation.
In multistage sampling , or multistage cluster sampling, you draw a sample from a population using smaller and smaller groups at each stage.
This method is often used to collect data from a large, geographically spread group of people in national surveys, for example. You take advantage of hierarchical groupings (e.g., from state to city to neighborhood) to create a sample that’s less expensive and time-consuming to collect data from.
No, the steepness or slope of the line isn’t related to the correlation coefficient value. The correlation coefficient only tells you how closely your data fit on a line, so two datasets with the same correlation coefficient can have very different slopes.
To find the slope of the line, you’ll need to perform a regression analysis .
Correlation coefficients always range between -1 and 1.
The sign of the coefficient tells you the direction of the relationship: a positive value means the variables change together in the same direction, while a negative value means they change together in opposite directions.
The absolute value of a number is equal to the number without its sign. The absolute value of a correlation coefficient tells you the magnitude of the correlation: the greater the absolute value, the stronger the correlation.
These are the assumptions your data must meet if you want to use Pearson’s r :
- Both variables are on an interval or ratio level of measurement
- Data from both variables follow normal distributions
- Your data have no outliers
- Your data is from a random or representative sample
- You expect a linear relationship between the two variables
Quantitative research designs can be divided into two main categories:
- Correlational and descriptive designs are used to investigate characteristics, averages, trends, and associations between variables.
- Experimental and quasi-experimental designs are used to test causal relationships .
Qualitative research designs tend to be more flexible. Common types of qualitative design include case study , ethnography , and grounded theory designs.
A well-planned research design helps ensure that your methods match your research aims, that you collect high-quality data, and that you use the right kind of analysis to answer your questions, utilizing credible sources . This allows you to draw valid , trustworthy conclusions.
The priorities of a research design can vary depending on the field, but you usually have to specify:
- Your research questions and/or hypotheses
- Your overall approach (e.g., qualitative or quantitative )
- The type of design you’re using (e.g., a survey , experiment , or case study )
- Your sampling methods or criteria for selecting subjects
- Your data collection methods (e.g., questionnaires , observations)
- Your data collection procedures (e.g., operationalization , timing and data management)
- Your data analysis methods (e.g., statistical tests or thematic analysis )
A research design is a strategy for answering your research question . It defines your overall approach and determines how you will collect and analyze data.
Questionnaires can be self-administered or researcher-administered.
Self-administered questionnaires can be delivered online or in paper-and-pen formats, in person or through mail. All questions are standardized so that all respondents receive the same questions with identical wording.
Researcher-administered questionnaires are interviews that take place by phone, in-person, or online between researchers and respondents. You can gain deeper insights by clarifying questions for respondents or asking follow-up questions.
You can organize the questions logically, with a clear progression from simple to complex, or randomly between respondents. A logical flow helps respondents process the questionnaire easier and quicker, but it may lead to bias. Randomization can minimize the bias from order effects.
Closed-ended, or restricted-choice, questions offer respondents a fixed set of choices to select from. These questions are easier to answer quickly.
Open-ended or long-form questions allow respondents to answer in their own words. Because there are no restrictions on their choices, respondents can answer in ways that researchers may not have otherwise considered.
A questionnaire is a data collection tool or instrument, while a survey is an overarching research method that involves collecting and analyzing data from people using questionnaires.
The third variable and directionality problems are two main reasons why correlation isn’t causation .
The third variable problem means that a confounding variable affects both variables to make them seem causally related when they are not.
The directionality problem is when two variables correlate and might actually have a causal relationship, but it’s impossible to conclude which variable causes changes in the other.
Correlation describes an association between variables : when one variable changes, so does the other. A correlation is a statistical indicator of the relationship between variables.
Causation means that changes in one variable brings about changes in the other (i.e., there is a cause-and-effect relationship between variables). The two variables are correlated with each other, and there’s also a causal link between them.
While causation and correlation can exist simultaneously, correlation does not imply causation. In other words, correlation is simply a relationship where A relates to B—but A doesn’t necessarily cause B to happen (or vice versa). Mistaking correlation for causation is a common error and can lead to false cause fallacy .
Controlled experiments establish causality, whereas correlational studies only show associations between variables.
- In an experimental design , you manipulate an independent variable and measure its effect on a dependent variable. Other variables are controlled so they can’t impact the results.
- In a correlational design , you measure variables without manipulating any of them. You can test whether your variables change together, but you can’t be sure that one variable caused a change in another.
In general, correlational research is high in external validity while experimental research is high in internal validity .
A correlation is usually tested for two variables at a time, but you can test correlations between three or more variables.
A correlation coefficient is a single number that describes the strength and direction of the relationship between your variables.
Different types of correlation coefficients might be appropriate for your data based on their levels of measurement and distributions . The Pearson product-moment correlation coefficient (Pearson’s r ) is commonly used to assess a linear relationship between two quantitative variables.
A correlational research design investigates relationships between two variables (or more) without the researcher controlling or manipulating any of them. It’s a non-experimental type of quantitative research .
A correlation reflects the strength and/or direction of the association between two or more variables.
- A positive correlation means that both variables change in the same direction.
- A negative correlation means that the variables change in opposite directions.
- A zero correlation means there’s no relationship between the variables.
Random error is almost always present in scientific studies, even in highly controlled settings. While you can’t eradicate it completely, you can reduce random error by taking repeated measurements, using a large sample, and controlling extraneous variables .
You can avoid systematic error through careful design of your sampling , data collection , and analysis procedures. For example, use triangulation to measure your variables using multiple methods; regularly calibrate instruments or procedures; use random sampling and random assignment ; and apply masking (blinding) where possible.
Systematic error is generally a bigger problem in research.
With random error, multiple measurements will tend to cluster around the true value. When you’re collecting data from a large sample , the errors in different directions will cancel each other out.
Systematic errors are much more problematic because they can skew your data away from the true value. This can lead you to false conclusions ( Type I and II errors ) about the relationship between the variables you’re studying.
Random and systematic error are two types of measurement error.
Random error is a chance difference between the observed and true values of something (e.g., a researcher misreading a weighing scale records an incorrect measurement).
Systematic error is a consistent or proportional difference between the observed and true values of something (e.g., a miscalibrated scale consistently records weights as higher than they actually are).
On graphs, the explanatory variable is conventionally placed on the x-axis, while the response variable is placed on the y-axis.
- If you have quantitative variables , use a scatterplot or a line graph.
- If your response variable is categorical, use a scatterplot or a line graph.
- If your explanatory variable is categorical, use a bar graph.
The term “ explanatory variable ” is sometimes preferred over “ independent variable ” because, in real world contexts, independent variables are often influenced by other variables. This means they aren’t totally independent.
Multiple independent variables may also be correlated with each other, so “explanatory variables” is a more appropriate term.
The difference between explanatory and response variables is simple:
- An explanatory variable is the expected cause, and it explains the results.
- A response variable is the expected effect, and it responds to other variables.
There are 4 main types of extraneous variables :
- Demand characteristics : environmental cues that encourage participants to conform to researchers’ expectations.
- Experimenter effects : unintentional actions by researchers that influence study outcomes.
- Situational variables : environmental variables that alter participants’ behaviors.
- Participant variables : any characteristic or aspect of a participant’s background that could affect study results.
An extraneous variable is any variable that you’re not investigating that can potentially affect the dependent variable of your research study.
A confounding variable is a type of extraneous variable that not only affects the dependent variable, but is also related to the independent variable.
In a factorial design, multiple independent variables are tested.
If you test two variables, each level of one independent variable is combined with each level of the other independent variable to create different conditions.
Within-subjects designs have many potential threats to internal validity , but they are also very statistically powerful .
Advantages:
- Only requires small samples
- Statistically powerful
- Removes the effects of individual differences on the outcomes
Disadvantages:
- Internal validity threats reduce the likelihood of establishing a direct relationship between variables
- Time-related effects, such as growth, can influence the outcomes
- Carryover effects mean that the specific order of different treatments affect the outcomes
While a between-subjects design has fewer threats to internal validity , it also requires more participants for high statistical power than a within-subjects design .
- Prevents carryover effects of learning and fatigue.
- Shorter study duration.
- Needs larger samples for high power.
- Uses more resources to recruit participants, administer sessions, cover costs, etc.
- Individual differences may be an alternative explanation for results.
Yes. Between-subjects and within-subjects designs can be combined in a single study when you have two or more independent variables (a factorial design). In a mixed factorial design, one variable is altered between subjects and another is altered within subjects.
In a between-subjects design , every participant experiences only one condition, and researchers assess group differences between participants in various conditions.
In a within-subjects design , each participant experiences all conditions, and researchers test the same participants repeatedly for differences between conditions.
