
- Ask a Librarian
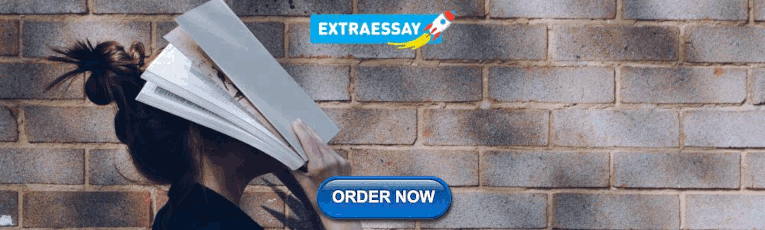
Research: Overview & Approaches
- Getting Started with Undergraduate Research
- Planning & Getting Started
- Building Your Knowledge Base
- Locating Sources
- Reading Scholarly Articles
- Creating a Literature Review
- Productivity & Organizing Research
- Scholarly and Professional Relationships
Introduction to Empirical Research
Databases for finding empirical research, guided search, google scholar, examples of empirical research, sources and further reading.
- Interpretive Research
- Action-Based Research
- Creative & Experimental Approaches
Your Librarian

- Introductory Video This video covers what empirical research is, what kinds of questions and methods empirical researchers use, and some tips for finding empirical research articles in your discipline.

- Guided Search: Finding Empirical Research Articles This is a hands-on tutorial that will allow you to use your own search terms to find resources.

- Study on radiation transfer in human skin for cosmetics
- Long-Term Mobile Phone Use and the Risk of Vestibular Schwannoma: A Danish Nationwide Cohort Study
- Emissions Impacts and Benefits of Plug-In Hybrid Electric Vehicles and Vehicle-to-Grid Services
- Review of design considerations and technological challenges for successful development and deployment of plug-in hybrid electric vehicles
- Endocrine disrupters and human health: could oestrogenic chemicals in body care cosmetics adversely affect breast cancer incidence in women?
- << Previous: Scholarly and Professional Relationships
- Next: Interpretive Research >>
- Last Updated: Jun 20, 2024 1:41 PM
- URL: https://guides.lib.purdue.edu/research_approaches
- USC Libraries
- Research Guides
- Identify Empirical Articles
*Education: Identify Empirical Articles
- Google Scholar tips and instructions
- Newspaper Articles
- Video Tutorials
- EDUC 508 Library Session
- Statistics and Data
- Tests & Measurements
- Citation Managers
- APA Style This link opens in a new window
- Scan & Deliver and Interlibrary Loan
- Educational Leadership
- Global Executive Ed.D.
- Marriage & Family Therapy
- Organizational Change & Leadership
- Literacy Education
- Accreditation
- Journal Ranking Metrics
- Publishing Your Research
- Education and STEM Databases
How to Recognize Empirical Journal Articles
Definition of an empirical study: An empirical research article reports the results of a study that uses data derived from actual observation or experimentation. Empirical research articles are examples of primary research.
Parts of a standard empirical research article: (articles will not necessary use the exact terms listed below.)
- Abstract ... A paragraph length description of what the study includes.
- Introduction ...Includes a statement of the hypotheses for the research and a review of other research on the topic.
- Who are participants
- Design of the study
- What the participants did
- What measures were used
- Results ...Describes the outcomes of the measures of the study.
- Discussion ...Contains the interpretations and implications of the study.
- References ...Contains citation information on the material cited in the report. (also called bibliography or works cited)
Characteristics of an Empirical Article:
- Empirical articles will include charts, graphs, or statistical analysis.
- Empirical research articles are usually substantial, maybe from 8-30 pages long.
- There is always a bibliography found at the end of the article.
Type of publications that publish empirical studies:
- Empirical research articles are published in scholarly or academic journals
- These journals are also called “peer-reviewed,” or “refereed” publications.
Examples of such publications include:
- American Educational Research Journal
- Computers & Education
- Journal of Educational Psychology
Databases that contain empirical research: (selected list only)
- List of other useful databases by subject area
This page is adapted from Eric Karkhoff's Sociology Research Guide: Identify Empirical Articles page (Cal State Fullerton Pollak Library).
Sample Empirical Articles
Roschelle, J., Feng, M., Murphy, R. F., & Mason, C. A. (2016). Online Mathematics Homework Increases Student Achievement. AERA Open . ( L INK TO ARTICLE )
Lester, J., Yamanaka, A., & Struthers, B. (2016). Gender microaggressions and learning environments: The role of physical space in teaching pedagogy and communication. Community College Journal of Research and Practice , 40(11), 909-926. ( LINK TO ARTICLE )
- << Previous: Newspaper Articles
- Next: Workshops and Webinars >>
- Last Updated: Jul 19, 2024 11:15 AM
- URL: https://libguides.usc.edu/education
- Privacy Policy

