Open-Ended vs. Closed Questions in User Research
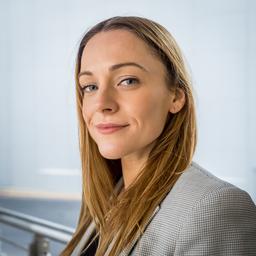
January 26, 2024 2024-01-26
- Email article
- Share on LinkedIn
- Share on Twitter
When conducting user research, asking questions helps you uncover insights. However, how you ask questions impacts what and how much you can discover .
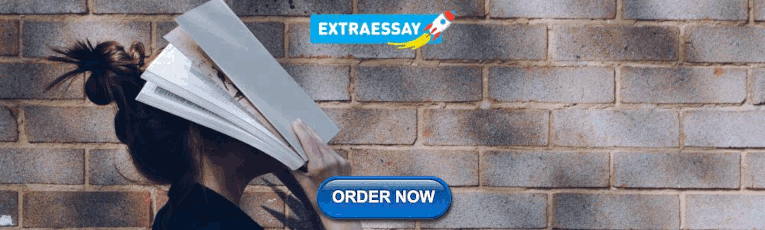
In This Article:
Open-ended vs. closed questions, why asking open-ended questions is important, how to ask open-ended questions.
There are two types of questions we can use in research studies: open-ended and closed.
Open-ended questions allow participants to give a free-form text answer. Closed questions (or closed-ended questions) restrict participants to one of a limited set of possible answers.
Open-ended questions encourage exploration of a topic; a participant can choose what to share and in how much detail. Participants are encouraged to give a reasoned response rather than a one-word answer or a short phrase.
Examples of open-ended questions include:
- Walk me through a typical day.
- Tell me about the last time you used the website.
- What are you thinking?
- How did you feel about using the website to do this task?
Note that the first two open-ended questions are commands but act as questions. These are common questions asked in user interviews to get participants to share stories. Questions 3 and 4 are common questions that a usability-test facilitator may ask during and after a user attempts a task, respectively.
Closed questions have a short and limited response. Examples of closed questions include:
- What’s your job title?
- Have you used the website before?
- Approximately, how many times have you used the website?
- When was the last time you used the website?
Strictly speaking, questions 3 and 4 would only be considered “closed” if they were accompanied by answer options, such as (a) never, (b) once, (c) two times or more. This is because the number of times and days could be infinite. That being said, in UX, we treat questions like these as closed questions.
In the dialog between a facilitator and a user below, closed questions provide a short, clarifying response, while open-ended questions result in the user describing an experience.
Using Closed Questions in Surveys
Closed questions are heavily utilized in surveys because the responses can be analyzed statistically (and surveys are usually a quantitative exercise). When used in surveys, they often take the form of multiple-choice questions or rating-scale items , rather than open-text questions. This way, the respondent has the answer options provided, and researchers can easily quantify how popular certain responses are. That being said, some closed questions could be answered through an open-text field to provide a better experience for the respondent. Consider the following closed questions:
- In which industry do you work?
- What is your gender?
Both questions could be presented as multiple-choice questions in a survey. However, the respondent might find it more comfortable to share their industry and gender in a free-text field if they feel the survey does not provide an option that directly aligns with their situation or if there are too many options to review.
Another reason closed questions are used in surveys is that they are much easier to answer than open-ended ones. A survey with many open-ended questions will usually have a lower completion rate than one with more closed questions.
Using Closed Questions in Interviews and Usability Tests
Closed questions are used occasionally in interviews and usability tests to get clarification and extra details. They are often used when asking followup questions. For example, a facilitator might ask:
- Has this happened to you before?
- When was the last time this happened?
- Was this a different time than the time you mentioned previously?
Closed questions help facilitators gather important details. However, they should be used sparingly in qualitative research as they can limit what you can learn.
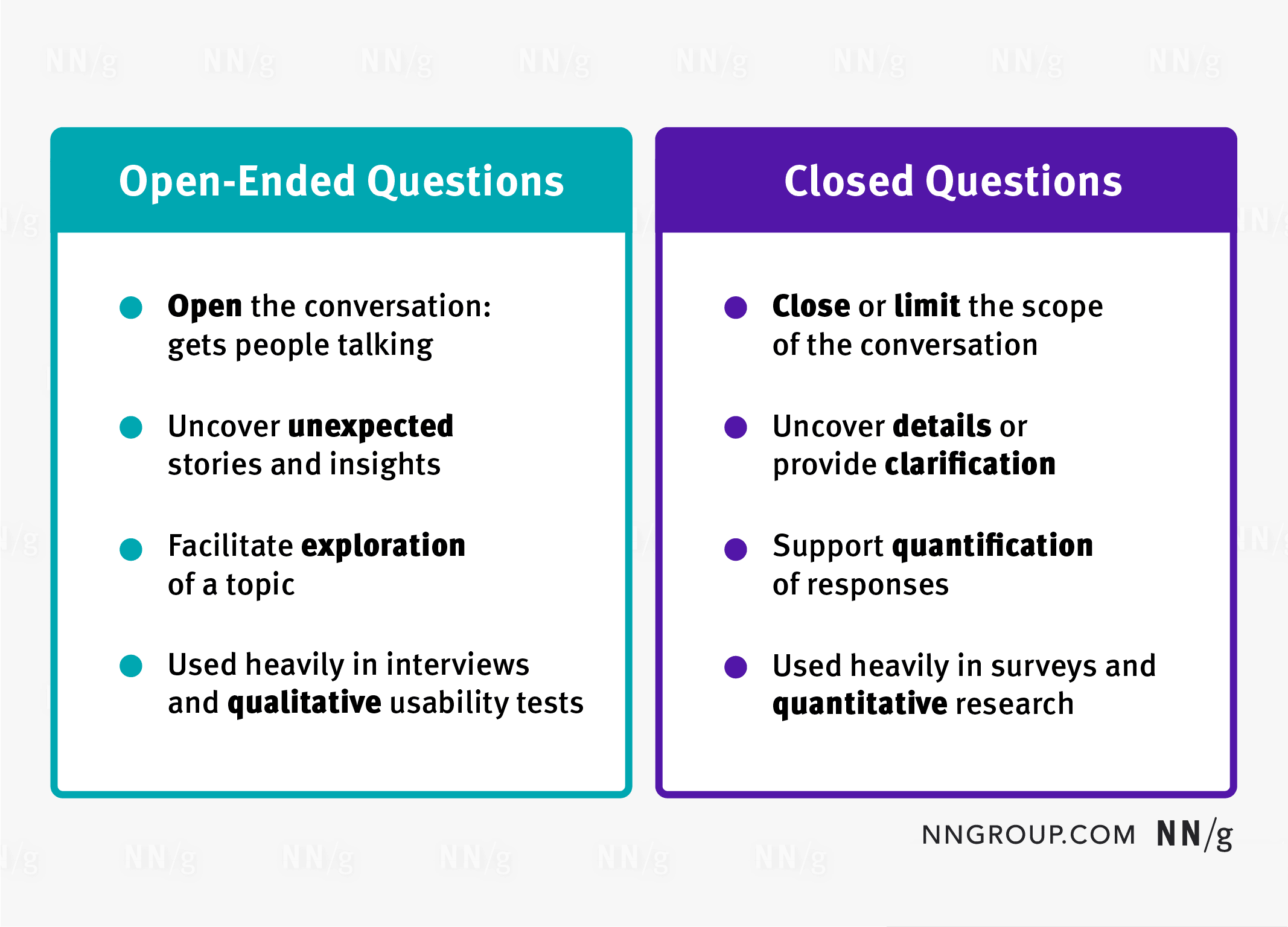
The greatest benefit of open-ended questions is that they allow you to find more than you anticipate. You don’t know what you don’t know. People may share motivations you didn’t expect and mention behaviors and concerns you knew nothing about. When you ask people to explain things, they often reveal surprising mental models , problem-solving strategies, hopes, and fears.
On the other hand, closed questions stop the conversation. If an interviewer or usability-test facilitator were to ask only closed questions, the conversation would be stilted and surface-level. The facilitator might not learn important things they didn’t think to ask because closed questions eliminate surprises: what you expect is what you get.
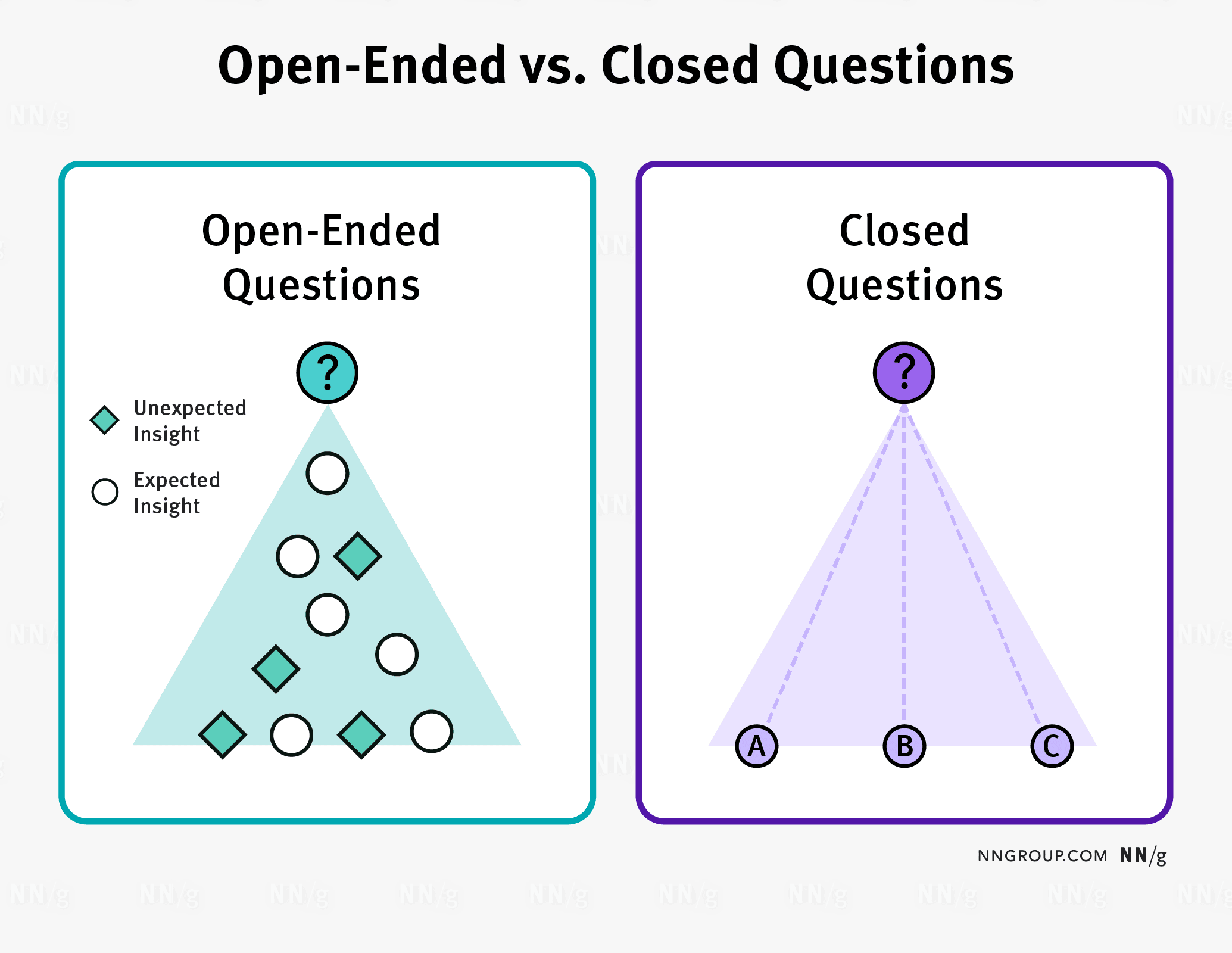
Closed Questions Can Sometimes Be Leading
When you ask closed questions, you may accidentally reveal what you’re interested in and prime participants to volunteer only specific information. This is why researchers use the funnel technique , where the session or followup questions begin with broad, open-ended questions before introducing specific, closed questions.
Not all closed questions are leading. That being said, it’s easy for a closed question to become leading if it suggests an answer.
The table below shows examples of leading closed questions . Reworking a question so it’s not leading often involves making it open-ended, as shown in column 2 of the table below.
One way to spot a leading, closed question is to look at how the question begins. Leading closed questions often start with the words “did,” “was,” or “is.” Open-ended questions often begin with “how” or “what.”
New interviewers and usability-test facilitators often struggle to ask enough open-ended questions. A new interviewer might be tempted to ask many factual, closed questions in quick succession, such as the following:
- Do you have children?
- Do you work?
- How old are you?
- Do you ever [insert behavior]?
However, these questions could be answered in response to a broad, open-ended question like Tell me a bit about yourself .
When constructing an interview guide for a user interview, try to think of a broad, open-ended version of a closed question that might get the participant talking about the question you want answered, like in the example above.
When asking questions in a usability test, try to favor questions that begin with “how,” or “what,” over “do,” or “did” like in the table below.
Another tip to help you ask open-ended questions is to use one of the following question stems :
- Walk me through [how/what]...
- Tell me a bit about…
- Tell me about a time where…
Finally, you can ask open-ended questions when probing. Probing questions are open-ended and are used in response to what a participant shares. They are designed to solicit more information. You can use the following probing questions in interviews and usability tests.
- Tell me more about that.
- What do you mean by that?
- Can you expand on that?
- What do you think about that?
- Why do you think that?
Ask open-ended questions in conversations with users to discover unanticipated answers and important insights. Use closed questions to gather additional small details, gain clarification, or when you want to analyze responses quantitatively.
Related Topics
- Research Methods Research Methods
Learn More:
Please accept marketing cookies to view the embedded video. https://www.youtube.com/watch?v=LpV3tMy_WZ0
Open vs. Closed Questions in User Research
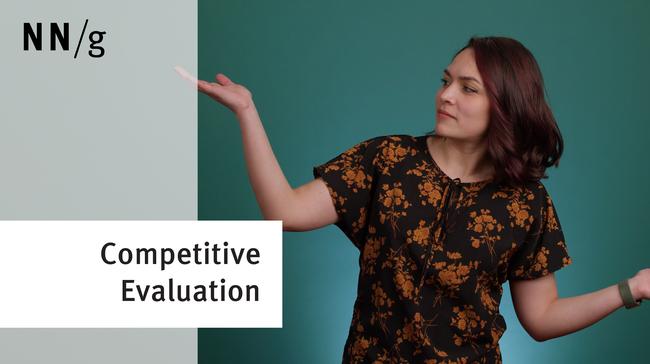
Competitive Reviews vs. Competitive Research
Therese Fessenden · 4 min
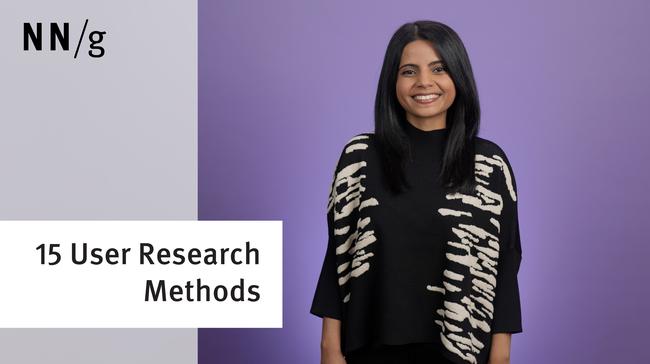
15 User Research Methods to Know Beyond Usability Testing
Samhita Tankala · 3 min
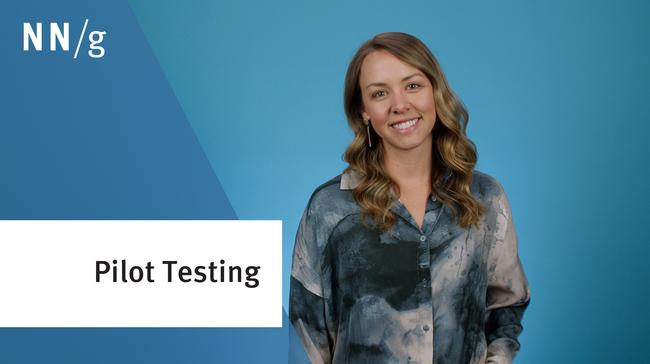
Always Pilot Test User Research Studies
Kim Flaherty · 3 min
Related Articles:
Field Studies Done Right: Fast and Observational
Jakob Nielsen · 3 min
Screening Participants for User-Research Studies
Maddie Brown · 7 min
Should You Run a Survey?
Maddie Brown · 6 min
The Funnel Technique in Qualitative User Research
Maria Rosala and Kate Moran · 7 min
Card Sorting: Pushing Users Beyond Terminology Matches
Samhita Tankala · 5 min
Card Sorting: Uncover Users' Mental Models for Better Information Architecture
Samhita Tankala and Katie Sherwin · 11 min
Root out friction in every digital experience, super-charge conversion rates, and optimize digital self-service
Uncover insights from any interaction, deliver AI-powered agent coaching, and reduce cost to serve
Increase revenue and loyalty with real-time insights and recommendations delivered to teams on the ground
Know how your people feel and empower managers to improve employee engagement, productivity, and retention
Take action in the moments that matter most along the employee journey and drive bottom line growth
Whatever they’re saying, wherever they’re saying it, know exactly what’s going on with your people
Get faster, richer insights with qual and quant tools that make powerful market research available to everyone
Run concept tests, pricing studies, prototyping + more with fast, powerful studies designed by UX research experts
Track your brand performance 24/7 and act quickly to respond to opportunities and challenges in your market
Explore the platform powering Experience Management
- Free Account
- Product Demos
- For Digital
- For Customer Care
- For Human Resources
- For Researchers
- Financial Services
- All Industries
Popular Use Cases
- Customer Experience
- Employee Experience
- Net Promoter Score
- Voice of Customer
- Customer Success Hub
- Product Documentation
- Training & Certification
- XM Institute
- Popular Resources
- Customer Stories
- Artificial Intelligence
- Market Research
- Partnerships
- Marketplace
The annual gathering of the experience leaders at the world’s iconic brands building breakthrough business results, live in Salt Lake City.
- English/AU & NZ
- Español/Europa
- Español/América Latina
- Português Brasileiro
- REQUEST DEMO
- Experience Management
- What is a survey?
- Open Ended Questions
Try Qualtrics for free
Your quick guide to open-ended questions in surveys.
17 min read In this guide, find out how you can use open-ended survey questions to glean more meaningful insights from your research, as well as how to analyse them and best practices.
When you want to get more comprehensive responses to a survey – answers beyond just yes or no – you’ll want to consider open-ended questions.
But what are open-ended questions? In this guide, we’ll go through what open-ended questions are, including how they can help gather information and provide greater context to your research findings.
Take a Self-Guided Product Tour of XM for Strategic UX
What are open-ended questions?
Open-ended questions can offer you incredibly helpful insights into your respondent’s viewpoints. Here’s an explanation below of what they are and what they can do:
Free-form and not governed by simple one word answers (e.g. yes or no responses), an open-ended question allows respondents to answer in open-text format, giving them the creative thinking, freedom and space to answer in as much (or as little) detail as they like.
Open-ended questions help you to see things from the respondent’s perspective, as you get feedback in their own words instead of stock answers. Also, as you’re getting more meaningful answers and accurate responses, you can better analyze sentiment amongst your audience.
Open-ended versus closed-ended questions
Open-ended questions provide more qualitative research data; contextual insights that accentuate quantitative information. With open-ended questions, you get more meaningful user research data.
Closed-ended questions, on the other hand, provide quantitative data ; limited insight but easy to analyze and compile into reports. Market researchers often add commentary to this kind of data to provide readers with background and further food for thought.
Here are the main differences with examples of open-ended and closed-ended questions:
For example, an open-ended question might be: “What do you think of statistical analysis software?”.
Whereas closed-ended questions would simply be: “Do you use statistical analysis software?” or “Have you used statistical analysis software in the past?”.
Open-ended questions afford much more freedom to respondents and can result in deeper and more meaningful insights. A closed question can be useful and fast, but doesn’t provide much context. Open-ended questions are helpful for understanding the “why”.
When and why should you use an open-ended question?
Open-ended questions are great for going more in-depth on a topic. Closed-ended questions may tell you the “what,” but open-ended questions will tell you the “why.”
Another benefit of open-ended questions is that they allow you to get answers from your respondents in their words. For example, it can help to know the language that customers use to describe a product of feature, so that the company can match the language in their product description to increase discoverability.
Open-ended questions can also help you to learn things you didn’t expect, especially as they encourage creativity, and get answers to slightly more complex issues. For example, you could ask the question “What are the main reasons you canceled your subscription?” as a closed-ended question by providing a list of reasons (too expensive, don’t use it anymore). However, you are limited only to reasons that you can think of. But if you don’t know why people are canceling, then it might be better to ask as an open-ended question.
You might ask open-ended questions when you are doing a pilot out preliminary research to validate a product idea. You can then use that information to generate closed-ended questions for a larger follow-up study.
However, it can be wise to limit the overall number of open-ended questions in a survey because they are burdensome.
In terms of what provides more valuable information, only you can decide that based on the requirements of your research study. You also have to take into account variables such as the cost and scale of your research study, as well as when you need the information. Open-ended questions can provide you with more context, but they’re also more information to sift through, whereas closed-ended questions provide you with a tidy, finite response.
If you still prefer the invaluable responses and data from open-ended questions, using software like Qualtrics Text IQ can automate this complicated process. Through AI technology Text IQ can understand sentiment and articulate thousands of open-ended responses into simplified dashboards.
Open-ended question examples
While there are no set rules to the number of open-ended questions you can ask, of course you want to ask an open-ended question that correlates with your research objective.
Here are a few examples of open-ended survey questions related to your product:
- What do you like most about this product?
- What do you like least about this product?
- How does our product compare to competitor products?
- If someone asked you about our product, what would you say to them?
- How can we improve our product?
You could even supplement closed-ended questions with an open-ended question to get more detail, e.g. “How often do you use our product?” — with a multiple choice, single word answers approach. These might be simple answers such as “Frequently”, “Sometimes”, “Never” — and if a respondent answers “Never”, you could follow with: “If you have never used our product, why not?”. This is a really easy way to understand why potential customers don’t use your product.
Also, incorporating open-ended questions into your surveys can provide useful information for salespeople throughout the sales process. For example, you might uncover insights that help your salespeople to reposition your products or improve the way they sell to new customers based on what existing customers feel. Though you might get helpful answers from a closed-ended question, open-ended questions give you more than a surface-level insight into their sentiments, emotions and thoughts.
It doesn’t need to be complicated, it can be as simple as what you see below. The survey doesn’t need to speak for itself, let your survey respondents say everything.
Asking open-ended questions: Crafting question that generate the best insights
Open responses can be difficult to quantify. Framing them correctly is key to getting useful data from your answers. Below are some open ended questions examples of what to avoid.
1. Avoid questions that are too broad or vague
Example : “What changes has your company made in the last five years due to external events?”
Problem : There are too many potential responses to this query, which means you’ll get too broad a range of answers. What kind of changes are being referred to, economic, strategic, personnel etc.? What external events are useful to know about? Don’t overwhelm your respondent with an overly broadquestion – ask the right questions and get precise answers.
Solution : Target your questions with a specific clarification of what you want. For example, “What policy changes has your company made about working from home in the last 6 months as a result of the COVID-19 pandemic?”. Alternatively, use a close-ended question, or offer examples to give respondents something to work from.
2. Make sure that the purpose of the question is clear
Example : “Why did you buy our product?”
Problem : This type of unclear-purpose question can lead to short, unhelpful answers. “Because I needed it” or “I fancied it” don’t necessarily give you data to work with.
Solution : Make it clear what you actually want to know. “When you bought our product, how did you intend to use it?” or “What are the main reasons you purchased [Our Brand] instead of another brand?” might be two alternatives that provide more context.
3. Keep questions simple and quick to answer
Example : “Please explain the required process that your brand uses to manage its contact center (i.e. technical software stack, approval process, employee review, data security, management, compliance management etc.). Please be as detailed as possible.”
Problem : The higher the level of effort, the lower the chances of getting a good range of responses or high quality answers. It’s unlikely that a survey respondent will take the time to give a detailed answer on something that’s not their favorite subject. This results in either short, unhelpful answers, or even worse, the respondent quits the survey and decides not to participate after seeing the length of time and effort required. This can end up causing bias with the type of respondents that answer the survey.
Solution : If you really need the level of detail, there are a few options to try. You can break up the question into multiple questions or share some information on why you really need this insight. You could offer a different way of submitting an answer, such as a voice to text or video recording functionality, or make the question optional to help respondents to keep progressing through the survey. Possibly the best solution is to change from open-ended questions in a survey to a qualitative research method, such as focus groups or one-to-one interviews, where lengthier responses and more effort are expected.
4. Ask only one question at a time
Example : “When was the last time you used our product? How was your experience?”
Problem : Too many queries at once can cause a feeling of mental burden in your respondents, which means you risk losing their interest. Some survey takers might read the first question but miss the second, or forget about it when writing their response.
Solution : Only ask one thing at a time!
5. Don’t ask for a minimum word count
Example : “Please provide a summary of why you chose our brand over a competitor brand. [Minimum 50 characters].”
Problem : Even though making a minimum word count might seem like a way to get higher quality responses, this is often not the case. Respondents may well give up, or type gibberish to fill in the word count. Ideally, the responses you gather will be the natural response of the person you’re surveying – mandating a word count impedes this.
Solution : Leave off the word count. If you need to encourage longer responses, you can expand the text box size to fit more words in. Offer speech to text or video recording options to encourage lengthier responses, and explain why you need a detailed answer.
6. Don’t ask an open-ended question when a closed-ended question would be enough
Example : “Where are you from?”
Problem : It’s harder to control the data you’ll collect when you use an open question when a closed one would work. For example, someone could respond to the above question with “The US”, “The United States” or “America”.
Solution : To save time and effort on both your side and the participant’s side, use a drop-down with standardized responses.
7. Limit the total number of open-ended questions you ask
Example : “How do you feel about product 1?” “How do you feel about product 2?” “How do you feel about product 3?”
Problem : An open question requires more thought and effort than a closed one. Respondents can usually answer 4-6 closed questions in the same time as only 1 open one, and prefer to be able to answer quickly.
Solution : To reduce survey fatigue,lower drop-off rates, and save costs, only ask as many questions as you think you can get an answer for. Limit open-ended questions for ones where you really need context. Unless your respondents are highly motivated, keep it to 5 open-ended questions or fewer. Space them out to keep drop-offs to a minimum.
8. Don’t force respondents to answer open-ended questions
Example : “How could your experience today have been improved? Please provide a detailed response.”
Problem : A customer may not have any suggestions for improvements. By requiring an answer, though, the customer is now forced to think of something that can be improved even if it would not make them more likely to use the service again. Making these respondents answer means you risk bias. It could lead to prioritizing unnecessary improvements.
Solution : Give respondents the option to say “No” or “Not applicable” or “I don’t know” to queries, or to skip the question entirely.
How to analyze the results from open-ended questions
Step 1: collect and structure your responses.
Online survey tools can simplify the process of creating and sending questionnaires, as well as gathering responses to open-ended questions. These tools often have simple, customisable templates to make the process much more efficient and tailored to your requirements.
Some solutions offer different targeting variables, from geolocation to customer segments and site behavior. This allows you to offer customized promotions to drive conversions and gather the right feedback at every stage in the online journey.
Upon receipt, your data should be in a clear, structured format and you can then export it to a CSV or Excel file before automatic analysis. At this point, you’ll want to check the data (spelling, duplication, symbols) so that it’s easier for a machine to process and analyze.
Step 2: Use text analytics
One method that’s increasingly applied to open-ended responses is automation. These new tools make it easy to extract data from open-text question responses with minimal human intervention. It makes an open-ended question response as accessible and easy to analyze as that of a closed question, but with more detail provided.
For example, you could use automated coding via artificial intelligence to look into buckets of responses to your open-ended questions and assign them accordingly for review. This can save a great deal of time, but the accuracy depends on your choice of solution.
Alternatively, you could use sentiment analysis — a form of natural language processing — to systematically identify, extract and quantify information. With sentiment analysis, you can determine whether responses are positive or negative, which can be really useful for unstructured responses or for quick, large-scale reviews.
Some solutions also offer custom programming so you can apply your own code to analyze survey results, giving complete flexibility and accuracy.
Step 3: Visualize your results
With the right data analysis and visualization tools, you can see your survey results in the format most applicable to you and your stakeholders. For example, C-Suite may want to see information displayed using graphs rather than tables — whereas your research team might want a comprehensive breakdown of responses, including response percentages for each question.
This might be easier for a survey with closed-ended questions, but with the right analysis for open-ended questions’ responses, you can easily collate response data that’s easy to quantify.
With the survey tools that exist today, it’s incredibly easy to import and analyze data at scale to uncover trends and develop actionable insights. You can also apply your own programming code and data visualization techniques to get the information you need. No matter whether you’re using open-ended questions or getting one-word answers in emojis, you’re able to surface the most useful insights for action.
Ask the right open-ended questions with Qualtrics
With Qualtrics’ survey software , used by more than 13,000 brands and 99 of the top 100 business schools, you can get answers to the most important market, brand, customer, and product questions with ease. Choose from a huge range of multiple-choice questions (both open-ended questions and closed-ended) and tailor your survey to get the most in-depth responses to your queries.
You can build a positive relationship with your respondents and get a deeper understanding of what they think and feel with Qualtrics-powered surveys. The best part? It’s completely free to get started with.
Product Tour: XM for Strategic UX
Related resources
Post event survey questions 10 min read, best survey software 16 min read, close-ended questions 7 min read, survey vs questionnaire 12 min read, response bias 13 min read, double barreled question 11 min read, likert scales 14 min read, request demo.
Ready to learn more about Qualtrics?

Learn and Grow
Open-Ended Questions: +20 Examples, Tips and Comparison

By Caitriona Maria
November 11, 2023
Open-ended questions allow for a wide range of responses, unlike closed-ended questions with limited response options. They are often used in surveys or interviews to gather qualitative data, providing more detailed and insightful information than closed-ended questions.
Let’s explore the definition, purpose, and benefits of open-ended questions and tips for crafting and asking effective ones.
See next: Close-Ended Questions: Examples, Tips, and When To Use
What Are Open-Ended Questions?
An open-ended question encourages a full, meaningful answer using the subject’s knowledge, experience, attitude, and feelings.
A good open-ended question should be broad enough to invite thoughtful responses yet specific enough to provide direction. It should avoid leading the respondent to a particular answer, eliminating bias.
Additionally, the language should be simple and clear to ensure understanding and comfort for the respondent. Lastly, it should be relevant and purposeful to align with the overall objectives of the survey or interview .
Characteristics of Effective Open-Ended Questions
- Non-leading question
- Relevant to the topic or issue discussed
- Should allow for a variety of free-form responses
- Cannot be answered with a “ yes ” or “no,” “true” or “false” response
Examples of Open-Ended Questions
Here are some examples of open-ended questions that can be used in surveys, discussions, or interviews:
- What do you believe are the biggest challenges facing our society today?
- Can you describe a time when you felt most fulfilled in your work?
- How has your perspective on [topic] evolved over time?
- What does [concept] mean to you?
- How do you see technology shaping our future?
- Can you share a personal experience that has shaped your values and beliefs?
- In your opinion, what are the most important qualities of a leader?
- What factors do you consider when making important decisions?
- How can we better address issues of diversity and inclusion in our community?
- What impact do you think [policy/decision] will have on our environment?
Tips for Crafting Effective Open-Ended Questions
Crafting effective open-ended questions requires careful consideration of the wording and structure. Here are some tips to keep in mind:
Start With “What,” “How” or “Why”
Beginning a question with “what,” “how,” or “why” encourages the respondent to think critically and provide a detailed answer.
Other common words and phrases that an open-ended question include “describe,” “tell me about,” and “what do you think about…”
Avoid Leading or Loaded Questions
Leading questions or loaded questions can bias the respondent towards a certain answer and limit the range of responses. It is important to avoid using language that suggests a preferred or expected answer.
For example, instead of asking, “Don’t you agree that…?” a more effective open-ended question would be, “What is your opinion on…?”
Use Simple and Clear Language
Open-ended questions should be easy to understand and answer. Using complicated or technical language can confuse the respondent and result in incomplete or inaccurate responses.
Be Specific and Direct
It is important to be specific with open-ended questions to gather relevant and useful information. Avoiding broad or vague questions can help elicit more focused and detailed responses.
Consider the Order of Questions
The order of questions in a survey or interview can impact the responses. It is often best to start with closed-ended questions before moving on to open-ended ones, as this can help warm up and engage the respondent.
For instance, in the initial stages, closed questions can be helpful to gather information about customer demographics such as age, marital status, religion, and so on.
Tips for Asking Open-Ended Questions
When asking open-ended questions, here are some other tips to keep in mind.
Appropriateness
Decide if an open-ended question is necessary or appropriate for the situation.
Consider the purpose of the question and evaluate if a closed-ended question may be more suit.
Don’t Ask Too Many Open-Ended Questions
It is important to balance open-ended and closed-ended questions to gather relevant information effectively.
Too many open-ended questions can overwhelm respondents and lead to incomplete answers, or they might abandon the survey altogether.
Consider using open-ended and closed-ended questions to gather detailed responses and specific data or statistics.
Change Close-Ended to Open-Ended Questions
Sometimes, a closed-ended question can be rephrased to elicit more detailed, open-ended responses.
For example, instead of asking, “Do you like our product?” which only allows for a yes or no answer, you could ask, “What do you like or dislike about our product?”
Listen Carefully to Responses
When using open-ended questions, it is important to listen and take note of the responses actively. This can provide valuable insights and help identify areas for improvement or potential new ideas.
Allow Time for Responses
Open-ended questions require more thought and reflection, so give respondents enough time to formulate their responses. Avoid rushing them or interrupting them before they have finished speaking.
Advantages of Open-Ended Questions
Open-ended questions offer several advantages over other question forms. Here are some key benefits:
1. Encourages Thoughtful Responses
Open-ended questions require the respondent to think and provide a more detailed answer rather than simply selecting from a list of predetermined options.
This allows for more thoughtful and insightful responses, providing a deeper understanding of the subject matter.
2. Allows for Individual Perspectives
Since open-ended questions do not limit the response options, they allow individuals to express their unique perspectives and experiences.
Open-ended questions can provide diverse answers and a more holistic view of the topic.
3. Provides Rich and Detailed Data
The open-ended nature of these questions allows for a wider range of responses, providing richer and more detailed data compared to closed-ended questions.
This can be especially useful in qualitative research and allows researchers to uncover deeper meaning and understanding.
4. Promotes Engagement
Open-ended questions often require the respondent to provide longer answers, which can promote engagement and interest in the topic being discussed.
Open Ended Questions Vs. Close Ended Questions
Closed-ended questions can be answered with a simple “yes” or “no” or are limited to a predetermined set of options. Unlike open-ended questions, they do not allow respondents to expand on their answers or provide additional information.
Common closed-ended questions include multiple-choice, ranking scale, or binary questions (yes/no).
These questions are often used in quantitative research, where the objective is to gather statistical data. For instance, they are commonly found in surveys where data needs to be analyzed swiftly and uniformly.
These questions provide a straightforward way for researchers to categorize responses and draw conclusions from the data. However, they may not offer the depth and nuance of information that open-ended questions can provide.
Examples of Close-Ended Questions
As mentioned, closed-ended questions are useful for gathering specific data or statistics. Here are some examples:
- Do you agree or disagree with the statement?
- On a scale of 1 to 10, how satisfied are you with your job?
- What is your age?
- Which of the following options best describes your educational background?
- How much is your monthly phone bill? Select from the range below.
- Have you ever used our product/service before?
- Would you consider using our product/service again?
- Do you prefer [option A] or [option B] for [specific situation]?
- Were you happy with your purchase?
- Was this helpful?
Open Ended Questions to Ask Your Customers or Clients
- What do you value most in a product/service?
- How has our product/service improved your business?
- Can you explain how our product/service has helped you achieve a specific goal?
- What improvements or changes would you like to see in our product/service?
- How likely are you to recommend our product/service to others ? Why or why not?
- Can you share a specific experience or interaction with our brand that stands out in your mind?
- What do you think sets us apart from our competitors?
- Can you describe a time when our product/service exceeded your expectations?
- How has using our product/service impacted your daily routine or workflow?
- In your opinion, what is the biggest benefit of our product/service?
Open-ended questions are valuable for gathering qualitative data and gaining deeper insights into a topic. They offer several advantages over closed-ended questions, including promoting engagement and providing rich, detailed data.
By following the tips provided, researchers can craft practical, open-ended questions that elicit thoughtful and meaningful responses from participants.
Caitriona Maria is an education writer and founder of TPR Teaching, crafting inspiring pieces that promote the importance of developing new skills. For 7 years, she has been committed to providing students with the best learning opportunities possible, both domestically and abroad. Dedicated to unlocking students' potential, Caitriona has taught English in several countries and continues to explore new cultures through her travels.
Nerdy Types of Men Make ‘the Best Husbands’ According to Millenial Women
Close-ended questions: +20 examples, tips and comparison, leave a comment cancel reply.
Save my name, email, and website in this browser for the next time I comment.
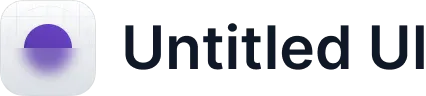
The Importance of Open-Ended Questions: How to Make the Most of Them
Understand what open-ended questions are in the realm of user research, and how you can use them to derive meaningful insights from your users.
Aishwarya N K
January 27, 2024

In this Article
Short on time? Get an AI generated summary of this article instead
AI-generated article summary
Understanding Open-Ended Questions in UX Research
While surveys and data analysis provide insights into what users do, they often fall short in revealing why they do it. To bridge this gap, open-ended questions are essential. These questions encourage users to express their thoughts in their own words, providing deeper insights into their motivations and emotions.
What are Open-Ended Questions? Open-ended questions prompt detailed responses rather than simple ""yes"" or ""no"" answers. They typically start with ""how,"" ""what,"" ""why,"" or ""tell me about,"" fostering a nuanced understanding of participants' perspectives. They are commonly used in qualitative research, user interviews, and surveys.
Open-Ended vs. Closed-Ended Questions Open-Ended Questions: Best for exploring topics in-depth, building rapport, and understanding complex subjects. Closed-Ended Questions: Suitable for collecting quantifiable data, efficiency in surveys, and verifying specific information. Advantages of Open-Ended Questions Rich Insights: Encourage detailed and nuanced responses. User-Centric Exploration: Allow participants to share unanticipated experiences. Flexibility: Adapt questioning based on participant responses. Contextual Understanding: Provide context for behaviors and preferences. Uncovering Unconscious Needs: Reveal needs participants may not consciously recognize. Engagement: Foster a collaborative atmosphere, enhancing participant investment. Validating Quantitative Data: Offer qualitative context to numerical findings. Iterative Design Improvement: Inform design optimizations based on user insights. Building Empathy: Deepen understanding of emotional user experiences. Enhancing User-Centered Design: Prioritize user perspectives to create better-aligned products. Tips for Asking Open-Ended Questions Start with openers like ""How,"" ""What,"" or ""Why."" Be clear and concise to ensure understanding. Avoid leading questions to promote genuine responses. Encourage elaboration by following up on responses. Use scenario-based questions for concrete responses. Tailor questions to the participant's context and background. Create a comfortable environment for open sharing. Maintain neutral language to avoid bias. Mix question types to keep engagement high. Keep questions brief to avoid confusion. Allow for silence to encourage deeper thinking. Be mindful of your tone and non-verbal cues.
Get fast AI summaries of customer calls and feedback with magic summarize in Decode
We spend hours crafting surveys, designing questionnaires, and analyzing data with laser focus. However, the cold, hard truth is that this data can only tell you what users do, not why they do it. To bridge the gap and understand the motivations and emotions that truly drive user behavior, we need to delve deeper. Open-ended questions are your secret tool to help you understand by inviting users to express themselves in their own words.
What is an open-ended question?
Open-ended questions are queries that prompt a detailed, extensive response rather than a simple "yes" or "no" answer. These questions encourage participants to share their thoughts, opinions, and experiences in their own words, fostering a more comprehensive and nuanced understanding of their perspective.
Open-ended questions typically begin with words like "how," "what," "why," or "tell me about," allowing respondents to express themselves freely and provide detailed insights. This approach is commonly used in qualitative research, user interviews , surveys, and discussions to draw out rich and diverse information from participants.
Open-ended questions vs closed-ended questions

When should you ask open and closed-ended questions?
The choice between open-ended and closed-ended questions depends on the goals of your research, the information you seek, and the stage of your interaction with participants. Here's a general guide:
Open-ended questions are suitable for:
Exploration and understanding: Use open-ended questions when you want to explore a topic in-depth and gain a comprehensive understanding of participants' perspectives.
Early research stages: At the beginning of your research or interview, open-ended questions help build rapport and allow participants to share their thoughts freely.
Complex subjects: For complex or nuanced topics where you want detailed responses, open-ended questions encourage participants to elaborate.
Closed-ended questions are suitable for:
Collecting quantitative data: When you need specific, quantifiable data or want to gather responses that are easy to categorize, closed-ended questions with predefined answer options are effective.
Survey design : In surveys, closed-ended questions are often used for efficiency, especially when dealing with a large number of participants.
Verification: When you need to verify specific information or test hypotheses, closed-ended questions can provide clear, standardized responses.
Mixed approach:
In many cases, a combination of open-ended and closed-ended questions is beneficial. Start with open-ended questions to gather rich insights, and then use closed-ended questions to quantify and categorize specific aspects. This balanced approach allows for depth and structure in your research.
Advantages of open-ended questions
Rich insights.
Open-ended questions encourage participants to provide detailed and nuanced responses, offering researchers deeper insights into their thoughts, feelings, and experiences. This richness of information goes beyond simple yes/no answers.
User-centric exploration
By allowing participants to express themselves freely, open-ended questions empower users to share aspects of their experiences that researchers may not have anticipated. This user-centric approach ensures that the study explores areas relevant to participants.
Flexibility and adaptability
Open-ended questions provide flexibility, allowing researchers to adapt the line of questioning based on participants' responses. This adaptability ensures that researchers can follow interesting leads and delve deeper into specific topics as they arise.
Understanding context
Participants can provide context and elaborate on their responses, helping researchers understand the reasons behind certain behaviors or preferences. This contextual information is valuable for making informed design decisions.
Uncovering unconscious needs
Participants might reveal needs, desires, or challenges they were not consciously aware of when responding to open-ended questions. This uncovering of subconscious aspects adds a layer of depth to the research findings.
Encouraging engagement
Open-ended questions engage participants more actively in the research process. This engagement fosters a collaborative atmosphere, making participants feel heard and valued, which can contribute to more honest and thoughtful responses.
Validating quantitative data
When used in conjunction with quantitative methods, open-ended questions can help validate or provide context for numerical data. They offer a qualitative dimension that complements quantitative findings, providing a more comprehensive understanding.
Iterative design improvement
Insights gathered from open-ended questions can inform iterative design improvements. Understanding users' perspectives and preferences at a deeper level enables designers to refine and optimize products for enhanced user satisfaction.
Building empathy
Open-ended questions contribute to building empathy with users. Researchers gain a more profound understanding of the emotional aspects of user experiences, fostering a human-centric approach to UX design .
Enhancing user-centered design
Overall, open ended questions support a user-centered design approach by prioritizing the user's voice and perspective. This leads to products and experiences that are more aligned with user needs and expectations.
How to ask open-ended questions
Asking effective open-ended questions is crucial to elicit detailed and insightful responses. Here are some tips on how to ask open-ended questions:
Start with "How," "What," "Why," or "Tell me about"
These question starters encourage participants to provide detailed information rather than a simple yes or no.
For example: Instead of asking, "Did you enjoy the experience?" ask, "How would you describe your experience?"
Be clear and concise
Frame your questions in a clear and straightforward manner to ensure participants understand what you're asking.
For example: Instead of a vague question like, "Anything else you want to share?" be more specific, such as, "Is there any specific aspect of the product that stood out to you?"
Avoid leading questions
Refrain from phrasing questions in a way that leads them to give a particular answer. Avoiding leading questions ensures that participants provide unbiased and genuine responses.
For example: Instead of asking, "Don't you think the new feature is great?" ask, "What are your thoughts on the new feature?"
Encourage elaboration
Follow up on responses with additional probes to encourage participants to elaborate on their answers. This helps you delve deeper into their perspectives.
For example: If a participant mentions a positive experience, follow up with, "Can you provide more details on what made that aspect enjoyable for you?"
Use scenario-based questions
Presenting hypothetical scenarios can prompt participants to think about situations in a more concrete and detailed manner.
For example: "Imagine you are using this product in a real-world scenario. How do you envision it fitting into your daily routine?"
Consider context
Tailor your questions to the context of the research and the participant's background. This ensures relevance and encourages thoughtful responses.
For example: If researching a mobile app, ask, "How do you typically use mobile apps for [specific purpose]?"
Create a comfortable environment
Establish a rapport with participants to make them feel comfortable sharing their thoughts openly. A relaxed atmosphere encourages open-ended responses.
Use neutral language
Ensure that your questions are phrased in a neutral manner to avoid influencing participants' responses. This helps in obtaining unbiased and honest feedback.
For example: Instead of saying, "Don't you find the interface confusing?" ask, "How would you describe your experience with the interface?"
Vary question types
Mix open-ended questions with other question types in your research. This adds variety to the interaction and helps maintain participant engagement.
Limit question length
Keep your questions concise and to the point. Long-winded questions can confuse participants and may lead to less detailed responses.
For example: Instead of a lengthy question like, "Considering your past experiences, including the challenges you may have faced, can you describe how this product compares?" break it down into more focused queries.
Allow for silence
After posing an open-ended question, give participants time to gather their thoughts and respond. Silence can be a powerful tool, encouraging participants to share more detailed insights.
Be mindful of your tone
Pay attention to your tone and non-verbal cues. A friendly and non-judgmental demeanor helps participants feel more comfortable sharing their perspectives.
{{cta-trial}}
Frequently Asked Questions
What are open-ended questions and closed-ended questions.
Open-ended questions are designed to elicit detailed and subjective responses, encouraging participants to express their thoughts in their own words. These questions typically begin with words like "how," "what," or "why." In contrast, closed-ended questions have predefined answer options, often requiring a simple "yes" or "no" or selecting from a list.
Why do we use open-ended questions?
Open-ended questions are crucial in research and communication as they promote richer, qualitative insights. By allowing participants to share their perspectives freely, researchers can uncover nuanced details, emotions, and unexpected insights. Open-ended questions are particularly useful when exploring complex topics or seeking in-depth information.
What is an open-ended question example?
Some examples of an open-ended question include:
- How would you describe your overall experience using our website?
- Can you share any suggestions for improving the navigation on our app?
- What factors influence your decision when choosing a [product/service]?
- What features would you like to see in future versions of our product?
Lorem ipsum dolor sit amet, consectetur adipiscing elit. Suspendisse varius enim in eros elementum tristique. Duis cursus, mi quis viverra ornare, eros dolor interdum nulla, ut commodo diam libero vitae erat. Aenean faucibus nibh et justo cursus id rutrum lorem imperdiet. Nunc ut sem vitae risus tristique posuere.
With lots of unique blocks, you can easily build a page without coding.
Click on Study templates
Start from scratch
Add blocks to the content
Saving the Template
Publish the Template
Aishwarya tries to be a meticulous writer who dots her i’s and crosses her t’s. She brings the same diligence while curating the best restaurants in Bangalore. When she is not dreaming about her next scuba dive, she can be found evangelizing the Lord of the Rings to everyone in earshot.
Senior Product Marketing Specialist
Related Articles
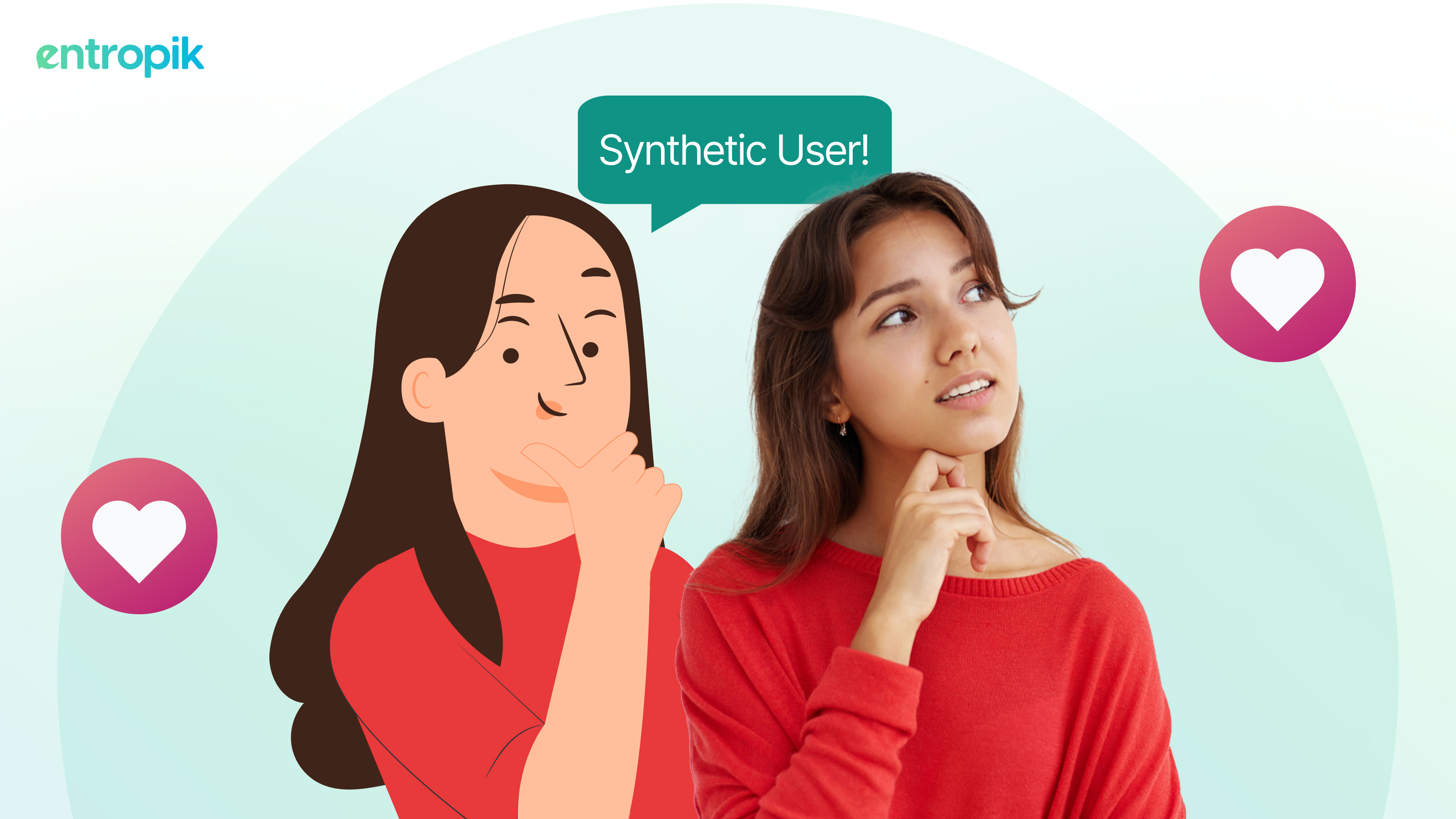
Synthetic Users: Revolutionizing UX Testing and Digital Performance
Synthetic users simulate real behavior to enhance UX testing and digital performance, enabling businesses to optimize experiences and detect issues pre-emptively.
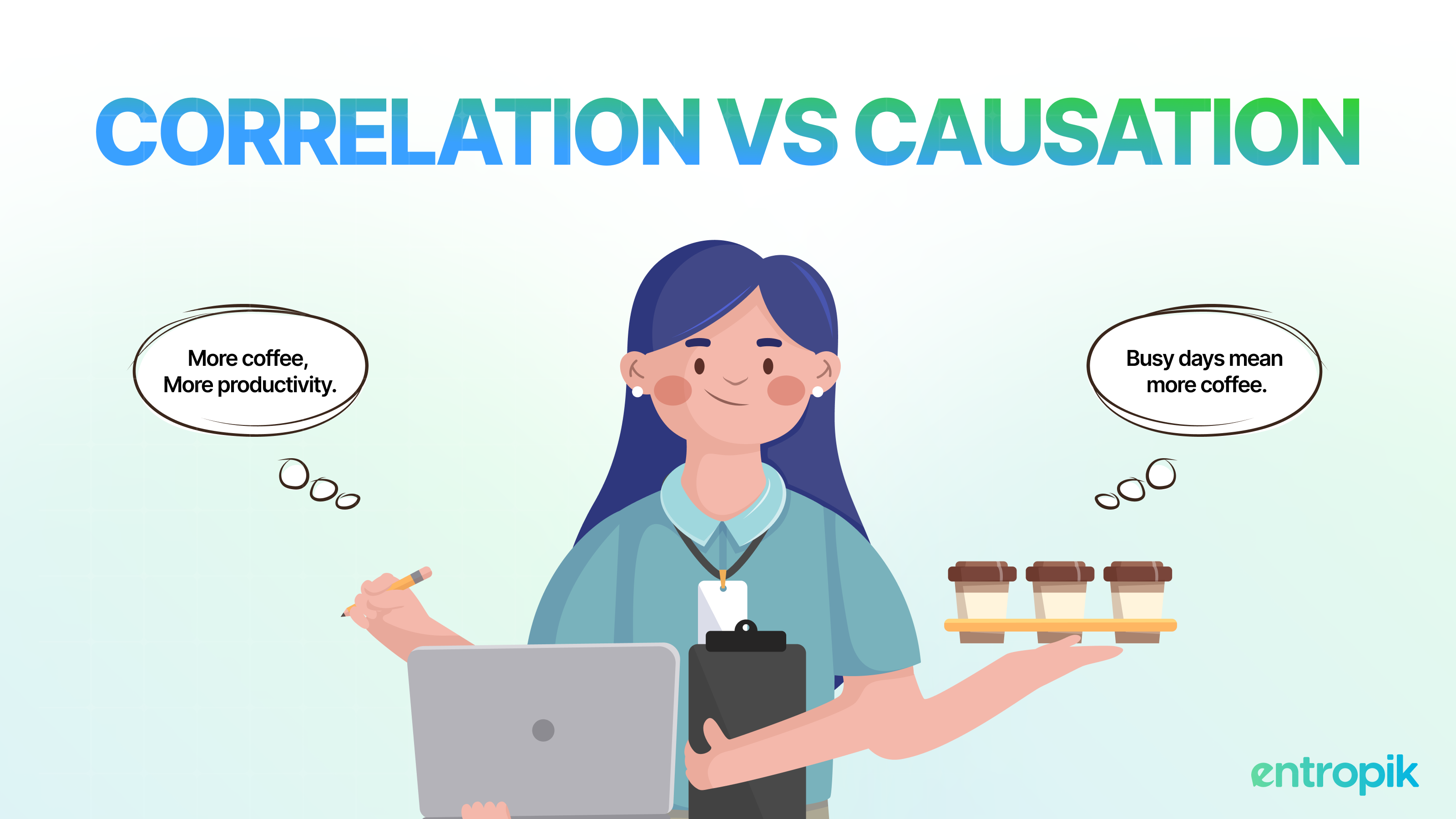
Correlation vs Causation: Applied in UX Research
Discover the critical distinctions between correlation and causation and why they matter in UX Research.

Subjective vs Objective Research: A Competitive Analysis
Explore the differences between subjective and objective research methods, their impact on data analysis, and how to apply each approach effectively.

Predictive Analytics: Harnessing the Power to Foresee the Future
AI-Driven Predictive Analytics: The Key to Forecasting Market Trends, Boosting Efficiency, and Accelerating Business Growth

Mastering the Art of Conceptual Framework in Market Research
Learn how a conceptual framework in market research guides your study, connects theory with practice and ensures a clear structure for effective analysis and results.

The Ultimate User Testing Guide
Explore our guide on user testing, covering its importance, methods, and how to enhance user experience through actionable insights. Read more!
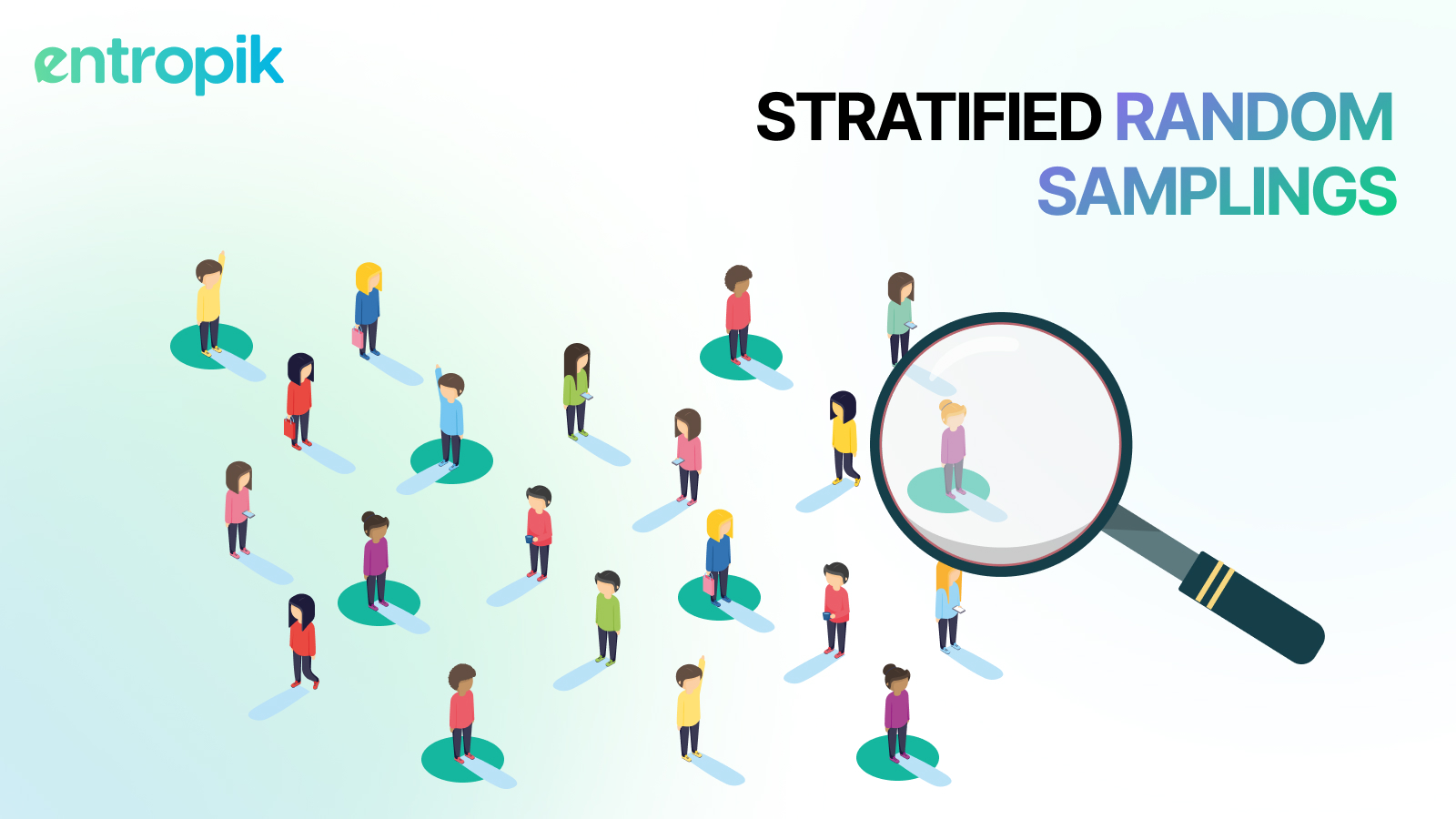
Stratified Random Sampling: A Complete Guide with Definition, Method, and Examples
Master Stratified Random Sampling: A Step-by-Step Guide to Boost Precision in Research and Decision-Making for Researchers and Product Managers

Building Customer Loyalty: Best Practices and Strategies
Customer loyalty is a cornerstone of any successful business. This comprehensive guide will explore various strategies and tactics to help you cultivate a loyal customer base.