The word “between” means that you’re comparing different conditions between groups, while the word “within” means you’re comparing different conditions within the same group.
Random assignment is used in experiments with a between-groups or independent measures design. In this research design, there’s usually a control group and one or more experimental groups. Random assignment helps ensure that the groups are comparable.
In general, you should always use random assignment in this type of experimental design when it is ethically possible and makes sense for your study topic.
To implement random assignment , assign a unique number to every member of your study’s sample .
Then, you can use a random number generator or a lottery method to randomly assign each number to a control or experimental group. You can also do so manually, by flipping a coin or rolling a dice to randomly assign participants to groups.
Random selection, or random sampling , is a way of selecting members of a population for your study’s sample.
In contrast, random assignment is a way of sorting the sample into control and experimental groups.
Random sampling enhances the external validity or generalizability of your results, while random assignment improves the internal validity of your study.
In experimental research, random assignment is a way of placing participants from your sample into different groups using randomization. With this method, every member of the sample has a known or equal chance of being placed in a control group or an experimental group.
“Controlling for a variable” means measuring extraneous variables and accounting for them statistically to remove their effects on other variables.
Researchers often model control variable data along with independent and dependent variable data in regression analyses and ANCOVAs . That way, you can isolate the control variable’s effects from the relationship between the variables of interest.
Control variables help you establish a correlational or causal relationship between variables by enhancing internal validity .
If you don’t control relevant extraneous variables , they may influence the outcomes of your study, and you may not be able to demonstrate that your results are really an effect of your independent variable .
A control variable is any variable that’s held constant in a research study. It’s not a variable of interest in the study, but it’s controlled because it could influence the outcomes.
Including mediators and moderators in your research helps you go beyond studying a simple relationship between two variables for a fuller picture of the real world. They are important to consider when studying complex correlational or causal relationships.
Mediators are part of the causal pathway of an effect, and they tell you how or why an effect takes place. Moderators usually help you judge the external validity of your study by identifying the limitations of when the relationship between variables holds.
If something is a mediating variable :
- It’s caused by the independent variable .
- It influences the dependent variable
- When it’s taken into account, the statistical correlation between the independent and dependent variables is higher than when it isn’t considered.
A confounder is a third variable that affects variables of interest and makes them seem related when they are not. In contrast, a mediator is the mechanism of a relationship between two variables: it explains the process by which they are related.
A mediator variable explains the process through which two variables are related, while a moderator variable affects the strength and direction of that relationship.
There are three key steps in systematic sampling :
- Define and list your population , ensuring that it is not ordered in a cyclical or periodic order.
- Decide on your sample size and calculate your interval, k , by dividing your population by your target sample size.
- Choose every k th member of the population as your sample.
Systematic sampling is a probability sampling method where researchers select members of the population at a regular interval – for example, by selecting every 15th person on a list of the population. If the population is in a random order, this can imitate the benefits of simple random sampling .
Yes, you can create a stratified sample using multiple characteristics, but you must ensure that every participant in your study belongs to one and only one subgroup. In this case, you multiply the numbers of subgroups for each characteristic to get the total number of groups.
For example, if you were stratifying by location with three subgroups (urban, rural, or suburban) and marital status with five subgroups (single, divorced, widowed, married, or partnered), you would have 3 x 5 = 15 subgroups.
You should use stratified sampling when your sample can be divided into mutually exclusive and exhaustive subgroups that you believe will take on different mean values for the variable that you’re studying.
Using stratified sampling will allow you to obtain more precise (with lower variance ) statistical estimates of whatever you are trying to measure.
For example, say you want to investigate how income differs based on educational attainment, but you know that this relationship can vary based on race. Using stratified sampling, you can ensure you obtain a large enough sample from each racial group, allowing you to draw more precise conclusions.
In stratified sampling , researchers divide subjects into subgroups called strata based on characteristics that they share (e.g., race, gender, educational attainment).
Once divided, each subgroup is randomly sampled using another probability sampling method.
Cluster sampling is more time- and cost-efficient than other probability sampling methods , particularly when it comes to large samples spread across a wide geographical area.
However, it provides less statistical certainty than other methods, such as simple random sampling , because it is difficult to ensure that your clusters properly represent the population as a whole.
There are three types of cluster sampling : single-stage, double-stage and multi-stage clustering. In all three types, you first divide the population into clusters, then randomly select clusters for use in your sample.
- In single-stage sampling , you collect data from every unit within the selected clusters.
- In double-stage sampling , you select a random sample of units from within the clusters.
- In multi-stage sampling , you repeat the procedure of randomly sampling elements from within the clusters until you have reached a manageable sample.
Cluster sampling is a probability sampling method in which you divide a population into clusters, such as districts or schools, and then randomly select some of these clusters as your sample.
The clusters should ideally each be mini-representations of the population as a whole.
If properly implemented, simple random sampling is usually the best sampling method for ensuring both internal and external validity . However, it can sometimes be impractical and expensive to implement, depending on the size of the population to be studied,
If you have a list of every member of the population and the ability to reach whichever members are selected, you can use simple random sampling.
The American Community Survey is an example of simple random sampling . In order to collect detailed data on the population of the US, the Census Bureau officials randomly select 3.5 million households per year and use a variety of methods to convince them to fill out the survey.
Simple random sampling is a type of probability sampling in which the researcher randomly selects a subset of participants from a population . Each member of the population has an equal chance of being selected. Data is then collected from as large a percentage as possible of this random subset.
Quasi-experimental design is most useful in situations where it would be unethical or impractical to run a true experiment .
Quasi-experiments have lower internal validity than true experiments, but they often have higher external validity as they can use real-world interventions instead of artificial laboratory settings.
A quasi-experiment is a type of research design that attempts to establish a cause-and-effect relationship. The main difference with a true experiment is that the groups are not randomly assigned.
Blinding is important to reduce research bias (e.g., observer bias , demand characteristics ) and ensure a study’s internal validity .
If participants know whether they are in a control or treatment group , they may adjust their behavior in ways that affect the outcome that researchers are trying to measure. If the people administering the treatment are aware of group assignment, they may treat participants differently and thus directly or indirectly influence the final results.
- In a single-blind study , only the participants are blinded.
- In a double-blind study , both participants and experimenters are blinded.
- In a triple-blind study , the assignment is hidden not only from participants and experimenters, but also from the researchers analyzing the data.
Blinding means hiding who is assigned to the treatment group and who is assigned to the control group in an experiment .
A true experiment (a.k.a. a controlled experiment) always includes at least one control group that doesn’t receive the experimental treatment.
However, some experiments use a within-subjects design to test treatments without a control group. In these designs, you usually compare one group’s outcomes before and after a treatment (instead of comparing outcomes between different groups).
For strong internal validity , it’s usually best to include a control group if possible. Without a control group, it’s harder to be certain that the outcome was caused by the experimental treatment and not by other variables.
An experimental group, also known as a treatment group, receives the treatment whose effect researchers wish to study, whereas a control group does not. They should be identical in all other ways.
Individual Likert-type questions are generally considered ordinal data , because the items have clear rank order, but don’t have an even distribution.
Overall Likert scale scores are sometimes treated as interval data. These scores are considered to have directionality and even spacing between them.
The type of data determines what statistical tests you should use to analyze your data.
A Likert scale is a rating scale that quantitatively assesses opinions, attitudes, or behaviors. It is made up of 4 or more questions that measure a single attitude or trait when response scores are combined.
To use a Likert scale in a survey , you present participants with Likert-type questions or statements, and a continuum of items, usually with 5 or 7 possible responses, to capture their degree of agreement.
In scientific research, concepts are the abstract ideas or phenomena that are being studied (e.g., educational achievement). Variables are properties or characteristics of the concept (e.g., performance at school), while indicators are ways of measuring or quantifying variables (e.g., yearly grade reports).
The process of turning abstract concepts into measurable variables and indicators is called operationalization .
There are various approaches to qualitative data analysis , but they all share five steps in common:
- Prepare and organize your data.
- Review and explore your data.
- Develop a data coding system.
- Assign codes to the data.
- Identify recurring themes.
The specifics of each step depend on the focus of the analysis. Some common approaches include textual analysis , thematic analysis , and discourse analysis .
There are five common approaches to qualitative research :
- Grounded theory involves collecting data in order to develop new theories.
- Ethnography involves immersing yourself in a group or organization to understand its culture.
- Narrative research involves interpreting stories to understand how people make sense of their experiences and perceptions.
- Phenomenological research involves investigating phenomena through people’s lived experiences.
- Action research links theory and practice in several cycles to drive innovative changes.
Hypothesis testing is a formal procedure for investigating our ideas about the world using statistics. It is used by scientists to test specific predictions, called hypotheses , by calculating how likely it is that a pattern or relationship between variables could have arisen by chance.
