Have a language expert improve your writing
Run a free plagiarism check in 10 minutes, generate accurate citations for free.
- Knowledge Base
Methodology
- Random vs. Systematic Error | Definition & Examples
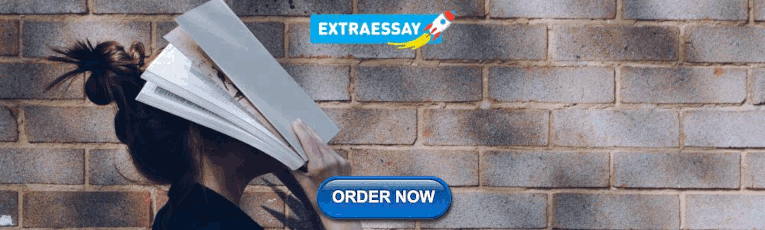
Random vs. Systematic Error | Definition & Examples
Published on May 7, 2021 by Pritha Bhandari . Revised on June 22, 2023.
In scientific research, measurement error is the difference between an observed value and the true value of something. It’s also called observation error or experimental error.
There are two main types of measurement error:
Random error is a chance difference between the observed and true values of something (e.g., a researcher misreading a weighing scale records an incorrect measurement).
- Systematic error is a consistent or proportional difference between the observed and true values of something (e.g., a miscalibrated scale consistently registers weights as higher than they actually are).
By recognizing the sources of error, you can reduce their impacts and record accurate and precise measurements. Gone unnoticed, these errors can lead to research biases like omitted variable bias or information bias .
Table of contents
Are random or systematic errors worse, random error, reducing random error, systematic error, reducing systematic error, other interesting articles, frequently asked questions about random and systematic error.
In research, systematic errors are generally a bigger problem than random errors.
Random error isn’t necessarily a mistake, but rather a natural part of measurement. There is always some variability in measurements, even when you measure the same thing repeatedly, because of fluctuations in the environment, the instrument, or your own interpretations.
But variability can be a problem when it affects your ability to draw valid conclusions about relationships between variables . This is more likely to occur as a result of systematic error.
Precision vs accuracy
Random error mainly affects precision , which is how reproducible the same measurement is under equivalent circumstances. In contrast, systematic error affects the accuracy of a measurement, or how close the observed value is to the true value.
Taking measurements is similar to hitting a central target on a dartboard. For accurate measurements, you aim to get your dart (your observations) as close to the target (the true values) as you possibly can. For precise measurements, you aim to get repeated observations as close to each other as possible.
Random error introduces variability between different measurements of the same thing, while systematic error skews your measurement away from the true value in a specific direction.
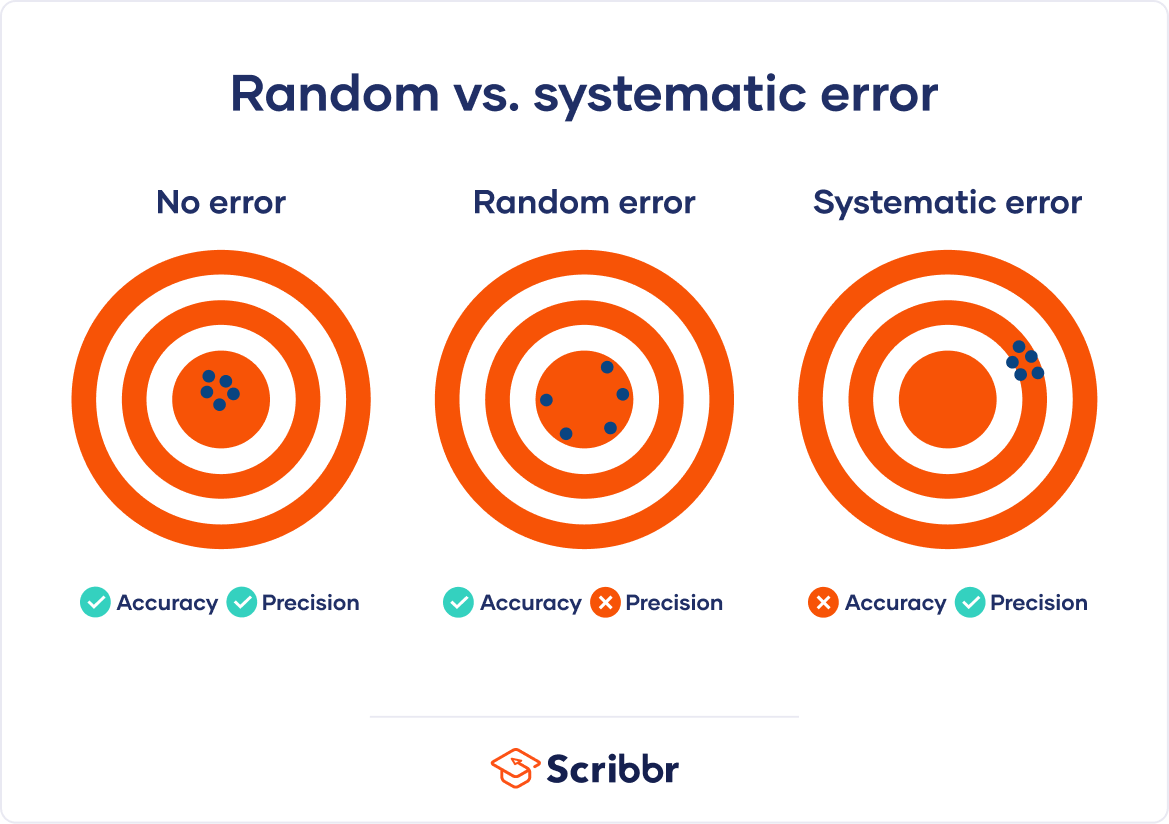
When you only have random error, if you measure the same thing multiple times, your measurements will tend to cluster or vary around the true value. Some values will be higher than the true score, while others will be lower. When you average out these measurements, you’ll get very close to the true score.
For this reason, random error isn’t considered a big problem when you’re collecting data from a large sample—the errors in different directions will cancel each other out when you calculate descriptive statistics . But it could affect the precision of your dataset when you have a small sample.
Systematic errors are much more problematic than random errors because they can skew your data to lead you to false conclusions. If you have systematic error, your measurements will be biased away from the true values. Ultimately, you might make a false positive or a false negative conclusion (a Type I or II error ) about the relationship between the variables you’re studying.
Prevent plagiarism. Run a free check.
Random error affects your measurements in unpredictable ways: your measurements are equally likely to be higher or lower than the true values.
In the graph below, the black line represents a perfect match between the true scores and observed scores of a scale. In an ideal world, all of your data would fall on exactly that line. The green dots represent the actual observed scores for each measurement with random error added.
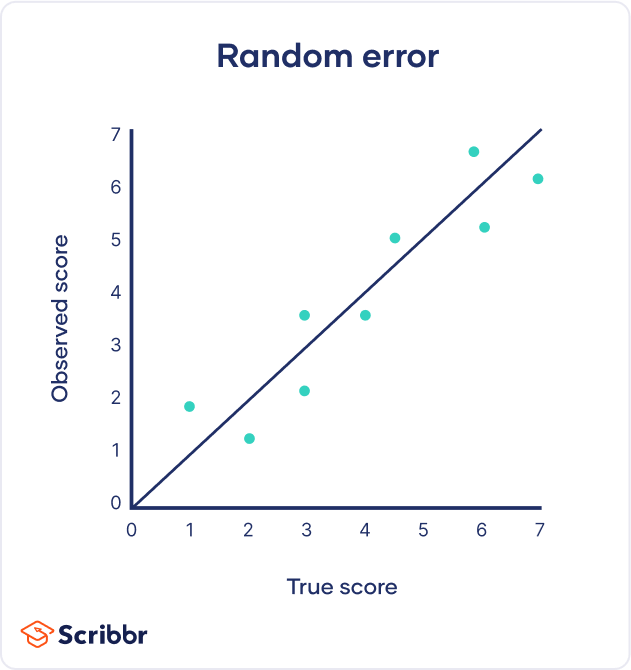
Random error is referred to as “noise”, because it blurs the true value (or the “signal”) of what’s being measured. Keeping random error low helps you collect precise data.
Sources of random errors
Some common sources of random error include:
- natural variations in real world or experimental contexts.
- imprecise or unreliable measurement instruments.
- individual differences between participants or units.
- poorly controlled experimental procedures.
Random error source | Example |
---|---|
Natural variations in context | In an about memory capacity, your participants are scheduled for memory tests at different times of day. However, some participants tend to perform better in the morning while others perform better later in the day, so your measurements do not reflect the true extent of memory capacity for each individual. |
Imprecise instrument | You measure wrist circumference using a tape measure. But your tape measure is only accurate to the nearest half-centimeter, so you round each measurement up or down when you record data. |
Individual differences | You ask participants to administer a safe electric shock to themselves and rate their pain level on a 7-point rating scale. Because pain is subjective, it’s hard to reliably measure. Some participants overstate their levels of pain, while others understate their levels of pain. |
Random error is almost always present in research, even in highly controlled settings. While you can’t eradicate it completely, you can reduce random error using the following methods.
Take repeated measurements
A simple way to increase precision is by taking repeated measurements and using their average. For example, you might measure the wrist circumference of a participant three times and get slightly different lengths each time. Taking the mean of the three measurements, instead of using just one, brings you much closer to the true value.
Increase your sample size
Large samples have less random error than small samples. That’s because the errors in different directions cancel each other out more efficiently when you have more data points. Collecting data from a large sample increases precision and statistical power .
Control variables
In controlled experiments , you should carefully control any extraneous variables that could impact your measurements. These should be controlled for all participants so that you remove key sources of random error across the board.
Systematic error means that your measurements of the same thing will vary in predictable ways: every measurement will differ from the true measurement in the same direction, and even by the same amount in some cases.
Systematic error is also referred to as bias because your data is skewed in standardized ways that hide the true values. This may lead to inaccurate conclusions.
Types of systematic errors
Offset errors and scale factor errors are two quantifiable types of systematic error.
An offset error occurs when a scale isn’t calibrated to a correct zero point. It’s also called an additive error or a zero-setting error.
A scale factor error is when measurements consistently differ from the true value proportionally (e.g., by 10%). It’s also referred to as a correlational systematic error or a multiplier error.
You can plot offset errors and scale factor errors in graphs to identify their differences. In the graphs below, the black line shows when your observed value is the exact true value, and there is no random error.
The blue line is an offset error: it shifts all of your observed values upwards or downwards by a fixed amount (here, it’s one additional unit).
The purple line is a scale factor error: all of your observed values are multiplied by a factor—all values are shifted in the same direction by the same proportion, but by different absolute amounts.
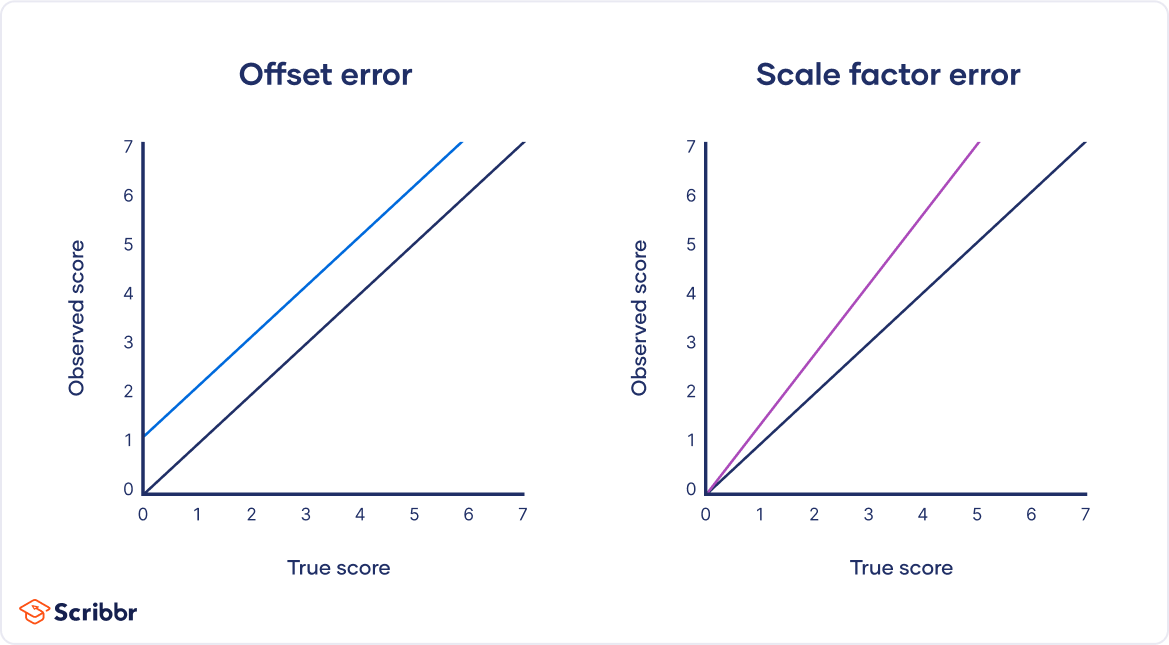
Sources of systematic errors
The sources of systematic error can range from your research materials to your data collection procedures and to your analysis techniques. This isn’t an exhaustive list of systematic error sources, because they can come from all aspects of research.
Response bias occurs when your research materials (e.g., questionnaires ) prompt participants to answer or act in inauthentic ways through leading questions . For example, social desirability bias can lead participants try to conform to societal norms, even if that’s not how they truly feel.
Your question states: “Experts believe that only systematic actions can reduce the effects of climate change. Do you agree that individual actions are pointless?”
Experimenter drift occurs when observers become fatigued, bored, or less motivated after long periods of data collection or coding, and they slowly depart from using standardized procedures in identifiable ways.
Initially, you code all subtle and obvious behaviors that fit your criteria as cooperative. But after spending days on this task, you only code extremely obviously helpful actions as cooperative.
Sampling bias occurs when some members of a population are more likely to be included in your study than others. It reduces the generalizability of your findings, because your sample isn’t representative of the whole population.
Receive feedback on language, structure, and formatting
Professional editors proofread and edit your paper by focusing on:
- Academic style
- Vague sentences
- Style consistency
See an example
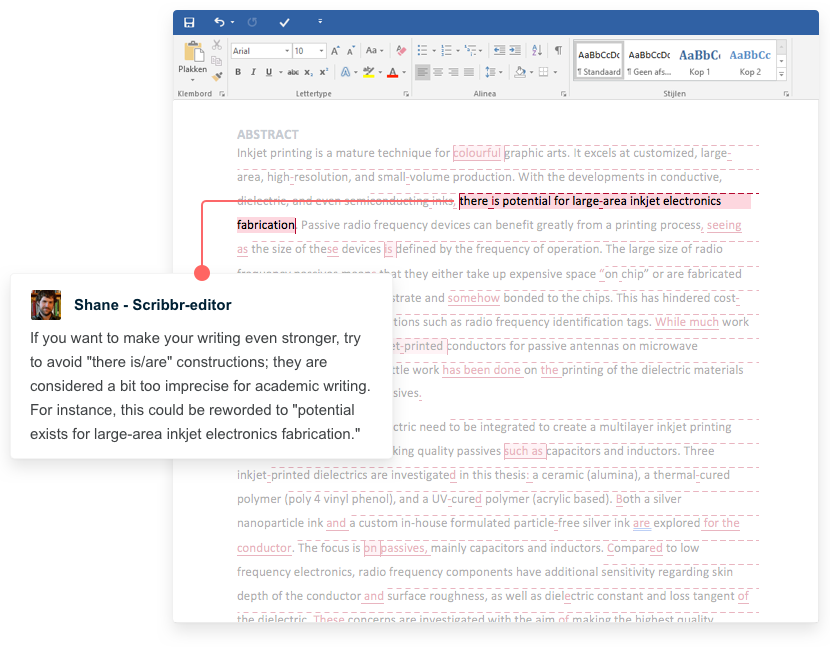
You can reduce systematic errors by implementing these methods in your study.
Triangulation
Triangulation means using multiple techniques to record observations so that you’re not relying on only one instrument or method.
For example, if you’re measuring stress levels, you can use survey responses, physiological recordings, and reaction times as indicators. You can check whether all three of these measurements converge or overlap to make sure that your results don’t depend on the exact instrument used.
Regular calibration
Calibrating an instrument means comparing what the instrument records with the true value of a known, standard quantity. Regularly calibrating your instrument with an accurate reference helps reduce the likelihood of systematic errors affecting your study.
You can also calibrate observers or researchers in terms of how they code or record data. Use standard protocols and routine checks to avoid experimenter drift.
Randomization
Probability sampling methods help ensure that your sample doesn’t systematically differ from the population.
In addition, if you’re doing an experiment, use random assignment to place participants into different treatment conditions. This helps counter bias by balancing participant characteristics across groups.
Wherever possible, you should hide the condition assignment from participants and researchers through masking (blinding) .
Participants’ behaviors or responses can be influenced by experimenter expectancies and demand characteristics in the environment, so controlling these will help you reduce systematic bias.
If you want to know more about statistics , methodology , or research bias , make sure to check out some of our other articles with explanations and examples.
- Normal distribution
- Degrees of freedom
- Null hypothesis
- Discourse analysis
- Control groups
- Mixed methods research
- Non-probability sampling
- Quantitative research
- Ecological validity
Research bias
- Rosenthal effect
- Implicit bias
- Cognitive bias
- Selection bias
- Negativity bias
- Status quo bias
Random and systematic error are two types of measurement error.
Systematic error is a consistent or proportional difference between the observed and true values of something (e.g., a miscalibrated scale consistently records weights as higher than they actually are).
Systematic error is generally a bigger problem in research.
With random error, multiple measurements will tend to cluster around the true value. When you’re collecting data from a large sample , the errors in different directions will cancel each other out.
Systematic errors are much more problematic because they can skew your data away from the true value. This can lead you to false conclusions ( Type I and II errors ) about the relationship between the variables you’re studying.
Random error is almost always present in scientific studies, even in highly controlled settings. While you can’t eradicate it completely, you can reduce random error by taking repeated measurements, using a large sample, and controlling extraneous variables .
You can avoid systematic error through careful design of your sampling , data collection , and analysis procedures. For example, use triangulation to measure your variables using multiple methods; regularly calibrate instruments or procedures; use random sampling and random assignment ; and apply masking (blinding) where possible.
Cite this Scribbr article
If you want to cite this source, you can copy and paste the citation or click the “Cite this Scribbr article” button to automatically add the citation to our free Citation Generator.
Bhandari, P. (2023, June 22). Random vs. Systematic Error | Definition & Examples. Scribbr. Retrieved September 4, 2024, from https://www.scribbr.com/methodology/random-vs-systematic-error/
Is this article helpful?
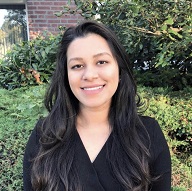
Pritha Bhandari
Other students also liked, reliability vs. validity in research | difference, types and examples, what is a controlled experiment | definitions & examples, extraneous variables | examples, types & controls, what is your plagiarism score.

An official website of the United States government
The .gov means it’s official. Federal government websites often end in .gov or .mil. Before sharing sensitive information, make sure you’re on a federal government site.
The site is secure. The https:// ensures that you are connecting to the official website and that any information you provide is encrypted and transmitted securely.
- Publications
- Account settings
Preview improvements coming to the PMC website in October 2024. Learn More or Try it out now .
- Advanced Search
- Journal List
- BMC Med Res Methodol

A tutorial on methodological studies: the what, when, how and why
Lawrence mbuagbaw.
1 Department of Health Research Methods, Evidence and Impact, McMaster University, Hamilton, ON Canada
2 Biostatistics Unit/FSORC, 50 Charlton Avenue East, St Joseph’s Healthcare—Hamilton, 3rd Floor Martha Wing, Room H321, Hamilton, Ontario L8N 4A6 Canada
3 Centre for the Development of Best Practices in Health, Yaoundé, Cameroon
Daeria O. Lawson
Livia puljak.
4 Center for Evidence-Based Medicine and Health Care, Catholic University of Croatia, Ilica 242, 10000 Zagreb, Croatia
David B. Allison
5 Department of Epidemiology and Biostatistics, School of Public Health – Bloomington, Indiana University, Bloomington, IN 47405 USA
Lehana Thabane
6 Departments of Paediatrics and Anaesthesia, McMaster University, Hamilton, ON Canada
7 Centre for Evaluation of Medicine, St. Joseph’s Healthcare-Hamilton, Hamilton, ON Canada
8 Population Health Research Institute, Hamilton Health Sciences, Hamilton, ON Canada
Associated Data
Data sharing is not applicable to this article as no new data were created or analyzed in this study.
Methodological studies – studies that evaluate the design, analysis or reporting of other research-related reports – play an important role in health research. They help to highlight issues in the conduct of research with the aim of improving health research methodology, and ultimately reducing research waste.
We provide an overview of some of the key aspects of methodological studies such as what they are, and when, how and why they are done. We adopt a “frequently asked questions” format to facilitate reading this paper and provide multiple examples to help guide researchers interested in conducting methodological studies. Some of the topics addressed include: is it necessary to publish a study protocol? How to select relevant research reports and databases for a methodological study? What approaches to data extraction and statistical analysis should be considered when conducting a methodological study? What are potential threats to validity and is there a way to appraise the quality of methodological studies?
Appropriate reflection and application of basic principles of epidemiology and biostatistics are required in the design and analysis of methodological studies. This paper provides an introduction for further discussion about the conduct of methodological studies.
The field of meta-research (or research-on-research) has proliferated in recent years in response to issues with research quality and conduct [ 1 – 3 ]. As the name suggests, this field targets issues with research design, conduct, analysis and reporting. Various types of research reports are often examined as the unit of analysis in these studies (e.g. abstracts, full manuscripts, trial registry entries). Like many other novel fields of research, meta-research has seen a proliferation of use before the development of reporting guidance. For example, this was the case with randomized trials for which risk of bias tools and reporting guidelines were only developed much later – after many trials had been published and noted to have limitations [ 4 , 5 ]; and for systematic reviews as well [ 6 – 8 ]. However, in the absence of formal guidance, studies that report on research differ substantially in how they are named, conducted and reported [ 9 , 10 ]. This creates challenges in identifying, summarizing and comparing them. In this tutorial paper, we will use the term methodological study to refer to any study that reports on the design, conduct, analysis or reporting of primary or secondary research-related reports (such as trial registry entries and conference abstracts).
In the past 10 years, there has been an increase in the use of terms related to methodological studies (based on records retrieved with a keyword search [in the title and abstract] for “methodological review” and “meta-epidemiological study” in PubMed up to December 2019), suggesting that these studies may be appearing more frequently in the literature. See Fig. 1 .

Trends in the number studies that mention “methodological review” or “meta-
epidemiological study” in PubMed.
The methods used in many methodological studies have been borrowed from systematic and scoping reviews. This practice has influenced the direction of the field, with many methodological studies including searches of electronic databases, screening of records, duplicate data extraction and assessments of risk of bias in the included studies. However, the research questions posed in methodological studies do not always require the approaches listed above, and guidance is needed on when and how to apply these methods to a methodological study. Even though methodological studies can be conducted on qualitative or mixed methods research, this paper focuses on and draws examples exclusively from quantitative research.
The objectives of this paper are to provide some insights on how to conduct methodological studies so that there is greater consistency between the research questions posed, and the design, analysis and reporting of findings. We provide multiple examples to illustrate concepts and a proposed framework for categorizing methodological studies in quantitative research.
What is a methodological study?