How to Create Buyer Personas That Drive Product and Marketing Success?
Develop detailed, data-backed buyer personas to improve product development, refine marketing strategies, and deliver personalized experiences.
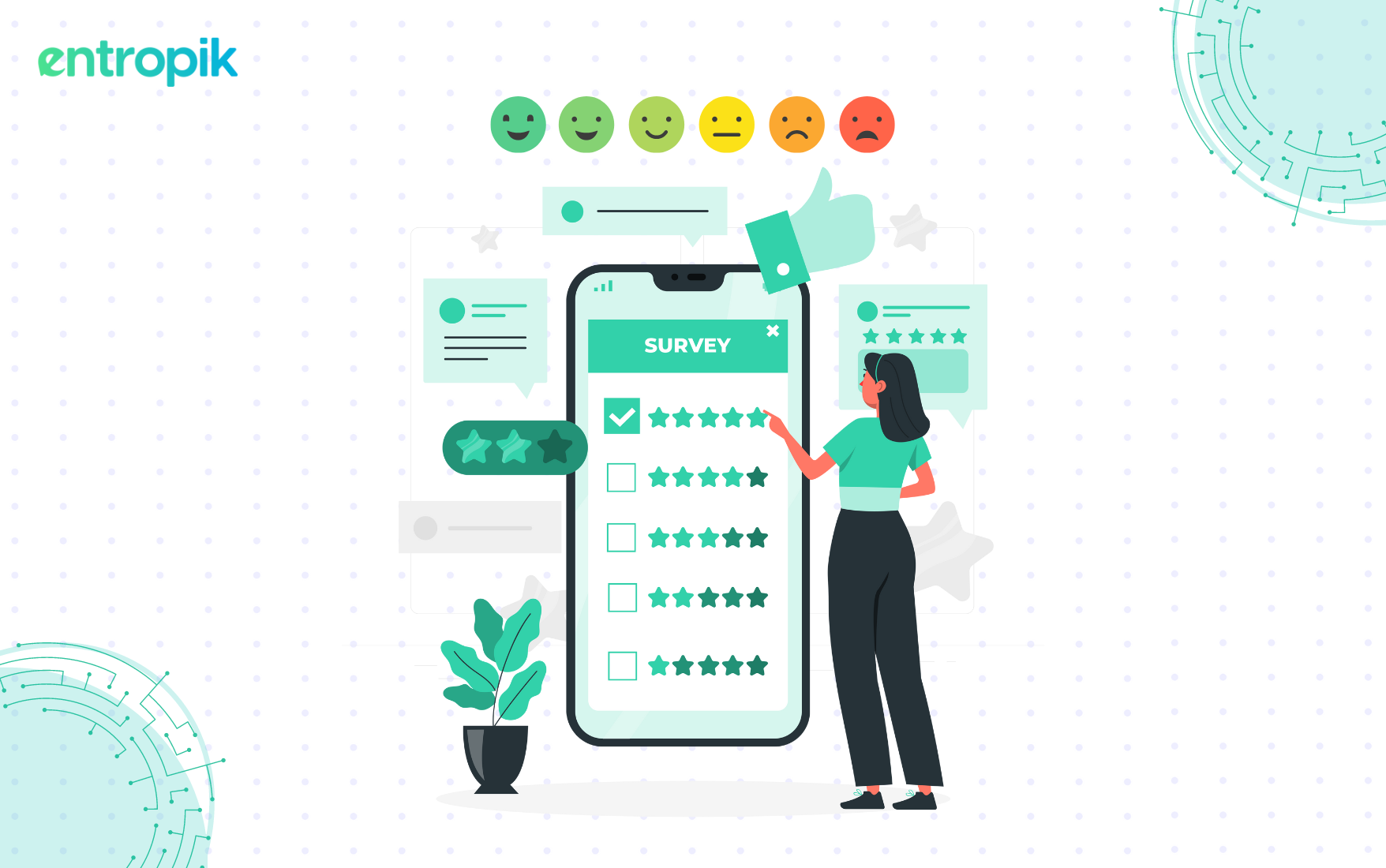
What is a Survey? Benefits, Types, Blocks, Use Cases, and More
Discover the power of surveys: types, benefits, use cases, and how to create effective surveys using the AI-powered platform Decode.
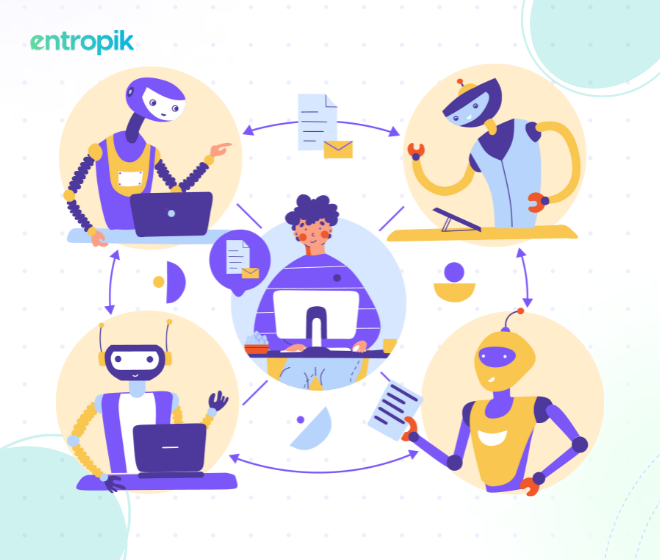
Top AI Events You Do Not Want to Miss in 2024
Here are all the top AI events for 2024, curated in one convenient place just for you.
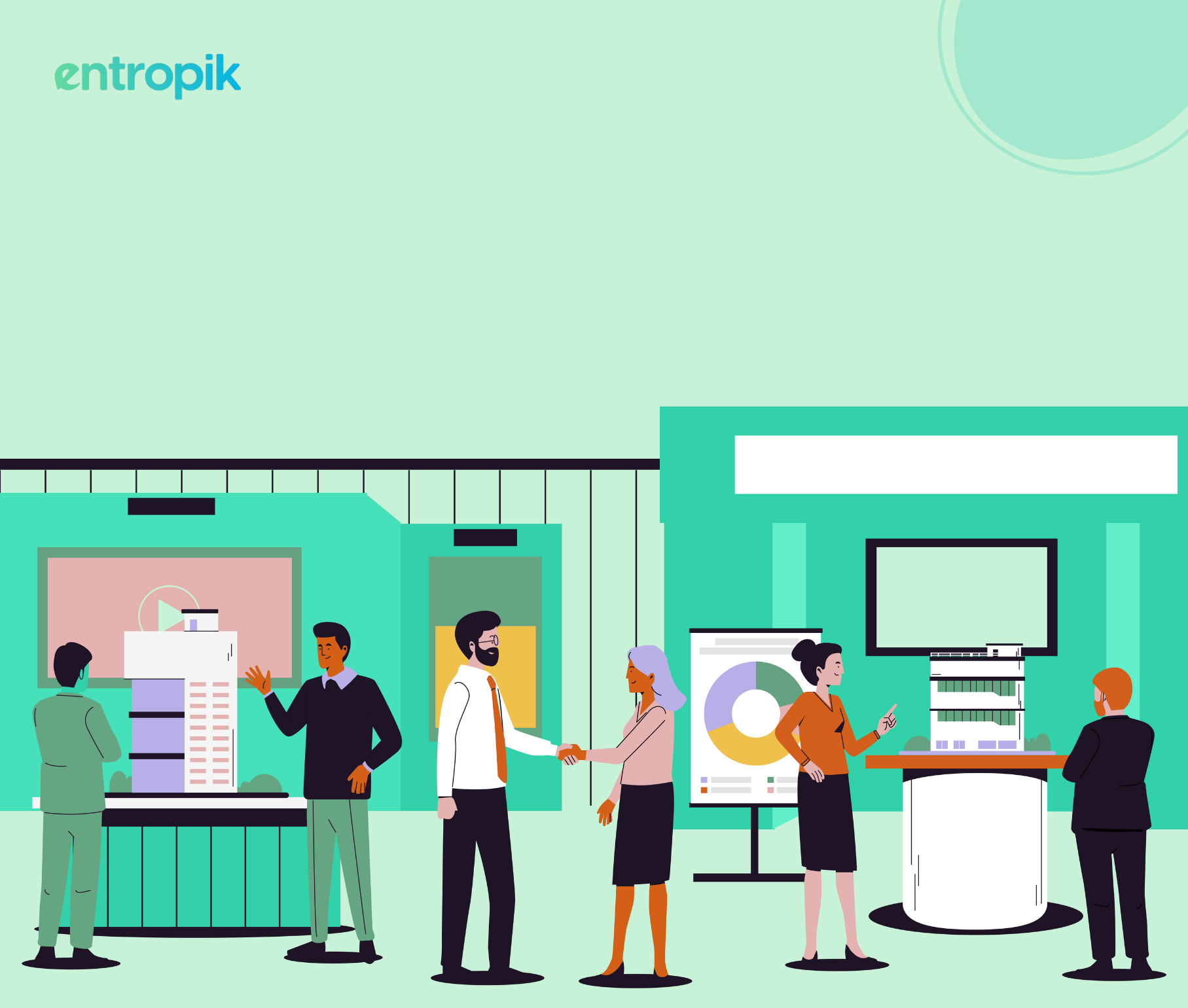
Top Insights Events You Do Not Want to Miss in 2024
Here are all the top Insights events for 2024, curated in one convenient place just for you.
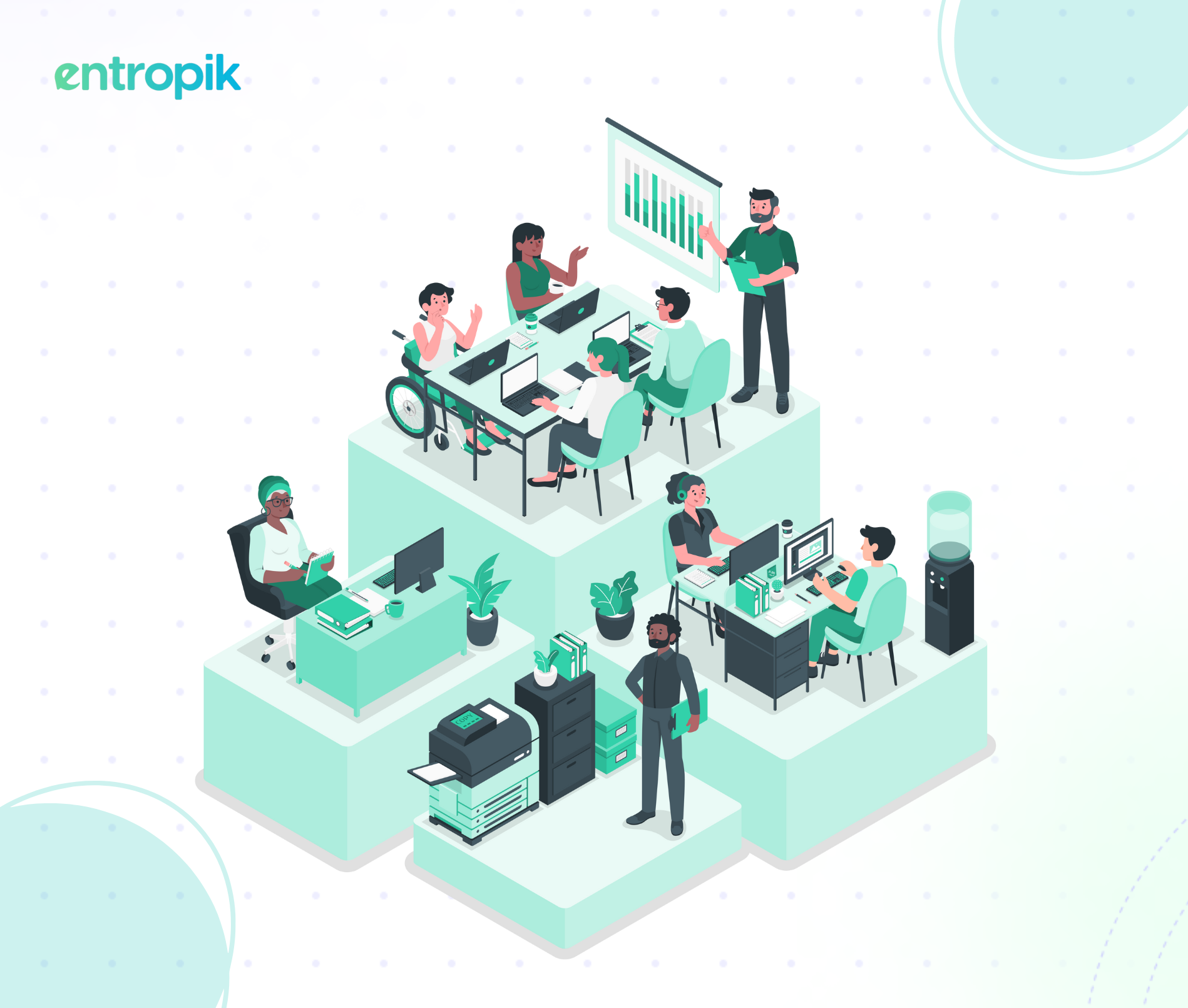
Top CX Events You Do Not Want to Miss in 2024
Here are all the top CX events for 2024, curated in one convenient place just for you.
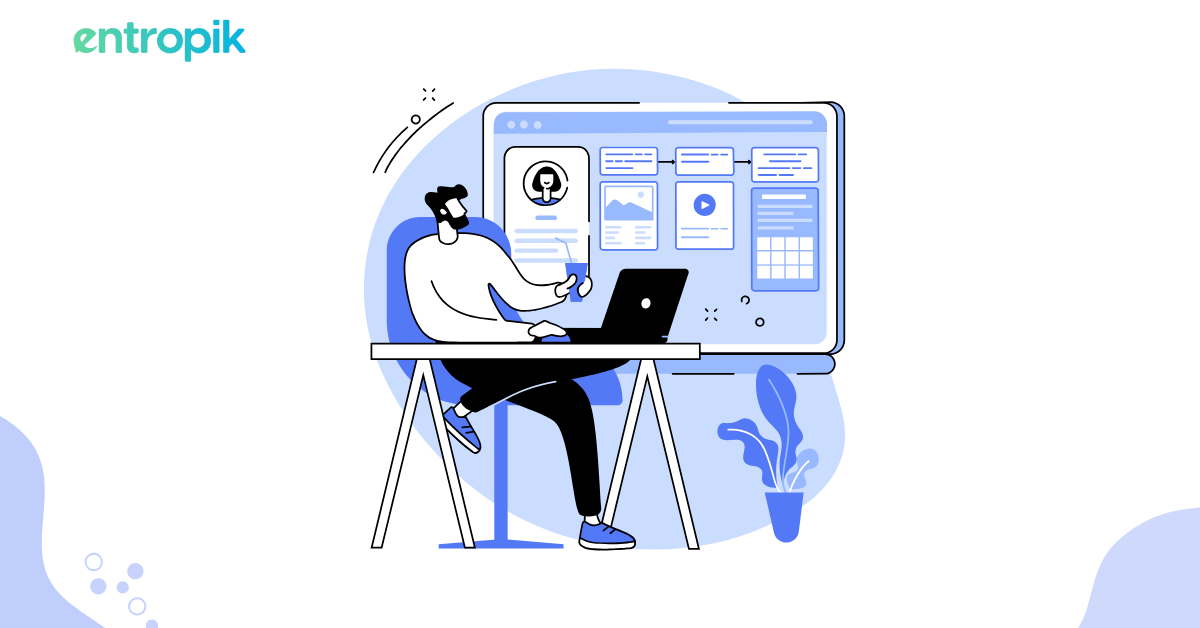
How to Build an Experience Map: A Complete Guide
An experience map is essential for businesses, as it highlights the customer journey, uncovering insights to improve user experiences and address pain points. Read to find more!

Everything You Need to Know about Intelligent Scoring
Are you curious about Intelligent Scoring and how it differs from regular scoring? Discover its applications and benefits. Read on to learn more!

Qualitative Research Methods and Its Advantages In Modern User Research
Discover how to leverage qualitative research methods, including moderated sessions, to gain deep user insights and enhance your product and UX decisions.
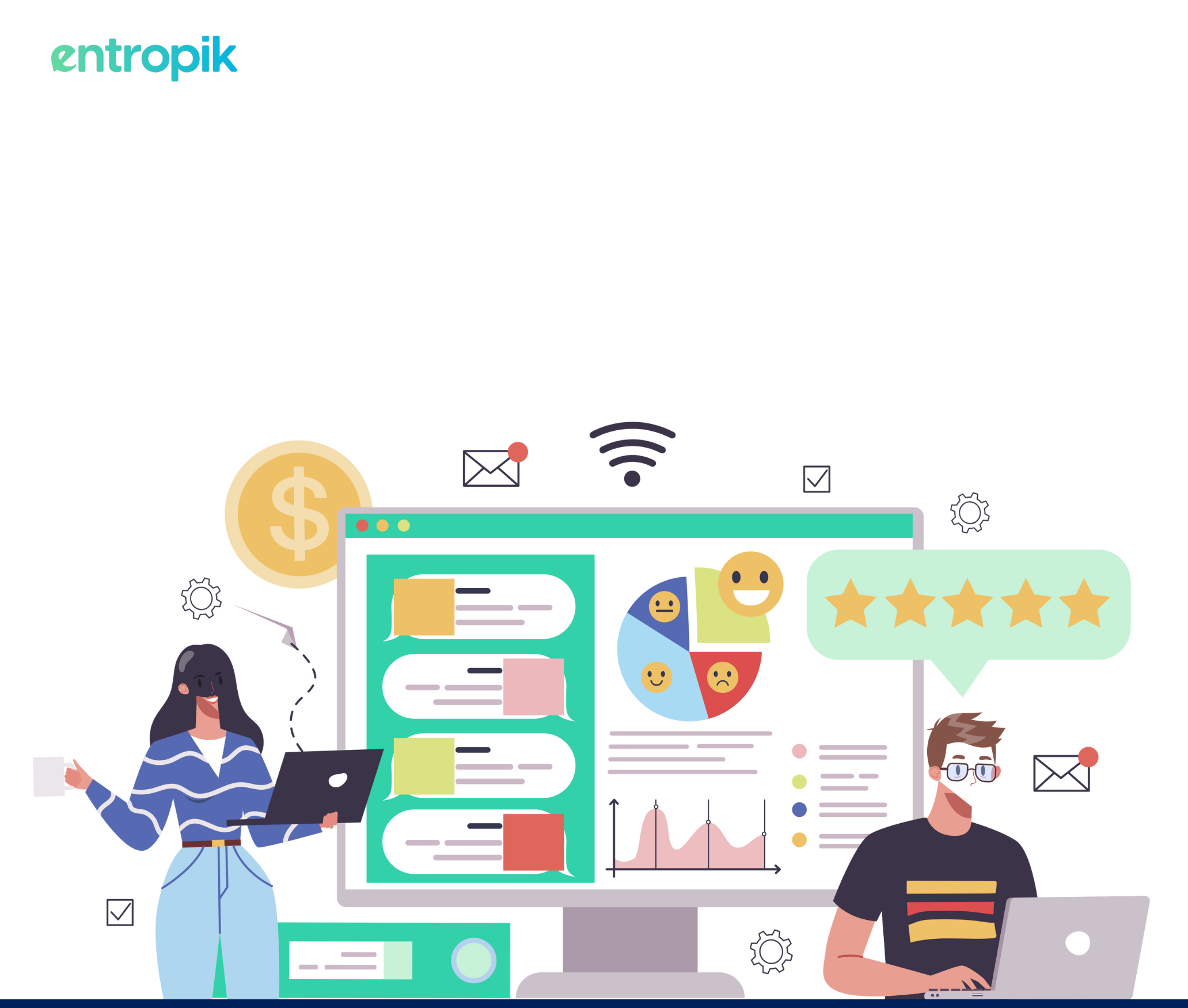
The 10 Best Customer Experience Platforms to Transform Your CX
Explore the top 10 CX platforms to revolutionize customer interactions, enhance satisfaction, and drive business growth.
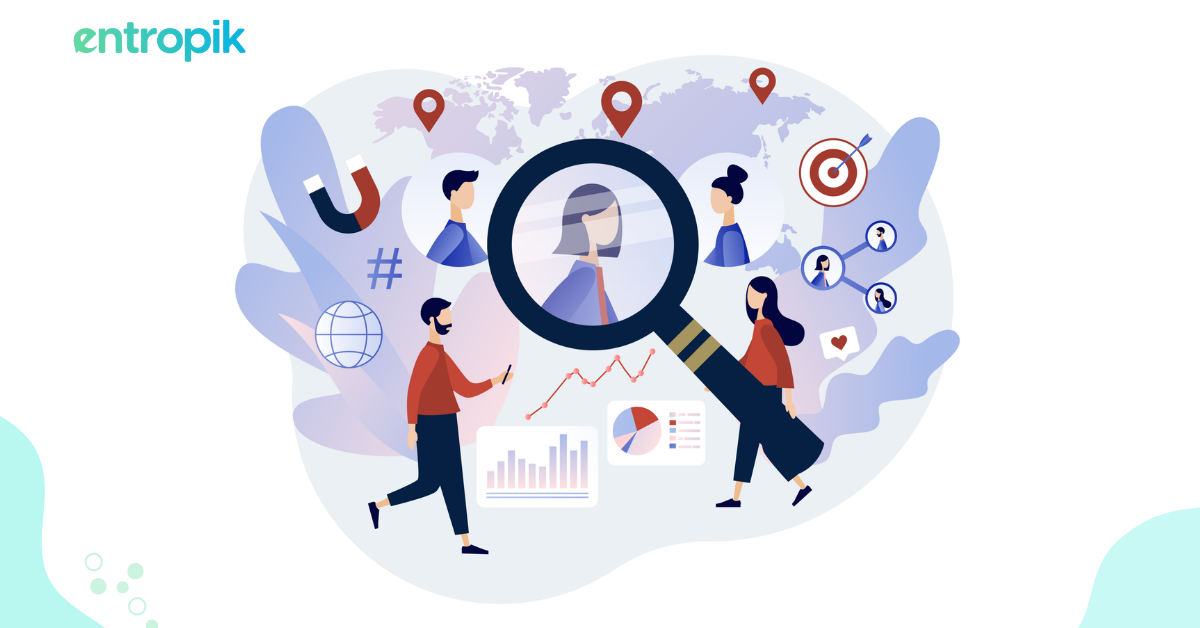
TAM SAM SOM: What It Means and How to Calculate It?
Understanding TAM, SAM, SOM helps businesses gauge market potential. Learn their definitions and how to calculate them for better business decisions and strategy.

Understanding Likert Scales: Advantages, Limitations, and Questions
Using Likert scales can help you understand how your customers view and rate your product. Here's how you can use them to get the feedback you need.

Mastering the 80/20 Rule to Transform User Research
Find out how the Pareto Principle can optimize your user research processes and lead to more impactful results with the help of AI.
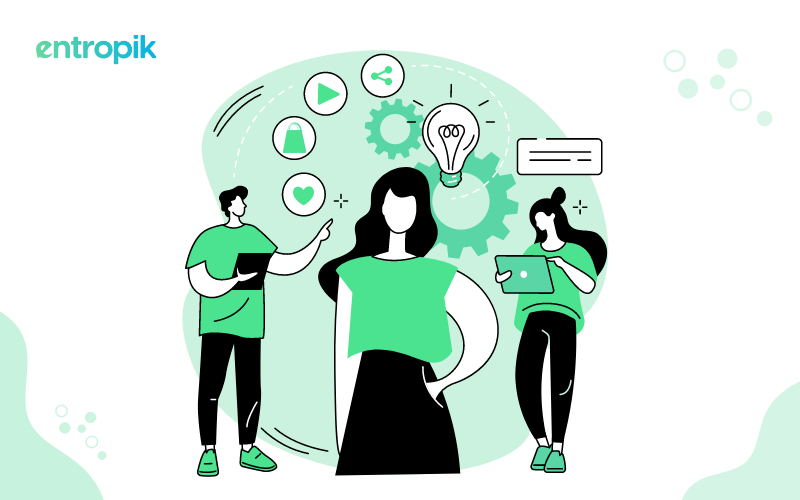
Understanding Consumer Psychology: The Science Behind What Makes Us Buy
Gain a comprehensive understanding of consumer psychology and learn how to apply these insights to inform your research and strategies.

A Guide to Website Footers: Best Design Practices & Examples
Explore the importance of website footers, design best practices, and how to optimize them using UX research for enhanced user engagement and navigation.
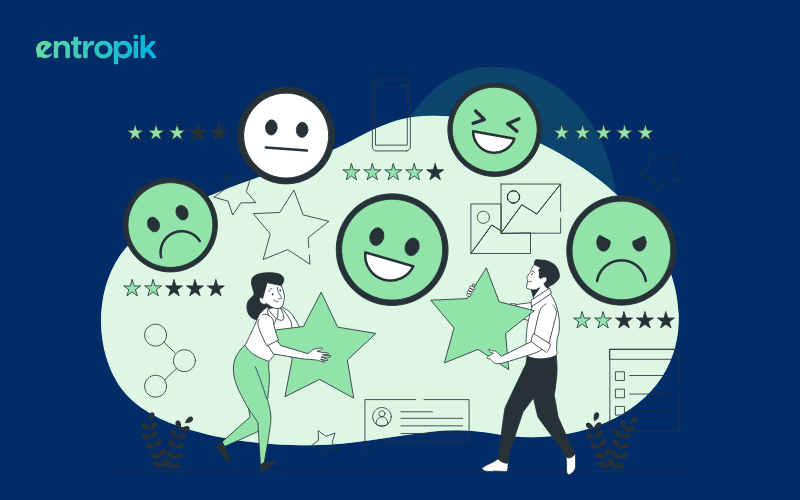
Customer Effort Score: Definition, Examples, Tips
A great customer score can lead to dedicated, engaged customers who can end up being loyal advocates of your brand. Here's what you need to know about it.

How to Detect and Address User Pain Points for Better Engagement
Understanding user pain points can help you provide a seamless user experiences that makes your users come back for more. Here's what you need to know about it.

What is Quota Sampling? Definition, Types, Examples, and How to Use It?
Discover Quota Sampling: Learn its process, types, and benefits for accurate consumer insights and informed marketing decisions. Perfect for researchers and brand marketers!
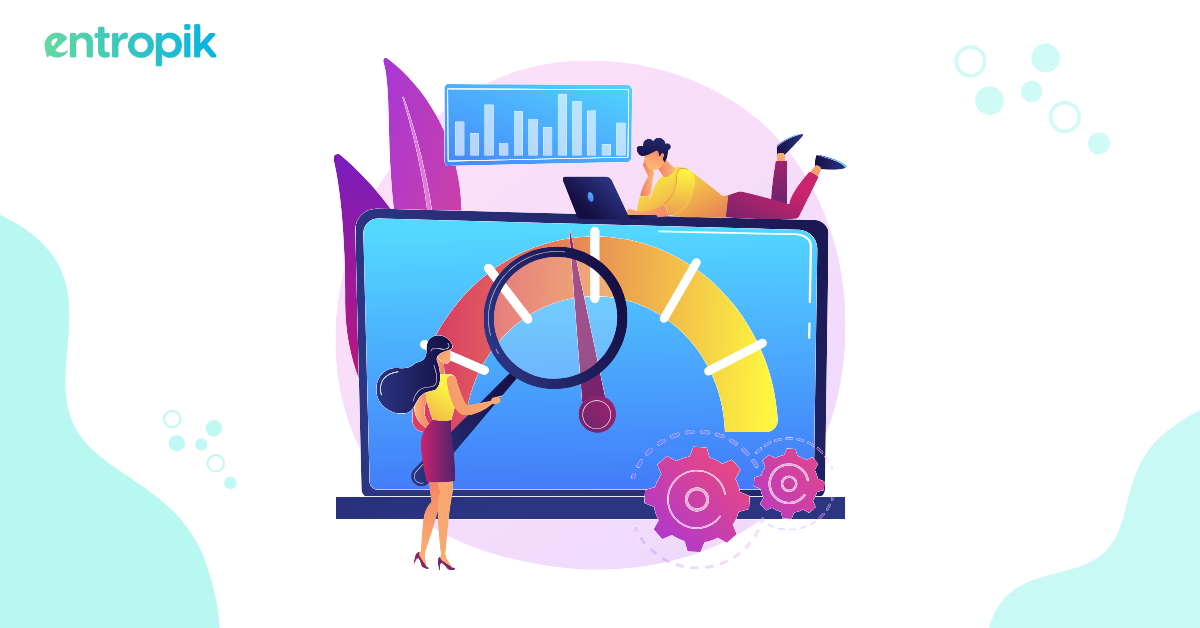
What Is Accessibility Testing? A Comprehensive Guide
Ensure inclusivity and compliance with accessibility standards through thorough testing. Improve user experience and mitigate legal risks. Learn more.
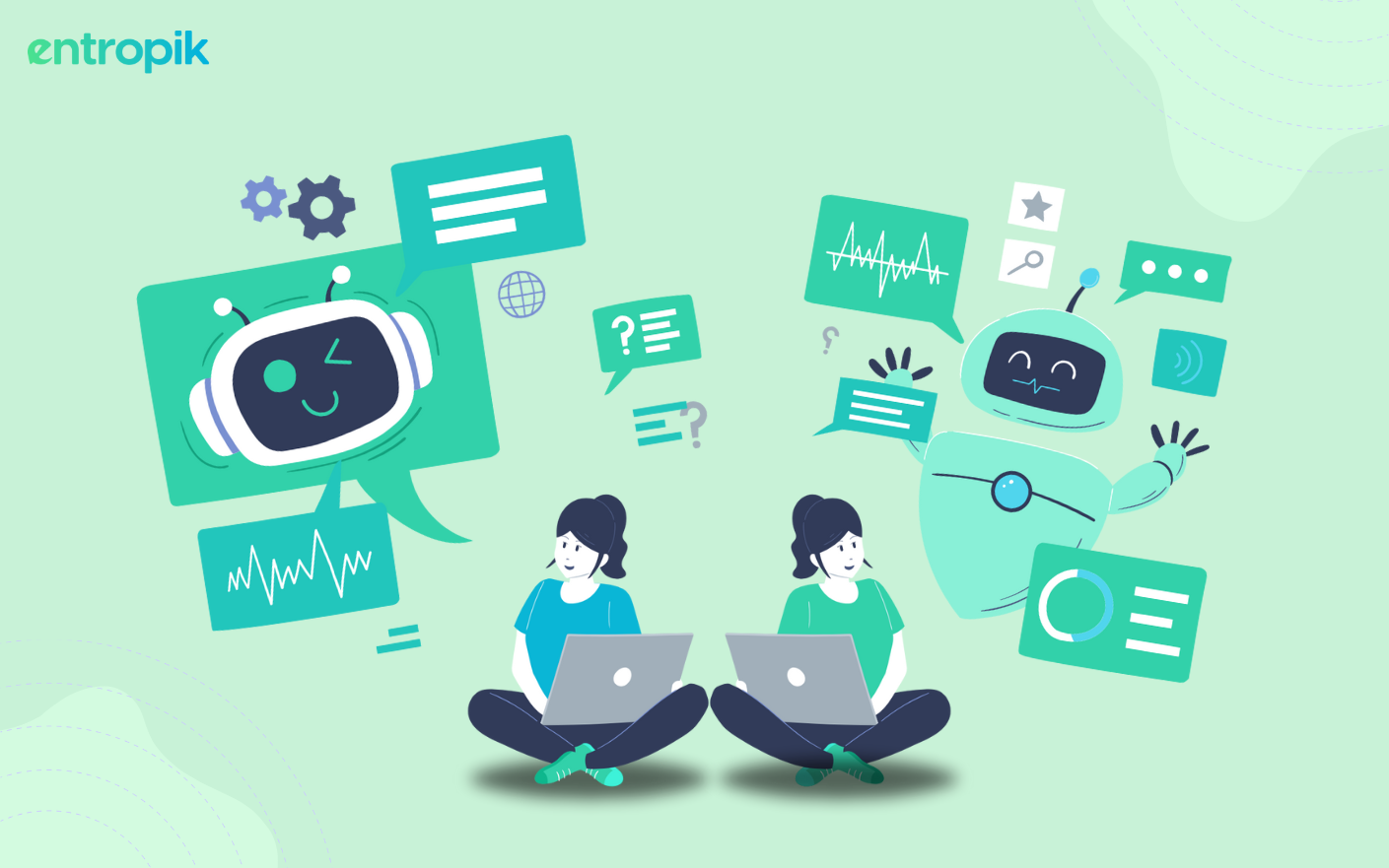
Maximizing Your Research Efficiency with AI Transcriptions
Explore how AI transcription can transform your market research by delivering precise and rapid insights from audio and video recordings.
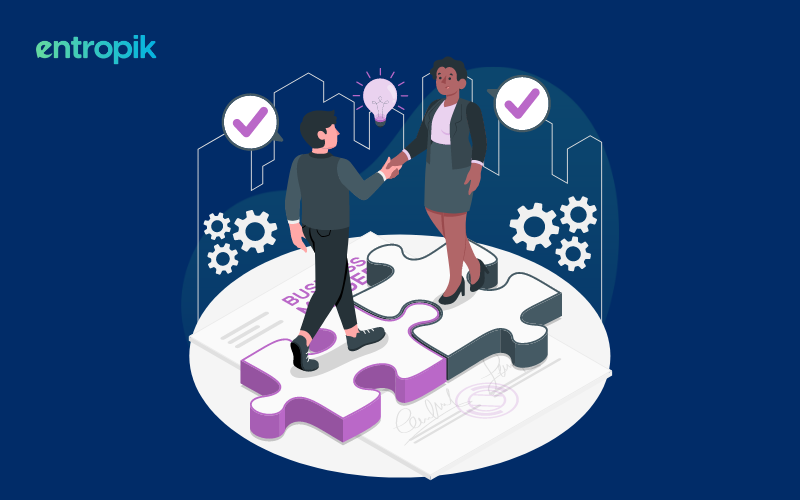
Understanding the False Consensus Effect: How to Manage it
The false consensus effect can cause incorrect assumptions and ultimately, the wrong conclusions. Here's how you can overcome it.

5 Banking Customer Experience Trends to Watch Out for in 2024
Discover the top 5 banking customer experience trends to watch out for in 2024. Stay ahead in the evolving financial landscape.
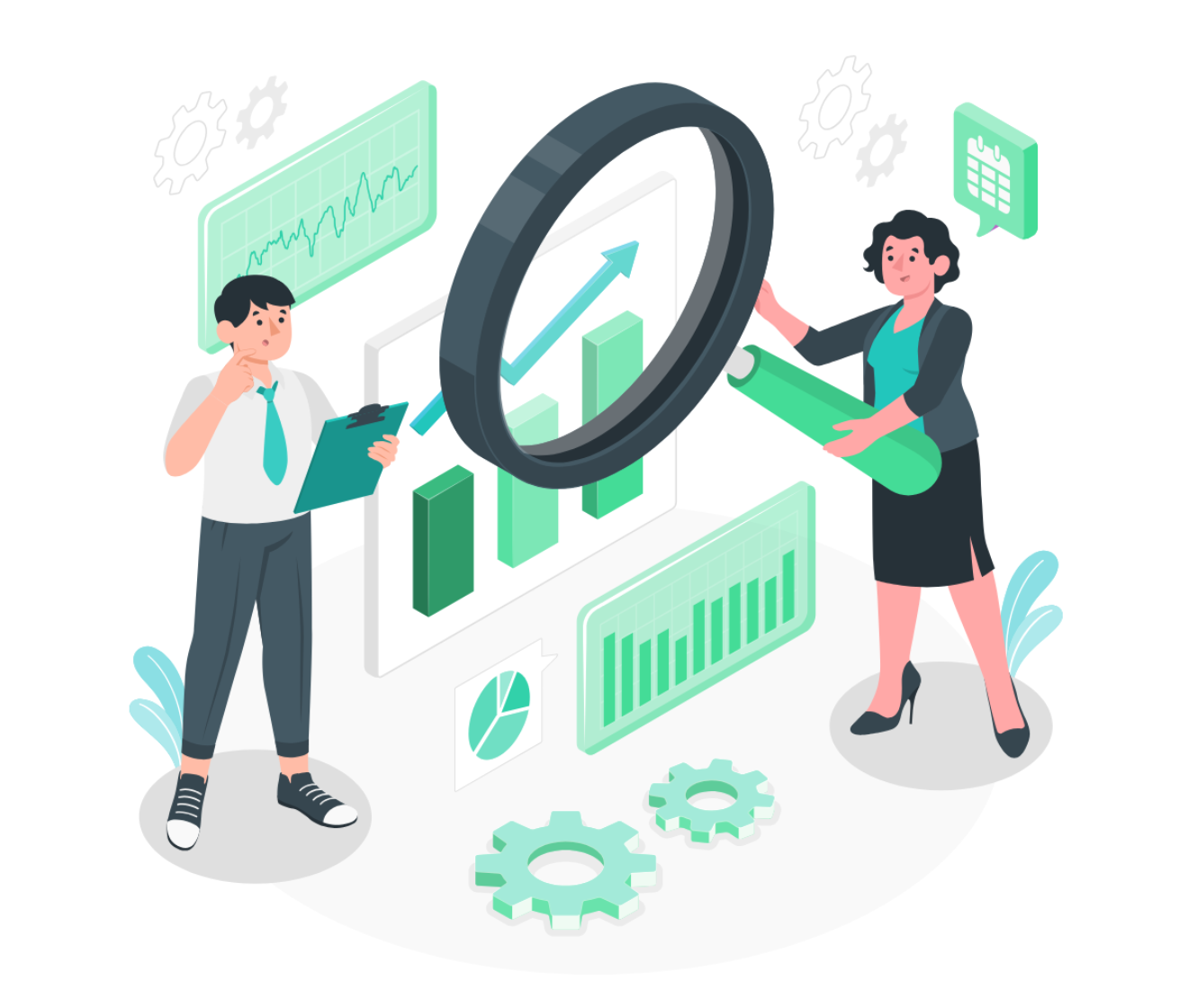
The Ultimate Guide to Regression Analysis: Definition, Types, Usage & Advantages
Master Regression Analysis: Learn types, uses & benefits in consumer research for precise insights & strategic decisions.
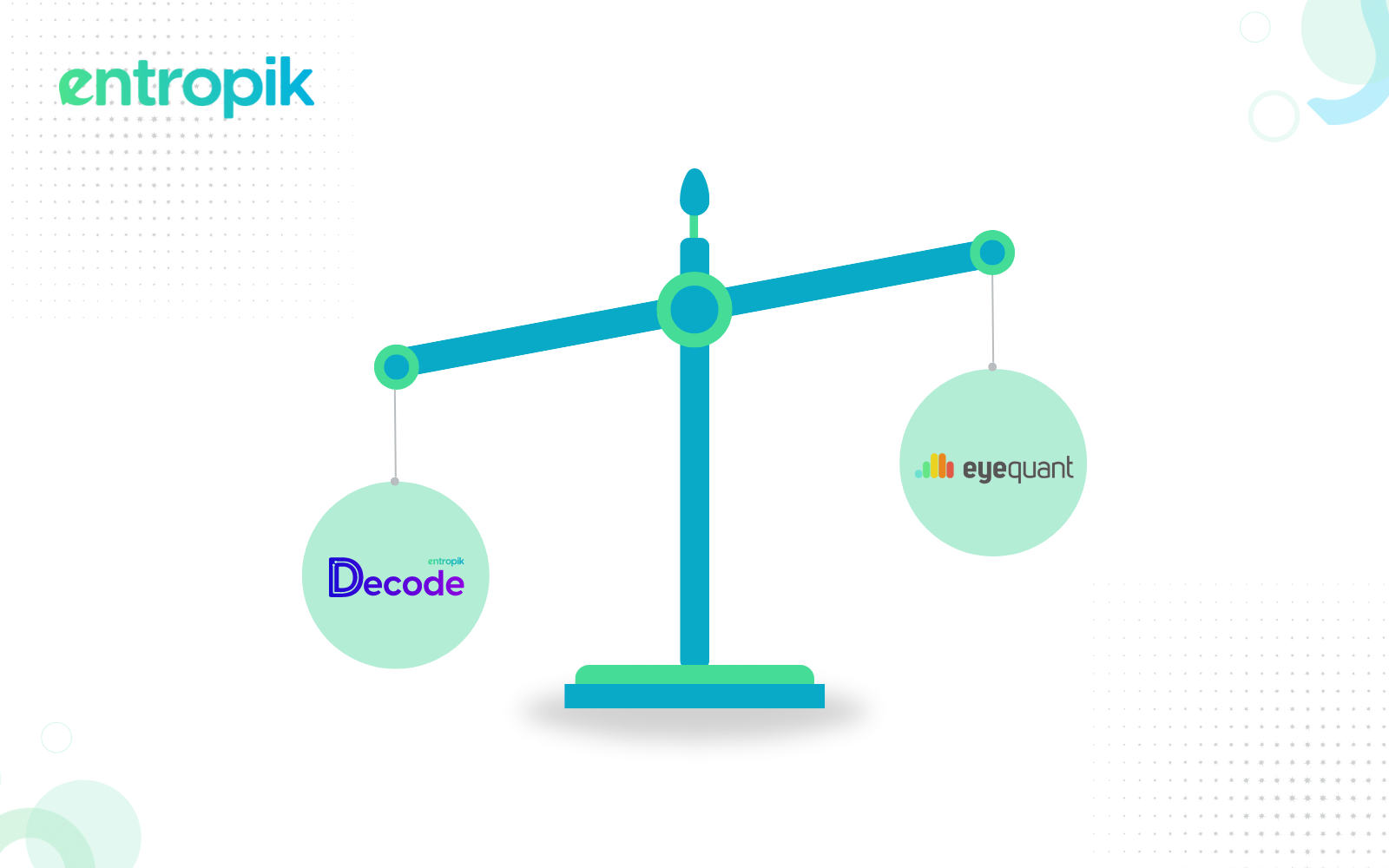
EyeQuant Alternative
Meet Qatalyst, your best eyequant alternative to improve user experience and an AI-powered solution for all your user research needs.

EyeSee Alternative
Embrace the Insights AI revolution: Meet Decode, your innovative solution for consumer insights, offering a compelling alternative to EyeSee.
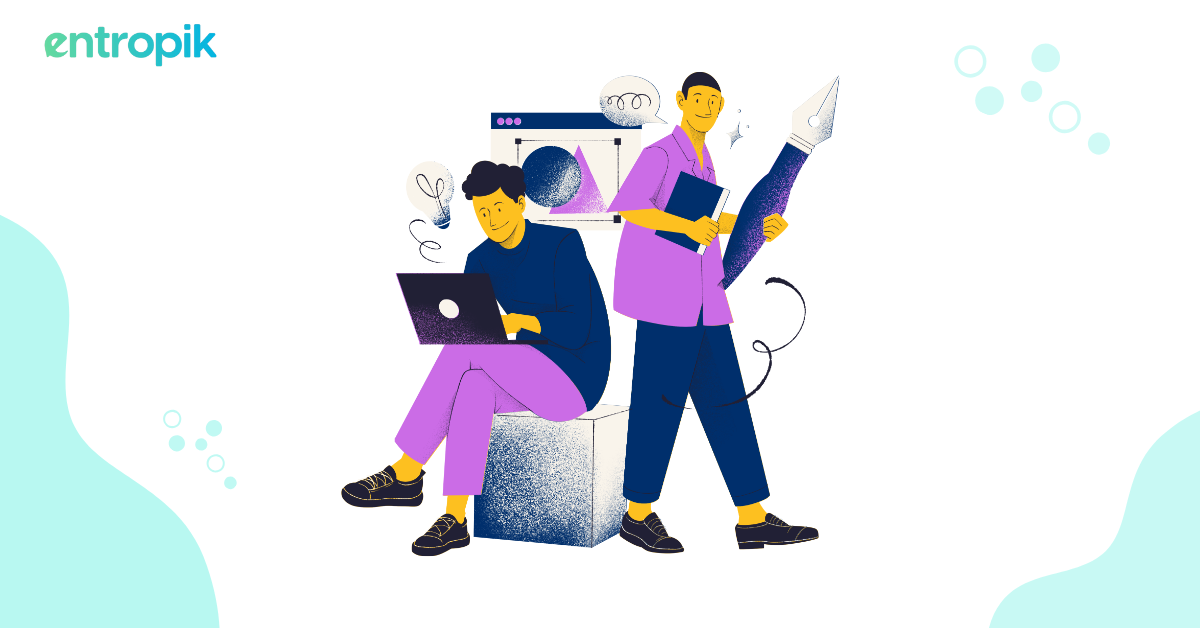
Skeuomorphism in UX Design: Is It Dead?
Skeuomorphism in UX design creates intuitive interfaces using familiar real-world visuals to help users easily understand digital products. Do you know how?
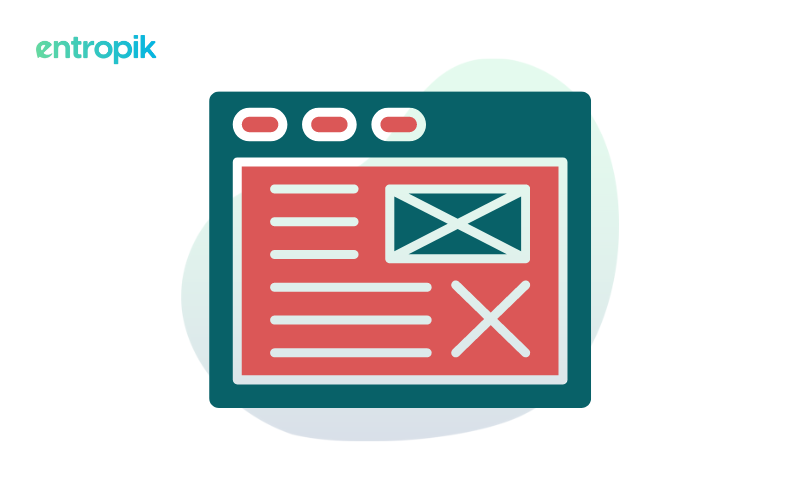
Top 6 Wireframe Tools and Ways to Test Your Designs
Wireframe tools assist designers in planning and visualizing the layout of their websites. Look through this list of wireframing tools to find the one that suits you best.
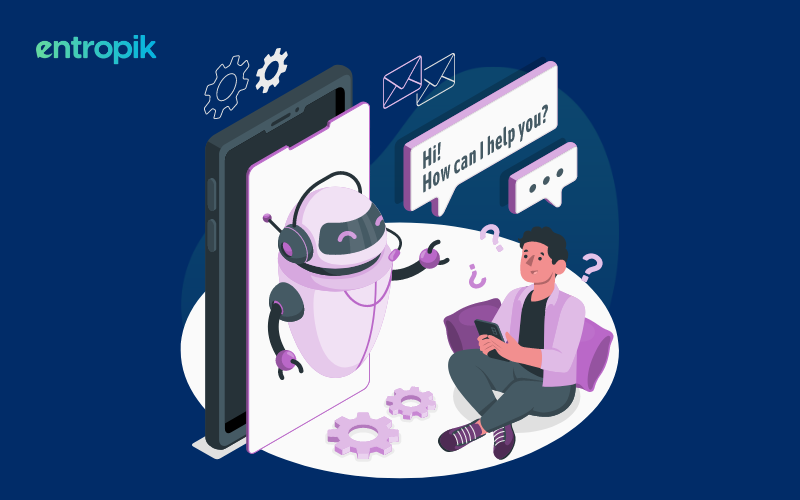
Revolutionizing Customer Interaction: The Power of Conversational AI
Conversational AI enhances customer service across various industries, offering intelligent, context-aware interactions that drive efficiency and satisfaction. Here's how.
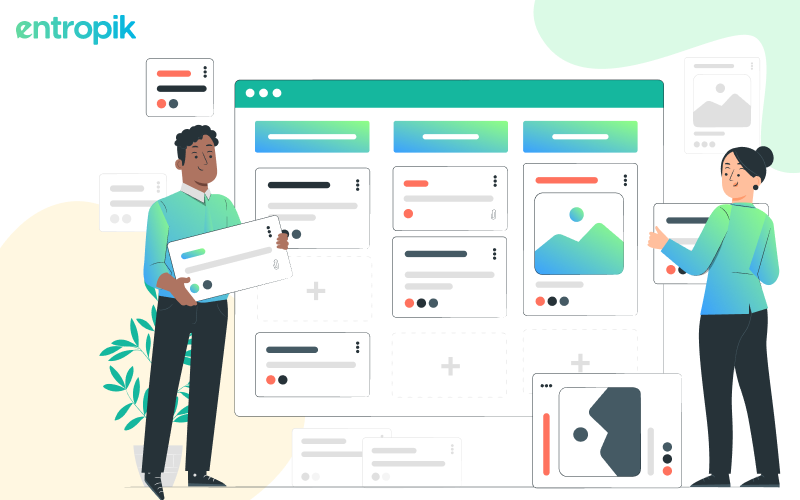
User Story Mapping: A Powerful Tool for User-Centered Product Development
Learn about user story mapping and how it can be used for successful product development with this blog.
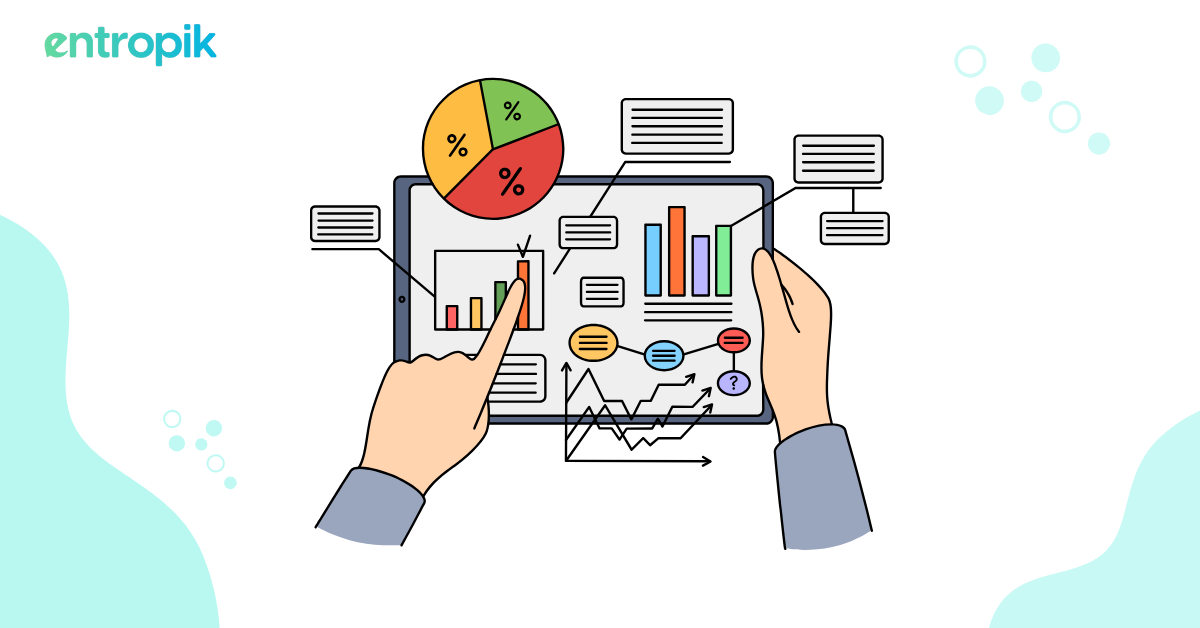
What is Research Hypothesis: Definition, Types, and How to Develop
Read the blog to learn how a research hypothesis provides a clear and focused direction for a study and helps formulate research questions.

Understanding Customer Retention: How to Keep Your Customers Coming Back
Understanding customer retention is key to building a successful brand that has repeat, loyal customers. Here's what you need to know about it.
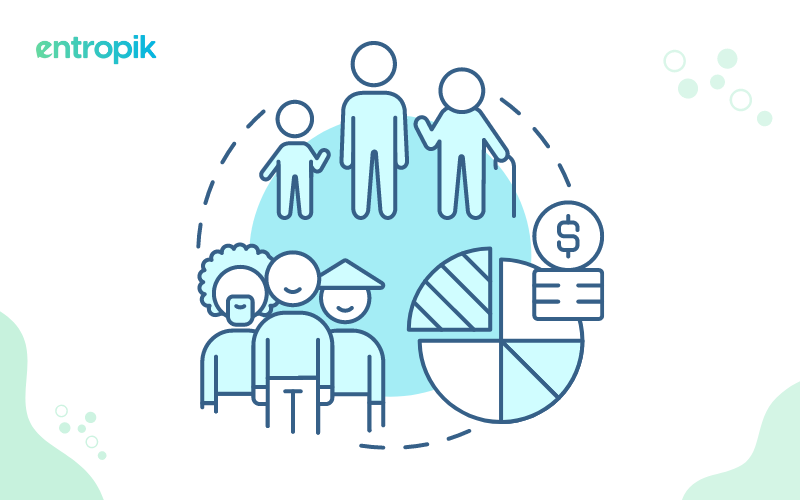
Demographic Segmentation: How Brands Can Use it to Improve Marketing Strategies
Read this blog to learn what demographic segmentation means, its importance, and how it can be used by brands.

Mastering Product Positioning: A UX Researcher's Guide
Read this blog to understand why brands should have a well-defined product positioning and how it affects the overall business.

Discrete Vs. Continuous Data: Everything You Need To Know
Explore the differences between discrete and continuous data and their impact on business decisions and customer insights.
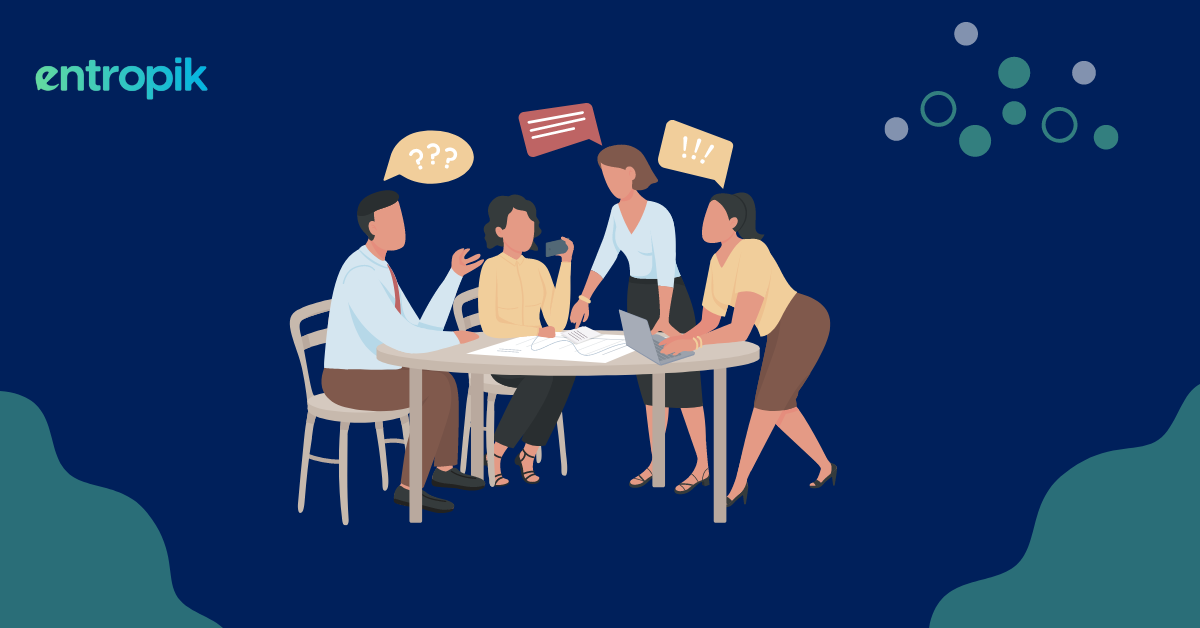
50+ Employee Engagement Survey Questions
Understand how an employee engagement survey provides insights into employee satisfaction and motivation, directly impacting productivity and retention.

What is Experimental Research: Definition, Types & Examples
Understand how experimental research enables researchers to confidently identify causal relationships between variables and validate findings, enhancing credibility.

A Guide to Interaction Design
Interaction design can help you create engaging and intuitive user experiences, improving usability and satisfaction through effective design principles. Here's how.
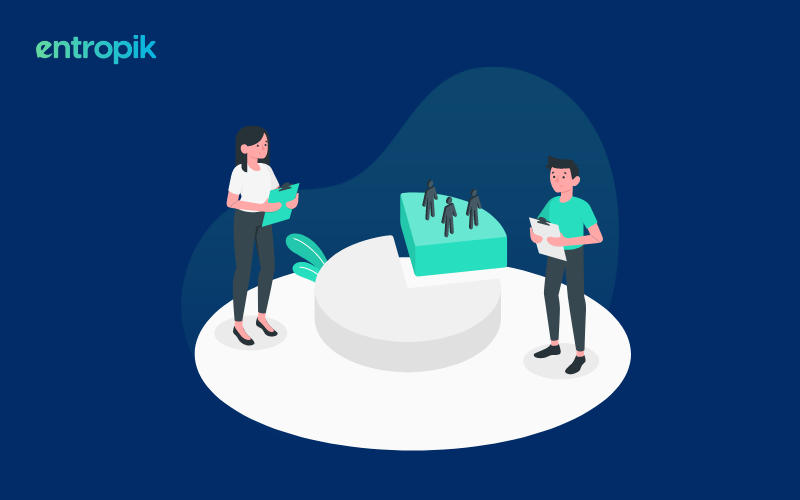
Exploring the Benefits of Stratified Sampling
Understanding stratified sampling can improve research accuracy by ensuring diverse representation across key subgroups. Here's how.
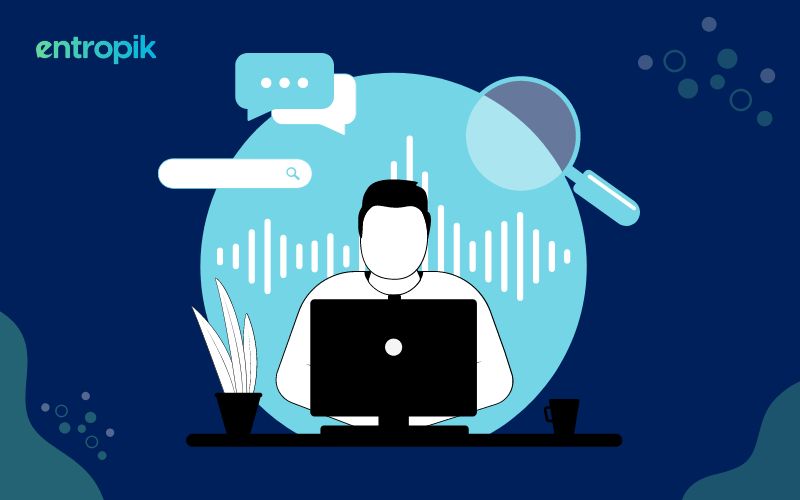
A Guide to Voice Recognition in Enhancing UX Research
Learn the importance of using voice recognition technology in user research for enhanced user feedback and insights.
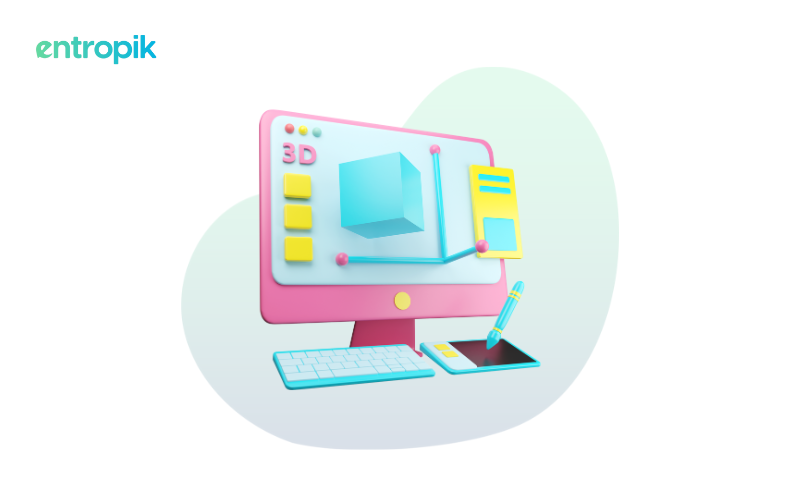
The Ultimate Figma Design Handbook: Design Creation and Testing
The Ultimate Figma Design Handbook covers setting up Figma, creating designs, advanced features, prototyping, and testing designs with real users.

The Power of Organization: Mastering Information Architectures
Understanding the art of information architectures can enhance user experiences by organizing and structuring digital content effectively, making information easy to find and navigate. Here's how.
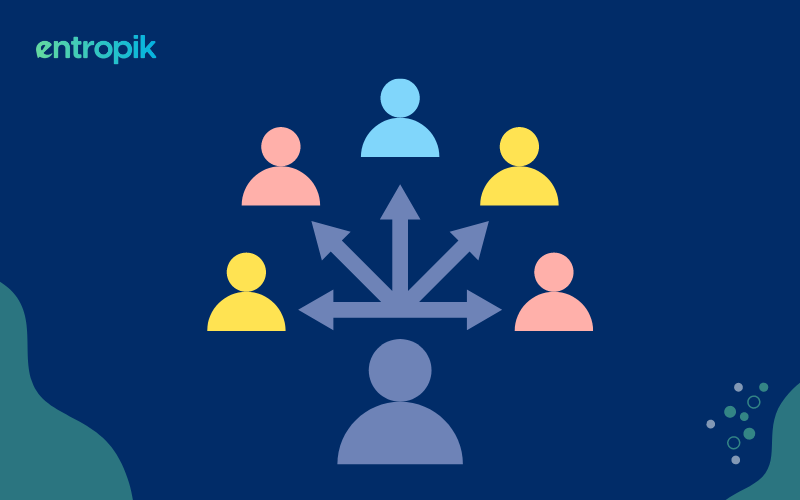
Convenience Sampling: Examples, Benefits, and When To Use It
Read the blog to understand how convenience sampling allows for quick and easy data collection with minimal cost and effort.
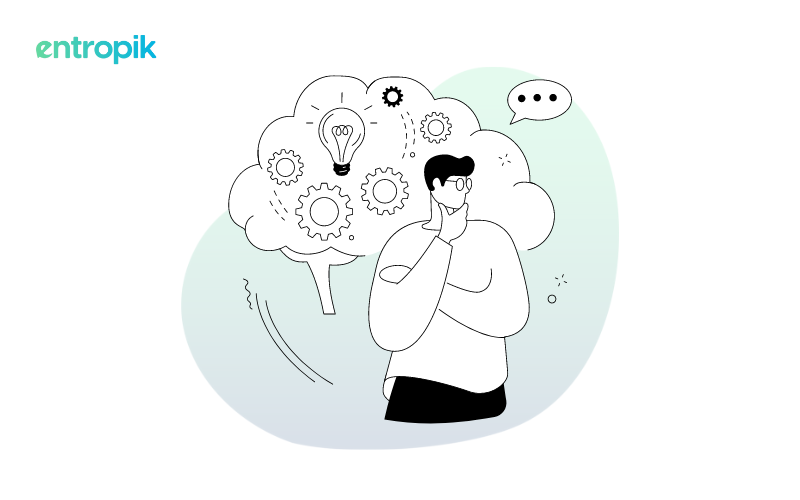
What is Critical Thinking, and How Can it be Used in Consumer Research?
Learn how critical thinking enhances consumer research and discover how Decode's AI-driven platform revolutionizes data analysis and insights.