Operationalization means turning abstract conceptual ideas into measurable observations.
For example, the concept of social anxiety isn’t directly observable, but it can be operationally defined in terms of self-rating scores, behavioral avoidance of crowded places, or physical anxiety symptoms in social situations.
Before collecting data , it’s important to consider how you will operationalize the variables that you want to measure.
When conducting research, collecting original data has significant advantages:
- You can tailor data collection to your specific research aims (e.g. understanding the needs of your consumers or user testing your website)
- You can control and standardize the process for high reliability and validity (e.g. choosing appropriate measurements and sampling methods )
However, there are also some drawbacks: data collection can be time-consuming, labor-intensive and expensive. In some cases, it’s more efficient to use secondary data that has already been collected by someone else, but the data might be less reliable.
Data collection is the systematic process by which observations or measurements are gathered in research. It is used in many different contexts by academics, governments, businesses, and other organizations.
There are several methods you can use to decrease the impact of confounding variables on your research: restriction, matching, statistical control and randomization.
In restriction , you restrict your sample by only including certain subjects that have the same values of potential confounding variables.
In matching , you match each of the subjects in your treatment group with a counterpart in the comparison group. The matched subjects have the same values on any potential confounding variables, and only differ in the independent variable .
In statistical control , you include potential confounders as variables in your regression .
In randomization , you randomly assign the treatment (or independent variable) in your study to a sufficiently large number of subjects, which allows you to control for all potential confounding variables.
A confounding variable is closely related to both the independent and dependent variables in a study. An independent variable represents the supposed cause , while the dependent variable is the supposed effect . A confounding variable is a third variable that influences both the independent and dependent variables.
Failing to account for confounding variables can cause you to wrongly estimate the relationship between your independent and dependent variables.
To ensure the internal validity of your research, you must consider the impact of confounding variables. If you fail to account for them, you might over- or underestimate the causal relationship between your independent and dependent variables , or even find a causal relationship where none exists.
Yes, but including more than one of either type requires multiple research questions .
For example, if you are interested in the effect of a diet on health, you can use multiple measures of health: blood sugar, blood pressure, weight, pulse, and many more. Each of these is its own dependent variable with its own research question.
You could also choose to look at the effect of exercise levels as well as diet, or even the additional effect of the two combined. Each of these is a separate independent variable .
To ensure the internal validity of an experiment , you should only change one independent variable at a time.
No. The value of a dependent variable depends on an independent variable, so a variable cannot be both independent and dependent at the same time. It must be either the cause or the effect, not both!
You want to find out how blood sugar levels are affected by drinking diet soda and regular soda, so you conduct an experiment .
- The type of soda – diet or regular – is the independent variable .
- The level of blood sugar that you measure is the dependent variable – it changes depending on the type of soda.
Determining cause and effect is one of the most important parts of scientific research. It’s essential to know which is the cause – the independent variable – and which is the effect – the dependent variable.
In non-probability sampling , the sample is selected based on non-random criteria, and not every member of the population has a chance of being included.
Common non-probability sampling methods include convenience sampling , voluntary response sampling, purposive sampling , snowball sampling, and quota sampling .
Probability sampling means that every member of the target population has a known chance of being included in the sample.
Probability sampling methods include simple random sampling , systematic sampling , stratified sampling , and cluster sampling .
Using careful research design and sampling procedures can help you avoid sampling bias . Oversampling can be used to correct undercoverage bias .
Some common types of sampling bias include self-selection bias , nonresponse bias , undercoverage bias , survivorship bias , pre-screening or advertising bias, and healthy user bias.
Sampling bias is a threat to external validity – it limits the generalizability of your findings to a broader group of people.
A sampling error is the difference between a population parameter and a sample statistic .
A statistic refers to measures about the sample , while a parameter refers to measures about the population .
Populations are used when a research question requires data from every member of the population. This is usually only feasible when the population is small and easily accessible.
Samples are used to make inferences about populations . Samples are easier to collect data from because they are practical, cost-effective, convenient, and manageable.
There are seven threats to external validity : selection bias , history, experimenter effect, Hawthorne effect , testing effect, aptitude-treatment and situation effect.
The two types of external validity are population validity (whether you can generalize to other groups of people) and ecological validity (whether you can generalize to other situations and settings).
The external validity of a study is the extent to which you can generalize your findings to different groups of people, situations, and measures.
Cross-sectional studies cannot establish a cause-and-effect relationship or analyze behavior over a period of time. To investigate cause and effect, you need to do a longitudinal study or an experimental study .
Cross-sectional studies are less expensive and time-consuming than many other types of study. They can provide useful insights into a population’s characteristics and identify correlations for further research.
Sometimes only cross-sectional data is available for analysis; other times your research question may only require a cross-sectional study to answer it.
Longitudinal studies can last anywhere from weeks to decades, although they tend to be at least a year long.
The 1970 British Cohort Study , which has collected data on the lives of 17,000 Brits since their births in 1970, is one well-known example of a longitudinal study .
Longitudinal studies are better to establish the correct sequence of events, identify changes over time, and provide insight into cause-and-effect relationships, but they also tend to be more expensive and time-consuming than other types of studies.
Longitudinal studies and cross-sectional studies are two different types of research design . In a cross-sectional study you collect data from a population at a specific point in time; in a longitudinal study you repeatedly collect data from the same sample over an extended period of time.
Longitudinal study | Cross-sectional study |
---|---|
observations | Observations at a in time |
Observes the multiple times | Observes (a “cross-section”) in the population |
Follows in participants over time | Provides of society at a given point |
There are eight threats to internal validity : history, maturation, instrumentation, testing, selection bias , regression to the mean, social interaction and attrition .
Internal validity is the extent to which you can be confident that a cause-and-effect relationship established in a study cannot be explained by other factors.
In mixed methods research , you use both qualitative and quantitative data collection and analysis methods to answer your research question .
The research methods you use depend on the type of data you need to answer your research question .
- If you want to measure something or test a hypothesis , use quantitative methods . If you want to explore ideas, thoughts and meanings, use qualitative methods .
- If you want to analyze a large amount of readily-available data, use secondary data. If you want data specific to your purposes with control over how it is generated, collect primary data.
- If you want to establish cause-and-effect relationships between variables , use experimental methods. If you want to understand the characteristics of a research subject, use descriptive methods.
A confounding variable , also called a confounder or confounding factor, is a third variable in a study examining a potential cause-and-effect relationship.
A confounding variable is related to both the supposed cause and the supposed effect of the study. It can be difficult to separate the true effect of the independent variable from the effect of the confounding variable.
In your research design , it’s important to identify potential confounding variables and plan how you will reduce their impact.
Discrete and continuous variables are two types of quantitative variables :
- Discrete variables represent counts (e.g. the number of objects in a collection).
- Continuous variables represent measurable amounts (e.g. water volume or weight).
Quantitative variables are any variables where the data represent amounts (e.g. height, weight, or age).
Categorical variables are any variables where the data represent groups. This includes rankings (e.g. finishing places in a race), classifications (e.g. brands of cereal), and binary outcomes (e.g. coin flips).
You need to know what type of variables you are working with to choose the right statistical test for your data and interpret your results .
You can think of independent and dependent variables in terms of cause and effect: an independent variable is the variable you think is the cause , while a dependent variable is the effect .
In an experiment, you manipulate the independent variable and measure the outcome in the dependent variable. For example, in an experiment about the effect of nutrients on crop growth:
- The independent variable is the amount of nutrients added to the crop field.
- The dependent variable is the biomass of the crops at harvest time.
Defining your variables, and deciding how you will manipulate and measure them, is an important part of experimental design .
Experimental design means planning a set of procedures to investigate a relationship between variables . To design a controlled experiment, you need:
- A testable hypothesis
- At least one independent variable that can be precisely manipulated
- At least one dependent variable that can be precisely measured
When designing the experiment, you decide:
- How you will manipulate the variable(s)
- How you will control for any potential confounding variables
- How many subjects or samples will be included in the study
- How subjects will be assigned to treatment levels
Experimental design is essential to the internal and external validity of your experiment.
I nternal validity is the degree of confidence that the causal relationship you are testing is not influenced by other factors or variables .
External validity is the extent to which your results can be generalized to other contexts.
The validity of your experiment depends on your experimental design .
Reliability and validity are both about how well a method measures something:
- Reliability refers to the consistency of a measure (whether the results can be reproduced under the same conditions).
- Validity refers to the accuracy of a measure (whether the results really do represent what they are supposed to measure).
If you are doing experimental research, you also have to consider the internal and external validity of your experiment.