Any study that describes or analyzes methods (design, conduct, analysis or reporting) in published (or unpublished) literature is a methodological study. Consequently, the scope of methodological studies is quite extensive and includes, but is not limited to, topics as diverse as: research question formulation [ 11 ]; adherence to reporting guidelines [ 12 – 14 ] and consistency in reporting [ 15 ]; approaches to study analysis [ 16 ]; investigating the credibility of analyses [ 17 ]; and studies that synthesize these methodological studies [ 18 ]. While the nomenclature of methodological studies is not uniform, the intents and purposes of these studies remain fairly consistent – to describe or analyze methods in primary or secondary studies. As such, methodological studies may also be classified as a subtype of observational studies.
Parallel to this are experimental studies that compare different methods. Even though they play an important role in informing optimal research methods, experimental methodological studies are beyond the scope of this paper. Examples of such studies include the randomized trials by Buscemi et al., comparing single data extraction to double data extraction [ 19 ], and Carrasco-Labra et al., comparing approaches to presenting findings in Grading of Recommendations, Assessment, Development and Evaluations (GRADE) summary of findings tables [ 20 ]. In these studies, the unit of analysis is the person or groups of individuals applying the methods. We also direct readers to the Studies Within a Trial (SWAT) and Studies Within a Review (SWAR) programme operated through the Hub for Trials Methodology Research, for further reading as a potential useful resource for these types of experimental studies [ 21 ]. Lastly, this paper is not meant to inform the conduct of research using computational simulation and mathematical modeling for which some guidance already exists [ 22 ], or studies on the development of methods using consensus-based approaches.
When should we conduct a methodological study?
Methodological studies occupy a unique niche in health research that allows them to inform methodological advances. Methodological studies should also be conducted as pre-cursors to reporting guideline development, as they provide an opportunity to understand current practices, and help to identify the need for guidance and gaps in methodological or reporting quality. For example, the development of the popular Preferred Reporting Items of Systematic reviews and Meta-Analyses (PRISMA) guidelines were preceded by methodological studies identifying poor reporting practices [ 23 , 24 ]. In these instances, after the reporting guidelines are published, methodological studies can also be used to monitor uptake of the guidelines.
These studies can also be conducted to inform the state of the art for design, analysis and reporting practices across different types of health research fields, with the aim of improving research practices, and preventing or reducing research waste. For example, Samaan et al. conducted a scoping review of adherence to different reporting guidelines in health care literature [ 18 ]. Methodological studies can also be used to determine the factors associated with reporting practices. For example, Abbade et al. investigated journal characteristics associated with the use of the Participants, Intervention, Comparison, Outcome, Timeframe (PICOT) format in framing research questions in trials of venous ulcer disease [ 11 ].
How often are methodological studies conducted?
There is no clear answer to this question. Based on a search of PubMed, the use of related terms (“methodological review” and “meta-epidemiological study”) – and therefore, the number of methodological studies – is on the rise. However, many other terms are used to describe methodological studies. There are also many studies that explore design, conduct, analysis or reporting of research reports, but that do not use any specific terms to describe or label their study design in terms of “methodology”. This diversity in nomenclature makes a census of methodological studies elusive. Appropriate terminology and key words for methodological studies are needed to facilitate improved accessibility for end-users.
Why do we conduct methodological studies?
Methodological studies provide information on the design, conduct, analysis or reporting of primary and secondary research and can be used to appraise quality, quantity, completeness, accuracy and consistency of health research. These issues can be explored in specific fields, journals, databases, geographical regions and time periods. For example, Areia et al. explored the quality of reporting of endoscopic diagnostic studies in gastroenterology [ 25 ]; Knol et al. investigated the reporting of p -values in baseline tables in randomized trial published in high impact journals [ 26 ]; Chen et al. describe adherence to the Consolidated Standards of Reporting Trials (CONSORT) statement in Chinese Journals [ 27 ]; and Hopewell et al. describe the effect of editors’ implementation of CONSORT guidelines on reporting of abstracts over time [ 28 ]. Methodological studies provide useful information to researchers, clinicians, editors, publishers and users of health literature. As a result, these studies have been at the cornerstone of important methodological developments in the past two decades and have informed the development of many health research guidelines including the highly cited CONSORT statement [ 5 ].
Where can we find methodological studies?
Methodological studies can be found in most common biomedical bibliographic databases (e.g. Embase, MEDLINE, PubMed, Web of Science). However, the biggest caveat is that methodological studies are hard to identify in the literature due to the wide variety of names used and the lack of comprehensive databases dedicated to them. A handful can be found in the Cochrane Library as “Cochrane Methodology Reviews”, but these studies only cover methodological issues related to systematic reviews. Previous attempts to catalogue all empirical studies of methods used in reviews were abandoned 10 years ago [ 29 ]. In other databases, a variety of search terms may be applied with different levels of sensitivity and specificity.
Some frequently asked questions about methodological studies
In this section, we have outlined responses to questions that might help inform the conduct of methodological studies.
Q: How should I select research reports for my methodological study?
A: Selection of research reports for a methodological study depends on the research question and eligibility criteria. Once a clear research question is set and the nature of literature one desires to review is known, one can then begin the selection process. Selection may begin with a broad search, especially if the eligibility criteria are not apparent. For example, a methodological study of Cochrane Reviews of HIV would not require a complex search as all eligible studies can easily be retrieved from the Cochrane Library after checking a few boxes [ 30 ]. On the other hand, a methodological study of subgroup analyses in trials of gastrointestinal oncology would require a search to find such trials, and further screening to identify trials that conducted a subgroup analysis [ 31 ].
The strategies used for identifying participants in observational studies can apply here. One may use a systematic search to identify all eligible studies. If the number of eligible studies is unmanageable, a random sample of articles can be expected to provide comparable results if it is sufficiently large [ 32 ]. For example, Wilson et al. used a random sample of trials from the Cochrane Stroke Group’s Trial Register to investigate completeness of reporting [ 33 ]. It is possible that a simple random sample would lead to underrepresentation of units (i.e. research reports) that are smaller in number. This is relevant if the investigators wish to compare multiple groups but have too few units in one group. In this case a stratified sample would help to create equal groups. For example, in a methodological study comparing Cochrane and non-Cochrane reviews, Kahale et al. drew random samples from both groups [ 34 ]. Alternatively, systematic or purposeful sampling strategies can be used and we encourage researchers to justify their selected approaches based on the study objective.
Q: How many databases should I search?
A: The number of databases one should search would depend on the approach to sampling, which can include targeting the entire “population” of interest or a sample of that population. If you are interested in including the entire target population for your research question, or drawing a random or systematic sample from it, then a comprehensive and exhaustive search for relevant articles is required. In this case, we recommend using systematic approaches for searching electronic databases (i.e. at least 2 databases with a replicable and time stamped search strategy). The results of your search will constitute a sampling frame from which eligible studies can be drawn.
Alternatively, if your approach to sampling is purposeful, then we recommend targeting the database(s) or data sources (e.g. journals, registries) that include the information you need. For example, if you are conducting a methodological study of high impact journals in plastic surgery and they are all indexed in PubMed, you likely do not need to search any other databases. You may also have a comprehensive list of all journals of interest and can approach your search using the journal names in your database search (or by accessing the journal archives directly from the journal’s website). Even though one could also search journals’ web pages directly, using a database such as PubMed has multiple advantages, such as the use of filters, so the search can be narrowed down to a certain period, or study types of interest. Furthermore, individual journals’ web sites may have different search functionalities, which do not necessarily yield a consistent output.
Q: Should I publish a protocol for my methodological study?
A: A protocol is a description of intended research methods. Currently, only protocols for clinical trials require registration [ 35 ]. Protocols for systematic reviews are encouraged but no formal recommendation exists. The scientific community welcomes the publication of protocols because they help protect against selective outcome reporting, the use of post hoc methodologies to embellish results, and to help avoid duplication of efforts [ 36 ]. While the latter two risks exist in methodological research, the negative consequences may be substantially less than for clinical outcomes. In a sample of 31 methodological studies, 7 (22.6%) referenced a published protocol [ 9 ]. In the Cochrane Library, there are 15 protocols for methodological reviews (21 July 2020). This suggests that publishing protocols for methodological studies is not uncommon.
Authors can consider publishing their study protocol in a scholarly journal as a manuscript. Advantages of such publication include obtaining peer-review feedback about the planned study, and easy retrieval by searching databases such as PubMed. The disadvantages in trying to publish protocols includes delays associated with manuscript handling and peer review, as well as costs, as few journals publish study protocols, and those journals mostly charge article-processing fees [ 37 ]. Authors who would like to make their protocol publicly available without publishing it in scholarly journals, could deposit their study protocols in publicly available repositories, such as the Open Science Framework ( https://osf.io/ ).
Q: How to appraise the quality of a methodological study?
A: To date, there is no published tool for appraising the risk of bias in a methodological study, but in principle, a methodological study could be considered as a type of observational study. Therefore, during conduct or appraisal, care should be taken to avoid the biases common in observational studies [ 38 ]. These biases include selection bias, comparability of groups, and ascertainment of exposure or outcome. In other words, to generate a representative sample, a comprehensive reproducible search may be necessary to build a sampling frame. Additionally, random sampling may be necessary to ensure that all the included research reports have the same probability of being selected, and the screening and selection processes should be transparent and reproducible. To ensure that the groups compared are similar in all characteristics, matching, random sampling or stratified sampling can be used. Statistical adjustments for between-group differences can also be applied at the analysis stage. Finally, duplicate data extraction can reduce errors in assessment of exposures or outcomes.
Q: Should I justify a sample size?
A: In all instances where one is not using the target population (i.e. the group to which inferences from the research report are directed) [ 39 ], a sample size justification is good practice. The sample size justification may take the form of a description of what is expected to be achieved with the number of articles selected, or a formal sample size estimation that outlines the number of articles required to answer the research question with a certain precision and power. Sample size justifications in methodological studies are reasonable in the following instances:
- Comparing two groups
- Determining a proportion, mean or another quantifier
- Determining factors associated with an outcome using regression-based analyses
For example, El Dib et al. computed a sample size requirement for a methodological study of diagnostic strategies in randomized trials, based on a confidence interval approach [ 40 ].
Q: What should I call my study?
A: Other terms which have been used to describe/label methodological studies include “ methodological review ”, “methodological survey” , “meta-epidemiological study” , “systematic review” , “systematic survey”, “meta-research”, “research-on-research” and many others. We recommend that the study nomenclature be clear, unambiguous, informative and allow for appropriate indexing. Methodological study nomenclature that should be avoided includes “ systematic review” – as this will likely be confused with a systematic review of a clinical question. “ Systematic survey” may also lead to confusion about whether the survey was systematic (i.e. using a preplanned methodology) or a survey using “ systematic” sampling (i.e. a sampling approach using specific intervals to determine who is selected) [ 32 ]. Any of the above meanings of the words “ systematic” may be true for methodological studies and could be potentially misleading. “ Meta-epidemiological study” is ideal for indexing, but not very informative as it describes an entire field. The term “ review ” may point towards an appraisal or “review” of the design, conduct, analysis or reporting (or methodological components) of the targeted research reports, yet it has also been used to describe narrative reviews [ 41 , 42 ]. The term “ survey ” is also in line with the approaches used in many methodological studies [ 9 ], and would be indicative of the sampling procedures of this study design. However, in the absence of guidelines on nomenclature, the term “ methodological study ” is broad enough to capture most of the scenarios of such studies.
Q: Should I account for clustering in my methodological study?
A: Data from methodological studies are often clustered. For example, articles coming from a specific source may have different reporting standards (e.g. the Cochrane Library). Articles within the same journal may be similar due to editorial practices and policies, reporting requirements and endorsement of guidelines. There is emerging evidence that these are real concerns that should be accounted for in analyses [ 43 ]. Some cluster variables are described in the section: “ What variables are relevant to methodological studies?”
A variety of modelling approaches can be used to account for correlated data, including the use of marginal, fixed or mixed effects regression models with appropriate computation of standard errors [ 44 ]. For example, Kosa et al. used generalized estimation equations to account for correlation of articles within journals [ 15 ]. Not accounting for clustering could lead to incorrect p -values, unduly narrow confidence intervals, and biased estimates [ 45 ].
Q: Should I extract data in duplicate?
A: Yes. Duplicate data extraction takes more time but results in less errors [ 19 ]. Data extraction errors in turn affect the effect estimate [ 46 ], and therefore should be mitigated. Duplicate data extraction should be considered in the absence of other approaches to minimize extraction errors. However, much like systematic reviews, this area will likely see rapid new advances with machine learning and natural language processing technologies to support researchers with screening and data extraction [ 47 , 48 ]. However, experience plays an important role in the quality of extracted data and inexperienced extractors should be paired with experienced extractors [ 46 , 49 ].
Q: Should I assess the risk of bias of research reports included in my methodological study?
A : Risk of bias is most useful in determining the certainty that can be placed in the effect measure from a study. In methodological studies, risk of bias may not serve the purpose of determining the trustworthiness of results, as effect measures are often not the primary goal of methodological studies. Determining risk of bias in methodological studies is likely a practice borrowed from systematic review methodology, but whose intrinsic value is not obvious in methodological studies. When it is part of the research question, investigators often focus on one aspect of risk of bias. For example, Speich investigated how blinding was reported in surgical trials [ 50 ], and Abraha et al., investigated the application of intention-to-treat analyses in systematic reviews and trials [ 51 ].
Q: What variables are relevant to methodological studies?
A: There is empirical evidence that certain variables may inform the findings in a methodological study. We outline some of these and provide a brief overview below:
- Country: Countries and regions differ in their research cultures, and the resources available to conduct research. Therefore, it is reasonable to believe that there may be differences in methodological features across countries. Methodological studies have reported loco-regional differences in reporting quality [ 52 , 53 ]. This may also be related to challenges non-English speakers face in publishing papers in English.
- Authors’ expertise: The inclusion of authors with expertise in research methodology, biostatistics, and scientific writing is likely to influence the end-product. Oltean et al. found that among randomized trials in orthopaedic surgery, the use of analyses that accounted for clustering was more likely when specialists (e.g. statistician, epidemiologist or clinical trials methodologist) were included on the study team [ 54 ]. Fleming et al. found that including methodologists in the review team was associated with appropriate use of reporting guidelines [ 55 ].
- Source of funding and conflicts of interest: Some studies have found that funded studies report better [ 56 , 57 ], while others do not [ 53 , 58 ]. The presence of funding would indicate the availability of resources deployed to ensure optimal design, conduct, analysis and reporting. However, the source of funding may introduce conflicts of interest and warrant assessment. For example, Kaiser et al. investigated the effect of industry funding on obesity or nutrition randomized trials and found that reporting quality was similar [ 59 ]. Thomas et al. looked at reporting quality of long-term weight loss trials and found that industry funded studies were better [ 60 ]. Kan et al. examined the association between industry funding and “positive trials” (trials reporting a significant intervention effect) and found that industry funding was highly predictive of a positive trial [ 61 ]. This finding is similar to that of a recent Cochrane Methodology Review by Hansen et al. [ 62 ]
- Journal characteristics: Certain journals’ characteristics may influence the study design, analysis or reporting. Characteristics such as journal endorsement of guidelines [ 63 , 64 ], and Journal Impact Factor (JIF) have been shown to be associated with reporting [ 63 , 65 – 67 ].
- Study size (sample size/number of sites): Some studies have shown that reporting is better in larger studies [ 53 , 56 , 58 ].
- Year of publication: It is reasonable to assume that design, conduct, analysis and reporting of research will change over time. Many studies have demonstrated improvements in reporting over time or after the publication of reporting guidelines [ 68 , 69 ].
- Type of intervention: In a methodological study of reporting quality of weight loss intervention studies, Thabane et al. found that trials of pharmacologic interventions were reported better than trials of non-pharmacologic interventions [ 70 ].
- Interactions between variables: Complex interactions between the previously listed variables are possible. High income countries with more resources may be more likely to conduct larger studies and incorporate a variety of experts. Authors in certain countries may prefer certain journals, and journal endorsement of guidelines and editorial policies may change over time.
Q: Should I focus only on high impact journals?
A: Investigators may choose to investigate only high impact journals because they are more likely to influence practice and policy, or because they assume that methodological standards would be higher. However, the JIF may severely limit the scope of articles included and may skew the sample towards articles with positive findings. The generalizability and applicability of findings from a handful of journals must be examined carefully, especially since the JIF varies over time. Even among journals that are all “high impact”, variations exist in methodological standards.
Q: Can I conduct a methodological study of qualitative research?
A: Yes. Even though a lot of methodological research has been conducted in the quantitative research field, methodological studies of qualitative studies are feasible. Certain databases that catalogue qualitative research including the Cumulative Index to Nursing & Allied Health Literature (CINAHL) have defined subject headings that are specific to methodological research (e.g. “research methodology”). Alternatively, one could also conduct a qualitative methodological review; that is, use qualitative approaches to synthesize methodological issues in qualitative studies.
Q: What reporting guidelines should I use for my methodological study?
A: There is no guideline that covers the entire scope of methodological studies. One adaptation of the PRISMA guidelines has been published, which works well for studies that aim to use the entire target population of research reports [ 71 ]. However, it is not widely used (40 citations in 2 years as of 09 December 2019), and methodological studies that are designed as cross-sectional or before-after studies require a more fit-for purpose guideline. A more encompassing reporting guideline for a broad range of methodological studies is currently under development [ 72 ]. However, in the absence of formal guidance, the requirements for scientific reporting should be respected, and authors of methodological studies should focus on transparency and reproducibility.
Q: What are the potential threats to validity and how can I avoid them?
A: Methodological studies may be compromised by a lack of internal or external validity. The main threats to internal validity in methodological studies are selection and confounding bias. Investigators must ensure that the methods used to select articles does not make them differ systematically from the set of articles to which they would like to make inferences. For example, attempting to make extrapolations to all journals after analyzing high-impact journals would be misleading.
Many factors (confounders) may distort the association between the exposure and outcome if the included research reports differ with respect to these factors [ 73 ]. For example, when examining the association between source of funding and completeness of reporting, it may be necessary to account for journals that endorse the guidelines. Confounding bias can be addressed by restriction, matching and statistical adjustment [ 73 ]. Restriction appears to be the method of choice for many investigators who choose to include only high impact journals or articles in a specific field. For example, Knol et al. examined the reporting of p -values in baseline tables of high impact journals [ 26 ]. Matching is also sometimes used. In the methodological study of non-randomized interventional studies of elective ventral hernia repair, Parker et al. matched prospective studies with retrospective studies and compared reporting standards [ 74 ]. Some other methodological studies use statistical adjustments. For example, Zhang et al. used regression techniques to determine the factors associated with missing participant data in trials [ 16 ].
With regard to external validity, researchers interested in conducting methodological studies must consider how generalizable or applicable their findings are. This should tie in closely with the research question and should be explicit. For example. Findings from methodological studies on trials published in high impact cardiology journals cannot be assumed to be applicable to trials in other fields. However, investigators must ensure that their sample truly represents the target sample either by a) conducting a comprehensive and exhaustive search, or b) using an appropriate and justified, randomly selected sample of research reports.
Even applicability to high impact journals may vary based on the investigators’ definition, and over time. For example, for high impact journals in the field of general medicine, Bouwmeester et al. included the Annals of Internal Medicine (AIM), BMJ, the Journal of the American Medical Association (JAMA), Lancet, the New England Journal of Medicine (NEJM), and PLoS Medicine ( n = 6) [ 75 ]. In contrast, the high impact journals selected in the methodological study by Schiller et al. were BMJ, JAMA, Lancet, and NEJM ( n = 4) [ 76 ]. Another methodological study by Kosa et al. included AIM, BMJ, JAMA, Lancet and NEJM ( n = 5). In the methodological study by Thabut et al., journals with a JIF greater than 5 were considered to be high impact. Riado Minguez et al. used first quartile journals in the Journal Citation Reports (JCR) for a specific year to determine “high impact” [ 77 ]. Ultimately, the definition of high impact will be based on the number of journals the investigators are willing to include, the year of impact and the JIF cut-off [ 78 ]. We acknowledge that the term “generalizability” may apply differently for methodological studies, especially when in many instances it is possible to include the entire target population in the sample studied.
Finally, methodological studies are not exempt from information bias which may stem from discrepancies in the included research reports [ 79 ], errors in data extraction, or inappropriate interpretation of the information extracted. Likewise, publication bias may also be a concern in methodological studies, but such concepts have not yet been explored.
A proposed framework
In order to inform discussions about methodological studies, the development of guidance for what should be reported, we have outlined some key features of methodological studies that can be used to classify them. For each of the categories outlined below, we provide an example. In our experience, the choice of approach to completing a methodological study can be informed by asking the following four questions:
- What is the aim?
A methodological study may be focused on exploring sources of bias in primary or secondary studies (meta-bias), or how bias is analyzed. We have taken care to distinguish bias (i.e. systematic deviations from the truth irrespective of the source) from reporting quality or completeness (i.e. not adhering to a specific reporting guideline or norm). An example of where this distinction would be important is in the case of a randomized trial with no blinding. This study (depending on the nature of the intervention) would be at risk of performance bias. However, if the authors report that their study was not blinded, they would have reported adequately. In fact, some methodological studies attempt to capture both “quality of conduct” and “quality of reporting”, such as Richie et al., who reported on the risk of bias in randomized trials of pharmacy practice interventions [ 80 ]. Babic et al. investigated how risk of bias was used to inform sensitivity analyses in Cochrane reviews [ 81 ]. Further, biases related to choice of outcomes can also be explored. For example, Tan et al investigated differences in treatment effect size based on the outcome reported [ 82 ].
Methodological studies may report quality of reporting against a reporting checklist (i.e. adherence to guidelines) or against expected norms. For example, Croituro et al. report on the quality of reporting in systematic reviews published in dermatology journals based on their adherence to the PRISMA statement [ 83 ], and Khan et al. described the quality of reporting of harms in randomized controlled trials published in high impact cardiovascular journals based on the CONSORT extension for harms [ 84 ]. Other methodological studies investigate reporting of certain features of interest that may not be part of formally published checklists or guidelines. For example, Mbuagbaw et al. described how often the implications for research are elaborated using the Evidence, Participants, Intervention, Comparison, Outcome, Timeframe (EPICOT) format [ 30 ].
Sometimes investigators may be interested in how consistent reports of the same research are, as it is expected that there should be consistency between: conference abstracts and published manuscripts; manuscript abstracts and manuscript main text; and trial registration and published manuscript. For example, Rosmarakis et al. investigated consistency between conference abstracts and full text manuscripts [ 85 ].
In addition to identifying issues with reporting in primary and secondary studies, authors of methodological studies may be interested in determining the factors that are associated with certain reporting practices. Many methodological studies incorporate this, albeit as a secondary outcome. For example, Farrokhyar et al. investigated the factors associated with reporting quality in randomized trials of coronary artery bypass grafting surgery [ 53 ].
Methodological studies may also be used to describe methods or compare methods, and the factors associated with methods. Muller et al. described the methods used for systematic reviews and meta-analyses of observational studies [ 86 ].
Some methodological studies synthesize results from other methodological studies. For example, Li et al. conducted a scoping review of methodological reviews that investigated consistency between full text and abstracts in primary biomedical research [ 87 ].
Some methodological studies may investigate the use of names and terms in health research. For example, Martinic et al. investigated the definitions of systematic reviews used in overviews of systematic reviews (OSRs), meta-epidemiological studies and epidemiology textbooks [ 88 ].
In addition to the previously mentioned experimental methodological studies, there may exist other types of methodological studies not captured here.
- 2. What is the design?
Most methodological studies are purely descriptive and report their findings as counts (percent) and means (standard deviation) or medians (interquartile range). For example, Mbuagbaw et al. described the reporting of research recommendations in Cochrane HIV systematic reviews [ 30 ]. Gohari et al. described the quality of reporting of randomized trials in diabetes in Iran [ 12 ].