Home » Research Paper – Structure, Examples and Writing Guide
Research Paper – Structure, Examples and Writing Guide
Table of Contents

Research Paper
Definition:
Research Paper is a written document that presents the author’s original research, analysis, and interpretation of a specific topic or issue.
It is typically based on Empirical Evidence, and may involve qualitative or quantitative research methods, or a combination of both. The purpose of a research paper is to contribute new knowledge or insights to a particular field of study, and to demonstrate the author’s understanding of the existing literature and theories related to the topic.
Structure of Research Paper
The structure of a research paper typically follows a standard format, consisting of several sections that convey specific information about the research study. The following is a detailed explanation of the structure of a research paper:
The title page contains the title of the paper, the name(s) of the author(s), and the affiliation(s) of the author(s). It also includes the date of submission and possibly, the name of the journal or conference where the paper is to be published.
The abstract is a brief summary of the research paper, typically ranging from 100 to 250 words. It should include the research question, the methods used, the key findings, and the implications of the results. The abstract should be written in a concise and clear manner to allow readers to quickly grasp the essence of the research.
Introduction
The introduction section of a research paper provides background information about the research problem, the research question, and the research objectives. It also outlines the significance of the research, the research gap that it aims to fill, and the approach taken to address the research question. Finally, the introduction section ends with a clear statement of the research hypothesis or research question.
Literature Review
The literature review section of a research paper provides an overview of the existing literature on the topic of study. It includes a critical analysis and synthesis of the literature, highlighting the key concepts, themes, and debates. The literature review should also demonstrate the research gap and how the current study seeks to address it.
The methods section of a research paper describes the research design, the sample selection, the data collection and analysis procedures, and the statistical methods used to analyze the data. This section should provide sufficient detail for other researchers to replicate the study.
The results section presents the findings of the research, using tables, graphs, and figures to illustrate the data. The findings should be presented in a clear and concise manner, with reference to the research question and hypothesis.
The discussion section of a research paper interprets the findings and discusses their implications for the research question, the literature review, and the field of study. It should also address the limitations of the study and suggest future research directions.
The conclusion section summarizes the main findings of the study, restates the research question and hypothesis, and provides a final reflection on the significance of the research.
The references section provides a list of all the sources cited in the paper, following a specific citation style such as APA, MLA or Chicago.
How to Write Research Paper
You can write Research Paper by the following guide:
- Choose a Topic: The first step is to select a topic that interests you and is relevant to your field of study. Brainstorm ideas and narrow down to a research question that is specific and researchable.
- Conduct a Literature Review: The literature review helps you identify the gap in the existing research and provides a basis for your research question. It also helps you to develop a theoretical framework and research hypothesis.
- Develop a Thesis Statement : The thesis statement is the main argument of your research paper. It should be clear, concise and specific to your research question.
- Plan your Research: Develop a research plan that outlines the methods, data sources, and data analysis procedures. This will help you to collect and analyze data effectively.
- Collect and Analyze Data: Collect data using various methods such as surveys, interviews, observations, or experiments. Analyze data using statistical tools or other qualitative methods.
- Organize your Paper : Organize your paper into sections such as Introduction, Literature Review, Methods, Results, Discussion, and Conclusion. Ensure that each section is coherent and follows a logical flow.
- Write your Paper : Start by writing the introduction, followed by the literature review, methods, results, discussion, and conclusion. Ensure that your writing is clear, concise, and follows the required formatting and citation styles.
- Edit and Proofread your Paper: Review your paper for grammar and spelling errors, and ensure that it is well-structured and easy to read. Ask someone else to review your paper to get feedback and suggestions for improvement.
- Cite your Sources: Ensure that you properly cite all sources used in your research paper. This is essential for giving credit to the original authors and avoiding plagiarism.
Research Paper Example