A sample is a subset of individuals from a larger population . Sampling means selecting the group that you will actually collect data from in your research. For example, if you are researching the opinions of students in your university, you could survey a sample of 100 students.
In statistics, sampling allows you to test a hypothesis about the characteristics of a population.
Quantitative research deals with numbers and statistics, while qualitative research deals with words and meanings.
Quantitative methods allow you to systematically measure variables and test hypotheses . Qualitative methods allow you to explore concepts and experiences in more detail.
Methodology refers to the overarching strategy and rationale of your research project . It involves studying the methods used in your field and the theories or principles behind them, in order to develop an approach that matches your objectives.
Methods are the specific tools and procedures you use to collect and analyze data (for example, experiments, surveys , and statistical tests ).
In shorter scientific papers, where the aim is to report the findings of a specific study, you might simply describe what you did in a methods section .
In a longer or more complex research project, such as a thesis or dissertation , you will probably include a methodology section , where you explain your approach to answering the research questions and cite relevant sources to support your choice of methods.
Ask our team
Want to contact us directly? No problem. We are always here for you.
- Email [email protected]
- Start live chat
- Call +1 (510) 822-8066
- WhatsApp +31 20 261 6040
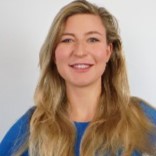
Our team helps students graduate by offering:
- A world-class citation generator
- Plagiarism Checker software powered by Turnitin
- Innovative Citation Checker software
- Professional proofreading services
- Over 300 helpful articles about academic writing, citing sources, plagiarism, and more
Scribbr specializes in editing study-related documents . We proofread:
- PhD dissertations
- Research proposals
- Personal statements
- Admission essays
- Motivation letters
- Reflection papers
- Journal articles
- Capstone projects
Scribbr’s Plagiarism Checker is powered by elements of Turnitin’s Similarity Checker , namely the plagiarism detection software and the Internet Archive and Premium Scholarly Publications content databases .
The add-on AI detector is powered by Scribbr’s proprietary software.
The Scribbr Citation Generator is developed using the open-source Citation Style Language (CSL) project and Frank Bennett’s citeproc-js . It’s the same technology used by dozens of other popular citation tools, including Mendeley and Zotero.
You can find all the citation styles and locales used in the Scribbr Citation Generator in our publicly accessible repository on Github .
Experimental Method In Psychology
Saul Mcleod, PhD
Editor-in-Chief for Simply Psychology
BSc (Hons) Psychology, MRes, PhD, University of Manchester
Saul Mcleod, PhD., is a qualified psychology teacher with over 18 years of experience in further and higher education. He has been published in peer-reviewed journals, including the Journal of Clinical Psychology.
Learn about our Editorial Process
Olivia Guy-Evans, MSc
Associate Editor for Simply Psychology
BSc (Hons) Psychology, MSc Psychology of Education
Olivia Guy-Evans is a writer and associate editor for Simply Psychology. She has previously worked in healthcare and educational sectors.
On This Page:
The experimental method involves the manipulation of variables to establish cause-and-effect relationships. The key features are controlled methods and the random allocation of participants into controlled and experimental groups .
What is an Experiment?
An experiment is an investigation in which a hypothesis is scientifically tested. An independent variable (the cause) is manipulated in an experiment, and the dependent variable (the effect) is measured; any extraneous variables are controlled.
An advantage is that experiments should be objective. The researcher’s views and opinions should not affect a study’s results. This is good as it makes the data more valid and less biased.
There are three types of experiments you need to know:
1. Lab Experiment
A laboratory experiment in psychology is a research method in which the experimenter manipulates one or more independent variables and measures the effects on the dependent variable under controlled conditions.
A laboratory experiment is conducted under highly controlled conditions (not necessarily a laboratory) where accurate measurements are possible.
The researcher uses a standardized procedure to determine where the experiment will take place, at what time, with which participants, and in what circumstances.
Participants are randomly allocated to each independent variable group.
Examples are Milgram’s experiment on obedience and Loftus and Palmer’s car crash study .
- Strength : It is easier to replicate (i.e., copy) a laboratory experiment. This is because a standardized procedure is used.
- Strength : They allow for precise control of extraneous and independent variables. This allows a cause-and-effect relationship to be established.
- Limitation : The artificiality of the setting may produce unnatural behavior that does not reflect real life, i.e., low ecological validity. This means it would not be possible to generalize the findings to a real-life setting.
- Limitation : Demand characteristics or experimenter effects may bias the results and become confounding variables .
2. Field Experiment
A field experiment is a research method in psychology that takes place in a natural, real-world setting. It is similar to a laboratory experiment in that the experimenter manipulates one or more independent variables and measures the effects on the dependent variable.
However, in a field experiment, the participants are unaware they are being studied, and the experimenter has less control over the extraneous variables .
Field experiments are often used to study social phenomena, such as altruism, obedience, and persuasion. They are also used to test the effectiveness of interventions in real-world settings, such as educational programs and public health campaigns.
An example is Holfing’s hospital study on obedience .
- Strength : behavior in a field experiment is more likely to reflect real life because of its natural setting, i.e., higher ecological validity than a lab experiment.
- Strength : Demand characteristics are less likely to affect the results, as participants may not know they are being studied. This occurs when the study is covert.
- Limitation : There is less control over extraneous variables that might bias the results. This makes it difficult for another researcher to replicate the study in exactly the same way.
3. Natural Experiment
A natural experiment in psychology is a research method in which the experimenter observes the effects of a naturally occurring event or situation on the dependent variable without manipulating any variables.
Natural experiments are conducted in the day (i.e., real life) environment of the participants, but here, the experimenter has no control over the independent variable as it occurs naturally in real life.
Natural experiments are often used to study psychological phenomena that would be difficult or unethical to study in a laboratory setting, such as the effects of natural disasters, policy changes, or social movements.
For example, Hodges and Tizard’s attachment research (1989) compared the long-term development of children who have been adopted, fostered, or returned to their mothers with a control group of children who had spent all their lives in their biological families.
Here is a fictional example of a natural experiment in psychology:
Researchers might compare academic achievement rates among students born before and after a major policy change that increased funding for education.
In this case, the independent variable is the timing of the policy change, and the dependent variable is academic achievement. The researchers would not be able to manipulate the independent variable, but they could observe its effects on the dependent variable.
- Strength : behavior in a natural experiment is more likely to reflect real life because of its natural setting, i.e., very high ecological validity.
- Strength : Demand characteristics are less likely to affect the results, as participants may not know they are being studied.
- Strength : It can be used in situations in which it would be ethically unacceptable to manipulate the independent variable, e.g., researching stress .
- Limitation : They may be more expensive and time-consuming than lab experiments.
- Limitation : There is no control over extraneous variables that might bias the results. This makes it difficult for another researcher to replicate the study in exactly the same way.
Key Terminology
Ecological validity.
The degree to which an investigation represents real-life experiences.
Experimenter effects
These are the ways that the experimenter can accidentally influence the participant through their appearance or behavior.
Demand characteristics
The clues in an experiment lead the participants to think they know what the researcher is looking for (e.g., the experimenter’s body language).
Independent variable (IV)
The variable the experimenter manipulates (i.e., changes) is assumed to have a direct effect on the dependent variable.
Dependent variable (DV)
Variable the experimenter measures. This is the outcome (i.e., the result) of a study.
Extraneous variables (EV)
All variables which are not independent variables but could affect the results (DV) of the experiment. EVs should be controlled where possible.
Confounding variables
Variable(s) that have affected the results (DV), apart from the IV. A confounding variable could be an extraneous variable that has not been controlled.
Random Allocation
Randomly allocating participants to independent variable conditions means that all participants should have an equal chance of participating in each condition.
The principle of random allocation is to avoid bias in how the experiment is carried out and limit the effects of participant variables.
Order effects
Changes in participants’ performance due to their repeating the same or similar test more than once. Examples of order effects include:
(i) practice effect: an improvement in performance on a task due to repetition, for example, because of familiarity with the task;
(ii) fatigue effect: a decrease in performance of a task due to repetition, for example, because of boredom or tiredness.