Some methodological studies are analytical wherein “analytical studies identify and quantify associations, test hypotheses, identify causes and determine whether an association exists between variables, such as between an exposure and a disease.” [ 89 ] In the case of methodological studies all these investigations are possible. For example, Kosa et al. investigated the association between agreement in primary outcome from trial registry to published manuscript and study covariates. They found that larger and more recent studies were more likely to have agreement [ 15 ]. Tricco et al. compared the conclusion statements from Cochrane and non-Cochrane systematic reviews with a meta-analysis of the primary outcome and found that non-Cochrane reviews were more likely to report positive findings. These results are a test of the null hypothesis that the proportions of Cochrane and non-Cochrane reviews that report positive results are equal [ 90 ].
- 3. What is the sampling strategy?
Methodological reviews with narrow research questions may be able to include the entire target population. For example, in the methodological study of Cochrane HIV systematic reviews, Mbuagbaw et al. included all of the available studies ( n = 103) [ 30 ].
Many methodological studies use random samples of the target population [ 33 , 91 , 92 ]. Alternatively, purposeful sampling may be used, limiting the sample to a subset of research-related reports published within a certain time period, or in journals with a certain ranking or on a topic. Systematic sampling can also be used when random sampling may be challenging to implement.
- 4. What is the unit of analysis?
Many methodological studies use a research report (e.g. full manuscript of study, abstract portion of the study) as the unit of analysis, and inferences can be made at the study-level. However, both published and unpublished research-related reports can be studied. These may include articles, conference abstracts, registry entries etc.
Some methodological studies report on items which may occur more than once per article. For example, Paquette et al. report on subgroup analyses in Cochrane reviews of atrial fibrillation in which 17 systematic reviews planned 56 subgroup analyses [ 93 ].
This framework is outlined in Fig. 2 .

A proposed framework for methodological studies
Conclusions
Methodological studies have examined different aspects of reporting such as quality, completeness, consistency and adherence to reporting guidelines. As such, many of the methodological study examples cited in this tutorial are related to reporting. However, as an evolving field, the scope of research questions that can be addressed by methodological studies is expected to increase.
In this paper we have outlined the scope and purpose of methodological studies, along with examples of instances in which various approaches have been used. In the absence of formal guidance on the design, conduct, analysis and reporting of methodological studies, we have provided some advice to help make methodological studies consistent. This advice is grounded in good contemporary scientific practice. Generally, the research question should tie in with the sampling approach and planned analysis. We have also highlighted the variables that may inform findings from methodological studies. Lastly, we have provided suggestions for ways in which authors can categorize their methodological studies to inform their design and analysis.
Acknowledgements
Abbreviations.
CONSORT | Consolidated Standards of Reporting Trials |
EPICOT | Evidence, Participants, Intervention, Comparison, Outcome, Timeframe |
GRADE | Grading of Recommendations, Assessment, Development and Evaluations |
PICOT | Participants, Intervention, Comparison, Outcome, Timeframe |
PRISMA | Preferred Reporting Items of Systematic reviews and Meta-Analyses |
SWAR | Studies Within a Review |
SWAT | Studies Within a Trial |
Authors’ contributions
LM conceived the idea and drafted the outline and paper. DOL and LT commented on the idea and draft outline. LM, LP and DOL performed literature searches and data extraction. All authors (LM, DOL, LT, LP, DBA) reviewed several draft versions of the manuscript and approved the final manuscript.
This work did not receive any dedicated funding.
Availability of data and materials
Ethics approval and consent to participate.
Not applicable.
Consent for publication
Competing interests.
DOL, DBA, LM, LP and LT are involved in the development of a reporting guideline for methodological studies.
Publisher’s Note
Springer Nature remains neutral with regard to jurisdictional claims in published maps and institutional affiliations.
Table of Contents
- 1.1. Overview
- 1.2. Accuracy vs Precision
- 1.3. Notation: Significant Figures and Roundoff
- 1.4. Types of Errors/Uncertainties
- 1.5. Estimating Measurement Errors
- 1.6.1. Independent Quantities
- 1.6.2. Dependent Quantities, Covariance
- 1.6.3. Examples
- 1.7. Mean, Least Squares
- 1.8. Least Square Fitting, Parameter Determination
Related Topics
- Previous: Modern Lab Experiments
- Next: 2. Collection of useful tools
Quick search
1. summary of error analysis and statistical methods ¶, 1.1. overview ¶.
There are two main types of experiments in physics:
One tries to determine some physical quantity with the highest precision possible e.g. the speed of light, the elementary charge, the gravitational constant etc. In other words the experiments goal is to determine some parameters.
The goal of the other type of experiment is to test whether a particular theory or theoretical model agrees with experiment. These type pf experiments test a hypothesis.
Very often these two experiment types have a large overlap. Most experiments carried out in intermediate lab is of the first type where you have to determine a physical quantity with the highest accuracy and precision possible. This quantity e.g. Planck’s constant is determined from various other directly quantities such as voltages, currents, distances etc. When you perform the same measurement repeatedly you will observe that you will get slightly different results from which you can determine an average value. We are assuming that the experimental conditions are completely reproducible in such a way that by repeating the same measurement an infinite number of times you would obtain the true value with infinite precision and accuracy. In reality this is not possible and you need to estimate the true value from the existing measurements, together with a good estimate of the range of values within which you expect the true value to lie. This last quantity you can call the experimental uncertainty or error or precision. How you obtain these best estimates from a finite number of measurements is the subject of statistics and error analysis.
There are many excellent books on statistics and error analysis in experimental physics (a classical book is Data Reduction and Error Analysis for the Physical Sciences 3rd Edition,by Philip Bevington and D. Keith Robinson , or An Introduction to Error Analysis: The Study of Uncertainties in Physical Measurements by John R. Taylor. Another more advanced book : Statistical Methods in Experimental Physics: 2nd Edition by Frederick James) which discuss measurement errors and uncertainties and how statistical methods can be used to obtain best estimates on quantities extracted from measurements. The following sections are basically a very short summary of the main results developed in these books. You should use these techniques to analyze your data to obtain reasonable estimates for your measurement values and their uncertainties.
1.2. Accuracy vs Precision ¶
There is a difference between what one means with accuracy and precision . The accuracy is a measure of how close the experimental value is to the true one. The precision determined how reproducible your experimental value is. It is often useful to determine the relative precision where the uncertainty of your result is expressed as a fraction of the value of the result. A good experiment needs to consider accuracy and precision simultaneously. An experiment can be very precisie but if e.g. the calibration of your instrument is wrong then your result will be inaccurate. While an imprecise experiment might be accurate but you are not going to learn anything new from it.
If you have an experimental result where you determined e.g. Planck’s constant, in the discussion of the results you should compare the accuracy of your result (how much it deviates from the currently accepted much more precise value) compared to the precision of your result. If the published value lies within your estimated uncertainty then you can be happy as your experiment agrees within its precision. If not, you need to try to understand what is going on, did you discover new physics or was there something else that lead to the results that contributed to the deviation or is your estimate of the precision of your result overly optimistic ?
1.3. Notation: Significant Figures and Roundoff ¶
The precision of an experimental value is indicated by the number of digits used to represent the results including the uncertainty of the result. Here are the rules regarding significant figures when you use scientific notation ( \(1234 = 1.234\cdot10^3\) )
The leftmost non-zero digit is the most significant one
The rightmost digit is the least significant one
All digits between the least and the most significant digits are counted as significant digits or significant figures.
When quoting an experimental result the number of significant figures should be approximately 1 more than that dictated by the experimental precision to avoid rounding errors in later calculations. E.g. you determined the elementary charge to be \(1.624\pm\ 10^{-19}\ C\) with an uncertainty of \(2.5\ 10^{-21}\ C\) then you would quote that result as \(Q = (1.624\ \pm 0.025)\cdot 10^{-19}\ C\) .
If you have a large uncertainty in the above example e.g. \(8.7\ 10^{-21}\ C\) the you would quote the result as \(Q = (1.62\ \pm 0.09)\cdot 10^{-19}\ C\) . You let the uncertainty define the precision to which you quote the result.
When your drop insignificant figures the last digit retained should be rounded according to the following rule (the computer can do this for you):
If the fraction is larger that 0.5 increment the new least significant digit.
If the fraction is less than 0.5 do not increment
If the fraction is equal 0.5 increment the new least significant digit only of it is odd.
Examples: 1.234 is rounded to 1.23, 1.236 is rounded to 1.24, 1.235 is rounded to 1.24 and 1.225 is rounded to 1.22
1.4. Types of Errors/Uncertainties ¶
There are two main type of errors which can have a variety of reasons:
Random errors: these are due to fluctuations of observations that lead to different results each time the experiment is repeated. They require repeated measurements in order to achieve a precision which corresponds to the desired accuracy of the experiment. Random errors can only be reduced by improving the experimental method and refining the experiment techniques employed. Random errors can be due to instrumental limitations which can be reduced by using better instruments or due to inherent limitation of the measurement technique. For example, if you count the number of random processes occurring during a time intervall, if the time intervall is too short and the total number of counts too small the relative precision will be limited, even though you might have the best instrument available.
Systematic Errors: this type of error is responsible for the deviation of even the most precise measurement from the tru value. These errors can be very difficult to determine and a large part of designing and analyzing an experiment is spent on reducing and determining its systematic error.
1.5. Estimating Measurement Errors ¶
There are two important indices of the uncertainty in a measured quantity, the maximum error and the standard error (note these names can be different in different books).
Maximum error: This is supposed to indicate the range within which the true value definitely lies. With a single measurement, it is really the only type of error that can be estimated.
Standard error: If you have a large number \(N\) of measurements of a single quantity, \(t\) , will produce a “normal” or “Gaussian” distribution curve as shown below. The ordinate, \(n(t)\) , is the number of observations per unit range of \(t\) whose values lie between \(t\) and \(t+dt\) . The equation for the curve is:

Fig. 1.1 Gaussian distribution curve. ¶
This curve is symmetrical about the mean value, \(\bar t\) . \(N\) is the total number of observations, and \(\sigma\) is a measure of the width of the curve and is called the standard deviation or standard error . For this distribution \(\sigma\) is the RMS value of the departures of the individual \(t\) measurements from the mean, \(\bar t\) . The square of the RMS value is also the so-called variance of \(t\) :
You can verify that 67% of all measurements of \(t\) lie within \(\bar t \pm \sigma\) , 95% within \(\bar t \pm 2\sigma\) and 99.7 % within \(\bar t \pm 3\sigma\) . In reality \(\bar t\) and \(\sigma\) are not known and need to be estimated from the data.
An important application is the estimate of the uncertainty of a counting experiment where the number of randomly occurring events are counted during a certain time intervall (e.g. particle emitted by a radioactive source):
Lets assume you have a single measurement with the result, \(N_{exp}\) counts. One assumes now that \(N_{exp}\) represents the mean for this measurement that you would obtain if you repeated this it many times, but which you actually do not do. This is of course a rather coarse approximation but the only one possible. In that case the estimate of the standard deviation for this measurement would be \(\sigma_{N} = \sqrt{N_{exp}}\) , a really important result which is widely applied .
1.6. Combination of Errors ¶
1.6.1. independent quantities ¶.
The discussion in this section is what you will use most of the time when you perform the error analysis of your data.
Usually in an experiment, the final result is derived from a number of measured, and usually independent quantities, \(x, y, z, ...\) , connected to the required quantity, F, by some mathematical formula. It is therefore necessary to determine how errors/uncertainties in the measured quantities affect the final result.
In general, if \(F = f(x, y, z, .......)\) , then we can estimate the maximal error by:
where \(\delta x\) is the maximal error of the quantity \(x\) .
We can determine the standard error \(\sigma_F\) using the following expression:
This assumes that all quantities \(x,y,z,...\) are independent of each other. Typically you will quote the standard error.
1.6.2. Dependent Quantities, Covariance ¶
The error analysis of data with measurements that are possibly correlated is a bit more complex than the previous examples and is illustrated using a simple example.
We will first consider a new quantity \(y_i = a x_{i1} + b x_{i2}\) which is linear combination of two experimental quantities \(x_{i1}, x_{i2}\) that are determined during measurement \(i\) of a total of \(N\) experiments, hence \(i = 1,2,3...,N\) . First we can calculate the mean:
Next we can calculate the variance of \(y\) :
where \(cov(x_1,x_2) = \frac{1}{N}\sum_{i=1}^N \left((x_{i1}- \bar x_1)(x_{i2} - \bar x_2)\right)\) is the covariance of \(x_1\) and \(x_2\) . It is a measure of the correlation between the two variables and can be positive, negative or zero. If \(x_1\) and \(x_2\) are completely independent of each other their covariance is 0. This is a simple example of a linear function of measured quantities which can be generalized to the general function \(F = f(x_1, x_2, x_3, ..., x_n)\) as follows:
1.6.3. Examples ¶
Here a a few examples of how to practically apply the above expressions. They are taken from the analysis of experiments that you are analyzing:
You have measured an angle \(\theta \pm \sigma_\theta\) e.g. in a spectroscope where \(\theta = \theta_{deg}, \theta_{min}\) is in degrees( \(\theta_{deg}\) ) and minutes( \(\theta_{min})\) and \(\sigma_\theta\) is in minutes. During your analysis you need to calculate the cosine of this angle and need to know the error in the cosine. How to you proceed?
Convert the degrees and minutes to radians: \(\theta_r = \frac{\pi}{180}\left(\theta_{deg} + \frac{\theta_{min}}{60}\right)\) , \(\sigma_{\theta_r} = \frac{\pi}{180}\left(\frac{\sigma_{\theta}}{60}\right)\)
Apply (1.3) where \(f\) is \(\cos{\theta}\) and x is \(\theta\) and no other variables.
The result should be \(\sigma_{\cos} = \sqrt{ \left( \frac{d\cos}{d\theta}\right) ^2 \sigma_r^2 } = \sin{\theta_r}\sigma_r\)
You encounter a problem where you need to estimate the uncertainty of a quantity that contains the square root of an experimentally determined count rate \(R = N/\Delta t\) where \(N\) is the number of counts observed in the time \(\Delta t\) . Assuming an uncertainty of \(\sigma_t\) for the time interval what is the uncertainty of \(\sqrt{R}\) ?
Calculate the uncertainty of \(N\) using the fact that it is the result of a counting experiment: \(\sigma_N = \sqrt{N}\) .
We need therefore to determine the uncertainty of the function \(f(N, {\Delta t}) = \sqrt{\frac{N}{\Delta t}}\)
This is a function of 2 variables ( \(N\) and \(\Delta t\) ) so you need to calculate the corresponding partial derivatives according to equation (1.3) as follows:
\(\frac{df}{dN} = \frac{1}{2\sqrt{N \Delta t}}\) and \(\frac{df}{d\Delta t} = \frac{-1\sqrt{N}}{2\sqrt{\Delta t^3}}\)
Combining the derivatives and uncertainties you get:
\(\sigma_f = \sqrt{\left(\frac{1}{2\sqrt{N \Delta t}} \right)^2 N + \left(\frac{-1\sqrt{N}}{2\sqrt{\Delta t^3}}\right)^2 \sigma_t^2}\)
This a bit more complex but basically the same procedure as before.
This example shows how to use the covariance matrix that is returned by every fit. This matrix contains information on the correlation between fit parameters. The simple error propagation (1.3) is only valid if the quantities \(x, y, z\) are independent from each other. When these quantities are fit parameters, e.g. the slope and offset of a line, or the parameters of a polynomial, they are most likely not independent. In this case you need to take into account their correlation as described in (1.8) .
As an example we try to determine the location of a peak and its uncertainty:
Start by fitting the data points around the the top of the peak with a parabola (in this way we approximate the top part of the peak with a parabola) \(y = a_0 + a_1 x + a_2 x^2\)
Determine the location of the maximum \(x_m\) by solving: \(\left. \frac{dy}{dx}\right|_{x_m} = 0\) , resulting in \(x_m = -\frac{a_1}{2a_2}\) . The determined peak position is now a function of 2 variables \(x_m(a_1, a_2)\) .
These fit parameters also have their own errors \(\sigma_{a_1}, \sigma_{a_2}\) which are NOT independent and we need to use equation (1.8) :
First we calculate the two partial derivatives \(\frac{\partial x_m}{\partial a_1} = \frac{1}{2 a_2}\) and \(\frac{\partial x_m}{\partial a_2} = -\frac{a_1}{2 a_2^2}\) . Since \(x_m\) is independent of \(a_0\) all its partial derivatives are 0.
Using eq. (1.8) we get therefore:
\(\sigma_{x_m}^2 = C_{11}\left(\frac{\partial x_m}{\partial a_1}\right) ^2 + 2C_{12}\left(\frac{\partial x_m}{\partial a_1}\right)\left(\frac{\partial x_m}{\partial a_2}\right) + C_{22}\left(\frac{\partial x_m}{\partial a_2}\right) ^2\)
Where the \(C_{ij}\) are the covariance matrix elements : cov[i,j] . Inserting the expressions for the derivatives results in:
\(\sigma_{x_m}^2 = C_{11}\left(\frac{1}{2 a_2}\right) ^2 - 2C_{12}\left(\frac{1}{2 a_2}\right)\left(\frac{a_1}{2 a_2^2}\right) + C_{22}\left(\frac{a_1}{2 a_2^2}\right) ^2\)
This is a similar expression as eq. (1.3) since \(C_{ii} = \sigma_{a_i}^2\) but with an additional term that can be negative. The final correlated error can therefore be smaller than the one obtained from (1.3) for quantities that are correlated.
1.7. Mean, Least Squares ¶
Assume that you have measured a set of \(N\) data points of the same quantity \(x\) . These represent a finite, random selection from an imaginary population of an infinite number of data points, distributed according to e.g. a Gaussian distribution function, characterized by the mean, \(\mu\) , and the variance \(\sigma\) . The probability of observing a value \(x_i\) for the \(i^{th}\) data point is then:

Fig. 1.2 A sample \(x_1, x_2, ...\) and its distribution curve. ¶
In reality we do not know the true mean for a distribution but have to estimate it. The question then is what is the best method to approximate the true mean given only a finite set of data points. If we know the the data are distributed according a gaussian with a certain \(\sigma\) we can determine the total probability or likelihood to observe the given set of data points assuming a value \(\mu'\) for the mean.
The best value for \(\mu'\) is the one for which \(P_{tot}\) is a maximum. This is an example of the method of maximum likelihood . In order for \(P_{tot}\) to be maximal the expression:
needs to be minimal and therefore \(\partial X/\partial \mu' = 0.\) This leads then to the expected result:
Here \(\sigma_{\mu'}\) is the standard error of the mean calculated using error propagation as outlined (1.4) , and \(\sigma\) has been estimated from the data as shown above.
If each data point has a different error, one has to assume that the set of measurements is a sample drawn from a set of different distributions with the same mean but different \(\sigma's\) . In that case the maximal likelihood is obtained by minimizing
which leads to the definition of the so-called weighted mean:
1.8. Least Square Fitting, Parameter Determination ¶
In the introduction of this chapter the two main type of experiments were listed as parameter determination and hypothesis testing. Both of these often lead to the problem that you have measured a quantity \(y_i\) that depends on another measured quantity \(x_i\) . Let’s assume that the experimental uncertainty \(\sigma_{y_i} \ll \sigma_{x_i}\) and that \(\sigma_{x_i}\) can be neglected. In that case we can assume that the set of experimental \(y_i\) -values represents a random sample of data drawn from distributions centered around a value of \(y(x_i)\) with a width of \(\sigma_i\) . The determination of the function \(y = f(x_i)\) can now be either the subject of parameter determination, of hypothesis testing or a combination of both. For parameter determination, we further assume that one can write \(y(x_i) = f(x_i, a_0, a_1, ..., a_n)\) where \(a_i\) are parameters that need to be determined from the experimental data and the goal of the fitting process is the determination of the best set of parameters that reproduce the experimental data set.

Fig. 1.3 Experimental points \((x_1,y_1), (x_2,y_2) ...\) , together with their distribution functions from which they were drawn and the function \(f(x)\) which should be estimated from the data. ¶
In order to determine the parameters \(a_0, a_1, ..., a_n\) one maximizes the total likelihood for obtaining the observed set of data \((x_i, y_i, \sigma_i)\) with respect to these parameters .
Maximizing \(P(a_0, a_1, ... , a_n)\) corresponds to minimizing the quantity \(\chi^2\) :
This forms the basis of least square fitting. Different fit algorithms have been developed depending on whether the fit function is linear in the parameters (linear fit) or non linear. They differ in the method of finding the minimum but all return the parameters at the minimum together with their variances (standard errors) and their covariances in form of the so-called covariance matrix. All fitting routines will return the quantity \(\chi^2\) and \(\chi^2_\nu = \chi^2/\nu\) where \(\nu = N - n\) the number of degrees of freedom of the fit. \(\chi^2_\nu\) is also called the reduced \(\chi^2\) . Since a data set is considered a random sample the value of \(\chi^2\) is also a random variable following its own distribution function:
This can be used to either have a measure of the quality of the fit and/or how well the chosen fitting function \(f(x_i, a_0, a_1, ..., a_n)\) represents the true functional relationship \(y(x)\) . The latter is an important part of hypothesis testing. Since a distribution function does not give a probability for a specific value of \(\chi^2_\nu\) but a probability density, one chooses an integral over the distribution function as a measure for the quality of a fit (also called the goodness of a fit) or a test of a hypothesis:
Here \(P(\chi^2,\nu)\) is the probability that another random set of \(N\) data points drawn from the same parent distribution would yield an equal or larger value of \(\chi^2\) than the one obtained.
For a fitting function that is a good approximation to the true function and with realistic error estimates one should obtain an average \(\chi^2\) -value for which \(P(\chi^2,\nu)\approx 0.5\) . A poorer fit will produce a larger value of \(\chi^2_\nu\) with a much smaller value of \(P(\chi^2,\nu)\) . In general fits with \(P(\chi^2,\nu)\) reasonably cose to 0.5 have a reduced \(\chi^2_\nu \approx 1\) or typically < 1.5.
The interpretation of \(\chi^2_\nu\) is always somewhat ambiguous as it depends on the quality of the data as well as on the choice of the fitting function.
In general, fitting a function which is non-linear in the parameters is more challenging as reasonable initial values need to be assigned to the fit parameters. Also if a minimum in \(\chi^2_\nu\) has been found it is not necessarily a global minimum. Therefore the parameters and the value of \(\chi^2_\nu\) need to be studied. If parameters are highly correlated it is also possible that a fit fails, i.e. a matrix cannot be inverted and a minimum cannot be found, this is usually a sign of an ill posed problem.
Error Analysis
Introduction, significant figures, the idea of error, classification of error, a. mean value, b. measuring error, maximum error, probable error, average deviation, standard deviation, c. standard deviation, propagation of errors.
- Original Research
- Open access
- Published: 30 January 2018
Teaching and learning mathematics through error analysis
- Sheryl J. Rushton 1
Fields Mathematics Education Journal volume 3 , Article number: 4 ( 2018 ) Cite this article
44k Accesses
25 Citations
12 Altmetric
Metrics details
For decades, mathematics education pedagogy has relied most heavily on teachers, demonstrating correctly worked example exercises as models for students to follow while practicing their own exercises. In more recent years, incorrect exercises have been introduced for the purpose of student-conducted error analysis. Combining the use of correctly worked exercises with error analysis has led researchers to posit increased mathematical understanding. Combining the use of correctly worked exercises with error analysis has led researchers to posit increased mathematical understanding.
A mixed method design was used to investigate the use of error analysis in a seventh-grade mathematics unit on equations and inequalities. Quantitative data were used to establish statistical significance of the effectiveness of using error analysis and qualitative methods were used to understand participants’ experience with error analysis.