How Business Intelligence Tools Transform User Research & Product Management
This blog explains how Business Intelligence (BI) tools can transform user research and product management by providing data-driven insights for better decision-making.
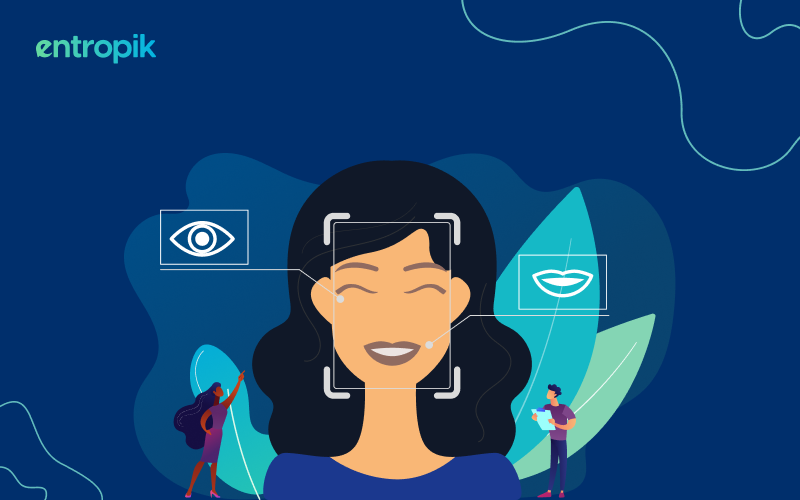
What is Face Validity? Definition, Guide and Examples
Read this blog to explore face validity, its importance, and the advantages of using it in market research.
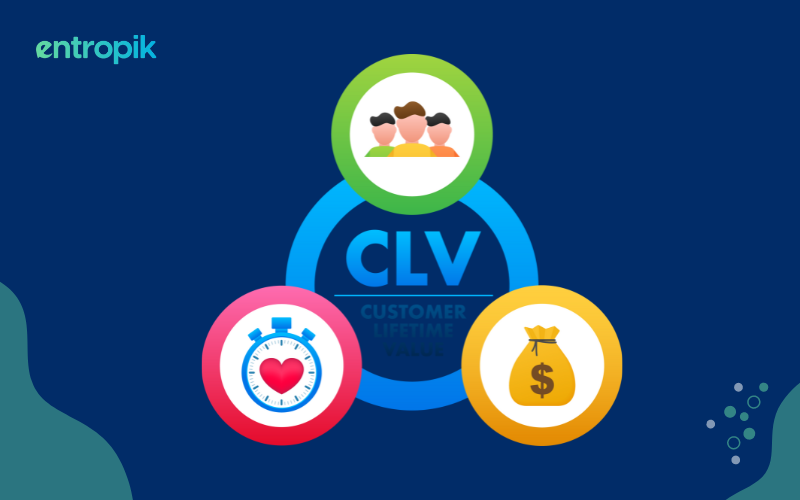
What is Customer Lifetime Value, and How To Calculate It?
Read this blog to understand how Customer Lifetime Value (CLV) can help your business optimize marketing efforts, improve customer retention, and increase profitability.
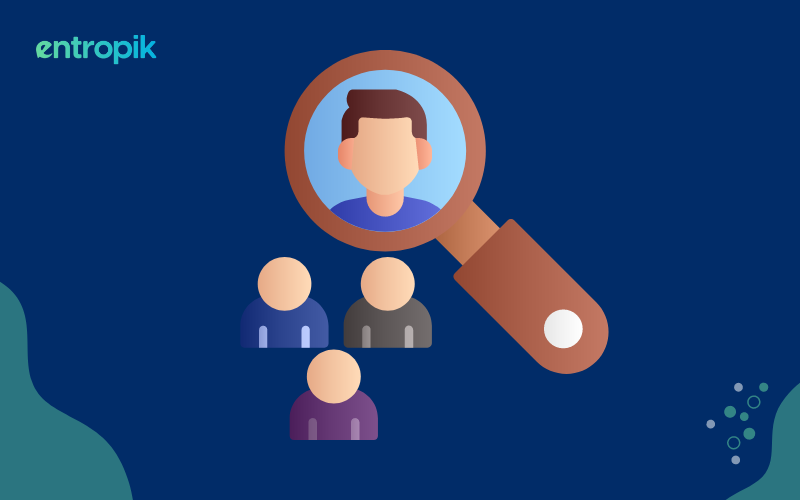
Systematic Sampling: Definition, Examples, and Types
Explore how systematic sampling helps researchers by providing a structured method to select representative samples from larger populations, ensuring efficiency and reducing bias.
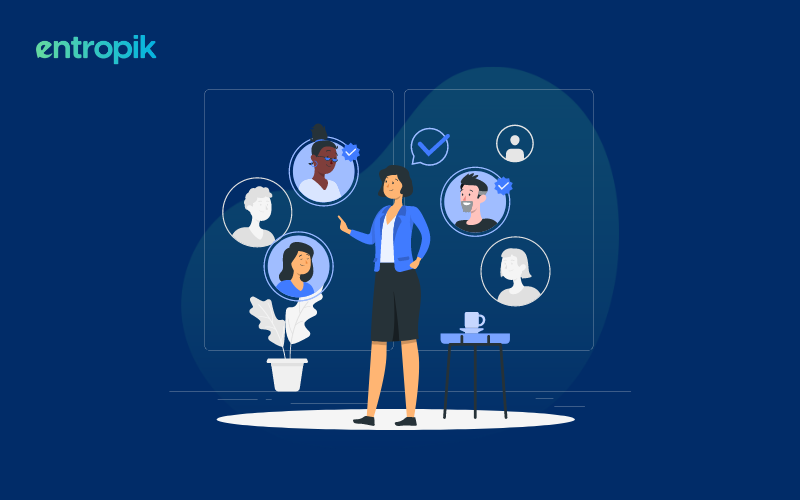
Understanding Selection Bias: A Guide
Selection bias can affect the type of respondents you choose for the study and ultimately the quality of responses you receive. Here’s all you need to know about it.
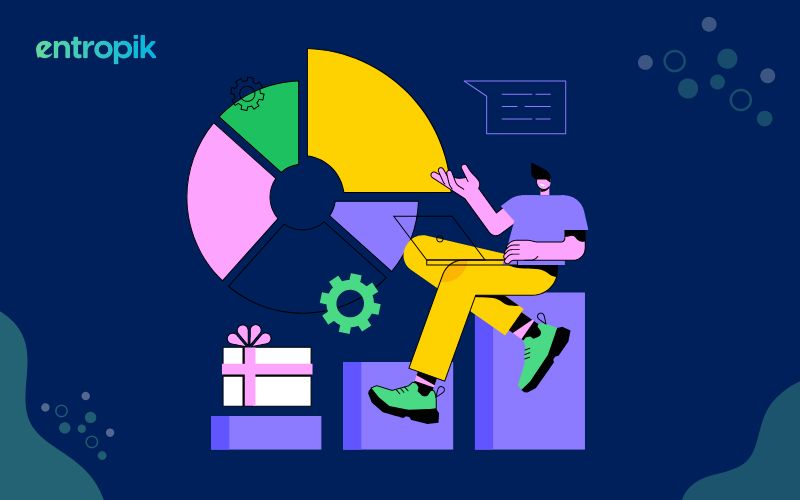
A Guide to Designing an Effective Product Strategy
Read this blog to explore why a well-defined product strategy is required for brands while developing or refining a product.
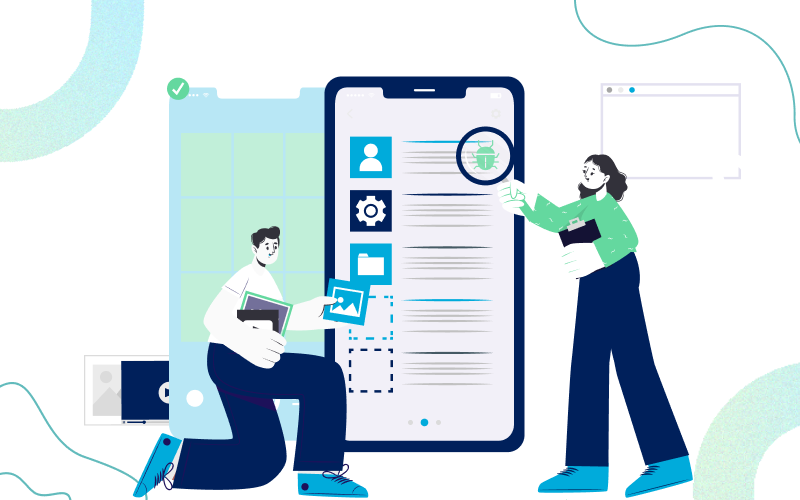
A Guide to Minimum Viable Product (MVP) in UX: Definition, Strategies, and Examples
Discover what an MVP is, why it's crucial in UX, strategies for creating one, and real-world examples from top companies like Dropbox and Airbnb.

Asking Close Ended Questions: A Guide
Asking the right close ended questions is they key to getting quantitiative data from your users. Her's how you should do it.
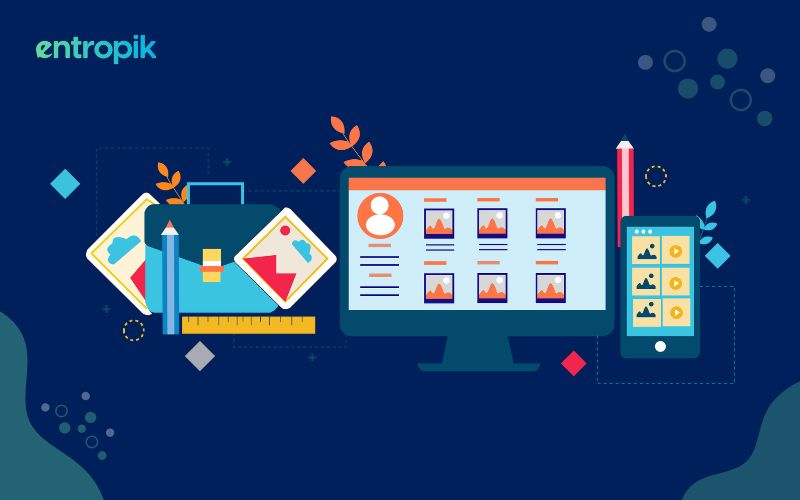
Creating Website Mockups: Your Ultimate Guide to Effective Design
Read this blog to learn website mockups- tools, examples and how to create an impactful website design.
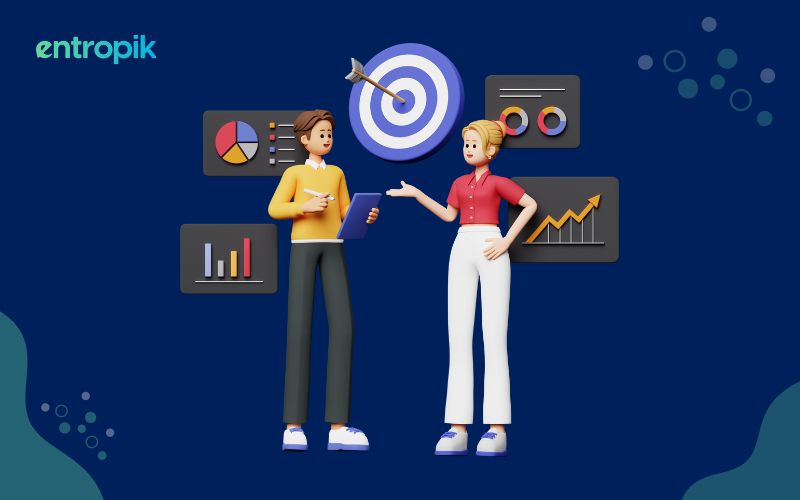
Understanding Your Target Market And Its Importance In Consumer Research
Read this blog to learn about the importance of creating products and services to suit the needs of your target audience.
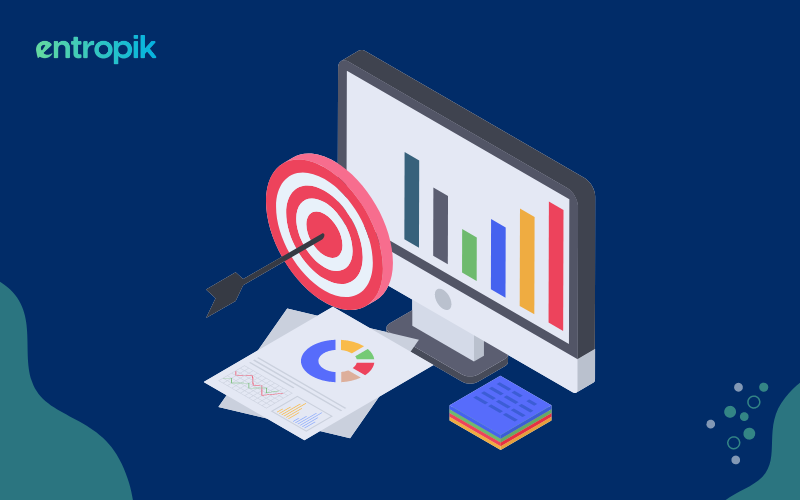
What Is a Go-To-Market Strategy And How to Create One?
Check out this blog to learn how a go-to-market strategy helps businesses enter markets smoothly, attract more customers, and stand out from competitors.
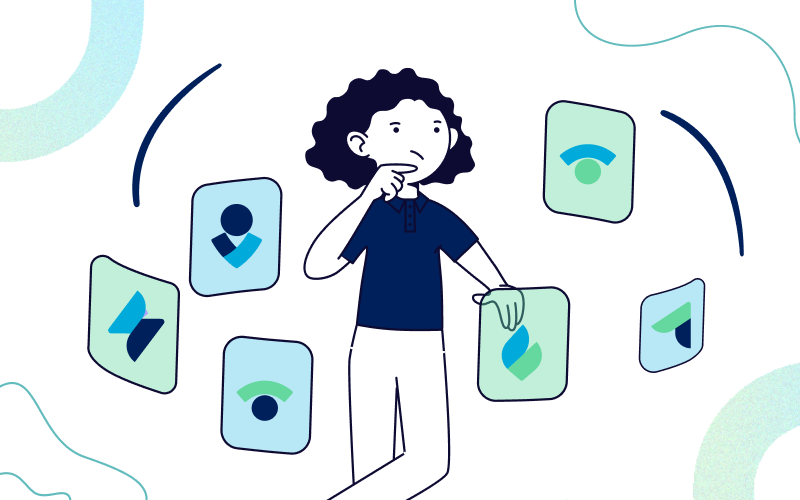
What is Confirmation Bias in Consumer Research?
Learn how confirmation bias affects consumer research, its types, impacts, and practical tips to avoid it for more accurate and reliable insights.
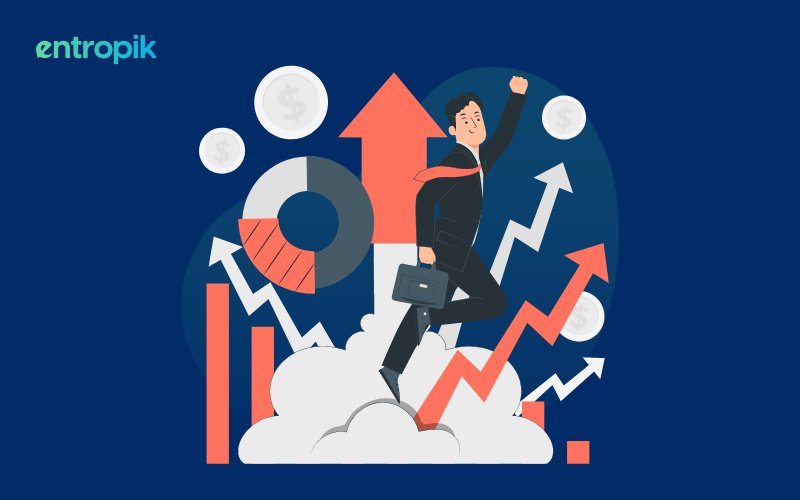
Market Penetration: The Key to Business Success
Understanding market penetration is key to cracking the code to sustained business growth and competitive advantage in any industry. Here's all you need to know about it.
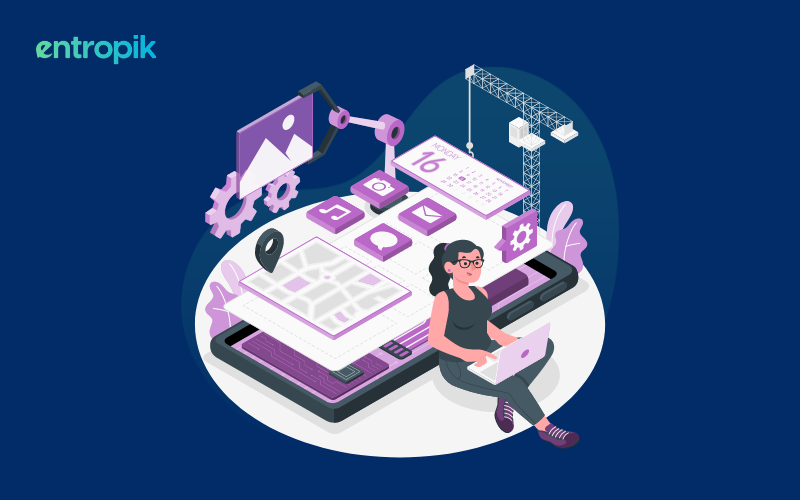
How to Create an Effective User Interface
Having a simple, clear user interface helps your users find what they really want, improving the user experience. Here's how you can achieve it.
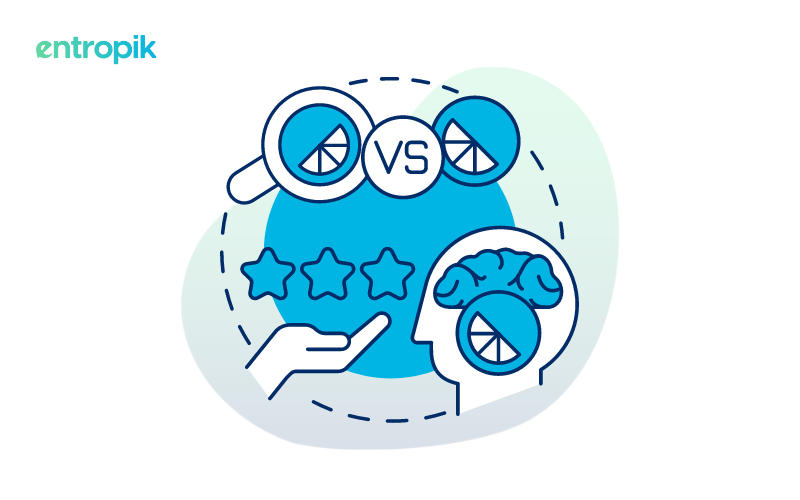
Product Differentiation and What It Means for Your Business
Discover how product differentiation helps businesses stand out with unique features, innovative designs, and exceptional customer experiences.
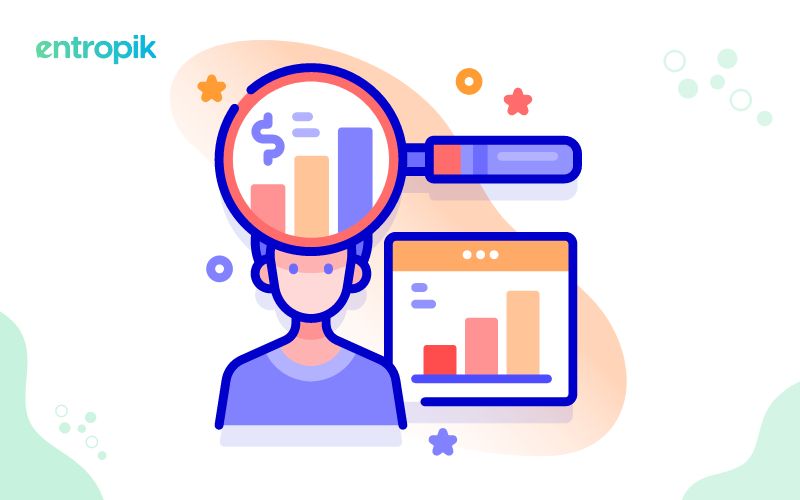
What is Ethnographic Research? Definition, Types & Examples
Read this blog to understand Ethnographic research, its relevance in today’s business landscape and how you can leverage it for your business.
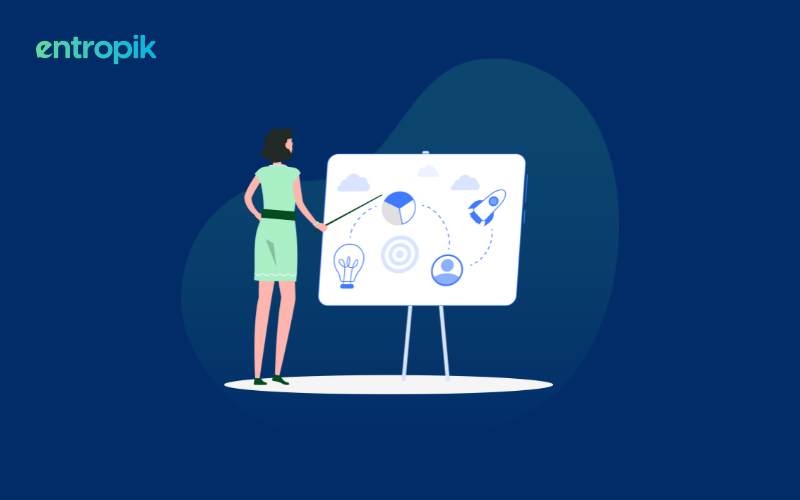
Product Roadmap: The 2024 Guide [with Examples]
Read this blog to understand how a product roadmap can align stakeholders by providing a clear product development and delivery plan.
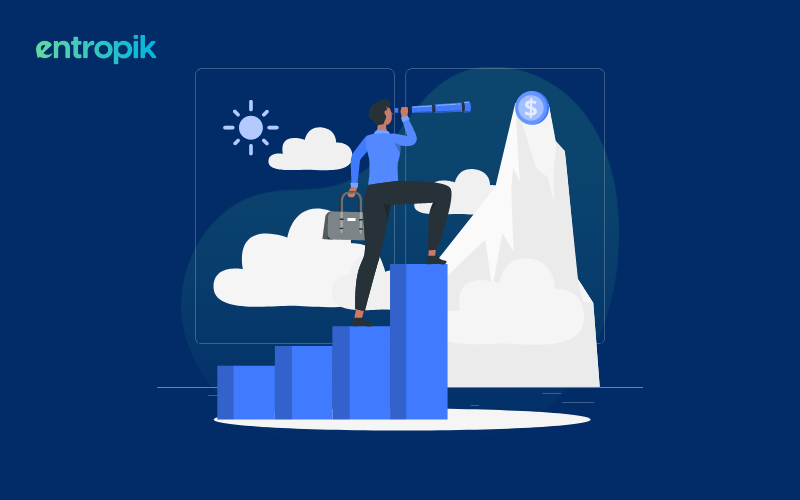
Product Market Fit: Making Your Products Stand Out in a Crowded Market
Delve into the concept of product-market fit, explore its significance, and equip yourself with practical insights to achieve it effectively.
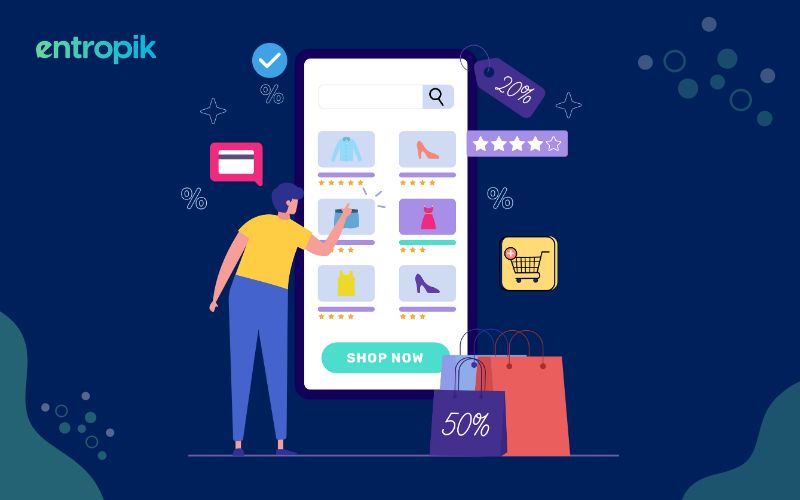
Consumer Behavior in Online Shopping: A Comprehensive Guide
Ever wondered how online shopping behavior can influence successful business decisions? Read on to learn more.

How to Conduct a First Click Test?
Why are users leaving your site so fast? Learn how First Click Testing can help. Discover quick fixes for frustration and boost engagement.
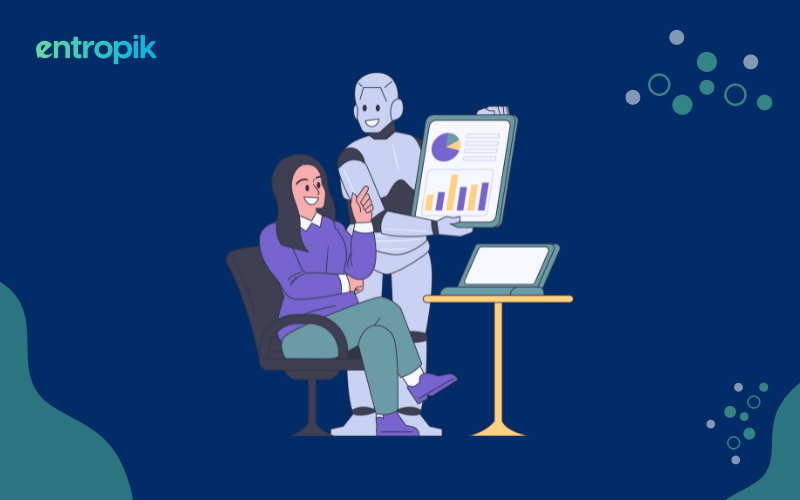
What is Market Intelligence? Methods, Types, and Examples
Read the blog to understand how marketing intelligence helps you understand consumer behavior and market trends to inform strategic decision-making.
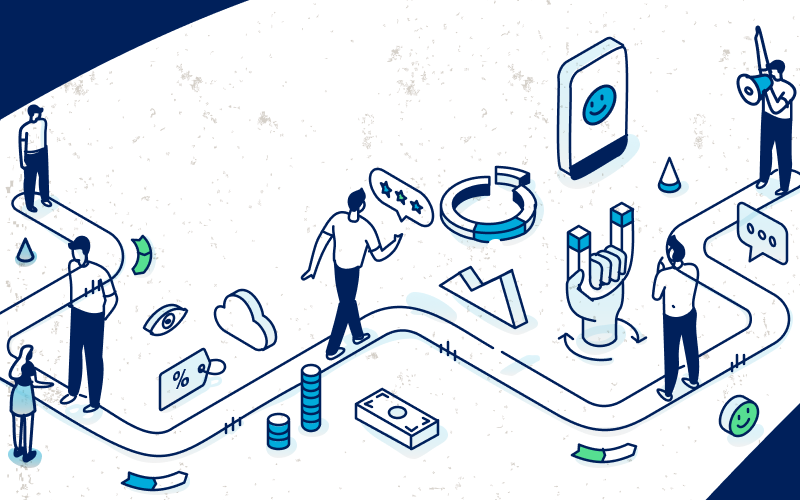
What is a Longitudinal Study? Definition, Types, and Examples
Is your long-term research strategy unclear? Learn how longitudinal studies decode complexity. Read on for insights.
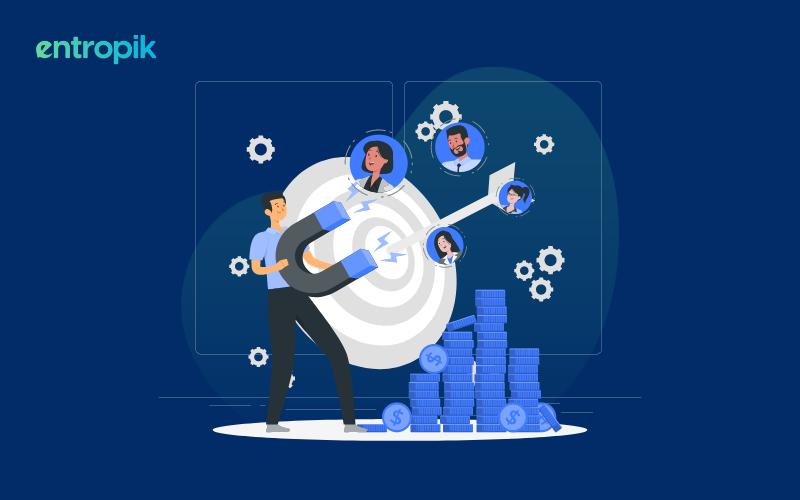
What Is the Impact of Customer Churn on Your Business?
Understanding and reducing customer churn is the key to building a healthy business that keeps customers satisfied. Here's all you need to know about it.
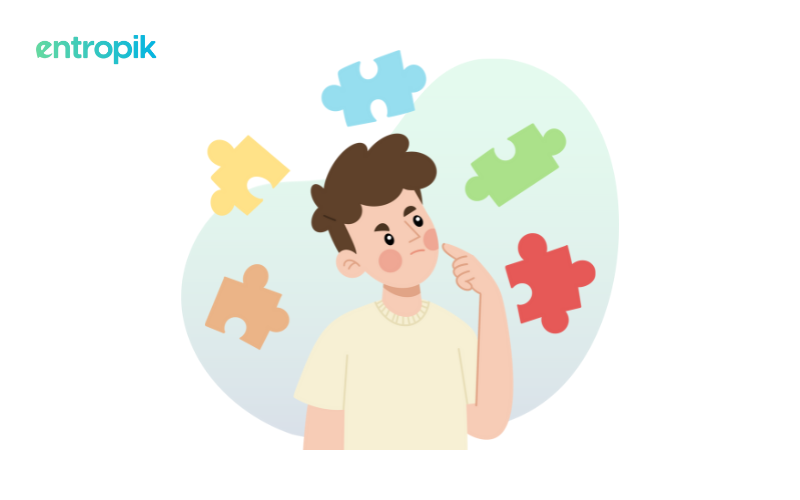
The Ultimate Design Thinking Guide
Discover the power of design thinking in UX design for your business. Learn the process and key principles in our comprehensive guide.
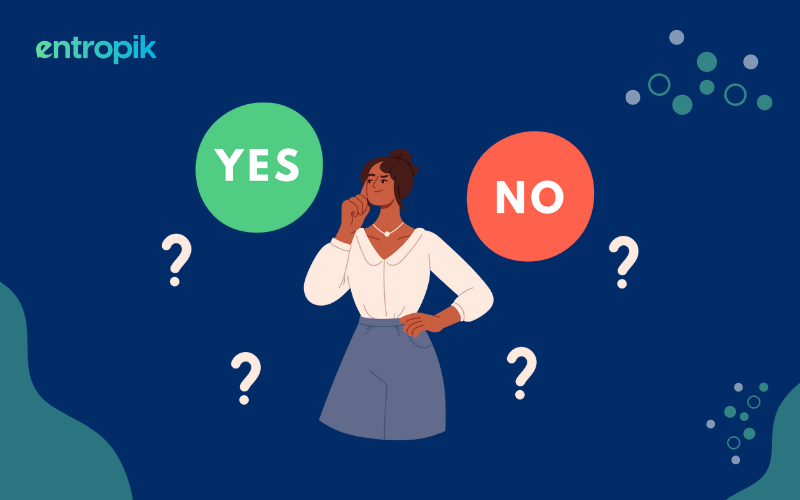
100+ Yes Or No Survey Questions Examples
Yes or no survey questions simplify responses, aiding efficiency, clarity, standardization, quantifiability, and binary decision-making. Read some examples!

What is Customer Segmentation? The ULTIMATE Guide
Explore how customer segmentation targets diverse consumer groups by tailoring products, marketing, and experiences to their preferred needs.
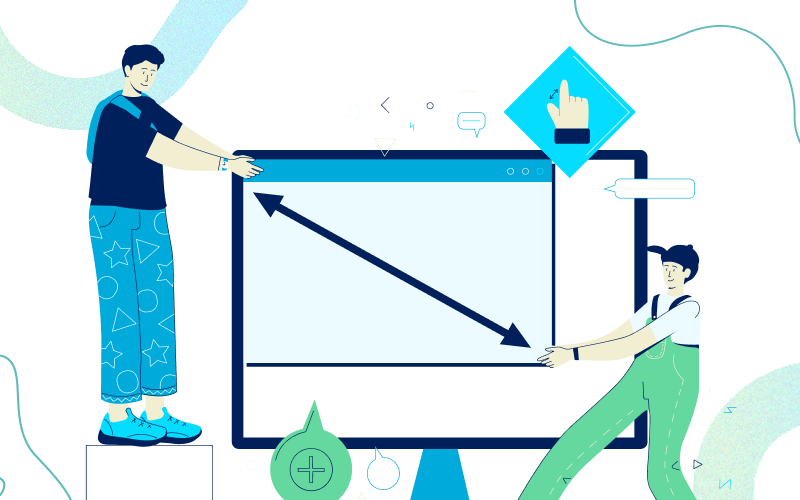
Crafting User-Centric Websites Through Responsive Web Design
Find yourself reaching for your phone instead of a laptop for regular web browsing? Read on to find out what that means & how you can leverage it for business.

How Does Product Placement Work? Examples and Benefits
Read the blog to understand how product placement helps advertisers seek subtle and integrated ways to promote their products within entertainment content.
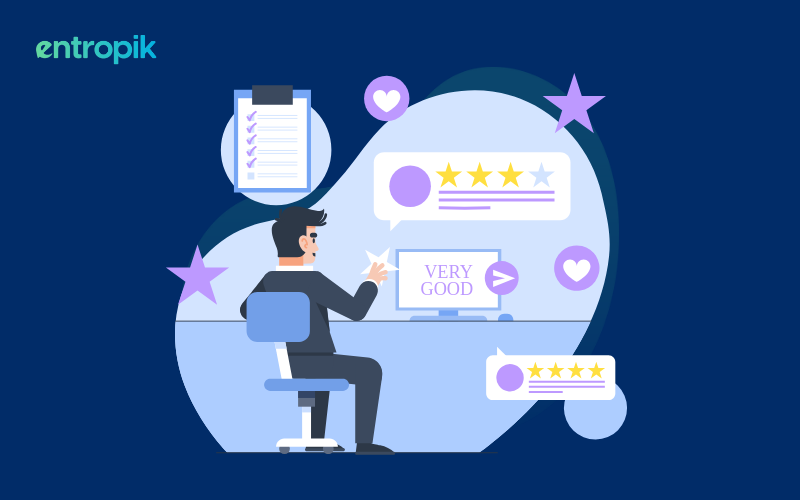
The Importance of Reputation Management, and How it Can Make or Break Your Brand
A good reputation management strategy is crucial for any brand that wants to keep its customers loyal. Here's how brands can focus on it.

A Comprehensive Guide to Human-Centered Design
Are you putting the human element at the center of your design process? Read this blog to understand why brands must do so.

How to Leverage Customer Insights to Grow Your Business
Genuine insights are becoming increasingly difficult to collect. Read on to understand the challenges and what the future holds for customer insights.
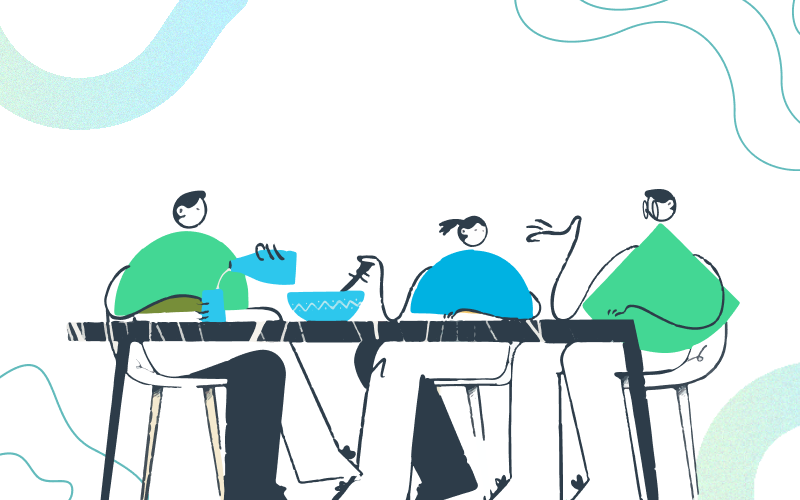
The Complete Guide to Behavioral Segmentation
Struggling to reach your target audience effectively? Discover how behavioral segmentation can transform your marketing approach. Read more in our blog!

Creating a Unique Brand Identity: How to Make Your Brand Stand Out
Creating a great brand identity goes beyond creating a memorable logo - it's all about creating a consistent and unique brand experience for your cosnumers. Here's everything you need to know about building one.

Understanding the Product Life Cycle: A Comprehensive Guide
Understanding the product life cycle, or the stages a product goes through from its launch to its sunset can help you understand how to market it at every stage to create the most optimal marketing strategies.
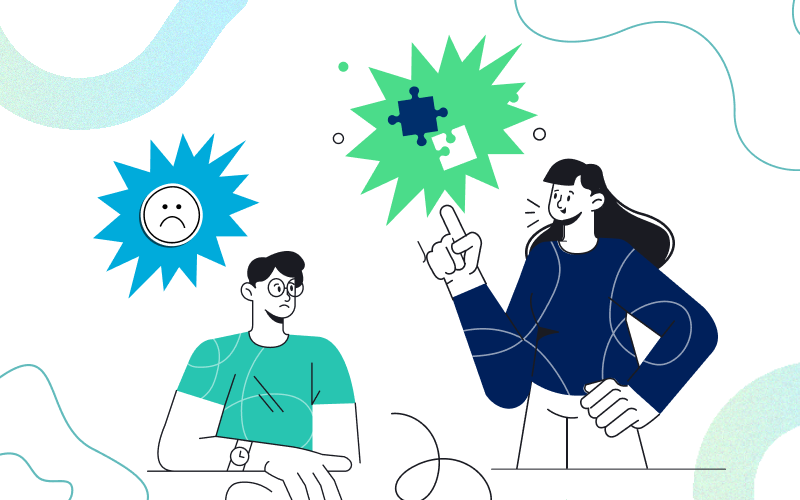
Empathy vs. Sympathy in UX Research
Are you conducting UX research and seeking guidance on conducting user interviews with empathy or sympathy? Keep reading to discover the best approach.

What is Exploratory Research, and How To Conduct It?
Read this blog to understand how exploratory research can help you uncover new insights, patterns, and hypotheses in a subject area.

First Impressions & Why They Matter in User Research
Ever wonder if first impressions matter in user research? The answer might surprise you. Read on to learn more!

Cluster Sampling: Definition, Types & Examples
Read this blog to understand how cluster sampling tackles the challenge of efficiently collecting data from large, spread-out populations.
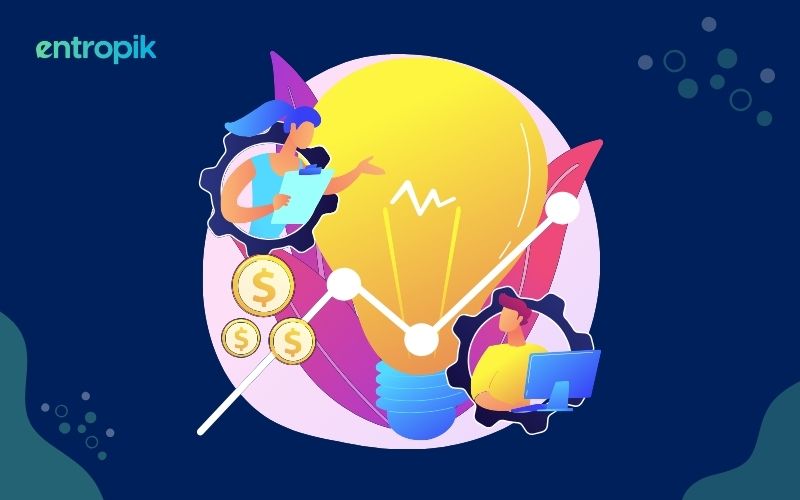
Top Six Market Research Trends
Curious about where market research is headed? Read on to learn about the changes surrounding this field in 2024 and beyond.
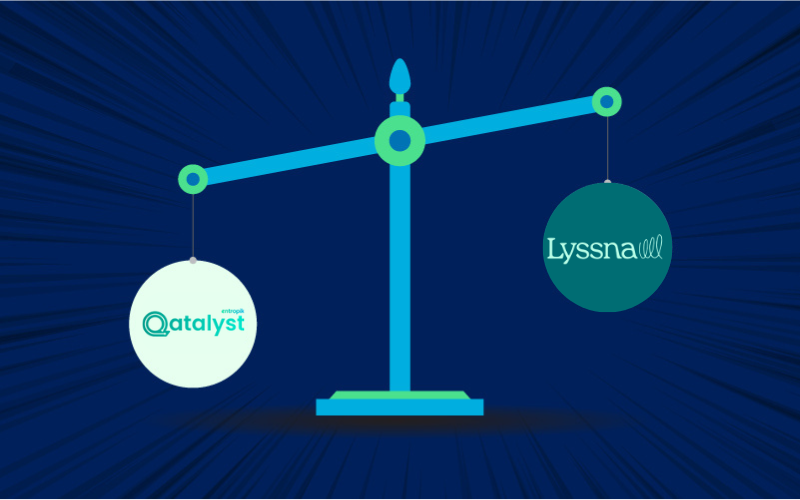
Lyssna Alternative
Meet Qatalyst, your best lyssna alternative to usability testing, to create a solution for all your user research needs.

What is Feedback Loop? Definition, Importance, Types, and Best Practices
Struggling to connect with your customers? Read the blog to learn how feedback loops can solve your problem!

UI vs. UX Design: What’s The Difference?
Learn how UI solves the problem of creating an intuitive and visually appealing interface and how UX addresses broader issues related to user satisfaction and overall experience with the product or service.

The Impact of Conversion Rate Optimization on Your Business
Understanding conversion rate optimization can help you boost your online business. Read more to learn all about it.
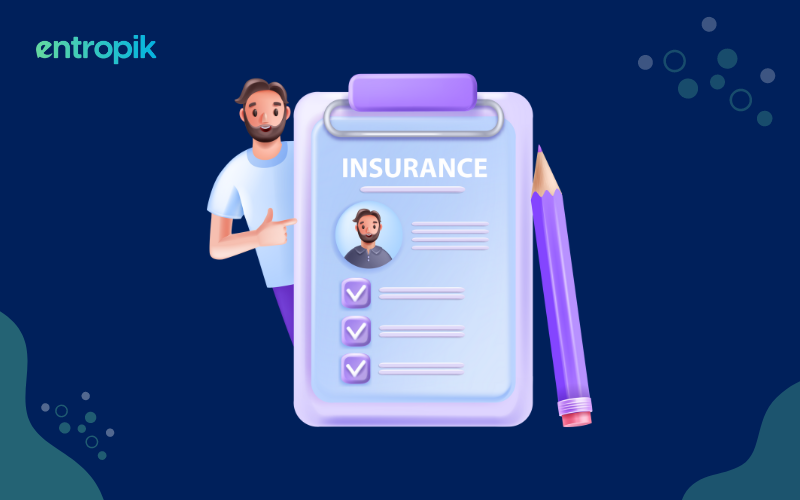
Insurance Questionnaire: Tips, Questions and Significance
Leverage this pre-built customizable questionnaire template for insurance to get deep insights from your audience.

UX Research Plan Template
Read on to understand why you need a UX Research Plan and how you can use a fully customizable template to get deep insights from your users!
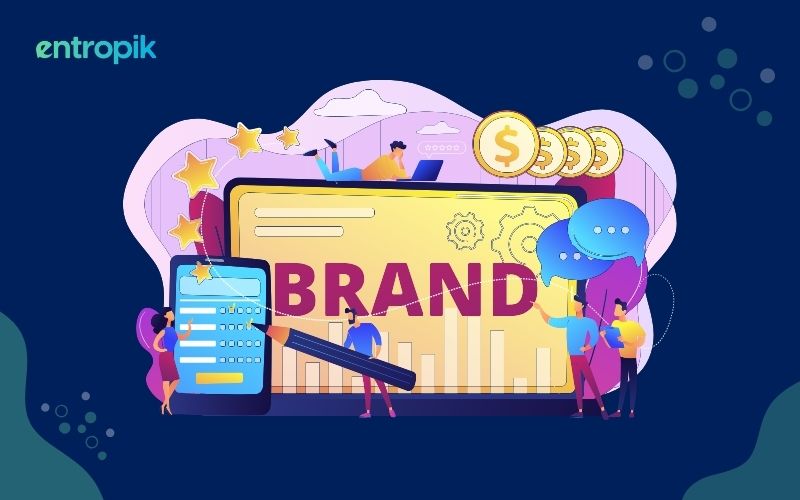
Brand Experience: What it Means & Why It Matters
Have you ever wondered how users navigate the travel industry for your research insights? Read on to understand user experience in the travel sector.

Validity in Research: Definitions, Types, Significance, and Its Relationship with Reliability
Is validity ensured in your research process? Read more to explore the importance and types of validity in research.
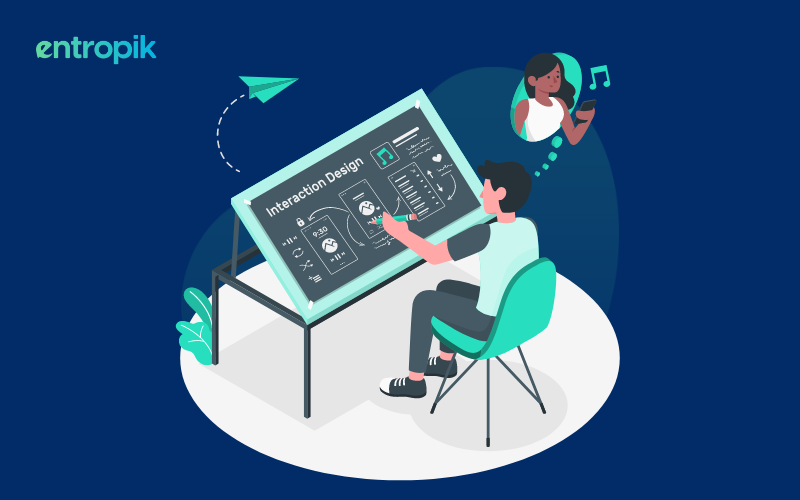
The Role of UI Designers in Creating Delightful User Interfaces
UI designers help to create aesthetic and functional experiences for users. Here's all you need to know about them.

Top Usability Testing Tools to Try
Using usability testing tools can help you understand user preferences and behaviors and ultimately, build a better digital product. Here are the top tools you should be aware of.

Understanding User Experience in Travel Market Research
Ever wondered how users navigate the travel industry for your research insights? Read on to understand user experience in the travel sector.
Maximize Your Research Potential
Experience why teams worldwide trust our Consumer & User Research solutions.
Book a Demo


Qualitative research: open-ended and closed-ended questions
12 april 2019 • 2160 words, 9 min. read Latest update : 30 march 2021

By Pierre-Nicolas Schwab

Our guide to market research can be downloaded free of charge
From a very young age, we have been taught what open-ended , and closed-ended questions are. How are these terms applied to qualitative research methods , and in particular to interviews?
Kathryn J. Roulston reveals her definitions of an open-ended and closed-ended question in qualitative interviews in the SAGE Encyclopedia on Qualitative Research Methods . If you want to better understand how qualitative methods fit within a market research approach, we suggest you take a look at our step-by-step guide to market research which can be downloaded in our white papers section (free of charge and direct; we won’t ask you any contact details first).
credits : Shutterstock
Introduction
- Closed-ended question
- Open-ended question
Examples of closed and open-ended questions for satisfaction research
Examples of closed and open-ended questions for innovation research, some practical advice.
Let us begin by pointing out that open and closed-ended questions do not at first glance serve the same purpose in market research. Instead, open-ended questions are used in qualitative research (see the video above for more information) and closed-ended questions are used in quantitative research. But this is not an absolute rule.
In this article, you will, therefore, discover the definitions of closed and open-ended questions. We will also explain how to use them. Finally, you will find examples of how to reformulate closed-ended questions into open-ended questions in the case of :
- satisfaction research
- innovation research
Essential elements to remember
Open-ended questions:
- for qualitative research (interviews and focus groups)
- very useful in understanding in detail the respondent and his or her position concerning a defined topic/situation
- particularly helpful in revealing new aspects , sub-themes, issues, and so forth that are unknown or unidentified
Closed-ended questions:
- for quantitative research (questionnaires and surveys)
- suitable for use with a wide range of respondents
- allow a standardised analysis of the data
- are intended to confirm the hypotheses (previously stated in the qualitative part)
A closed-ended question
A closed-ended question offers, as its name suggests, a limited number of answers. For example, the interviewee may choose a response from a panel of given proposals or a simple “yes” or “no”. They are intended to provide a precise, clearly identifiable and easily classified answer.
This type of question is used in particular during interviews whose purpose is to be encoded according to pre-established criteria. There is no room for free expression, as is the case for open-ended questions. Often, this type of question is integrated into 1-to-1 interview guides and focus groups and allows the interviewer to collect the same information from a wide range of respondents in the same format. Indeed, closed-ended questions are designed and oriented to follow a pattern and framework predefined by the interviewer.
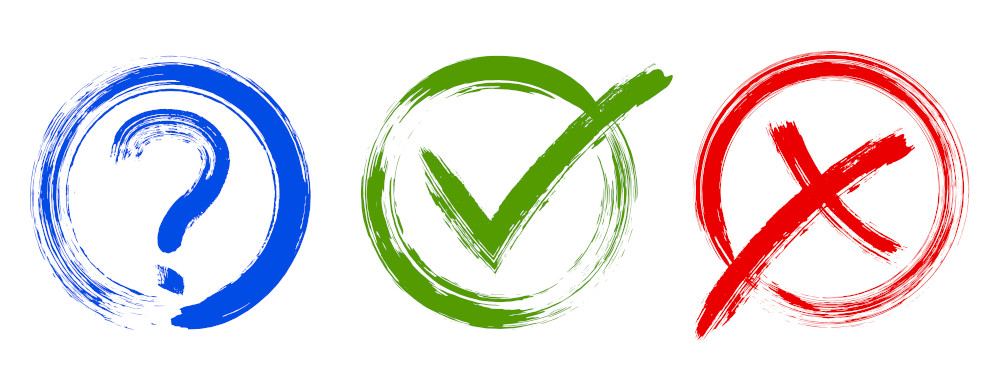
Two forms of closed-ended questions were identified by the researchers: specific closed-ended questions , where respondents are offered choice answers, and implicit closed-ended questions , which include assumptions about the answers that can be provided by respondents.
A specific closed-ended question would be formulated as follows, for example: “how many times a week do you eat pasta: never, once or twice a week, 3 to 4 times, 5 times a week or more?” The adapted version in the form of an implicit closed-ended question would be formulated as follows: “how many times a week do you eat pasta? ». The interviewer then assumes that the answers will be given in figures.
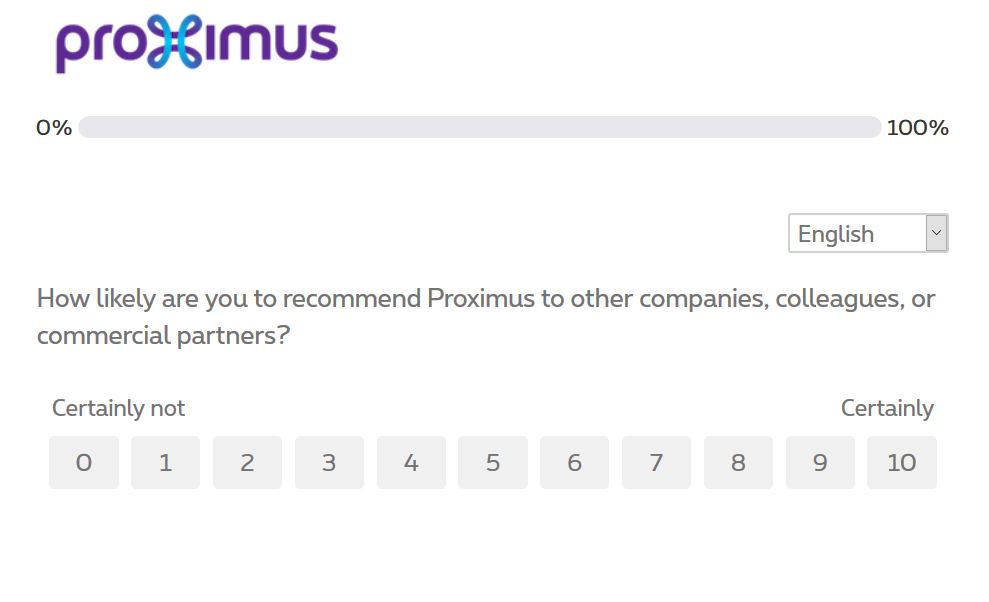
The Net Promoter Score (or NPS) is an example of closed question (see example above)
While some researchers consider the use of closed-ended questions to be restrictive, others see in these questions – combined with open-ended questions – the possibility of generating different data for analysis. How these closed-ended questions can be used, formulated, sequenced, and introduced in interviews depends heavily upon the studies and research conducted upstream.
[call-to-action-read id=”35845″]
In what context are closed-ended questions used?
- Quantitative research (tests, confirmation of the qualitative research and so on).
- Research with a large panel of respondents (> 100 people)
- Recurrent research whose results need to be compared
- When you need confirmation, and the possible answers are limited in effect
An open-ended question
An open-ended question is a question that allows the respondent to express himself or herself freely on a given subject. This type of question is, as opposed to closed-ended questions, non-directive and allows respondents to use their own terms and direct their response at their convenience.
Open-ended questions, and therefore without presumptions, can be used to see which aspect stands out from the answers and thus could be interpreted as a fact, behaviour, reaction, etc. typical to a defined panel of respondents.
For example, we can very easily imagine open-ended questions such as “describe your morning routine”. Respondents are then free to describe their routine in their own words, which is an important point to consider. Indeed, the vocabulary used is also conducive to analysis and will be an element to be taken into account when adapting an interview guide, for example, and/or when developing a quantitative questionnaire.