Related Articles
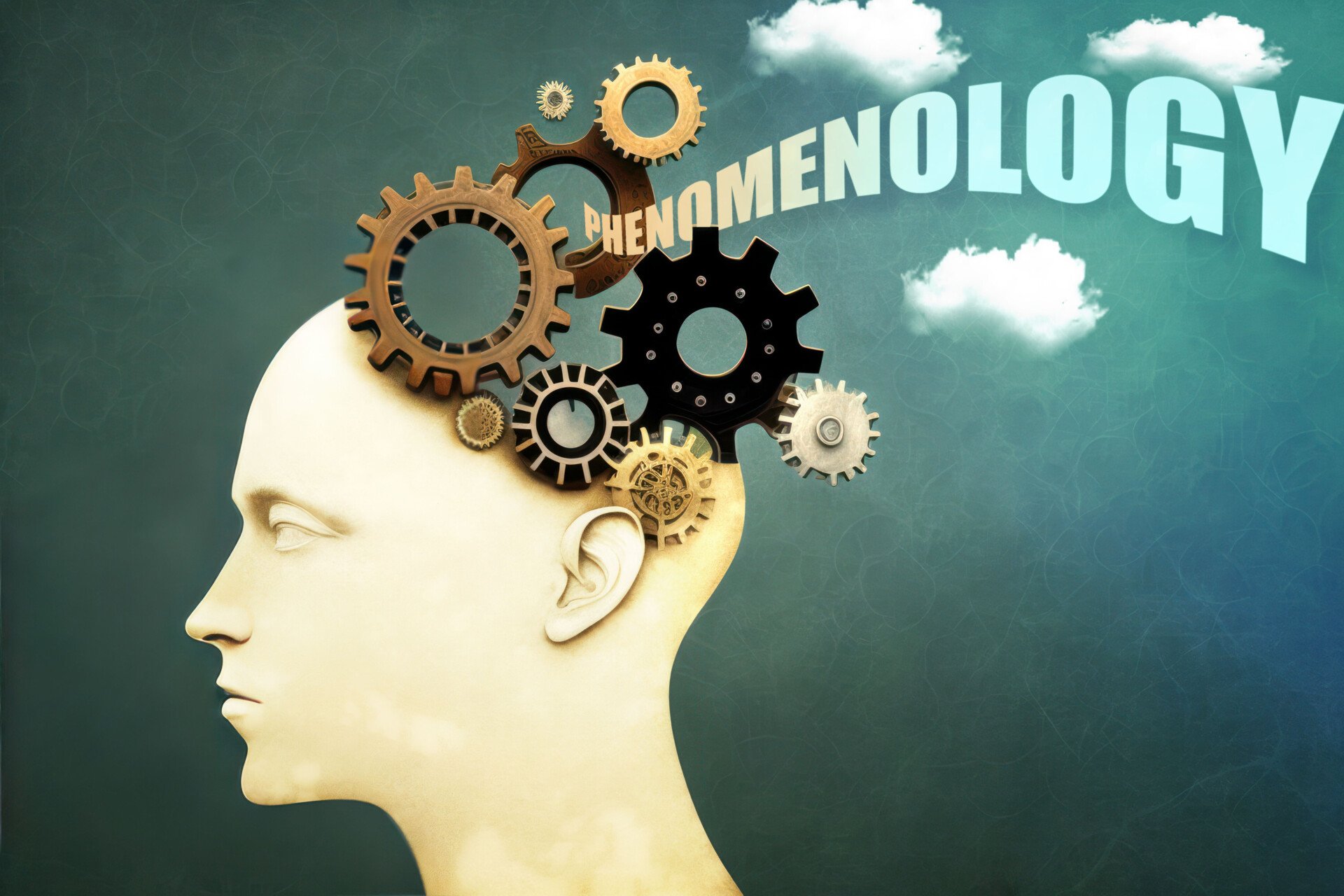
Research Methodology
Phenomenology In Qualitative Research
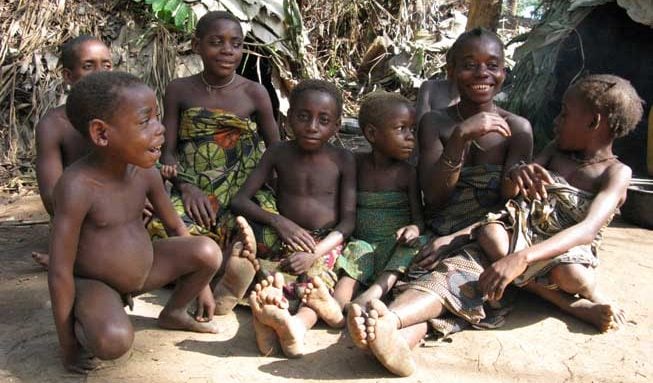
Ethnography In Qualitative Research
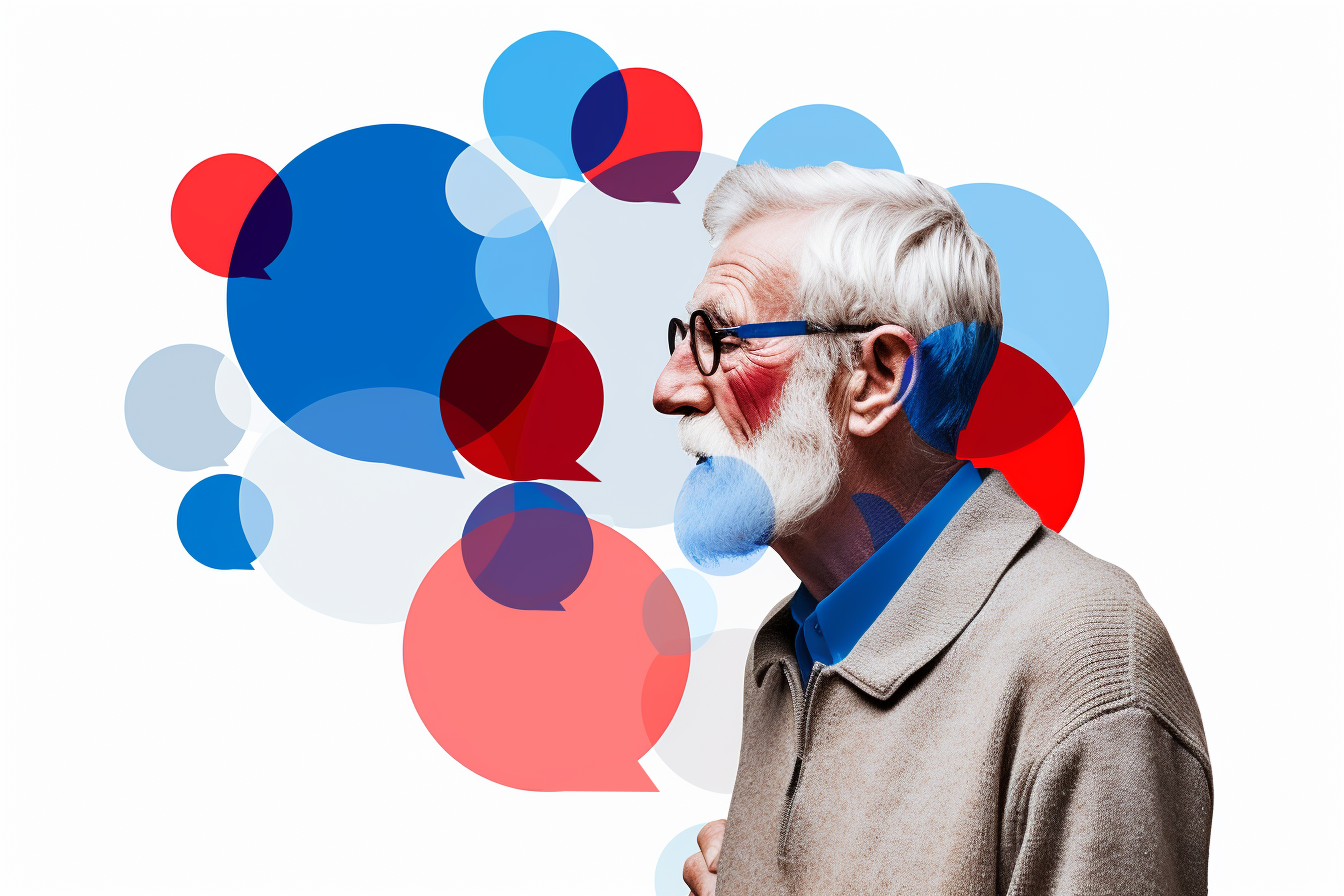
Narrative Analysis In Qualitative Research
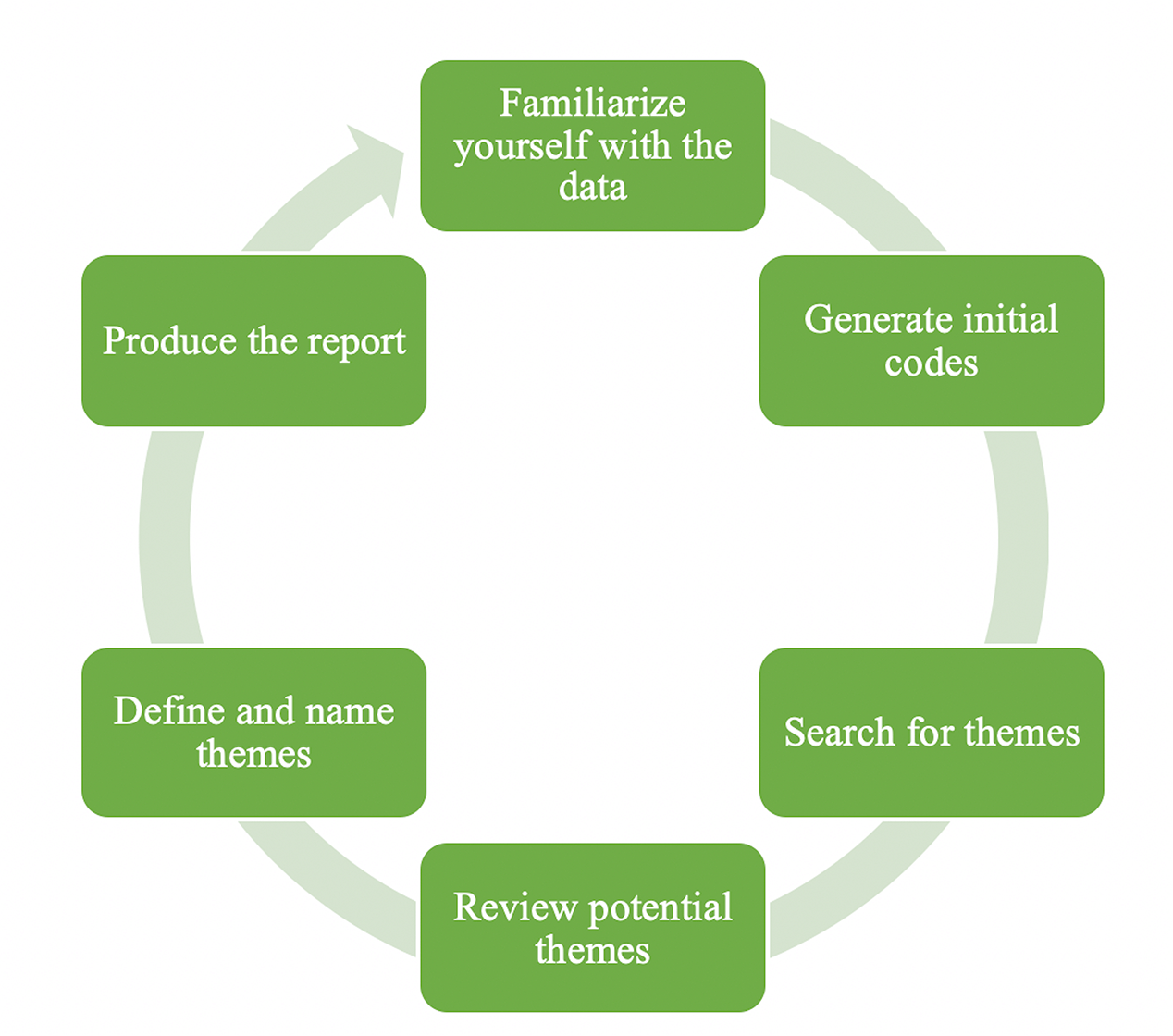
Thematic Analysis: A Step by Step Guide
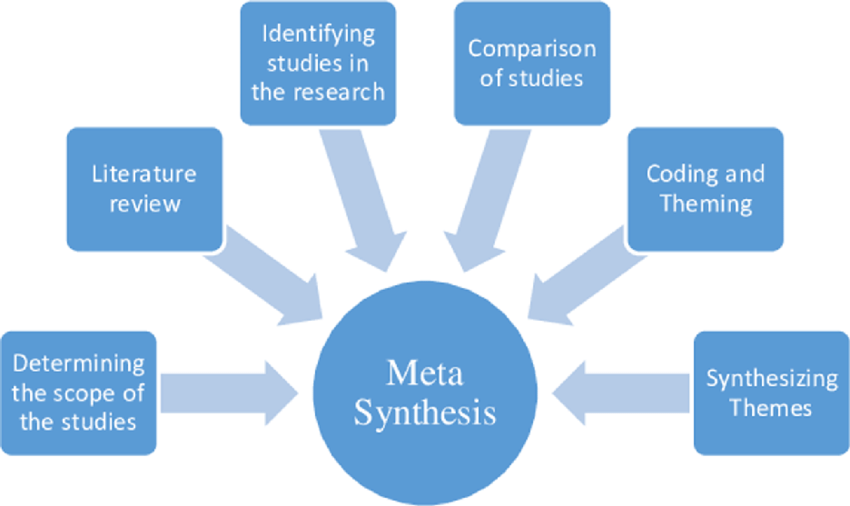
Metasynthesis Of Qualitative Research
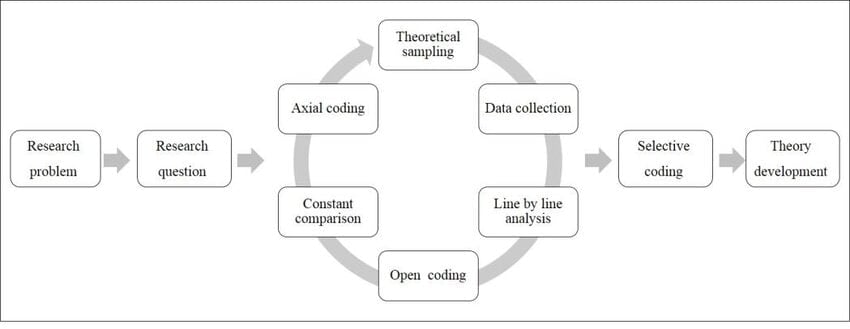
Grounded Theory In Qualitative Research: A Practical Guide

- Science Notes Posts
- Contact Science Notes
- Todd Helmenstine Biography
- Anne Helmenstine Biography
- Free Printable Periodic Tables (PDF and PNG)
- Periodic Table Wallpapers
- Interactive Periodic Table
- Periodic Table Posters
- Science Experiments for Kids
- How to Grow Crystals
- Chemistry Projects
- Fire and Flames Projects
- Holiday Science
- Chemistry Problems With Answers
- Physics Problems
- Unit Conversion Example Problems
- Chemistry Worksheets
- Biology Worksheets
- Periodic Table Worksheets
- Physical Science Worksheets
- Science Lab Worksheets
- My Amazon Books
Control Group Definition and Examples

The control group is the set of subjects that does not receive the treatment in a study. In other words, it is the group where the independent variable is held constant. This is important because the control group is a baseline for measuring the effects of a treatment in an experiment or study. A controlled experiment is one which includes one or more control groups.
- The experimental group experiences a treatment or change in the independent variable. In contrast, the independent variable is constant in the control group.
- A control group is important because it allows meaningful comparison. The researcher compares the experimental group to it to assess whether or not there is a relationship between the independent and dependent variable and the magnitude of the effect.
- There are different types of control groups. A controlled experiment has one more control group.
Control Group vs Experimental Group
The only difference between the control group and experimental group is that subjects in the experimental group receive the treatment being studied, while participants in the control group do not. Otherwise, all other variables between the two groups are the same.
Control Group vs Control Variable