The results determined that there was no significant difference in posttest scores. However, there was a significant difference in delayed posttest scores.
In general, the teacher and students found the use of error analysis to be beneficial in the learning process.
For decades, mathematics education pedagogy has relied most heavily on teachers demonstrating correctly worked example exercises as models for students to follow while practicing their own exercises [ 3 ]. In more recent years, incorrect exercises have been introduced for the purpose of student-conducted error analysis [ 17 ]. Conducting error analysis aligns with the Standards of Mathematical Practice [ 18 , 19 ] and the Mathematics Teaching Practices [ 18 ]. Researchers posit a result of increased mathematical understanding when these practices are used with a combination of correctly and erroneously worked exercises [ 1 , 4 , 8 , 11 , 15 , 16 , 18 , 19 , 23 ].
Review of literature
Correctly worked examples consist of a problem statement with the steps taken to reach a solution along with the final result and are an effective method for the initial acquisitions of procedural skills and knowledge [ 1 , 11 , 26 ]. Cognitive load theory [ 1 , 11 , 25 ] explains the challenge of stimulating the cognitive process without overloading the student with too much essential and extraneous information that will limit the working memory and leave a restricted capacity for learning. Correctly worked examples focus the student’s attention on the correct solution procedure which helps to avoid the need to search their prior knowledge for solution methods. Correctly worked examples free the students from performance demands and allow them to concentrate on gaining new knowledge [ 1 , 11 , 16 ].
Error analysis is an instructional strategy that holds promise of helping students to retain their learning [ 16 ]. Error analysis consists of being presented a problem statement with the steps taken to reach a solution in which one or more of the steps are incorrect, often called erroneous examples [ 17 ]. Students analyze and explain the errors and then complete the exercise correctly providing reasoning for their own solution. Error analysis leads students to enact two Standards of Mathematical Practice, namely, (a) make sense of problems and persevere in solving them and (b) attend to precision [ 19 ].
Another of the Standards of Mathematical Practice suggests that students learn to construct viable arguments and comment on the reasoning of others [ 19 ]. According to Große and Renkl [ 11 ], students who attempted to establish a rationale for the steps of the solution learned more than those who did not search for an explanation. Teachers can assist in this practice by facilitating meaningful mathematical discourse [ 18 ]. “Arguments do not have to be lengthy, they simply need to be clear, specific, and contain data or reasoning to back up the thinking” [ 20 ]. Those data and reasons could be in the form of charts, diagrams, tables, drawings, examples, or word explanations.
Researchers [ 7 , 21 ] found the process of explaining and justifying solutions for both correct and erroneous examples to be more beneficial for achieving learning outcomes than explaining and justifying solutions to correctly worked examples only. They also found that explaining why an exercise is correct or incorrect fostered transfer and led to better learning outcomes than explaining correct solutions only. According to Silver et al. [ 22 ], students are able to form understanding by classifying procedures into categories of correct examples and erroneous examples. The students then test their initial categories against further correct and erroneous examples to finally generate a set of attributes that defines the concept. Exposing students to both correctly worked examples and error analysis is especially beneficial when a mathematical concept is often done incorrectly or is easily confused [ 11 ].
Große and Renkl [ 11 ] suggested in their study involving university students in Germany that since errors are inherent in human life, introducing errors in the learning process encourages students to reflect on what they know and then be able to create clearer and more complete explanations of the solutions. The presentation of “incorrect knowledge can induce cognitive conflicts which prompt the learner to build up a coherent knowledge structure” [ 11 ]. Presenting a cognitive conflict through erroneously worked exercises triggers learning episodes through reflection and explanations, which leads to deeper understanding [ 29 ]. Error analysis “can foster a deeper and more complete understanding of mathematical content, as well as of the nature of mathematics itself” [ 4 ].
Several studies have been conducted on the use of error analysis in mathematical units [ 1 , 16 , 17 ]. The study conducted for this article differed from these previous studies in mathematical content, number of teachers and students involved in the study, and their use of a computer or online component. The most impactful differences between the error analysis studies conducted in the past and this article’s study are the length of time between the posttest and the delayed posttest and the use of qualitative data to add depth to the findings. The previous studies found students who conducted error analysis work did not perform significantly different on the posttest than students who received a more traditional approach to learning mathematics. However, the students who conducted error analysis outperformed the control group in each of the studies on delayed posttests that were given 1–2 weeks after the initial posttest.
Loibl and Rummel [ 15 ] discovered that high school students became aware of their knowledge gaps in a general manner by attempting an exercise and failing. Instruction comparing the erroneous work with correctly worked exercises filled the learning gaps. Gadgil et al. [ 9 ] conducted a study in which students who compared flawed work to expertly done work were more likely to repair their own errors than students who only explained the expertly done work. This discovery was further supported by other researchers [8, 14, 24]. Each of these researchers found students ranging from elementary mathematics to university undergraduate medical school who, when given correctly worked examples and erroneous examples, learned more than students who only examined correctly worked examples. This was especially true when the erroneous examples were similar to the kinds of errors that they had committed [ 14 ]. Stark et al. [ 24 ] added that it is important for students to receive sufficient scaffolding in correctly worked examples before and alongside of the erroneous examples.
The purpose of this study was to explore whether seventh-grade mathematics students could learn better from the use of both correctly worked examples and error analysis than from the more traditional instructional approach of solving their exercises in which the students are instructed with only correctly worked examples. The study furthered previous research on the subject of learning from the use of both correctly worked examples and error analysis by also investigating the feedback from the teacher’s and students’ experiences with error analysis. The following questions were answered in this study:
What was the difference in mathematical achievement when error analysis was included in students’ lessons and assignments versus a traditional approach of learning through correct examples only?
What kind of benefits or disadvantages did the students and teacher observe when error analysis was included in students’ lessons and assignments versus a traditional approach of learning through correct examples only?
A mixed method design was used to investigate the use of error analysis in a seventh-grade mathematics unit on equations and inequalities. Quantitative data were used to establish statistical significance of the effectiveness of using error analysis and qualitative methods were used to understand participants’ experience with error analysis [ 6 , 27 ].
Participants
Two-seventh-grade mathematics classes at an International Baccalaureate (IB) school in a suburban charter school in Northern Utah made up the control and treatment groups using a convenience grouping. One class of 26 students was the control group and one class of 27 students was the treatment group.
The same teacher taught both the groups, so a comparison could be made from the teacher’s point of view of how the students learned and participated in the two different groups. At the beginning of the study, the teacher was willing to give error analysis a try in her classroom; however, she was not enthusiastic about using this strategy. She could not visualize how error analysis could work on a daily basis. By the end of the study, the teacher became very enthusiastic about using error analysis in her seventh grade mathematics classes.
The total group of participants involved 29 males and 24 females. About 92% of the participants were Caucasian and the other 8% were of varying ethnicities. Seventeen percent of the student body was on free or reduced lunch. Approximately 10% of the students had individual education plans (IEP).
A pretest and posttest were created to contain questions that would test for mathematical understanding on equations and inequalities using Glencoe Math: Your Common Core Edition CCSS [ 5 ] as a resource. The pretest was reused as the delayed posttest. Homework assignments were created for both the control group and the treatment group from the Glencoe Math: Your Common Core Edition CCSS textbook. However, the researcher rewrote two to three of the homework exercises as erroneous examples for the treatment group to find the error and fix the exercise with justifications (see Figs. 1 , 2 ). Students from both groups used an Assignment Time Log to track the amount of time which they spent on their homework assignments.
Example of the rewritten homework exercises as equation erroneous examples
Example of the rewritten homework exercises as inequality erroneous examples
Both the control and the treatment groups were given the same pretest for an equations and inequality unit. The teacher taught both the control and treatment groups the information for the new concepts in the same manner. The majority of the instruction was done using the direct instruction strategy. The students in both groups were allowed to work collaboratively in pairs or small groups to complete the assignments after instruction had been given. During the time she allotted for answering questions from the previous assignment, she would only show the control group the exercises worked correctly. However, for the treatment group, the teacher would write errors which she found in the students’ work on the board. She would then either pair up the students or create small groups and have the student discuss what errors they noticed and how they would fix them. Often, the teacher brought the class together as a whole to discuss what they discovered and how they could learn from it.
The treatment group was given a homework assignment with the same exercises as the control group, but including the erroneous examples. Students in both the control and treatment groups were given the Assignment Time Log to keep a record of how much time was spent completing each homework assignment.
At the end of each week, both groups took the same quiz. The quizzes for the control group received a grade, and the quiz was returned without any further attention. If a student asked how to do an exercise, the teacher only showed the correct example. The teacher graded the quizzes for the treatment group using the strategy found in the Teaching Channel’s video “Highlighting Mistakes: A Grading Strategy” [ 2 ]. She marked the quizzes by highlighting the mistakes; no score was given. The students were allowed time in class or at home to make corrections with justifications.
The same posttest was administered to both groups at the conclusion of the equation and inequality chapter, and a delayed posttest was administered 6 weeks later. The delayed posttest also asked the students in the treatment group to respond to an open-ended request to “Please provide some feedback on your experience”. The test scores were analyzed for significant differences using independent samples t tests. The responses to the open-ended request were coded and analyzed for similarities and differences, and then, used to determine the students’ perceptions of the benefits or disadvantages of using error analysis in their learning.
At the conclusion of gathering data from the assessments, the researcher interviewed the teacher to determine the differences which the teacher observed in the preparation of the lessons and students’ participation in the lessons [ 6 ]. The interview with the teacher contained a variety of open-ended questions. These are the questions asked during the interview: (a) what is your opinion of using error analysis in your classroom at the conclusion of the study versus before the study began? (b) describe a typical classroom discussion in both the control group class and the treatment group class, (c) talk about the amount of time you spent grading, preparing, and teaching both groups, and (d) describe the benefits or disadvantages of using error analysis on a daily basis compared to not using error analysis in the classroom. The responses from the teacher were entered into a computer, coded, and analyzed for thematic content [ 6 , 27 ]. The themes that emerged from coding the teacher’s responses were used to determine the kind of benefits or disadvantages observed when error analysis was included in students’ lessons and assignments versus a traditional approach of learning through correct examples only from the teacher’s point of view.
Findings and discussion
Mathematical achievement.
Preliminary analyses were carried out to evaluate assumptions for the t test. Those assumptions include: (a) the independence, (b) normality tested using the Shapiro–Wilk test, and (c) homogeneity of variance tested using the Levene Statistic. All assumptions were met.
The Levene Statistic for the pretest scores ( p > 0.05) indicated that there was not a significant difference in the groups. Independent samples t tests were conducted to determine the effect error analysis had on student achievement determined by the difference in the means of the pretest and posttest and of the pretest and delayed posttest. There was no significant difference in the scores from the posttest for the control group ( M = 8.23, SD = 5.67) and the treatment group ( M = 9.56, SD = 5.24); t (51) = 0.88, p = 0.381. However, there was a significant difference in the scores from the delayed posttest for the control group ( M = 5.96, SD = 4.90) and the treatment group ( M = 9.41, SD = 4.77); t (51) = 2.60, p = 0.012. These results suggest that students can initially learn mathematical concepts through a variety of methods. Nevertheless, the retention of the mathematical knowledge is significantly increased when error analysis is added to the students’ lessons, assignments, and quizzes. It is interesting to note that the difference between the means from the pretest to the posttest was higher in the treatment group ( M = 9.56) versus the control group ( M = 8.23), implying that even though there was not a significant difference in the means, the treatment group did show a greater improvement.
The Assignment Time Log was completed by only 19% of the students in the treatment group and 38% of the students in the control group. By having such a small percentage of each group participate in tracking the time spent completing homework assignment, the results from the t test analysis cannot be used in any generalization. However, the results from the analysis were interesting. The mean time spent doing the assignments for each group was calculated and analyzed using an independent samples t test. There was no significant difference in the amount of time students which spent on their homework for the control group ( M = 168.30, SD = 77.41) and the treatment group ( M = 165.80, SD = 26.53); t (13) = 0.07, p = 0.946. These results suggest that the amount of time that students spent on their homework was close to the same whether they had to do error analyses (find the errors, fix them, and justify the steps taken) or solve each exercise in a traditional manner of following correctly worked examples. Although the students did not spend a significantly different amount of time outside of class doing homework, the treatment group did spend more time during class working on quiz corrections and discussing error which could attribute to the retention of knowledge.
Feedback from participants
All students participating in the current study submitted a signed informed consent form. Students process mathematical procedures better when they are aware of their own errors and knowledge gaps [ 15 ]. The theoretical model of using errors that students make themselves and errors that are likely due to the typical knowledge gaps can also be found in works by other researchers such as Kawasaki [ 14 ] and VanLehn [ 29 ]. Highlighting errors in the students’ own work and in typical errors made by others allowed the participants in the treatment group the opportunity to experience this theoretical model. From their experiences, the participants were able to give feedback to help the researcher delve deeper into what the thoughts were of the use of error analysis in their mathematics classes than any other study provided [ 1 , 4 , 7 , 8 , 9 , 11 , 14 , 15 , 16 , 17 , 21 , 23 , 24 , 25 , 26 , 29 ]. Overall, the teacher and students found the use of error analysis in the equations and inequalities unit to be beneficial. The teacher pointed out that the discussions in class were deeper in the treatment group’s class. When she tried to facilitate meaningful mathematical discourse [ 18 ] in the control group class, the students were unable to get to the same level of critical thinking as the treatment group discussions. In the open-ended question at the conclusion of the delayed posttest (“Please provide some feedback on your experience.”), the majority (86%) of the participants from the treatment group indicated that the use of erroneous examples integrated into their lessons was beneficial in helping them recognize their own mistakes and understanding how to correct those mistakes. One student reported, “I realized I was doing the same mistakes and now knew how to fix it”. Several (67%) of the students indicated learning through error analysis made the learning process easier for them. A student commented that “When I figure out the mistake then I understand the concept better, and how to do it, and how not to do it”.
When students find and correct the errors in exercises, while justifying themselves, they are being encouraged to learn to construct viable arguments and critique the reasoning of others [ 19 ]. This study found that explaining why an exercise is correct or incorrect fostered transfer and led to better learning outcomes than explaining correct solutions only. However, some of the higher level students struggled with the explanation component. According to the teacher, many of these higher level students who typically do very well on the homework and quizzes scored lower on the unit quizzes and tests than the students expected due to the requirement of explaining the work. In the past, these students had not been justifying their thinking and always got correct answers. Therefore, providing reasons for erroneous examples and justifying their own process were difficult for them.
Often teachers are resistant to the idea of using error analysis in their classroom. Some feel creating erroneous examples and highlighting errors for students to analyze is too time-consuming [ 28 ]. The teacher in this study taught both the control and treatment groups, which allowed her the perspective to compare both methods. She stated, “Grading took about the same amount of time whether I gave a score or just highlighted the mistakes”. She noticed that having the students work on their errors from the quizzes and having them find the errors in the assignments and on the board during class time ultimately meant less work for her and more work for the students.
Another reason behind the reluctance to use error analysis is the fact that teachers are uncertain about exposing errors to their students. They are fearful that the discussion of errors could lead their students to make those same errors and obtain incorrect solutions [ 28 ]. Yet, most of the students’ feedback stated the discussions in class and the error analyses on the assignments and quizzes helped them in working homework exercises correctly. Specifically, they said figuring out what went wrong in the exercise helped them solve that and other exercises. One student said that error analysis helped them “do better in math on the test, and I actually enjoyed it”. Nevertheless, 2 of the 27 participating students in the treatment group had negative comments about learning through error analysis. One student did not feel that correcting mistakes showed them anything, and it did not reinforce the lesson. The other student stated being exposed to error analysis did, indeed, confuse them. The student kept thinking the erroneous example was a correct answer and was unsure about what they were supposed to do to solve the exercise.
When the researcher asked the teacher if there were any benefits or disadvantages to using error analysis in teaching the equations and inequalities unit, she said that she thoroughly enjoyed teaching using the error analysis method and was planning to implement it in all of her classes in the future. In fact, she found that her “hands were tied” while grading the control group quizzes and facilitating the lessons. She said, “I wanted to have the students find their errors and fix them, so we could have a discussion about what they were doing wrong”. The students also found error analysis to have more benefits than disadvantages. Other than one student whose response was eliminated for not being on topic and the two students with negative comments, the other 24 of the students in the treatment group had positive comments about their experience with error analysis. When students had the opportunity to analyze errors in worked exercises (error analysis) through the assignments and quizzes, they were able to get a deeper understanding of the content and, therefore, retained the information longer than those who only learned through correct examples.
Discussions generated in the treatment group’s classroom afforded the students the opportunity to critically reason through the work of others and to develop possible arguments on what had been done in the erroneous exercise and what approaches might be taken to successfully find a solution to the exercise. It may seem surprising that an error as simple as adding a number when it should have been subtracted could prompt a variety of questions and lead to the students suggesting possible ways to solve and check to see if the solution makes sense. In an erroneous exercise presented to the treatment group, the students were provided with the information that two of the three angles of a triangle were 35° and 45°. The task was to write and solve an equation to find the missing measure. The erroneous exercise solver had created the equation: x + 35 + 45 = 180. Next was written x + 80 = 180. The solution was x = 260°. In the discussion, the class had on this exercise, the conclusion was made that the error occurred when 80 was added to 180 to get a sum of 260. However, the discussion progressed finding different equations and steps that could have been taken to discover the missing angle measure to be 100° and why 260° was an unreasonable solution. Another approach discussed by the students was to recognize that to say the missing angle measure was 260° contradicted with the fact that one angle could not be larger than the sum of the angle measures of a triangle. Analyzing the erroneous exercises gave the students the opportunity of engaging in the activity of “explaining” and “fixing” the errors of the presented exercise as well as their own errors, an activity that fostered the students’ learning.
The students participating in both the control and treatment groups from the two-seventh-grade mathematics classes at the IB school in a suburban charter school in Northern Utah initially learned the concepts taught in the equations and inequality unit statistically just as well with both methods of teaching. The control group had the information taught to them with the use of only correctly worked examples. If they had a question about an exercise which they did wrong, the teacher would show them how to do the exercise correctly and have a discussion on the steps required to obtain the correct solutions. On their assignments and quizzes, the control group was expected to complete the work by correctly solving the equations and inequalities in the exercise, get a score on their work, and move on to the next concept. On the other hand, the students participating in the treatment group were given erroneous examples within their assignments and asked to find the errors, explain what had been done wrong, and then correctly solve the exercise with justifications for the steps they chose to use. During lessons, the teacher put erroneous examples from the students’ work on the board and generated paired, small groups, or whole group discussion of what was wrong with the exercise and the different ways to do it correctly. On the quizzes, the teacher highlighted the errors and allowed the students to explain the errors and justify the correct solution.
Both the method of teaching using error analysis and the traditional method of presenting the exercise and having the students solve it proved to be just as successful on the immediate unit summative posttest. However, the delayed posttest given 6 weeks after the posttest showed that the retention of knowledge was significantly higher for the treatment group. It is important to note that the fact that the students in the treatment group were given more time to discuss the exercises in small groups and as a whole class could have influenced the retention of mathematical knowledge just as much or more than the treatment of using error analysis. Researchers have proven academic advantages of group work for students, in large part due to the perception of students having a secure support system, which cannot be obtained when working individually [ 10 , 12 , 13 ].
The findings of this study supported the statistical findings of other researchers [ 1 , 16 , 17 ], suggesting that error analysis may aid in providing a richer learning experience that leads to a deeper understanding of equations and inequalities for long-term knowledge. The findings of this study also investigated the teacher’s and students’ perceptions of using error analysis in their teaching and learning. The students and teacher used for this study were chosen to have the same teacher for both the control and treatment groups. Using the same teacher for both groups, the researcher was able to determine the teacher’s attitude toward the use of error analysis compared to the non-use of error analysis in her instruction. The teacher’s comments during the interview implied that she no longer had an unenthusiastic and skeptical attitude toward the use of error analysis on a daily basis in her classroom. She was “excited to implement the error analysis strategy into the rest of her classes for the rest of the school year”. She observed error analysis to be an effective way to deal with common misconceptions and offer opportunities for students to reflect on their learning from their errors. The process of error analysis assisted the teacher in supporting productive struggle in learning mathematics [ 18 ] and created opportunity for students to have deep discussions about alternative ways to solve exercises. Error analysis also aided in students’ discovery of their own errors and gave them possible ways to correct those errors. Learning through the use of error analysis was enjoyable for many of the participating students.
According to the NCTM [ 18 ], effective teaching of mathematics happens when a teacher implements exercises that will engage students in solving and discussing tasks that promote mathematical reasoning and problem solving. Providing erroneous examples allowed discussion, multiple entry points, and varied solution strategies. Both the teacher and the students participating in the treatment group came to the conclusion that error analysis is a beneficial strategy to use in the teaching and learning of mathematics. Regardless of the two negative student comments about error analysis not being helpful for them, this researcher recommends the use of error analysis in teaching and learning mathematics.
The implications of the treatment of teaching students mathematics through the use of error analysis are that students’ learning could be fostered and retention of content knowledge may be longer. When a teacher is able to have their students’ practice critiquing the reasoning of others and creating viable arguments [ 19 ] by analyzing errors in mathematics, the students not only are able to meet the Standard of Mathematical Practice, but are also creating a lifelong skill of analyzing the effectiveness of “plausible arguments, distinguish correct logic or reasoning from that which is flawed, and—if there is a flaw in an argument—explain what it is” ([ 19 ], p. 7).
Limitations and future research
This study had limitations. The sample size was small to use the same teacher for both groups. Another limitation was the length of the study only encompassed one unit. Using error analysis could have been a novelty and engaged the students more than it would when the novelty wore off. Still another limitation was the study that was conducted at an International Baccalaureate (IB) school in a suburban charter school in Northern Utah, which may limit the generalization of the findings and implications to other schools with different demographics.
This study did not have a separation of conceptual and procedural questions on the assessments. For a future study, the creation of an assessment that would be able to determine if error analysis was more helpful in teaching conceptual mathematics or procedural mathematics could be beneficial to teachers as they plan their lessons. Another suggestion for future research would be to gather more data using several teachers teaching both the treatment group and the control group.