As we detail in our market research whitepaper , one of the recommendations to follow when using open-ended questions is to start by asking more general questions and end with more detailed questions. For example, after describing a typical day, the interviewer may ask for clarification on one of the aspects mentioned by the respondent. Also, open-ended questions can also be directed so that the interviewee evokes his or her feelings about a situation he or she may have mentioned earlier.
In what context are open-ended questions used?
- Mainly in qualitative research (interviews and focus groups)
- To recruit research participants
- During research to test a design, a proof-of-concept, a prototype, and so on, it is essential to be able to identify the most appropriate solution.
- Analysis of consumers and purchasing behaviour
- Satisfaction research , reputation, customer experience and loyalty research, and so forth.
- To specify the hypotheses that will enable the quantitative questionnaire to be drawn up and to propose a series of relevant answers (to closed-ended questions ).
It is essential for the interviewer to give respondents a framework when using open-ended questions. Without this context, interviewees could be lost in the full range of possible responses, and this could interfere with the smooth running of the interview. Another critical point concerning this type of question is the analytical aspect that follows. Indeed, since respondents are free to formulate their answers, the data collected will be less easy to classify according to fixed criteria.
The use of open-ended questions in quantitative questionnaires
Rules are made to be broken; it is well known. Most quantitative questionnaires, therefore, contain free fields in which the respondent is invited to express his or her opinions in a more “free” way. But how to interpret these answers?
When the quantity of answers collected is small (about ten) it will be easy to proceed manually, possibly by coding (for more information on the coding technique, go here ). You will thus quickly identify the main trends and recurring themes.
On the other hand, if you collect hundreds or even thousands of answers, the analysis of these free answers will be much more tedious. How can you do it? In this case, we advise you to use a semantic analysis tool. This is most often an online solution, specific to a language, which is based on an NLP (Natural Language Processing) algorithm. This algorithm will, very quickly, analyse your corpus and bring out the recurring themes . It is not a question here of calculating word frequencies, but instead of working on semantics to analyse the repetition of a subject.
Of course, the use of open-ended questions in interviews does not exclude the use of closed-ended questions. Alternating these two types of questions in interviews, whether 1-to-1 interviews, group conversations or focus groups, is conducive not only to maintaining a specific dynamic during the interview but also to be able to frame specific responses while leaving certain fields of expression free. In general, it is interesting for the different parties that the interview ends with an open-ended question where the interviewer asks the interviewee if he or she has anything to add or if he or she has any questions.
In this type of research, you confront the respondent with a new, innovative product or service. It is therefore important not to collect superficial opinions but to understand in depth the respondent’s attitude towards the subject of the market research.
As you will have understood, open-ended questions are particularly suitable for qualitative research (1-to-1 interviews and focus groups). How should they be formulated?
The Five W’s; (who did what, where, when, and why ) questioning method should be used rigorously and sparingly :
- Who? Who? What? Where? When? How? How much? “are particularly useful for qualitative research and allow you to let your interlocutor develop and elaborate a constructed and informative answer.
- Use the CIT (Critical Incident Technique) method with formulations that encourage your interviewer to go into the details of an experience: “Can you describe/tell me…? “, ” What did you feel? “, ” According to you… “
- Avoid asking “Why?”: this question may push the interviewer into a corner, and the interviewer may seek logical reasoning for his or her previous answer. Be gentle with your respondents by asking them to tell you more, to give you specific examples, for example.
In contrast, closed-ended questions are mainly used and adapted to quantitative questionnaires since they facilitate the analysis of the results by framing the participants’ answers.
Image: Shutterstock
- Market research methods
Pour offrir les meilleures expériences, nous utilisons des technologies telles que les cookies pour stocker et/ou accéder aux informations des appareils. Le fait de consentir à ces technologies nous permettra de traiter des données telles que le comportement de navigation ou les ID uniques sur ce site. Le fait de ne pas consentir ou de retirer son consentement peut avoir un effet négatif sur certaines caractéristiques et fonctions.
Open-Ended Questions – A Complete Guide

Open-ended questions are invaluable for gathering meaningful insights. Unlike closed-ended questions that limit responses, open-ended questions allow people to answer in their own words.
This gives them the freedom to provide more detailed and thoughtful responses, reveal attitudes and emotions, and share unexpected perspectives.
In this comprehensive guide, you will learn what open-ended questions are, why they are so effective for research, how to phrase open-ended questions, where to use open-ended questions, tips for success with open-ended questions, etc
Whether you are conducting formal research or having an informal discussion, open-ended questions can help you explore topics more deeply, foster engaging dialogue, and develop nuanced understandings of people’s experiences and beliefs.
Let’s get started.
Table of Contents
What are open-ended questions.
Open-ended questions are questions that require more than a simple yes/no or one-word response.
They are designed to encourage the respondent to provide an explanatory, descriptive answer using their own words. Unlike closed-ended questions that limit the response options, open-ended questions give people the flexibility to respond however they want.
Some examples of open-ended questions are:
- How did you feel when you experienced that?
- What factors influenced your decision to purchase this product?
- Could you describe your typical day?
These types of questions cannot be answered with a pre-determined set of responses. They push respondents to think deeper and share more details, opinions, and examples in their unique voice.
In contrast, closed-ended questions limit the answer to a binary yes/no, a numerical rating, or a choice among several fixed options. For example:
- Did you enjoy this product? (Yes/No)
- On a scale of 1-10, how would you rate this experience?
- What is your age range? (18-24, 25-34, 35-44 etc.)
While closed-ended questions can be useful in some cases, they do not gather the type of rich, descriptive data that open-ended questions produce.
Open-ended questions give respondents the freedom to fully express themselves and take the conversation in new, unexpected directions.
Benefits of open-ended questions
1. allow for detailed, expansive responses.
Open-ended questions allow respondents to provide much more detail and explanation in their answers. Unlike closed-ended questions that limit responses to a few words or a rating, open-ended questions give people the flexibility to fully express themselves.
- Respondents can provide important context around their experiences, thought processes, and motivations. This gives color, texture and background to their answers.
- They can offer detailed descriptions with vivid language, examples, and anecdotes that bring their responses to life. This creates a fuller picture.
- Explanations are encouraged, allowing them to articulate their reasoning, describe causes and effects, and make connections. This provides greater logic and insight.
2. Reveal Deeper Insights About Thoughts, Feelings, Opinions
By giving respondents freedom of expression, open-ended questions reveal deeper insights about their perspectives, mindsets, and emotions.
- They illuminate people’s internal motivations, fears, dreams, and beliefs that drive their behaviors and decisions. This provides a window into the psyche.
- They uncover complex reasoning and weighing of pros and cons that led to conclusions. This highlights nuanced thought processes.
- They give glimpses into emotional experiences and psychological influences beneath the surface. This builds empathy and understanding.
3. Promote Open Dialogue and Two-Way Communication
The flexible nature of open-ended questions allows for a smoother, more natural give-and-take conversation.
- They facilitate an easy back-and-forth flow as respondents expand on ideas and the questioner asks follow-up questions.
- Long pauses or awkward silences are prevented by the open-ended structure keeping the discussion moving.
- Unexpected insights can organically arise through this open dialogue, rather than sticking to a rigid script.
4. Help Build Rapport and Trust
By giving respondents freedom to share, open-ended questions demonstrate genuine interest in their point of view.
- This helps build rapport as respondents feel heard, respected and engaged.
- It establishes trust and willingness to be vulnerable, facilitating more honest, thoughtful responses.
- The questioner gains credibility by prioritizing the respondent’s complete perspective rather than fishing for certain answers.
5. Uncover More Information
Open-ended questions are ideal for gathering comprehensive information on topics where closed-ended questions would fall short.
- In research, the flexibility allows discovery of unexpected themes, sentiments and behaviors.
- In counseling, they permit clients to share anxieties and surface emotional needs on their own terms.
- In interviews, they help build a complete profile based on real narratives rather than superficial data points.
The expansive nature of responses to open-ended questions contains insights and intelligence that other question types cannot reveal. This makes them invaluable for in-depth qualitative research across fields.
How to Phrase Open-Ended Questions
1. use interrogative words.
Forming open-ended questions using interrogative words like who, what, when, where, why and how is an effective strategy to elicit detailed, explanatory responses.
These question words encourage people to provide more thoughtful answers beyond just yes/no or one-word replies. For example, asking “Why did you make that decision?” or “How did you feel when that happened?” pushes respondents to reflect more deeply instead of reacting instinctually.
They have to describe their motivations, thought processes, emotions and experiences in order to fully answer the question.
Phrasing open-ended questions without using interrogative words often enables respondents to get away with shorter, more closed responses. The interrogative wording forces them to delve deeper and share more.
2. Begin Questions With Phrases Like “Tell Me About…”
Inviting descriptive responses by beginning open-ended questions with phrases like “Tell me about…” or “Describe…” is another impactful technique.
This wording establishes clear expectations that an extensive, in-depth explanation is desired. Respondents recognize they have the freedom and permission to share details, context, examples, and backstories without worrying about providing the “right” simple answer.
The “Tell me about…” or “Describe…” preamble signals that the questioner is interested in hearing their full perspective, not just surface-level facts.
Starting open-ended questions in this way empowers respondents to open up comfortably without reservations about response length or format. It gets them primed to be thoughtful and reflective.
3. Keep Language Open and Non-Leading
Wording open-ended questions in an open, neutral way avoids biasing or leading respondents toward particular responses.
Closed-ended questions often have baked-in assumptions or apply pressure to answer in a socially desirable way. Open-ended questions should completely avoid this by using objective, non-judgmental language.
Don’t impose any preconceived notions or make respondents feel like there is a “correct” answer they should give. Let their experiences, thought processes, attitudes and beliefs emerge organically without being influenced.
Keeping questions open-ended both linguistically and psychologically empower respondents to share their authentic perspectives, even if unexpected or contrary to assumptions.
4. Use Probing Follow-Up Questions
Following up on initial open-ended questions with probing questions is an excellent tactic to gather more details and encourage elaboration.
For example, asking “Can you expand on that concept?” or “You mentioned [X] – what exactly do you mean by that?” demonstrates interest and pushes them to build on their original response with more depth, examples, context and clarity.
Phrasing follow-ups using words like “elaborate,” “explain” or “describe” challenges respondents to dive deeper into their thought processes and unpack their statements further.
Not accepting their original response at face value pressures them to provide richer descriptions and concrete evidence to back up their claims. This develops a fruitful dialogue rather than a one-off question.
When to Use Open-Ended Questions
1. during jtbd interviews.
Leveraging open-ended questions is incredibly effective throughout Jobs-to-Be-Done interviews to reveal the complete backstory and motivation surrounding customers’ purchases.
The non-restrictive format gives customers the latitude to comprehensively describe the circumstances, emotions, frustrations and needs leading up to acquiring a product or service.
Skilled interviewers utilize probing open-ended follow-ups to encourage vivid narratives and details about the full context around purchase occasions, rather than just superficial factors. Customers can elaborate extensively about their decision journey, thought processes, usage situations, pain points with previous options, requirements, and perceived risks.
This provides a holistic understanding of the “job” the product was “hired” to do. Letting customers explain freely without constraints uncovers unexpected insights about usage behaviors, delighters, substitutes, and more that closed-ended questions would not organically reveal.
2. For Qualitative Market Research
For qualitative market research, open-ended inquiry delivers profoundly detailed understandings of how consumers truly perceive brands, make purchasing decisions and experience products day-to-day.
The flexible format provides space for target consumers to explain in their own words their affiliations with brands, product/service usage occasions, decision motivations, pain points, moments of delight, desired outcomes and more.
Researchers can deeply explore responses using “why” and “how” probes to uncover the psychological, emotional, social and functional factors driving consumer behaviours.
This reveals strategic opportunities around positioning, messaging, feature development and customer experience design.
While quantitative data establishes surface-level consumer trends, open-ended engagement provides meaningful qualitative context and language to inform smart strategy and create deep consumer connections.
3. To Gather Customer Feedback
Companies use open-ended questions to gather candid qualitative customer feedback that pinpoints priorities for improvement.
Customers can explain frustrations, positive/negative experiences, emotional pain points and desires in their own words without being limited by pre-determined response options.
Probing follow-up questions explores feedback more deeply to identify root causes of pain points versus superficial irritants.
Customers also have space to provide suggestions to resolve issues and share moments that delight them. This constructive feedback is synthesized to guide enhancements across the customer journey, from marketing to product features to post-purchase experience and support.
Closed-ended satisfaction scales fail to provide the rich narratives and insights needed to address problems and identify what matters most to customers.
Common Mistakes to Avoid With Open-Ended Questions
1. asking more than one question at a time.
Asking multiple questions at once is an extremely common mistake when using open-ended questions that significantly hinders their effectiveness.
Overwhelming respondents with compound, complex or overlapping questions leaves them confused about which aspect to focus their response on. This results in vague, generalized answers that gloss over the nuances of each inquiry rather than providing the specific, deep insights each question warrants.
Even elaborate responses to multi-part questions often lack the laser focus and structure needed to extract key themes.
Additionally, blending different lines of inquiry into one big question makes it challenging to analyze and utilize the unstructured feedback. It’s far more effective to ask one open-ended question at a time, give the respondent space to answer thoroughly, and use strategic follow-ups to progressively build understanding.
This disciplined approach avoids cognitive overload and provides the detail required to drive meaningful dialogue and derive actionable conclusions.
2. Using Closed-Ended Phrasing
It’s vital to pay close attention to the exact wording used when phrasing open-ended questions. Even subtle vocabulary issues can inadvertently create closed-ended questions that limit responses to yes/no, agree/disagree or basic data.
Leading with verbs like “Do,” “Does,” “Is,” “Are,” or “Did” prompts closed responses rather than explanations. Asking “Why was that good?” or “How did you like it?” implies there was liking rather than allowing them to evaluate freely.
The language itself should remain open and non-leading to empower respondents to share whatever perspectives or experiences emerge naturally, without assumptions.
Carefully phrasing questions with neutral language like “Tell me about…” or “What factors influenced…” ensures responses contain unfiltered insights rather than confirmations of preconceived notions.
3. Not Allowing Enough Time for Responses
Rushing respondents to answer open-ended questions defeats their purpose of gathering in-depth insights and often results in abrupt, incomplete responses lacking meaningful substance.
After asking an open-ended question, it’s essential to proactively give respondents adequate silent time to gather thoughts, reflect on experiences, and articulate responses before interrupting.
If responses seem cursory, ask probing follow-up questions to draw out the rich details and explanations that open-ended inquiries are designed for.
Making respondents feel pressed for time can also discourage sharing personal anecdotes or discussing sensitive topics that require vulnerability. Allowing ample time upfront ultimately saves effort compared to trying to recover depth through multiple ineffective follow-up questions after initially rushing the pace. Patience pays off by enabling thorough responses and productive, unhurried dialogue.
4. Neglecting Active Listening and Follow-Up
Failing to actively listen and ask follow-up questions after posing open-ended inquiries squanders their potential for deep, revealing discussion.
Without planned follow-up, even thoughtful responses often remain surface-level and leave underlying perspectives unaddressed.
Strategic probing follow-ups based on active listening are essential to dive deeper into relevant themes, gather illuminating examples and stories, understand nuanced thinking, and uncover subtle emotions.
They show interest in the respondent’s vantage point rather than just checking a box.
Simply letting responses conclude without probing for more is a lost opportunity to build understanding and interpersonal connections. Follow-ups demonstrate curiosity, clarify ambiguities, and encourage vulnerability through elaboration in their own authentic voice.
Tips for Success with Open-Ended Questions
1. listen fully without interrupting.
Allowing respondents to answer open-ended questions without interruption demonstrates exemplary active listening skills and gives space for thoughtful, unfiltered responses.
Jumping in too soon with follow-ups or tangents cuts off the initial flow of insight and risks losing unexpected revelations still percolating.
Silence after asking an open-ended question can feel awkward, but resisting the temptation to immediately fill gaps leads to stronger dialogue and understanding in the long run.
Even if responses seem slow to develop, interrupting can fluster respondents and inhibit substantive sharing.
By listening patiently and without judgment from start to conclusion, you signal genuine interest in understanding their full perspective, making them more willing to open up candidly. This level of care and focus builds crucial trust and rapport that supports ongoing vulnerable sharing.
2. Ask Follow-Up Questions to Probe Deeper
Thoughtful, strategic open-ended follow-up questions are essential to probe initial responses more deeply for vivid examples, explanatory backstories and illuminating details that bring insight to life.
Questions like “What drove that decision?” or “How did that make you feel?” demonstrate curiosity to learn more rather than passively accepting surface-level responses.
Drawing out more textures, emotions, contexts and narratives helps co-construct meaning and perspective-taking.
Follow a logical path of inquiry without bombarding respondents with tangents. Look for gaps to fill or opportunities to clarify and expand based on active listening.
Continue probing with empathy and tact until satisfied with the depth and specificity of understanding. Mastering open-ended follow-up techniques leads to richer discoveries.
3. Remain Objective and Non-Judgmental
When facilitating responses to open-ended questions, it’s vital that tone, body language and verbal reactions remain completely neutral and non-judgmental, even if responses provoke internal surprise.
Any hint of subjectivity could shut down honesty, making respondents hesitant to share freely in the future.
Maintain engaged, affirmative eye contact and posture regardless of your personal feelings to foster a safe space. Never explicitly express disapproval, disagreement or shock.
The goal is unfiltered insight into the respondent’s perspectives, not conformity with your own. Leave your biases aside to have an authentic, open-minded exchange. Let responses speak for themselves without revealing your own hand through unnecessary commentary.
4. Adjust Based on Situational Context
Successful use of open-ended questions requires reading situational contexts and adjusting questioning and follow-up techniques accordingly.
In formal research interviews, maintain more structure and focus by sticking to clear lines of inquiry. In informal dialogue, conversations can flow more organically based on responses, following intriguing tangents.
Consider factors like relationship status, power dynamics, setting formality, time constraints, response styles and emotional energy when deciding how tightly or loosely to guide the discussion.
Get clarification if responses seem unclear. With sensitive topics, tread carefully and give space. Frame questions and probe with situational awareness to enhance positive outcomes.
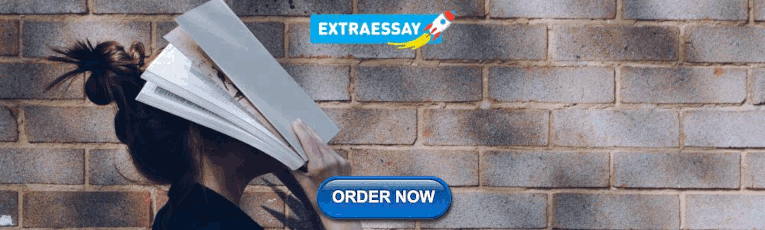
5. Balance Open and Closed-Ended Questions
Both open and closed-ended questions play important complementary roles in gathering complete, multi-faceted information.
Open-ended questions uncover deep qualitative insights through descriptive responses in the respondent’s own words. Closed-ended questions efficiently gather quantifiable data, opinions and facts.
Relying solely on open-ended questions can lead to aimless rambling, while overusing closed-ended questions results in thin data lacking context.
Develop mastery in blending, sequencing and transitioning smoothly between the two approaches. For example, use open-ended questions to explore themes and closed-ended questions to confirm conclusions. Balance them artfully based on the situation.
6. Use Proper Tone, Body Language, Eye Contact
Warm, conversational tone and friendly body language while asking open-ended questions and listening to responses help build crucial rapport and willingness to open up.
Make regular eye contact to show engagement, leaning in slightly to signal interest in learning the respondent’s perspective.
Avoid crossed arms or distracted glances at notes or devices which can seem closed-off. Reflect the respondent’s emotional tenor – if anxiety emerges around a topic, adopt a reassuring tone.
Your nonverbals should make respondents feel heard, respected and comfortable revealing their authentic self without fear of judgment. A caring presence breeds candidness.
7. Take Notes on Key Information
During open-ended questioning dialogues, take concise notes on main discussion themes, powerful respondent quotes, follow-up topics, body language and insights that resonate rather than attempting to transcribe responses.
Verbatim transcription is inefficient in capturing the core essence. Prioritize highlighting the main takeaways, defined terms, compelling stories and emotional moments that leave an impression.
Review, organize and reflect on notes soon after while memory remains fresh to consolidate learnings and plan the next steps.
Quality selective note-taking aids meaningful analysis of the wealth of unstructured qualitative information generated through open-ended engagement.
Frequently Asked Questions About Open-Ended Questions
1. Q: What is the difference between open-ended and closed-ended questions?
A: Open-ended questions elicit an explanatory response with detailed narrative, context and emotions. Closed-ended questions limit responses to a short phrase or numerical rating.
2. Q: When should I use open-ended questions versus closed-ended questions?
A: Use open-ended questions when seeking qualitative insights and detailed perspectives. Use closed-ended for quantifiable data or confirming hypotheses. Use both to balance breadth and depth.
3. Q: What phrases help encourage detailed responses to open-ended questions?
A: Phrases like “tell me more about “, “describe your experience”, and “explain your perspective on” encourage elaboration. Avoid yes/no phrasing.
4. Q: How can I avoid influencing the respondent’s answers to open-ended questions?
A: Use neutral language. Don’t lead towards expected or desired responses. Allow free expression without judgment or imposition of assumptions.
5. Q: How many open-ended questions should I ask at a time?
A: Ask one open-ended question at a time. Allow thorough response then ask focused follow-up questions to build understanding.
6. Q: How can I get respondents to open up more with open-ended questions?
A: Active listening, empathy, and non-judgment encourage openness. Probing gently with follow up questions signals interest in understanding their full perspective.
7. Q: What are some examples of good open-ended questions for research interviews?
A: “Tell me about your experience using this product”, “How did this make you feel?”, “What factors influenced your decision?”
8. Q: How can I tailor open-ended questions based on the situation and respondent?
A: Consider formality of setting, time constraints, rapport level, demographics, tone of conversation and emotions when crafting relevant, thoughtful questions.
9. Q: What listening skills are important for gathering the most from open-ended questions?
A: Focused, active listening without interruption. Probing follow-ups to draw out details. Objectivity. Empathy. Situational awareness.
10. Q: How can I remember to use open-ended questions more often?
A: Actively monitor your language for closed-ended phrasing. Pause after asking one question. Prepare follow-up questions in advance.
- A/B Testing
- Company News
- Conversion Rate Optimisation
- CRO Tools and Resources
- Experimentation Articles
- Google Analytics
- Personalization
- Usability Testing
- User Research
- The Ultimate Guide To Integrating Voice of the Customer into CRO
- Scaling User Research For Enterprise CRO
- Understanding the Core Concepts of CRO Personalization
- Dynamic Content Personalization: Tips and Best Practices
- The Impact of AB Testing on User Retention
- Open-Ended Questions - A Complete Guide
- Close-Ended Questions - A Complete Guide
- Explore vs Exploit: Finding the Balance in CRO
- Top 19 Best CRO Books (recommended by experts)
- The 7Ps Of Marketing
- Qualitative vs Quantitative Research: When to Use Each
- Introduction To MultiVariate Testing: A Complete Guide (2023)
- What Is A CRO Test? Definition, Types & Examples
- One-Tailed vs Two-Tailed Tests: What You Should Know

Is your CRO programme delivering the impact you hoped for ?
Benchmark your CRO now for immediate, free report packed with ACTIONABLE insights you and your team can implement today to increase conversion.
Takes only two minutes
If your CRO programme is not delivering the highest ROI of all of your marketing spend, then we should talk.
Before you go...

“The world’s leading companies are using experimentation to generate better results for the whole business.
Download our latest ebook to discover how businesses have made the shift from CRO to experimentation and how you can too.
Join the next one!
Be the first to know about future events with top speakers from the CRO industry.
An official website of the United States government
Official websites use .gov A .gov website belongs to an official government organization in the United States.
Secure .gov websites use HTTPS A lock ( Lock Locked padlock icon ) or https:// means you've safely connected to the .gov website. Share sensitive information only on official, secure websites.
- Publications
- Account settings
- Advanced Search
- Journal List