Note : The below example research paper is for illustrative purposes only and is not an actual research paper. Actual research papers may have different structures, contents, and formats depending on the field of study, research question, data collection and analysis methods, and other factors. Students should always consult with their professors or supervisors for specific guidelines and expectations for their research papers.
Research Paper Example sample for Students:
Title: The Impact of Social Media on Mental Health among Young Adults
Abstract: This study aims to investigate the impact of social media use on the mental health of young adults. A literature review was conducted to examine the existing research on the topic. A survey was then administered to 200 university students to collect data on their social media use, mental health status, and perceived impact of social media on their mental health. The results showed that social media use is positively associated with depression, anxiety, and stress. The study also found that social comparison, cyberbullying, and FOMO (Fear of Missing Out) are significant predictors of mental health problems among young adults.
Introduction: Social media has become an integral part of modern life, particularly among young adults. While social media has many benefits, including increased communication and social connectivity, it has also been associated with negative outcomes, such as addiction, cyberbullying, and mental health problems. This study aims to investigate the impact of social media use on the mental health of young adults.
Literature Review: The literature review highlights the existing research on the impact of social media use on mental health. The review shows that social media use is associated with depression, anxiety, stress, and other mental health problems. The review also identifies the factors that contribute to the negative impact of social media, including social comparison, cyberbullying, and FOMO.
Methods : A survey was administered to 200 university students to collect data on their social media use, mental health status, and perceived impact of social media on their mental health. The survey included questions on social media use, mental health status (measured using the DASS-21), and perceived impact of social media on their mental health. Data were analyzed using descriptive statistics and regression analysis.
Results : The results showed that social media use is positively associated with depression, anxiety, and stress. The study also found that social comparison, cyberbullying, and FOMO are significant predictors of mental health problems among young adults.
Discussion : The study’s findings suggest that social media use has a negative impact on the mental health of young adults. The study highlights the need for interventions that address the factors contributing to the negative impact of social media, such as social comparison, cyberbullying, and FOMO.
Conclusion : In conclusion, social media use has a significant impact on the mental health of young adults. The study’s findings underscore the need for interventions that promote healthy social media use and address the negative outcomes associated with social media use. Future research can explore the effectiveness of interventions aimed at reducing the negative impact of social media on mental health. Additionally, longitudinal studies can investigate the long-term effects of social media use on mental health.
Limitations : The study has some limitations, including the use of self-report measures and a cross-sectional design. The use of self-report measures may result in biased responses, and a cross-sectional design limits the ability to establish causality.
Implications: The study’s findings have implications for mental health professionals, educators, and policymakers. Mental health professionals can use the findings to develop interventions that address the negative impact of social media use on mental health. Educators can incorporate social media literacy into their curriculum to promote healthy social media use among young adults. Policymakers can use the findings to develop policies that protect young adults from the negative outcomes associated with social media use.
References :
- Twenge, J. M., & Campbell, W. K. (2019). Associations between screen time and lower psychological well-being among children and adolescents: Evidence from a population-based study. Preventive medicine reports, 15, 100918.
- Primack, B. A., Shensa, A., Escobar-Viera, C. G., Barrett, E. L., Sidani, J. E., Colditz, J. B., … & James, A. E. (2017). Use of multiple social media platforms and symptoms of depression and anxiety: A nationally-representative study among US young adults. Computers in Human Behavior, 69, 1-9.
- Van der Meer, T. G., & Verhoeven, J. W. (2017). Social media and its impact on academic performance of students. Journal of Information Technology Education: Research, 16, 383-398.
Appendix : The survey used in this study is provided below.
Social Media and Mental Health Survey
- How often do you use social media per day?
- Less than 30 minutes
- 30 minutes to 1 hour
- 1 to 2 hours
- 2 to 4 hours
- More than 4 hours
- Which social media platforms do you use?
- Others (Please specify)
- How often do you experience the following on social media?
- Social comparison (comparing yourself to others)
- Cyberbullying
- Fear of Missing Out (FOMO)
- Have you ever experienced any of the following mental health problems in the past month?
- Do you think social media use has a positive or negative impact on your mental health?