Adams, D.M., McLaren, B.M., Durkin, K., Mayer, R.E., Rittle-Johnson, B., Isotani, S., van Velsen, M.: Using erroneous examples to improve mathematics learning with a web-based tutoring system. Comput. Hum. Behav. 36 , 401–411 (2014)
Article Google Scholar
Alcala, L.: Highlighting mistakes: a grading strategy. The teaching channel. https://www.teachingchannel.org/videos/math-test-grading-tips
Atkinson, R.K., Derry, S.J., Renkl, A., Wortham, D.: Learning from examples: instructional Principles from the worked examples research. Rev. Educ. Res. 70 (2), 181–214 (2000)
Borasi, R.: Exploring mathematics through the analysis of errors. Learn. Math. 7 (3), 2–8 (1987)
Google Scholar
Carter, J.A., Cuevas, G.J., Day, R., Malloy, C., Kersaint, G., Luchin, B.M., Willard, T.: Glencoe math: your common core edition CCSS. Glencoe/McGraw-Hill, Columbus (2013)
Creswell, J.: Research design: qualitative, quantitative, and mixed methods approaches, 4th edn. Sage Publications, Thousand Oaks (2014)
Curry, L. A.: The effects of self-explanations of correct and incorrect solutions on algebra problem-solving performance. In: Proceedings of the 26th annual conference of the cognitive science society, vol. 1548. Erlbaum, Mahwah (2004)
Durkin, K., Rittle-Johnson, B.: The effectiveness of using incorrect examples to support learning about decimal magnitude. Learn. Instr. 22 (3), 206–214 (2012)
Gadgil, S., Nokes-Malach, T.J., Chi, M.T.: Effectiveness of holistic mental model confrontation in driving conceptual change. Learn. Instr. 22 (1), 47–61 (2012)
Gaudet, A.D., Ramer, L.M., Nakonechny, J., Cragg, J.J., Ramer, M.S.: Small-group learning in an upper-level university biology class enhances academic performance and student attitutdes toward group work. Public Libr. Sci. One 5 , 1–9 (2010)
Große, C.S., Renkl, A.: Finding and fixing errors in worked examples: can this foster learning outcomes? Learn. Instr. 17 (6), 612–634 (2007)
Janssen, J., Kirschner, F., Erkens, G., Kirschner, P.A., Paas, F.: Making the black box of collaborative learning transparent: combining process-oriented and cognitive load approaches. Educ. Psychol. Rev. 22 , 139–154 (2010)
Johnson, D.W., Johnson, R.T.: An educational psychology success story: social interdependence theory and cooperative learning. Educ. Res. 38 , 365–379 (2009)
Kawasaki, M.: Learning to solve mathematics problems: the impact of incorrect solutions in fifth grade peers’ presentations. Jpn. J. Dev. Psychol. 21 (1), 12–22 (2010)
Loibl, K., Rummel, N.: Knowing what you don’t know makes failure productive. Learn. Instr. 34 , 74–85 (2014)
McLaren, B.M., Adams, D., Durkin, K., Goguadze, G., Mayer, R.E., Rittle-Johnson, B., Van Velsen, M.: To err is human, to explain and correct is divine: a study of interactive erroneous examples with middle school math students. 21st Century learning for 21st Century skills, pp. 222–235. Springer, Berlin (2012)
Chapter Google Scholar
McLaren, B.M., Adams, D.M., Mayer, R.E.: Delayed learning effects with erroneous examples: a study of learning decimals with a web-based tutor. Int. J. Artif. Intell. Educ. 25 (4), 520–542 (2015)
National Council of Teachers of Mathematics (NCTM): Principles to actions: ensuring mathematical success for all. Author, Reston (2014)
National Governors Association Center for Best Practices & Council of Chief State School Officers (NGA Center and CCSSO): Common core state standards. Authors, Washington, DC (2010)
O’Connell, S., SanGiovanni, J.: Putting the practices into action: Implementing the common core standards for mathematical practice K-8. Heinemann, Portsmouth (2013)
Siegler, R.S.: Microgenetic studies of self-explanation. Microdevelopment: transition processes in development and learning, pp. 31–58. Cambridge University Press, New York (2002)
Silver, H.F., Strong, R.W., Perini, M.J.: The strategic teacher: Selecting the right research-based strategy for every lesson. ASCD, Alexandria (2009)
Sisman, G.T., Aksu, M.: A study on sixth grade students’ misconceptions and errors in spatial measurement: length, area, and volume. Int. J. Sci. Math. Educ. 14 (7), 1293–1319 (2015)
Stark, R., Kopp, V., Fischer, M.R.: Case-based learning with worked examples in complex domains: two experimental studies in undergraduate medical education. Learn. Instr. 21 (1), 22–33 (2011)
Sweller, J.: Cognitive load during problem solving: effects on learning. Cognitive Sci. 12 , 257–285 (1988)
Sweller, J., Cooper, G.A.: The use of worked examples as a substitute for problem solving in learning algebra. Cognit. Instr. 2 (1), 59–89 (1985)
Tashakkori, A., Teddlie, C.: Sage handbook of mixed methods in social & behavioral research, 2nd edn. Sage Publications, Thousand Oaks (2010)
Book Google Scholar
Tsovaltzi, D., Melis, E., McLaren, B.M., Meyer, A.K., Dietrich, M., Goguadze, G.: Learning from erroneous examples: when and how do students benefit from them? Sustaining TEL: from innovation to learning and practice, pp. 357–373. Springer, Berlin (2010)
VanLehn, K.: Rule-learning events in the acquisition of a complex skill: an evaluation of CASCADE. J. Learn. Sci. 8 (1), 71–125 (1999)
Download references
Acknowledgements
Not applicable.
Competing interests
The author declares that no competing interests.
Availability of data and materials
A spreadsheet of the data will be provided as an Additional file 1 : Error analysis data.
Consent for publication
Ethics approval and consent to participate.
All students participating in the current study submitted a signed informed consent form.
Publisher’s Note
Springer Nature remains neutral with regard to jurisdictional claims in published maps and institutional affiliations.
Author information
Authors and affiliations.
Weber State University, 1351 Edvalson St. MC 1304, Ogden, UT, 84408, USA
Sheryl J. Rushton
You can also search for this author in PubMed Google Scholar
Corresponding author
Correspondence to Sheryl J. Rushton .
Additional file
Additional file 1:.
Error analysis data.
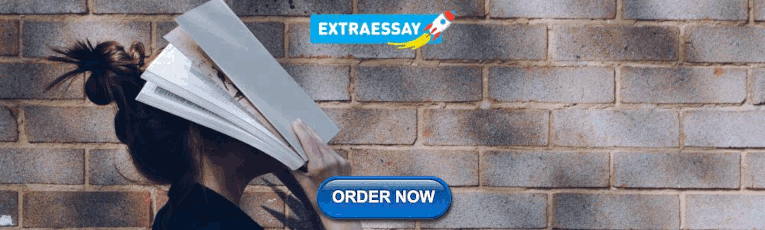
Rights and permissions
Open Access This article is distributed under the terms of the Creative Commons Attribution 4.0 International License ( http://creativecommons.org/licenses/by/4.0/ ), which permits unrestricted use, distribution, and reproduction in any medium, provided you give appropriate credit to the original author(s) and the source, provide a link to the Creative Commons license, and indicate if changes were made.
Reprints and permissions
About this article
Cite this article.
Rushton, S.J. Teaching and learning mathematics through error analysis. Fields Math Educ J 3 , 4 (2018). https://doi.org/10.1186/s40928-018-0009-y
Download citation
Received : 07 March 2017
Accepted : 16 January 2018
Published : 30 January 2018
DOI : https://doi.org/10.1186/s40928-018-0009-y
Share this article
Anyone you share the following link with will be able to read this content:
Sorry, a shareable link is not currently available for this article.
Provided by the Springer Nature SharedIt content-sharing initiative
- Error analysis
- Correct and erroneous examples
- Mathematics teaching practices
- Standards of mathematical practices
Numbers, Facts and Trends Shaping Your World
Read our research on:
Full Topic List
Regions & Countries
- Publications
- Our Methods
- Short Reads
- Tools & Resources
Read Our Research On:
Key things to know about U.S. election polling in 2024
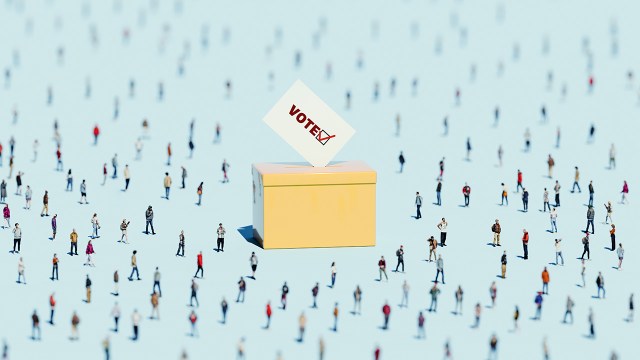
Confidence in U.S. public opinion polling was shaken by errors in 2016 and 2020. In both years’ general elections, many polls underestimated the strength of Republican candidates, including Donald Trump. These errors laid bare some real limitations of polling.
In the midterms that followed those elections, polling performed better . But many Americans remain skeptical that it can paint an accurate portrait of the public’s political preferences.
Restoring people’s confidence in polling is an important goal, because robust and independent public polling has a critical role to play in a democratic society. It gathers and publishes information about the well-being of the public and about citizens’ views on major issues. And it provides an important counterweight to people in power, or those seeking power, when they make claims about “what the people want.”
The challenges facing polling are undeniable. In addition to the longstanding issues of rising nonresponse and cost, summer 2024 brought extraordinary events that transformed the presidential race . The good news is that people with deep knowledge of polling are working hard to fix the problems exposed in 2016 and 2020, experimenting with more data sources and interview approaches than ever before. Still, polls are more useful to the public if people have realistic expectations about what surveys can do well – and what they cannot.
With that in mind, here are some key points to know about polling heading into this year’s presidential election.
Probability sampling (or “random sampling”). This refers to a polling method in which survey participants are recruited using random sampling from a database or list that includes nearly everyone in the population. The pollster selects the sample. The survey is not open for anyone who wants to sign up.
Online opt-in polling (or “nonprobability sampling”). These polls are recruited using a variety of methods that are sometimes referred to as “convenience sampling.” Respondents come from a variety of online sources such as ads on social media or search engines, websites offering rewards in exchange for survey participation, or self-enrollment. Unlike surveys with probability samples, people can volunteer to participate in opt-in surveys.
Nonresponse and nonresponse bias. Nonresponse is when someone sampled for a survey does not participate. Nonresponse bias occurs when the pattern of nonresponse leads to error in a poll estimate. For example, college graduates are more likely than those without a degree to participate in surveys, leading to the potential that the share of college graduates in the resulting sample will be too high.
Mode of interview. This refers to the format in which respondents are presented with and respond to survey questions. The most common modes are online, live telephone, text message and paper. Some polls use more than one mode.
Weighting. This is a statistical procedure pollsters perform to make their survey align with the broader population on key characteristics like age, race, etc. For example, if a survey has too many college graduates compared with their share in the population, people without a college degree are “weighted up” to match the proper share.
How are election polls being conducted?
Pollsters are making changes in response to the problems in previous elections. As a result, polling is different today than in 2016. Most U.S. polling organizations that conducted and publicly released national surveys in both 2016 and 2022 (61%) used methods in 2022 that differed from what they used in 2016 . And change has continued since 2022.
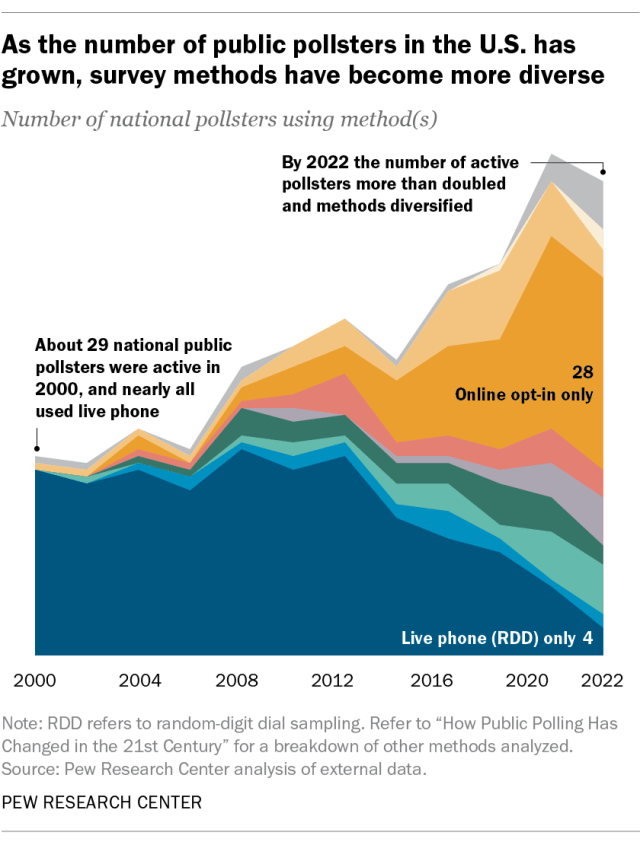
One change is that the number of active polling organizations has grown significantly, indicating that there are fewer barriers to entry into the polling field. The number of organizations that conduct national election polls more than doubled between 2000 and 2022.
This growth has been driven largely by pollsters using inexpensive opt-in sampling methods. But previous Pew Research Center analyses have demonstrated how surveys that use nonprobability sampling may have errors twice as large , on average, as those that use probability sampling.
The second change is that many of the more prominent polling organizations that use probability sampling – including Pew Research Center – have shifted from conducting polls primarily by telephone to using online methods, or some combination of online, mail and telephone. The result is that polling methodologies are far more diverse now than in the past.
(For more about how public opinion polling works, including a chapter on election polls, read our short online course on public opinion polling basics .)
All good polling relies on statistical adjustment called “weighting,” which makes sure that the survey sample aligns with the broader population on key characteristics. Historically, public opinion researchers have adjusted their data using a core set of demographic variables to correct imbalances between the survey sample and the population.
But there is a growing realization among survey researchers that weighting a poll on just a few variables like age, race and gender is insufficient for getting accurate results. Some groups of people – such as older adults and college graduates – are more likely to take surveys, which can lead to errors that are too sizable for a simple three- or four-variable adjustment to work well. Adjusting on more variables produces more accurate results, according to Center studies in 2016 and 2018 .
A number of pollsters have taken this lesson to heart. For example, recent high-quality polls by Gallup and The New York Times/Siena College adjusted on eight and 12 variables, respectively. Our own polls typically adjust on 12 variables . In a perfect world, it wouldn’t be necessary to have that much intervention by the pollster. But the real world of survey research is not perfect.
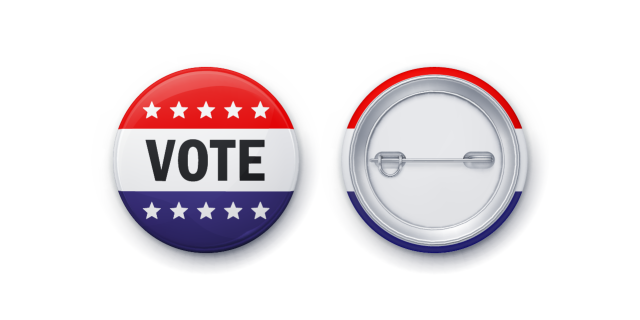
Predicting who will vote is critical – and difficult. Preelection polls face one crucial challenge that routine opinion polls do not: determining who of the people surveyed will actually cast a ballot.
Roughly a third of eligible Americans do not vote in presidential elections , despite the enormous attention paid to these contests. Determining who will abstain is difficult because people can’t perfectly predict their future behavior – and because many people feel social pressure to say they’ll vote even if it’s unlikely.
No one knows the profile of voters ahead of Election Day. We can’t know for sure whether young people will turn out in greater numbers than usual, or whether key racial or ethnic groups will do so. This means pollsters are left to make educated guesses about turnout, often using a mix of historical data and current measures of voting enthusiasm. This is very different from routine opinion polls, which mostly do not ask about people’s future intentions.
When major news breaks, a poll’s timing can matter. Public opinion on most issues is remarkably stable, so you don’t necessarily need a recent poll about an issue to get a sense of what people think about it. But dramatic events can and do change public opinion , especially when people are first learning about a new topic. For example, polls this summer saw notable changes in voter attitudes following Joe Biden’s withdrawal from the presidential race. Polls taken immediately after a major event may pick up a shift in public opinion, but those shifts are sometimes short-lived. Polls fielded weeks or months later are what allow us to see whether an event has had a long-term impact on the public’s psyche.
How accurate are polls?
The answer to this question depends on what you want polls to do. Polls are used for all kinds of purposes in addition to showing who’s ahead and who’s behind in a campaign. Fair or not, however, the accuracy of election polling is usually judged by how closely the polls matched the outcome of the election.
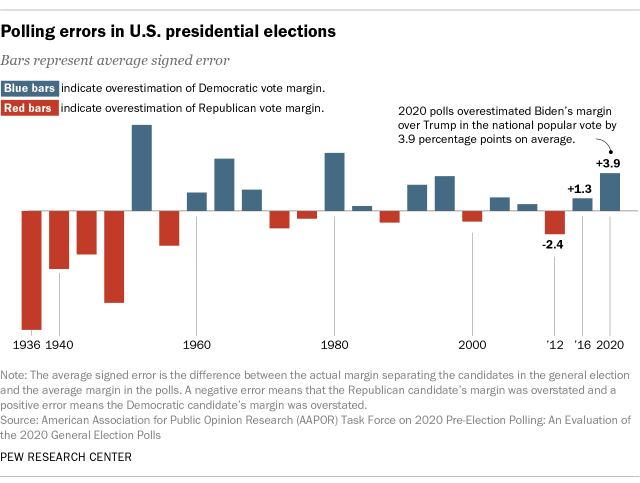
By this standard, polling in 2016 and 2020 performed poorly. In both years, state polling was characterized by serious errors. National polling did reasonably well in 2016 but faltered in 2020.
In 2020, a post-election review of polling by the American Association for Public Opinion Research (AAPOR) found that “the 2020 polls featured polling error of an unusual magnitude: It was the highest in 40 years for the national popular vote and the highest in at least 20 years for state-level estimates of the vote in presidential, senatorial, and gubernatorial contests.”
How big were the errors? Polls conducted in the last two weeks before the election suggested that Biden’s margin over Trump was nearly twice as large as it ended up being in the final national vote tally.
Errors of this size make it difficult to be confident about who is leading if the election is closely contested, as many U.S. elections are .
Pollsters are rightly working to improve the accuracy of their polls. But even an error of 4 or 5 percentage points isn’t too concerning if the purpose of the poll is to describe whether the public has favorable or unfavorable opinions about candidates , or to show which issues matter to which voters. And on questions that gauge where people stand on issues, we usually want to know broadly where the public stands. We don’t necessarily need to know the precise share of Americans who say, for example, that climate change is mostly caused by human activity. Even judged by its performance in recent elections, polling can still provide a faithful picture of public sentiment on the important issues of the day.
The 2022 midterms saw generally accurate polling, despite a wave of partisan polls predicting a broad Republican victory. In fact, FiveThirtyEight found that “polls were more accurate in 2022 than in any cycle since at least 1998, with almost no bias toward either party.” Moreover, a handful of contrarian polls that predicted a 2022 “red wave” largely washed out when the votes were tallied. In sum, if we focus on polling in the most recent national election, there’s plenty of reason to be encouraged.
Compared with other elections in the past 20 years, polls have been less accurate when Donald Trump is on the ballot. Preelection surveys suffered from large errors – especially at the state level – in 2016 and 2020, when Trump was standing for election. But they performed reasonably well in the 2018 and 2022 midterms, when he was not.
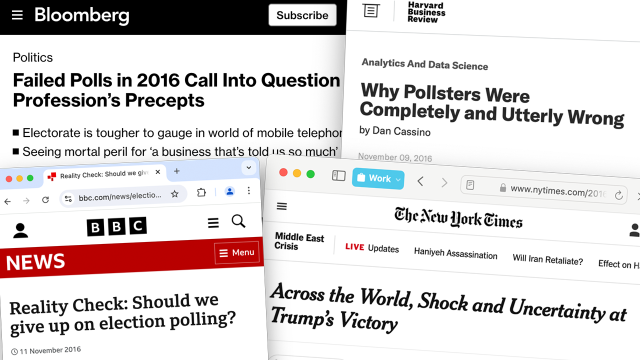
During the 2016 campaign, observers speculated about the possibility that Trump supporters might be less willing to express their support to a pollster – a phenomenon sometimes described as the “shy Trump effect.” But a committee of polling experts evaluated five different tests of the “shy Trump” theory and turned up little to no evidence for each one . Later, Pew Research Center and, in a separate test, a researcher from Yale also found little to no evidence in support of the claim.
Instead, two other explanations are more likely. One is about the difficulty of estimating who will turn out to vote. Research has found that Trump is popular among people who tend to sit out midterms but turn out for him in presidential election years. Since pollsters often use past turnout to predict who will vote, it can be difficult to anticipate when irregular voters will actually show up.
The other explanation is that Republicans in the Trump era have become a little less likely than Democrats to participate in polls . Pollsters call this “partisan nonresponse bias.” Surprisingly, polls historically have not shown any particular pattern of favoring one side or the other. The errors that favored Democratic candidates in the past eight years may be a result of the growth of political polarization, along with declining trust among conservatives in news organizations and other institutions that conduct polls.
Whatever the cause, the fact that Trump is again the nominee of the Republican Party means that pollsters must be especially careful to make sure all segments of the population are properly represented in surveys.
The real margin of error is often about double the one reported. A typical election poll sample of about 1,000 people has a margin of sampling error that’s about plus or minus 3 percentage points. That number expresses the uncertainty that results from taking a sample of the population rather than interviewing everyone . Random samples are likely to differ a little from the population just by chance, in the same way that the quality of your hand in a card game varies from one deal to the next.
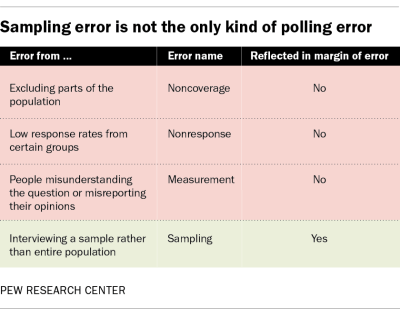
The problem is that sampling error is not the only kind of error that affects a poll. Those other kinds of error, in fact, can be as large or larger than sampling error. Consequently, the reported margin of error can lead people to think that polls are more accurate than they really are.
There are three other, equally important sources of error in polling: noncoverage error , where not all the target population has a chance of being sampled; nonresponse error, where certain groups of people may be less likely to participate; and measurement error, where people may not properly understand the questions or misreport their opinions. Not only does the margin of error fail to account for those other sources of potential error, putting a number only on sampling error implies to the public that other kinds of error do not exist.
Several recent studies show that the average total error in a poll estimate may be closer to twice as large as that implied by a typical margin of sampling error. This hidden error underscores the fact that polls may not be precise enough to call the winner in a close election.
Other important things to remember
Transparency in how a poll was conducted is associated with better accuracy . The polling industry has several platforms and initiatives aimed at promoting transparency in survey methodology. These include AAPOR’s transparency initiative and the Roper Center archive . Polling organizations that participate in these organizations have less error, on average, than those that don’t participate, an analysis by FiveThirtyEight found .
Participation in these transparency efforts does not guarantee that a poll is rigorous, but it is undoubtedly a positive signal. Transparency in polling means disclosing essential information, including the poll’s sponsor, the data collection firm, where and how participants were selected, modes of interview, field dates, sample size, question wording, and weighting procedures.
There is evidence that when the public is told that a candidate is extremely likely to win, some people may be less likely to vote . Following the 2016 election, many people wondered whether the pervasive forecasts that seemed to all but guarantee a Hillary Clinton victory – two modelers put her chances at 99% – led some would-be voters to conclude that the race was effectively over and that their vote would not make a difference. There is scientific research to back up that claim: A team of researchers found experimental evidence that when people have high confidence that one candidate will win, they are less likely to vote. This helps explain why some polling analysts say elections should be covered using traditional polling estimates and margins of error rather than speculative win probabilities (also known as “probabilistic forecasts”).
National polls tell us what the entire public thinks about the presidential candidates, but the outcome of the election is determined state by state in the Electoral College . The 2000 and 2016 presidential elections demonstrated a difficult truth: The candidate with the largest share of support among all voters in the United States sometimes loses the election. In those two elections, the national popular vote winners (Al Gore and Hillary Clinton) lost the election in the Electoral College (to George W. Bush and Donald Trump). In recent years, analysts have shown that Republican candidates do somewhat better in the Electoral College than in the popular vote because every state gets three electoral votes regardless of population – and many less-populated states are rural and more Republican.
For some, this raises the question: What is the use of national polls if they don’t tell us who is likely to win the presidency? In fact, national polls try to gauge the opinions of all Americans, regardless of whether they live in a battleground state like Pennsylvania, a reliably red state like Idaho or a reliably blue state like Rhode Island. In short, national polls tell us what the entire citizenry is thinking. Polls that focus only on the competitive states run the risk of giving too little attention to the needs and views of the vast majority of Americans who live in uncompetitive states – about 80%.