Open-ended interview questions and saturation
Susan c weller, ben vickers, h russell bernard, alyssa m blackburn, stephen borgatti, clarence c gravlee, jeffrey c johnson.
- Author information
- Article notes
- Copyright and License information
Competing Interests: The authors have declared that no competing interests exist.
‡ These authors also contributed equally to this work.
* E-mail: [email protected]
Contributed equally.
Received 2018 Feb 16; Accepted 2018 May 22; Collection date 2018.
This is an open access article distributed under the terms of the Creative Commons Attribution License , which permits unrestricted use, distribution, and reproduction in any medium, provided the original author and source are credited.
Sample size determination for open-ended questions or qualitative interviews relies primarily on custom and finding the point where little new information is obtained (thematic saturation). Here, we propose and test a refined definition of saturation as obtaining the most salient items in a set of qualitative interviews (where items can be material things or concepts, depending on the topic of study) rather than attempting to obtain all the items . Salient items have higher prevalence and are more culturally important. To do this, we explore saturation, salience, sample size, and domain size in 28 sets of interviews in which respondents were asked to list all the things they could think of in one of 18 topical domains. The domains—like kinds of fruits (highly bounded) and things that mothers do (unbounded)—varied greatly in size. The datasets comprise 20–99 interviews each (1,147 total interviews). When saturation was defined as the point where less than one new item per person would be expected, the median sample size for reaching saturation was 75 (range = 15–194). Thematic saturation was, as expected, related to domain size. It was also related to the amount of information contributed by each respondent but, unexpectedly, was reached more quickly when respondents contributed less information. In contrast, a greater amount of information per person increased the retrieval of salient items. Even small samples ( n = 10) produced 95% of the most salient ideas with exhaustive listing, but only 53% of those items were captured with limited responses per person (three). For most domains, item salience appeared to be a more useful concept for thinking about sample size adequacy than finding the point of thematic saturation. Thus, we advance the concept of saturation in salience and emphasize probing to increase the amount of information collected per respondent to increase sample efficiency.
Introduction
Open-ended questions are used alone or in combination with other interviewing techniques to explore topics in depth, to understand processes, and to identify potential causes of observed correlations. Open-ended questions may produce lists, short answers, or lengthy narratives, but in all cases, an enduring question is: How many interviews are needed to be sure that the range of salient items (in the case of lists) and themes (in the case of narratives) are covered. Guidelines for collecting lists, short answers, and narratives often recommend continuing interviews until saturation is reached. The concept of theoretical saturation —the point where the main ideas and variations relevant to the formulation of a theory have been identified—was first articulated by Glaser and Strauss [ 1 , 2 ] in the context of how to develop grounded theory. Most of the literature on analyzing qualitative data, however, deals with observable thematic saturation —the point during a series of interviews where few or no new ideas, themes, or codes appear [ 3 – 6 ].
Since the goal of research based on qualitative data is not necessarily to collect all or most ideas and themes but to collect the most important ideas and themes, salience may provide a better guide to sample size adequacy than saturation. Salience (often called cultural or cognitive salience) can be measured by the frequency of item occurrence (prevalence) or the order of mention [ 7 , 8 ]. These two indicators tend to be correlated [ 9 ]. In a set of lists of birds, for example, robins are reported more frequently and appear earlier in responses than are penguins. Salient terms are also more prevalent in everyday language [ 10 – 12 ]. Item salience also may be estimated by combining an item’s frequency across lists with its rank/position on individual lists [ 13 – 16 ].
In this article, we estimate the point of complete thematic saturation and the associated sample size and domain size for 28 sets of interviews in which respondents were asked to list all the things they could think of in one of 18 topical domains. The domains—like kinds of fruits (highly bounded) and things that mothers do (unbounded)—varied greatly in size. We also examine the impact of the amount of information produced per respondent on saturation and on the number of unique items obtained by comparing results generated by asking respondents to name all the relevant things they can with results obtained from a limited number of responses per question, as with standard open-ended questioning. Finally, we introduce an additional type of saturation based on the relative salience of items and themes— saturation in salience —and we explore whether the most salient items are captured at minimal sample sizes. A key conclusion is that saturation may be more meaningfully and more productively conceived of as the point where the most salient ideas have been obtained .
Recent research on saturation
Increasingly, researchers are applying systematic analysis and sampling theory to untangle the problems of saturation and sample size in the enormous variety of studies that rely on qualitative data—including life-histories, discourse analysis, ethnographic decision modeling, focus groups, grounded theory, and more. For example, Guest et al.[ 17 ] and others[ 18 – 19 ] found that about 12–16 interviews were adequate to achieve thematic saturation. Similarly, Hagaman and Wutich [ 20 ] found that they could reliably retrieve the three most salient themes from each of the four sites in the first 16 interviews.
Galvin[ 21 ] and Fugard and Potts[ 22 ] framed the sample size problem for qualitative data in terms of the likelihood that a specific idea or theme will or will not appear in a set of interviews, given the prevalence of those ideas in the population. They used traditional statistical theory to show that small samples retrieve only the most prevalent themes and that larger samples are more sensitive and can retrieve less prevalent themes as well. This framework can be applied to the expectation of observing or not observing almost anything. Here it would apply to the likelihood of observing a theme in a set of narrative responses, but it applies equally well for situations such as behavioral observations, where specific behaviors are being observed and sampled[ 23 ]. For example, to obtain ideas or themes that would be reported by about one out of five people (0.20 prevalence) or a behavior with the same prevalence, there is a 95% likelihood of seeing those themes or behaviors at least once in 14 interviews—if those themes or behaviors are independent.
Saturation and sample size have also begun to be examined with multivariate models and simulations. Tran et al. [ 24 ] estimated thematic saturation and the total number of themes from open-ended questions in a large survey and then simulated data to test predictions about sample size and saturation. They assumed that items were independent and found that sample sizes greater than 50 would add less than one new theme per additional person interviewed.
Similarly, Lowe et al. [ 25 ] estimated saturation and domain size in two examples and in simulated datasets, testing the effect of various parameters. Lowe et al. found that responses were not independent across respondents and that saturation may never be reached. In this context, non-independence refers to the fact that some responses are much more likely than others to be repeated across people. Instead of complete saturation, they suggested using a goal such as obtaining a percentage of the total domain that one would like to capture (e.g., 90%) and the average prevalence of items one would like to observe to estimate the appropriate sample size. For example, to obtain 90% of items with an average prevalence of 0.20, a sample size of 36 would be required. Van Rijnsoever [ 26 ] used simulated datasets to study the accumulation of themes across sample size increments and assessed the effect of different sampling strategies, item prevalence, and domain size on saturation. Van Rijnsoever’s results indicated that the point of saturation was dependent on the prevalence of the items.
As modeling estimates to date have been based on only one or two real-world examples, it is clear that more empirical examples are needed. Here, we use 28 real-world examples to estimate the impact of sample size, domain size, and amount of information per respondent on saturation and on the total number of items obtained. Using the proportion of people in a sample that mentioned an item as a measure of salience, we find that even small samples may adequately capture the most salient items.
Materials and methods
The datasets comprise 20–99 interviews each (1,147 total interviews). Each example elicits multiple responses from each individual in response to an open-ended question (“Name all the … you can think of”) or a question with probes (“What other … are there?”).
Data were obtained by contacting researchers who published analyses of free lists. Examples with 20 or more interviews were selected so that saturation could be examined incrementally through a range of sample sizes. Thirteen published examples were obtained on: illness terms [ 27 ] (in English and in Spanish); birds, flowers, and fabrics [ 28 ]; recreational/street drugs and fruits [ 29 ]; things mothers do (online, face-to-face, and written administration) and racial and ethnic groups [ 30 ] (online, face-to-face, and written administration). Fifteen unpublished classroom educational examples were obtained on: soda pops (Weller, n.d.); holidays (two replications), things that might appear in a living room, characteristics of a good leader (two replications), a good team (two replications), and a good team player (Johnson, n.d.); and bad words, industries (two replications), cultural industries (two replications), and scary things (Borgatti, n.d.). (Original data appear online in S1 Appendix The Original Data for the 28 Examples.)
Some interviews were face to face, some were written responses, and some were administered on-line. Investigators varied in their use of prompts, using nonspecific (What other … are there?), semantic (repeating prior responses and then asking for others), and/or alphabetic prompts (going through the alphabet and asking for others). Brewer [ 29 ] and Gravlee et al. [ 30 ] specifically examined the effect of prompting on response productivity, although the Brewer et al. examples in these analyses contain results before extensive prompting and the Gravlee et al. examples contain results after prompting. The 28 examples, their topic, source, sample size, the question used in the original data collection, and the three most frequently mentioned items appear in Table 1 . All data were collected and analyzed without personal identifying information.
Table 1. The examples.
For each example, statistical models describe the pattern of obtaining new or unique items with incremental increases in sample size. Individual lists were first analyzed with Flame [ 31 , 32 ] to provide the list of unique items for each example and the Smith [ 14 ] and Sutrop [ 15 ] item salience scores. Duplicate items due to spelling, case errors, spacing, or variations were combined.
To help develop an interviewing stopping rule, a simple model was used to predict the unique number of items contributed by each additional respondent. Generalized linear models (GLM, log-linear models for count data) were used to predict the unique number of items added by each respondent (incrementing sample size), because number of unique items added by each respondent (count data) is approximately Poisson distributed. For each example, models were fit with ordinary least squares linear regression, Poisson, and negative binomial probability distributions. Respondents were assumed to be in random order, in the order in which they occurred in each dataset, although in some cases they were in the order they were interviewed. Goodness-of-fit was compared across the three models with minimized deviants (the Akaike Information Criterion, AIC) to find the best-fitting model [ 33 ]. Using the best-fitting model for each example, the point of saturation was estimated as the point where the expected number of new items was one or less. Sample size and domain size were estimated at the point of saturation, and total domain size was estimated for an infinite sample size from the model for each example as the limit of a geometric series (assuming a negative slope).
Because the GLM models above used only incremental sample size to predict the total number of unique items (domain size) and ignored variation in the number of items provided by each person and variation in item salience, an additional analysis was used to estimate domain size while accounting for subject and item heterogeneity. For that analysis, domain size was estimated with a capture-recapture estimation technique used for estimating the size of hidden populations. Domain size was estimated from the total number of items on individual lists and the number of matching items between pairs of lists with a log-linear analysis. For example, population size can be estimated from the responses of two people as the product of their number of responses divided by the number of matching items (assumed to be due to chance). If Person#1 named 15 illness terms and Person#2 named 31 terms and they matched on five illnesses, there would be 41 unique illness terms and the estimated total number of illness terms based on these two people would be (15 x 31) /5 = 93.
A log-linear solution generalizes this logic from a 2 x 2 table to a 2 K table [ 34 ]. the capture–recapture solution estimates total population size for hidden populations using the pattern of recapture (matching) between pairs of samples (respondents) to estimate the population size. An implementation in R with GLM uses a log-linear form to estimate population size based on recapture rates (Rcapture [ 35 , 36 ]). In this application, it is assumed that the population does not change between interviews (closed population) and models are fit with: (1) no variation across people or items (M 0 ); (2) variation only across respondents (M t ); (3) variation only across items (M h ); and (4) variation due to an interaction between people and items (M ht ). For each model, estimates were fit with binomial, Chao’s lower bound estimate, Poisson, Darroch log normal, and gamma distributions [ 35 ]. Variation among items (heterogeneity) is a test for a difference in the probabilities of item occurrence and, in this case, is equivalent to a test for a difference in item salience among the items. Due to the large number of combinations needed to estimate these models, Rcapture software estimates are provided for all four models only up to a sample of size 10. For larger sample sizes (all examples in this study had sample sizes of 20 or larger), only model 1 with no effects for people or items (the binomial model) and model 3 with item effects (item salience differences) were tested. Therefore, models were fit at size 10, to test all four models and then at the total available sample size.
Descriptive information for the examples appears in Table 2 . The first four columns list the name of the example, the sample size in the original study, the mean list length (with the range of the list length across respondents), and the total number of unique items obtained. For the Holiday1 example, interviews requested names of holidays (“Write down all the holidays you can think of”), there were 24 respondents, the average number of holidays listed per person (list length) was 13 (ranging from five to 29), and 62 unique holidays were obtained.
Table 2. Estimated point of saturation and domain size.
nbi = Negative binomial-identity, p = Poisson-log ; c = Chao’s Lower bound; g = gamma
Predicting thematic saturation from sample size
The free-list counts showed a characteristic descending curve where an initial person listed new themes and each additional person repeated some themes already reported and added new items, but fewer and fewer new items were added with incremental increases in sample size. All examples were fit using the GLM log-link and identity-link with normal, Poisson, and negative binomial distributions. The negative binomial model resulted in a better fit than the Poisson (or identity-link models) for most full-listing examples, providing the best fit to the downward sloping curve with a long tail. Of the 28 examples, only three were not best fit by negative binomial log-link models: the best-fitting model for two examples was the Poisson log-link model (GoodTeam1 and GoodTeam2Player) and one was best fit by the negative binomial identity-link model (CultInd1).
Sample size was a significant predictor of the number of new items for 21 of the 28 examples. Seven examples did not result in a statistically significant fit (Illnesses-US, Holiday2, Industries1, Industries2, GoodTLeader, GoodTeam2Player, and GoodTeam3). The best-fitting model was used to predict the point of saturation and domain size for all 28 examples ( S2 Appendix GLM Statistical Model Results for the 28 Examples).
Using the best-fitting GLM models we estimated the predicted sample size for reaching saturation. Saturation was defined as the point where less than one new item would be expected for each additional person interviewed. Using the models to solve for the sample size (X) when only one item was obtained per person (Y = 1) and rounding up to the nearest integer, provided the point of saturation (Y≤1.0). Table 2 , column five, reports the sample size where saturation was reached (N SAT ). For Holiday1, one or fewer new items were obtained per person when X = 16.98. Rounding up to the next integer provides the saturation point (N SAT = 17). For the Fruit domain, saturation occurred at a sample size of 15.
Saturation was reached at sample sizes of 15–194, with a median sample size of 75. Only five examples (Holiday1, Fruits, Birds, Flowers, and Drugs) reached saturation within the original study sample size and most examples did not reach saturation even after four or five dozen interviews. A more liberal definition of saturation, defined as the point where less than two new items would be expected for each additional person (solving for Y≤2), resulted in a median sample size for reaching saturation of 50 (range 10–146).
Some domains were well bounded and were elicited with small sample sizes. Some were not. In fact, most of the distributions exhibited a very long tail—where many items were mentioned by only one or two people. Fig 1 shows the predicted curves for all examples for sample sizes of 1 to 50. Saturation is the point where the descending curve crosses Y = 1 (or Y = 2). Although the expected number of unique ideas or themes obtained for successive respondents tends to decrease as the sample size increases, this occurs rapidly in some domains and slowly or not at all in other domains. Fruits, Holiday1, and Illness-G are domains with the three bottom-most curves and the steepest descent, indicating that saturation was reached rapidly and with small sample sizes. The three top-most curves are the Moms-F2F, Industries1, and Industries2 domains, which reached saturation at very large sample sizes or essentially did not reach saturation.
Fig 1. The number of unique items provided with increasing sample size.
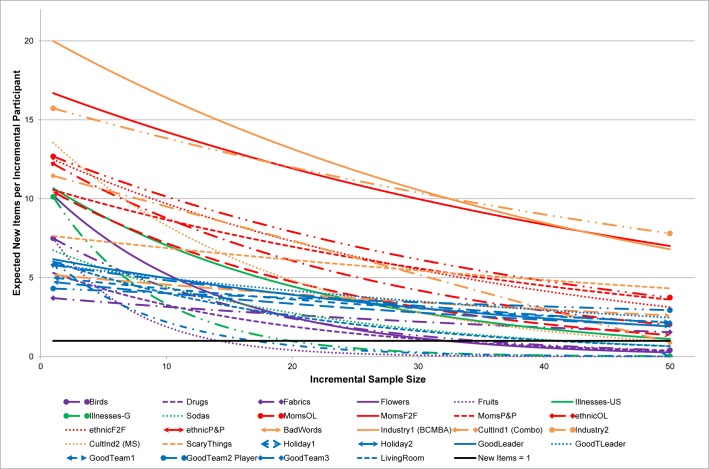
Estimating domain size
Because saturation appeared to be related to domain size and some investigators state that a percentage of the domain might be a better standard [ 25 ], domain size was also estimated. First, total domain size was estimated with the GLM models obtained above. Domain size was estimated at the point of saturation by cumulatively summing the number of items obtained for sample sizes n = 1, n = 2, n = 3, … to N SAT . For the Holiday1 sample, summing the number of predicted unique items for sample sizes n = 1 to n = 17 should yield 51 items ( Table 2 , Domain Size at Saturation, D SAT ). Thus, the model predicted that approximately 51 holidays would be obtained by the time saturation was reached.
The total domain size was estimated using a geometric series, summing the estimated number of unique items obtained cumulatively across people in an infinitely large sample. For the Holiday1 domain, the total domain size was estimated as 56 (see Table 2 , Total Domain Size D TOT ). So for the Holiday1 domain, although the total domain size was estimated to be 57, the model predicted that saturation occurred when the sample size reached 17, and at that point 51 holidays should be retrieved. Model predictions were close to the empirical data, as 62 holidays were obtained with a sample of 24.
Larger sample sizes were needed to reach saturation in larger domains; the largest domains were MomsF2F, Industries1, and Industries2 each estimated to have about 1,000 items and more than 100 interviews needed to approach saturation. Saturation (Y≤1) tended to occur at about 90% of the total domain size. For Fruits, the domain size at saturation was 51 and the total domain size was estimated at 53 (51/53 = 96%) and for MomsF2F, domain size at saturation was 904 and total domain size was 951 (95%).
Second, total domain size was estimated using a capture-recapture log-linear model with a parameter for item heterogeneity [ 35 , 36 ]. A descending, concave curve is diagnostic of item heterogeneity and was present in almost all of the examples. The estimated population sizes using R-Capture appear in the last column of Table 2 . When the gamma distribution provided the best fit to the response data, the domain size increased by an order of magnitude as did the standard error on that estimate. When responses fit a gamma distribution, the domain may be extremely large and may not readily reach saturation.
Inclusion of the pattern of matching items across people with a parameter for item heterogeneity (overlap in items between people due to salience) resulted in larger population size estimates than those above without heterogeneity. Estimation from the first two respondents was not helpful and provided estimates much lower than those from any of the other methods. The simple model without subject or item effects (the binomial model) did not fit any of the examples. Estimation from the first 10 respondents in each example suggested that more variation was due to item heterogeneity than to item and subject heterogeneity, so we report only the estimated domain size with the complete samples accounting for item heterogeneity in salience.
Overall, the capture–recapture estimates incorporating the effect of salience were larger than the GLM results above without a parameter for salience. For Fruits, the total domain size was estimated as 45 from the first two people; as 88 (gamma distribution estimate) from the first 10 people with item heterogeneity and as 67 (Chao lower bound estimate) with item and subject heterogeneity; and using the total sample ( n = 33) the binomial model (without any heterogeneity parameters) estimated the domain size as 62 (but did not fit the data) and with item heterogeneity the domain size was estimated as 73 (the best-fitting model used the Chao lower bound estimate). Thus, the total domain size for Fruits estimated with a simple GLM model was 53 and with a capture–recapture model (including item heterogeneity) was 73 ( Table 2 , last column). Similarly, the domain size for Holiday1 was estimated at 57 with the simple GLM model and 100 with capture-recapture model. Domain size estimates suggest that even the simplest domains can be large and that inclusion of item heterogeneity increases domain size estimates.
Saturation and the number of responses per person
The original examples used an exhaustive listing of responses to obtain about a half dozen (GoodLeader and GoodTeam2Player) to almost three dozen responses per person (Industries1 and Industries2). A question is whether saturation and the number of unique ideas obtained might be affected by the number of responses per person. Since open-ended questions may obtain only a few responses, we limited the responses to a maximum of three per person, truncating lists to see the effect on the number of items obtained at different sample sizes and the point of saturation.
When more information (a greater number of responses) was collected per person, more unique items were obtained even at smaller sample sizes ( Table 3 ). The amount of information retrieved per sample can be conceived of in terms of bits of information per sample and is roughly the average number of responses per person times the sample size so that, with all other things being equal, larger sample sizes with less probing should approach the same amount of information obtained with smaller samples and more probing. So, for a given sample size, a study with six responses per person should obtain twice as much information as a study with three responses per person. In the GoodLeader, GoodTeam1, and GoodTeam2Player examples, the average list length was approximately six and when the sample size was 10 (6 x 10 = 60 bits of information), approximately twice as many items were obtained as when lists were truncated to three responses (3 x 10 = 30 bits of information).
Table 3. Comparison of number of unique items obtained with full free lists and with three or fewer responses.
Increasing the sample size proportionately increases the amount of information, but not always. For Scary Things, 5.6 bits more information were collected per person with full listing (16.9 average list length) than with three or fewer responses per person (3.0 list length); and the number of items obtained in a sample size of 10 with full listing (102) was roughly 5.6 times greater than that obtained with three responses per person (18 items). However, at a sample size of 20 the number of unique items with free lists was only 4.5 times larger (153) than the number obtained with three responses per person (34). Across examples , interviews that obtained more information per person were more productive and obtained more unique items overall even with smaller sample sizes than did interviews with only three responses per person .
Using the same definition of saturation (the point where less than one new item would be expected for each additional person interviewed), less information per person resulted in reaching saturation at much smaller sample sizes. Fig 2 shows the predicted curves for all examples when the number of responses per person is three (or fewer). The Holiday examples reached saturation (fewer than one new item per person) with a sample size of 17 (Holiday1) with 13.0 average responses per person and 87 (Holiday2) with 17.8 average responses ( Table 2 ), but reached saturation with a sample size of only 9 (Holiday 1 and Holiday2) when there were a maximum of three responses per person ( Table 3 , last column). With three or fewer responses per person, the median sample size for reaching saturation was 16 (range: 4–134). Thus, fewer responses per person resulted in reaching saturation at smaller sample sizes and resulted in fewer domain items.
Fig 2. The number of unique items provided with increasing sample size when there are three or fewer responses per person.
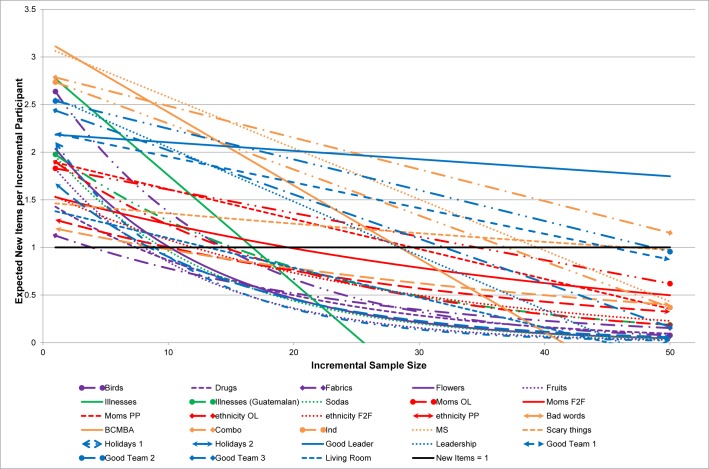
Salience and sample size
Saturation did not seem to be a useful guide for determining a sample size stopping point, because it was sensitive both to domain size and the number of responses per person. Since a main goal of open-ended interviews is to obtain the most important ideas and themes, it seemed reasonable to consider item salience as an alternative guide to assist with determining sample size adequacy. Here, the question would be: Whether or not complete saturation is achieved, are the most salient ideas and themes captured in small samples?
A simple and direct measure of item salience is the proportion of people in a sample that mentioned an item [ 37 ]. However, we examined the correlation between the sample proportions and two salience indices that combine the proportion of people mentioning an item with the item’s list position [ 13 – 15 ]. Because the item frequency distributions have long tails—there are many items mentioned by only one or two people—we focused on only those items mentioned by two or more people (24–204 items) and used the full lists provided by each respondent. The average Spearman correlation between the Smith and Sutrop indices in the 28 examples was 0.95 (average Pearson correlation 0.96, 95%CI: 0.92, 0.98), between the Smith index and the sample proportions was 0.89 (average Pearson 0.96, 95%CI: 0.915, 0.982), and between the Sutrop index and the sample proportions was 0.86 (average Pearson 0.88 95%CI: 0.753, 0.943). Thus, the three measures were highly correlated in 28 examples that varied in content, number of items, and sample size—validating the measurement of a single construct.
To test whether the most salient ideas and themes were captured in smaller samples or with limited probing, we used the sample proportions to estimate item salience and compared the set of most salient items across sample sizes and across more and less probing. Specifically, we defined a set of salient items for each example as those mentioned by 20% or more in the sample of size 20 (because all examples had at least 20) with full-listing (because domains were more detailed). We compared the set of salient items with the set of items obtained at smaller sample sizes and with fewer responses per person.
The set size for salient items (prevalence ≥ 20%) was not related to overall domain size, but was an independent characteristic of each domain and whether there were core or prototypical items with higher salience. Most domains had about two dozen items mentioned by 20% or more of the original listing sample ( n = 20), but some domains had only a half dozen or fewer items (GoodLeader, GoodTeam2Player, GoodTeam3). With full listing, 26 of 28 examples captured more than 95% of the salient ideas in the first 10 interviews: 18 examples captured 100%, eight examples captured 95–99%, one example captured 91%, and one captured 80% ( Table 4 ). With a maximum of three responses per person, about two-thirds of the salient items (68%) were captured with 20 interviews and about half of the items (53%) were captured in the first 10 interviews. With a sample size of 20, a greater number of responses per person resulted in approximately 50% more items than with three responses per person. Extensive probing resulted in a greater capture of salient items even with smaller sample sizes.
Table 4. Capture of salient items with full free list and with three or fewer responses.
Summary and discussion.
The strict notion of complete saturation as the point where few or no new ideas are observed is not a useful concept to guide sample size decisions, because it is sensitive to domain size and the amount of information contributed by each respondent. Larger sample sizes are necessary to reach saturation for large domains and it is difficult to know, when starting a study, just how large the domain or set of ideas will be. Also, when respondents only provide a few responses or codes per person, saturation may be reached quickly. So, if complete thematic saturation is observed, it is difficult to know whether the domain is small or whether the interviewer did only minimal probing.
Rather than attempting to reach complete saturation with an incremental sampling plan, a more productive focus might be on gaining more depth with probing and seeking the most salient ideas. Rarely do we need all the ideas and themes, rather we tend to be looking for important or salient ideas. A greater number of responses per person resulted in the capture of a greater number of salient items. With exhaustive listing, the first 10 interviews obtained 95% of the salient ideas (defined here as item prevalence of 0.20 or more), while only 53% of those ideas were obtained in 10 interviews with three or fewer responses per person.
We used a simple statistical model to predict the number of new items added by each additional person and found that complete saturation was not a helpful concept for free-lists, as the median sample size was 75 to get fewer than one new idea per person. It is important to note that we assumed that interviews were in a random order or were in the order that the interviews were conducted and were not reordered to any kind of optimum. The reordering of respondents to maximally fit a saturation curve may make it appear that saturation has been reached at a smaller sample size [ 31 ].
Most of the examples examined in this study needed sample sizes larger than most qualitative researchers use to reach saturation. Mason’s [ 6 ] review of 298 PhD dissertations in the United Kingdom, all based on qualitative data, found a mean sample size of 27 (range 1–95). Here, few of the examples reached saturation with less than four dozen interviews. Even with large sample sizes, some domains may continue to add new items. For very large domains, an incremental sampling strategy may lead to dozens and dozens of interviews and still not reach complete saturation. The problem is that most domains have very long tails in the distribution of observed items, with many items mentioned by only one or two people. A more liberal definition of complete saturation (allowing up to two new items per person) allowed for saturation to occur at smaller sample sizes, but saturation still did not occur until a median sample size of 50.
In the examples we studied, most domains were large and domain size affected when saturation occurred. Unfortunately, there did not seem to be a good or simple way at the outset to tell if a domain would be large or small. Most domains were much larger than expected, even on simple topics. Domain size varied by substantive content, sample, and degree of heterogeneity in salience. Domain size and saturation were sample dependent, as the holiday examples showed. Also, domain size estimates did not mean that there are only 73 fruits, rather the pattern of naming fruits—for this particular sample—indicated a set size of 73.
It was impossible to know, when starting, if a topic or domain was small and would require 15 interviews to reach saturation or if the domain was large and would require more than 100 interviews to reach saturation. Although eight of the examples had sample sizes of 50–99, sample sizes in qualitative studies are rarely that large. Estimates of domain size were even larger when models incorporated item heterogeneity (salience). The Fruit example had an estimated domain size of 53 without item heterogeneity, but 73 with item heterogeneity. The estimated size of the Fabric domain increased from 210 to 753 when item heterogeneity was included.
The number of responses per person affected both saturation and the number of obtained items. A greater number of responses per person resulted in a greater yield of domain items. The bits of information obtained in a sample can be approximated by the product of the average number of responses per person (list length) and the number of people in a sample. However, doubling the sample size did not necessarily double the unique items obtained because of item salience and sampling variability. When only a few items are obtained from each person, only the most salient items tend to be provided by each person and fewer items are obtained overall.
Brewer [ 29 ] explored the effect of probing or prompting on interview yield. Brewer examined the use of a few simple prompts: simply asking for more responses, providing alphabetical cues, or repeating the last response(s) and asking again for more information. Semantic cueing, repeating prior responses and asking for more information, increased the yield by approximately 50%. The results here indicated a similar pattern. When more information was elicited per person , about 50% more domain items were retrieved than when people provided a maximum of three responses.
Interviewing to obtain multiple responses also affects saturation. With few responses per person, complete saturation was reached rapidly. Without extensive interview probing, investigators may reach saturation quickly and assume they have a sample sufficient to retrieve most of the domain items. Unfortunately, different degrees of salience among items may cause strong effects for respondents to repeat similar ideas—the most salient ideas—without elaborating on less salient or less prevalent ideas, resulting in a set of only the ideas with the very highest salience. If an investigator wishes to obtain most of the ideas that are relevant in a domain , a small sample with extensive probing (listing) will prove much more productive than a large sample with casual or no probing .
Recently, Galvin [ 21 ] and Fugard and Potts [ 22 ] framed sample size estimation for qualitative interviewing in terms of binomial probabilities. However, results for the 28 examples with multiple responses per person suggest that this may not be appropriate because of the interdependencies among items due to salience. The capture–recapture analysis indicated that none of the 28 examples fit the binomial distribution. Framing the sample size problem in terms that a specific idea or theme will or will not appear in a set of interviews may facilitate thinking about sample size, but such estimates may be misleading.
If a binomial distribution is assumed, sample size can be estimated from the prevalence of an idea in the population, from how confident you want to be in obtaining these ideas, and from how many times you would like these ideas to minimally appear across participants in your interviews. A binomial estimate assumes independence (no difference in salience across items) and predicts that if an idea or theme actually occurs in 20% of the population, there is a 90% or higher likelihood of obtaining those themes at least once in 11 interviews and a 95% likelihood in 14 interviews. In contrast, our results indicated that the heterogeneity in salience across items causes these estimates to underestimate the necessary sample size as items with ≥20% prevalence were captured in 10 interviews in only 64% of the samples with full listing and in only 4% (one) of samples with three or fewer responses.
Lowe et al. [ 25 ] also found that items were not independent and that binomial estimates significantly underestimated sample size. They proposed sample size estimation from the desired proportion of items at a given average prevalence. Their formula predicts that 36 interviews would be necessary to capture 90% of items with an average prevalence of 0.20, regardless of degree of heterogeneity in salience, domain size, or amount of information provided per respondent. Although they included a parameter for non-independence, their model does not seem to be accurate for cases with limited responses or for large domains.
Conclusions
In general , probing and prompting during an interview seems to matter more than the number of interviews . Thematic saturation may be an illusion and may result from a failure to use in-depth probing during the interview. A small sample ( n = 10) can collect some of the most salient ideas, but a small sample with extensive probing can collect most of the salient ideas. A larger sample ( n = 20) is more sensitive and can collect more prevalent and more salient ideas, as well as less prevalent ideas, especially with probing. Some domains, however, may not have items with high prevalence. Several of the domains examined had only a half dozen or fewer items with prevalence of 20% or more. The direct link between salience and population prevalence offers a rationale for sample size and facilitates study planning. If the goal is to get a few widely held ideas, a small sample size will suffice. If the goal is to explore a larger range of ideas, a larger sample size or extensive probing is needed. Sample sizes of one to two dozen interviews should be sufficient with exhaustive probing (listing interviews), especially in a coherent domain. Empirically observed stabilization of item salience may indicate an adequate sample size.
A next step would be to test whether these conclusions and recommendations hold for other types of open-ended questions, such as narratives, life histories, and open-ended questions in large surveys. Open-ended survey questions are inefficient and result in thin or sparse data with few responses per person because of a lack of prompting. Tran et al. [ 24 ] reported item prevalence of 0.025 in answers in a large Internet survey suggesting few responses per person. In contrast, we used an item prevalence of 0.20 and higher to identify the most salient items in each domain and the highest prevalence in each domain ranged from 0.30 to 0.80 ( Table 1 ). Inefficiency in open-ended survey questions is likely due to the dual purpose of the questions: They try to define the range of possible answers and get the respondent’s answer. A better approach might be to precede survey development with a dozen free-listing interviews to get the range of possible responses and then use that content to design structured survey questions.
Another avenue for investigation is how our findings on thematic saturation compare to theoretical saturation in grounded theory studies [ 2 , 38 , 39 ]. Grounded theory studies rely on theoretical sampling–-an iterative procedure in which a single interview is coded for themes; the next respondent is selected to discover new themes and relationships between themes; and so on, until no more relevant themes or inter-relationships are discovered and a theory is built to explain the facts/themes of the case under study. In contrast this study examined thematic saturation, the simple accumulation of ideas and themes, and found that saturation in salience was more attainable–-perhaps more important—than thematic saturation.
Supporting information
Acknowledgments.
We would like to thank Devon Brewer and Kristofer Jennings for providing feedback on an earlier version of this manuscript. We would also like to thank Devon Brewer for providing data from his studies on free-lists.
Data Availability
All relevant data are available as an Excel file in the Supporting Information files.
Funding Statement
This project was partially supported by the Agency for Healthcare Research and Quality (R24HS022134). Funding for the original data sets was from the National Science Foundation (#BCS-0244104) for Gravlee et al. (2013), from the National Institute on Drug Abuse (R29DA10640) for Brewer et al. (2002), and from the Air Force Office of Scientific Research for Brewer (1995). Content is solely the responsibility of the authors and does not necessarily represent the official views of the funding agencies.
- 1. Glaser BG. The constant comparative method of qualitative analysis. Soc Probl. 1965; 12: 436−445. [ Google Scholar ]
- 2. Glaser BG, Strauss AL. The discovery of grounded theory: Strategies for qualitative research. New Brunswick, NJ: Aldine, 1967. [ Google Scholar ]
- 3. Lincoln YS, Guba EG. Naturalistic inquiry. Beverly Hills, CA: Sage, 1985. [ Google Scholar ]
- 4. Morse JM. Strategies for sampling In: Morse JM, editor, Qualitative Nursing Research: A Contemporary Dialogue. Rockville, MD: Aspen Press, 1989, pp. 117–131. [ Google Scholar ]
- 5. Sandelowski M. Sample size in qualitative research. Res Nurs Health. 1995; 18:179−183. [ DOI ] [ PubMed ] [ Google Scholar ]
- 6. Mason M. Sample size and saturation in PhD studies using qualitative interviews. Forum: Qualitative Social Research 2010; 11. http://nbn-resolving.de/urn:nbn:de:0114-fqs100387 (accessed December 26, 2017).
- 7. Thompson EC, Juan Z. Comparative cultural salience: measures using free-list data. Field Methods. 2006; 18: 398–412. [ Google Scholar ]
- 8. Romney A, D'Andrade R. Cognitive aspects of English kin terms. Am Anthro. 1964; 66: 146–170. [ Google Scholar ]
- 9. Bousfield WA, Barclay WD. The relationship between order and frequency of occurrence of restricted associative responses. J Exp Psych. 1950; 40: 643–647. [ DOI ] [ PubMed ] [ Google Scholar ]
- 10. Geeraerts D. Theories of lexical semantics. Oxford University Press, 2010. [ Google Scholar ]
- 11. Hajibayova L. Basic-Level Categories a Review. J of Info Sci. 2013; 1–12. [ Google Scholar ]
- 12. Berlin Brent. Ethnobiological classification In: Rosch E, Lloyd BB, eds. Cognition and Categorization. Hillsdale, NJ: Erlbaum; 1978, pp 9–26. [ Google Scholar ]
- 13. Smith JJ, Furbee L, Maynard K, Quick S, Ross L. Salience counts: A domain analysis of English color terms. J Linguistic Anthro. 1995; 5(2): 203–216. [ Google Scholar ]
- 14. Smith JJ, Borgatti SP. Salience counts-and so does accuracy: Correcting and updating a measure for free-list-item salience. J Linguistic Anthro. 1997; 7: 208–209. [ Google Scholar ]
- 15. Sutrop U. List task and a cognitive salience index. Field Methods. 2001;13(3): 263–276. [ Google Scholar ]
- 16. Robbins MC, Nolan JM, Chen D. An improved measure of cognitive salience in free listing tasks: a Marshallese example. Field Methods. 2017;29:395−9:395. [ Google Scholar ]
- 17. Guest G, Bunce A, Johnson L. How many interviews are enough? An experiment with data saturation and variability. Field Methods. 2006; 18: 59–82. [ Google Scholar ]
- 18. Coenen M, Stamm TA, Stucki G, Cieza A. Individual interviews and focus groups in patients with rheumatoid arthritis: A comparison of two qualitative methods. Quality of Life Research. 2012; 21:359–70. doi: 10.1007/s11136-011-9943-2 [ DOI ] [ PubMed ] [ Google Scholar ]
- 19. Francis JJ, Johnston M, Robertson C, Glidewell L, Entwistle V, Eccles MP, et al. What is an adequate sample size? Operationalising data saturation for theory-based interview studies. Psychol Health. 2010; 25:1229–45. doi: 10.1080/08870440903194015 [ DOI ] [ PubMed ] [ Google Scholar ]
- 20. Hagaman A K, Wutich A. How many interviews are enough to identify metathemes in multisited and cross-cultural research? Another perspective on Guest, Bunce, and Johnson’s (2006) landmark study. Field Methods. 2017; 29:23−41. [ Google Scholar ]
- 21. Galvin R. How many interviews are enough? Do qualitative interviews in building energy consumption research produce reliable knowledge? J of Building Engineering, 2015; 1: 2–12. [ Google Scholar ]
- 22. Fugard AJ, Potts HW. Supporting thinking on sample sizes for thematic analyses: a quantitative tool. Int J Soc Res Methodol. 2015; 18: 669–684. [ Google Scholar ]
- 23. Bernard HR, Killworth PD. Sampling in time allocation research. Ethnology. 1993; 32:207–15. [ Google Scholar ]
- 24. Tran VT, Porcher R, Tran VC, Ravaud P. Predicting data saturation in qualitative surveys with mathematical models from ecological research. J Clin Epi. 2017; February;82:71–78.e2. doi: 10.1016/j.jclinepi.2016.10.001 Epub 2016 Oct 24. [ DOI ] [ PubMed ] [ Google Scholar ]
- 25. Lowe A, Norris AC, Farris AJ, Babbage DR. Quantifying thematic saturation in qualitative data analysis. Field Methods. 2018; 30 (in press, online first: http://journals.sagepub.com/doi/full/10.1177/1525822X17749386 ). [ Google Scholar ]
- 26. Van Rijnsoever FJ. (I Can’t Get No) Saturation: A simulation and guidelines for sample sizes in qualitative research. PLoS ONE. 2017; 12: e0181689 doi: 10.1371/journal.pone.0181689 [ DOI ] [ PMC free article ] [ PubMed ] [ Google Scholar ]
- 27. Weller S. New data on intracultural variability: the hot-cold concept of medicine and illness. Hum Organ. 1983; 42: 249–257. [ Google Scholar ]
- 28. Brewer DD. Cognitive indicators of knowledge in semantic domains. J of Quant Anthro. 1995; 5: 107–128. [ Google Scholar ]
- 29. Brewer DD. Supplementary interviewing techniques to maximize output in free listing tasks. Field Methods. 2002; 14: 108–118. [ Google Scholar ]
- 30. Gravlee CC, Bernard HR, Maxwell CR, Jacobsohn A. Mode effects in free-list elicitation: comparing oral, written, and web-based data collection. Soc Sci Comput Rev. 2013; 31: 119–132. [ Google Scholar ]
- 31. Pennec F, Wencelius J, Garine E, Bohbot H. Flame v1.2—Free-list analysis under Microsoft Excel (Software and English User Guide), 2014. Available from: https://www.researchgate.net/publication/261704624_Flame_v12_-_Free-List_Analysis_Under_Microsoft_Excel_Software_and_English_User_Guide (10/19/17) [ Google Scholar ]
- 32. Borgatti SP. Software review: FLAME (Version 1.1). Field Methods. 2015; 27:199–205. [ Google Scholar ]
- 33. SAS Institute Inc. GENMOD SAS/STAT® 13.1 User’s Guide. Cary, NC: SAS Institute Inc., 2013. [ Google Scholar ]
- 34. Bishop Y, Feinberg S, Holland P. Discrete multivariate statistics: Theory and practice, MIT Press, Cambridge, 1975. [ Google Scholar ]
- 35. Baillargeon S, Rivest LP. Rcapture: loglinear models for capture-recapture in R. J Statistical Software. 2007; 19: 1–31. [ Google Scholar ]
- 36. Rivest LP, Baillargeon S: Package ‘Rcapture’ Loglinear models for capture-recapture experiments, in CRAN, R, Documentation Feb 19, 2015.
- 37. Weller SC, Romney AK. Systematic data collection (Vol. 10). Sage, 1988. [ Google Scholar ]
- 38. Morse M. Theoretical saturation In Lewis-Beck MS, Bryman A, Liao TF, editors. The Sage encyclopedia of social science research methods. Thousand Oaks, CA: Sage, 2004, p1123 Available from http://sk.sagepub.com/reference/download/socialscience/n1011.pdf [ Google Scholar ]
- 39. Tay, I. To what extent should data saturation be used as a quality criterion in qualitative research? Linked in 2014. Available from https://www.linkedin.com/pulse/20140824092647-82509310-to-what-extent-should-data-saturation-be-used-as-a-quality-criterion-in-qualitative-research
Associated Data
This section collects any data citations, data availability statements, or supplementary materials included in this article.
Supplementary Materials
Data availability statement.
- View on publisher site
- PDF (1.9 MB)
- Collections
Similar articles
Cited by other articles, links to ncbi databases.
- Download .nbib .nbib
- Format: AMA APA MLA NLM
Add to Collections
No internet connection.
All search filters on the page have been cleared., your search has been saved..
- Sign in to my profile My Profile
Reader's guide
Entries a-z, subject index.
- Survey: Open-Ended Questions
- By: Dalal Albudaiwi
- In: The SAGE Encyclopedia of Communication Research Methods
- Chapter DOI: https:// doi. org/10.4135/9781483381411.n608
- Subject: Communication and Media Studies , Sociology
- Show page numbers Hide page numbers
Open-ended questions are questions that do not provide participants with a predetermined set of answer choices, instead allowing the participants to provide responses in their own words. Open-ended questions are often used in qualitative research methods and exploratory studies. Qualitative studies that utilize open-ended questions allow researchers to take a holistic and comprehensive look at the issues being studied because open-ended responses permit respondents to provide more options and opinions, giving the data more diversity than would be possible with a closed-question or forced-choice survey measure. This entry expands on the many benefits of open-ended survey questions before examining the steps to writing well-constructed open-ended questions.
Advantages of Open-Ended Questions
A survey asking for a closed-ended response (e.g., “agree” or “disagree”) may use language not entirely appropriate or understood by the participants and may force the participants to select an answer that is not a completely accurate representation of their thoughts on the subject. By using open-ended questions, participants are able to express and articulate opinions that may be extreme, unusual, or simply ones that the researcher did not think about when creating the survey. This often provides researchers rich, relevant data for their studies.
Open-ended questions also help participants to freely share their personal experiences, especially if the topic is sensitive or concerns personal matters. For example, participants can be more expressive when answering questions about subjects like sexual harassment and religion affiliation. When asked about personal issues via open-ended questions, participants will often share their unique, personal experiences. Having a variety of personal stories by participants allows researchers to identify certain words and expressions that will prove useful to them when analyzing their data because it will enable them to explore the topic in-depth and through different angles. Researchers can also support the results of their study by quoting participants’ responses.
Respondents will typically provide ideas that widen the researcher’s understanding of the topic of the study. This can lead to new aspects of the topic that can be investigated in future studies. For [Page 1716] topics in which opinions and knowledge are not well-established, open-ended responses permit the respondent to express some levels of uncertainty. The researcher can then probe the participant further about his or her response in order to more fully understand what the participant knows or feels about the topic.
Open-ended questions also encourage and permit an emotional response on the part of the respondent. A decline of a response or a qualification of a response can indicate a hesitancy or reluctance to discuss an issue. The resistance to discuss is something that can be explored or may become evident in the nature of the response. Unlike a limited response question, the open-ended survey can explore or incorporate the ability to permit an expression of these limitations or concerns.
Perhaps the greatest benefit of an open-ended survey question is that it provides each participant with a sense of individuality. A response on a Likert scale creates a common metric for responses but lacks the individuality of an open-ended response that encourages articulation, creativity, and uniqueness of expression about an issue. The more personalized or individualized the nature of the circumstances under investigation, the greater the ability to capture and express that opinion when using open-ended responses. While the nature of the direct comparison may prove difficult because of the variability of responses, that variation may provide more naturalness to the understanding of the issues. Such an understanding may prove vital in generating a more complete understanding and representation of the underlying issues and considerations of the sample.
Constructing Open-Ended Questions
Having explained the importance of open-ended questions, it is equally important that researchers form these questions accurately and in a way that makes them easily understood by the participants, being careful to avoid using jargon or words with multiple meanings or interpretations. Asking vague or ambiguous questions may confuse or mislead participants, resulting in irrelevant data that will complicate the data analysis process for researchers.
On the other hand, sometimes researchers intentionally design questions to be more ambiguous to examine certain variables or issues. Depending on the purpose of the study, forming ambiguous or indirect questions may help the researcher to obtain other relevant data. An open-ended response permits the participant to generate or supply the definition of the context or the stimulus. When a topic or wording becomes polarized, the use of an open-ended response permits respondents to generate a response that reflects a bias or a predisposed attitude. The open-ended response permits the respondent to express a position or define the context in the manner the respondent chooses.
It is important to note that not all surveys require open-ended questions to collect valid and useful data. For example, if a researcher wants to know which presidential candidate the sample supports, an open-ended response may not prove necessary. A question that supplies a list of limited options may prove more useful in such a scenario. Such a survey matches the goal of the study and is considered “natural” because that is how votes during an election are conducted. The sample has experience with this format and expects such a method of data collection. If the goal is simply capturing which candidate has more support, as opposed to understanding why a candidate is supported, a closed-ended or limited-choice survey proves appropriate.
Dalal Albudaiwi
See also Survey: Leading Questions ; Survey: Multiple-Choice Questions ; Survey: Negative-Wording Questions ; Survey: Questionnaire ; Survey Instructions ; Survey Questions, Writing and Phrasing of ; Survey Wording
Further Readings
Allen, M., Titsworth, S., & Hunt, S. K. (2009). Quantitative research in communication. Los Angeles, CA: Sage.
Blair, J., Czaja, R. F., & Blair, E. (2013). Designing surveys: A guide to decisions and procedures (3rd ed.). Beverly Hills, CA: Sage.
Harris, D. F. (2014). The complete guide to writing questionnaires: How to get better information for better decisions. Portland, OR: I&M Press.
Holtz-Bacha, C., & Stomback, J. (Eds.). (2012). Opinion polls and the media: Reflecting and shaping public opinions. Basingstoke, UK: Palgrave Macmillan.
[Page 1717] Punch, K. F. (2005). Introduction to social research-quantitative & qualitative approaches. Beverly Hills, CA: Sage.
Wildemuth, B. M. (Eds.). (2009). Applications of social research method to questions in information and library science . Santa Barbara, CA: Libraries Unlimited.
- Survey: Negative-Wording Questions
- Survey: Questionnaire
- Authoring: Telling a Research Story
- Body Image and Eating Disorders
- Hypothesis Formulation
- Methodology, Selection of
- Program Assessment
- Research Ideas, Sources of
- Research Project, Planning of
- Research Question Formulation
- Research Topic, Definition of
- Research, Inspiration for
- Social Media: Blogs, Microblogs, and Twitter
- Testability
- Acknowledging the Contribution of Others
- Activism and Social Justice
- Anonymous Source of Data
- Authorship Bias
- Authorship Credit
- Confidentiality and Anonymity of Participants
- Conflict of Interest in Research
- Controversial Experiments
- Copyright Issues in Research
- Cultural Sensitivity in Research
- Data Security
- Debriefing of Participants
- Deception in Research
- Ethical Issues, International Research
- Ethics Codes and Guidelines
- Fraudulent and Misleading Data
- Funding Research
- Health Care Disparities
- Human Subjects, Treatment of
- Informed Consent
- Institutional Review Board
- Organizational Ethics
- Peer Review
- Plagiarism, Self-
- Privacy of Information
- Privacy of Participants
- Public Behavior, Recording of
- Reliability, Unitizing
- Research Ethics and Social Values
- Researcher-Participant Relationships
- Social Implications of Research
- Archive Searching for Research
- Bibliographic Research
- Databases, Academic
- Foundation and Government Research Collections
- Library Research
- Literature Review, The
- Literature Reviews, Foundational
- Literature Reviews, Resources for
- Literature Reviews, Strategies for
- Literature Sources, Skeptical and Critical Stance Toward
- Literature, Determining Quality of
- Literature, Determining Relevance of
- Meta-Analysis
- Publications, Scholarly
- Search Engines for Literature Search
- Vote Counting Literature Review Methods
- Abstract or Executive Summary
- Academic Journals
- Alternative Conference Presentation Formats
- American Psychological Association (APA) Style
- Archiving Data
- Blogs and Research
- Chicago Style
- Citations to Research
- Evidence-Based Policy Making
- Invited Publication
- Limitations of Research
- Modern Language Association (MLA) Style
- Narrative Literature Review
- New Media Analysis
- News Media, Writing for
- Panel Presentations and Discussion
- Pay to Review and/or Publish
- Peer Reviewed Publication
- Poster Presentation of Research
- Primary Data Analysis
- Publication Style Guides
- Publication, Politics of
- Publications, Open-Access
- Publishing a Book
- Publishing a Journal Article
- Research Report, Organization of
- Research Reports, Objective
- Research Reports, Subjective
- Scholarship of Teaching and Learning
- Secondary Data
- Submission of Research to a Convention
- Submission of Research to a Journal
- Title of Manuscript, Selection of
- Visual Images as Data Within Qualitative Research
- Writer’s Block
- Writing a Discussion Section
- Writing a Literature Review
- Writing a Methods Section
- Writing a Results Section
- Writing Process, The
- Coding of Data
- Content Analysis, Definition of
- Content Analysis, Process of
- Content Analysis: Advantages and Disadvantages
- Conversation Analysis
- Critical Analysis
- Discourse Analysis
- Interaction Analysis, Quantitative
- Intercoder Reliability
- Intercoder Reliability Coefficients, Comparison of
- Intercoder Reliability Standards: Reproducibility
- Intercoder Reliability Standards: Stability
- Intercoder Reliability Techniques: Cohen’s Kappa
- Intercoder Reliability Techniques: Fleiss System
- Intercoder Reliability Techniques: Holsti Method
- Intercoder Reliability Techniques: Krippendorf Alpha
- Intercoder Reliability Techniques: Percent Agreement
- Intercoder Reliability Techniques: Scott’s Pi
- Metrics for Analysis, Selection of
- Narrative Analysis
- Observational Research Methods
- Observational Research, Advantages and Disadvantages
- Observer Reliability
- Rhetorical and Dramatism Analysis
- Unobtrusive Analysis
- Association of Internet Researchers (AoIR)
- Computer-Mediated Communication (CMC)
- Internet as Cultural Context
- Internet Research and Ethical Decision Making
- Internet Research, Privacy of Participants
- Online and Offline Data, Comparison of
- Online Communities
- Online Data, Collection and Interpretation of
- Online Data, Documentation of
- Online Data, Hacking of
- Online Interviews
- Online Social Worlds
- Social Networks, Online
- Correspondence Analysis
- Cutoff Scores
- Data Cleaning
- Data Reduction
- Data Trimming
- Facial Affect Coding System
- Factor Analysis
- Factor Analysis-Oblique Rotation
- Factor Analysis: Confirmatory
- Factor Analysis: Evolutionary
- Factor Analysis: Exploratory
- Factor Analysis: Internal Consistency
- Factor Analysis: Parallelism Test
- Factor Analysis: Rotated Matrix
- Factor Analysis: Varimax Rotation
- Implicit Measures
- Measurement Levels
- Measurement Levels, Interval
- Measurement Levels, Nominal/Categorical
- Measurement Levels, Ordinal
- Measurement Levels, Ratio
- Observational Measurement: Face Features
- Observational Measurement: Proxemics and Touch
- Observational Measurement: Vocal Qualities
- Organizational Identification
- Outlier Analysis
- Physiological Measurement
- Physiological Measurement: Blood Pressure
- Physiological Measurement: Genital Blood Volume
- Physiological Measurement: Heart Rate
- Physiological Measurement: Pupillary Response
- Physiological Measurement: Skin Conductance
- Reaction Time
- Reliability of Measurement
- Reliability, Cronbach’s Alpha
- Reliability, Knuder-Richardson
- Reliability, Split-half
- Scales, Forced Choice
- Scales, Likert Statement
- Scales, Open-Ended
- Scales, Rank Order
- Scales, Semantic Differential
- Scales, True/False
- Scaling, Guttman
- Standard Score
- Time Series Notation
- Validity, Concurrent
- Validity, Construct
- Validity, Face and Content
- Validity, Halo Effect
- Validity, Measurement of
- Validity, Predictive
- Variables, Conceptualization
- Variables, Operationalization
- Z Transformation
- Confederates
- Generalization
- Imagined Interactions
- Interviewees
- Matched Groups
- Matched Individuals
- Random Assignment of Participants
- Respondents
- Response Style
- Treatment Groups
- Vulnerable Groups
- Experience Sampling Method
- Sample Versus Population
- Sampling Decisions
- Sampling Frames
- Sampling, Internet
- Sampling, Methodological Issues in
- Sampling, Multistage
- Sampling, Nonprobability
- Sampling, Probability
- Sampling, Special Population
- Opinion Polling
- Sampling, Random
- Survey Instructions
- Survey Questions, Writing and Phrasing of
- Survey Response Rates
- Survey Wording
- Survey: Contrast Questions
- Survey: Demographic Questions
- Survey: Dichotomous Questions
- Survey: Filter Questions
- Survey: Follow-up Questions
- Survey: Leading Questions
- Survey: Multiple-Choice Questions
- Survey: Sampling Issues
- Survey: Structural Questions
- Surveys, Advantages and Disadvantages of
- Surveys, Using Others’
- Under-represented Group
- Alternative News Media
- Analytic Induction
- Archival Analysis
- Artifact Selection
- Autoethnography
- Axial Coding
- Burkean Analysis
- Close Reading
- Coding, Fixed
- Coding, Flexible
- Computer-Assisted Qualitative Data Analysis Software (CAQDAS)
- Covert Observation
- Critical Ethnography
- Critical Incident Method
- Critical Race Theory
- Cultural Studies and Communication
- Demand Characteristics
- Ethnographic Interview
- Ethnography
- Ethnomethodology
- Fantasy Theme Analysis
- Feminist Analysis
- Field Notes
- First Wave Feminism
- Fisher Narrative Paradigm
- Focus Groups
- Frame Analysis
- Garfinkling
- Gender-Specific Language
- Grounded Theory
- Hermeneutics
- Historical Analysis
- Informant Interview
- Interaction Analysis, Qualitative
- Interpretative Research
- Interviews for Data Gathering
- Interviews, Recording and Transcribing
- Marxist Analysis
- Meta-ethnography
- Metaphor Analysis
- Narrative Interviewing
- Naturalistic Observation
- Negative Case Analysis
- Neo-Aristotelian Method
- New Media and Participant Observation
- Participant Observer
- Pentadic Analysis
- Performance Research
- Phenomenological Traditions
- Poetic Analysis
- Postcolonial Analysis
- Power in Language
- Pronomial Use-Solidarity
- Psychoanalytic Approaches to Rhetoric
- Public Memory
- Qualitative Data
- Queer Methods
- Queer Theory
- Researcher-Participant Relationships in Observational Research
- Respondent Interviews
- Rhetoric as Epistemic
- Rhetoric, Aristotle’s: Ethos
- Rhetoric, Aristotle’s: Logos
- Rhetoric, Aristotle’s: Pathos
- Rhetoric, Isocrates’
- Rhetorical Artifact
- Rhetorical Method
- Rhetorical Theory
- Second Wave Feminism
- Snowball Subject Recruitment
- Social Constructionism
- Social Network Analysis
- Spontaneous Decision Making
- Symbolic Interactionism
- Terministic Screens
- Textual Analysis
- Thematic Analysis
- Theoretical Traditions
- Third-Wave Feminism
- Transcription Systems
- Triangulation
- Turning Point Analysis
- Unobtrusive Measurement
- Visual Materials, Analysis of
- t -Test, Independent Samples
- t -Test, One Sample
- t -Test, Paired Samples
- Analysis of Covariance (ANCOVA)
- Analysis of Ranks
- Analysis of Variance (ANOVA)
- Bonferroni Correction
- Decomposing Sums of Squares
- Eta Squared
- Factorial Analysis of Variance
- McNemar Test
- One-Tailed Test
- One-Way Analysis of Variance
- Post Hoc Tests
- Post Hoc Tests: Duncan Multiple Range Test
- Post Hoc Tests: Least Significant Difference
- Post Hoc Tests: Scheffe Test
- Post Hoc Tests: Student-Newman-Keuls Test
- Post Hoc Tests: Tukey Honestly Significance Difference Test
- Repeated Measures
- Between-Subjects Design
- Blocking Variable
- Control Groups
- Counterbalancing
- Cross-Sectional Design
- Degrees of Freedom
- Delayed Measurement
- Ex Post Facto Designs
- Experimental Manipulation
- Experiments and Experimental Design
- External Validity
- Extraneous Variables, Control of
- Factor, Crossed
- Factor, Fixed
- Factor, Nested
- Factor, Random
- Factorial Designs
- False Negative
- False Positive
- Field Experiments
- Hierarchical Model
- Individual Difference
- Internal Validity
- Laboratory Experiments
- Latin Square Design
- Longitudinal Design
- Manipulation Check
- Measures of Variability
- Median Split of Sample
- Mixed Level Design
- Multitrial Design
- Null Hypothesis
- One-Group Pretest–Posttest Design
- Orthogonality
- Overidentified Model
- Pilot Study
- Population/Sample
- Power Curves
- Quantitative Research, Purpose of
- Quantitative Research, Steps for
- Quasi-Experimental Design
- Random Assignment
- Replication
- Research Proposal
- Sampling Theory
- Sampling, Determining Size
- Solomon Four-Group Design
- Stimulus Pre-test
- Two-Group Pretest–Posttest Design
- Two-Group Random Assignment Pretest–Posttest Design
- Variables, Control
- Variables, Dependent
- Variables, Independent
- Variables, Latent
- Variables, Marker
- Variables, Mediating Types
- Variables, Moderating Types
- Within-Subjects Design
- Analysis of Residuals
- Bivariate Statistics
- Bootstrapping
- Confidence Interval
- Conjoint Analysis
- Contrast Analysis
- Correlation, Pearson
- Correlation, Point-Biserial
- Correlation, Spearman
- Covariance/Variance Matrix
- Cramér’s V
- Discriminant Analysis
- Kendall’s Tau
- Kruskal-Wallis Test
- Linear Regression
- Linear Versus Nonlinear Relationships
- Multicollinearity
- Multiple Regression
- Multiple Regression: Block Analysis
- Multiple Regression: Covariates in Multiple Regression
- Multiple Regression: Multiple R
- Multiple Regression: Standardized Regression Coefficient
- Partial Correlation
- Phi Coefficient
- Semi-Partial r
- Simple Bivariate Correlation
- Categorization
- Cluster Analysis
- Data Transformation
- Errors of Measurement
- Errors of Measurement: Attenuation
- Errors of Measurement: Ceiling and Floor Effects
- Errors of Measurement: Dichotomization of a Continuous Variable
- Errors of Measurement: Range Restriction
- Errors of Measurement: Regression Toward the Mean
- Frequency Distributions
- Heterogeneity of Variance
- Heteroskedasticity
- Homogeneity of Variance
- Hypothesis Testing, Logic of
- Intraclass Correlation
- Mean, Arithmetic
- Mean, Geometric
- Mean, Harmonic
- Measures of Central Tendency
- Mortality in Sample
- Normal Curve Distribution
- Relationships Between Variables
- Sensitivity Analysis
- Significance Test
- Simple Descriptive Statistics
- Standard Deviation and Variance
- Standard Error
- Standard Error, Mean
- Statistical Power Analysis
- Type I error
- Type II error
- Univariate Statistics
- Variables, Categorical
- Variables, Continuous
- Variables, Defining
- Variables, Interaction of
- Autoregressive, Integrative, Moving Average (ARIMA) Models
- Binomial Effect Size Display
- Cloze Procedure
- Cross Validation
- Cross-Lagged Panel Analysis
- Curvilinear Relationship
- Effect Sizes
- Hierarchical Linear Modeling
- Lag Sequential Analysis
- Log-Linear Analysis
- Logistic Analysis
- Margin of Error
- Markov Analysis
- Maximum Likelihood Estimation
- Meta-Analysis: Estimation of Average Effect
- Meta-Analysis: Fixed Effects Analysis
- Meta-Analysis: Literature Search Issues
- Meta-Analysis: Model Testing
- Meta-Analysis: Random Effects Analysis
- Meta-Analysis: Statistical Conversion to Common Metric
- Multivariate Analysis of Variance (MANOVA)
- Multivariate Statistics
- Ordinary Least Squares
- Path Analysis
- Probit Analysis
- Structural Equation Modeling
- Time-Series Analysis
- Acculturation
- African American Communication and Culture
- Agenda Setting
- Applied Communication
- Argumentation Theory
- Asian/Pacific American Communication Studies
- Bad News, Communication of
- Basic Course in Communication
- Business Communication
- Communication and Aging Research
- Communication and Culture
- Communication and Evolution
- Communication and Future Studies
- Communication and Human Biology
- Communication and Technology
- Communication Apprehension
- Communication Assessment
- Communication Competence
- Communication Education
- Communication Ethics
- Communication History
- Communication Privacy Management Theory
- Communication Skills
- Communication Theory
- Conflict, Mediation, and Negotiation
- Corporate Communication
- Crisis Communication
- Cross-Cultural Communication
- Cyberchondria
- Dark Side of Communication
- Debate and Forensics
- Development of Communication in Children
- Digital Media and Race
- Digital Natives
- Dime Dating
- Disability and Communication
- Distance Learning
- Educational Technology
- Emergency Communication
- Empathic Listening
- English as a Second Language
- Environmental Communication
- Family Communication
- Feminist Communication Studies
- Film Studies
- Financial Communication
- Freedom of Expression
- Game Studies
- Gender and Communication
- GLBT Communication Studies
- GLBT Social Media
- Group Communication
- Health Communication
- Health Literacy
- Human-Computer Interaction
- Instructional Communication
- Intercultural Communication
- Intergenerational Communication
- Intergroup Communication
- International Communication
- International Film
- Interpersonal Communication
- Intrapersonal Communication
- Language and Social Interaction
- Latino Communication
- Legal Communication
- Managerial Communication
- Mass Communication
- Massive Multiplayer Online Games
- Massive Open Online Courses
- Media and Technology Studies
- Media Diffusion
- Media Effects Research
- Media Literacy
- Message Production
- Multiplatform Journalism
- Native American or Indigenous Peoples Communication
- Nonverbal Communication
- Organizational Communication
- Parasocial Communication
- Patient-Centered Communication
- Peace Studies
- Performance Studies
- Personal Relationship Studies
- Philosophy of Communication
- Political Communication
- Political Debates
- Political Economy of Media
- Popular Communication
- Pornography and Research
- Public Address
- Public Relations
- Reality Television
- Relational Dialectics Theory
- Religious Communication
- Rhetorical Genre
- Risk Communication
- Robotic Communication
- Science Communication
- Selective Exposure
- Service Learning
- Small Group Communication
- Social Cognition
- Social Network Systems
- Social Presence
- Social Relationships
- Spirituality and Communication
- Sports Communication
- Strategic Communication
- Structuration Theory
- Training and Development in Organizations
- Video Games
- Visual Communication Studies
- Wartime Communication
- Academic Journal Structure
- Citation Analyses
- Communication Journals
- Interdisciplinary Journals
- Professional Communication Organizations (NCA, ICA, Central, etc.)
Sign in to access this content
Get a 30 day free trial, more like this, sage recommends.
We found other relevant content for you on other Sage platforms.
Have you created a personal profile? Login or create a profile so that you can save clips, playlists and searches
- Sign in/register
Navigating away from this page will delete your results
Please save your results to "My Self-Assessments" in your profile before navigating away from this page.
Sign in to my profile
Please sign into your institution before accessing your profile
Sign up for a free trial and experience all Sage Learning Resources have to offer.
You must have a valid academic email address to sign up.
Get off-campus access
- View or download all content my institution has access to.
Sign up for a free trial and experience all Sage Learning Resources has to offer.
- view my profile
- view my lists
Why Are Open-Ended Questions Important In Qualitative Research?
Nov 8, 2023 | User Acceptance Testing , User Research
Qualitative research is crucial in understanding the complexities of human behaviour, experiences, and perspectives.
Table of Contents
It allows researchers to explore the richness and depth of individuals’ thoughts, feelings, decision making process and motivations.
One of the critical tools in qualitative research is the use of open-ended questions. Open-ended questions invite respondents to provide detailed and personalised responses—allowing for a more nuanced understanding of the topic at hand.
This article aims to explore the importance of open-ended questions in qualitative research and share some actionable tips for crafting practical questions. So, let’s dig in!
What is qualitative research?
Before delving into the significance of open-ended questions, let’s first understand what qualitative research entails.
Qualitative research is an exploratory approach that aims to understand the meaning and interpretation individuals attach to their experiences.
Unlike quantitative research, which focuses on numerical data and statistical analysis, qualitative research emphasises in capturing the richness and depth of human experiences through methods like interviews, think aloud usability test, focus groups, and observations.
Objectives of qualitative research in usability testing
In the context of usability testing, qualitative research helps uncover users’ thoughts, emotions, and attitudes towards a product or service.
Fundamentally, it provides valuable insights into user behaviour, preferences, pain points, and areas for improvement.
By leveraging open-ended questions, researchers can uncover the underlying reasons behind users’ actions and gain a deeper understanding of their needs and expectations.
Differences between qualitative and quantitative research methods
Qualitative and quantitative research methods typically differ in their approaches, data collection techniques, and analysis.
For context, quantitative research focuses on numerical data, statistical analysis, and generalizability, while qualitative research seeks to explore and understand specific contexts, meanings, and interpretations.
Furthermore, qualitative research is more subjective, allowing for greater depth and richness of data, while quantitative research prioritises objectivity and generalizability.
What are open-ended questions?
Open-ended questions are questions that don’t have predefined or limited answer options. They encourage respondents to provide detailed and personalised responses, allowing them to express their thoughts, feelings, and experiences in their own words.
Unlike closed-ended questions, which may be answered with a simple “yes” or “no” or by selecting from a list of options, open-ended questions invite respondents to provide more elaborate and nuanced responses.
Characteristics of open-ended questions
Open-ended questions are characterised by several key elements that distinguish them from closed-ended questions, namely:
- Freedom of response: Respondents can express themselves freely with open-ended questions because there are no predetermined answer options.
- Richness of information: Open-ended questions encourage respondents to provide detailed and in-depth responses, providing researchers with a wealth of information.
- Flexibility: Open-ended questions give respondents the flexibility to respond in a way that makes sense to them, allowing for diverse perspectives and insights.
- Exploration of complexity: These questions help explore complex phenomena, opinions, and experiences that cannot be easily captured by closed-ended questions.
Importance of open-ended questions in qualitative research
Open-ended questions play a vital role in qualitative research for several reasons, namely:
Encouraging detailed responses
Open-ended questions enable respondents to provide more detailed and nuanced responses. By avoiding predetermined options, researchers can capture the richness and complexity of individuals’ thoughts, feelings, and experiences.
This depth of information is invaluable in gaining a comprehensive understanding of the research topic.
Facilitating a deeper understanding
Open-ended questions provide researchers with a better understanding of participants’ perspectives, beliefs, attitudes, and experiences.
By allowing individuals to express themselves freely, researchers can gain insights into the underlying reasons behind their actions and decision-making processes.
This deeper understanding is crucial for uncovering the underlying motivations and meanings that drive human behaviour.
Flexibility and adaptability
Open-ended questions offer flexibility and adaptability in qualitative research. They give participants a platform to present fresh themes, concepts, and viewpoints that the researcher might not have anticipated.
This flexibility allows for the emergence of unexpected insights and encourages a more exploratory and dynamic research process.
Tips for crafting effective open-ended questions
Open-ended questions, designed to elicit rich and authentic responses, are essential tools for researchers seeking to unravel the depth of participant perspectives.
Here are some actionable tips to help you master the art of crafting effective, open-ended questions:
1. Align questions with objectives
Before penning down your open-ended questions, it’s crucial to align them with the overarching objectives of your research. Clear alignment ensures that each question serves a purpose in contributing to the depth and breadth of your study.
For example, if your objective is to understand user satisfaction with a new software interface, frame questions that specifically address different aspects of the UX design , such as navigation, font readability, and functionality.
2. Clarity and comprehension
Ambiguity in questions can hinder the quality of responses. Participants should easily comprehend the intent of each question, allowing them to provide insightful and relevant answers.
Always ensure that your questions are clear, concise, and free of jargon. Test your questions beforehand on a diverse audience to identify any potential confusion and refine them accordingly.
3. Maintain neutrality
A neutral tone in your questions is essential to minimise bias. Participants should feel free to express their genuine opinions without worrying about the researcher’s judgment.
Avoid injecting personal opinions, judgements, or assumptions into your questions. Instead, present inquiries in an objective and non-directive manner to foster an open and honest exchange.
4. Encourage openness
Creating an environment that encourages participants to open up is vital for qualitative research. Open-ended questions should invite participants to share their thoughts and experiences freely.
Begin questions with phrases that signal openness, such as “Tell me about…” or “Describe your experience with…” Such prompts set the stage for participants to share their perspectives openly.
5. Use probing questions
While open-ended questions provide an initial exploration, supplementing them with probing questions allows researchers to delve deeper into specific aspects.
Probing questions guide participants to elaborate on their initial responses.
After receiving an open-ended response, follow up with probing questions that seek clarification, ask for examples, or explore the participant’s feelings in more detail.
This layered approach enriches the data collected.
6. Frame questions that encourage respondents to share stories
Human experiences are often best expressed through stories. Crafting questions that invite participants to share narratives can provide a deeper understanding of their perspectives.
Furthermore, always ask questions that prompt participants to recount specific experiences or share anecdotes related to the topic. Remember, stories add context, emotion, and a human touch to the research data.
All things considered, the effectiveness of open-ended questions lies not only in their form but in the thoughtful application of these tips.
Common mistakes to avoid with open-ended questions
Pitfalls lurk along this path of crafting and using open-ended questions. It is important to be mindful of the common mistakes to ensure the authenticity and reliability of the data collected.
Let’s explore these potential pitfalls and learn how to navigate around them, shall we?
1. Leading questions
Leading questions subtly guide participants toward a particular response, often unintentionally injecting the researcher’s bias into the inquiry.
These questions can steer participants away from expressing their genuine thoughts and experiences.
Craft open-ended questions with a neutral tone, avoiding any language that may suggest a preferred answer. By maintaining objectivity, researchers create a safe space for participants to share their perspectives without feeling influenced.
Example of a Leading Question:
Leading: “Don’t you think the new feature significantly improved your user experience?”
Revised: “How has the new feature impacted your user experience?”
2. Double-barreled questions
Double-barreled questions address more than one issue in a single inquiry, potentially causing confusion for participants. This can lead to ambiguous or unreliable responses as participants may not clearly distinguish between the two issues presented.
Always break down complex inquiries into single-issue questions, as this not only enhances clarity but also allows participants to provide specific and focused responses to each component of the question.
Example of a Double-Barreled Question:
Double-barreled: “How satisfied are you with the product’s functionality and design?”
Revised: “How satisfied are you with the product’s functionality? How about its design?”
3. Overly complex questions
Complex questions, laden with jargon or convoluted language, can overwhelm participants. When faced with complexity, participants may struggle to comprehend the question, leading to vague or incomplete responses that do not truly reflect their experiences.
Frame questions in clear and straightforward language to ensure participants easily grasp the intent. A well-understood question encourages participants to provide thoughtful and meaningful responses.
Example of an Overly Complex Question:
Complex: “In what ways do the multifaceted functionalities of the application contribute to your overall user satisfaction?”
Revised: “How do the application’s features contribute to your overall satisfaction?”
In summary, open-ended questions are indispensable tools in qualitative research.
They allow UX researchers to explore the complexity and diversity of human experiences, thoughts, and perspectives.
Open-ended questions provide valuable insights that go beyond mere numerical data. It encourages detailed and personalised responses,.
Remember to align the questions with your research objectives, ensuring clarity and neutrality and encouraging openness and storytelling.
Researchers often learn more about their subjects and find valuable insights that drive meaningful research outcomes when they use open-ended questions.
Uncover powerful insights with open-ended questions. Learn more about our UX Research services and how we can help you design experiences that resonate with your users.
Related posts:
- Field Studies vs Usability Testing: Which Is Better?
Qualitative Usability Testing Tips
- Key Roles in Building An Enterprise UX Team
- How to Create an Orchestrated Omnichannel User Experience
UX insights delivered straight to you like an exclusive club once a month
You have successfully subscribed, recent articles, 5 stages of design thinking process you need to know.
Sep 5, 2024
Staying ahead of the curve means continually innovating and adapting. Entrepreneurs and mobile app developers often face the challenge of creating solutions that not only solve real problems— but also deliver exceptional user experiences. This is where design thinking...
10 Ways Fintechs Use Experience Design to Surpass Banking
Aug 4, 2024
Malaysia’s Fintech landscape is booming, surpassing even the banking sector. Fintech companies place a lot of importance and emphasis on creating the perfect digital experience design ensuring that their customers receive the best user experience (UX) possible. Only...
Quantitative Usability Testing Tips You Should Consider
Feb 27, 2024
UI/UX designers aim to not only create visually appealing interfaces but also ensure that users can interact with their designs effortlessly. In this quest, quantitative usability testing is a fundamental tenet. This in-depth guide seeks to delve into the intricacies...
Nov 30, 2023
Usability testing is a crucial part of the UX design process that helps businesses understand how users interact with their products and identify areas for improvement. One effective approach to usability testing is qualitative testing, which focuses on gathering...
How To Use Generative AI Tools and Applications For Your Research Workflow
Nov 29, 2023
Within this rapidly evolving technological landscape, unique Generative AI tools and applications have emerged as invaluable companions for both seasoned UX designers and curious user researchers. These tools offer a mosaic of innovative possibilities, serving as...
Thank you for visiting nature.com. You are using a browser version with limited support for CSS. To obtain the best experience, we recommend you use a more up to date browser (or turn off compatibility mode in Internet Explorer). In the meantime, to ensure continued support, we are displaying the site without styles and JavaScript.
- View all journals
- Explore content
- About the journal
- Publish with us
- Sign up for alerts
- Published: 15 September 2022
Interviews in the social sciences
- Eleanor Knott ORCID: orcid.org/0000-0002-9131-3939 1 ,
- Aliya Hamid Rao ORCID: orcid.org/0000-0003-0674-4206 1 ,
- Kate Summers ORCID: orcid.org/0000-0001-9964-0259 1 &
- Chana Teeger ORCID: orcid.org/0000-0002-5046-8280 1
Nature Reviews Methods Primers volume 2 , Article number: 73 ( 2022 ) Cite this article
757k Accesses
113 Citations
191 Altmetric
Metrics details
- Interdisciplinary studies
In-depth interviews are a versatile form of qualitative data collection used by researchers across the social sciences. They allow individuals to explain, in their own words, how they understand and interpret the world around them. Interviews represent a deceptively familiar social encounter in which people interact by asking and answering questions. They are, however, a very particular type of conversation, guided by the researcher and used for specific ends. This dynamic introduces a range of methodological, analytical and ethical challenges, for novice researchers in particular. In this Primer, we focus on the stages and challenges of designing and conducting an interview project and analysing data from it, as well as strategies to overcome such challenges.
Similar content being viewed by others
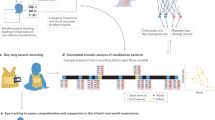
The fundamental importance of method to theory
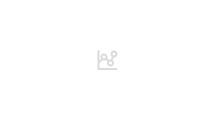
How ‘going online’ mediates the challenges of policy elite interviews
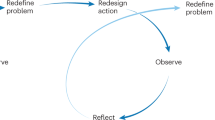
Participatory action research
Introduction.