- Very positive
- Somewhat positive
- Somewhat negative
- Very negative
- In your opinion, which factors contribute to the negative impact of social media on mental health?
- Social comparison
- In your opinion, what interventions could be effective in reducing the negative impact of social media on mental health?
- Education on healthy social media use
- Counseling for mental health problems caused by social media
- Social media detox programs
- Regulation of social media use
Thank you for your participation!
Applications of Research Paper
Research papers have several applications in various fields, including:
- Advancing knowledge: Research papers contribute to the advancement of knowledge by generating new insights, theories, and findings that can inform future research and practice. They help to answer important questions, clarify existing knowledge, and identify areas that require further investigation.
- Informing policy: Research papers can inform policy decisions by providing evidence-based recommendations for policymakers. They can help to identify gaps in current policies, evaluate the effectiveness of interventions, and inform the development of new policies and regulations.
- Improving practice: Research papers can improve practice by providing evidence-based guidance for professionals in various fields, including medicine, education, business, and psychology. They can inform the development of best practices, guidelines, and standards of care that can improve outcomes for individuals and organizations.
- Educating students : Research papers are often used as teaching tools in universities and colleges to educate students about research methods, data analysis, and academic writing. They help students to develop critical thinking skills, research skills, and communication skills that are essential for success in many careers.
- Fostering collaboration: Research papers can foster collaboration among researchers, practitioners, and policymakers by providing a platform for sharing knowledge and ideas. They can facilitate interdisciplinary collaborations and partnerships that can lead to innovative solutions to complex problems.
When to Write Research Paper
Research papers are typically written when a person has completed a research project or when they have conducted a study and have obtained data or findings that they want to share with the academic or professional community. Research papers are usually written in academic settings, such as universities, but they can also be written in professional settings, such as research organizations, government agencies, or private companies.
Here are some common situations where a person might need to write a research paper:
- For academic purposes: Students in universities and colleges are often required to write research papers as part of their coursework, particularly in the social sciences, natural sciences, and humanities. Writing research papers helps students to develop research skills, critical thinking skills, and academic writing skills.
- For publication: Researchers often write research papers to publish their findings in academic journals or to present their work at academic conferences. Publishing research papers is an important way to disseminate research findings to the academic community and to establish oneself as an expert in a particular field.
- To inform policy or practice : Researchers may write research papers to inform policy decisions or to improve practice in various fields. Research findings can be used to inform the development of policies, guidelines, and best practices that can improve outcomes for individuals and organizations.
- To share new insights or ideas: Researchers may write research papers to share new insights or ideas with the academic or professional community. They may present new theories, propose new research methods, or challenge existing paradigms in their field.
Purpose of Research Paper
The purpose of a research paper is to present the results of a study or investigation in a clear, concise, and structured manner. Research papers are written to communicate new knowledge, ideas, or findings to a specific audience, such as researchers, scholars, practitioners, or policymakers. The primary purposes of a research paper are:
- To contribute to the body of knowledge : Research papers aim to add new knowledge or insights to a particular field or discipline. They do this by reporting the results of empirical studies, reviewing and synthesizing existing literature, proposing new theories, or providing new perspectives on a topic.
- To inform or persuade: Research papers are written to inform or persuade the reader about a particular issue, topic, or phenomenon. They present evidence and arguments to support their claims and seek to persuade the reader of the validity of their findings or recommendations.
- To advance the field: Research papers seek to advance the field or discipline by identifying gaps in knowledge, proposing new research questions or approaches, or challenging existing assumptions or paradigms. They aim to contribute to ongoing debates and discussions within a field and to stimulate further research and inquiry.
- To demonstrate research skills: Research papers demonstrate the author’s research skills, including their ability to design and conduct a study, collect and analyze data, and interpret and communicate findings. They also demonstrate the author’s ability to critically evaluate existing literature, synthesize information from multiple sources, and write in a clear and structured manner.