Fortunately, this is not how most pollsters view the world . As the noted political scientist Sidney Verba explained, “Surveys produce just what democracy is supposed to produce – equal representation of all citizens.”
- Survey Methods
- Trust, Facts & Democracy
- Voter Files
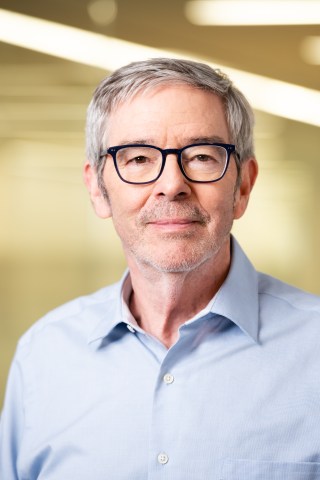
Scott Keeter is a senior survey advisor at Pew Research Center .
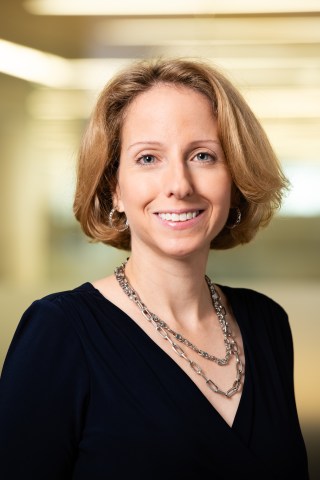
Courtney Kennedy is Vice President of Methods and Innovation at Pew Research Center .
How do people in the U.S. take Pew Research Center surveys, anyway?
How public polling has changed in the 21st century, what 2020’s election poll errors tell us about the accuracy of issue polling, a field guide to polling: election 2020 edition, methods 101: how is polling done around the world, most popular.
901 E St. NW, Suite 300 Washington, DC 20004 USA (+1) 202-419-4300 | Main (+1) 202-857-8562 | Fax (+1) 202-419-4372 | Media Inquiries
Research Topics
- Email Newsletters
ABOUT PEW RESEARCH CENTER Pew Research Center is a nonpartisan fact tank that informs the public about the issues, attitudes and trends shaping the world. It conducts public opinion polling, demographic research, media content analysis and other empirical social science research. Pew Research Center does not take policy positions. It is a subsidiary of The Pew Charitable Trusts .
© 2024 Pew Research Center
Investigation for Applicability of Deep Learning Based Prediction Model in Energy Consumption Analysis
- Original Research
- Published: 02 September 2024
- Volume 5 , article number 853 , ( 2024 )
Cite this article
- Brijesh Singh 1 ,
- Jitendra Kumar Seth ORCID: orcid.org/0000-0001-7997-3443 2 ,
- Devansh Kumar Srivastava 1 ,
- Anchal Kumar Singh 1 &
- Aman Mishra 1
The electricity demand is rising in our day-to-day life and business; therefore, it is important to forecast electricity consumption to balance the demand and supply chain. The prediction mechanism will enable us to meet the demand quickly and use the available resources optimally. It will help us to reduce the waste of energy. Several statistical methods have been proposed to solve this issue, but they do not achieve the goal due to tedious topology and monitoring mechanisms. This research aims to forecast the load hourly or annually without rigorous monitoring and with fewer resources using Deep Learning models. In this work, Recurrent Neural Network (RNN), Long-Short Term Memory (LSTM), and Gated Recurrent Unit (GRU) based models are implemented. Their performances are measured using different evaluation metrics such as Mean Squared Error (MSE), Root Mean Square Error (RMSE) and Mean Absolute Error (MAE) to identify the best-performing model to forecast the load. The dataset is formed by collecting data from several open-source platforms containing the required parameters affecting the load day or night throughout all seasons. The proposed model can be utilized in the optimal production and supply of energy by accurately forecasting the energy load at a time.
This is a preview of subscription content, log in via an institution to check access.
Access this article
Subscribe and save.
- Get 10 units per month
- Download Article/Chapter or eBook
- 1 Unit = 1 Article or 1 Chapter
- Cancel anytime
Price excludes VAT (USA) Tax calculation will be finalised during checkout.
Instant access to the full article PDF.
Rent this article via DeepDyve
Institutional subscriptions
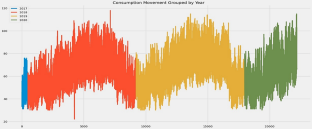
Similar content being viewed by others
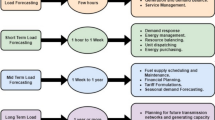
Advances in Deep Learning Techniques for Short-term Energy Load Forecasting Applications: A Review
Long-Short Term Memory Model with Univariate Input for Forecasting Individual Household Electricity Consumption
Energy Demand Forecasting Using Deep Learning
Explore related subjects.
- Artificial Intelligence
Data availability
Data availability copyright is not required. Dataset is taken from open source.
Hogarty TH. The US Energy Information Administration and coal data. Energy Policy. 1978;6(2):168–9.
Article Google Scholar
Jordan MI, Mitchell TM. Machine learning: Trends, perspectives, and prospects. Science. 2015;349(6245):255–60.
Article MathSciNet Google Scholar
Mitchell TM. Machine learning. New York: McGraw-Hill; 1997.
Google Scholar
Rizvi S, Abu-Siada A. Active Power Sharing in a Micro-Grid with Multiple Grid Connections. Designs. 2022;6(2):24. https://doi.org/10.3390/designs6020024 .
Wang Y-N. Approximate-based Internal Model Control Strategy. Acta Automatica Sinica. 2009;34(2):172–9. https://doi.org/10.3724/sp.j.1004.2008.00172 .
Amral N, Özveren C, King D. Short term load forecasting using multiple linear regression. In Proceedings of the 42nd International Universities Power Engineering Conference, (UPEC 2007), Brighton, UK, 4–6 September 2007; pp. 1192–1198.
Hahn H, Meyer-Nieberg S, Pickl S. Electric load forecasting methods: tools for decision making. Eur J Oper Res. 2009;199:902–7.
Gross G, Galiana FD. Short-term load forecasting. Proc IEEE. 1987;75(12):1558–73.
Krogh B, de Llinas E, Lesser D. Design and implementation of an on-line load forecasting algorithm. IEEE Trans Power Appar Syst. 1982;9:3284–9.
Papalexopoulos AD, Hesterberg TC. A regression-based approach to short-term system load forecasting. IEEE Trans Power Syst. 1990;5:1535–47.
Ahmad T, Chen H. Short and medium-term forecasting of cooling and heating load demand in building environment with data- mining based approaches. Energy Build. 2018;166:460–76.
Fan C, Xiao F, Zhao Y. A short-term building cooling load prediction method using deep learning algorithms. Appl Energy. 2017;195:222–33.
Seyedzadeh S, et al. Machine learning for estimation of building energy consumption and performance: a review. Vis Eng. 2018;6:1–20.
Kuster C, Rezgui Y, Mourshed M. Electrical load forecasting models: a critical systematic review. Sustain Cities Soc. 2017;35:257–70.
Esenyel NM, Akın M. Comparing Accuracy Performance of ELM, ARMA and ARMA-GARCH Model In Predicting Exchange Rate Return. Alphanumeric J. 2017;5(1):1. https://doi.org/10.17093/alphanumeric.298658 .
Rani P, Distance ARIMA. Improved Traffic Prediction by Applying KNN and Euclidean Distance ARIMA (Ke-Arima) Approach. Int J Comp Appl. 2018;182(3):23–9. https://doi.org/10.5120/ijca2018917488 .
Nair V, Hinton GE. Rectified linear units improve restricted boltzmann machines, in Proceedings of the 27th International Conference on Machine Learning (ICML ’10), pp. 807–814, June 2010.
Yi B, Kim. An Enhanced Algorithm of RNN Using Trend in Time-Series. Symmetry. 2019;11(7):912. https://doi.org/10.3390/sym11070912 .
Chen Y. Voltages prediction algorithm based on LSTM recurrent neural network. Optik. 2020;220:164869. https://doi.org/10.1016/j.ijleo.2020.164869 .
Bai C. AGA-GRU: An Optimized GRU Neural Network Model Based on Adaptive Genetic Algorithm. J Phys: Conf Ser. 2020;1651(1):012146.
Arafet K, Berlanga R. Digital Twins in Solar Farms: An Approach through Time Series and Deep Learning. Algorithms. 2021;14(5):156. https://doi.org/10.3390/a14050156 .
Ramos P, Oliveira J. A Procedure for Identification of Ap- propriate State Space and ARIMA Models Based on Time-Series Cross-Validation. Algorithms. 2016;9(4):76. https://doi.org/10.3390/a9040076 .
Nivethitha S, Raman G, Ramamritham K. A deep learning framework for building energy consumption forecast. Renew Sustain Energy Rev. 2021. https://doi.org/10.1016/j.rser.2020.110591 .
Download references
The authors declare that funding is not applicable in this research.
Author information
Authors and affiliations.
Department of EEE, KIET Group of Institutions, Ghaziabad, India
Brijesh Singh, Devansh Kumar Srivastava, Anchal Kumar Singh & Aman Mishra
Department of IT, KIET Group of Institutions, Ghaziabad, India
Jitendra Kumar Seth
You can also search for this author in PubMed Google Scholar
Corresponding author
Correspondence to Jitendra Kumar Seth .
Ethics declarations
Conflict of interest.
There is no conflict of interest. This article does not contain any studies with human participants or animals performed by any of the authors.
Additional information
Publisher’s note.
Springer Nature remains neutral with regard to jurisdictional claims in published maps and institutional affiliations.
Rights and permissions
Springer Nature or its licensor (e.g. a society or other partner) holds exclusive rights to this article under a publishing agreement with the author(s) or other rightsholder(s); author self-archiving of the accepted manuscript version of this article is solely governed by the terms of such publishing agreement and applicable law.
Reprints and permissions
About this article
Singh, B., Seth, J.K., Srivastava, D.K. et al. Investigation for Applicability of Deep Learning Based Prediction Model in Energy Consumption Analysis. SN COMPUT. SCI. 5 , 853 (2024). https://doi.org/10.1007/s42979-024-03221-5
Download citation
Received : 01 July 2023
Accepted : 12 August 2024
Published : 02 September 2024
DOI : https://doi.org/10.1007/s42979-024-03221-5
Share this article
Anyone you share the following link with will be able to read this content:
Sorry, a shareable link is not currently available for this article.
Provided by the Springer Nature SharedIt content-sharing initiative
- Load forecasting
- Recurrent neural network
- Gated recurrent unit
- Deep learning
Advertisement
- Find a journal
- Publish with us
- Track your research
- Open access
- Published: 05 September 2024
Impact of narrative nursing cognition, self-efficacy, and social support on the practices of registered nurses in China: a structural equation modeling analysis
- Li Zhang 1 ,
- Qiang Han 1 ,
- Lin Nan 2 &
- Huiyun Yang 3
BMC Nursing volume 23 , Article number: 624 ( 2024 ) Cite this article
Metrics details
Narrative nursing is a novel approach according with humanistic care, which has been shown to be effective in improving health outcomes for both patients and nurses. Nevertheless, few studies have investigated the status of narrative nursing practice among nurses, and a comprehensive understanding of factors influencing this practice remains elusive.
This was an observational, cross-sectional study using convenience sampling method.
After obtaining the informed consent, a total of 931 registered nurses from three hospitals in China were investigated. Data were collected using the Social Support Rating Scale, the General Self-efficacy Scale, and the Knowledge-Attitude-Practice Survey of Clinical Nurses on Narrative Nursing. All the scales were validated in the Chinese population. The questionnaire results were verified by an independent investigator. Factors influencing narrative nursing practice were determined through a series of analyses, including independent sample t-tests, one-way ANOVA, and Pearson correlations. Subsequently, path analysis was performed and a structural equation model was established.
The score of narrative nursing practice in this study was 30.26 ± 5.32. The structural equation model showed a good fit, with a Root Mean Square Error of Approximation (RMSEA) of 0.007 (90%CI: 0.000, 0.047). Both social support and narrative nursing attitude could directly affect narrative nursing practice (βsocial support = 0.08, P < 0.001; βattitude = 0.54, P < 0.001) and indirectly influence it via self-efficacy ( β social support = 0.04, P < 0.001; β attitude = 0.06, P < 0.001). In addition, narrative nursing knowledge ( β = 0.08, P < 0.001) and the nurses’ growth environment ( β =-0.06, P < 0.001) also affected the practice of narrative nursing.
Narrative nursing in China is at a medium level and could be influenced by several personal and environmental factors. This study highlighted the critical role of nursing management in the advancement of narrative nursing practices. Nurse managers should prioritize specialized training and cultivate supportive environments for nurses to improve their narrative nursing practices.
Peer Review reports
Nurses are the largest group of healthcare professionals, and their initial assessment and subsequent care are critical for favorable health outcomes [ 1 ]. Humanistic care is the cornerstone of nursing practice [ 2 ], which is also a vital approach to promoting global health, according to the statements by the International Council of Nurses [ 3 ]. However, with the rise of science-centered medicine, the emphasis on humanistic care has diminished [ 4 ]. Research has revealed that 76.9% of medical disputes arise from a lack of humanistic care [ 5 ], which can adversely affect both patients and nurses/nurse practitioners. For instance, patients who do not receive sufficient humanistic care may feel insecure, express dissatisfaction with hospitalization, and encounter strained doctor-patient relationships [ 6 ]. Nurses, on the other hand, may suffer from anxiety and depression [ 7 ] and experience job burnout [ 8 ]. Therefore, practicing humanistic care has extremely important significance.
Narrative nursing is a key practice in humanistic care, which aims to provide patient- and family-centered care by interpreting narrative tales and integrating knowledge, beliefs, and values within the nurse-patient interaction context [ 9 ]. Narrative nursing can be augmented by focusing on the analysis of unfolding case studies, utilizing interactive teaching methods, and incorporating the practical realities of nursing care [ 10 ]. Storytelling is the core technique of narrative nursing, which requires nurses to continuously inquire about the issues encountered by patients and families and assess their needs and preferences for patient- and family-centered care [ 9 ]. Narrative nursing is considered successful when patients and their families feel cared for. Narrative nursing helps patients cope with disease and restores their meaning in life through focused listening and reactive nursing practices [ 11 ]. Patients receiving narrative nursing reported calmness of mind [ 12 ], relief from depression and anxiety [ 13 ], and improvement in their quality of life [ 14 ]. Additionally, narrative nursing has the potential to reduce nurses’ work-related stress [ 15 ] and mitigate the ethical challenges faced in clinical practice [ 16 ].
Although narrative nursing has obvious advantages, the implementation process still faces a series of challenges, such as the lack of standardized clinical nurse-patient communication models and significant differences in mutual understanding levels between nurses and patients [ 17 ]. To address these issues, it is imperative to identify the potential factors influencing the effectiveness of narrative nursing and develop targeted strategies accordingly.
According to Bandura’s theory, personal cognition, which encompasses knowledge and attitudes, directly affects behavioral activities by predicting and modulating behavior [ 18 ]. Previous research corroborates this finding, demonstrating that healthcare professionals’ cognitive attitudes toward trauma-informed care practices positively impact their implementation of such practices [ 19 ]. In this study, nurses’ personal cognition of their knowledge and attitudes toward narrative nursing were also expected to influence their narrative nursing practices. In addition, a positive attitude towards narrative nursing can boost nurses’ confidence in their capabilities, indirectly enhancing narrative nursing practices through increased self-efficacy [ 20 ]. Therefore, self-efficacy may serve as a mediating factor in the relationship between nurses’ attitudes toward narrative nursing and their narrative nursing practices.
Social support also influenced narrative nursing practices. Social support, defined as emotional and tangible assistance provided by family, friends, colleagues, and other significant individuals [ 21 ], is understood through main and buffering effect models [ 22 ]. The main effects model suggests that social support invariably benefits individuals, regardless of their stress or distress levels. Research has shown that individuals who receive social support are less likely to experience compassion fatigue and can manage stress more effectively to promote narrative nursing [ 23 ]. Furthermore, high social support can help nursing personnel proactively prevent adverse performances and maintain a more positive emotional experience, effectively mitigating the physical and psychological impacts of occupational stress [ 24 ]. By enhancing nurses’ self-efficacy through positive experiences, social support can indirectly improve the quality and efficiency of narrative nursing [ 25 ]. Thus, theoretically, social support may indirectly influence narrative nursing practices through self-efficacy. Thus, we hypothesized that the practice of narrative nursing is contingent upon the synergistic effects of personal factors, such as knowledge and attitude regarding narrative nursing, and environmental factors, such as social support, which indirectly affect narrative nursing practice through self-efficacy. A theoretical model illustrating these relationships is presented in Fig. 1 .
Due to that the existing knowledge gap regarding the current state and factors influencing narrative nursing practices, we conducted this cross-sectional study to primarily understand these aspects, offering empirical evidence for subsequent longitudinal and intervention-based studies. This study aimed to identify and understand how personal factors, such as knowledge and attitude, and environmental elements, such as social support, influence the practice of narrative nursing.
Three hypotheses were proposed: (1) personal factors, including both knowledge and attitude, directly impact the practice of narrative nursing, (2) environmental factors, such as social support, also directly affect the practice of narrative nursing, and (3) self-efficacy serves as a mediator between personal and environmental factors that influence the practice of narrative nursing.

Hypothetical model of the relationships among personal factors, environmental factors, and narrative nursing practice
Study design and participants
This study was designed as a multi-center cross-sectional investigation using convenience sampling to obtain sufficient samples for developing our model. From March to October 2023, we surveyed 1091 nurses at three hospitals in Shanxi Province, China, and collected 931 questionnaires with a response rate of 85.34%. Nurses meeting the following criteria were invited to participate: (1) possession of a valid nursing practice license, (2) a minimum of one year of nursing experience providing care to sober patients, and (3) voluntary commitment to participate in this study. Individuals who (1) were not scheduled for duty during the survey period, (2) did not directly engage in nursing services (e.g., nurse managers), and (3) were advanced students and interns were excluded. All the participants provided written informed consent.
Setting and procedure
Having secured the consent of the nurse managers and nursing supervisors at each selected hospital, an electronic questionnaire was developed to facilitate an online survey using Sojump. Sojump is the most commonly used online survey platform in China and offers features such as data encryption, server stability, automated backups, and user-friendly interface. To prevent duplicate responses, the questionnaire was designed to allow for only one response per unique cell phone number or IP address. All the questions were configured as mandatory to ensure that the survey could not be submitted with any missing responses. Prior to the official survey launch, comprehensive training materials were provided to nursing leaders at the three hospitals, outlining the study’s objectives and importance, and detailing the precautions and response methods to be employed when completing the questionnaire. Subsequently, the survey data were verified by independent data processors to ensure completeness and reliability of the questionnaire.
Ethical considerations
This study adhered to the principle of the Declaration of Helsinki. Ethical approval was granted by the Medical Ethics Committee of The Second Affiliated Hospital of Xi’an Jiaotong University (Approval No. 2023004). Before the commencement of the investigation, participants were given an informed consent form. They were thoroughly informed of the voluntary and confidential nature of their involvement in this study. Emphasis was placed on the fact that their participation was entirely optional and that they had the unconditional right to withdraw from the study at any point without consequences or explanation. Additionally, they were assured that all collected data would remain anonymous and that their privacy would be strictly protected throughout the research process.
Sample size
According to Comrey and Lee [ 26 ], a sample size of approximately 300 is considered adequate for factor analysis. To further assess the statistical power, we conducted a post hoc analysis using G*Power 3.1 software to determine whether the sample size was sufficient. The analysis revealed that with 931 participants, a power of 100% was achieved at a significance level of 0.05 (two-side).
Social-demographic characteristics
A self-designed questionnaire was initially developed based on a literature review and considerations of clinical significance and was subsequently pilot tested by an independent statistician. A questionnaire was used to collect sociodemographic characteristics, including gender, age, marital status, working department, working years, educational level, growth environment, monthly income (RMB), and job satisfaction. Specifically, the growth environment was categorized as urban or rural, which referred to the places where the participants were born and raised.
Knowledge-attitude-practice survey of clinical nurses on narrative nursing
The Knowledge-Attitude-Practice Survey of Clinical Nurses on Narrative Nursing was used to evaluate the narrative nursing practice, knowledge and attitude of participants in this study [ 27 ]. The questionnaire consists of three subscales: practice (8 items), knowledge (6 items), and the attitude (8 items). Each item was rated on a scale of 1 to 5 points, based on a 5-level Likert scale scoring method. The total scores for Practice, Knowledge, and Attitude were to 8–40 points, 6–30 points, and 8–40 points, respectively. A higher score indicated a higher the level of practice, knowledge, or attitude towards narrative nursing. This scale has revealed good reliability and validity in previous study (Cronbach’s α = 0.844) [ 28 ], and in this study, the Cronbach’s α for practice, knowledge, attitude subscales were 0.835, 0.735, 0.891, respectively.
Perceived social support rating scale
The Perceived Social Support Rating Scale was employed to evaluate the social support received by nurses [ 29 ]. This scale comprises three dimensions: subjective, objective, and support utilization. It comprises 10 items, each item is scored on a scale from 1 to 7, with higher scores indicating a greater level of social support. This scale has demonstrated strong validity and reliability in the Chinese population (Cronbach’s α = 0.949) [ 30 ]. The Cronbach’s α of the scale in this study was 0.806.
General self-efficacy scale
The Chinese version of the General Self-efficacy Scale was used to evaluate nurses’ confidence in their capacity to participate in narrative approaches [ 31 ]. This scale consists of a single dimension with10 items. Each item is rated on a scale of 1–4, allowing for a total possible score range of 10–40 points. This scale has been validated in a Chinese population, with a previous study reporting a Cronbach’s α of 0.903 [ 32 ]. The Cronbach’s α of the scale in this study was 0.941.
Data analysis
Statistical analysis was conducted using IBM SPSS version 26.0 and AMOS version 20. The normality of the variables was evaluated by assessing the skewness and kurtosis. The variables were considered to be normally distributed if the skewness value was less than or equal to 2 or the kurtosis value was less than or equal to 4 [ 33 ]. All continuous data in our study adhered to a normal distribution. Thus, parametric tests were conducted. Specifically, independent sample t-tests (dichotomous variables), one-way ANOVA (polytomous variables), and Pearson’s correlations (continuous variables) were performed. Variables that exhibited significant differences during the univariate analysis ( P < 0.2) [ 34 ] and potential factors identified through clinical experience were incorporated into the structural equation model. The structural equation model was conducted using bootstrap resampling techniques to detect direct and indirect relationships among the factors were analyzed, presenting standardized regression coefficients ( β ) and their corresponding p-values for direct, indirect, and total effects. Variables with non-significant coefficients were excluded from the model. Model fit was assessed using appropriate model fit indices [ 35 ]: a relative chi-square (χ2/df) test of less than 3 (indicates a good fit if P > 0.05); adjusted goodness-of-fit index (AGFI) (adjusted measure of how well the model fits the data); comparative fit index (CFI) (compares the fit of the proposed model to a null model); normative fit index (NFI) (compares the fit of the model to a model where all variables are uncorrelated); incremental fit index (IFI) (similar to CFI, accounts for the degrees of freedom); and Tucker-Lewis Index (TLI) (like CFI, assesses model fit); all above 0.90, goodness-of-fit index (GFI) (overall measure of how well the model fits the data) above 0.80, and Root Mean Square Error of Approximation (RMSEA) (estimates the error of approximation in the model) less than 0.08. A p-values of less than 0.05 (two-tailed) were considered statistically significant.
A total of 931 participants were included in this study, with a slightly higher proportion of middle-aged individuals. Most participants held a bachelor’s degree or higher (81.95%), and their monthly income was mainly less than 5,000 RMB (46.19%). The other details are presented in Table 1 .