In-depth interviews are a qualitative research method that follow a deceptively familiar logic of human interaction: they are conversations where people talk with each other, interact and pose and answer questions 1 . An interview is a specific type of interaction in which — usually and predominantly — a researcher asks questions about someone’s life experience, opinions, dreams, fears and hopes and the interview participant answers the questions 1 .
Interviews will often be used as a standalone method or combined with other qualitative methods, such as focus groups or ethnography, or quantitative methods, such as surveys or experiments. Although interviewing is a frequently used method, it should not be viewed as an easy default for qualitative researchers 2 . Interviews are also not suited to answering all qualitative research questions, but instead have specific strengths that should guide whether or not they are deployed in a research project. Whereas ethnography might be better suited to trying to observe what people do, interviews provide a space for extended conversations that allow the researcher insights into how people think and what they believe. Quantitative surveys also give these kinds of insights, but they use pre-determined questions and scales, privileging breadth over depth and often overlooking harder-to-reach participants.
In-depth interviews can take many different shapes and forms, often with more than one participant or researcher. For example, interviews might be highly structured (using an almost survey-like interview guide), entirely unstructured (taking a narrative and free-flowing approach) or semi-structured (using a topic guide ). Researchers might combine these approaches within a single project depending on the purpose of the interview and the characteristics of the participant. Whatever form the interview takes, researchers should be mindful of the dynamics between interviewer and participant and factor these in at all stages of the project.
In this Primer, we focus on the most common type of interview: one researcher taking a semi-structured approach to interviewing one participant using a topic guide. Focusing on how to plan research using interviews, we discuss the necessary stages of data collection. We also discuss the stages and thought-process behind analysing interview material to ensure that the richness and interpretability of interview material is maintained and communicated to readers. The Primer also tracks innovations in interview methods and discusses the developments we expect over the next 5–10 years.
We wrote this Primer as researchers from sociology, social policy and political science. We note our disciplinary background because we acknowledge that there are disciplinary differences in how interviews are approached and understood as a method.
Experimentation
Here we address research design considerations and data collection issues focusing on topic guide construction and other pragmatics of the interview. We also explore issues of ethics and reflexivity that are crucial throughout the research project.
Research design
Participant selection.
Participants can be selected and recruited in various ways for in-depth interview studies. The researcher must first decide what defines the people or social groups being studied. Often, this means moving from an abstract theoretical research question to a more precise empirical one. For example, the researcher might be interested in how people talk about race in contexts of diversity. Empirical settings in which this issue could be studied could include schools, workplaces or adoption agencies. The best research designs should clearly explain why the particular setting was chosen. Often there are both intrinsic and extrinsic reasons for choosing to study a particular group of people at a specific time and place 3 . Intrinsic motivations relate to the fact that the research is focused on an important specific social phenomenon that has been understudied. Extrinsic motivations speak to the broader theoretical research questions and explain why the case at hand is a good one through which to address them empirically.
Next, the researcher needs to decide which types of people they would like to interview. This decision amounts to delineating the inclusion and exclusion criteria for the study. The criteria might be based on demographic variables, like race or gender, but they may also be context-specific, for example, years of experience in an organization. These should be decided based on the research goals. Researchers should be clear about what characteristics would make an individual a candidate for inclusion in the study (and what would exclude them).
The next step is to identify and recruit the study’s sample . Usually, many more people fit the inclusion criteria than can be interviewed. In cases where lists of potential participants are available, the researcher might want to employ stratified sampling , dividing the list by characteristics of interest before sampling.
When there are no lists, researchers will often employ purposive sampling . Many researchers consider purposive sampling the most useful mode for interview-based research since the number of interviews to be conducted is too small to aim to be statistically representative 4 . Instead, the aim is not breadth, via representativeness, but depth via rich insights about a set of participants. In addition to purposive sampling, researchers often use snowball sampling . Both purposive and snowball sampling can be combined with quota sampling . All three types of sampling aim to ensure a variety of perspectives within the confines of a research project. A goal for in-depth interview studies can be to sample for range, being mindful of recruiting a diversity of participants fitting the inclusion criteria.
Study design
The total number of interviews depends on many factors, including the population studied, whether comparisons are to be made and the duration of interviews. Studies that rely on quota sampling where explicit comparisons are made between groups will require a larger number of interviews than studies focused on one group only. Studies where participants are interviewed over several hours, days or even repeatedly across years will tend to have fewer participants than those that entail a one-off engagement.
Researchers often stop interviewing when new interviews confirm findings from earlier interviews with no new or surprising insights (saturation) 4 , 5 , 6 . As a criterion for research design, saturation assumes that data collection and analysis are happening in tandem and that researchers will stop collecting new data once there is no new information emerging from the interviews. This is not always possible. Researchers rarely have time for systematic data analysis during data collection and they often need to specify their sample in funding proposals prior to data collection. As a result, researchers often draw on existing reports of saturation to estimate a sample size prior to data collection. These suggest between 12 and 20 interviews per category of participant (although researchers have reported saturation with samples that are both smaller and larger than this) 7 , 8 , 9 . The idea of saturation has been critiqued by many qualitative researchers because it assumes that meaning inheres in the data, waiting to be discovered — and confirmed — once saturation has been reached 7 . In-depth interview data are often multivalent and can give rise to different interpretations. The important consideration is, therefore, not merely how many participants are interviewed, but whether one’s research design allows for collecting rich and textured data that provide insight into participants’ understandings, accounts, perceptions and interpretations.
Sometimes, researchers will conduct interviews with more than one participant at a time. Researchers should consider the benefits and shortcomings of such an approach. Joint interviews may, for example, give researchers insight into how caregivers agree or debate childrearing decisions. At the same time, they may be less adaptive to exploring aspects of caregiving that participants may not wish to disclose to each other. In other cases, there may be more than one person interviewing each participant, such as when an interpreter is used, and so it is important to consider during the research design phase how this might shape the dynamics of the interview.
Data collection
Semi-structured interviews are typically organized around a topic guide comprised of an ordered set of broad topics (usually 3–5). Each topic includes a set of questions that form the basis of the discussion between the researcher and participant (Fig. 1 ). These topics are organized around key concepts that the researcher has identified (for example, through a close study of prior research, or perhaps through piloting a small, exploratory study) 5 .
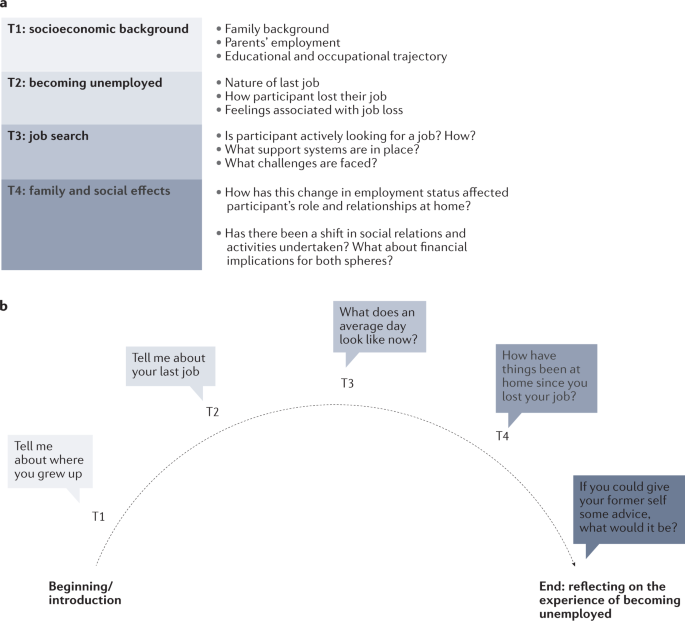
a | Elaborated topics the researcher wants to cover in the interview and example questions. b | An example topic arc. Using such an arc, one can think flexibly about the order of topics. Considering the main question for each topic will help to determine the best order for the topics. After conducting some interviews, the researcher can move topics around if a different order seems to make sense.
Topic guide
One common way to structure a topic guide is to start with relatively easy, open-ended questions (Table 1 ). Opening questions should be related to the research topic but broad and easy to answer, so that they help to ease the participant into conversation.
After these broad, opening questions, the topic guide may move into topics that speak more directly to the overarching research question. The interview questions will be accompanied by probes designed to elicit concrete details and examples from the participant (see Table 1 ).
Abstract questions are often easier for participants to answer once they have been asked more concrete questions. In our experience, for example, questions about feelings can be difficult for some participants to answer, but when following probes concerning factual experiences these questions can become less challenging. After the main themes of the topic guide have been covered, the topic guide can move onto closing questions. At this stage, participants often repeat something they have said before, although they may sometimes introduce a new topic.
Interviews are especially well suited to gaining a deeper insight into people’s experiences. Getting these insights largely depends on the participants’ willingness to talk to the researcher. We recommend designing open-ended questions that are more likely to elicit an elaborated response and extended reflection from participants rather than questions that can be answered with yes or no.
Questions should avoid foreclosing the possibility that the participant might disagree with the premise of the question. Take for example the question: “Do you support the new family-friendly policies?” This question minimizes the possibility of the participant disagreeing with the premise of this question, which assumes that the policies are ‘family-friendly’ and asks for a yes or no answer. Instead, asking more broadly how a participant feels about the specific policy being described as ‘family-friendly’ (for example, a work-from-home policy) allows them to express agreement, disagreement or impartiality and, crucially, to explain their reasoning 10 .
For an uninterrupted interview that will last between 90 and 120 minutes, the topic guide should be one to two single-spaced pages with questions and probes. Ideally, the researcher will memorize the topic guide before embarking on the first interview. It is fine to carry a printed-out copy of the topic guide but memorizing the topic guide ahead of the interviews can often make the interviewer feel well prepared in guiding the participant through the interview process.
Although the topic guide helps the researcher stay on track with the broad areas they want to cover, there is no need for the researcher to feel tied down by the topic guide. For instance, if a participant brings up a theme that the researcher intended to discuss later or a point the researcher had not anticipated, the researcher may well decide to follow the lead of the participant. The researcher’s role extends beyond simply stating the questions; it entails listening and responding, making split-second decisions about what line of inquiry to pursue and allowing the interview to proceed in unexpected directions.
Optimizing the interview
The ideal place for an interview will depend on the study and what is feasible for participants. Generally, a place where the participant and researcher can both feel relaxed, where the interview can be uninterrupted and where noise or other distractions are limited is ideal. But this may not always be possible and so the researcher needs to be prepared to adapt their plans within what is feasible (and desirable for participants).
Another key tool for the interview is a recording device (assuming that permission for recording has been given). Recording can be important to capture what the participant says verbatim. Additionally, it can allow the researcher to focus on determining what probes and follow-up questions they want to pursue rather than focusing on taking notes. Sometimes, however, a participant may not allow the researcher to record, or the recording may fail. If the interview is not recorded we suggest that the researcher takes brief notes during the interview, if feasible, and then thoroughly make notes immediately after the interview and try to remember the participant’s facial expressions, gestures and tone of voice. Not having a recording of an interview need not limit the researcher from getting analytical value from it.
As soon as possible after each interview, we recommend that the researcher write a one-page interview memo comprising three key sections. The first section should identify two to three important moments from the interview. What constitutes important is up to the researcher’s discretion 9 . The researcher should note down what happened in these moments, including the participant’s facial expressions, gestures, tone of voice and maybe even the sensory details of their surroundings. This exercise is about capturing ethnographic detail from the interview. The second part of the interview memo is the analytical section with notes on how the interview fits in with previous interviews, for example, where the participant’s responses concur or diverge from other responses. The third part consists of a methodological section where the researcher notes their perception of their relationship with the participant. The interview memo allows the researcher to think critically about their positionality and practice reflexivity — key concepts for an ethical and transparent research practice in qualitative methodology 11 , 12 .
Ethics and reflexivity
All elements of an in-depth interview can raise ethical challenges and concerns. Good ethical practice in interview studies often means going beyond the ethical procedures mandated by institutions 13 . While discussions and requirements of ethics can differ across disciplines, here we focus on the most pertinent considerations for interviews across the research process for an interdisciplinary audience.
Ethical considerations prior to interview
Before conducting interviews, researchers should consider harm minimization, informed consent, anonymity and confidentiality, and reflexivity and positionality. It is important for the researcher to develop their own ethical sensitivities and sensibilities by gaining training in interview and qualitative methods, reading methodological and field-specific texts on interviews and ethics and discussing their research plans with colleagues.
Researchers should map the potential harm to consider how this can be minimized. Primarily, researchers should consider harm from the participants’ perspective (Box 1 ). But, it is also important to consider and plan for potential harm to the researcher, research assistants, gatekeepers, future researchers and members of the wider community 14 . Even the most banal of research topics can potentially pose some form of harm to the participant, researcher and others — and the level of harm is often highly context-dependent. For example, a research project on religion in society might have very different ethical considerations in a democratic versus authoritarian research context because of how openly or not such topics can be discussed and debated 15 .
The researcher should consider how they will obtain and record informed consent (for example, written or oral), based on what makes the most sense for their research project and context 16 . Some institutions might specify how informed consent should be gained. Regardless of how consent is obtained, the participant must be made aware of the form of consent, the intentions and procedures of the interview and potential forms of harm and benefit to the participant or community before the interview commences. Moreover, the participant must agree to be interviewed before the interview commences. If, in addition to interviews, the study contains an ethnographic component, it is worth reading around this topic (see, for example, Murphy and Dingwall 17 ). Informed consent must also be gained for how the interview will be recorded before the interview commences. These practices are important to ensure the participant is contributing on a voluntary basis. It is also important to remind participants that they can withdraw their consent at any time during the interview and for a specified period after the interview (to be decided with the participant). The researcher should indicate that participants can ask for anything shared to be off the record and/or not disseminated.
In terms of anonymity and confidentiality, it is standard practice when conducting interviews to agree not to use (or even collect) participants’ names and personal details that are not pertinent to the study. Anonymizing can often be the safer option for minimizing harm to participants as it is hard to foresee all the consequences of de-anonymizing, even if participants agree. Regardless of what a researcher decides, decisions around anonymity must be agreed with participants during the process of gaining informed consent and respected following the interview.
Although not all ethical challenges can be foreseen or planned for 18 , researchers should think carefully — before the interview — about power dynamics, participant vulnerability, emotional state and interactional dynamics between interviewer and participant, even when discussing low-risk topics. Researchers may then wish to plan for potential ethical issues, for example by preparing a list of relevant organizations to which participants can be signposted. A researcher interviewing a participant about debt, for instance, might prepare in advance a list of debt advice charities, organizations and helplines that could provide further support and advice. It is important to remember that the role of an interviewer is as a researcher rather than as a social worker or counsellor because researchers may not have relevant and requisite training in these other domains.
Box 1 Mapping potential forms of harm
Social: researchers should avoid causing any relational detriment to anyone in the course of interviews, for example, by sharing information with other participants or causing interview participants to be shunned or mistreated by their community as a result of participating.
Economic: researchers should avoid causing financial detriment to anyone, for example, by expecting them to pay for transport to be interviewed or to potentially lose their job as a result of participating.
Physical: researchers should minimize the risk of anyone being exposed to violence as a result of the research both from other individuals or from authorities, including police.
Psychological: researchers should minimize the risk of causing anyone trauma (or re-traumatization) or psychological anguish as a result of the research; this includes not only the participant but importantly the researcher themselves and anyone that might read or analyse the transcripts, should they contain triggering information.
Political: researchers should minimize the risk of anyone being exposed to political detriment as a result of the research, such as retribution.
Professional/reputational: researchers should minimize the potential for reputational damage to anyone connected to the research (this includes ensuring good research practices so that any researchers involved are not harmed reputationally by being involved with the research project).
The task here is not to map exhaustively the potential forms of harm that might pertain to a particular research project (that is the researcher’s job and they should have the expertise most suited to mapping such potential harms relative to the specific project) but to demonstrate the breadth of potential forms of harm.
Ethical considerations post-interview
Researchers should consider how interview data are stored, analysed and disseminated. If participants have been offered anonymity and confidentiality, data should be stored in a way that does not compromise this. For example, researchers should consider removing names and any other unnecessary personal details from interview transcripts, password-protecting and encrypting files and using pseudonyms to label and store all interview data. It is also important to address where interview data are taken (for example, across borders in particular where interview data might be of interest to local authorities) and how this might affect the storage of interview data.
Examining how the researcher will represent participants is a paramount ethical consideration both in the planning stages of the interview study and after it has been conducted. Dissemination strategies also need to consider questions of anonymity and representation. In small communities, even if participants are given pseudonyms, it might be obvious who is being described. Anonymizing not only the names of those participating but also the research context is therefore a standard practice 19 . With particularly sensitive data or insights about the participant, it is worth considering describing participants in a more abstract way rather than as specific individuals. These practices are important both for protecting participants’ anonymity but can also affect the ability of the researcher and others to return ethically to the research context and similar contexts 20 .
Reflexivity and positionality
Reflexivity and positionality mean considering the researcher’s role and assumptions in knowledge production 13 . A key part of reflexivity is considering the power relations between the researcher and participant within the interview setting, as well as how researchers might be perceived by participants. Further, researchers need to consider how their own identities shape the kind of knowledge and assumptions they bring to the interview, including how they approach and ask questions and their analysis of interviews (Box 2 ). Reflexivity is a necessary part of developing ethical sensibility as a researcher by adapting and reflecting on how one engages with participants. Participants should not feel judged, for example, when they share information that researchers might disagree with or find objectionable. How researchers deal with uncomfortable moments or information shared by participants is at their discretion, but they should consider how they will react both ahead of time and in the moment.
Researchers can develop their reflexivity by considering how they themselves would feel being asked these interview questions or represented in this way, and then adapting their practice accordingly. There might be situations where these questions are not appropriate in that they unduly centre the researchers’ experiences and worldview. Nevertheless, these prompts can provide a useful starting point for those beginning their reflexive journey and developing an ethical sensibility.
Reflexivity and ethical sensitivities require active reflection throughout the research process. For example, researchers should take care in interview memos and their notes to consider their assumptions, potential preconceptions, worldviews and own identities prior to and after interviews (Box 2 ). Checking in with assumptions can be a way of making sure that researchers are paying close attention to their own theoretical and analytical biases and revising them in accordance with what they learn through the interviews. Researchers should return to these notes (especially when analysing interview material), to try to unpack their own effects on the research process as well as how participants positioned and engaged with them.
Box 2 Aspects to reflect on reflexively
For reflexive engagement, and understanding the power relations being co-constructed and (re)produced in interviews, it is necessary to reflect, at a minimum, on the following.
Ethnicity, race and nationality, such as how does privilege stemming from race or nationality operate between the researcher, the participant and research context (for example, a researcher from a majority community may be interviewing a member of a minority community)
Gender and sexuality, see above on ethnicity, race and nationality
Social class, and in particular the issue of middle-class bias among researchers when formulating research and interview questions
Economic security/precarity, see above on social class and thinking about the researcher’s relative privilege and the source of biases that stem from this
Educational experiences and privileges, see above
Disciplinary biases, such as how the researcher’s discipline/subfield usually approaches these questions, possibly normalizing certain assumptions that might be contested by participants and in the research context
Political and social values
Lived experiences and other dimensions of ourselves that affect and construct our identity as researchers
In this section, we discuss the next stage of an interview study, namely, analysing the interview data. Data analysis may begin while more data are being collected. Doing so allows early findings to inform the focus of further data collection, as part of an iterative process across the research project. Here, the researcher is ultimately working towards achieving coherence between the data collected and the findings produced to answer successfully the research question(s) they have set.
The two most common methods used to analyse interview material across the social sciences are thematic analysis 21 and discourse analysis 22 . Thematic analysis is a particularly useful and accessible method for those starting out in analysis of qualitative data and interview material as a method of coding data to develop and interpret themes in the data 21 . Discourse analysis is more specialized and focuses on the role of discourse in society by paying close attention to the explicit, implicit and taken-for-granted dimensions of language and power 22 , 23 . Although thematic and discourse analysis are often discussed as separate techniques, in practice researchers might flexibly combine these approaches depending on the object of analysis. For example, those intending to use discourse analysis might first conduct thematic analysis as a way to organize and systematize the data. The object and intention of analysis might differ (for example, developing themes or interrogating language), but the questions facing the researcher (such as whether to take an inductive or deductive approach to analysis) are similar.
Preparing data
Data preparation is an important step in the data analysis process. The researcher should first determine what comprises the corpus of material and in what form it will it be analysed. The former refers to whether, for example, alongside the interviews themselves, analytic memos or observational notes that may have been taken during data collection will also be directly analysed. The latter refers to decisions about how the verbal/audio interview data will be transformed into a written form, making it suitable for processes of data analysis. Typically, interview audio recordings are transcribed to produce a written transcript. It is important to note that the process of transcription is one of transformation. The verbal interview data are transformed into a written transcript through a series of decisions that the researcher must make. The researcher should consider the effect of mishearing what has been said or how choosing to punctuate a sentence in a particular way will affect the final analysis.
Box 3 shows an example transcript excerpt from an interview with a teacher conducted by Teeger as part of her study of history education in post-apartheid South Africa 24 (Box 3 ). Seeing both the questions and the responses means that the reader can contextualize what the participant (Ms Mokoena) has said. Throughout the transcript the researcher has used square brackets, for example to indicate a pause in speech, when Ms Mokoena says “it’s [pause] it’s a difficult topic”. The transcription choice made here means that we see that Ms Mokoena has taken time to pause, perhaps to search for the right words, or perhaps because she has a slight apprehension. Square brackets are also included as an overt act of communication to the reader. When Ms Mokoena says “ja”, the English translation (“yes”) of the word in Afrikaans is placed in square brackets to ensure that the reader can follow the meaning of the speech.
Decisions about what to include when transcribing will be hugely important for the direction and possibilities of analysis. Researchers should decide what they want to capture in the transcript, based on their analytic focus. From a (post)positivist perspective 25 , the researcher may be interested in the manifest content of the interview (such as what is said, not how it is said). In that case, they may choose to transcribe intelligent verbatim . From a constructivist perspective 25 , researchers may choose to record more aspects of speech (including, for example, pauses, repetitions, false starts, talking over one another) so that these features can be analysed. Those working from this perspective argue that to recognize the interactional nature of the interview setting adequately and to avoid misinterpretations, features of interaction (pauses, overlaps between speakers and so on) should be preserved in transcription and therefore in the analysis 10 . Readers interested in learning more should consult Potter and Hepburn’s summary of how to present interaction through transcription of interview data 26 .
The process of analysing semi-structured interviews might be thought of as a generative rather than an extractive enterprise. Findings do not already exist within the interview data to be discovered. Rather, researchers create something new when analysing the data by applying their analytic lens or approach to the transcripts. At a high level, there are options as to what researchers might want to glean from their interview data. They might be interested in themes, whereby they identify patterns of meaning across the dataset 21 . Alternatively, they may focus on discourse(s), looking to identify how language is used to construct meanings and therefore how language reinforces or produces aspects of the social world 27 . Alternatively, they might look at the data to understand narrative or biographical elements 28 .
A further overarching decision to make is the extent to which researchers bring predetermined framings or understandings to bear on their data, or instead begin from the data themselves to generate an analysis. One way of articulating this is the extent to which researchers take a deductive approach or an inductive approach to analysis. One example of a truly inductive approach is grounded theory, whereby the aim of the analysis is to build new theory, beginning with one’s data 6 , 29 . In practice, researchers using thematic and discourse analysis often combine deductive and inductive logics and describe their process instead as iterative (referred to also as an abductive approach ) 30 , 31 . For example, researchers may decide that they will apply a given theoretical framing, or begin with an initial analytic framework, but then refine or develop these once they begin the process of analysis.
Box 3 Excerpt of interview transcript (from Teeger 24 )
Interviewer : Maybe you could just start by talking about what it’s like to teach apartheid history.
Ms Mokoena : It’s a bit challenging. You’ve got to accommodate all the kids in the class. You’ve got to be sensitive to all the racial differences. You want to emphasize the wrongs that were done in the past but you also want to, you know, not to make kids feel like it’s their fault. So you want to use the wrongs of the past to try and unite the kids …
Interviewer : So what kind of things do you do?
Ms Mokoena : Well I normally highlight the fact that people that were struggling were not just the blacks, it was all the races. And I give examples of the people … from all walks of life, all races, and highlight how they suffered as well as a result of apartheid, particularly the whites… . What I noticed, particularly my first year of teaching apartheid, I noticed that the black kids made the others feel responsible for what happened… . I had a lot of fights…. A lot of kids started hating each other because, you know, the others are white and the others were black. And they started saying, “My mother is a domestic worker because she was never allowed an opportunity to get good education.” …
Interviewer : I didn’t see any of that now when I was observing.
Ms Mokoena : … Like I was saying I think that because of the re-emphasis of the fact that, look, everybody did suffer one way or the other, they sort of got to see that it was everybody’s struggle … . They should now get to understand that that’s why we’re called a Rainbow Nation. Not everybody agreed with apartheid and not everybody suffered. Even all the blacks, not all blacks got to feel what the others felt . So ja [yes], it’s [pause] it’s a difficult topic, ja . But I think if you get the kids to understand why we’re teaching apartheid in the first place and you show the involvement of all races in all the different sides , then I think you have managed to teach it properly. So I think because of my inexperience then — that was my first year of teaching history — so I think I — maybe I over-emphasized the suffering of the blacks versus the whites [emphasis added].
Reprinted with permission from ref. 24 , Sage Publications.
From data to codes
Coding data is a key building block shared across many approaches to data analysis. Coding is a way of organizing and describing data, but is also ultimately a way of transforming data to produce analytic insights. The basic practice of coding involves highlighting a segment of text (this may be a sentence, a clause or a longer excerpt) and assigning a label to it. The aim of the label is to communicate some sort of summary of what is in the highlighted piece of text. Coding is an iterative process, whereby researchers read and reread their transcripts, applying and refining their codes, until they have a coding frame (a set of codes) that is applied coherently across the dataset and that captures and communicates the key features of what is contained in the data as it relates to the researchers’ analytic focus.
What one codes for is entirely contingent on the focus of the research project and the choices the researcher makes about the approach to analysis. At first, one might apply descriptive codes, summarizing what is contained in the interviews. It is rarely desirable to stop at this point, however, because coding is a tool to move from describing the data to interpreting the data. Suppose the researcher is pursuing some version of thematic analysis. In that case, it might be that the objects of coding are aspects of reported action, emotions, opinions, norms, relationships, routines, agreement/disagreement and change over time. A discourse analysis might instead code for different types of speech acts, tropes, linguistic or rhetorical devices. Multiple types of code might be generated within the same research project. What is important is that researchers are aware of the choices they are making in terms of what they are coding for. Moreover, through the process of refinement, the aim is to produce a set of discrete codes — in which codes are conceptually distinct, as opposed to overlapping. By using the same codes across the dataset, the researcher can capture commonalities across the interviews. This process of refinement involves relabelling codes and reorganizing how and where they are applied in the dataset.
From coding to analysis and writing
Data analysis is also an iterative process in which researchers move closer to and further away from the data. As they move away from the data, they synthesize their findings, thus honing and articulating their analytic insights. As they move closer to the data, they ground these insights in what is contained in the interviews. The link should not be broken between the data themselves and higher-order conceptual insights or claims being made. Researchers must be able to show evidence for their claims in the data. Figure 2 summarizes this iterative process and suggests the sorts of activities involved at each stage more concretely.
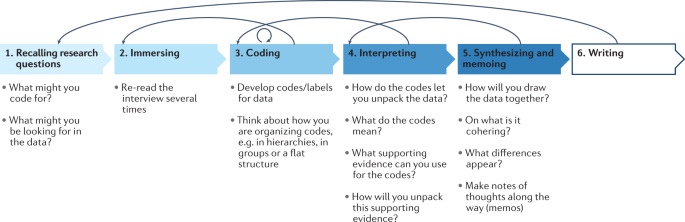
As well as going through steps 1 to 6 in order, the researcher will also go backwards and forwards between stages. Some stages will themselves be a forwards and backwards processing of coding and refining when working across different interview transcripts.
At the stage of synthesizing, there are some common quandaries. When dealing with a dataset consisting of multiple interviews, there will be salient and minority statements across different participants, or consensus or dissent on topics of interest to the researcher. A strength of qualitative interviews is that we can build in these nuances and variations across our data as opposed to aggregating them away. When exploring and reporting data, researchers should be asking how different findings are patterned and which interviews contain which codes, themes or tropes. Researchers should think about how these variations fit within the longer flow of individual interviews and what these variations tell them about the nature of their substantive research interests.
A further consideration is how to approach analysis within and across interview data. Researchers may look at one individual code, to examine the forms it takes across different participants and what they might be able to summarize about this code in the round. Alternatively, they might look at how a code or set of codes pattern across the account of one participant, to understand the code(s) in a more contextualized way. Further analysis might be done according to different sampling characteristics, where researchers group together interviews based on certain demographic characteristics and explore these together.
When it comes to writing up and presenting interview data, key considerations tend to rest on what is often termed transparency. When presenting the findings of an interview-based study, the reader should be able to understand and trace what the stated findings are based upon. This process typically involves describing the analytic process, how key decisions were made and presenting direct excerpts from the data. It is important to account for how the interview was set up and to consider the active part that the researcher has played in generating the data 32 . Quotes from interviews should not be thought of as merely embellishing or adding interest to a final research output. Rather, quotes serve the important function of connecting the reader directly to the underlying data. Quotes, therefore, should be chosen because they provide the reader with the most apt insight into what is being discussed. It is good practice to report not just on what participants said, but also on the questions that were asked to elicit the responses.
Researchers have increasingly used specialist qualitative data analysis software to organize and analyse their interview data, such as NVivo or ATLAS.ti. It is important to remember that such software is a tool for, rather than an approach or technique of, analysis. That said, software also creates a wide range of possibilities in terms of what can be done with the data. As researchers, we should reflect on how the range of possibilities of a given software package might be shaping our analytical choices and whether these are choices that we do indeed want to make.
Applications
This section reviews how and why in-depth interviews have been used by researchers studying gender, education and inequality, nationalism and ethnicity and the welfare state. Although interviews can be employed as a method of data collection in just about any social science topic, the applications below speak directly to the authors’ expertise and cutting-edge areas of research.
When it comes to the broad study of gender, in-depth interviews have been invaluable in shaping our understanding of how gender functions in everyday life. In a study of the US hedge fund industry (an industry dominated by white men), Tobias Neely was interested in understanding the factors that enable white men to prosper in the industry 33 . The study comprised interviews with 45 hedge fund workers and oversampled women of all races and men of colour to capture a range of experiences and beliefs. Tobias Neely found that practices of hiring, grooming and seeding are key to maintaining white men’s dominance in the industry. In terms of hiring, the interviews clarified that white men in charge typically preferred to hire people like themselves, usually from their extended networks. When women were hired, they were usually hired to less lucrative positions. In terms of grooming, Tobias Neely identifies how older and more senior men in the industry who have power and status will select one or several younger men as their protégés, to include in their own elite networks. Finally, in terms of her concept of seeding, Tobias Neely describes how older men who are hedge fund managers provide the seed money (often in the hundreds of millions of dollars) for a hedge fund to men, often their own sons (but not their daughters). These interviews provided an in-depth look into gendered and racialized mechanisms that allow white men to flourish in this industry.
Research by Rao draws on dozens of interviews with men and women who had lost their jobs, some of the participants’ spouses and follow-up interviews with about half the sample approximately 6 months after the initial interview 34 . Rao used interviews to understand the gendered experience and understanding of unemployment. Through these interviews, she found that the very process of losing their jobs meant different things for men and women. Women often saw job loss as being a personal indictment of their professional capabilities. The women interviewed often referenced how years of devaluation in the workplace coloured their interpretation of their job loss. Men, by contrast, were also saddened by their job loss, but they saw it as part and parcel of a weak economy rather than a personal failing. How these varied interpretations occurred was tied to men’s and women’s very different experiences in the workplace. Further, through her analysis of these interviews, Rao also showed how these gendered interpretations had implications for the kinds of jobs men and women sought to pursue after job loss. Whereas men remained tied to participating in full-time paid work, job loss appeared to be a catalyst pushing some of the women to re-evaluate their ties to the labour force.
In a study of workers in the tech industry, Hart used interviews to explain how individuals respond to unwanted and ambiguously sexual interactions 35 . Here, the researcher used interviews to allow participants to describe how these interactions made them feel and act and the logics of how they interpreted, classified and made sense of them 35 . Through her analysis of these interviews, Hart showed that participants engaged in a process she termed “trajectory guarding”, whereby they sought to monitor unwanted and ambiguously sexual interactions to avoid them from escalating. Yet, as Hart’s analysis proficiently demonstrates, these very strategies — which protect these workers sexually — also undermined their workplace advancement.
Drawing on interviews, these studies have helped us to understand better how gendered mechanisms, gendered interpretations and gendered interactions foster gender inequality when it comes to paid work. Methodologically, these studies illuminate the power of interviews to reveal important aspects of social life.
Nationalism and ethnicity
Traditionally, nationalism has been studied from a top-down perspective, through the lens of the state or using historical methods; in other words, in-depth interviews have not been a common way of collecting data to study nationalism. The methodological turn towards everyday nationalism has encouraged more scholars to go to the field and use interviews (and ethnography) to understand nationalism from the bottom up: how people talk about, give meaning, understand, navigate and contest their relation to nation, national identification and nationalism 36 , 37 , 38 , 39 . This turn has also addressed the gap left by those studying national and ethnic identification via quantitative methods, such as surveys.
Surveys can enumerate how individuals ascribe to categorical forms of identification 40 . However, interviews can question the usefulness of such categories and ask whether these categories are reflected, or resisted, by participants in terms of the meanings they give to identification 41 , 42 . Categories often pitch identification as a mutually exclusive choice; but identification might be more complex than such categories allow. For example, some might hybridize these categories or see themselves as moving between and across categories 43 . Hearing how people talk about themselves and their relation to nations, states and ethnicities, therefore, contributes substantially to the study of nationalism and national and ethnic forms of identification.
One particular approach to studying these topics, whether via everyday nationalism or alternatives, is that of using interviews to capture both articulations and narratives of identification, relations to nationalism and the boundaries people construct. For example, interviews can be used to gather self–other narratives by studying how individuals construct I–we–them boundaries 44 , including how participants talk about themselves, who participants include in their various ‘we’ groupings and which and how participants create ‘them’ groupings of others, inserting boundaries between ‘I/we’ and ‘them’. Overall, interviews hold great potential for listening to participants and understanding the nuances of identification and the construction of boundaries from their point of view.
Education and inequality
Scholars of social stratification have long noted that the school system often reproduces existing social inequalities. Carter explains that all schools have both material and sociocultural resources 45 . When children from different backgrounds attend schools with different material resources, their educational and occupational outcomes are likely to vary. Such material resources are relatively easy to measure. They are operationalized as teacher-to-student ratios, access to computers and textbooks and the physical infrastructure of classrooms and playgrounds.
Drawing on Bourdieusian theory 46 , Carter conceptualizes the sociocultural context as the norms, values and dispositions privileged within a social space 45 . Scholars have drawn on interviews with students and teachers (as well as ethnographic observations) to show how schools confer advantages on students from middle-class families, for example, by rewarding their help-seeking behaviours 47 . Focusing on race, researchers have revealed how schools can remain socioculturally white even as they enrol a racially diverse student population. In such contexts, for example, teachers often misrecognize the aesthetic choices made by students of colour, wrongly inferring that these students’ tastes in clothing and music reflect negative orientations to schooling 48 , 49 , 50 . These assessments can result in disparate forms of discipline and may ultimately shape educators’ assessments of students’ academic potential 51 .
Further, teachers and administrators tend to view the appropriate relationship between home and school in ways that resonate with white middle-class parents 52 . These parents are then able to advocate effectively for their children in ways that non-white parents are not 53 . In-depth interviews are particularly good at tapping into these understandings, revealing the mechanisms that confer privilege on certain groups of students and thereby reproduce inequality.
In addition, interviews can shed light on the unequal experiences that young people have within educational institutions, as the views of dominant groups are affirmed while those from disadvantaged backgrounds are delegitimized. For example, Teeger’s interviews with South African high schoolers showed how — because racially charged incidents are often framed as jokes in the broader school culture — Black students often feel compelled to ignore and keep silent about the racism they experience 54 . Interviews revealed that Black students who objected to these supposed jokes were coded by other students as serious or angry. In trying to avoid such labels, these students found themselves unable to challenge the racism they experienced. Interviews give us insight into these dynamics and help us see how young people understand and interpret the messages transmitted in schools — including those that speak to issues of inequality in their local school contexts as well as in society more broadly 24 , 55 .
The welfare state
In-depth interviews have also proved to be an important method for studying various aspects of the welfare state. By welfare state, we mean the social institutions relating to the economic and social wellbeing of a state’s citizens. Notably, using interviews has been useful to look at how policy design features are experienced and play out on the ground. Interviews have often been paired with large-scale surveys to produce mixed-methods study designs, therefore achieving both breadth and depth of insights.
In-depth interviews provide the opportunity to look behind policy assumptions or how policies are designed from the top down, to examine how these play out in the lives of those affected by the policies and whose experiences might otherwise be obscured or ignored. For example, the Welfare Conditionality project used interviews to critique the assumptions that conditionality (such as, the withdrawal of social security benefits if recipients did not perform or meet certain criteria) improved employment outcomes and instead showed that conditionality was harmful to mental health, living standards and had many other negative consequences 56 . Meanwhile, combining datasets from two small-scale interview studies with recipients allowed Summers and Young to critique assumptions around the simplicity that underpinned the design of Universal Credit in 2020, for example, showing that the apparently simple monthly payment design instead burdened recipients with additional money management decisions and responsibilities 57 .
Similarly, the Welfare at a (Social) Distance project used a mixed-methods approach in a large-scale study that combined national surveys with case studies and in-depth interviews to investigate the experience of claiming social security benefits during the COVID-19 pandemic. The interviews allowed researchers to understand in detail any issues experienced by recipients of benefits, such as delays in the process of claiming, managing on a very tight budget and navigating stigma and claiming 58 .
These applications demonstrate the multi-faceted topics and questions for which interviews can be a relevant method for data collection. These applications highlight not only the relevance of interviews, but also emphasize the key added value of interviews, which might be missed by other methods (surveys, in particular). Interviews can expose and question what is taken for granted and directly engage with communities and participants that might otherwise be ignored, obscured or marginalized.
Reproducibility and data deposition
There is a robust, ongoing debate about reproducibility in qualitative research, including interview studies. In some research paradigms, reproducibility can be a way of interrogating the rigour and robustness of research claims, by seeing whether these hold up when the research process is repeated. Some scholars have suggested that although reproducibility may be challenging, researchers can facilitate it by naming the place where the research was conducted, naming participants, sharing interview and fieldwork transcripts (anonymized and de-identified in cases where researchers are not naming people or places) and employing fact-checkers for accuracy 11 , 59 , 60 .
In addition to the ethical concerns of whether de-anonymization is ever feasible or desirable, it is also important to address whether the replicability of interview studies is meaningful. For example, the flexibility of interviews allows for the unexpected and the unforeseen to be incorporated into the scope of the research 61 . However, this flexibility means that we cannot expect reproducibility in the conventional sense, given that different researchers will elicit different types of data from participants. Sharing interview transcripts with other researchers, for instance, downplays the contextual nature of an interview.
Drawing on Bauer and Gaskell, we propose several measures to enhance rigour in qualitative research: transparency, grounding interpretations and aiming for theoretical transferability and significance 62 .
Researchers should be transparent when describing their methodological choices. Transparency means documenting who was interviewed, where and when (without requiring de-anonymization, for example, by documenting their characteristics), as well as the questions they were asked. It means carefully considering who was left out of the interviews and what that could mean for the researcher’s findings. It also means carefully considering who the researcher is and how their identity shaped the research process (integrating and articulating reflexivity into whatever is written up).
Second, researchers should ground their interpretations in the data. Grounding means presenting the evidence upon which the interpretation relies. Quotes and extracts should be extensive enough to allow the reader to evaluate whether the researcher’s interpretations are grounded in the data. At each step, researchers should carefully compare their own explanations and interpretations with alternative explanations. Doing so systematically and frequently allows researchers to become more confident in their claims. Here, researchers should justify the link between data and analysis by using quotes to justify and demonstrate the analytical point, while making sure the analytical point offers an interpretation of quotes (Box 4 ).
An important step in considering alternative explanations is to seek out disconfirming evidence 4 , 63 . This involves looking for instances where participants deviate from what the majority are saying and thus bring into question the theory (or explanation) that the researcher is developing. Careful analysis of such examples can often demonstrate the salience and meaning of what appears to be the norm (see Table 2 for examples) 54 . Considering alternative explanations and paying attention to disconfirming evidence allows the researcher to refine their own theories in respect of the data.
Finally, researchers should aim for theoretical transferability and significance in their discussions of findings. One way to think about this is to imagine someone who is not interested in the empirical study. Articulating theoretical transferability and significance usually takes the form of broadening out from the specific findings to consider explicitly how the research has refined or altered prior theoretical approaches. This process also means considering under what other conditions, aside from those of the study, the researcher thinks their theoretical revision would be supported by and why. Importantly, it also includes thinking about the limitations of one’s own approach and where the theoretical implications of the study might not hold.
Box 4 An example of grounding interpretations in data (from Rao 34 )
In an article explaining how unemployed men frame their job loss as a pervasive experience, Rao writes the following: “Unemployed men in this study understood unemployment to be an expected aspect of paid work in the contemporary United States. Robert, a white unemployed communications professional, compared the economic landscape after the Great Recession with the tragic events of September 11, 2001:
Part of your post-9/11 world was knowing people that died as a result of terrorism. The same thing is true with the [Great] Recession, right? … After the Recession you know somebody who was unemployed … People that really should be working.
The pervasiveness of unemployment rendered it normal, as Robert indicates.”
Here, the link between the quote presented and the analytical point Rao is making is clear: the analytical point is grounded in a quote and an interpretation of the quote is offered 34 .
Limitations and optimizations
When deciding which research method to use, the key question is whether the method provides a good fit for the research questions posed. In other words, researchers should consider whether interviews will allow them to successfully access the social phenomena necessary to answer their question(s) and whether the interviews will do so more effectively than other methods. Table 3 summarizes the major strengths and limitations of interviews. However, the accompanying text below is organized around some key issues, where relative strengths and weaknesses are presented alongside each other, the aim being that readers should think about how these can be balanced and optimized in relation to their own research.
Breadth versus depth of insight
Achieving an overall breadth of insight, in a statistically representative sense, is not something that is possible or indeed desirable when conducting in-depth interviews. Instead, the strength of conducting interviews lies in their ability to generate various sorts of depth of insight. The experiences or views of participants that can be accessed by conducting interviews help us to understand participants’ subjective realities. The challenge, therefore, is for researchers to be clear about why depth of insight is the focus and what we should aim to glean from these types of insight.
Naturalistic or artificial interviews
Interviews make use of a form of interaction with which people are familiar 64 . By replicating a naturalistic form of interaction as a tool to gather social science data, researchers can capitalize on people’s familiarity and expectations of what happens in a conversation. This familiarity can also be a challenge, as people come to the interview with preconceived ideas about what this conversation might be for or about. People may draw on experiences of other similar conversations when taking part in a research interview (for example, job interviews, therapy sessions, confessional conversations, chats with friends). Researchers should be aware of such potential overlaps and think through their implications both in how the aims and purposes of the research interview are communicated to participants and in how interview data are interpreted.
Further, some argue that a limitation of interviews is that they are an artificial form of data collection. By taking people out of their daily lives and asking them to stand back and pass comment, we are creating a distance that makes it difficult to use such data to say something meaningful about people’s actions, experiences and views. Other approaches, such as ethnography, might be more suitable for tapping into what people actually do, as opposed to what they say they do 65 .
Dynamism and replicability
Interviews following a semi-structured format offer flexibility both to the researcher and the participant. As the conversation develops, the interlocutors can explore the topics raised in much more detail, if desired, or pass over ones that are not relevant. This flexibility allows for the unexpected and the unforeseen to be incorporated into the scope of the research.
However, this flexibility has a related challenge of replicability. Interviews cannot be reproduced because they are contingent upon the interaction between the researcher and the participant in that given moment of interaction. In some research paradigms, replicability can be a way of interrogating the robustness of research claims, by seeing whether they hold when they are repeated. This is not a useful framework to bring to in-depth interviews and instead quality criteria (such as transparency) tend to be employed as criteria of rigour.
Accessing the private and personal
Interviews have been recognized for their strength in accessing private, personal issues, which participants may feel more comfortable talking about in a one-to-one conversation. Furthermore, interviews are likely to take a more personable form with their extended questions and answers, perhaps making a participant feel more at ease when discussing sensitive topics in such a context. There is a similar, but separate, argument made about accessing what are sometimes referred to as vulnerable groups, who may be difficult to make contact with using other research methods.
There is an associated challenge of anonymity. There can be types of in-depth interview that make it particularly challenging to protect the identities of participants, such as interviewing within a small community, or multiple members of the same household. The challenge to ensure anonymity in such contexts is even more important and difficult when the topic of research is of a sensitive nature or participants are vulnerable.
Increasingly, researchers are collaborating in large-scale interview-based studies and integrating interviews into broader mixed-methods designs. At the same time, interviews can be seen as an old-fashioned (and perhaps outdated) mode of data collection. We review these debates and discussions and point to innovations in interview-based studies. These include the shift from face-to-face interviews to the use of online platforms, as well as integrating and adapting interviews towards more inclusive methodologies.
Collaborating and mixing
Qualitative researchers have long worked alone 66 . Increasingly, however, researchers are collaborating with others for reasons such as efficiency, institutional incentives (for example, funding for collaborative research) and a desire to pool expertise (for example, studying similar phenomena in different contexts 67 or via different methods). Collaboration can occur across disciplines and methods, cases and contexts and between industry/business, practitioners and researchers. In many settings and contexts, collaboration has become an imperative 68 .
Cheek notes how collaboration provides both advantages and disadvantages 68 . For example, collaboration can be advantageous, saving time and building on the divergent knowledge, skills and resources of different researchers. Scholars with different theoretical or case-based knowledge (or contacts) can work together to build research that is comparative and/or more than the sum of its parts. But such endeavours also carry with them practical and political challenges in terms of how resources might actually be pooled, shared or accounted for. When undertaking such projects, as Morse notes, it is worth thinking about the nature of the collaboration and being explicit about such a choice, its advantages and its disadvantages 66 .
A further tension, but also a motivation for collaboration, stems from integrating interviews as a method in a mixed-methods project, whether with other qualitative researchers (to combine with, for example, focus groups, document analysis or ethnography) or with quantitative researchers (to combine with, for example, surveys, social media analysis or big data analysis). Cheek and Morse both note the pitfalls of collaboration with quantitative researchers: that quality of research may be sacrificed, qualitative interpretations watered down or not taken seriously, or tensions experienced over the pace and different assumptions that come with different methods and approaches of research 66 , 68 .
At the same time, there can be real benefits of such mixed-methods collaboration, such as reaching different and more diverse audiences or testing assumptions and theories between research components in the same project (for example, testing insights from prior quantitative research via interviews, or vice versa), as long as the skillsets of collaborators are seen as equally beneficial to the project. Cheek provides a set of questions that, as a starting point, can be useful for guiding collaboration, whether mixed methods or otherwise. First, Cheek advises asking all collaborators about their assumptions and understandings concerning collaboration. Second, Cheek recommends discussing what each perspective highlights and focuses on (and conversely ignores or sidelines) 68 .
A different way to engage with the idea of collaboration and mixed methods research is by fostering greater collaboration between researchers in the Global South and Global North, thus reversing trends of researchers from the Global North extracting knowledge from the Global South 69 . Such forms of collaboration also align with interview innovations, discussed below, that seek to transform traditional interview approaches into more participatory and inclusive (as part of participatory methodologies).
Digital innovations and challenges
The ongoing COVID-19 pandemic has centred the question of technology within interview-based fieldwork. Although conducting synchronous oral interviews online — for example, via Zoom, Skype or other such platforms — has been a method used by a small constituency of researchers for many years, it became (and remains) a necessity for many researchers wanting to continue or start interview-based projects while COVID-19 prevents face-to-face data collection.
In the past, online interviews were often framed as an inferior form of data collection for not providing the kinds of (often necessary) insights and forms of immersion face-to-face interviews allow 70 , 71 . Online interviews do tend to be more decontextualized than interviews conducted face-to-face 72 . For example, it is harder to recognize, engage with and respond to non-verbal cues 71 . At the same time, they broaden participation to those who might not have been able to access or travel to sites where interviews would have been conducted otherwise, for example people with disabilities. Online interviews also offer more flexibility in terms of scheduling and time requirements. For example, they provide more flexibility around precarious employment or caring responsibilities without having to travel and be away from home. In addition, online interviews might also reduce discomfort between researchers and participants, compared with face-to-face interviews, enabling more discussion of sensitive material 71 . They can also provide participants with more control, enabling them to turn on and off the microphone and video as they choose, for example, to provide more time to reflect and disconnect if they so wish 72 .
That said, online interviews can also introduce new biases based on access to technology 72 . For example, in the Global South, there are often urban/rural and gender gaps between who has access to mobile phones and who does not, meaning that some population groups might be overlooked unless researchers sample mindfully 71 . There are also important ethical considerations when deciding between online and face-to-face interviews. Online interviews might seem to imply lower ethical risks than face-to-face interviews (for example, they lower the chances of identification of participants or researchers), but they also offer more barriers to building trust between researchers and participants 72 . Interacting only online with participants might not provide the information needed to assess risk, for example, participants’ access to a private space to speak 71 . Just because online interviews might be more likely to be conducted in private spaces does not mean that private spaces are safe, for example, for victims of domestic violence. Finally, online interviews prompt further questions about decolonizing research and engaging with participants if research is conducted from afar 72 , such as how to include participants meaningfully and challenge dominant assumptions while doing so remotely.
A further digital innovation, modulating how researchers conduct interviews and the kinds of data collected and analysed, stems from the use and integration of (new) technology, such as WhatsApp text or voice notes to conduct synchronous or asynchronous oral or written interviews 73 . Such methods can provide more privacy, comfort and control to participants and make recruitment easier, allowing participants to share what they want when they want to, using technology that already forms a part of their daily lives, especially for young people 74 , 75 . Such technology is also emerging in other qualitative methods, such as focus groups, with similar arguments around greater inclusivity versus traditional offline modes. Here, the digital challenge might be higher for researchers than for participants if they are less used to such technology 75 . And while there might be concerns about the richness, depth and quality of written messages as a form of interview data, Gibson reports that the reams of transcripts that resulted from a study using written messaging were dense with meaning to be analysed 75 .
Like with online and face-to-face interviews, it is important also to consider the ethical questions and challenges of using such technology, from gaining consent to ensuring participant safety and attending to their distress, without cues, like crying, that might be more obvious in a face-to-face setting 75 , 76 . Attention to the platform used for such interviews is also important and researchers should be attuned to the local and national context. For example, in China, many platforms are neither legal nor available 76 . There, more popular platforms — like WeChat — can be highly monitored by the government, posing potential risks to participants depending on the topic of the interview. Ultimately, researchers should consider trade-offs between online and offline interview modalities, being attentive to the social context and power dynamics involved.
The next 5–10 years
Continuing to integrate (ethically) this technology will be among the major persisting developments in interview-based research, whether to offer more flexibility to researchers or participants, or to diversify who can participate and on what terms.
Pushing the idea of inclusion even further is the potential for integrating interview-based studies within participatory methods, which are also innovating via integrating technology. There is no hard and fast line between researchers using in-depth interviews and participatory methods; many who employ participatory methods will use interviews at the beginning, middle or end phases of a research project to capture insights, perspectives and reflections from participants 77 , 78 . Participatory methods emphasize the need to resist existing power and knowledge structures. They broaden who has the right and ability to contribute to academic knowledge by including and incorporating participants not only as subjects of data collection, but as crucial voices in research design and data analysis 77 . Participatory methods also seek to facilitate local change and to produce research materials, whether for academic or non-academic audiences, including films and documentaries, in collaboration with participants.
In responding to the challenges of COVID-19, capturing the fraught situation wrought by the pandemic and the momentum to integrate technology, participatory researchers have sought to continue data collection from afar. For example, Marzi has adapted an existing project to co-produce participatory videos, via participants’ smartphones in Medellin, Colombia, alongside regular check-in conversations/meetings/interviews with participants 79 . Integrating participatory methods into interview studies offers a route by which researchers can respond to the challenge of diversifying knowledge, challenging assumptions and power hierarchies and creating more inclusive and collaborative partnerships between participants and researchers in the Global North and South.
Brinkmann, S. & Kvale, S. Doing Interviews Vol. 2 (Sage, 2018). This book offers a good general introduction to the practice and design of interview-based studies.
Silverman, D. A Very Short, Fairly Interesting And Reasonably Cheap Book About Qualitative Research (Sage, 2017).
Yin, R. K. Case Study Research And Applications: Design And Methods (Sage, 2018).
Small, M. L. How many cases do I need?’ On science and the logic of case selection in field-based research. Ethnography 10 , 5–38 (2009). This article convincingly demonstrates how the logic of qualitative research differs from quantitative research and its goal of representativeness.
Google Scholar
Gerson, K. & Damaske, S. The Science and Art of Interviewing (Oxford Univ. Press, 2020).
Glaser, B. G. & Strauss, A. L. The Discovery Of Grounded Theory: Strategies For Qualitative Research (Aldine, 1967).
Braun, V. & Clarke, V. To saturate or not to saturate? Questioning data saturation as a useful concept for thematic analysis and sample-size rationales. Qual. Res. Sport Exerc. Health 13 , 201–216 (2021).
Guest, G., Bunce, A. & Johnson, L. How many interviews are enough? An experiment with data saturation and variability. Field Methods 18 , 59–82 (2006).
Vasileiou, K., Barnett, J., Thorpe, S. & Young, T. Characterising and justifying sample size sufficiency in interview-based studies: systematic analysis of qualitative health research over a 15-year period. BMC Med. Res. Methodol. 18 , 148 (2018).
Silverman, D. How was it for you? The Interview Society and the irresistible rise of the (poorly analyzed) interview. Qual. Res. 17 , 144–158 (2017).
Jerolmack, C. & Murphy, A. The ethical dilemmas and social scientific tradeoffs of masking in ethnography. Sociol. Methods Res. 48 , 801–827 (2019).
MathSciNet Google Scholar
Reyes, V. Ethnographic toolkit: strategic positionality and researchers’ visible and invisible tools in field research. Ethnography 21 , 220–240 (2020).
Guillemin, M. & Gillam, L. Ethics, reflexivity and “ethically important moments” in research. Qual. Inq. 10 , 261–280 (2004).
Summers, K. For the greater good? Ethical reflections on interviewing the ‘rich’ and ‘poor’ in qualitative research. Int. J. Soc. Res. Methodol. 23 , 593–602 (2020). This article argues that, in qualitative interview research, a clearer distinction needs to be drawn between ethical commitments to individual research participants and the group(s) to which they belong, a distinction that is often elided in existing ethics guidelines.
Yusupova, G. Exploring sensitive topics in an authoritarian context: an insider perspective. Soc. Sci. Q. 100 , 1459–1478 (2019).
Hemming, J. in Surviving Field Research: Working In Violent And Difficult Situations 21–37 (Routledge, 2009).
Murphy, E. & Dingwall, R. Informed consent, anticipatory regulation and ethnographic practice. Soc. Sci. Med. 65 , 2223–2234 (2007).
Kostovicova, D. & Knott, E. Harm, change and unpredictability: the ethics of interviews in conflict research. Qual. Res. 22 , 56–73 (2022). This article highlights how interviews need to be considered as ethically unpredictable moments where engaging with change among participants can itself be ethical.
Andersson, R. Illegality, Inc.: Clandestine Migration And The Business Of Bordering Europe (Univ. California Press, 2014).
Ellis, R. What do we mean by a “hard-to-reach” population? Legitimacy versus precarity as barriers to access. Sociol. Methods Res. https://doi.org/10.1177/0049124121995536 (2021).
Article Google Scholar
Braun, V. & Clarke, V. Thematic Analysis: A Practical Guide (Sage, 2022).
Alejandro, A. & Knott, E. How to pay attention to the words we use: the reflexive review as a method for linguistic reflexivity. Int. Stud. Rev. https://doi.org/10.1093/isr/viac025 (2022).
Alejandro, A., Laurence, M. & Maertens, L. in International Organisations and Research Methods: An Introduction (eds Badache, F., Kimber, L. R. & Maertens, L.) (Michigan Univ. Press, in the press).
Teeger, C. “Both sides of the story” history education in post-apartheid South Africa. Am. Sociol. Rev. 80 , 1175–1200 (2015).
Crotty, M. The Foundations Of Social Research: Meaning And Perspective In The Research Process (Routledge, 2020).
Potter, J. & Hepburn, A. Qualitative interviews in psychology: problems and possibilities. Qual. Res. Psychol. 2 , 281–307 (2005).
Taylor, S. What is Discourse Analysis? (Bloomsbury Publishing, 2013).
Riessman, C. K. Narrative Analysis (Sage, 1993).
Corbin, J. M. & Strauss, A. Grounded theory research: Procedures, canons and evaluative criteria. Qual. Sociol. 13 , 3–21 (1990).
Timmermans, S. & Tavory, I. Theory construction in qualitative research: from grounded theory to abductive analysis. Sociol. Theory 30 , 167–186 (2012).
Fereday, J. & Muir-Cochrane, E. Demonstrating rigor using thematic analysis: a hybrid approach of inductive and deductive coding and theme development. Int. J. Qual. Meth. 5 , 80–92 (2006).
Potter, J. & Hepburn, A. Eight challenges for interview researchers. Handb. Interview Res. 2 , 541–570 (2012).
Tobias Neely, M. Fit to be king: how patrimonialism on Wall Street leads to inequality. Socioecon. Rev. 16 , 365–385 (2018).
Rao, A. H. Gendered interpretations of job loss and subsequent professional pathways. Gend. Soc. 35 , 884–909 (2021). This article used interview data from unemployed men and women to illuminate how job loss becomes a pivotal moment shaping men’s and women’s orientation to paid work, especially in terms of curtailing women’s participation in paid work.
Hart, C. G. Trajectory guarding: managing unwanted, ambiguously sexual interactions at work. Am. Sociol. Rev. 86 , 256–278 (2021).
Goode, J. P. & Stroup, D. R. Everyday nationalism: constructivism for the masses. Soc. Sci. Q. 96 , 717–739 (2015).
Antonsich, M. The ‘everyday’ of banal nationalism — ordinary people’s views on Italy and Italian. Polit. Geogr. 54 , 32–42 (2016).
Fox, J. E. & Miller-Idriss, C. Everyday nationhood. Ethnicities 8 , 536–563 (2008).
Yusupova, G. Cultural nationalism and everyday resistance in an illiberal nationalising state: ethnic minority nationalism in Russia. Nations National. 24 , 624–647 (2018).
Kiely, R., Bechhofer, F. & McCrone, D. Birth, blood and belonging: identity claims in post-devolution Scotland. Sociol. Rev. 53 , 150–171 (2005).
Brubaker, R. & Cooper, F. Beyond ‘identity’. Theory Soc. 29 , 1–47 (2000).
Brubaker, R. Ethnicity Without Groups (Harvard Univ. Press, 2004).
Knott, E. Kin Majorities: Identity And Citizenship In Crimea And Moldova From The Bottom-Up (McGill Univ. Press, 2022).
Bucher, B. & Jasper, U. Revisiting ‘identity’ in international relations: from identity as substance to identifications in action. Eur. J. Int. Relat. 23 , 391–415 (2016).
Carter, P. L. Stubborn Roots: Race, Culture And Inequality In US And South African Schools (Oxford Univ. Press, 2012).
Bourdieu, P. in Cultural Theory: An Anthology Vol. 1, 81–93 (eds Szeman, I. & Kaposy, T.) (Wiley-Blackwell, 2011).
Calarco, J. M. Negotiating Opportunities: How The Middle Class Secures Advantages In School (Oxford Univ. Press, 2018).
Carter, P. L. Keepin’ It Real: School Success Beyond Black And White (Oxford Univ. Press, 2005).
Carter, P. L. ‘Black’ cultural capital, status positioning and schooling conflicts for low-income African American youth. Soc. Probl. 50 , 136–155 (2003).
Warikoo, N. K. The Diversity Bargain Balancing Acts: Youth Culture in the Global City (Univ. California Press, 2011).
Morris, E. W. “Tuck in that shirt!” Race, class, gender and discipline in an urban school. Sociol. Perspect. 48 , 25–48 (2005).
Lareau, A. Social class differences in family–school relationships: the importance of cultural capital. Sociol. Educ. 60 , 73–85 (1987).
Warikoo, N. Addressing emotional health while protecting status: Asian American and white parents in suburban America. Am. J. Sociol. 126 , 545–576 (2020).
Teeger, C. Ruptures in the rainbow nation: how desegregated South African schools deal with interpersonal and structural racism. Sociol. Educ. 88 , 226–243 (2015). This article leverages ‘ deviant ’ cases in an interview study with South African high schoolers to understand why the majority of participants were reluctant to code racially charged incidents at school as racist.
Ispa-Landa, S. & Conwell, J. “Once you go to a white school, you kind of adapt” black adolescents and the racial classification of schools. Sociol. Educ. 88 , 1–19 (2015).
Dwyer, P. J. Punitive and ineffective: benefit sanctions within social security. J. Soc. Secur. Law 25 , 142–157 (2018).
Summers, K. & Young, D. Universal simplicity? The alleged simplicity of Universal Credit from administrative and claimant perspectives. J. Poverty Soc. Justice 28 , 169–186 (2020).
Summers, K. et al. Claimants’ Experiences Of The Social Security System During The First Wave Of COVID-19 . https://www.distantwelfare.co.uk/winter-report (2021).
Desmond, M. Evicted: Poverty And Profit In The American City (Crown Books, 2016).
Reyes, V. Three models of transparency in ethnographic research: naming places, naming people and sharing data. Ethnography 19 , 204–226 (2018).
Robson, C. & McCartan, K. Real World Research (Wiley, 2016).
Bauer, M. W. & Gaskell, G. Qualitative Researching With Text, Image And Sound: A Practical Handbook (SAGE, 2000).
Lareau, A. Listening To People: A Practical Guide To Interviewing, Participant Observation, Data Analysis And Writing It All Up (Univ. Chicago Press, 2021).
Lincoln, Y. S. & Guba, E. G. Naturalistic Inquiry (Sage, 1985).
Jerolmack, C. & Khan, S. Talk is cheap. Sociol. Methods Res. 43 , 178–209 (2014).
Morse, J. M. Styles of collaboration in qualitative inquiry. Qual. Health Res. 18 , 3–4 (2008).
ADS Google Scholar
Lamont, M. et al. Getting Respect: Responding To Stigma And Discrimination In The United States, Brazil And Israel (Princeton Univ. Press, 2016).
Cheek, J. Researching collaboratively: implications for qualitative research and researchers. Qual. Health Res. 18 , 1599–1603 (2008).
Botha, L. Mixing methods as a process towards indigenous methodologies. Int. J. Soc. Res. Methodol. 14 , 313–325 (2011).
Howlett, M. Looking at the ‘field’ through a zoom lens: methodological reflections on conducting online research during a global pandemic. Qual. Res. https://doi.org/10.1177/1468794120985691 (2021).
Reñosa, M. D. C. et al. Selfie consents, remote rapport and Zoom debriefings: collecting qualitative data amid a pandemic in four resource-constrained settings. BMJ Glob. Health 6 , e004193 (2021).
Mwambari, D., Purdeková, A. & Bisoka, A. N. Covid-19 and research in conflict-affected contexts: distanced methods and the digitalisation of suffering. Qual. Res. https://doi.org/10.1177/1468794121999014 (2021).
Colom, A. Using WhatsApp for focus group discussions: ecological validity, inclusion and deliberation. Qual. Res. https://doi.org/10.1177/1468794120986074 (2021).
Kaufmann, K. & Peil, C. The mobile instant messaging interview (MIMI): using WhatsApp to enhance self-reporting and explore media usage in situ. Mob. Media Commun. 8 , 229–246 (2020).
Gibson, K. Bridging the digital divide: reflections on using WhatsApp instant messenger interviews in youth research. Qual. Res. Psychol. 19 , 611–631 (2020).
Lawrence, L. Conducting cross-cultural qualitative interviews with mainland Chinese participants during COVID: lessons from the field. Qual. Res. https://doi.org/10.1177/1468794120974157 (2020).
Ponzoni, E. Windows of understanding: broadening access to knowledge production through participatory action research. Qual. Res. 16 , 557–574 (2016).
Kong, T. S. Gay and grey: participatory action research in Hong Kong. Qual. Res. 18 , 257–272 (2018).
Marzi, S. Participatory video from a distance: co-producing knowledge during the COVID-19 pandemic using smartphones. Qual. Res. https://doi.org/10.1177/14687941211038171 (2021).
Kvale, S. & Brinkmann, S. InterViews: Learning The Craft Of Qualitative Research Interviewing (Sage, 2008).
Rao, A. H. The ideal job-seeker norm: unemployment and marital privileges in the professional middle-class. J. Marriage Fam. 83 , 1038–1057 (2021).
Rivera, L. A. Ivies, extracurriculars and exclusion: elite employers’ use of educational credentials. Res. Soc. Stratif. Mobil. 29 , 71–90 (2011).
Download references
Acknowledgements
The authors are grateful to the MY421 team and students for prompting how best to frame and communicate issues pertinent to in-depth interview studies.
Author information
Authors and affiliations.
Department of Methodology, London School of Economics, London, UK
Eleanor Knott, Aliya Hamid Rao, Kate Summers & Chana Teeger
You can also search for this author in PubMed Google Scholar
Contributions
The authors contributed equally to all aspects of the article.
Corresponding author
Correspondence to Eleanor Knott .
Ethics declarations
Competing interests.
The authors declare no competing interests.
Peer review
Peer review information.
Nature Reviews Methods Primers thanks Jonathan Potter and the other, anonymous, reviewer(s) for their contribution to the peer review of this work.
Additional information
Publisher’s note.
Springer Nature remains neutral with regard to jurisdictional claims in published maps and institutional affiliations.
A pre-written interview outline for a semi-structured interview that provides both a topic structure and the ability to adapt flexibly to the content and context of the interview and the interaction between the interviewer and participant. Others may refer to the topic guide as an interview protocol.
Here we refer to the participants that take part in the study as the sample. Other researchers may refer to the participants as a participant group or dataset.
This involves dividing a population into smaller groups based on particular characteristics, for example, age or gender, and then sampling randomly within each group.
A sampling method where the guiding logic when deciding who to recruit is to achieve the most relevant participants for the research topic, in terms of being rich in information or insights.
Researchers ask participants to introduce the researcher to others who meet the study’s inclusion criteria.
Similar to stratified sampling, but participants are not necessarily randomly selected. Instead, the researcher determines how many people from each category of participants should be recruited. Recruitment can happen via snowball or purposive sampling.
A method for developing, analysing and interpreting patterns across data by coding in order to develop themes.
An approach that interrogates the explicit, implicit and taken-for-granted dimensions of language as well as the contexts in which it is articulated to unpack its purposes and effects.
A form of transcription that simplifies what has been said by removing certain verbal and non-verbal details that add no further meaning, such as ‘ums and ahs’ and false starts.
The analytic framework, theoretical approach and often hypotheses, are developed prior to examining the data and then applied to the dataset.
The analytic framework and theoretical approach is developed from analysing the data.
An approach that combines deductive and inductive components to work recursively by going back and forth between data and existing theoretical frameworks (also described as an iterative approach). This approach is increasingly recognized not only as a more realistic but also more desirable third alternative to the more traditional inductive versus deductive binary choice.
A theoretical apparatus that emphasizes the role of cultural processes and capital in (intergenerational) social reproduction.
Rights and permissions
Springer Nature or its licensor holds exclusive rights to this article under a publishing agreement with the author(s) or other rightsholder(s); author self-archiving of the accepted manuscript version of this article is solely governed by the terms of such publishing agreement and applicable law.
Reprints and permissions
About this article
Cite this article.
Knott, E., Rao, A.H., Summers, K. et al. Interviews in the social sciences. Nat Rev Methods Primers 2 , 73 (2022). https://doi.org/10.1038/s43586-022-00150-6
Download citation
Accepted : 14 July 2022
Published : 15 September 2022
DOI : https://doi.org/10.1038/s43586-022-00150-6
Share this article
Anyone you share the following link with will be able to read this content:
Sorry, a shareable link is not currently available for this article.
Provided by the Springer Nature SharedIt content-sharing initiative
This article is cited by
What the textbooks don’t teach about the reality of running a digitally enabled health study: a phenomenological interview study.
- Meghan Bradway
- Elia Garbarron
- Eirik Årsand
BMC Digital Health (2024)
Development of a digital intervention for psychedelic preparation (DIPP)
- Rosalind G. McAlpine
- Matthew D. Sacchet
- Sunjeev K. Kamboj
Scientific Reports (2024)
‘Life Minus Illness = Recovery’: A Phenomenological Study About Experiences and Meanings of Recovery Among Individuals with Serious Mental Illness from Southern India
- Srishti Hegde
- Shalini Quadros
- Vinita A. Acharya
Community Mental Health Journal (2024)
Can dry rivers provide a good quality of life? Integrating beneficial and detrimental nature’s contributions to people over time
- Néstor Nicolás-Ruiz
- María Luisa Suárez
- Cristina Quintas-Soriano
Ambio (2024)
Is e-business breaking down barriers for Bangladesh’s young female entrepreneurs during the COVID-19 pandemic? A qualitative study
- Md. Fouad Hossain Sarker
- Sayed Farrukh Ahmed
- Md. Salman Sohel
SN Social Sciences (2024)
Quick links
- Explore articles by subject
- Guide to authors
- Editorial policies
Sign up for the Nature Briefing newsletter — what matters in science, free to your inbox daily.