Characteristics of Research Paper
Research papers have several characteristics that distinguish them from other forms of academic or professional writing. Here are some common characteristics of research papers:
- Evidence-based: Research papers are based on empirical evidence, which is collected through rigorous research methods such as experiments, surveys, observations, or interviews. They rely on objective data and facts to support their claims and conclusions.
- Structured and organized: Research papers have a clear and logical structure, with sections such as introduction, literature review, methods, results, discussion, and conclusion. They are organized in a way that helps the reader to follow the argument and understand the findings.
- Formal and objective: Research papers are written in a formal and objective tone, with an emphasis on clarity, precision, and accuracy. They avoid subjective language or personal opinions and instead rely on objective data and analysis to support their arguments.
- Citations and references: Research papers include citations and references to acknowledge the sources of information and ideas used in the paper. They use a specific citation style, such as APA, MLA, or Chicago, to ensure consistency and accuracy.
- Peer-reviewed: Research papers are often peer-reviewed, which means they are evaluated by other experts in the field before they are published. Peer-review ensures that the research is of high quality, meets ethical standards, and contributes to the advancement of knowledge in the field.
- Objective and unbiased: Research papers strive to be objective and unbiased in their presentation of the findings. They avoid personal biases or preconceptions and instead rely on the data and analysis to draw conclusions.
Advantages of Research Paper
Research papers have many advantages, both for the individual researcher and for the broader academic and professional community. Here are some advantages of research papers:
- Contribution to knowledge: Research papers contribute to the body of knowledge in a particular field or discipline. They add new information, insights, and perspectives to existing literature and help advance the understanding of a particular phenomenon or issue.
- Opportunity for intellectual growth: Research papers provide an opportunity for intellectual growth for the researcher. They require critical thinking, problem-solving, and creativity, which can help develop the researcher’s skills and knowledge.
- Career advancement: Research papers can help advance the researcher’s career by demonstrating their expertise and contributions to the field. They can also lead to new research opportunities, collaborations, and funding.
- Academic recognition: Research papers can lead to academic recognition in the form of awards, grants, or invitations to speak at conferences or events. They can also contribute to the researcher’s reputation and standing in the field.
- Impact on policy and practice: Research papers can have a significant impact on policy and practice. They can inform policy decisions, guide practice, and lead to changes in laws, regulations, or procedures.
- Advancement of society: Research papers can contribute to the advancement of society by addressing important issues, identifying solutions to problems, and promoting social justice and equality.
Limitations of Research Paper
Research papers also have some limitations that should be considered when interpreting their findings or implications. Here are some common limitations of research papers:
- Limited generalizability: Research findings may not be generalizable to other populations, settings, or contexts. Studies often use specific samples or conditions that may not reflect the broader population or real-world situations.
- Potential for bias : Research papers may be biased due to factors such as sample selection, measurement errors, or researcher biases. It is important to evaluate the quality of the research design and methods used to ensure that the findings are valid and reliable.
- Ethical concerns: Research papers may raise ethical concerns, such as the use of vulnerable populations or invasive procedures. Researchers must adhere to ethical guidelines and obtain informed consent from participants to ensure that the research is conducted in a responsible and respectful manner.
- Limitations of methodology: Research papers may be limited by the methodology used to collect and analyze data. For example, certain research methods may not capture the complexity or nuance of a particular phenomenon, or may not be appropriate for certain research questions.
- Publication bias: Research papers may be subject to publication bias, where positive or significant findings are more likely to be published than negative or non-significant findings. This can skew the overall findings of a particular area of research.
- Time and resource constraints: Research papers may be limited by time and resource constraints, which can affect the quality and scope of the research. Researchers may not have access to certain data or resources, or may be unable to conduct long-term studies due to practical limitations.
About the author
Muhammad Hassan
Researcher, Academic Writer, Web developer
You may also like