Univariate analysis
The independent sample t-test showed that there were significant differences in growth environment (MD = 0.94, 95%CI: 0.26 ~ 1.62, t = 2.71, P = 0.007) and having experience of hospitalized or accompanied (MD = 1.39, 95%CI: 0.55 ~ 2.23, t = 3.23, P = 0.001). For the one-way ANOVA, age ( F = 4.08, P = 0.017), working years ( F = 4.97, P = 0.007), workload ( F = 6.46, P = 0.002), job satisfaction ( F = 26.14, P < 0.001), and narrative nursing contact were significant factors ( F = 25.17, P < 0.001) (Table 1 ). These factors were used to validate of the structural equation model.
Correlations between variables
Correlations between the measurement variables were analyzed using Pearson’s correlation coefficient analysis (Table 2 ). We observed positive correlations among all the individual variables. In this study, the correlation coefficient between the latent variables ranged from 0.20 to 0.68, and the squared value was smaller than every AVE value; thus, discriminant validity was secured. Narrative nursing knowledge ( r = 0.41, P < 0.001), narrative nursing attitude ( r = 0.68, P < 0.001), social support ( r = 0.35, P < 0.001), and self-efficacy ( r = 0.43, P < 0.001) were statistically significant.
Structural equation model
Table 3 details the model’s results, revealing that nurses’ attitudes toward narrative nursing indirectly influenced narrative nursing practice through self-efficacy, with a desirable fitting index (Table 4 ). This relationship involved a direct effect of 0.54 ( P < 0.001), an indirect effect of 0.06, and a total effect of 0.60 ( P < 0.001). Knowledge of narrative nursing directly influenced narrative nursing practice with an effect of 0.08 ( P < 0.001). Social support has an indirect impact on narrative nursing practice through self-efficacy, with a direct effect of 0.08 ( P < 0.001), an indirect effect of 0.04, and a total effect of 0.12 ( P < 0.001). In addition, the growth environment was also associated with narrative nursing practice ( β =-0.06, P < 0.01). The refined model is presented in Fig. 2 .

Hypothesized Model of narrative nursing knowledge, narrative nursing attitude, social support, self-efficacy, growth environment, and narrative nursing practice
Summary of main results
The average score for nurses’ narrative nursing practice in this study was 30.26 ± 5.32, slightly higher than the previous study that investigated a total of 1993 nurses in China (28.27 ± 5.48) [ 36 ]. The structural equation model showed that narrative nursing attitudes and social support directly affected narrative nursing practice and indirectly influenced it via self-efficacy. In addition, narrative nursing knowledge and the growth environment were found to have an impact on narrative nursing practice. This study clarified the relationships between personal and environmental factors and narrative nursing practice, thus providing further insight into factors that may improve narrative nursing practice.
Model results
Attitude is central to the daily implementation of humanistic care, while knowledge provides basic theoretical guidance for nurses to engage in humanistic care practices [ 37 ]. In previous empirical research, both attitude and knowledge were found to significantly affect nursing practices, including the prevention of catheter-associated tract infections [ 38 ] and medical device-related pressure injuries [ 39 ]. This study confirmed that both narrative nursing knowledge and attitude could directly affect the practice of narrative nursing, thus verifying Hypothesis 1.
It has been proved that self-efficacy is intricately linked to the humanistic practices of narrative nursing [ 40 ]. This study further found that self-efficacy acted as a mediating factor in narrative nursing attitudes and practice, verifying our hypothesis 3. One possible mechanism is that self-efficacy is a dynamic process that can be modified by attitude, knowledge, feedback, and experience [ 41 ]. Once nurses gained higher levels of self-efficacy, they can effectively use their skills to accomplish challenging tasks [ 42 ]. Therefore, it is highly advisable to intensify and prioritize training and educational programs focused on narrative nursing, as this will significantly bolster nurses’ self-efficacy in engaging in narrative nursing practices.
Consistent with previous research findings [ 43 ], this study confirmed that social support can directly affect narrative nursing practice. Additionally, it could indirectly influence narrative nursing practice through self-efficacy, thus verifying hypotheses 2 and 3. Previous studies have highlighted that a strong social support network can provide emotional bolstering, guidance, and tangible assistance, which can exert a positive influence on an individual’s beliefs, performance, and overall functioning [ 43 ]. Hospital nurses who receive greater social support may experience higher personal achievement and lower levels of emotional exhaustion and burnout [ 44 ], which could increase job satisfaction and promote humanistic care [ 45 ]. In particular, social support within the workplace, such as advocacy for narrative nursing and the development of narrative nursing skills, can effectively increase the humanistic qualities of nursing staff and promote the practice of narrative nursing [ 46 ]. In addition, healthy workplace characteristics, such as harmonious nurse–physician relationships, may influence work performance [ 47 ]. These findings underscore the importance of reinforcing social support and nurturing nursing staff’s enthusiasm for narrative nursing, which can significantly enhance the quality of narrative nursing practice.
Notably, this study identified an association between nurses’ growth environment and their practice of narrative nursing, with nurses in urban areas exhibiting better narrative nursing practices, which has not been clearly stated in previous studies. A large-scale longitudinal study of American adults spanning various stages of adulthood, has found that urban-rural differences in the expression of these five personality traits [ 48 ]. City dwellers tend to score higher on cheerfulness and mental health [ 48 ], which are significant factors that affect the execution of humanistic care [ 45 ]. This could explain the findings of the present study. Accordingly, it is imperative that nursing managers focus on cultivating positive personality traits in nurses and promoting their psychological well-being. Training programs should be tailored to cater to a diverse range of nurse personalities while also enhancing their professional knowledge and fostering a deeper passion for nursing. Thus, the provision of humanistic care may be improved, encouraging narrative nursing practice.
Limitations
This study has several limitations. First, although we analyzed the research data based on a theoretical model, the cross-sectional design limited the inference of causality. Additional longitudinal and intervention studies are necessary to validate these findings. Second, despite being a multicenter study, we only included hospitals from one province. Therefore, questions might be raised about how the results can be generalized to other regions or countries. Future research should aim to replicate our study in different regions or countries to assess the consistency of our findings and determine the extent to which they can be generalized. Third, the variables in this study were measured using self-report questionnaires, and the results may have been overestimated, underestimated, or deviated.
Conclusions
This study identified crucial factors from both the personal and environmental perspectives that could directly or indirectly influence narrative nursing practice. The path relationships in our model highlight the necessity of a comprehensive strategy to promote the practice of narrative nursing. This includes specialized training that enhances their knowledge and skills in narrative care, as well as the creation of a supportive environment that encourages and facilitates the implementation of humanistic care. The findings offer a compelling rationale for policymakers and nurse managers to integrate narrative nursing into healthcare settings. In doing so, they can contribute to the provision of patient-centered care that is compassionate, effective, and aligned with the principles of narrative nursing.
Implications for future research
The global healthcare sector has long faced challenges stemming from the scarcity and unequal distribution of nursing personnel [ 49 ]. In 2022, the registered nurses in China only covered 3.7 per 1,000 individuals [ 50 ]. This scarcity has seriously affected the quality of both clinical care and humanistic care [ 51 ]. The findings suggest that a multifaceted approach should be developed to promote the practice of narrative nursing in China. First, it is recommended that nursing programs tailored to narrative nursing, including art-based narrative training [ 52 ] and reflective practice [ 10 ] to improve nurses’ knowledge of narrative nursing and foster an awareness and a desire to engage in such practices. These practices should be integrated into undergraduate and postgraduate nursing curricula to ensure a solid foundation for narrative nursing. In addition, simulation training for narrative nursing listening [ 53 ] and the use of narrative nursing case studies [ 54 ] can enhance nurses’ self-efficacy in implementing narrative nursing. By providing hands-on experience and practical examples, these methods enable nurses to apply narrative techniques in controlled environments, thereby enhancing their confidence in their abilities [ 10 ]. Moreover, within medical settings, fostering a supportive environment is crucial for promoting the practice of narrative nursing [ 55 ]. Therefore, nursing managers should provide more support for nurses. These include providing resources, addressing concerns, and creating a supportive environment that values and encourages narrative nursing. Subsequently, a narrative-based nursing model should be developed to emphasize the concept of patient-centered care [ 56 ], while also incorporating the considerations of cost-effectiveness.
Data availability
The datasets generated and/or analyzed during the current study are available from the corresponding author on reasonable request.
World Health Organization. (2024). Nursing and Midwifery. https://www.who.int/news-room/fact-sheets/detail/nursing-and-midwifery . Assessed 10 August 2024.
Watson J. The theory of human caring: retrospective and prospective. Nurs Sci Q. 1997;10(1):49–52. https://doi.org/10.1177/089431849701000114 .
Article CAS PubMed Google Scholar
International Council of Nurses. (2024). Nurses: A voice to lead. https://www.icn.ch/sites/default/files/inlinefiles/ICN_IND_Toolkit_English_FINAL_low%20res_0.pdf . Assessed 11 August 2024.
McCaffrey G. A humanism for nursing? Nurs Inq. 2019;26(2):e12281. https://doi.org/10.1111/nin.12281 .
Article PubMed Google Scholar
Chen G. The effective reduction of violence against doctors through the improvement of medical humanistic care. J Multidisciplinary Healthc. 2023;16:1403–7. https://doi.org/10.2147/JMDH.S411674 .
Article Google Scholar
Panagioti M, Geraghty K, Johnson J, Zhou A, Panagopoulou E, Chew-Graham C, Peters D, Hodkinson A, Riley R, Esmail A. Association between physician burnout and patient safety, professionalism, and patient satisfaction: a systematic review and Meta-analysis. Jama Intern Med. 2018;178(10):1317–31.
Article PubMed PubMed Central Google Scholar
Maharaj S, Lees T, Lal S. Prevalence and risk factors of depression, anxiety, and stress in a cohort of Australian nurses. Int J Environ Res Public Health. 2018;16(1):61. https://doi.org/10.3390/ijerph16010061 .
Shen A, Wang Y, Qiang W. A multicenter investigation of caring behaviors and burnout among oncology nurses in China. Cancer Nurs. 2020;43(5):E246–53. https://doi.org/10.1097/NCC.0000000000000680 .
Horton-Deutsch S, Sherwood G. Reflective practice: transforming education and improving outcomes. Indianapolis, IN: Sigma Theta Tau International; 2017.
Google Scholar
Fitzpatrick JJ. Narrative nursing: applications in practice, Education, and Research. Appl Nurs Res. 2017;37:67. https://doi.org/10.1016/j.apnr.2017.08.005 .
Zaharias G. What is narrative-based medicine? Narrative-based medicine. Can Fam Physician. 2018;64(3):176–80.
PubMed PubMed Central Google Scholar
Wise M, Marchand LR, Roberts LJ, Chih MY. Suffering in advanced cancer: a randomized control trial of a narrative intervention. J Palliat Med. 2018;21(2):200–7. https://doi.org/10.1089/jpm.2017.0007 .
Xu H, Xu G, Liu Y, Mu X, Liu Y, Hu H. Effect of narrative nursing intervention based on targeted nursing intervention on anxiety and nursing satisfaction of patients with malignant tumors undergoing chemotherapy. J Healthc Eng. 2021;2021(4438446). https://doi.org/10.1155/2021/4438446 .
Siverová J, Bužgová R. The effect of reminiscence therapy on quality of life, attitudes to ageing, and depressive symptoms in institutionalized elderly adults with cognitive impairment: a quasi-experimental study. Int J Ment Health Nurs. 2018;27(5):1430–9. https://doi.org/10.1111/inm.12442 .
Williams-Reade J, Freitas C, Lawson L. Narrative-informed medical family therapy: using narrative therapy practices in brief medical encounters. Families Syst Health. 2014;32(4):416–25. https://doi.org/10.1037/fsh0000082 .
Saeedi S, Jouybari L, Sanagoo A, Vakili MA. The effectiveness of narrative writing on the moral distress of intensive care nurses. Nurs Ethics. 2019;26(7–8):2195–203. https://doi.org/10.1177/0969733018806342 .
Höglander J, Holmström IK, Lövenmark A, Van Dulmen S, Eide H, Sundler AJ. Registered nurse-patient communication research: an integrative review for future directions in nursing research. J Adv Nurs. 2023;79(2):539–62. https://doi.org/10.1111/jan.15548 .
Bandura A. Self-efficacy: toward a unifying theory of behavioral change. Psychol Rev. 2001;84(2):191–215. https://doi.org/10.1037/0033-295x.84.2.191 .
Bruce MM, Kassam-Adams N, Rogers M, Anderson KM, Sluys KP, Richmond TS. Trauma providers’ knowledge, views, and practice of trauma-informed care. J Trauma Nurs. 2018;25(2):131–8. https://doi.org/10.1097/JTN.0000000000000356 .
Antonini M, Bellier-Teichmann T, O’reilly L, Cara C, Brousseau S, Weidmann J, Roulet-Schwab D, Ledoux I, Konishi M, Pasquier J, Delmas P. Effects of an educational intervention to strengthen humanistic practice on haemodialysis nurses’ caring attitudes and behaviours and quality of working life: a cluster randomised controlled trial. Bmc Nurs. 2021;20(1):255. https://doi.org/10.1186/s12912-021-00729-6 .
Zimet GD, Powell SS, Farley GK, Werkman S, Berkoff KA. Psychometric characteristics of the multidimensional scale of perceived social support. J Pers Assess. 1990;55(3–4):610–7. https://doi.org/10.1080/00223891.1990.9674095 .
Cohen S, Wills TA. Stress, social support, and the buffering hypothesis. Psychol Bull. 1985;98(2):310–57.
Liu Y, Aungsuroch Y, Gunawan J, Zeng D. Job stress, psychological capital, perceived social support, and occupational burnout among hospital nurses. J Nurs Scholarsh. 2021;53(4):511–8. https://doi.org/10.1111/jnu.12642 .
Fallah N, Abdolahzadeh F, Lotfi B. Examining the interconnections among entrepreneurial passion, need for achievement, social support, entrepreneurial self-efficacy, and entrepreneurial intention among foreign language learners: a moderated mediation model. Entrepreneurship Educ. 2023;6(3):319–38.
Liu Y, Aungsuroch Y. Work stress, perceived social support, self-efficacy and burnout among Chinese registered nurses. J Nurs Adm Manag. 2019;27(7):1445–53. https://doi.org/10.1111/jonm.12828 .
Comrey AL, Lee HB. A first course in factor analysis. Lawrence Erlbaum Associates; 1992.
Zhang L, Nan L, Han Q, Xue J, Dou Y, Li J. Analysis of the status quo and influencing factors of narrative nursing knowledge, attitude and behavior among nurses in tertiary hospitals. Chin Med Ethics. 2023;36(8):897–903. https://doi.org/10.12026/j.issn.1001-8565.2023.08.12 . (in Chinese).
Liao F, Hou M, Lin B, Li P. Analysis of the status quo and influencing factors of narrative nursing knowledge, attitude and practice of oncology nurses. Mod Clin Nurs. 2021;20(08):17–22.
Nolten PW. Conceptualization and measurement of social support: the development of the student social support scale. The University of Wisconsin-Madison; 1994.
Liu JW, Li FY, Lian YL. Reliability and validity of social support rating scale. J Xinjiang Med Univ. 2008;1:4–6. (in Chinese).
Zhang JX, Schwarzer R. Measuring optimistic self-beliefs: a Chinese adaptation of the general self-efficacy scale. Psychologia. 1995;38:174–81.
Cheng L, Ye Y, Zhong Z, Zhang F, Hu X, Cui R, Chen Q. Mediating effects of general self-efficacy on the relationship between the source of meaning in life and prosocial behaviours in vocational college nursing students: a cross-sectional study. PLoS ONE. 2020;15(12):e0243796. https://doi.org/10.1371/journal.pone.0243796 .
Article CAS PubMed PubMed Central Google Scholar
Kim JS, Kim J, Gelegjamts D. Knowledge, attitude and self-efficacy towards palliative care among nurses in Mongolia: a cross-sectional descriptive study. PLoS ONE. 2020;15(7):e0236390. https://doi.org/10.1371/journal.pone.0236390 .
Maldonado G, Greenland S. Simulation study of confounder-selection strategies. Am J Epidemiol. 1993;138(11):923–36.
Hooper D, Coughlan J, Mullen M. (2008). Structural equation modelling: Guidelines for determining model fit structural equation modelling: Guidelines for determining model fit. Electron J Bus Res Methods. 2008; 6 : 53–60. View Article.
Li XZ, Zhou BZ, Wang NY. Investigation on nurses’ cognition condition and influence factors about the narrative nursing in the tertiary hospitals. J Mod Med Health. 2024;40(4):634–8. (in Chinese).
Delmas P, O’Reilly L, Iglesias K, Cara C, Burnier M. Feasibility, acceptability, and preliminary effects of educational intervention to strengthen humanistic practice among hemodialysis nurses in the Canton of Vaud, Switzerland: a pilot study. Int J Hum Caring. 2016;20(1):31–43.
Mong I, Ramoo V, Ponnampalavanar S, Chong MC, Wan Nawawi WNF. Knowledge, attitude and practice in relation to catheter-associated urinary tract infection (CAUTI) prevention: a cross-sectional study. J Clin Nurs. 2022;31(1–2):209–19. https://doi.org/10.1111/jocn.15899 .
Zhang YB, He L, Gou L, Pei JH, Nan RL, Chen HX, Wang XL, Du YH, Yan H, Dou XM. Knowledge, attitude, and practice of nurses in intensive care unit on preventing medical device-related pressure injury: a cross-sectional study in western China. Int Wound J. 2021;18(6):777–86. https://doi.org/10.1111/iwj.13581 .
Bu M, Ma H, Zhai H, Ma Y, Xu N. Role of self-efficacy in nursing organizational climate: a way to develop nurses’ humanistic practice ability. J Nurs Adm Manag. 2022;30(7):2107–15. https://doi.org/10.1111/jonm.13516 .
Croy G, Garvey L, Willetts G, Wheelahan J, Hood K. Anxiety, flipped approach and self-efficacy: exploring nursing student outcomes. Nurse Educ Today. 2020;93:104534. https://doi.org/10.1016/j.nedt.2020.104534 .
Kim SM, Kim JH, Kwak JM. Psychometric properties of the Korean version of the nursing profession self-efficacy scale. J Nurs Res. 2022;30(2):e197. https://doi.org/10.1097/jnr.0000000000000481 .
Patterer AS, Yanagida T, Kühnel J, Korunka C. Daily receiving and providing of social support at work: identifying support exchange patterns in hierarchical data. Eur J Work Organizational Psychol. 2023;32(4):489–505.
Al-Hamdan Z, Bani Issa H. The role of organizational support and self-efficacy on work engagement among registered nurses in Jordan: a descriptive study. J Nurs Adm Manag. 2022;30(7):2154–64. https://doi.org/10.1111/jonm.13456 .
Liu X, Zhu H. Influencing factors of humanistic care ability and its dimensions among mental health workers during the COVID-19 pandemic: an online cross-sectional study. BMC Psychiatry. 2023;23(1):186. https://doi.org/10.1186/s12888-023-04656-5 .
Zhang T, Zhang L, Wen X, Li H. Level of humanistic care ability and its influencing factors among nursing aides in long-term care facilities. Geriatr Nurs. 2023;52:56–62. https://doi.org/10.1016/j.gerinurse.2023.05.007 .
Kittel AFD, Kunz RAC, Seufert T. Self-regulation in informal workplace learning: influence of organizational learning culture and job characteristics. Front Psychol. 2021;12:643748. https://doi.org/10.3389/fpsyg.2021.643748 .
Atherton OE, Willroth EC, Graham EK, Luo J, Mroczek DK, Lewis-Thames MW. Rural-urban differences in personality traits and well-being in adulthood. J Pers. 2024;92(1):73–87. https://doi.org/10.1111/jopy.12818 .
Anthony M. Editorial: nursing shortage. Home Healthc Now. 2024;42(1):5. https://doi.org/10.1097/NHH.0000000000001233 .
China Health Statistics Yearbook. (2022). http://www.nhc.gov.cn/mohwsbwstjxxzx/tjtjnj/202305/6ef68aac6bd14c1eb9375e01a0faa1fb/files/b05b3d958fc546d98261d165cea4adba.pdf Assessed 14 April 2024.
Ross J. Nursing shortage creating patient safety concerns. J Perianesthesia Nurs. 2022;37(4):565–7. https://doi.org/10.1016/j.jopan.2022.05.078 .
Laranjeira C, Carvalho P, Querido A. Digital Storytelling: art-based pedagogy to improve professional development for nurses. J Contin Educ Nurs. 2021;52(10):454–6. https://doi.org/10.3928/00220124-20210913-04 .
Orr FR. (2017). I know how it feels: A voice-hearing simulation to enhance nursing students’ empathy and self-efficacy (Doctoral dissertation).
Chiona BC, Msiska M. The self-efficacy of Malawian nursing educators towards the use of case study teaching method. Malawi Med J. 2023;35(2):89–94. https://doi.org/10.4314/mmj.v35i2.3 .
Chang WP. How social support affects the ability of clinical nursing personnel to cope with death. Appl Nurs Res. 2018;44:25–32. https://doi.org/10.1016/j.apnr.2018.09.005 .
Artioli G, Foà C, Taffurelli C. An integrated narrative nursing model: towards a new healthcare paradigm. Acta bio-medica. 2016;87(4–S):13–22.
PubMed Google Scholar
Download references
This study was funded by the National Natural Science Foundation of China (No. 22BGL317).
Author information
Authors and affiliations.
Department of Geriatric Digestive Surgery, The Second Affiliated Hospital of Xi’an Jiaotong University, Xi’an, Shaanxi Province, China
Li Zhang & Qiang Han
Cancer Center, The First Affiliated Hospital of Xi’an Jiaotong University, Xi’an, Shaanxi Province, China
Nursing Department, The Second Affiliated Hospital of Xi’an Jiaotong University, Xi’an, Shaanxi Province, China
Huiyun Yang
You can also search for this author in PubMed Google Scholar
Contributions
L Z conceptualized and designed the study, developed the methodology, implemented software tools, and drafted the original manuscript. Q H and L N conducted investigations, curated data, contributed to conceptualization and methodology, and drafted the original manuscript. HY provided supervision, validated the findings, and contributed to writing, reviewing, and editing the manuscript. All authors have approved the final draft.
Corresponding author
Correspondence to Huiyun Yang .
Ethics declarations
Ethical approval and consent to participate, consent for publication.
Not applicable.
Competing interests
The authors declare no competing interests.
Additional information
Publisher’s note.
Springer Nature remains neutral with regard to jurisdictional claims in published maps and institutional affiliations.
Rights and permissions
Open Access This article is licensed under a Creative Commons Attribution-NonCommercial-NoDerivatives 4.0 International License, which permits any non-commercial use, sharing, distribution and reproduction in any medium or format, as long as you give appropriate credit to the original author(s) and the source, provide a link to the Creative Commons licence, and indicate if you modified the licensed material. You do not have permission under this licence to share adapted material derived from this article or parts of it. The images or other third party material in this article are included in the article’s Creative Commons licence, unless indicated otherwise in a credit line to the material. If material is not included in the article’s Creative Commons licence and your intended use is not permitted by statutory regulation or exceeds the permitted use, you will need to obtain permission directly from the copyright holder. To view a copy of this licence, visit http://creativecommons.org/licenses/by-nc-nd/4.0/ .
Reprints and permissions
About this article
Cite this article.