Integrating open- and closed-ended questions on attitudes towards outgroups with different methods of text analysis
- Open access
- Published: 16 October 2023
- Volume 56 , pages 4802–4822, ( 2024 )
Cite this article
You have full access to this open access article
- Karolina Hansen ORCID: orcid.org/0000-0002-1556-4058 1 &
- Aleksandra Świderska ORCID: orcid.org/0000-0001-7252-4581 1
8916 Accesses
2 Citations
3 Altmetric
Explore all metrics
Researchers in behavioral sciences often use closed-ended questions, forcing participants to express even complex impressions or attitudes through a set of predetermined answers. Even if this has many advantages, people’s opinions can be much richer. We argue for assessing them using different methods, including open-ended questions. Manual coding of open-ended answers requires much effort, but automated tools help to analyze them more easily. In order to investigate how attitudes towards outgroups can be assessed and analyzed with different methods, we carried out two representative surveys in Poland. We asked closed- and open-ended questions about what Poland should do regarding the influx of refugees. While the attitudes measured with closed-ended questions were rather negative, those that emerged from open-ended answers were not only richer, but also more positive. Many themes that emerged in the manual coding were also identified in automated text analyses with Meaning Extraction Helper (MEH). Using Linguistic Inquiry and Word Count (LIWC) and Sentiment Analyzer from the Common Language Resources and Technology Infrastructure (CLARIN), we compared the difference between the studies in the emotional tone of the answers. Our research confirms the high usefulness of open-ended questions in surveys and shows how methods of textual data analysis help in understanding people’s attitudes towards outgroup members. Based on our methods comparison, researchers can choose a method or combine methods in a way that best fits their needs.
Similar content being viewed by others
How to Improve Coding for Open-Ended Survey Data: Lessons from the ANES
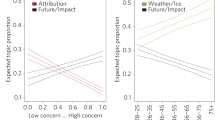
Explaining topic prevalence in answers to open-ended survey questions about climate change
A review of best practice recommendations for text analysis in r (and a user-friendly app).
Avoid common mistakes on your manuscript.
Should the UK leave EU? Should Poland let the refugees in? At first glance, a yes/no question in a poll would suffice to assess people’s opinions or predict the results of a referendum. However, asking about conditions under which the UK should leave the EU or Poland should accept refugees could allow for a better understanding of people’s attitudes. In the current research, we provide examples and guidance on what methods to use in the study of attitudes towards outgroups, focusing in particular on refugees as the example of an outgroup. We are comparing and integrating different methods as well as different approaches and tools for analysis of the written responses provided by participants: manual content analysis and three tools for automated text analysis (Meaning Extraction Helper, MEH; Linguistic Inquiry and Word Count, LIWC; and Sentiment Analyzer from the Common Language Resources and Technology Infrastructure, CLARIN).
Measuring attitudes towards outgroups
Most of the studies in behavioral sciences in general, and more specifically those measuring attitudes (to refugees, immigrants, climate change, and many others), use a top-down approach (e.g., Bansak et al., 2016 ; Esses et al., 2013 ; Wike et al., 2016 ). Within a top-down approach, researchers rely on existing theories that describe the relationships between specific variables to determine how these variables should be assessed (e.g., Forman et al., 2008 ). Such assessment is typically based on closed-ended questions, whereby researchers present participants with statements about a matter of interest and participants select an answer from predetermined options (Forman et al., 2008 ; Baburajan et al., 2020 ). In psychology, responses on a rating scale are especially common (Krosnick, 1999 ; Preston & Colman, 2000 ).
Relatively few studies let participants express what they think in their own words. This is possible by asking open-ended questions, which is characteristic of qualitative research (Forman et al., 2008 ). Responses to open-ended questions are then analyzed inductively within a bottom-up approach, that is, the researchers start with what is in the data to get to more abstract findings (Forman et al., 2008 ). The main benefit of using open-ended questions in research is that participants’ responses are freely constructed rather than suggested by the options provided by the researcher. This generates data that otherwise might not be possible to obtain from theory and the researchers' reasoning (e.g., Haddock & Zanna, 1998 ). Furthermore, previous research shows that by using open-ended questions researchers can better understand people's opinions (Geer, 1991 ).
Previous research suggests that the closed-ended format triggers a different response mode in participants than the open-ended format and participants draw on different memory or reasoning processes to answer closed- and open-ended questions (Connor Desai & Reimers, 2019 ; Schwarz et al., 1985 ). Anchoring effects and a stronger tendency to follow social norms in the case of closed-ended answers may explain the differences between answers to closed- and open-ended questions (Frew et al., 2003 ). In some studies, both formats gave broadly similar results, but open-ended responses were more detailed (e.g., Connor Desai & Reimers, 2019 ). In others, closed- and open-ended questions yielded different evaluations and different justifications for these evaluations (Frew et al., 2003 ). Prior results are therefore mixed.
We expect that when people’s opinions are ambivalent (as can occur with attitudes towards outgroups) or when the studied phenomena are complex and people’s opinions not well-formed, there might be differences between answers from closed- and open-ended questions. If, for instance, one wants to say “it depends,” then in an open-ended question they have a chance to do so, and in a closed-ended question they might adjust to the norm in their social context. The conclusions drawn from different types of questions could be more accurate and policies based on them might better reflect people’s attitudes than conclusions from only one type of questions. Furthermore, potential interventions or programs aimed at improving the attitudes towards outgroups might better target the right aspect of these attitudes and thus might be more effective.
Recently, the interest in open-ended questions has increased in different disciplines, mainly thanks to the development of tools for automated text analysis (e.g., Baburajan et al., 2022 ; Connor Desai & Reimers, 2019 ). There are a variety of tools for automated analysis of natural language, and there is also literature on these tools and examples of research using them. Other researchers have also written more general overviews about the approaches to and methods for analyzing language in behavioral sciences (e.g., Boyd & Schwartz, 2020 ; Rafaeli et al., 2019 ). However, we find that an empirical comparison of different ways of measuring attitudes towards outgroups and a comparison of the text analysis tools conducted on the same material is lacking.
Study context: Refugees in Poland
The goal of the current study was to compare different methods of assessing attitudes towards outgroup members and different methods of analyzing the acquired answers. To collect responses, we chose a socially important topic that evokes a variety of emotions in many countries: refugees. In 2018, according to the United Nations High Commissioner for Refugees (UNHCR), almost 70 million people worldwide were forcibly displaced, making it the highest number since the Second World War. At the moment of submitting this article (spring 2022), European countries are receiving Ukrainian refugees fleeing from their country after it was attacked by Russia. Reactions to the refugees and ideas of how they should be treated differ between countries. On the one hand, Germany opened its borders and already accepted about a million refugees from the Middle East in 2015 and 2016. On the other hand, Poland, Hungary, and the Czech Republic declared at that time that they would not accept any refugees. This strategy still holds today for the refugees from the Middle East who try to cross the border from Belarus into Poland, while the refugees from Ukraine arrive without major obstacles. In fact, in spring 2022 Poland has accepted about two million refugees from Ukraine just within a period of two weeks.
Before spring 2022, Poland had hosted only a handful of refugees. Direct contact with them was very rare. In 2017, 94% of Poles declared that they did not know any refugee personally (Stefaniak et al., 2017 ). Poles were rather welcoming to refugees in the spring of 2015, with 72% wanting to accept refugees in Poland. The same year, the refugees became a political topic in the parliamentary election campaign (Solska, 2017 ). These attitudes quickly shifted, and one year later, in the spring of 2016, only 33% of respondents wanted to accept refugees according to a Centre for Public Opinion Research (CBOS; https://www.cbos.pl/EN/about_us/about_us.php ) poll (CBOS, 2018 ), or 27% according to an Ariadna ( https://panelariadna.com/ ) national poll (Maison & Jasińska, 2017 ).
Although the above polls show overall negative attitudes towards refugees and although the Polish government has opposed admitting any to Poland until very recently, some studies suggested that the attitudes might be more complex and, if assessed in a different way, might not be as negative. A study that presented different profiles of refugees showed that “the vast majority of respondents in all surveyed countries neither categorically rejected nor categorically accepted all of their asylum-seeker profiles” (Bansak et al., 2016 , p. 221). Poland fell approximately in the middle, with 45% of respondents accepting refugees, and an acceptance rate ranging between 40 and 55% in the 15 studied countries.
A question arises as to why there are such different results for the same country in a similar period of time in different surveys. All of the surveys relied on closed-ended questions, but they were formulated in a slightly different way. As there were almost no refugees in Poland before spring 2022, the vast majority of Poles have never had contact with them (Stefaniak et al., 2017 ). Therefore, they might have been easily influenced by the way the questions were formulated. It might also be that Poles conditioned their support for refugees on the basis of their specific attributes (e.g., their religion or employability, as in Bansak et al., 2016 ), and this resulted in the variability of the answers.
Measuring attitudes towards refugees with open-ended questions
One exception to measuring attitudes towards refugees with closed-ended questions that utilized a bottom-up approach is a pilot study of refugee subgroups (Kotzur et al., 2019 ). In this pilot study, participants nominated meaningful categories of the subgroups of refugees (Kotzur et al., 2019 ). This allowed the researchers to investigate the stereotype content of a range of subgroups as identified by the participants themselves.
Another example of a study of attitudes towards refugees that used open-ended questions is an Australian study that asked the participants about their feelings, thoughts, and past experiences in relation to asylum seekers. Then, the participants quantitatively rated their own previously given open-ended answers on a continuum from negative to positive (Croucamp et al., 2017 ). The questionnaire did not contain separate closed-ended questions, so the researchers did not compare different ways of asking about attitudes towards refugees. However, as the authors noted, the “inclusion of a selection of participant-generated items allows insight into how the attitude processes emerge” (Croucamp et al., 2017 , p. 244).
In another Australian study, the questionnaire included open-ended questions about the respondents’ attitudes towards refugees in Port Augusta (Klocker, 2004 ). The responses were manually coded into categories. As the author pointed out, an advantage of the open-ended question was that it provided “respondents with the opportunity to frame the asylum debate in their own terms” (Klocker, 2004 , p. 4). In this case, the closed- and open-ended questions showed a similarly negative image of asylum seekers, but the open-ended questions allowed for a better understanding of the content of this image and the reasoning behind it.
The current research
As the aforementioned results show, letting the respondents state their opinion in their own words gives additional depth to the results. In the current research, we contrasted different data collection methods to better understand the complexity of attitudes towards refugees that may not be seen using only one of the methods. Analyzing textual data manually is time-consuming, and a large sample size understandingly can become a problem. Automated tools can help researchers with text analysis, but in order to rely on these tools, it is important to know how they compare to manual coding and to understand their advantages and limitations. The overarching aim of the current research was to aid this understanding.
In our approach, we combined the breadth and depth of information. As to breadth, we conducted two surveys on relatively large samples (ca. 250–300 participants in each) that were representative of the Polish population in terms of sex, age, and place of residence. We conducted Study 2 one year after Study 1 in order to analyze a time trend in the answers. As to depth, we asked participants to respond in their own words to the question What strategy should Poland adopt concerning refugees who want to come to Poland? Consequently, we acquired an extensive set of opinions that were self-formulated by participants. One way of analyzing such data is to do it manually, defining themes in a bottom-up or a top-down approach. For theme formulation, we used the bottom-up thematic coding done by two independent coders in each study. Furthermore, we tested various computerized methods of analyzing textual data. The current comparison of these methods is an empirical test of different approaches (content analysis, sentiment analysis) and programs (MEH, LIWC, Sentiment Analyzer) and is aimed at helping researchers in considering which approach(es) and tool(s) to choose.
Overall, by using closed- and open-ended responses and different methods of analysis, we show what could happen when seeing the results from only one angle and using only one of all the methods we used. Later on, we discuss how one could integrate the results of all methods, but we do not suggest that all methods should be used at the same time. We compare them, discuss the differences, and recommend using more than one.
In the current research, we used a convergent mixed-method research design with data transformation (Creswell et al., 2003 ; Fetters et al., 2013 ). Integration of the qualitative and quantitative data took place at the data collection stage, during the analysis phase, and during the interpretation of the results (Fig. 1 ). As we wanted to compare different methods of analysis of the same textual material, we also transformed the data from qualitative to quantitative form. While such transformations have been discussed in the literature (e.g., Caracelli & Greene, 1993 ; Tashakkori & Teddlie, 1998 ), there is still limited guidance on the topic. Our work helps to develop standards of practice for such transformations and analyses.
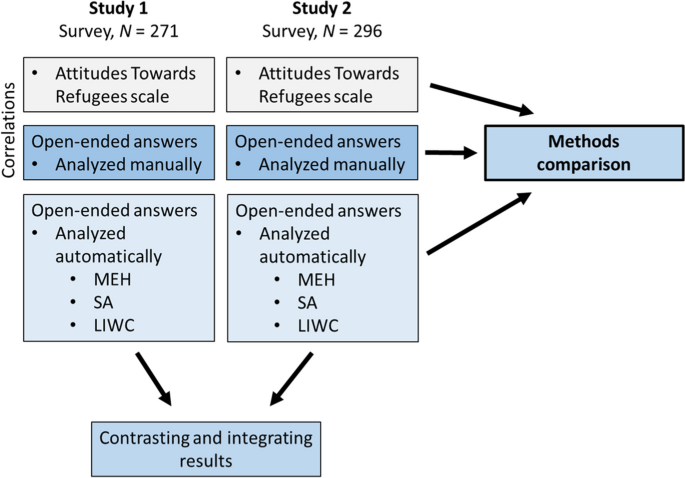
An outline of the present mixed-methods design. Note. MEH = Meaning Extraction Helper, SA = Sentiment Analyzer, LIWC = Linguistic Inquiry and Word Count
Methods of Studies 1 and 2
Participants.
We aimed to have at least 250 valid answers per study. According to the commercial research company that collected data, this sample size would be enough to reflect the demographics of the Polish population. We were also concerned with the feasibility of the study (financial resources) and of the manual text analysis (time and personal resources). We focused on comparing methods rather than statistical values, but for the simple statistical tests that we used, the achieved power was always above 99% (Faul et al., 2007 ).
Study 1 was completed online by 271 participants (53% women, 47% men) Footnote 1 , aged between 19 and 74 years ( M = 43.67, SD = 15.11). Study 2 was completed online by 296 participants (54% women, 46% men), aged between 18 and 75 years ( M = 43.48, SD = 15.68). All of them were Poles, and both samples were representative of the Polish adult population in terms of sex, age, and place of residence. The samples were collected using the computer-assisted web interviewing (CAWI) method. These were nationwide random-quota samples selected according to the representation in the population on the variables sex (2 categories) × age (5 categories) × size of place of residence (5 categories), i.e., in 50 strata in total. Table 1 presents the demographics of the Polish population as well as the demographics of our samples. Footnote 2 Participants received compensation in accordance with the company’s terms (points that could be exchanged for prizes). Both studies were approved by the Ethics Committee of the Faculty of Psychology, University of Warsaw.
Procedure and measures
After giving their informed consent and answering basic demographic questions (used to make the samples’ structure representative), within two larger survey studies Footnote 3 , participants responded to an open-ended question: What strategy, in your opinion, should Poland adopt concerning refugees who want to come to Poland?
Following the open-ended question, we assessed the respondents’ attitudes towards refugees with a five-item Attitudes Towards Refugees Scale (α Study1 = .97, α Study2 = .94), adapted from Eisnecker and Schupp ( 2016 ). The scale included five items, four starting with Do you think that the arrival of refugees to Poland would … (1) be good or bad for the Polish economy? (response scale: definitely bad to definitely good ), (2) enrich or threaten the cultural life in Poland? ( definitely threaten to definitely enrich ) , (3) make Poland a better or a worse country to live in ? ( definitely worse to definitely better ) , and (4) bring more opportunities or risks? ( definitely more risks to definitely more opportunities ). The fifth item asked, Do you think that Poland should accept some of the refugees coming to Europe? ( definitely not to definitely yes ). The corresponding response scales ranged from 1 to 100 in Study 1 and from 1 to 5 in Study 2, whereby lower numbers indicated more negative attitudes. Only the endpoints of the scales were labeled. We used the mean ratings of the five items of the scale as a dependent variable.
The development of the codebook for the manual coding of responses to the open-ended questions followed a bottom-up approach to the analysis of qualitative data (Creswell & Poth, 2016 ). That is, we began with multiple rounds of reading the responses to familiarize ourselves with the data. Afterwards, we started taking notes while reading to write down our initial impressions about participants’ attitudes towards refugees. The individual work was followed by a joint meeting devoted to a discussion of data, aided by the prepared notes. We first agreed that on the most general level, participants’ responses conveyed whether they were for or against accepting refugees. Therefore, the first step of coding (to be performed later by two independent coders in each study) became to determine whether the response’s author was overall (a) supportive of or (b) opposed to accepting refugees into the country. Two additional coding options were available for answers that (c) expressed a lack of any ideas on the matter (e.g., answers such as I don’t know ) or (d) appeared impossible to classify as being for or against accepting refugees (see online materials under the Open Science Framework [OSF] link and Table 3 below). At this stage, the coding was to resemble marking an answer on a scale with four (a, b, c, d) response options, where a given response can be assigned only a single code.
Further discussion about the data was focused on themes that seemed to frequently come up in the responses. We then decided that the coders should also code what recommendations participants had for the refugees themselves and/or for the receiving country. The codebook specified that the coders would mark 0 when a given theme was not mentioned in the text and 1 or 2 if it was. For most themes, only 0–1 coding was foreseen, but for some, we differentiated between different levels of the perceived strength of the answer with 0-1-2 coding. Here, any configuration of codes was possible—from all marked to none marked. This tentative plan was tested in the training phase of coding, when two coders (different people in the two studies) coded the first 10% of the responses and thus assessed the suitability of the codebook. Minor modifications were introduced based on the coders’ feedback. In the end, in Study 1 the answers opposed to the refugees could be classified into three subcategories ( refugees should be sent back home or to other countries, refugees should stay in their homeland and fight , and we should help Poles in need first ). The supportive answers could be divided into six subcategories of strategies. One denoted a general approval for various forms of assistance for refugees and the remaining five focused on approval under certain conditions: 1 = refugees should assimilate or 2 = be forced to assimilate ; refugees should be controlled by the state ; refugees should be isolated 1= from society or 2 = from each other ; refugees should 1 = work or 2 = be forced to work ; refugees should 1 = not receive any social benefits or 2 = only minimal benefits .
In Study 2, we went through a similar process of codebook development, but as a basis we used both the data and the codebook developed in Study 1. That is, while reading the responses and taking notes on them, we were checking whether the data seemed to preliminarily match the codebook or not, as well as what could be different. This led us to keep most of the categories from Study 1 and to add a few new themes. We included one new subcategory to the opposing strategies: we should help refugees in their countries . We also created two new subcategories for the supportive strategies: we should fulfill international agreements and we should accept only certain types of people .
Content analysis
The coders worked independently, treating every answer as a single unit of analysis. As sometimes the replies were highly complex or even internally contradictory, the coders could assign them to multiple subcategories simultaneously. In both studies, the coders started with coding 10% of the responses in order to ascertain that the codebook is a good match for the data and to practice using it. Afterwards, the coders met to discuss discrepancies, reach agreement, and clarify potential differences in their understanding of the categories. After the training stage, minor adjustments were introduced to the subcategories in the codebook to avoid further differences in understanding and to better reflect the content of the responses. Then, the coders coded the rest of the answers. At the end, the coders met again to arrive at final decisions where disagreements still emerged. We assessed the coders’ reliability after the training stage and for the main part of coding via computation of intraclass correlation coefficients (i.e., the absolute agreement). The results showed that in Study 1, in the training phase the coders reached reliabilities of α = .96, 95% CI [.92, .98] for the primary categories, and α = .64, 95% CI [.43, .81] for the secondary categories. For the main coding (after training), the reliabilities were in Study 1 α = 1, 95% CI [1, 1] for the primary categories, and α = .60, 95% CI [.52, .67] for the secondary categories. In Study 2, in the training phase the reliabilities were α = .95, 95% CI [.90, .98] for the primary categories, and α = .52, 95% CI [.28, .72] for the secondary categories. In the main coding phase in Study 2, the reliabilities were α = .87, 95% CI [.83, .90] and α = .68, 95% CI [.62, .73], respectively. Overall, the reliabilities were high to very high for the primary categories and noticeably lower for the secondary categories. However, in the secondary categories, some codes were less prevalent and one or two disagreements could strongly influence the reliability.
Automated text analyses
Besides the manual coding, we used three tools for automated text analysis. Each of them has advantages and limitations, and our goal was to test them on the same material and contrast their results. In future research, it might not be time-efficient to use all of them, but here, we wanted to present a practical comparison for other researchers, who can then decide which of the methods best fits their research.
Meaning Extraction Helper (MEH)
MEH is a tool that is used for the meaning extraction method (Boyd, 2019 ; Chung & Pennebaker, 2008 ). It uses automated text analysis to identify the most commonly used words in a text and determines how these words co-occur. Users can set the minimum number of words required for a text to be included in the analysis and the minimum observed percentage of a word (Boyd, 2019 ). The main MEH process occurs in three steps (Blackburn et al., 2018 ). First, the program automatically filters out a group of stop words (i.e., function words, low base rate words). Second, it identifies common content words (nouns, verbs, adjectives, and adverbs) in each text. Common content words are identified based on their frequency across the entire corpus that is being analyzed. MEH then assigns a binary score to each word. For example, if 10 common content words from the whole corpus are identified in a given text, a “1” will be assigned to each word and the remaining words will be assigned a “0”. In other words, the MEH generates a series of binary scores that represent common words for each text. Third, once the MEH has processed each word in each text, an output file is generated that identifies common words and shows which texts include them (or put differently, it shows each text as a row and indicates which words presented as columns are present or absent in it). Then, next steps of meaning extraction are performed outside of the MEH. The output file can be read into a statistical program (e.g., SPSS) to perform a principal component analysis (PCA) with varimax rotation and compute a set of components that identify common themes in the texts used. Based on this analysis, one can extract themes that emerge from the analyzed texts. Then, researchers can name the components using a bottom-up approach. Given the combination of statistical methods with qualitative interpretation of the components, the meaning extraction method constitutes a mixed-methods approach to studying language data. This methodology and the MEH software are recommended when conducting research in languages other than English, as the method does not involve translation until after the analyses have been conducted, which can help in cross-culturally appropriate text analysis (Ramirez-Esparza et al., 2008 ; Wagner et al., 2014 ).
Sentiment Analyzer
We manually corrected all responses for spelling and major grammatical errors. Subsequently, we used a tool available for Polish language called Sentiment Analyzer, part of the Common Language Resources and Technology Infrastructure, available online at https://ws.clarin-pl.eu/sentyment Footnote 4 . The tool’s development drew on a lexical semantic network for Polish, i.e., plWordNet 3.0 (Maziarz et al., 2013 ), which became one of the largest Polish dictionaries (Janz et al., 2017 ). plWordNet comprises lexical units (i.e., lemma, part of speech, and sense identifier, which together constitute a lexical meaning) and to a subset of these units, emotive annotations were added manually (Zaśko-Zielińska et al., 2015 ). In short, the annotators first identified the sentiment polarity of the lexical units (positive, negative, and neutral). Second, they assigned basic emotions following Plutchik’s ( 1980 ) wheel of emotions (joy, sadness, anger, fear, disgust, trust, and anticipation). Moreover, in the Polish linguistic tradition, basic emotions are associated with fundamental human values (Zaśko-Zielińska et al., 2015 ), and it may be difficult to separate emotions from values in language expression (Kaproń-Charzyńska, 2014 ). Therefore, six positive (utility, another’s good, beauty, truth, happiness, knowledge) and six negative (ugliness, error, harm, misfortune, futility, ignorance; Puzynina, 1992 ) values were incorporated into the unit descriptions.
Linguistic Inquiry and Word Count (LIWC)
To be able to analyze the responses in the LIWC program (Pennebaker et al., 2015 ), we translated them from Polish to English via Google Translate ( https://translate.google.pl/ ). Such approach was recommended to us by the LIWC developers for datasets in languages not covered by the software, and it has been shown to be effective in other studies (R. Boyd, personal communication, June 21, 2018). LIWC consists of the processing component that opens text files and the dictionaries. The program goes through each text in a file, word by word, and compares each word with the dictionary file, which consists of nearly 6400 units (words, word stems, and emoticons). If the word appears in the dictionary, it is automatically counted and classified into hierarchically-organized categories. At the end, LIWC calculates percentages of the categories. The categories include 21 linguistic dimensions (e.g., pronouns, verbs), 41 psychological constructs (e.g., perception, affect, and cognition), six personal concerns (e.g., work, home), and five informal language markers (e.g., swear words). In addition, LIWC provides the word count, general descriptor categories (e.g., words per sentence), and summary language variables (e.g., emotional tone; Pennebaker et al., 2015 ).
Automated text analyses: Short methods comparison
MEH allows researchers to automatically extract themes that emerge from open-ended answers. Its advantage is that it gives a quick impression of these themes and is language-independent, but the downside is that it does not take valence or negation into account. In sum, it can identify topics, but not emotions. Sentiment Analyzer allows its users to analyze valence, emotions, and associated values in the responses, and it is tailored for the Polish language, which is more grammatically complex than English. LIWC is to some extent similar to Sentiment Analyzer, but there are many more categories in LIWC. The downside is that LIWC is language-dependent and while it is available in a few languages, there is no official version for Polish. Additionally, both of the dictionary-based programs, Sentiment Analyzer and LIWC, have little or no capacity to account for context, irony and sarcasm, or idioms (Tausczik & Pennebaker, 2010 ). By using all these methods on the same material, we present their advantages and disadvantages in practice.
Results of Studies 1 and 2
Attitudes towards refugees scale.
The attitudes towards refugees were measured with five closed-ended statements of the Attitudes Towards Refugees Scale . They reached M = 38.42 ( SD = 28.62) on a response scale from 1 to 100 in Study 1, and M = 2.33 ( SD = 1.20) on a response scale from 1 to 5 in Study 2, where lower levels meant more negative attitudes. In both time points the results of one-sample t -tests showed that the means were significantly lower than the scales’ midpoints (38.42 vs. 50.5 in Study 1, and 2.33 vs. 3 in Study 2), with t (270) = −6.95, p < .001, 95% CI [−15.50, −8.66], Cohen’s d = −0.42, for Study 1, and t (295) = −9.55, p < .001, 95% CI [−0.81, −0.53], Cohen’s d = −0.56, for Study 2.
In order to compare the responses to the scale across our Studies 1 and 2, but also to compare it to studies of national polls (CBOS and Ariadna) conducted at a similar time, we concentrated on the question Do you think that Poland should accept some of the refugees coming to Europe? This question was very similar to questions asked in these polls and had the same anchors of the response scale ( definitely no to definitely yes ). We first recoded the 1–100 variable from Study 1 into a five-point scale (1–20 → 1, 21–40 → 2, 41–60 → 3, 61–80 → 4, 81–100 → 5). Then, in the same manner for Studies 1 and 2, we combined frequencies for responses of 4 and 5 into “supportive of accepting refugees” and frequencies for responses of 1 and 2 into “opposed,” and 3—the midpoint of the scale—we treated as “undecided.” When looking at the percentages in Study 1, 28% of the participants were supportive, 49% were opposed, and 23% were undecided (see Fig. 2 ). In Study 2, 26% of the participants were supportive, 56% were opposed, and 17% were undecided (see Fig. 2 ). The above results suggest that the participants’ attitudes expressed via the closed-ended statement were generally negative and were in opposition to allowing refugees in Poland. This attitude did not change significantly across Studies 1 and 2, as evidenced by the results of an analysis of variance (ANOVA) with Study (1 vs. 2) as the between-subjects factor and the mean responses to the aforementioned question from the scale as the dependent variable, F (1, 565) = 1.55, p = .39, η p 2 = .00 ( M Study1 = 2.56, SD = 1.41 vs. M Study2 = 2.45, SD = 1.49).
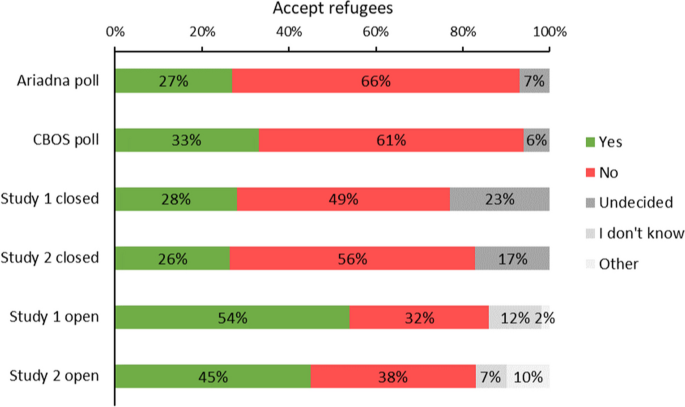
Results of two national polls, closed-ended answers from Studies 1 and 2, and manual coding of open-ended answers in Studies 1 and 2
Manual content analysis
The open-ended answers that we analyzed via the manual coding as well as with automated text analyses were of varying length (Table 2 ): some responses were very short (one to a few words) and some were very long (a paragraph), but most comprised one or two sentences.
As mentioned in the Methods section, in the manual content analysis of the answers to the open-ended question, there were two levels of codes: a primary code/category ( accept, do not accept, I don’t know, and other ), which all answers were ascribed one of, and a secondary code/category (e.g., send refugees home or to other countries ), any number of which was assigned to any given answer. The results of the content analysis revealed that in Study 1, 54% of the participants were supportive of accepting refugees and 32% were opposed (see Fig. 2 ). In Study 2, 45% of participants were supportive and 38% were opposed. The rest of the participants were undecided ( I don’t know answers: 12% in Study 1 and 7% in Study 2) or gave answers that were impossible to code as being supportive or opposed (2% and 10%, respectively). Footnote 5 The general categories were subdivided into more specific themes, as it was important for us to not only interpret the answers quantitatively in terms of percentages for and against, but also to examine their qualitative content (see Table 3 ). The themes were thus nested under the primary categories (e.g., refugees should assimilate was a subcategory of accept ). We report the prevalence percentages out of all answers, not only out of the given primary category.
Most of the answers stated that refugees should be accepted, but only 23% of all the answers in Study 1 and 7% in Study 2 explicitly described the support that the refugees should receive. The most frequent topic brought up in Study 1 was that refugees should work—30% of participants mentioned it, and 16% even said that refugees in Poland should be forced to work (see Table 3 ). The second most frequent category was to assist refugees in a variety of ways (23%). The third was a recommendation that they should assimilate into Polish society (22% mentioned assimilation, and 6% said refugees should be forced to assimilate). These themes did not surface to a similar extent in Study 2. Instead, the participants in Study 2 focused on the need to carefully select those who were to arrive (25%) and on the necessity for the authorities to control them (15%, see Table 3 ). In both time points, many of the “accept” answers were rather of the “yes, but…” kind and stated what refugees should do or under what conditions they should be accepted (see examples of comments fragments in Table 3 ).
Among people who were opposed to accepting refugees in Study 1, most said that Poland should send refugees back home or to other countries, while others indicated that refugees should have stayed in their homeland and fought. In Study 2, conducted a year later, an idea emerged that Poland should help the refugees in their home countries. This may be related to the fact that such an answer to the refugee situation was at that time mentioned in the media (e.g., Polsat News, 2017 ; TVN24, 2017 ).
First integration: Attitudes scale and manual coding
We wanted to triangulate and integrate the results from the closed- and open-ended answers. To this end, we followed the approach recommended in the literature (e.g., Onwuegbuzie & Teddlie, 2003 ) and we correlated the answers from the closed-ended questions with the codes stemming from the open-ended questions. We also added another analysis: mean comparisons using a series of t -tests. Footnote 6 For the correlations, we binarized a few codes that had a 0-1-2 coding into a 0-1 coding. All variables from the manual coding that were usable were included. From the first variable, we included only 0 ( do not accept refugees ) and 1 ( accept refugees ), without I don’t know and other . Then, we took each of the codes as a separate variable (0 = code absent, 1 = code present) and we ran Pearson’s correlations on each of these code variables with mean scores on the Attitudes Towards Refugees Scale (scale: 1–100 or 1–5). For the mean comparisons, we computed t -tests with codes treated as groups (i.e., we compared code absent vs. code present groups) with the Attitudes scale as a dependent variable. Having two studies, we could verify the results from Study 1 on the data from Study 2. Even though the frequencies of the themes shifted between Study 1 and 2, the results of the relationships between these measures as well as the results of the mean comparisons were similar in both studies.
The correlations showed that the results from the scale were strongly correlated with the results coded as the Accept refugees category (Table 4 , left). The mean comparisons showed clearly that people who said that refugees should be accepted were also on the Attitudes scale more welcoming than people who said that refugees should not be accepted (Fig. 3 ). The Cohen’s d value showed that the effect was very large (Table 4 , right). The correlations and mean comparisons also reflected the ambiguity of the answers that was visible in the manual coding. For example, mentioning that the refugees should assimilate (e.g., learn the Polish language) was correlated with positive attitudes towards them and people saying it had more positive attitudes towards refugees than people not mentioning it. However, saying that refugees should be forced to assimilate was not correlated with attitudes and there were no significant differences in attitudes.
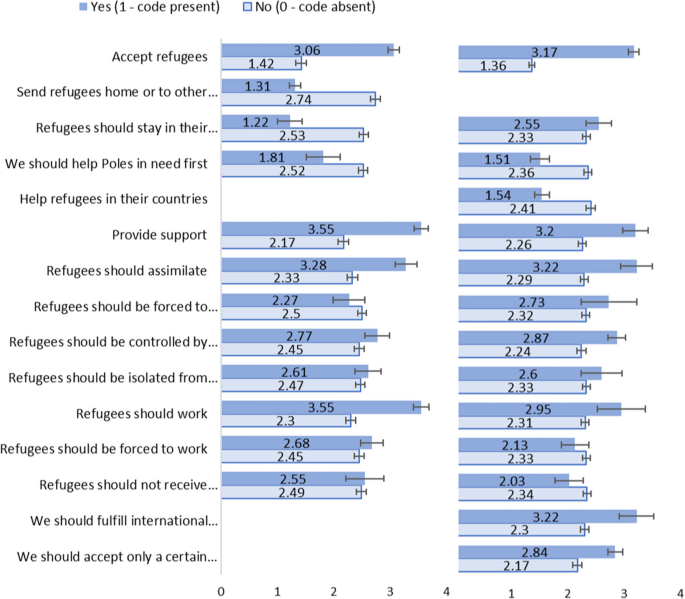
Mean differences in the Attitudes Towards Refugees Scale between code present and code absent in the results of the manual coding in Studies 1 and 2. Note. Error bars represent standard errors of the mean
Some correlations and mean differences were unsurprising, for example, that people who thought the refugees should be sent home had much less positive attitudes towards them. Some results were, nevertheless, intriguing considering the up-to-now understanding of attitudes towards refugees based on closed-ended answers, but less so given our results of the open-ended questions. For instance, mentioning that refugees should assimilate or should work, or that Poland should accept only a certain kind of people does not sound like an expression of positive attitudes towards refugees at first. However, letting participants express their opinion in their own words gave us insight into why these were associated with positive attitudes. People who indicated on the closed-ended questions that refugees could be enriching for the country and that we should accept them were often reasoning in their answers that refugees who will be well-integrated, will work, and will be deemed harmless could indeed enrich Poland and its economy and should be accepted.
As the next step of our analyses, we compared the manual coding of the open-ended answers with the results from MEH, an automated tool designed to extract themes from textual data. We set the minimum number of words required for a text to be included in the analysis to 2, and the minimum observed percentage to 3% (see Boyd, 2019 ; Ikizer et al., 2019 ). We conducted a PCA with a varimax rotation for each of the two studies. The variables entered were the content words that appeared in the texts. They were automatically coded for whether they were present in a given text or not (for more details, see Tables 2 and 3 in the Supplementary Material on OSF ). As PCAs on textual data can produce too many components, it is advisable to use a higher eigenvalue than the customary 1 for determining the number of factors. We used an eigenvalue of ≥ 1.5.
The results showed that four themes in Study 1 were above the eigenvalue of ≥ 1.5. These themes explained 29% of variance, which is fully acceptable with this type of data (Boyd, 2019 ). There were eight themes above the eigenvalue of ≥ 1.5 in Study 2 that explained 44% of variance (for more details see the Supplementary Material on OSF ). Each answer was quantified for the degree to which it fit (i.e., loaded on) each of the themes. In order to name the themes, we analyzed the words in the themes and sample texts that fit each of the themes the best. Table 5 presents the theme labels, example words, and (fragments of) the two highest-loading comments in each theme.
In Study 1, the four themes were: Support and work , Forced work , Let them in but… , and Language and assimilation . The two work-related themes reflected the work-related theme from the manual coding results (see Fig. 4 ), but were structured slightly differently. The first theme combined working with other types of support that the refugees should be provided. The second theme reflected the forced work theme of the manual coding but also incorporated the No social benefits theme. The Let them in but… theme was the broadest one, and it partly reflected our Accept category from the manual coding when subtracting the Provide support theme—it showed that even Poles who indicated that we should accept refugees listed many conditions under which it should happen. The Language and assimilation theme was partly similar to the manually coded Assimilation theme, but it was more focused on language only and it expressed both supportive statements about language courses that should be provided with rather unfriendly statements about the supposed lack of will of refugees to integrate and learn the local language.
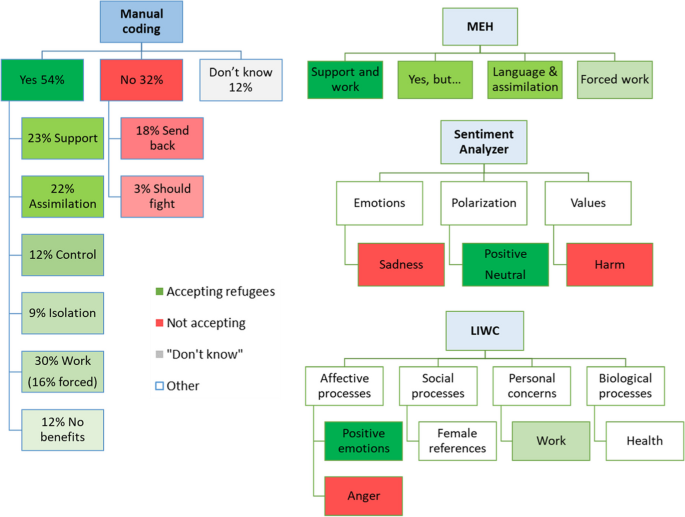
Categories that emerged from analyses of the same open-ended answers in Study 1 when using different methods. Note. MEH = Meaning Extraction Helper, LIWC = Linguistic Inquiry and Word Count
In Study 2, the eight themes that emerged were Support and assimilation, Forced work, Faking to flee from war—help abroad, Control and selection, Women and children only, Polish government should/shouldn't, Poland—bad place and time, and They don't want to come . Thus, there was some overlap between the themes from Study 1 and the categories from manual coding in Study 2, but new themes also emerged. Specifically, the new themes included statements that Poland is not the best country to invite refugees to and that refugees genuinely do not want to come to Poland, but to other EU countries. However, these themes were the smallest ones, with the least variance explained (see Table 5 and the Supplementary Material on OSF ). Again, even those themes that were to some extent similar to the manual coding included statements that were on the same topic but had different valence or intention.
To further explore the attitudes expressed in participants’ replies to the open-ended questions, we subjected the obtained texts to automated sentiment analysis. For this purpose, we selected a subset of variables that can be generated by Sentiment Analyzer, We chose those that also surfaced in participants’ responses in the manual coding and MEH. We considered only the responses coded as supportive of or opposed to accepting refugees into Poland, excluding “I don’t know” and other indecisive answers. Consequently, the Sentiment Analyzer variables included in the statistical analyses as the dependent variables were: polarity (positive, negative, neutral), five emotions (anticipation, fear, disgust, anger, sadness), and three values (harm, futility, utility). We conducted three multivariate analyses of variance (MANOVAs) on these three sets of variables with Study (1 vs. 2) and Response (for vs. against accepting refugees) as two between-participants factors.
The multivariate main effect of study was significant, F (3, 471) = 6.57, p < .001, η p 2 = .04, and the effect of response marginally significant, F (3, 471) = 2.28, p = .079, η p 2 = .01. These effects were qualified by a significant interaction, F (3, 471) = 2.96, p = .032, η p 2 = .02. In the univariate tests, the interaction was significant for positive polarity, F (1, 473) = 4.50, p = .034, η p 2 = .01 and neutral polarity, F (1, 473) = 5.81, p = .016, η p 2 = .01. The pairwise comparisons showed, for Study 1 versus Study 2, that responses for accepting refugees were more positive in Study 2 than in Study 1 ( M Study1 = 0.44, SD = 0.80 vs. M Study2 = 0.86, SD = 1.65, p = .002). Responses against accepting refugees were in Study 2 less neutral (i.e., stronger) than in Study 1 ( M Study1 = 7.80, SD = 12.34 vs. M Study2 = 3.95, SD = 4.20, p = .007). Further, comparing responses for and against refugees, only in Study 2 these responses differed: responses for accepting refugees were more positive than responses against refugees ( M for = 0.86, SD = 1.65 vs. M against = 0.42, SD = 0.94, p = .003). Responses for accepting refugees were also more neutral (i.e., weaker) than responses against accepting refugees ( M for = 8.54, SD = 11.64 vs. M against = 3.95, SD = 4.20, p < .001).
For emotions and values, only the multivariate main effect of study was significant, for emotions, F (5, 469) = 2.76, p = .018, η p 2 = .03, and for values, F (3, 471) = 5.40, p = .001, η p 2 = .03. In the univariate tests, the main effect of study was significant for the emotion of sadness, F (1, 473) = 5.08, p = .025, η p 2 = .01 and the value of harm, F (1, 473) = 7.50, p = .006, η p 2 = .02. References to sadness appeared more in Study 2 than in Study 1 ( M Study1 = .38, SD = 1.02 vs. M Study2 = .60, SD = 1.14). Similarly, references to harm appeared more in Study 2 than in Study 1 ( M Study1 = .34, SD = .94 vs. M Study2 = .60, SD = 1.24).
Taken together, the polarity of words that participants used in their responses may suggest less intense attitudes towards refugees in Study 1 than in Study 2. Simplifying, one could define them as overall more positive in Study 2. The results were, however, more complex and showed rather a larger polarisation than only positivity. The neutrality and positivity results were combined with the expression of these attitudes by participants that were (based on the manual coding) in favor or against accepting refugees. This showed that participants who were in favor of accepting refugees were even more positive in their answers in Study 2 than in Study 1. However, participants in Study 2 simultaneously expressed more concerns about having refugees in Poland. These results are partially in line with the results of our content analyses in that participants’ attitudes in Study 1 were overall more positive than a year later. On the other hand, although fewer participants were for the idea of accepting refugees in Study 2, perhaps they used stronger words to convey their approval than participants in Study 1. At the same time, participants in Study 2 might have been sad about the dire situation of refugees and the harm inflicted on them, which would fit the positive attitudes. Nonetheless, from the content analyses, we know that participants did not empathize with refugees, but rather were worried about the consequences of their arrival.
Sentiment Analyzer was the only tool at our disposal to analyze responses in Polish without translating them. It was intended to focus on, obviously, the linguistic expressions of feelings and emotions and their valence. We subsequently turned to LIWC, which is an established tool for more extensive automated text analysis. The program generates about 90 output variables for each text file, but not all of the available LIWC categories were pertinent to the present purpose. First, we used the overall word count from the output and reported it for the two studies in Table 2 . In order to determine which variables should be further analyzed in order to explore the underlying language structure of the answers to the open-ended questions, we again reviewed the results of the manual coding and MEH analyses. We compared them with examples of words, constituting LIWC categories, in the program’s dictionary (Pennebaker et al., 2015 ). This led us to identify affect (positive emotions, negative emotions, anxiety, anger, sadness), personal concerns (work, home, money), biological processes (body, health, power, risk), and social processes (family, male, female) as four potential categories of interest. As in the Sentiment Analyzer, the variables that make up the categories are conceptually related (e.g., Tausczik & Pennebaker, 2010 ; Pennebaker et al., 2015 ). We therefore conducted four MANOVAs (one per category) with the selected variables from each given category as the dependent variables. Study (1 vs. 2) and Response (for vs. against accepting refugees) were the between-participants factors.
The multivariate main effect of study was significant for affect, F (5, 469) = 3.63, p = .003, η p 2 = .04. For affect, the multivariate main effect of response and the interaction were marginally significant, F (5, 469) = 1.96, p = .084, η p 2 = .02 and F (5, 469) = 2.03, p = .074, η p 2 = .02, respectively. We discuss further only the significant result. Specifically, the univariate main effect of study was significant for positive emotions, F (1, 471) = 10.78, p = .001, η p 2 = .02 and anger, F (1, 471) = 4.41, p = .036, η p 2 = .01. The amount of positive emotions was higher in Study 2 ( M = 5.65, SD = 11.57) compared to Study 1 ( M = 2.84, SD = 5.06), while anger was lower in Study 2 ( M = .28, SD = 1.59) compared to Study 1 ( M = 1.02, SD = 6.77).
For personal concerns, the multivariate main effects of study and response were significant, F (3, 469) = 10.54, p < .001, η p 2 = .06 and F (3, 469) = 19.82, p < .001, η p 2 = .11, respectively. The interaction was significant as well, F (3, 469) = 12.75, p < .001, η p 2 = .08, but just for work in the univariate analysis, F (1, 471) = 36.19, p < .001, η p 2 = .07. The pairwise comparisons revealed that participants who were for accepting refugees mentioned work more often in Study 1 than in Study 2 ( M Study1 = 9.38, SD = 11.35 vs. M Study2 = 1.78, SD = 4.00, p < .001), and in Study 1 they mentioned it more than those who were against accepting refugees ( M = .90, SD = 2.76, p < .001).
For biological processes, the multivariate main effect turned out significant for response, F (4, 468) = 3.88, p = .004, η p 2 = .03, and the univariate main effect of response was significant for health, F (1, 471) = 12.27, p < .001, η p 2 = .03. That is, participants in favor of accepting refugees referred to health more ( M = .97, SD = 3.37) than participants who were against ( M = .09, SD = .52).
Finally, for social processes, the multivariate main effect of study was significant, F (3, 469) = 4.53, p = .004, η p 2 = .03 and response was marginally significant, F (3, 469) = 2.54, p = .056, η p 2 = .02). In terms of the univariate tests, the main effect of study was significant for the variable female, F (1, 471) = 13.39, p < .001, η p 2 = .04. There were more references to females in Study 2 ( M = 1.28, SD = 4.62) than in Study 1 ( M = .11, SD = .74).
In sum, the results of LIWC analyses converged with those from Sentiment Analyzer with regard to a generally more positive valence of responses in Study 2 compared to Study 1. The difference was that Sentiment Analyzer did not detect anger, while LIWC did not detect sadness when it comes to particular emotions in the answers. Therefore, the results of the automated analyses of the emotional underpinnings of the responses may be deemed quite inconclusive. This said, we did not explicitly code emotions, but instead inferred them post hoc so that we could explore the data with both Sentiment Analyzer and LIWC. Considering other LIWC variables, work did surface earlier in the manual coding and MEH analysis, in the participants’ opinion that refugees should have jobs or even be forced to work. This issue was indeed, like in LIWC results, more emphasized in Study 1. We have also noticed specifications as to who may be allowed to enter Poland, and an inclination to accept female refugees in Study 2 (see e.g., Table 5 ). A somewhat unexpected result concerned health in the responses of participants in favor of accepting refugees. The topic of health did not arise in other methods of analysis of our material. Nevertheless, it might have been related to the public debate about refugees in Poland, although especially its prejudiced iterations were delivered by certain politicians, who claimed that refugees pose danger as carriers of diseases (Gera, 2015 ).
Triangulation, integration, and methods comparison
In the current research, we used various methods to explore measuring attitudes towards outgroups on the example of attitudes towards refugees. The results of the Attitudes Towards Refugees Scale showed that the participants’ attitudes expressed via closed-ended statements were generally negative and participants were opposed to hosting refugees in Poland. The results of the manual content analysis of the answers to the open-ended question revealed a more positive view: roughly one third (32% and 38%) of the participants opposed accepting refugees. Although the results from the scale were strongly correlated with the results coded as the Accept refugees category, the qualitative analysis of the answers allowed us to observe many conditions under which the participants were willing to accept refugees. Such conditions were: an expectation that the refugees will assimilate, that they should work, or that they should be controlled by the state. Whereas the closed-ended answers and the percentages of the coded open-ended answers only showed that the attitudes were more negative in Study 2 than in Study 1, the content analysis of the open-ended questions also showed how the discourse and the topics mentioned changed between Study 1 and 2. For example, the main topic that the refugees should work present in Study 1 was less prominent in Study 2. Instead, the participants concentrated on the fact that Poland should accept only a certain number of refugees and a certain kind of people. This is in line with the extensive research on agenda setting, which shows that people emphasize in their responses what is on the media and this can shift even in a much shorter time than a year (e.g., Feezell, 2018 ).
The subsequent analysis conducted using MEH—an automated tool to extract themes from the text—yielded fewer themes than the manual coding, but the themes to some extent reflected some of the themes from the manual analysis. However, they were structured differently, as they often mixed positive statements (e.g., give them a chance to work ) and negative statements (e.g., put them in work camps ) as long as they were about the same topic (here: work). Manual coders observed that these were on the same topic, but intuitively divided them according to the supportive or oppressive intentions that they saw behind each statement. The results of the automated sentiment analysis with Sentiment Analyzer and LIWC provided us with a comparison of emotion words used in Studies 1 and 2 and by participants for and against accepting refugees. LIWC and Sentiment Analyzer to some extent showed that the general valence, or amount of positive emotions, was higher in Study 2 than in Study 1, which was contrary to the answers on the acceptance scale or to the percentages from the coded open-ended answers. The results from the Sentiment Analyzer combined with those from the manual coding were more detailed and showed rather that the response texts were more polarized and intense (more positive and less neutral) in Study 2 than in Study 1. From the thematic analysis and the manual coding, we saw that participants in Study 2 talked more about helping refugees in their countries or about accepting only a certain kind of people than in Study 1. On a linguistic level, words related to helping and accepting are positive, but how they were used was actually expressing more negative attitudes, for example, many participants said that Poland should send humanitarian help outside instead of accepting refugees to the country. In line with the other results, Sentiment Analyzer results also showed more sadness and harm in Study 2 than in Study 1. Using LIWC allowed us to compare more than just valence and emotions, and the results also showed that participants in Study 1 mentioned work more often than participants in Study 2, which reflects the manual coding and MEH analysis. In general, the results of LIWC and Sentiment Analyzer show advantages of the relatively quick and easy-to-use dictionary tools, but also the limitations of using and interpreting data based on one type of analysis only.
General discussion
The goal of the current research was twofold. First, we wanted to compare and integrate different methods of assessing attitudes towards outgroups, particularly to refugees. Second, we wanted to compare various methods of analyzing open-ended answers: manual content analysis and three automated text analysis tools (MEH, Sentiment Analyzer, and LIWC).
The results of the different methods partly converged, but each method also afforded a view of the data from a different angle. This conclusion is not historically new (see e.g., Geer, 1991 ; Krosnick, 1999 ). Furthermore, also other researchers have called for using open-ended questions, as these allow us to learn from participants’ ideas that researchers themselves would not come up with (e.g., Geer, 1991 ; Haddock & Zanna, 1998 ). In the current research we extend this with an observation that with open-ended questions one learns about explanations of people’s views and attitudes. These explanations are crucial to understanding attitudes, as basing an interpretation solely on closed-ended answers could lead researchers to interpret these attitudes incorrectly. With our research, we remind of these important, but in the last years largely forgotten, statements. We also show how to combine methods with the help of modern tools that allow for a relatively fast analysis of a large body of open-ended answers. We tested various tools on the same material and the researchers can choose which of the methods they want to use in their studies. If one decides to use more than one method or even all of them at the same time, it is important to thoughtfully integrate and interpret them. When the methods produce convergent results, the task of integrating them is relatively easy. But what if the methods generate ambiguous or even contradictory results? In the following section, we discuss our findings showing how the results coming from different methods can be integrated, how they complement each other, and what to do when the results differ across methods.
Comparing and integrating results of different methods
In the current research, closed-ended answers of the same participants were more negative than their open-ended answers. We think that this difference can be attributed to the format of the questions and to the fact that attitudes towards refugees are ambivalent, complex, and not well defined. When asked in an open format, participants can better explain their views and follow less the social norm (Connor Desai & Reimers, 2019 ; Frew et al., 2003 ). When integrating such results one must take into account the qualitative content of the open-ended answers. In our case, participants forced to answer on a scale chose to be more conservative in their answers, but when they could show the complexity of the issue and of their views, they stated more conditional answers as to not only whether to accept refugees, but how it should be done.
For the open-ended answers, we analyzed exactly the same content, so the differences we encountered in the results stem from the specific analysis methods and tools that we used. The manual analysis allowed for different levels of coding and for detecting indirect statements, irony, or negation. The results of MEH also produced some of the themes that emerged in the manual coding. These results agreed with each other to some extent and MEH could be seen as an alternative and quicker method of extracting meaning and creating themes. However, some of the themes were different as automatic meaning extraction does not take into account the valence of the answers. This was visible, for example, in the MEH-generated theme about learning the language, where some participants were writing about offering help, including language courses, others were stating that refugees should be forced to learn Polish, and still others were skeptical whether refugees would be able or willing to learn Polish. In order to integrate these partly disparate results, it is crucial to understand the content of the themes generated with a MEH analysis. To do so, it is important to look not only at the words in each theme but to carefully read the highly-loading responses from each extracted category (see also Ikizer et al., 2019 ). Researchers studying attitudes or other strongly valanced phenomena should either use MEH very carefully or use it in parallel with manual coding of at least some portion of the data.
Further cues to participants’ attitudes towards refugees came from the exact words they used in their written responses. Most importantly, automated text analyses allowed us to identify the emotional tone of the answers. They also provided an overview of the psychological constructs that surfaced while participants were expressing their views. The results of Sentiment Analyzer- and LIWC-based analyses indicated that on the linguistic level, participants emotions were more extreme and also more positive about refugees in Study 2 than in Study 1. Interestingly, the more positivity in Study 2 findings from two different software programs were not in line with the results from the closed-ended answers or from the manual coding, with these last two revealing more negative attitudes towards refugees in Study 2 compared to Study 1. How to reconcile these results? When the results were combined with the information from manual coding, the findings showed that it was mainly that in Study 2 participants expressed their views more intensely, more emotionally in general. Congruent with the above and with the results of manual coding, Sentiment Analyzer results showed more sadness and harm in Study 2 than in Study 1. Other Sentiment Analyzer and LIWC results concerned specific themes. These largely corresponded to what we found in manual coding as well as in automatic meaning extraction with MEH. In particular, LIWC, as a more comprehensive tool than Sentiment Analyzer, evidenced performance that was overall consistent with that of human coders. Furthermore, Sentiment Analyzer results showed that the responses of the participants whose answers were manually coded as accepting of refugees were also more positive, as shown by the automated analyses, than answers of participants who were against refugees. Overall, LIWC and Sentiment Analyzer are easy-to-use and time-efficient tools that complement the results from closed-ended questions. We, however, recommend using such fully automated tools in parallel with methods that capture the meaning and context of the responses.
To deepen our understanding of the participants’ attitudes and to compare the methods, we correlated the results from the closed-ended answers with the variables from the coding of the open-ended answers (as recommended in Onwuegbuzie & Teddlie, 2003 ). We also compared the means on the closed-ended scale for participants who mentioned or did not mention each given topic in their open-ended answer. The results of the correlations and mean comparisons showed similar results and can be treated as alternative methods of showing how the results of coded open-ended answers relate to closed-ended answers. Some correlations and mean comparisons merely showed a convergence of these methods with manual coding (e.g., participants who had positive attitudes towards refugees were also more supportive in their spontaneous answers), but some were surprising given the previous work on attitudes towards refugees conducted using closed-ended questions. However, these results were understandable and rational given the results of our open-ended questions. Similar results were obtained when combining codes from manual analysis with Sentiment Analyzer and LIWC variables. Overall, combining methods and letting participants express their opinion in their own words gives researchers insights into the reasoning behind the given answers and allows for a better understanding of attitudes.
Advantages and disadvantages of closed- and open-ended questions
In the current article, we showed the advantages of using open-ended questions for measuring attitudes and encouraged researchers to combine open- and closed-ended questions in their research. However, one should also consider the weaknesses and limitations of open-ended questions. While open-ended questions provide richer, more nuanced responses, it is much more difficult to get people to respond to them than to respond to closed-ended questions. Additionally, sometimes open-ended responses may just not be necessary. If one is measuring attitudes that are well-formed and that participants are certain about, it might not be necessary to use open-ended questions. Similarly, if one is conducting a series of studies and sees that over time the content of the answers stays similar, in the later studies it might not be needed to bother participants with responding to open-ended questions.
In our research, we compared different automated text analysis methods. They all are quicker than manual coding, but they also require some time investment. We devoted some time to pre- or post-processing (MEH: checking the themes; Sentiment Analyzer: correction of spelling before the analysis; LIWC: translation from English into Polish). However, some of these corrections, such as correcting spelling, are not obligatory; the quantity can make up for quality. Researchers who analyze many thousands of, for instance, tweets do not correct anything or they use only standard corrections of the most popular mistakes (Ikizer et al., 2019 ). This means losing some data, but with a very large dataset this does not constitute a big problem.
When it comes to the MEH analyses, they were very useful, objective, and relatively time-efficient. However, some features of the method itself influenced the results. Most importantly, such automated analyses as MEH detect the occurrence and co-occurrence of words without taking into account negation or context. Consequently, texts within a given theme may mention the same words and concepts, but can be expressing opposite intentions. Furthermore, the longer the text, the better MEH can classify it, so as a rule, the texts that are the highest-loading on a specific theme are rather the longer ones. Human coders are able to reliably extract themes and their valence manually even from short texts. All that said, we expect that the next years might bring new tools for sentiment analysis (e.g., similar to VADER, Hutto & Gilbert, 2014 ) that will overcome some of the limitations of the current tools.
Conclusions and implications
In the current research, the use of various methods applied to the same material allowed for contrasting them and looking at the advantages and limitations of each one. The manual coding allowed for the most detailed and context-sensitive analysis. This was manageable with the current dataset, but when working with large amounts of data collected automatically (e.g., from Twitter) manual coding would be impractical. The automated text analyses provided some approximation of the manual coding. However, we recommend using more than one of such tools at the same time. The results of each method separately converged only to some extent with each other and with the manual coding. Using two (or more) such tools would help diminish problems inherent to the automated methods, such as being either valence- or context-insensitive, or analyzing valence but focusing less on the topics mentioned. We can recommend using automated tools for large datasets, but with an additional manual analysis of parts of the most representative answers.
A direct real-world implication of our results is that instead of a simple yes or no to accepting refugees, there should be more space for discussion as to who should be accepted and how could the newcomers be integrated into the society. In order to do this, researchers and policymakers could use a broad array of methods of assessing and analyzing attitudes towards outgroups.
Data availability
The data and materials for all studies as well as the codebooks used for manual coding of answers are available at https://osf.io/3naj5/?view_only=f849eee116a5447db19290160f00ba39 .
Code availability
Code used to analyze the data is available at https://osf.io/3naj5/?view_only=f849eee116a5447db19290160f00ba39 .
The research company that collected the sample uses only two gender/sex categories: “man” and “woman.” In Polish there is just one word for both gender and sex: “płeć.”
In general, online panels suffer from coverage bias (i.e., do not include the offline population) and self-selection bias (i.e., include only people who sign up themselves for the panels). Our findings are thus generalizable to the Polish population that uses the Internet.
In Study 1, before the open-ended question, the participants were shown four scenarios describing a refugee and a few questions related to the scenarios. The scenarios had no effect on either the closed- or the open-ended responses, so we combined the data across the four conditions. In Study 2, we included a measure of dehumanization to deepen the understanding of the topic of forced work that emerged in Study 1. However, because in Study 2 this topic was not as prevalent as in Study 1, we excluded it from the analyses reported here. We provide all the data and results of the above analyses under the following link: https://osf.io/3naj5/?view_only=f849eee116a5447db19290160f00ba39 .
Sentiment Analyzer was not accessible through CLARIN’s web at the time of conducting our studies; instead, the analyses were carried out through personal communication with the tool’s creators (A. Janz, personal communication, February 7 & March 20, 2018).
Closed-ended answers in polls and in our closed-ended scale did not have the other category, so when counting only the yes/no/don’t know responses, the percentage of the yes answers would be even higher.
One should be cautious in interpreting the results of the t -tests due to the unequal distributions of the “code present” vs. “code absent” groups. These analyses are, however, replicated on both studies and are per se exploratory in nature.
Baburajan, V., e Silva, J. D. A., & Pereira, F. C. (2020). Open-ended versus closed-ended responses: A comparison study using topic modeling and factor analysis. IEEE Transactions on Intelligent Transportation Systems, 22 (4), 2123–2132. https://doi.org/10.1109/TITS.2020.3040904
Article Google Scholar
Baburajan, V., e Silva, J. D. A., & Pereira, F. C. (2022). Open vs closed-ended questions in attitudinal surveys–Comparing, combining, and interpreting using natural language processing. Transportation Research Part C: Emerging Technologies, 137 , 103589. https://doi.org/10.1016/j.trc.2022.103589
Bansak, K., Hainmueller, J., & Hangartner, D. (2016). How economic, humanitarian, and religious concerns shape European attitudes toward asylum seekers. Science, 354 (6309), 217–222. https://doi.org/10.1126/science.aag2147
Article PubMed Google Scholar
Blackburn, K. G., Yilmaz, G., & Boyd, R. L. (2018). Food for thought: Exploring how people think and talk about food online. Appetite, 123 , 390–401. https://doi.org/10.1016/j.appet.2018.01.022
Boyd, R. L. (2019). MEH: Meaning Extraction Helper (Version 2.1.07) [Software]. Retrieved January 27, 2019, from https://meh.ryanb.cc
Boyd, R. L., & Schwartz, H. A. (2020). Natural language analysis and the psychology of verbal behavior: The past, present, and future states of the field. Journal of Language and Social Psychology, 40 (1), 21–41. https://doi.org/10.1177/0261927X20967028
Article PubMed PubMed Central Google Scholar
Caracelli, V. J., & Greene, J. C. (1993). Data analysis strategies for mixed-method evaluation designs. Educational Evaluation and Policy Analysis, 15 (2), 195–207. https://doi.org/10.3102/01623737015002195
CBOS. (2018). Stosunek Polaków i Czechów do przyjmowania uchodźców. Komunikat z badań nr 87/2018 [Attitudes of Poles and Chechs towards accepting refugees. Research report no 87/2018]. Retrieved January 24, 2019, from https://www.cbos.pl/SPISKOM.POL/2018/K_087_18.PDF
Chung, C. K., & Pennebaker, J. W. (2008). Revealing dimensions of thinking in open-ended self-descriptions: An automated meaning extraction method for natural language. Journal of Research in Personality, 42 (1), 96–132. https://doi.org/10.1016/j.jrp.2007.04.006
Connor Desai, S., & Reimers, S. (2019). Comparing the use of open and closed questions for Web-based measures of the continued-influence effect. Behavior Research Methods, 51 (3), 1426–1440. https://doi.org/10.3758/s13428-018-1066-z
Creswell, J. W., & Poth, C. N. (2016). Qualitative inquiry and research design: Choosing among five approaches . Sage.
Google Scholar
Creswell, J. W., Plano Clark, V. L., Gutmann, M. L., & Hanson, W. E. (2003). Advanced mixed methods research designs. In A. Tashakkori & C. Teddlie (Eds.), Handbook of mixed methods in social and behavioral research (pp. 209–240). Sage.
Croucamp, C. J., O’connor, M., Pedersen, A., & Breen, L. J. (2017). Predicting community attitudes towards asylum seekers: A multi-component model. Australian Journal of Psychology, 69 (4), 237–246. https://doi.org/10.1111/ajpy.12149
Eisnecker, P., & Schupp, J. (2016). Flüchtlingszuwanderung: Mehrheit der Deutschen befürchtet negative Auswirkungen auf Wirtschaft und Gesellschaft [Influx of refugees: Most Germans fear negative effects on the economy and society]. DIW-Wochenbericht, 83 , 158–164.
Esses, V. M., Medianu, S., & Lawson, A. S. (2013). Uncertainty, threat, and the role of the media in promoting the dehumanization of immigrants and refugees. Journal of Social Issues, 69 (3), 518–536. https://doi.org/10.1111/josi.12027
Faul, F., Erdfelder, E., Lang, A. G., & Buchner, A. (2007). G* Power 3: A flexible statistical power analysis program for the social, behavioral, and biomedical sciences. Behavior Research Methods, 39 (2), 175–191. https://doi.org/10.3758/BF03193146
Feezell, J. T. (2018). Agenda setting through social media: The importance of incidental news exposure and social filtering in the digital era. Political Research Quarterly, 71 (2), 482–494. https://doi.org/10.1177/1065912917744895
Fetters, M. D., Curry, L. A., & Creswell, J. W. (2013). Achieving integration in mixed methods designs – Principles and practices. Health Services Research, 48 (6pt2), 2134–2156. https://doi.org/10.1111/1475-6773.12117
Forman, J., Creswell, J. W., Damschroder, L., Kowalski, C. P., & Krein, S. L. (2008). Qualitative research methods: Key features and insights gained from use in infection prevention research. American Journal of Infection Control, 36 (10), 764–771. https://doi.org/10.1016/j.ajic.2008.03.010
Frew, E. J., Whynes, D. K., & Wolstenholme, J. L. (2003). Eliciting willingness to pay: Comparing closed-ended with open-ended and payment scale formats. Medical Decision Making, 23 (2), 150–159. https://doi.org/10.1177/0272989X03251245
Geer, J. G. (1991). Do open-ended questions measure “salient” issues? Public Opinion Quarterly, 55 (3), 360–370. https://doi.org/10.1086/269268
Gera, V. (2015). Right-wing Polish leader: Migrants carry diseases to Europe. Associated Press. https://apnews.com/article/5da3f41c4d924be0a2434900649dd0e6 . Accessed 10 Mar 2022.
Haddock, G., & Zanna, M. P. (1998). On the use of open-ended measures to assess attitudinal components. British Journal of Social Psychology, 37 (2), 129–149. https://doi.org/10.1111/j.2044-8309.1998.tb01161.x
Hutto, C., & Gilbert, E. (2014). VADER: A parsimonious rule-based model for sentiment analysis of social media text. Proceedings of the International AAAI Conference on Web and Social Media , 8 (1), 216–225. https://doi.org/10.1609/icwsm.v8i1.14550
Ikizer, E. G., Ramírez-Esparza, N., & Boyd, R. L. (2019). # sendeanlat (# tellyourstory): Text analyses of tweets about sexual assault experiences. Sexuality Research and Social Policy, 16 (4), 463–475. https://doi.org/10.1007/s13178-018-0358-5
Janz, A., Kocoń, J., Piasecki, M., & Zaśko-Zielińska, M. (2017). plWordNet as a basis for large emotive lexicons of Polish. Proceedings of human language technologies as a challenge for computer science and linguistics (pp. 189–193) . Retrieved July 25, 2023, from http://ltc.amu.edu.pl/book2017/papers/SEM1-2.pdf
Kaproń-Charzyńska, I. (2014). Pragmatyczne aspekty słowotwórstwa. Funkcja ekspresywna i poetycka [The pragmatic aspects of word formation. The expressive and poetic function]. Mikołaj Kopernik University Press.
Klocker, N. (2004). Community antagonism towards asylum seekers in Port Augusta, South Australia. Australian Geographical Studies, 42 (1), 1–17. https://doi.org/10.1111/j.1467-8470.2004.00239.x
Kotzur, P. F., Schäfer, S. J., & Wagner, U. (2019). Meeting a nice asylum seeker: Intergroup contact changes stereotype content perceptions and associated emotional prejudices, and encourages solidarity-based collective action intentions. British Journal of Social Psychology, 58 (3), 668–690. https://doi.org/10.1111/bjso.12304
Krosnick, J. A. (1999). Survey research. Annual Review of Psychology, 50 (1), 537–567. https://doi.org/10.1146/annurev.psych.50.1.537
Maison, D., & Jasińska, S. (2017). Polacy na temat imigrantów Raport z badania ilościowego przeprowadzonego dla ZPP [Polish people on immigrants: A report from a quantitative study conducted for ZPP]. Retrieved May 4, 2017, from https://zpp.net.pl/wpcontent/uploads/2017/04/file-47b4a440754751a4b451b45461394605.pdf
Maziarz, M., Piasecki, M., & Szpakowicz, S. (2013). The chicken-and-egg problem in wordnet design: synonymy, synsets and constitutive relations. Language Resources and Evaluation, 47 (3), 769–796. https://doi.org/10.1007/s10579-012-9209-9
Onwuegbuzie, A. J., & Teddlie, C. (2003). A framework for analyzing data in mixed methods research. In A. Tashakkori & C. Teddlie (Eds.), Handbook of mixed methods in social and behavioral research (pp. 351–383). Sage.
Pennebaker, J. W., Boyd, R. L., Jordan, K., & Blackburn, K. (2015). The development and psychometric properties of LIWC2015 . University of Texas at Austin.
Plutchik, R. (1980). A general psychoevolutionary theory of emotion. In R. Plutchik & H. Kellerman (Eds.), Emotion: Theory, research, and experience. Volume 1: Theories of emotion (pp. 3–33). Academic.
Polsat News. (2017). Niemcy uruchamiają nowy program dla obcokrajowców. Dadzą imigrantom pieniądze za powrót do domu [Germany launches a new program for foreigners. They will give immigrants money to return home]. https://www.polsatnews.pl/wiadomosc/2017-01-23/niemcy-uruchamiaja-nowy-program-dla-obcokrajowcow-dadza-imigrantom-pieniadze-za-powrot-do-domu/ . Accessed 3 Jul, 2023.
Preston, C. C., & Colman, A. M. (2000). Optimal number of response categories in rating scales: Reliability, validity, discriminating power, and respondent preferences. Acta Psychologica, 104 (1), 1–15. https://doi.org/10.1016/S0001-6918(99)00050-5
Puzynina, J. (1992). Język wartości [Language of values]. Wydawnictwo Naukowe PWN.
Rafaeli, A., Ashtar, S., & Altman, D. (2019). Digital traces: New data, resources, and tools for psychological-science research. Current Directions in Psychological Science, 28 (6), 560–566. https://doi.org/10.1177/0963721419861410
Ramirez-Esparza, N., Chung, C., Kacewic, E., & Pennebaker, J. (2008). The psychology of word use in depression forums in English and in Spanish: Testing two text analytic approaches. Proceedings of the International AAAI Conference on Web and Social Media , 2 (1), 102–108. Retrieved March 3, 2022, https://ojs.aaai.org/index.php/ICWSM/article/view/18623
Schwarz, N., Hippler, H. J., Deutsch, B., & Strack, F. (1985). Response scales: Effects of category range on reported behavior and comparative judgments. Public Opinion Quarterly, 49 (3), 388–395. https://doi.org/10.1086/268936
Solska, J. (2017). Prof. Bilewicz o tym, dlaczego Polacy tak się boją uchodźców [Prof. Bilewicz about why Poles are so scared of the refugees]. Polityka. https://www.polityka.pl/tygodnikpolityka/spoleczenstwo/1706622,2,prof-bilewicz-o-tym-dlaczego-polacy-tak-sie-boja-uchodzcow.read . Accessed 10 Mar, 2022.
Stefaniak, A., Malinowska, K., & Witkowska, M. (2017). Kontakt międzygrupowy i dystans społeczny w Polskim Sondażu Uprzedzeń 3 [Intergroup contact and social distance in Polish Prejudice Survey 3]. Retrieved August 16, 2019, from http://cbu.psychologia.pl/wp-content/uploads/sites/410/2021/02/Stefaniak_Malinowska_Witkowska_DystansKontakt.pdf
Tashakkori, A., & Teddlie, C. (1998). Mixed methodology: Combining qualitative and quantitative approaches (vol. 46). Sage
Tausczik, Y. R., & Pennebaker, J. W. (2010). The psychological meaning of words: LIWC and computerized text analysis methods. Journal of Language and Social psychology, 29 (1), 24–54. https://doi.org/10.1177/0261927X0935167
TVN24. (2017). Jak każdy z nas może pomóc uchodźcom – przewodnik [How each of us can help refugees - a guide]. https://tvn24.pl/polska/jak-mozna-pomoc-uchodzcom-lista-organizacji-ra750709-2480249 . Accessed 3 Jul, 2023.
Wagner, W., Hansen, K., & Kronberger, N. (2014). Quantitative and qualitative research across cultures and languages: Cultural metrics and their application. Integrative Psychological and Behavioral Science, 48 (4), 418–434. https://doi.org/10.1007/s12124-014-9269-z
Wike, R., Stokes, B., & Simmons, K. (2016). Europeans fear wave of refugees will mean more terrorism, fewer jobs . Retrieved February 15, 2022, from https://www.politico.eu/wp-content/uploads/2016/07/Pew-Research-Center-EU-Refugees-and-National-Identity-Report-EMBARGOED-UNTIL-1800EDT-2200GMT-July-11-2016.pdf
Zaśko-Zielińska, M., Piasecki, M., & Szpakowicz, S. (2015). A large wordnet-based sentiment lexicon for Polish. Proceedings of the international conference recent advances in natural language processing (pp. 721–730). Retrieved July 25, 2023, from https://aclanthology.org/R15-1092.pdf
Download references
Acknowledgments
We thank Anna Fokina and Maksymilian Kropiński for their help in the manual coding of the open-ended answers, and Maciej Piasecki, Arkadiusz Janz, and others from the CLARIN team for their help with sentiment analysis. We are also grateful to Mikołaj Winiewski for supporting the data collection and to Sabina Toruńczyk-Ruiz for critical comments on an earlier draft of this manuscript.
This work was supported by the Faculty of Psychology, University of Warsaw, from the funds awarded by the Ministry of Science and Higher Education in the form of a subsidy for the maintenance and development of research potential in 2018 awarded to KH and AŚ, and in 2022 awarded to KH (501-D125-01-1250000 zlec. 5011000235) and AŚ (501-D125-01-1250000 zlec. 5011000619). Data collection for Study 2 was supported by the NCN Opus grant (2014/13/B/HS6/04077) awarded to Mikołaj Winiewski.
Author information
Authors and affiliations.
Faculty of Psychology, University of Warsaw, Stawki 5/7, 00-183, Warsaw, Poland
Karolina Hansen & Aleksandra Świderska
You can also search for this author in PubMed Google Scholar
Contributions
Both authors (KH and AŚ) contributed to all stages of the research: conceptualization, methodology, data curation, data analysis, and manuscript writing.
Corresponding author
Correspondence to Karolina Hansen .
Ethics declarations
Ethics approval.
The studies were performed in line with the principles of the Declaration of Helsinki. Approval was granted by the Ethics Committee of the Faculty of Psychology, University of Warsaw (decisions 5.03.2019 and 21.05.2019).
Consent to participate
Informed consent was obtained from all individual participants included in the studies.
Consent for publication
Not applicable. All studies were fully anonymous and there is no identifying information about the participants, either in the article or in the datasets. Participants consented to the use of their data for scientific purposes.
Conflict of interest
The authors have no competing interests to declare.
Additional information
Publisher's note.
Springer Nature remains neutral with regard to jurisdictional claims in published maps and institutional affiliations.
Rights and permissions
Open Access This article is licensed under a Creative Commons Attribution 4.0 International License, which permits use, sharing, adaptation, distribution and reproduction in any medium or format, as long as you give appropriate credit to the original author(s) and the source, provide a link to the Creative Commons licence, and indicate if changes were made. The images or other third party material in this article are included in the article's Creative Commons licence, unless indicated otherwise in a credit line to the material. If material is not included in the article's Creative Commons licence and your intended use is not permitted by statutory regulation or exceeds the permitted use, you will need to obtain permission directly from the copyright holder. To view a copy of this licence, visit http://creativecommons.org/licenses/by/4.0/ .
Reprints and permissions
About this article
Hansen, K., Świderska, A. Integrating open- and closed-ended questions on attitudes towards outgroups with different methods of text analysis. Behav Res 56 , 4802–4822 (2024). https://doi.org/10.3758/s13428-023-02218-x
Download citation
Accepted : 07 August 2023
Published : 16 October 2023
Issue Date : August 2024
DOI : https://doi.org/10.3758/s13428-023-02218-x
Share this article
Anyone you share the following link with will be able to read this content:
Sorry, a shareable link is not currently available for this article.
Provided by the Springer Nature SharedIt content-sharing initiative
- Closed-ended
- Natural language
- Text analysis
- Meaning extraction
- Sentiment analysis
- Find a journal
- Publish with us
- Track your research
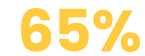
Yearly plans are up to 65% off for a limited Black Friday sale. ⏰
- Form Builder
- Survey Maker
- AI Form Generator
- AI Survey Tool
- AI Quiz Maker
- Store Builder
- WordPress Plugin