A control group is not the same thing as a control variable. A control variable or controlled variable is any factor that is held constant during an experiment. Examples of common control variables include temperature, duration, and sample size. The control variables are the same for both the control and experimental groups.
Types of Control Groups
There are different types of control groups:
- Placebo group : A placebo group receives a placebo , which is a fake treatment that resembles the treatment in every respect except for the active ingredient. Both the placebo and treatment may contain inactive ingredients that produce side effects. Without a placebo group, these effects might be attributed to the treatment.
- Positive control group : A positive control group has conditions that guarantee a positive test result. The positive control group demonstrates an experiment is capable of producing a positive result. Positive controls help researchers identify problems with an experiment.
- Negative control group : A negative control group consists of subjects that are not exposed to a treatment. For example, in an experiment looking at the effect of fertilizer on plant growth, the negative control group receives no fertilizer.
- Natural control group : A natural control group usually is a set of subjects who naturally differ from the experimental group. For example, if you compare the effects of a treatment on women who have had children, the natural control group includes women who have not had children. Non-smokers are a natural control group in comparison to smokers.
- Randomized control group : The subjects in a randomized control group are randomly selected from a larger pool of subjects. Often, subjects are randomly assigned to either the control or experimental group. Randomization reduces bias in an experiment. There are different methods of randomly assigning test subjects.
Control Group Examples
Here are some examples of different control groups in action:
Negative Control and Placebo Group
For example, consider a study of a new cancer drug. The experimental group receives the drug. The placebo group receives a placebo, which contains the same ingredients as the drug formulation, minus the active ingredient. The negative control group receives no treatment. The reason for including the negative group is because the placebo group experiences some level of placebo effect, which is a response to experiencing some form of false treatment.