Zhang, L., Han, Q., Nan, L. et al. Impact of narrative nursing cognition, self-efficacy, and social support on the practices of registered nurses in China: a structural equation modeling analysis. BMC Nurs 23 , 624 (2024). https://doi.org/10.1186/s12912-024-02292-2
Download citation
Received : 03 June 2024
Accepted : 26 August 2024
Published : 05 September 2024
DOI : https://doi.org/10.1186/s12912-024-02292-2
Share this article
Anyone you share the following link with will be able to read this content:
Sorry, a shareable link is not currently available for this article.
Provided by the Springer Nature SharedIt content-sharing initiative
- Narrative nursing
- Self-efficacy
- Social support
- Registered nurses
BMC Nursing
ISSN: 1472-6955
- General enquiries: [email protected]
Information
- Author Services
Initiatives
You are accessing a machine-readable page. In order to be human-readable, please install an RSS reader.
All articles published by MDPI are made immediately available worldwide under an open access license. No special permission is required to reuse all or part of the article published by MDPI, including figures and tables. For articles published under an open access Creative Common CC BY license, any part of the article may be reused without permission provided that the original article is clearly cited. For more information, please refer to https://www.mdpi.com/openaccess .
Feature papers represent the most advanced research with significant potential for high impact in the field. A Feature Paper should be a substantial original Article that involves several techniques or approaches, provides an outlook for future research directions and describes possible research applications.
Feature papers are submitted upon individual invitation or recommendation by the scientific editors and must receive positive feedback from the reviewers.
Editor’s Choice articles are based on recommendations by the scientific editors of MDPI journals from around the world. Editors select a small number of articles recently published in the journal that they believe will be particularly interesting to readers, or important in the respective research area. The aim is to provide a snapshot of some of the most exciting work published in the various research areas of the journal.
Original Submission Date Received: .
- Active Journals
- Find a Journal
- Proceedings Series
- For Authors
- For Reviewers
- For Editors
- For Librarians
- For Publishers
- For Societies
- For Conference Organizers
- Open Access Policy
- Institutional Open Access Program
- Special Issues Guidelines
- Editorial Process
- Research and Publication Ethics
- Article Processing Charges
- Testimonials
- Preprints.org
- SciProfiles
- Encyclopedia
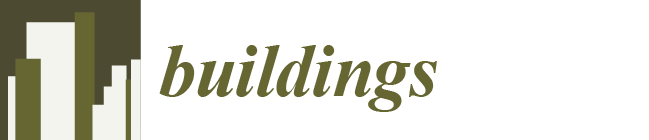
Article Menu
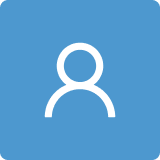
- Subscribe SciFeed
- Recommended Articles
- Google Scholar
- on Google Scholar
- Table of Contents
Find support for a specific problem in the support section of our website.
Please let us know what you think of our products and services.
Visit our dedicated information section to learn more about MDPI.
JSmol Viewer
Research on outlier detection methods for dam monitoring data based on post-data classification, 1. introduction, 2. data set pre-classification, 3. outlier detection after pre-classification of data sets, 3.1. 3-sigma rule for outlier detection, 3.2. k-medoids machine learning algorithm for outlier detection.
K-medoids Machine Learning Algorithm for Outlier Detection |
Input: Data set D = {x1, x2, …, xn}, Number of clusters K, Maximum number of iterations M |
Output: Clustering results clusters, Outliers outliers |
1: Select initial medoids as m ,m ;…,m |
2: Initialize total cost to infinity cost = ∞ |
3: iteration = 1 to M // |
4: For all x∈D and all m∈Medoids, calculate distance d(x,m)//Calculate the distance from each data point to each medoid |
5: For each x∈D, find min d(x,m) and assign x to the corresponding cluster//Assign each data point to the nearest medoid to form clusters |
6: For each cluster C, find the new center m′ = min ∑ d(x,x ) and calculate the cost cost // Recalculate the new center point (medoid) and cost for each cluster |
7: current cost <cost // Compare costs |
8: Update medoids |
9: Update total cost costtotal = ∑cost |
10: |
11: // Terminate if there is no cost improvement |
12: |
13: |
14: Set the threshold for determining outliers // Select based on the actual problem context |
15: Mark those points whose distance from the medoid exceeds the threshold as outliers // Mark outliers |
16: clusters and outliers // Return results |
3.3. Isolation Forest Machine Learning Algorithm for Outlier Detection
3.4. evaluation of outlier detection algorithm matching based on data set pre-classification, 4. conclusions, author contributions, data availability statement, acknowledgments, conflicts of interest.
- Jeon, J.; Lee, J.; Shin, D.; Park, H. Development of dam safety management system. Adv. Eng. Softw. 2009 , 40 , 554–563. [ Google Scholar ] [ CrossRef ]
- Abdelalim, A.M.; Said, S.O.; Alnaser, A.A.; Sharaf, A.; ElSamadony, A.; Kontoni, D.P.N.; Tantawy, M. Agent-Based Modeling for Construction Resource Positioning Using Digital Twin and BLE Technologies. Buildings 2024 , 14 , 1788. [ Google Scholar ] [ CrossRef ]
- Guo, M.; Qi, H.; Zhao, Y.; Liu, Y.; Zhao, J.; Zhang, Y. Design and Management of a Spatial Database for Monitoring Building Comfort and Safety. Buildings 2023 , 13 , 2982. [ Google Scholar ] [ CrossRef ]
- Cai, S.; Li, L.; Li, S.; Sun, R.; Yuan, G. An efficient approach for outlier detection from uncertain data streams based on maximal frequent patterns. Expert Syst. Appl. 2020 , 160 , 113646. [ Google Scholar ] [ CrossRef ]
- Erkuş, E.C.; Purutçuoğlu, V. Outlier detection and quasi-periodicity optimization algorithm: Frequency domain based outlier detection (FOD). Eur. J. Oper. Res. 2021 , 291 , 560–574. [ Google Scholar ] [ CrossRef ]
- Zhou, Z.Y.; Pi, D.C. Data streams oriented outlier detection method: A fast minimal infrequent pattern mining. Int. Arab J. Inf. Technol. 2021 , 18 , 864–870. [ Google Scholar ] [ CrossRef ]
- Huang, Y.; Liu, W.; Li, S.; Guo, Y.; Chen, W. Interpretable Single-dimension Outlier Detection (ISOD): An Unsupervised Outlier Detection Method Based on Quantiles and Skewness Coefficients. Appl. Sci. 2023 , 14 , 136. [ Google Scholar ] [ CrossRef ]
- Cai, S.; Li, L.; Li, Q.; Li, S.; Hao, S.; Sun, R. UWFP-Outlier: An efficient frequent-pattern-based outlier detection method for uncertain weighted data streams. Appl. Intell. 2020 , 50 , 3452–3470. [ Google Scholar ] [ CrossRef ]
- Ding, Y.; Nie, M.; Xu, Y.; Miao, H. A Classification Method of Earthquake Ground Motion Records Based on the Results of K-Means Clustering Analysis. Buildings 2024 , 14 , 1831. [ Google Scholar ] [ CrossRef ]
- Weckenmann, A.; Jiang, X.; Sommer, K.D.; Neuschaefer-Rube, U.; Seewig, J.; Shaw, L.; Estler, T. Multisensor data fusion in dimensional metrology. CIRP Ann. 2009 , 58 , 701–721. [ Google Scholar ] [ CrossRef ]
- Perez-Ramirez, C.A.; Amezquita-Sanchez, J.P.; Valtierra-Rodriguez, M.; Adeli, H.; Dominguez-Gonzalez, A.; Romero-Troncoso, R.J. Recurrent neural network model with Bayesian training and mutual information for response prediction of large buildings. Eng. Struct. 2019 , 178 , 603–615. [ Google Scholar ] [ CrossRef ]
- Xu, Y.; Lu, X.; Cetiner, B.; Taciroglu, E. Real-time regional seismic damage assessment framework based on long short-term memory neural network. Comput.-Aided Civ. Infrastruct. Eng. 2021 , 36 , 504–521. [ Google Scholar ] [ CrossRef ]
- Shin, S.P.; Kim, K.; Le, T.H.M. Feasibility of Advanced Reflective Cracking Prediction and Detection for Pavement Management Systems Using Machine Learning and Image Detection. Buildings 2024 , 14 , 1808. [ Google Scholar ] [ CrossRef ]
- Wu, J.; He, Y.; Xu, C.; Jia, X.; Huang, Y.; Chen, Q.; Huang, C.; Dadras Eslamlou, A.; Huang, S. Interpretability Analysis of Convolutional Neural Networks for Crack Detection. Buildings 2023 , 13 , 3095. [ Google Scholar ] [ CrossRef ]
- Lee, S.Y.; Jeon, J.S.; Le, T.H.M. Feasibility of Automated Black Ice Segmentation in Various Climate Conditions Using Deep Learning. Buildings 2023 , 13 , 767. [ Google Scholar ] [ CrossRef ]
- Wang, H.; Ye, Z.; Wang, D.; Jiang, H.; Liu, P. Synthetic datasets for rebar instance segmentation using mask r-cnn. Buildings 2023 , 13 , 585. [ Google Scholar ] [ CrossRef ]
- Raviolo, D.; Civera, M.; Zanotti Fragonara, L. A comparative analysis of optimization algorithms for finite element model updating on numerical and experimental benchmarks. Buildings 2023 , 13 , 3010. [ Google Scholar ] [ CrossRef ]
- Hacıefendioğlu, K.; Altunışık, A.C.; Abdioğlu, T. Deep Learning-Based Automated Detection of Cracks in Historical Masonry Structures. Buildings 2023 , 13 , 3113. [ Google Scholar ] [ CrossRef ]
- Quinlan, A.R.; Hall, I.M. BEDTools: A flexible suite of utilities for comparing genomic features. Bioinformatics 2010 , 26 , 841–842. [ Google Scholar ] [ CrossRef ]
- Chang, C.C.; Chow, C.C.; Tellier, L.C.; Vattikuti, S.; Purcell, S.M.; Lee, J.J. Second-generation PLINK: Rising to the challenge of larger and richer datasets. Gigascience 2015 , 4 , s13742-015. [ Google Scholar ] [ CrossRef ]
- Kingma, D.P.; Welling, M. Auto-encoding variational bayes. arXiv 2013 , arXiv:1312.6114. [ Google Scholar ]
- Jolliffe, I.T.; Cadima, J. Principal component analysis: A review and recent developments. Philos. Trans. R. Soc. A Math. Phys. Eng. Sci. 2016 , 374 , 20150202. [ Google Scholar ] [ CrossRef ] [ PubMed ]
- Batista, G.E.; Wang, X.; Keogh, E.J. A complexity-invariant distance measure for time series. In Proceedings of the 2011 SIAM International Conference on Data Mining, Phoenix, AZ, USA, 28–30 April 2011; Society for Industrial and Applied Mathematics: Philadelphia, PA, USA, 2011. [ Google Scholar ]
- Cuturi, M.; Blondel, M. Soft-dtw: A differentiable loss function for time-series. In Proceedings of the International Conference on Machine Learning, Sydney, Australia, 6–11 August 2017; pp. 894–903. [ Google Scholar ]
- Xi, X.; Keogh, E.; Shelton, C.; Wei, L.; Ratanamahatana, C.A. Fast time series classification using numerosity reduction. In Proceedings of the 23rd International Conference on Machine Learning, Pittsburgh, PA, USA, 25–29 June 2006; pp. 1033–1040. [ Google Scholar ]
- Keogh, E.; Kasetty, S. On the need for time series data mining benchmarks: A survey and empirical demonstration. Data Min. Knowl. Discov. 2003 , 7 , 349–371. [ Google Scholar ] [ CrossRef ]
- Ye, L.; Keogh, E. Time series shapelets: A new primitive for data mining. In Proceedings of the 15th ACM SIGKDD International Conference on Knowledge Discovery and Data Mining, Paris, France, 28 June–1 July 2009; pp. 947–956. [ Google Scholar ]
- Cleveland, R.B.; Cleveland, W.S.; McRae, J.E.; Terpenning, I. STL: A seasonal-trend decomposition. J. Off. Stat. 1990 , 6 , 3–73. [ Google Scholar ]
- Bakirtzis, S.; Qiu, K.; Wassell, I.; Fiore, M.; Zhang, J. Deep-learning-based multivariate time-series classification for indoor/outdoor detection. IEEE Internet Things J. 2022 , 9 , 24529–24540. [ Google Scholar ] [ CrossRef ]
- Bagnall, A.; Davis, L.; Hills, J.; Lines, J. Transformation based ensembles for time series classification. In Proceedings of the 2012 SIAM International Conference on Data Mining, Anaheim, CA, USA, 26–28 April 2012; pp. 307–331. [ Google Scholar ]
- Le Guennec, A.; Malinowski, S.; Tavenard, R. Data augmentation for time series classification using convolutional neural networks. In Proceedings of the ECML/PKDD Workshop on Advanced Analytics and Learning on Temporal Data, Riva Del Garda, Italy, 19–23 September 2016. [ Google Scholar ]
- Strygina, M.A.; Gritsuk, I.I. Hydrological safety and risk assessment of hydraulic structures. RUDN J. Eng. Res. 2018 , 19 , 317–324. [ Google Scholar ] [ CrossRef ]
- Su, X.; Yan, X.; Tsai, C.L. Linear regression. Wiley Interdiscip. Rev. Comput. Stat. 2012 , 4 , 275–294. [ Google Scholar ] [ CrossRef ]
- Zhang, D.; Zhang, D. Wavelet transform. In Fundamentals of Image Data Mining: Analysis, Features, Classification and Retrieval ; Springer: Cham, Switzerland, 2019; pp. 35–44. [ Google Scholar ]
- Pal, M. Random forest classifier for remote sensing classification. Int. J. Remote Sens. 2005 , 26 , 217–222. [ Google Scholar ] [ CrossRef ]
- Ma, C.; Xu, X.; Yang, J.; Cheng, L. Safety monitoring and management of reservoir and dams. Water 2023 , 15 , 1078. [ Google Scholar ] [ CrossRef ]
- Obi, J.C. A comparative study of several classification metrics and their performances on data. World J. Adv. Eng. Technol. Sci. 2023 , 8 , 308–314. [ Google Scholar ]
Click here to enlarge figure
Accuracy | Recall | F1 Score | |
---|---|---|---|
Sinusoidal Wave Cyclical Data Set | 1.00 | 0.90 | 0.95 |
Triangular Wave Cyclical Data Set | 0.67 | 1.00 | 0.80 |
Seasonal Cyclical Data Set | 0.85 | 0.85 | 0.85 |
Weakly Cyclical Growth Data Set | 1.00 | 1.00 | 1.00 |
Overall Accuracy | 0.86 |
Accuracy | Precision | Recall | F1 Score | |
---|---|---|---|---|
Sinusoidal Wave Cyclical Data Set | 0.9957 | 0.5600 | 0.4828 | 0.5185 |
Triangular Wave Cyclical Data Set | 0.9996 | 1.0000 | 0.9218 | 0.8195 |
Seasonal Cyclical Data Set | 0.9980 | 0.6867 | 0.9090 | 0.7843 |
Weakly Cyclical Growth Data Set | 0.7213 | 0.1500 | 0.0187 | 0.0351 |
Accuracy | Precision | Recall | F1 Score | |
---|---|---|---|---|
Sinusoidal Wave Cyclical Data Set | 0.9990 | 1.0000 | 0.7931 | 0.8846 |
Triangular Wave Cyclical Data Set | 0.9999 | 1.0000 | 0.4000 | 0.5714 |
Seasonal Cyclical Data Set | 0.9985 | 0.8500 | 0.7272 | 0.8095 |
Weakly Cyclical Growth Data Set | 0.4485 | 0.3030 | 0.8940 | 0.4526 |
Accuracy | Precision | Recall | F1 Score | |
---|---|---|---|---|
Sinusoidal Wave Cyclical Data Set | 0.9931 | 0.3968 | 0.8620 | 0.5434 |
Triangular Wave Cyclical Data Set | 0.9999 | 1.0000 | 0.4000 | 0.5714 |
Seasonal Cyclical Data Set | 0.9978 | 0.7727 | 0.7083 | 0.7391 |
Weakly Cyclical Growth Data Set | 0.7510 | 0.3000 | 0.0105 | 0.2020 |
The statements, opinions and data contained in all publications are solely those of the individual author(s) and contributor(s) and not of MDPI and/or the editor(s). MDPI and/or the editor(s) disclaim responsibility for any injury to people or property resulting from any ideas, methods, instructions or products referred to in the content. |
Share and Cite
Mao, Y.; Li, J.; Qi, Z.; Yuan, J.; Xu, X.; Jin, X.; Du, X. Research on Outlier Detection Methods for Dam Monitoring Data Based on Post-Data Classification. Buildings 2024 , 14 , 2758. https://doi.org/10.3390/buildings14092758
Mao Y, Li J, Qi Z, Yuan J, Xu X, Jin X, Du X. Research on Outlier Detection Methods for Dam Monitoring Data Based on Post-Data Classification. Buildings . 2024; 14(9):2758. https://doi.org/10.3390/buildings14092758
Mao, Yanpian, Jiachen Li, Zhiyong Qi, Jin Yuan, Xiaorong Xu, Xinxin Jin, and Xuhuang Du. 2024. "Research on Outlier Detection Methods for Dam Monitoring Data Based on Post-Data Classification" Buildings 14, no. 9: 2758. https://doi.org/10.3390/buildings14092758
Article Metrics
Article access statistics, further information, mdpi initiatives, follow mdpi.
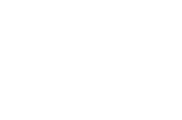
Subscribe to receive issue release notifications and newsletters from MDPI journals
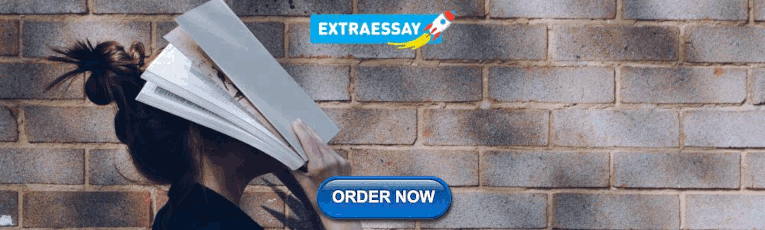
IMAGES
VIDEO
COMMENTS
5 USES OF UNCERTAINTY ANALYSIS (II) • Provide the only known basis for deciding whether: - Data agrees with theory - Tests from different facilities (jet engine performance) agree - Hypothesis has been appropriately assessed (resolved) - Phenomena measured are real • Provide basis for defining whether a closure check has been achieved - Is continuity satisfied (does the same ...
In this context, this study examined the post-graduate students' errors in writing research proposals. A textual analysis method was employed, where quantitative phase of analysis was followed ...
Reliability vs. Validity in Research | Difference, Types and Examples Reliability is about a method's consistency, and validity is about its accuracy.
Preface. This book is written as a guide for the presentation of experimental including a consistent treatment of experimental errors and inaccuracies. is meant for experimentalists in physics, astronomy, chemistry, life and engineering. However, it can be equally useful for theoreticians produce simulation data: they are often confronted with ...
values of a single quantity. These remaining deviations will be classed as random errors, and can be dealt with in a statistical manner. This document does not teach statistics in any formal sense, but it should help you to develop a working methodology for treating errors. 2 Determining random errors
4. Reducing errors in research. In their 2017 editorial Correcting the Medical Literature: "To Err Is Human, to Correct Divine", Christiansen and Flanagin urged researchers who discover errors to report them [7]. That step, though extremely important, will only help mitigate the effects of discovered errors on the published medical literature.
Alternatively, you can explore our Disciplines Hubs, including: Journal portfolios in each of our subject areas. Links to Books and Digital Library content from across Sage.
Abstract. Any data collected will be corrupted by errors; it is important to quantify these errors as the magnitude of the errors will influence the interpretation of the data. Errors arise in all four stages of the experimental process: calibration, acquisition, data analysis, and data combination. The chapter defines and explains how to ...
σ2 =. j ∂2χ2. ∂a2. j. 2. scan each parameter around minimum while others are fixed until ∆χ2 = 1 is reached. method #1 is much faster to calculate. method #2 is more generic and works even when the shape. of χ2 near minimum is not exactly parabollic. the scan of ∆χ2 = 1 defines a so-called one-sigma contour.
Key Words: error; bias; clinical research; trial design; study design; methodology; systematic review; meta-analysis; evidence-based medicine; Bayesian statistics; group sequential design; trial sequential analysis; data reporting CLINICAL RESEARCH with defined study methodology and statistical testing is a relatively recent addition to the field
1. Systematic Errors: Sometimes called constant errors. These uncertainties can result from errors in calibrating the measuring instruments, constant experimental conditions (e.g. temperature) different from those under which the instruments were calibrated, observational idiosyncracies (e.g. always reading a scale from an angle which is not
In this tutorial paper, we will use the term methodological study to refer to any study that reports on the design, conduct, analysis or reporting of primary or secondary research-related reports (such as trial registry entries and conference abstracts). In the past 10 years, there has been an increase in the use of terms related to ...
Another more advanced book : Statistical Methods in Experimental Physics: 2nd Edition by Frederick James) which discuss measurement errors and uncertainties and how statistical methods can be used to obtain best estimates on quantities extracted from measurements. The following sections are basically a very short summary of the main results ...
This review reveals that despite the criticism that this theory has received, it still plays a fundamental role in investigating, identifying and describing second language learners' errors and ...
Abstract. Some aspects of science, taken at the broadest level, are universal in empirical research. These include collecting, analyzing, and reporting data. In each of these aspects, errors can and do occur. In this work, we first discuss the importance of focusing on statistical and data errors to continually improve the practice of science.
The precision of a set of measurements is the spread in the distribution of measured values and can be estimated by using an index of dispersion (Chap. 5).Typically, the standard deviation serves as the metric of dispersion and is often expressed in percent:
89.332 + 1.1 = 90.432. should be rounded to get 90.4 (the tenths place is the last significant place in 1.1). After multiplication or division, the number of significant figures in the result is determined by the original number with the smallest number of significant figures. For example, (2.80) (4.5039) = 12.61092.
factual errors (also known as 'slips') are generally not due to inherent misunderstandings; slips may be due to memory deficits, impulsivity, or visual-motor integration problems and are easier to identify than conceptual errors.
Correctly worked examples consist of a problem statement with the steps taken to reach a solution along with the final result and are an effective method for the initial acquisitions of procedural skills and knowledge [1, 11, 26].Cognitive load theory [1, 11, 25] explains the challenge of stimulating the cognitive process without overloading the student with too much essential and extraneous ...
Kinematic calibration is necessary for the pose accuracy improvement of the parallel robots. However, difficulties occur in traditional calibration methods, such as exceeding a large number of erro...
Confidence in U.S. public opinion polling was shaken by errors in 2016 and 2020. In both years' general elections, many polls underestimated the strength of Republican candidates, including Donald Trump. These errors laid bare some real limitations of polling. In the midterms that followed those elections, polling performed better. But many ...
The electricity demand is rising in our day-to-day life and business; therefore, it is important to forecast electricity consumption to balance the demand and supply chain. The prediction mechanism will enable us to meet the demand quickly and use the available resources optimally. It will help us to reduce the waste of energy. Several statistical methods have been proposed to solve this issue ...
Background Narrative nursing is a novel approach according with humanistic care, which has been shown to be effective in improving health outcomes for both patients and nurses. Nevertheless, few studies have investigated the status of narrative nursing practice among nurses, and a comprehensive understanding of factors influencing this practice remains elusive. Design This was an observational ...
Safety monitoring of hydraulic structures is a critical task in the field of hydraulic engineering construction. This study developed a method for preprocessing and classifying monitoring data for the identification of gross errors in hydraulic structures. By utilizing linear regression and wavelet analysis techniques, it effectively differentiated various waveform characteristics in data sets ...