HubSpot CRM

Google Sheets

Google Analytics

Microsoft Excel

- Popular Forms
- Job Application Form Template
- Rental Application Form Template
- Hotel Accommodation Form Template
- Online Registration Form Template
- Employment Application Form Template
- Application Forms
- Booking Forms
- Consent Forms
- Contact Forms
- Donation Forms
- Customer Satisfaction Surveys
- Employee Satisfaction Surveys
- Evaluation Surveys
- Feedback Surveys
- Market Research Surveys
- Personality Quiz Template
- Geography Quiz Template
- Math Quiz Template
- Science Quiz Template
- Vocabulary Quiz Template
Try without registration Quick Start
Read engaging stories, how-to guides, learn about forms.app features.
Inspirational ready-to-use templates for getting started fast and powerful.
Spot-on guides on how to use forms.app and make the most out of it.

See the technical measures we take and learn how we keep your data safe and secure.
- Integrations
- Help Center
- Sign In Sign Up Free
- Open-ended questions: Tips, types & 35+ question examples
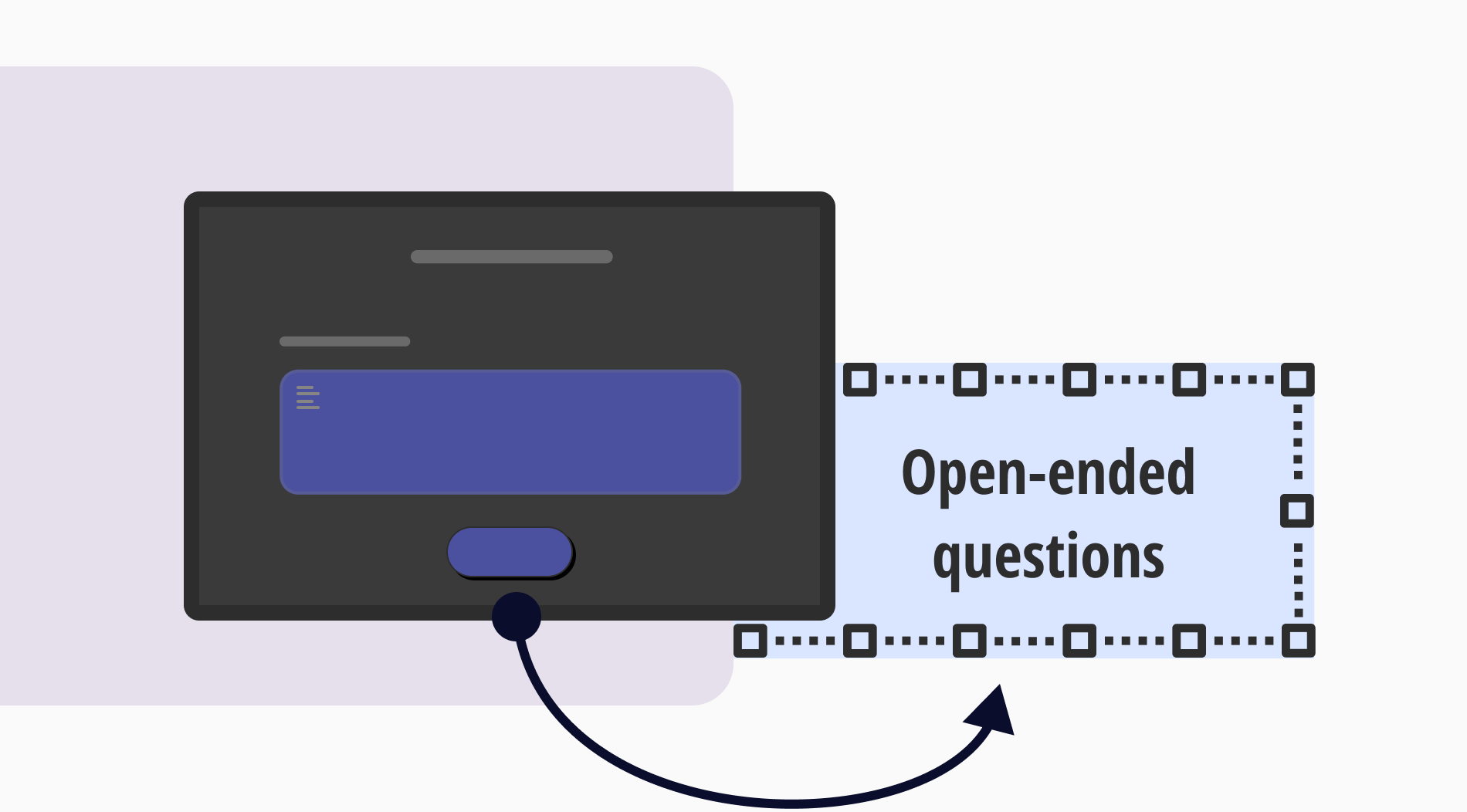
Ayşegül Nacu
Open-ended questions are powerful tools for gaining deeper insights into a specific topic. Open-ended questions, unlike closed-ended questions, which frequently elicit simple or brief replies, encourage deliberate and extensive responses . This can lead to an exchange of ideas between the survey creator and the survey taker.
Open-ended questions encourage critical thinking and creativity as well. They encourage people to think from different perspectives. By embracing the potential of open-ended questioning, people can obtain precious insights into relevant matters. These questions push the envelope of conventional knowledge and stoke the flame of creativity.
By reading this guide article for open-ended questions, you can expect to discover various tips for open-ended questions. Additionally, this article will likely offer practical examples to illustrate the effectiveness of them.
- What is an open-ended question?
Open-ended questions are thought-provoking questions to be answered in detail. These types of questions allow respondents to answer in the scope of their complete knowledge. Whether used in research, surveys, or interviews , open-ended questions provide a chance for discovery .
Survey creators benefit greatly from open-ended questions. Also, survey takers are more likely to feel valued when they are given the option to express their ideas in their own words. By allowing respondents to share their thoughts, experiences, and opinions with good open-ended questions, survey designers can create a more inclusive survey experience.
Types of open-ended questions
When seeking comprehensive and meaningful information, open-ended questions play a crucial role. In this section, we will explore diverse types of open-ended question types applicable across various contexts. By comprehending the distinct types of open-ended questions, you can customize your approach to extract the desired insights and responses from participants.
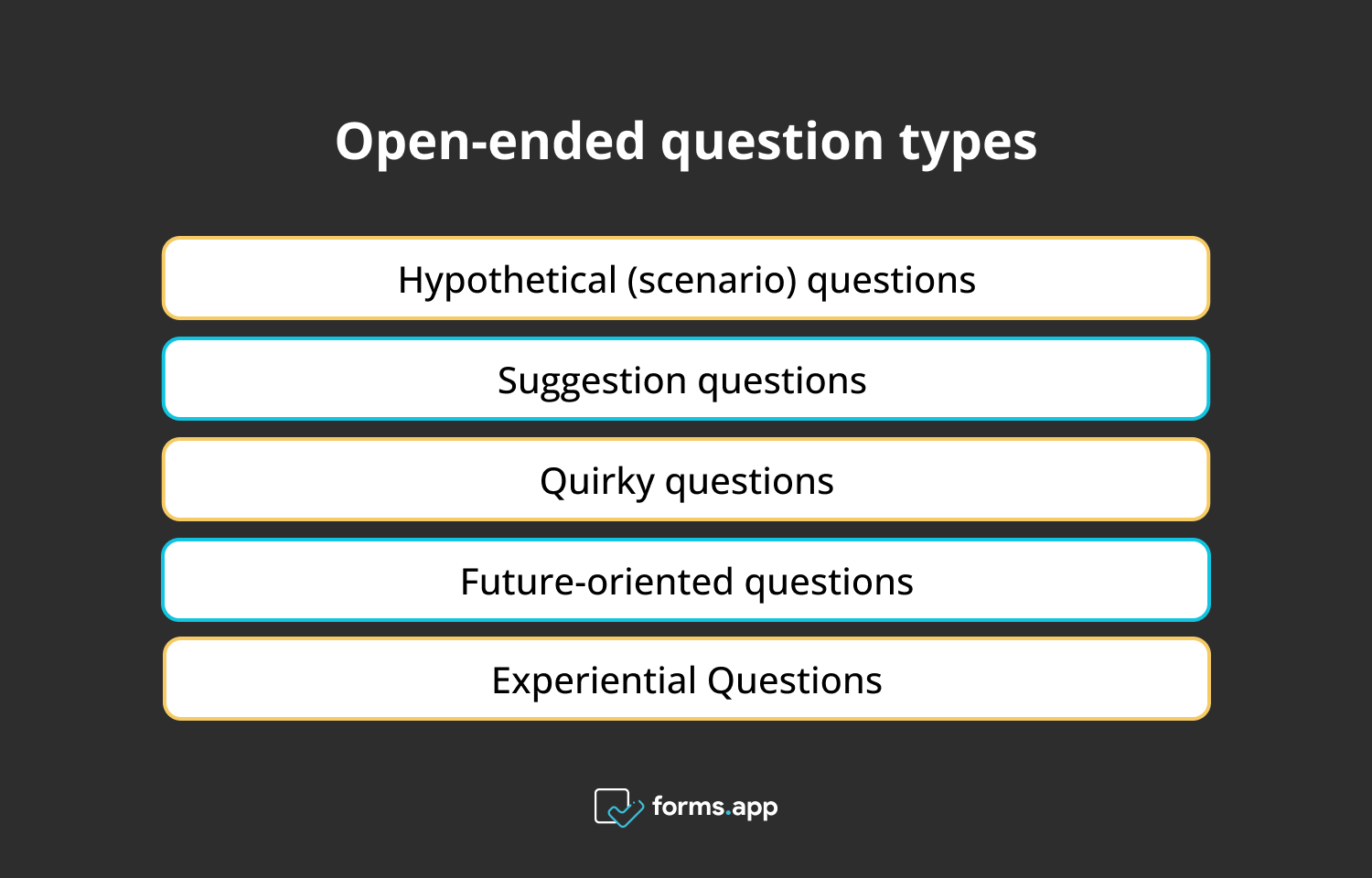
Open-ended question types
Every type of open-ended question has a specific function. Some are designed for hypothetical situations that stimulate critical thinking, while others are suggestion questions that inspire creativity, build rapport , and foster an engaging atmosphere.
- Hypothetical (scenario) questions: Hypothetical questions, also called as scenario questions provide the survey taker a fictional circumstance or scenario to ponder probable outcomes, viewpoints, or actions.
- Suggestion questions: These questions are designed to elicit creative and innovative responses. In suggestion questions, survey takers can express their genuine ideas freely.
- Quirky questions: Quirky open-ended questions can be used in icebreaker activities, team-building exercises, or just for fun! These types of questions improve the sense of humor.
- Future-oriented questions: Respondents speculate about future developments or possibilities when answering future-oriented questions. These questions encourage forward-thinking.
- Experiential Questions: These types of questions invite participants to share their personal experiences or stories in detail related to a particular theme or subject.
- Why and when do you need an open-ended question?
When you want to go further into a subject and learn more about it in depth, open-ended questions are essential. In circumstances when you want to collect extensive qualitative data , you might need open-ended questions. By encouraging participants to think broadly, these questions generate fruitful answers.
In general, open-ended questions are necessary when you want to go deeply into a subject , collect qualitative data and support creativity. We can say that open-ended questions are particularly useful in this regard. To sum up, open-ended questions offer versatile opportunities for usage across various contexts and purposes. Open-ended questions for surveys facilitate as a valuable tool in numerous fields.
- 35+ Open-ended question examples for your next form or survey
As the forms.app team, we are aware of the importance of gathering valuable data. In this section, we compiled 35+ open-ended questions in research examples to help you achieve this. You can improve your experiences and understanding of your target audience by getting inspired by these sample questions.
forms.app offers different question formats, such as long text, short text, and masked texts. You can utilize these question types to get data in the way you need.
Open-ended questions for research
Research open-ended questions are very useful in qualitative research approaches like focus groups and interviews. By facilitating a deeper exploration of the research topic, these questions help researchers probe further.
1. Can you explain any contradictions or inconsistencies you observed within the research topic? (Long text)
2. In your opinion, what are the long-term effects of the research project? (Long text)
3. Can you suggest areas of further research or exploration? (Short text)
4. What do you perceive as the main challenge of the research? (Short text)
5. How do you think cultural and environmental factors influence the study? (Long text)
6. Did the language of the research report affect your understanding? Why? (Long text)
7. What are the approaches or strategies employed during the research? (Short text)
Open-ended questions for sales
In the field of sales, open-ended questions are crafted to encourage customers to express their opinions . In this way, sales professionals gain access to valuable information and identify specific troubles.
8. What aspect of our product or service's potential excites you? (Short text)
9. Can you provide insights into our internal sales initiatives? (Long text)
10. What types of sales strategies do you want to see in the next year? (Long text)
11. What, in your opinion, is the most efficient means of fostering enduring relationships with customers? (Short text)
12. How do you measure and track your sales performance? (Long text)
13. Do you think social media affects your sales? If so, can you please indicate whether this effect is positive or negative? (Short text)
14. How do you evaluate the return on investment (ROI) for your work? (Long text)
Open-ended questions for customer surveys
Open-ended questions for customer surveys aim at nuanced customer feedback. In this way, companies can make informed decisions to enhance the customer experience and drive business growth.
15. Do you appreciate the communication within our company? Why? (Long text)
16. How would you recommend our product/service to others, and what specific value would you emphasize? (Long text)
17. If our product/service had a personality, how would you describe it? (Long text)
18. If you could change one thing about our product/service, what would it be? And why? (Long text)
19. Can you provide an example of a situation where our customer support team resolved a problem? (Short text)
20. What additional features or functionalities would you like to see in our product? (Short text)
21. Can you describe the relationship or connection you feel with our brand? (Long text)
Open-ended questions for employee surveys
Open-ended questions are important in capturing employee feedback. These types of questions are vital to take action to create a more positive and engaging work environment .
22. Can you describe a time when you felt your ideas or opinions were valued and included in the decision making process? (Long text)
23. How would you describe the company culture? (Long text)
24. Do you think your performance is effective in the company? Why? (Short text)
25. Between 1-10, how would you rate the level of trust and transparency between management and employees? ( Short text/Masked question type)
26. Can you share an example of a time when you felt genuinely recognized or appreciated for your work? (Long text)
27. If you could implement one change to enhance teamwork, what would it be? (Short text)
28. What factors contribute to your motivation? (Long text)
You can add a comment field to multiple-choice questions; thus, these questions function as open-ended questions.
Open-ended questions for friends
Open-ended questions can help you and your friends connect in meaningful ways and gain a deeper knowledge of one another. The introductory questions below are an excellent way to start if you want to strengthen your connection.
29. What is your favorite way to unwind and relax after a long day? (Short text)
30. If you could trade lives with any fictional character for a day, who would it be and why? (Long text)
31. What is your biggest fear, and how do you overcome it? (Long text)
32. What is your favorite ice cream topping? (Short text)
33. If you could have a humorous sound effect follow you wherever you go, what sound effect would it be, and when would it play? (Long text)
34. What is the silliest nickname you've ever had or given to someone else? (Short text)
35. What is one valuable lesson you've learned from your friendships? (Short text)
The masked text is intended to aid you if you want to collect responses in a particular way.
- How to ask open-ended questions on forms.app
Utilizing the capabilities and adaptability of forms.app, you can create forms that invite thorough and insightful input from your audience. By incorporating open-ended questions, you will enable your survey takers to reveal their detailed thoughts . In this section, we will provide guidance on how you can easily create forms with open-ended questions in forms.app.
1 - Sign in to your forms.app account or create a new account in case you don't have an account already.
2 - Choose a template or start from scratch.
3 - Select from open-ended question types such as long text and short text.
4 - Customize the question by clicking on the added field.
5 - Make sure the open-ended questions on your form are working properly by testing them using the preview option.
6 - Make your form's appearance and layout specific to your company's identity or the subject of your survey.
7 - Publish and distribute your form with your audience whenever you are satisfied with it via a variety of means, including email, social media, and embedding it on your website.
Encourage them to tell stories by posing questions that are compelling. Respondents will describe their encounters in depth.
- Open-ended questions vs. close-ended questions
Even though both open-ended and close-ended questions can be used in surveys for analysis, it is really important to differentiate the two types. In order to improve your surveys in this respect, we have compared the two types in detail in the table below.
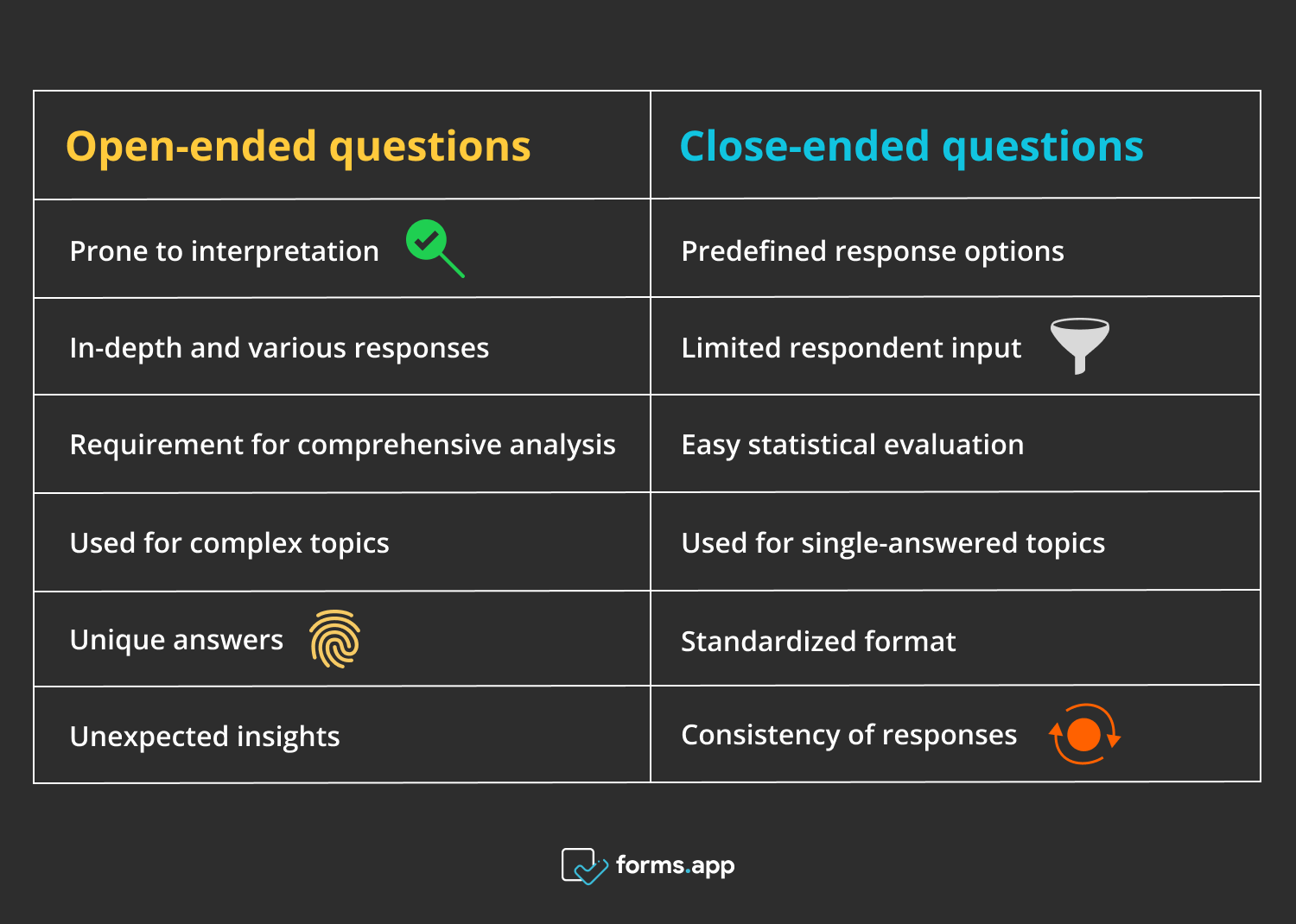
The comparison of open-ended and close-ended questions
- Key points to take away
The power of open-ended questions lies in their ability to go beyond restricted answers. With the help of open-ended questions, people will unlock richer insights regarding the topic.
- Give survey takers sufficient time: It's vital to allow survey takers enough time to think and respond when conducting surveys, including open-ended questions so that they can provide meaningful answers.
- Demonstrate open-mindedness: Avoid biased notions and provide assurance that there will be no misunderstandings and that the questionnaire will be handled objectively.
- Begin questions with "how," "what," and "why”: These questions will result in descriptive responses and prompt survey takers to elaborate.
- Follow up on the answers: Following up on respondents' answers demonstrates how much value you put on their ideas.
- Use symbolic representation: Utilizing metaphors, symbols, or imagery in your questions allows you to attract participants' interest and elicit more intense feelings or profound insights.
To promote survey participation and boost response rates, think about providing incentives or awards.
In conclusion, open-ended questions are crucial when attempting to investigate a subject, collect substantial qualitative data , and encourage participants to think critically. They are a valuable resource for an in-depth comprehension of a subject or phenomenon and for developing complete perspectives.
Open-ended questions can be a valuable tool for researchers, companies, and organization s to build unique perspectives and a comprehensive understanding of complicated events. Start learning more about forms.app's features today to realize the full potential of open-ended survey questions!
Ayşegül is a content writer at forms.app and a full-time translation project manager. She enjoys scrapbooking, reading, and traveling. With expertise in survey questions and survey types, she brings a versatile skill set to her endeavors.
- Form Features
- Data Collection
Table of Contents
Related posts.
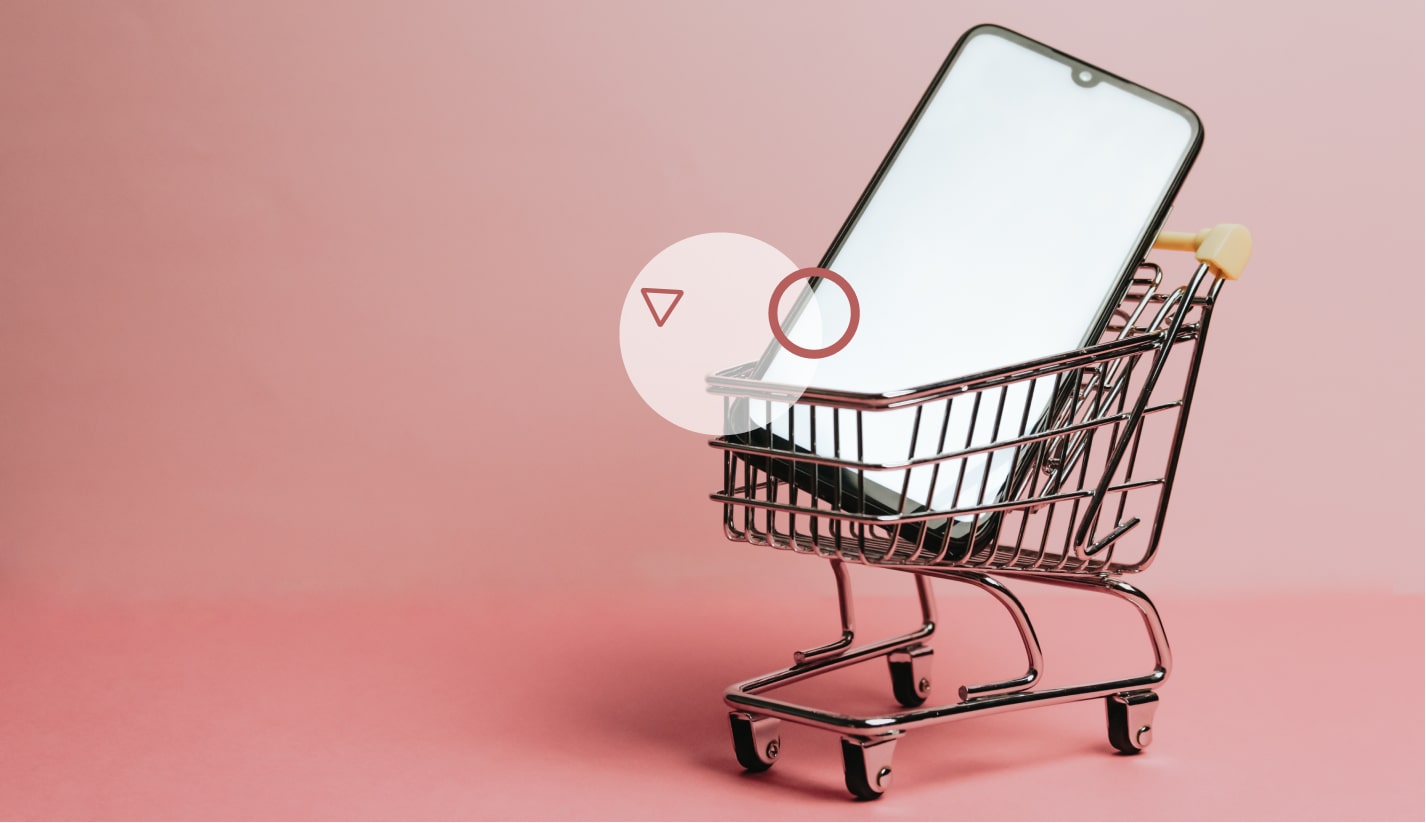
55 Amazing eCommerce survey questions for your business
Eren Eltemur
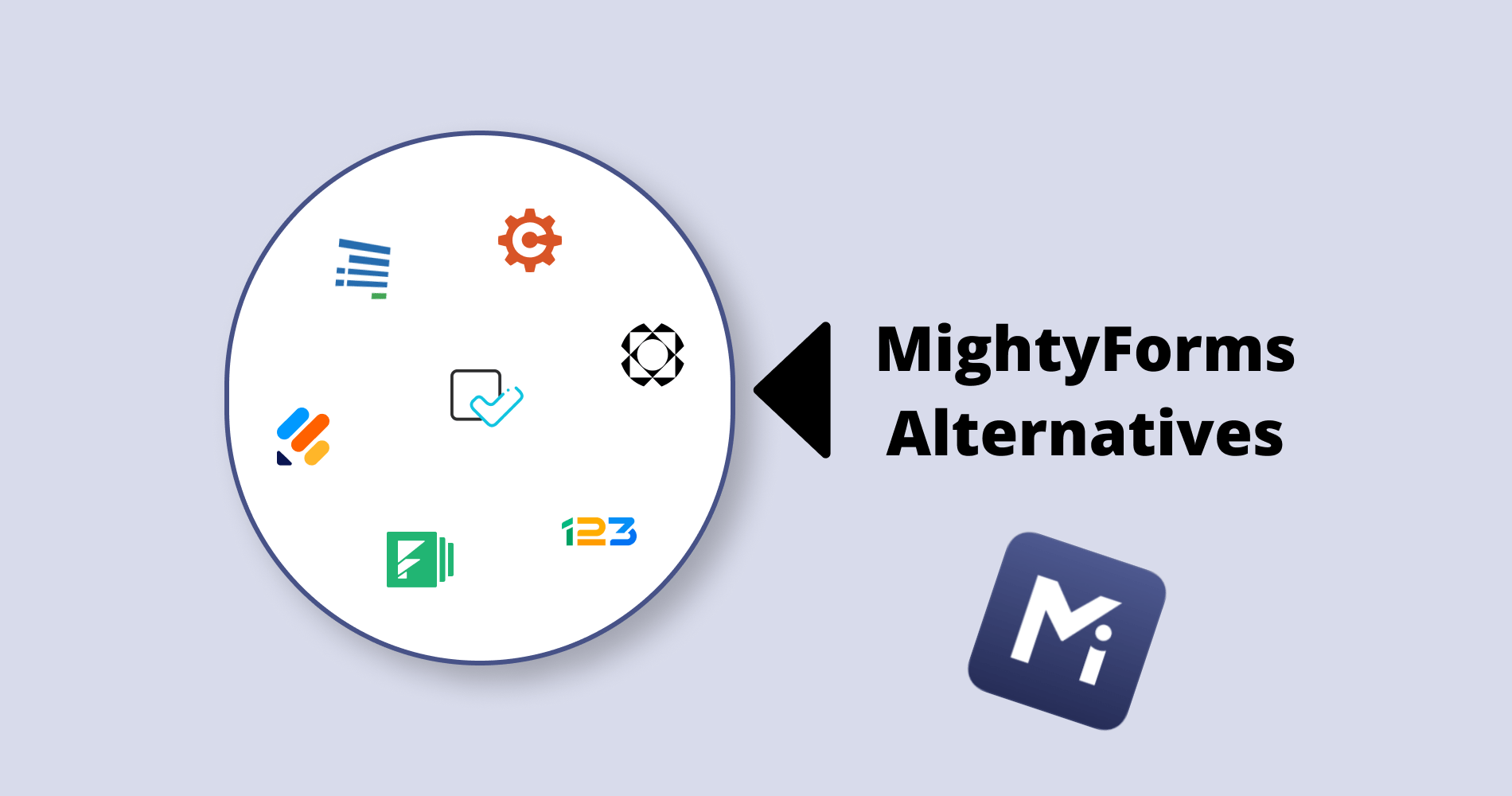
7 Best MightyForms alternatives to try in 2024
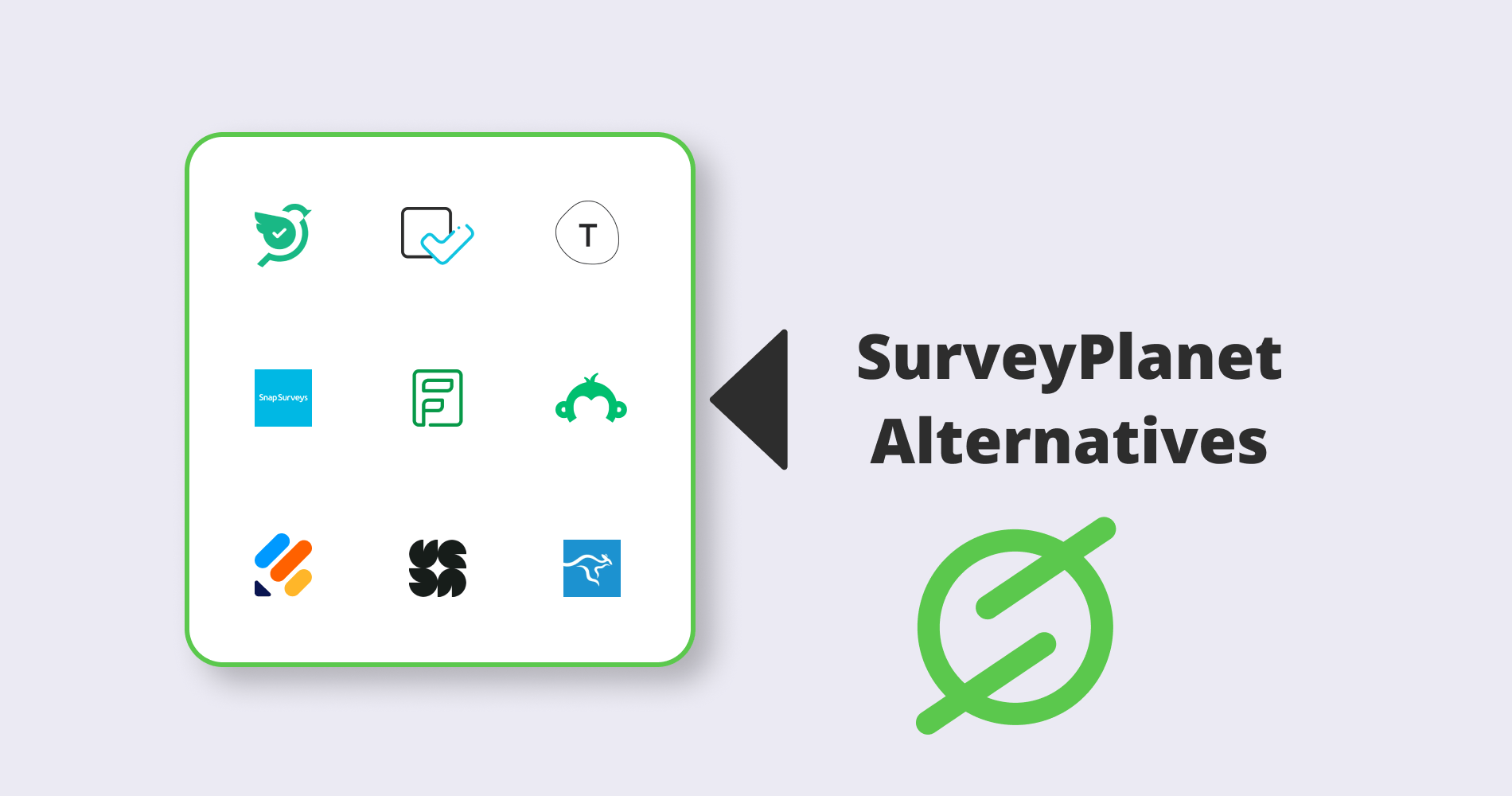
11 Great alternatives to SurveyPlanet in 2024 (pros & cons)
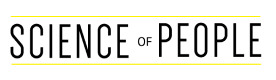
Open Ended Questions: 25 Examples for Customer Research
Unlock deeper insights with 30 open-ended questions for customer research. Learn how to use them effectively for rich feedback.
Make better business decisions
Pull data from any source
30 x faster analysis
Unrivalled customer insights
Australia's #1 Feedback Analytics platform
Imagine you've just completed the design of your customer survey. You've spent weeks crafting the perfect survey questions, and you're ready to launch.
But here's the catch – not all questions are created equal. While closed-ended questions provide straightforward, quantitative data, it's the open-ended questions that unlock deeper insights, revealing the true voice of your customers .
Open-ended questions are the secret sauce of effective customer research. They allow respondents to express their thoughts, feelings, and opinions in their own words, providing rich, detailed responses that paint a full picture of their experiences. In this article, we’ll dive into some open-ended question examples and explore why they are crucial for surveys, offering tips on how to use them effectively.
Table of Contents
What are open-ended questions, open-ended questions vs. closed-ended questions, 30 open-ended question examples, how to use open-ended questions for surveys and customer research.
Open-ended questions are those that cannot be answered with a simple "yes" or "no." Instead, they require respondents to provide more detailed and thoughtful answers. These questions typically start with words like "how," "what," "why," or "describe," inviting people to elaborate on their thoughts and feelings.
For example, instead of asking, "Did you find our product useful?" (a closed-ended question), you could ask, "What did you find most useful about our product?" This approach encourages respondents to provide specific feedback, offering deeper insights into their experiences and perceptions.
The importance of open-ended questions in research cannot be overstated. They allow you to:
Capture Detailed Responses: Open-ended questions provide rich, qualitative data that can reveal underlying motivations, emotions, and opinions.
Uncover Hidden Insights: By allowing respondents to express themselves freely, you can discover insights that you might not have anticipated.
Enhance Customer Understanding: These questions help you understand your customers' perspectives more deeply, enabling you to address their needs and improve their experience.
Understanding the difference between open-ended and closed-ended questions is crucial for designing effective surveys . Here's a quick comparison:
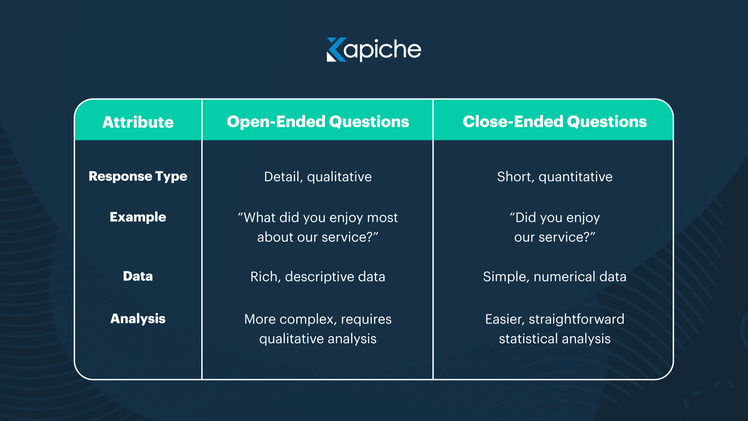
Closed-ended questions, such as those requiring "yes/no" or multiple-choice responses, are popular for their ease of analysis and quantifiable data. However, they often fall short in uncovering nuanced customer sentiments and detailed feedback. These questions tend to elicit a short, one-word answer. They tend to provide superficial data without delving into the underlying reasons or motivations behind respondents' choices.
Closed Versus Open Question Examples
For instance, consider a closed-ended question like, "Did you find our service satisfactory?" A respondent can answer with a simple "yes" or "no," providing little context or elaboration on what aspects of the service were satisfying or lacking. So rather than designing your survey for ease of analysis , it's best to swap your single-word answer with ones that will produce more detailed answers.
Let's break down three examples to illustrate this difference:
Satisfaction with Service:
Open-ended: "What aspects of our service did you find most satisfying?"
Closed-ended: "Were you satisfied with our service?" (Yes/No)
Product Feedback:
Open-ended: "How would you describe your experience with our product?"
Closed-ended: "Did you like our product?" (Yes/No)
Suggestions for Improvement:
Open-ended: "What suggestions do you have for improving our product?"
Closed-ended: "Would you like to see changes in our product?" (Yes/No)
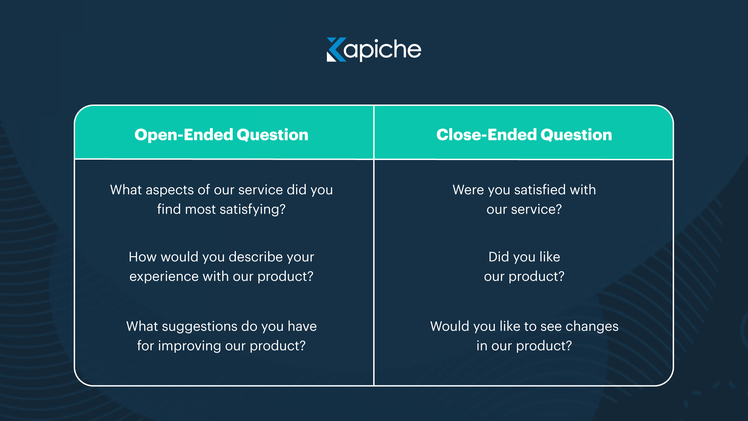
Open-ended questions encourage critical thinking and provide a depth of insight that closed-ended questions simply cannot match. They'll get you to the heart of your customer's perspective, offering a much more detailed response packed with nuances about their experiences and opinions.
Next, we'll dive into the heart of the article: examples of open-ended questions you can use in your surveys to gather valuable customer insights.
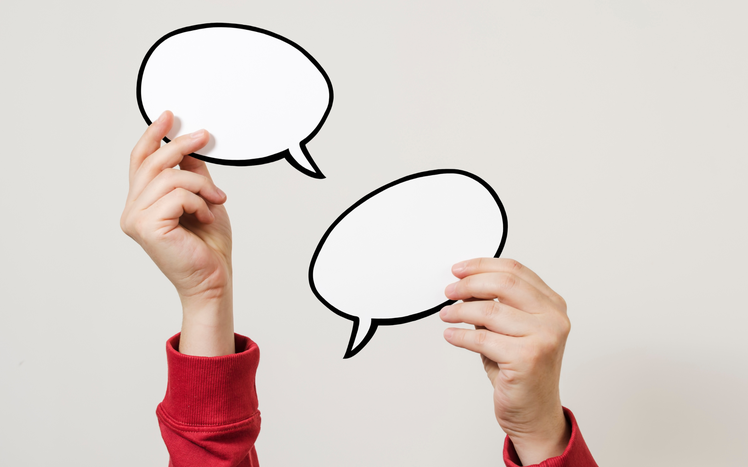
Open-ended questions are powerful tools for gathering detailed feedback and gaining a deeper understanding of your customers. Here are 30 examples of open-ended questions you can use in your surveys:
Customer Experience
These open-ended questions help gather detailed customer feedback on various touch points – from initial contact to post-purchase interactions. By asking about their customer experiences, you can identify strengths and themes for improvement with these in-depth responses.
"How would you describe your overall experience with our company?"
"What did you enjoy most about your experience with us?"
"How can we improve your next experience?"
Product Feedback
Product feedback questions are essential for understanding how customers perceive and use your products. Use these questions when you're looking to gather detailed opinions on product features, usability, and overall satisfaction. This feedback is invaluable for product development teams to make informed decisions about enhancements, new features, and addressing any issues that customers face.
"What features do you find most useful in our product?"
"What challenges did you face while using our product?"
"What improvements would you suggest for our product?"
Service Satisfaction
Service satisfaction questions focus on the quality and effectiveness of customer support and service interactions. These questions help you identify how well the service team is performing, with detailed insights on what improvements are needed. By understanding customer satisfaction with service, businesses can enhance their support processes, training, and overall customer service strategy.
"How would you describe the quality of our service?"
"What could we do to make our service more valuable to you?"
"Can you describe a recent interaction you had with our support team?"
Suggestions for Improvement
Gathering suggestions for improvement directly from customers provides actionable insights that can drive meaningful changes. These questions encourage customers to share their ideas and feedback on how your business can better meet their needs. Use this information when you're looking to prioritize enhancements that will have the most significant impact on customer satisfaction and business growth.
"What changes would you suggest for our product/service?"
"What additional features would you like to see?"
"How can we make your experience with us better?"
Customer Needs
Identifying and understanding customer needs is fundamental to delivering products and services that truly resonate with your audience. These questions help you uncover unmet needs and preferences, providing a clear direction for product development and marketing strategies.
"What problems are you hoping to solve with our product?"
"Are there any needs you have that our product/service does not currently meet?"
"What are your most important needs when using our service?"
Brand Perception
Understanding how customers perceive your brand is vital for shaping your brand strategy and positioning. These questions help you gather detailed feedback on brand attributes, strengths, and areas for improvement. With these insights around your brand sentiment , you'll be better equipped to head into brainstorming sessions with your team on how to refine your messaging, marketing efforts, and overall brand strategy to better resonate with your target audience.
"How would you describe our brand to a friend?"
"What comes to mind when you think of our company?"
"What words would you use to describe our brand?"
Competitor Comparison
Competitor comparison questions provide insights into how your business stacks up against others in the market. These questions help you better understand the competitive landscape from the customer's perspective. These insights are crucial for strategic planning, getting to the heart of your brand strengths and your weaknesses relative to competitors.
"How do we compare to other companies you have used?"
"What makes you choose us over other options available?"
"What do you think we do better or worse than our competitors?"
Purchase Decision
Understanding the factors that influence purchase decisions can help you refine your sales and marketing strategies. These questions dive into the motivations, concerns, and information needs of customers during the buying process. Use this feedback to optimize the customer journey, address barriers to purchase, and enhance the overall buying experience.
"What factors influenced your decision to purchase our product?"
"How did you first hear about us?"
"What concerns did you have before making your purchase?"
Customer Loyalty
Customer loyalty questions aim to uncover the reasons behind repeat business and long-term customer relationships. These questions help insights managers understand what drives loyalty and what might cause customers to switch to competitors. By identifying the key factors that contribute to loyalty, you can develop strategies to retain customers and foster long-term relationships.
"What keeps you coming back to us?"
"What would make you consider switching to another provider?"
"How likely are you to continue using our services?"
Overall Satisfaction
Overall satisfaction questions provide a broad view of how customers feel about your business and its offerings. These questions help you gauge general satisfaction levels and identify overarching themes in customer feedback. Understanding overall satisfaction is crucial for maintaining a positive customer relationship and ensuring that your business meets or exceeds customer expectations.
"How satisfied are you with your overall experience?"
"What could we do to improve your satisfaction?"
These examples illustrate the versatility and depth that open-ended questions can bring to your surveys. By using these questions, you can gather rich, qualitative data that provides a deeper understanding of your customers' thoughts, feelings, and experiences. To get the most out of your research efforts, make sure you have a customer experience dashboard set up that centralizes your data and helps you interpret themes and findings quickly.
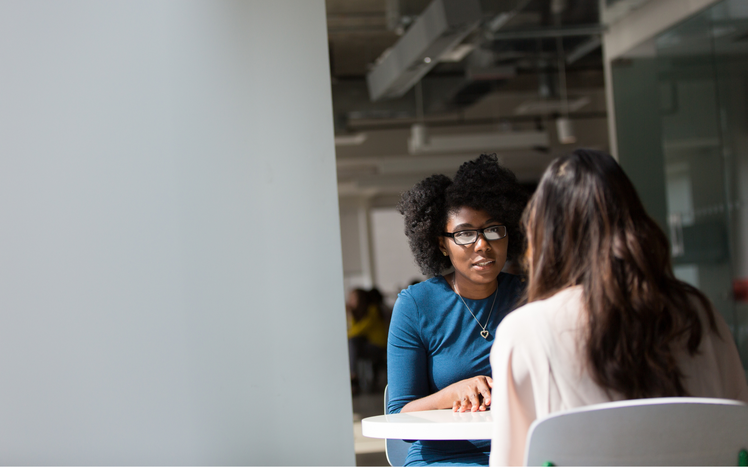
Open-ended questions play a crucial role in effective survey design and customer research. Here are some tips on how to best use them in your survey strategy:
Mix with Closed-Ended Questions: Combining open-ended and closed-ended questions can provide a balanced view and rich insights. Use closed-ended questions for quick insights and open-ended ones for detailed feedback.
Use Probing Questions: Use follow-up questions in your survey sequence to encourage respondents to elaborate on their answers. For example, "Can you tell me more about that?"
Analyze Responses Thoroughly: Qualitative data analysis tools, like Kapiche , can help you make sense of the responses. Look for common themes, patterns, and sentiments.
Encourage Honest Feedback: Assure respondents that their feedback is valuable and will be used to improve products or services. This can lead to more thoughtful and honest responses.
Keep Questions Relevant: Ensure that each question is relevant to your research objectives. Irrelevant questions can lead to disengaged respondents and lower-quality data.
Open-ended questions are invaluable for gaining a deeper understanding of your customers. By using them effectively, you can uncover insights that drive meaningful improvements and foster stronger customer relationships.
Effective survey design starts with asking the right questions. At Kapiche, we understand the power of open-ended questions in uncovering valuable customer insights. Our feedback analytics platform is designed to analyze unstructured data from open-ended responses, helping you uncover trends, sentiments, and actionable insights effortlessly.
Ready to transform your surveys with insightful open-ended questions? Discover how Kapiche can elevate your customer research efforts and drive meaningful improvements in your business. Watch an on-demand demo today and see how our platform can turn raw feedback into actionable intelligence.
You might also like
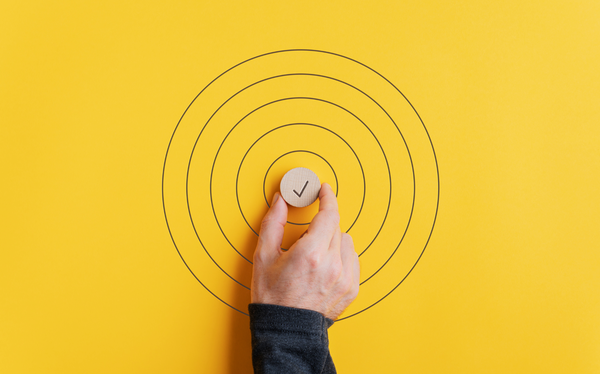
Product Updates
- Polling l Q&A l Quiz Tips
Higher Education
- Employee Training
- Internal Comms
Open-ended questions for 100% engagement
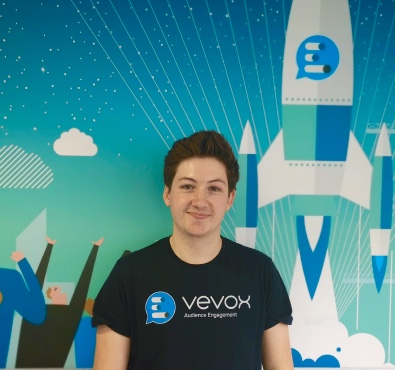
Open-ended questions can be a powerful tool to instigate critical thinking and invite discussions. By encouraging more than just yes-or-no answers, they help uncover deeper insights into the thoughts and feelings of an audience. Whether you're a business leader, educator, or team member, understanding how to leverage open-ended questions can positively improve your interactions and decision-making processes. Let's dive in to what open ended questions are and how you can effectively use them in classes and meetings.
What are open-ended questions and why use them?
Open-ended questions are designed to elicit detailed and thoughtful responses. They typically begin with "why," "how," or "what," and are an essential component of effective communication strategies, providing depth to discussions and feedback surveys.
Unlike closed-ended questions, which might limit responses to simple "yes" or "no" answers or a set of predetermined choices, open-ended questions invite elaboration. This allows the respondent to fully express themselves, providing valuable insights that might otherwise be missed. Open-ended questions creates a space for critical thinking with no right or wrong answers and it's a chance to spark sensible debates on a topic.
Crafting the perfect open-ended questions
Creating impactful open-ended questions requires thoughtful consideration. Aim for questions that invoke genuine interest and curiosity. This will elicit meaningful responses. Use verbs that evoke thought and emotion, such as "think," "feel," or "believe," to encourage students to provide more elaborate and considered answers.
While "why" questions can be insightful, they may also trigger defensiveness. Instead, focus on asking "what" or "how" to get to the root of decisions and opinions without putting the respondent on the spot. Using an anonymous Q&A or feedback tool can also be a chance to collate all of these answers to the questions in a safe space before discussing these verbally in a class setting.
Examples of open-ended questions for different scenarios and use cases
To demonstrate their versatility, we have provided a few examples of how open-ended questions can be tailored for various settings. From corporate setting to educational settings, these types of questions can be used in various ways to stimulate discussion on a topic, as icebreakers or to help promote better decision making within a group. Why not run open-ended questions with a word cloud poll ?
Open-ended questions for team meetings
- "How can we increase the effectiveness of our collaboration?"
- "What aspects of our next project excites you the most and why?"
- "How do you feel you can contribute to team projects?"
Open-ended questions for company surveys
- "How would you characterize the workplace atmosphere right now?"
- "What changes would enhance our work-life balance?"
- "What would you change about your role?"
Open-ended questions for brainstorming sessions
- "What strategies or tools might we explore to overcome this challenge?"
- "How do our current processes support our creative thinking?"
- "How do we overcome x challenge in wth world right now?"
Open-ended questions for classes or lectures
- "What topic sparks your curiosity the most, and what about it intrigues you?"
- "How might we adapt our learning environment to better suit diverse learning styles?"
- "What's your favourite thing about college life?"
How to implement open-ended questions with ease
Timing and setting play a crucial role in the effectiveness of open-ended questions. They excel in environments where in-depth discussion is the goal, such as debates, brainstorming sessions, icebreaker discussions or when seeking detailed feedback.
When conducting surveys or live interactions, ensure that the setting is conducive to open expression and you can avoid any fears of retribution or judgement. Encourage participation by creating a comfortable atmosphere and affirming that all perspectives are valued. Anonymous live polls or Q&A is a perfect way to start the flow of conversations and to avoid any inhibtions on getting stuck into a conversation. To draw out responses from quieter participants or those hesitant to share, create a supportive environment and use icebreakers to warm up the group. Encourage sharing by directly asking some willing members for their views or relating questions to personal experiences. Remind participants that their is no right or wrong and to be supportive of their peers.
Analyzing the impact of open-ended questions
To evaluate the success of your open-ended questions, observe the level of engagement, the depth of responses, and the variety of ideas presented. A good observation is to note if more silent or passive members of your audience have contributed as well. Using audience response systems you can measure the engagement to polls or Q&A discussion to gauge the level of interaction. Additionally, post-discussion feedback can offer insights into how participants felt about the openness and depth of the questions.
Start using open-ended questions to get increased engagement in your classes or meetings
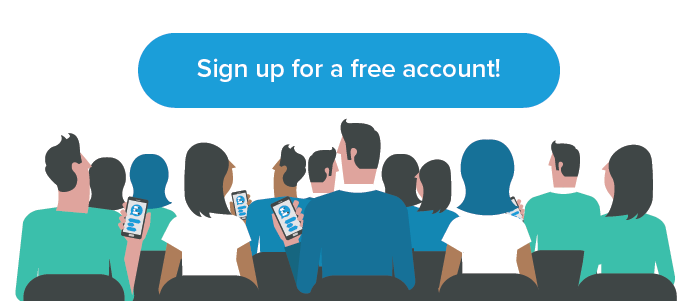
Related articles
From the blog.
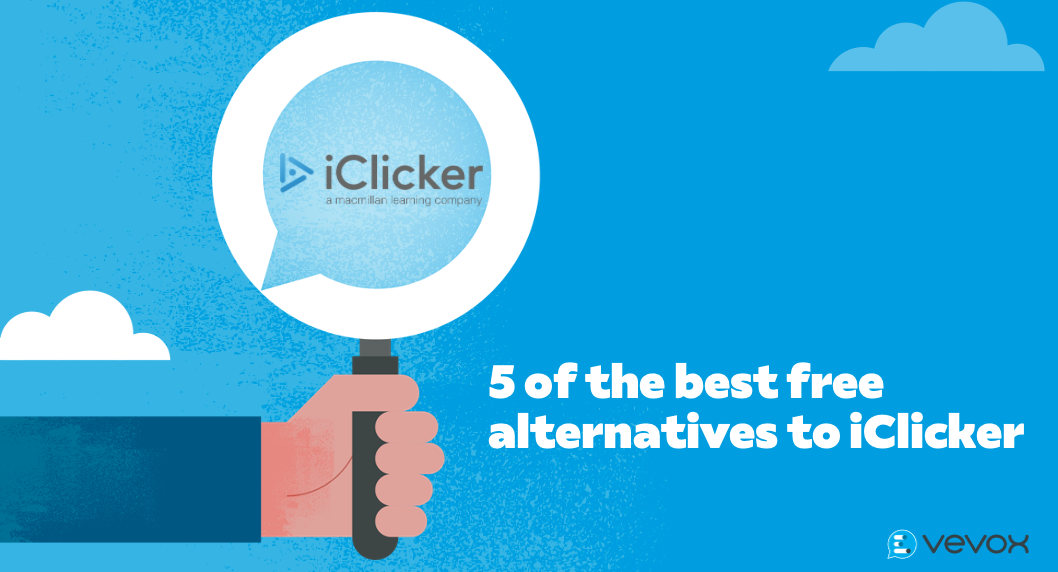
5 of the best free alternatives to iClicker
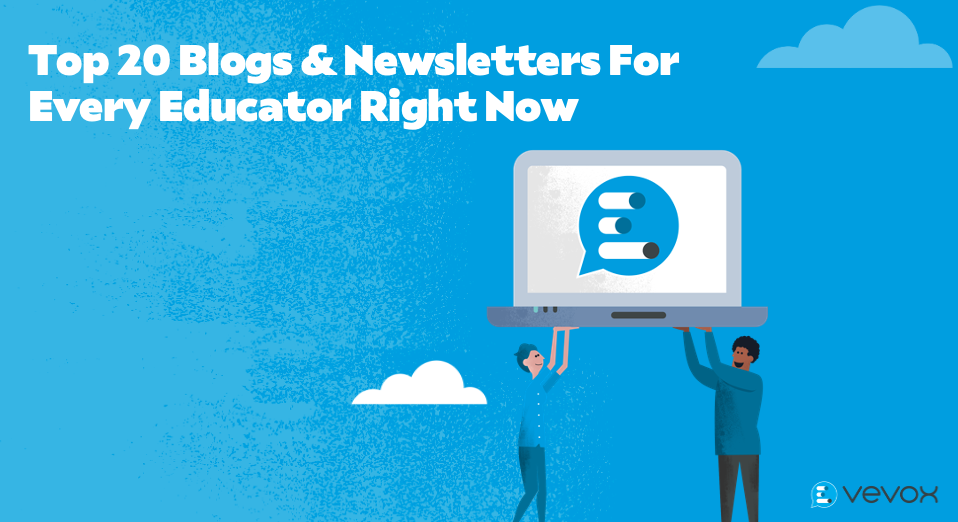
Top 20 Blogs & Newsletters Every Educator Should Follow
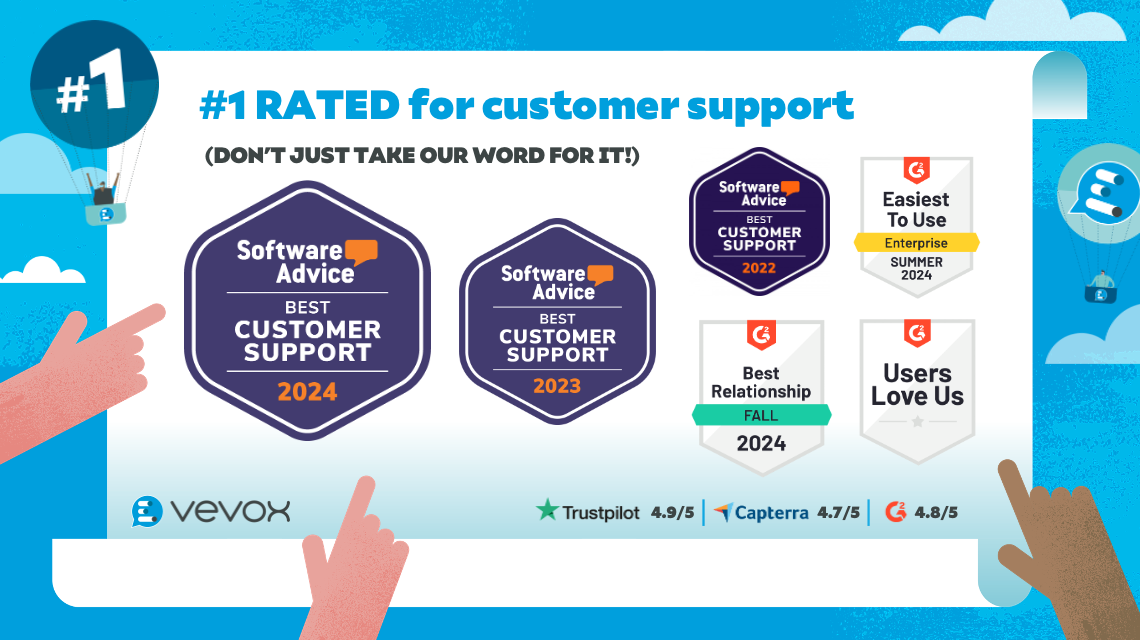
Award-winning service: Vevox again named top for customer support
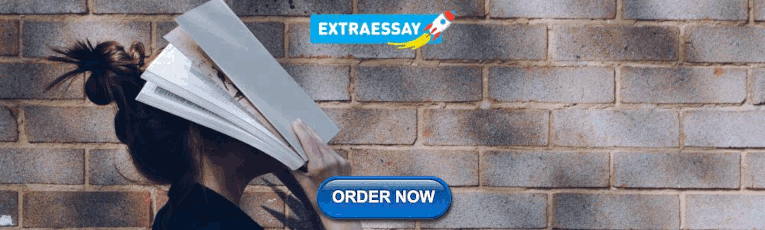
COMMENTS
Learn how to design, conduct, and analyze open-ended questions in qualitative research to gather rich and detailed data. Find out the benefits, types, and challenges of using open-ended questions and see examples of effective questions.
There are two types of questions we can use in research studies: open-ended and closed. Open-ended questions allow participants to give a free-form text answer. Closed questions (or closed-ended questions) restrict participants to one of a limited set of possible answers. Open-ended questions encourage exploration of a topic; a participant can ...
Overall, open-ended questions are powerful to gather information, foster communication, and gain deeper insights. Whether used in research, professional settings, or meaningful conversations, they enable individuals to explore ideas, share perspectives, critical thinking of a person, and engage in meaningful discussions.
Open-ended questions provide more qualitative research data; contextual insights that accentuate quantitative information. With open-ended questions, you get more meaningful user research data. Closed-ended questions, on the other hand, provide quantitative data; limited insight but easy to analyze and compile into reports. Market researchers ...
1. Encourages Thoughtful Responses. Open-ended questions require the respondent to think and provide a more detailed answer rather than simply selecting from a list of predetermined options. This allows for more thoughtful and insightful responses, providing a deeper understanding of the subject matter. 2.
Open-ended questions are crucial in research and communication as they promote richer, qualitative insights. By allowing participants to share their perspectives freely, researchers can uncover nuanced details, emotions, and unexpected insights. Open-ended questions are particularly useful when exploring complex topics or seeking in-depth ...
Learn the definitions, purposes, and examples of open-ended and closed-ended questions in qualitative and quantitative research methods. Find out how to use them in interviews, focus groups, and questionnaires for market research.
Learn what open-ended questions are, why they are effective for research, how to phrase them, when to use them, and tips for success. Open-ended questions allow respondents to provide detailed, expansive, and insightful answers in their own words.
Abstract. Sample size determination for open-ended questions or qualitative interviews relies primarily on custom and finding the point where little new information is obtained (thematic saturation). Here, we propose and test a refined definition of saturation as obtaining the most salient items in a set of qualitative interviews (where items ...
Reasons to Use Open-Ended Questions All open-ended questions are alike in that the respondent is not given answer choices. However, the reasons for using this structure and the level of cognitive effort needed to respond can vary. The following are seven examples that illustrate different reasons for open-ended questions. • 1.
Qualitative studies that utilize open-ended questions allow researchers to take a holistic and comprehensive look at the issues being studied because open-ended responses permit respondents to provide more options and opinions, giving the data more diversity than would be possible with a closed-question or forced-choice survey measure.
It allows researchers to explore the richness and depth of individuals' thoughts, feelings, decision making process and motivations. One of the critical tools in qualitative research is the use of open-ended questions. Open-ended questions invite respondents to provide detailed and personalised responses—allowing for a more nuanced ...
4. Open-ended questions can also provide a greater depth of insight that a closed-ended question. may not have. As Farber (2006) e xplains: agrees with this notion and adds that qualitative ...
One common way to structure a topic guide is to start with relatively easy, open-ended questions (Table 1). Opening questions should be related to the research topic but broad and easy to answer ...
This is possible by asking open-ended questions, which is characteristic of qualitative research (Forman et al., 2008). Responses to open-ended questions are then analyzed inductively within a bottom-up approach, that is, the researchers start with what is in the data to get to more abstract findings (Forman et al., 2008). The main benefit of ...
Open-ended questions for surveys facilitate as a valuable tool in numerous fields. 35+ Open-ended question examples for your next form or survey. As the forms.app team, we are aware of the importance of gathering valuable data. In this section, we compiled 35+ open-ended questions in research examples to help you achieve this.
Open-ended questions are questions that are designed to encourage people to share more than a one-word response and typically start with words like "what," "how," or "why.". Open-ended questions help people expound on an idea or issue and carry the conversation forward without getting stunted in potentially awkward silence or little ...
Revised on June 22, 2023. A questionnaire is a list of questions or items used to gather data from respondents about their attitudes, experiences, or opinions. Questionnaires can be used to collect quantitative and/or qualitative information. Questionnaires are commonly used in market research as well as in the social and health sciences.
Open Ended Questions: 25 Examples for Customer Research. Unlock deeper insights with 30 open-ended questions for customer research. Learn how to use them effectively for rich feedback. Imagine you've just completed the design of your customer survey. You've spent weeks crafting the perfect survey questions, and you're ready to launch.
Qualitative Research with Open-Ended Questions. By incorporating open-ended questions into our surveys, we can gather data that provides valuable insights into how people experience our cities. Qualitative research can help us understand the nuances of public opinion, behavior, and priorities, which can all inform our urban planning decisions.
Tailor your questions to accommodate cultural sensitivities and communication styles, ensuring your inquiries are respectful and effective. Leverage Open-Ended Questions for Enhanced Engagement. The art of asking open-ended questions is a valuable skill that can significantly improve the quality of feedback and discussion in any setting.