Positive and Negative Controls
For example, consider an experiment looking at whether a new drug kills bacteria. The experimental group exposes bacterial cultures to the drug. If the group survives, the drug is ineffective. If the group dies, the drug is effective.
The positive control group has a culture of bacteria that carry a drug resistance gene. If the bacteria survive drug exposure (as intended), then it shows the growth medium and conditions allow bacterial growth. If the positive control group dies, it indicates a problem with the experimental conditions. A negative control group of bacteria lacking drug resistance should die. If the negative control group survives, something is wrong with the experimental conditions.
- Bailey, R. A. (2008). Design of Comparative Experiments . Cambridge University Press. ISBN 978-0-521-68357-9.
- Chaplin, S. (2006). “The placebo response: an important part of treatment”. Prescriber . 17 (5): 16–22. doi: 10.1002/psb.344
- Hinkelmann, Klaus; Kempthorne, Oscar (2008). Design and Analysis of Experiments, Volume I: Introduction to Experimental Design (2nd ed.). Wiley. ISBN 978-0-471-72756-9.
- Pithon, M.M. (2013). “Importance of the control group in scientific research.” Dental Press J Orthod . 18 (6):13-14. doi: 10.1590/s2176-94512013000600003
- Stigler, Stephen M. (1992). “A Historical View of Statistical Concepts in Psychology and Educational Research”. American Journal of Education . 101 (1): 60–70. doi: 10.1086/444032
Related Posts
- Daily Crossword
- Word Puzzle
- Word Finder
- Word of the Day
- Synonym of the Day
- Word of the Year
- Language stories
- All featured
- Gender and sexuality
- All pop culture
- Writing hub
- Grammar essentials
- Commonly confused
- All writing tips
- Pop culture
- Writing tips
Advertisement
controlled experiment
- an experiment or trial that uses controls, usually separating the subjects into one or more control groups and experimental groups.
Discover More
Word history and origins.
Origin of controlled experiment 1
Example Sentences
In examining whether a school education improves the mental health of children, for example, no economist can design and run a controlled experiment.
Lysenko never undertook any controlled experiments on increased crop yield or the inheritance of acquired characteristics.
So, through a series of controlled experiments, the team nudged participants toward the minus sign.
While our controlled experiments showed DyRET could adapt its body successfully to surfaces it had walked on before, the real world is a much more variable and unpredictable place.
That is, the study wasn’t a randomized or controlled experiment.
A country of 300 million people is a permanent floating controlled experiment.
- To save this word, you'll need to log in. Log In
control experiment
Definition of control experiment
Examples of control experiment in a sentence.
These examples are programmatically compiled from various online sources to illustrate current usage of the word 'control experiment.' Any opinions expressed in the examples do not represent those of Merriam-Webster or its editors. Send us feedback about these examples.
Word History
1848, in the meaning defined above
Dictionary Entries Near control experiment
control electrode
control freak
Cite this Entry
“Control experiment.” Merriam-Webster.com Dictionary , Merriam-Webster, https://www.merriam-webster.com/dictionary/control%20experiment. Accessed 18 Jun. 2024.
Kids Definition
Kids definition of control experiment.
Subscribe to America's largest dictionary and get thousands more definitions and advanced search—ad free!
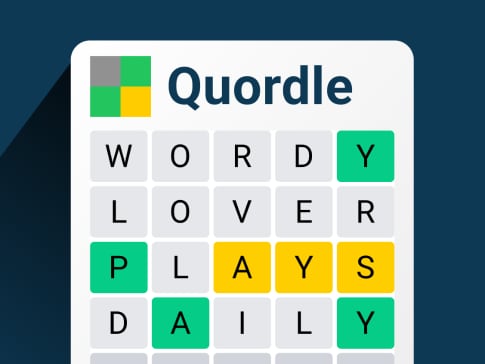
Can you solve 4 words at once?
Word of the day.
See Definitions and Examples »
Get Word of the Day daily email!
Popular in Grammar & Usage
Plural and possessive names: a guide, more commonly misspelled words, your vs. you're: how to use them correctly, every letter is silent, sometimes: a-z list of examples, more commonly mispronounced words, popular in wordplay, 8 words for lesser-known musical instruments, birds say the darndest things, 10 words from taylor swift songs (merriam's version), 10 scrabble words without any vowels, 12 more bird names that sound like insults (and sometimes are), games & quizzes.
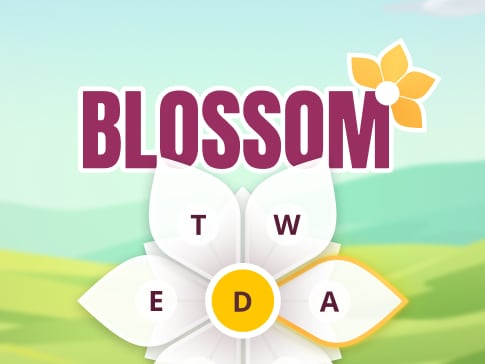
Scientific Method Vocabulary Terms
Cavan Images / Getty Images
- Scientific Method
- Chemical Laws
- Periodic Table
- Projects & Experiments
- Biochemistry
- Physical Chemistry
- Medical Chemistry
- Chemistry In Everyday Life
- Famous Chemists
- Activities for Kids
- Abbreviations & Acronyms
- Weather & Climate
- Ph.D., Biomedical Sciences, University of Tennessee at Knoxville
- B.A., Physics and Mathematics, Hastings College
Scientific experiments involve variables , controls, hypotheses , and a host of other concepts and terms that might be confusing.
Glossary of Science Terms
Here is a glossary of important science experiment terms and definitions:
- Central Limit Theorem: States that with a large enough sample, the sample mean will be normally distributed. A normally distributed sample mean is necessary to apply the t- test, so if you are planning to perform a statistical analysis of experimental data, it's important to have a sufficiently large sample.
- Conclusion: Determination of whether the hypothesis should be accepted or rejected.
- Control Group: Test subjects randomly assigned to not receive the experimental treatment.
- Control Variable: Any variable that does not change during an experiment. Also known as a constant variable.
- Data (singular: datum) : Facts, numbers, or values obtained in an experiment.
- Dependent Variable: The variable that responds to the independent variable. The dependent variable is the one being measured in the experiment. Also known as the dependent measure or responding variable.
- Double-Blind : When neither the researcher nor the subject knows whether the subject is receiving the treatment or a placebo. "Blinding" helps reduce biased results.
- Empty Control Group: A type of control group that does not receive any treatment, including a placebo.
- Experimental Group: Test subjects randomly assigned to receive the experimental treatment.
- Extraneous Variable: Extra variables (not independent, dependent, or control variables) that might influence an experiment but are not accounted for or measured or are beyond control. Examples might include factors you consider unimportant at the time of an experiment, such as the manufacturer of the glassware in a reaction or the color of paper used to make a paper airplane.
- Hypothesis: A prediction of whether the independent variable will have an effect on the dependent variable or a prediction of the nature of the effect.
- Independence or Independently: When one factor does not exert influence on another. For example, what one study participant does should not influence what another participant does. They make decisions independently. Independence is critical for a meaningful statistical analysis.
- Independent Random Assignment: Randomly selecting whether a test subject will be in a treatment or control group.
- Independent Variable : The variable that is manipulated or changed by the researcher.
- Independent Variable Levels: Changing the independent variable from one value to another (e.g., different drug doses, different amounts of time). The different values are called "levels."
- Inferential Statistics: Statistics (math) applied to infer characteristics of a population-based on a representative sample from the population.
- Internal Validity: When an experiment can accurately determine whether the independent variable produces an effect.
- Mean: The average calculated by adding all the scores and then dividing by the number of scores.
- Null Hypothesis : The "no difference" or "no effect" hypothesis, which predicts the treatment will not have an effect on the subject. The null hypothesis is useful because it is easier to assess with a statistical analysis than other forms of a hypothesis.
- Null Results (Nonsignificant Results): Results that do not disprove the null hypothesis. Null results don't prove the null hypothesis because the results may have resulted from a lack of power. Some null results are type 2 errors.
- p < 0.05: An indication of how often chance alone could account for the effect of the experimental treatment. A value p < 0.05 means that five times out of a hundred, you could expect this difference between the two groups purely by chance. Since the possibility of the effect occurring by chance is so small, the researcher may conclude the experimental treatment did indeed have an effect. Other p, or probability, values are possible. The 0.05 or 5% limit simply is a common benchmark of statistical significance.
- Placebo (Placebo Treatment): A fake treatment that should have no effect outside the power of suggestion. Example: In drug trials, test patients may be given a pill containing the drug or a placebo, which resembles the drug (pill, injection, liquid) but doesn't contain the active ingredient.
- Population: The entire group the researcher is studying. If the researcher cannot gather data from the population, studying large random samples taken from the population can be used to estimate how the population would respond.
- Power: The ability to observe differences or avoid making Type 2 errors.
- Random or Randomness : Selected or performed without following any pattern or method. To avoid unintentional bias, researchers often use random number generators or flip coins to make selections.
- Results: The explanation or interpretation of experimental data.
- Simple Experiment : A basic experiment designed to assess whether there is a cause and effect relationship or to test a prediction. A fundamental simple experiment might have only one test subject, compared with a controlled experiment , which has at least two groups.
- Single-Blind: When either the experimenter or subject is unaware whether the subject is getting the treatment or a placebo. Blinding the researcher helps prevent bias when the results are analyzed. Blinding the subject prevents the participant from having a biased reaction.
- Statistical Significance: Observation, based on the application of a statistical test, that a relationship probably is not due to pure chance. The probability is stated (e.g., p < 0.05) and the results are said to be statistically significant.
- T-Test: Common statistical data analysis applied to experimental data to test a hypothesis. The t -test computes the ratio between the difference between the group means and the standard error of the difference, a measure of the likelihood the group means could differ purely by chance. A rule of thumb is that the results are statistically significant if you observe a difference between the values that is three times larger than the standard error of the difference, but it's best to look up the ratio required for significance on a t-table .
- Type I Error (Type 1 Error): Occurs when you reject the null hypothesis, but it was actually true. If you perform the t -test and set p < 0.05, there is less than a 5% chance you could make a Type I error by rejecting the hypothesis based on random fluctuations in the data.
- Type II Error (Type 2 Error): Occurs when you accept the null hypothesis, but it was actually false. The experimental conditions had an effect, but the researcher failed to find it statistically significant.
- Null Hypothesis Examples
- Difference Between Independent and Dependent Variables
- Examples of Independent and Dependent Variables
- What Is a Double Blind Experiment?
- Example of a Permutation Test
- The Difference Between Type I and Type II Errors in Hypothesis Testing
- What Is a Hypothesis? (Science)
- Understanding Simple vs Controlled Experiments
- Six Steps of the Scientific Method
- The Difference Between Control Group and Experimental Group
- Null Hypothesis Definition and Examples
- What Is the Difference Between Alpha and P-Values?
- What Are the Elements of a Good Hypothesis?
- What Is an Experiment? Definition and Design
- What Is a P-Value?
- What are Controlled Experiments?
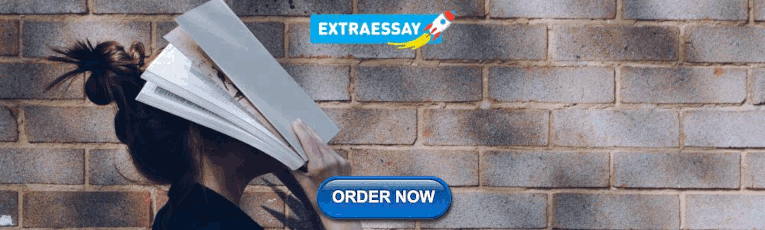
IMAGES
VIDEO
COMMENTS
Published on April 19, 2021 by Pritha Bhandari . Revised on June 22, 2023. In experiments, researchers manipulate independent variables to test their effects on dependent variables. In a controlled experiment, all variables other than the independent variable are controlled or held constant so they don't influence the dependent variable.
Search. A controlled experiment aims to demonstrate causation between variables by manipulating an independent variable while controlling all other factors that could influence the results. Its purpose is to show that changes in one variable (the independent variable) directly cause changes in another variable (the dependent variable).
There are two groups in the experiment, and they are identical except that one receives a treatment (water) while the other does not. The group that receives the treatment in an experiment (here, the watered pot) is called the experimental group, while the group that does not receive the treatment (here, the dry pot) is called the control group.The control group provides a baseline that lets ...
Controlled Experiment Definition. A controlled experiment is a scientific test that is directly manipulated by a scientist, in order to test a single variable at a time. The variable being tested is the independent variable, and is adjusted to see the effects on the system being studied. The controlled variables are held constant to minimize or ...
Controlled Experiment. A controlled experiment is simply an experiment in which all factors are held constant except for one: the independent variable. A common type of controlled experiment compares a control group against an experimental group. All variables are identical between the two groups except for the factor being tested.
A controlled experiment is a research study in which participants are randomly assigned to experimental and control groups. A controlled experiment allows researchers to determine cause and effect between variables. One drawback of controlled experiments is that they lack external validity (which means their results may not generalize to real ...
Control in experiments is critical for internal validity, which allows you to establish a cause-and-effect relationship between variables. Example: Experiment. You're studying the effects of colours in advertising. You want to test whether using green for advertising fast food chains increases the value of their products.
The steps are: Ask a question. Research the topic. Develop a hypothesis. Test with an experiment. Analyze data. Report conclusions. Developing a controlled experiment is one way to test a ...
Controlled Experiment . Controlled experiments have two groups of subjects. One group is the experimental group and it is exposed to your test. The other group is the control group, which is not exposed to the test.There are several methods of conducting a controlled experiment, but a simple controlled experiment is the most common. The simple controlled experiment has just the two groups: one ...
A controlled experiment is a comprehensive methodology employed in scientific research to reduce the effect of variables other than the independent variable. It involves segregating the subjects into two or more groups, including the control group that does not undergo any modifications in the independent variables, and the test group(s) that ...
A controlled experiment tries to form a link between the cause and the effect. If we are to study the effect of fertilizers on plant growth, the cause will be the 'fertilizer' and its effect would be the 'growth of the plant'. In other words:
Controlled Experiment Examples. 1. A good example of a controlled group would be an experiment to test the effects of a drug. The sample population would be divided into two, the group receiving the drug would be the experimental group while the group receiving the placebo would be the control group (Note that all the variables such as age, and ...
Actually, the term is "Sample Survey" and you may search online for it. I think the difference lies in the aim of the three types of studies, sample surveys want to get data for a parameter while observational studies and experiments want to convert some data into information, i.e., correlation and causation respectively.
A true experiment (a.k.a. a controlled experiment) always includes at least one control group that doesn't receive the experimental treatment. However, some experiments use a within-subjects design to test treatments without a control group. In these designs, you usually compare one group's outcomes before and after a treatment (instead of ...
1. Lab Experiment. A laboratory experiment in psychology is a research method in which the experimenter manipulates one or more independent variables and measures the effects on the dependent variable under controlled conditions. A laboratory experiment is conducted under highly controlled conditions (not necessarily a laboratory) where ...
The meaning of CONTROLLED EXPERIMENT is an experiment in which all the variable factors in an experimental group and a comparison control group are kept the same except for one variable factor in the experimental group that is changed or altered. How to use controlled experiment in a sentence.
For this reason, it's also known as a controlled variable or a constant variable. A single experiment may contain many control variables. Unlike the independent and dependent variables, control variables aren't a part of the experiment, but they are important because they could affect the outcome. Take a look at the difference between a ...
A scientific control is an experiment or observation designed to minimize the effects of variables other than the independent variable (i.e. confounding variables ). [1] This increases the reliability of the results, often through a comparison between control measurements and the other measurements. Scientific controls are a part of the ...
A control group is not the same thing as a control variable. A control variable or controlled variable is any factor that is held constant during an experiment. Examples of common control variables include temperature, duration, and sample size. The control variables are the same for both the control and experimental groups.
Controlled experiment definition: an experiment or trial that uses controls, usually separating the subjects into one or more control groups and experimental groups.. See examples of CONTROLLED EXPERIMENT used in a sentence.
The meaning of CONTROL EXPERIMENT is an experiment in which all variable factors have been kept constant and which is used as a standard of comparison to the experimental component in a controlled experiment.
Here is a glossary of important science experiment terms and definitions: Central Limit Theorem: States that with a large enough sample, the sample mean will be normally distributed. A normally distributed sample mean is necessary to apply the t- test, so if you are planning to perform a statistical analysis of experimental data, it's important ...
Controlled variables are quantities that a scientist wants to remain constant. If they were altered, it would greatly affect the experiment's results. Most experiments have more than one controlled variable. For example, if you are testing a new cold medicine, the controlled variable might be that the patient has a cold and a fever.
Over 30,000 field experiments with The Washington Post and Upworthy showed that readers prefer simpler headlines (e.g., more common words and more readable writing) over more complex ones. A follow-up mechanism experiment showed that readers from the general public paid more attention to, and processed more deeply, the simpler headlines compared to the complex headlines.