Research Paper Introduction – Writing Guide and...

Survey Instruments – List and Their Uses

Research Process – Steps, Examples and Tips

Informed Consent in Research – Types, Templates...

Theoretical Framework – Types, Examples and...

Data Interpretation – Process, Methods and...
- How It Works
- PhD thesis writing
- Master thesis writing
- Bachelor thesis writing
- Dissertation writing service
- Dissertation abstract writing
- Thesis proposal writing
- Thesis editing service
- Thesis proofreading service
- Thesis formatting service
- Coursework writing service
- Research paper writing service
- Architecture thesis writing
- Computer science thesis writing
- Engineering thesis writing
- History thesis writing
- MBA thesis writing
- Nursing dissertation writing
- Psychology dissertation writing
- Sociology thesis writing
- Statistics dissertation writing
- Buy dissertation online
- Write my dissertation
- Cheap thesis
- Cheap dissertation
- Custom dissertation
- Dissertation help
- Pay for thesis
- Pay for dissertation
- Senior thesis
- Write my thesis
Empirical Paper Writing From A To Z
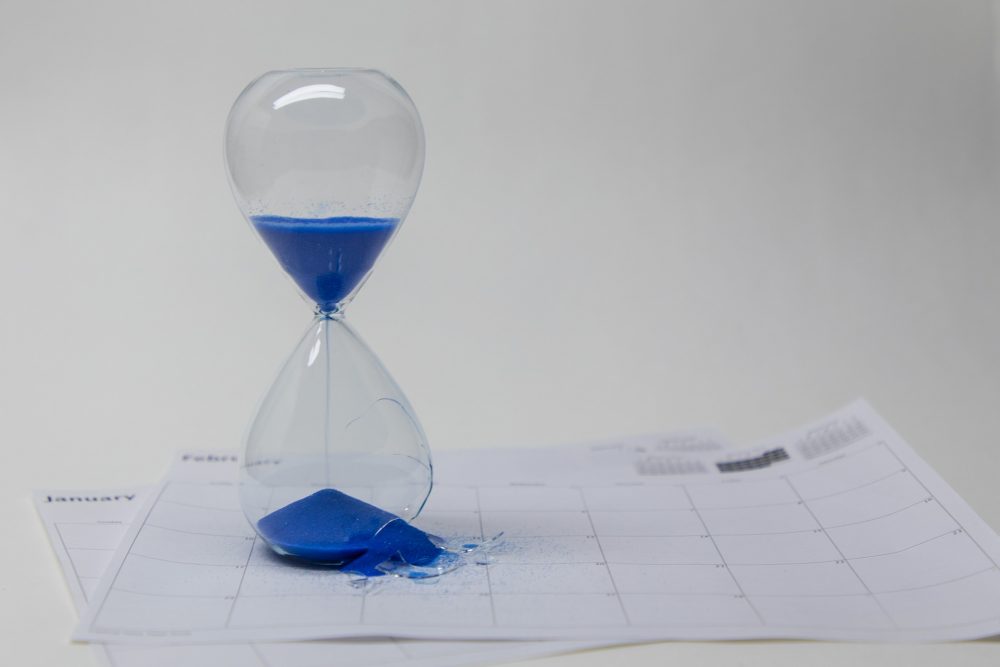
When asked to write an empirical paper, many learners don’t know where to start. That’s because most students don’t know much about this paper. Perhaps, you came across this article after searching for the meaning of this paper online. Well, you’ve come to the right place because this article will explain everything you want to know about this paper.
What is an Empirical Paper?
Importance of an empirical paper, how to write an empirical research paper, interesting empirical research topics to consider, useful tips and insights.
In most cases, humans decide based on evidence. Thus, most people decide based on what they can prove as valid or measure. When presented with plausible alternatives, most individuals tilt towards choices that have been proven effective. Empirical research adopts a similar approach.
In this study, researchers arrive at results by testing their empirical evidence. And they test the available evidence using quantitative and qualitative observation methods, as determined by the research nature. Perhaps, you’re torn between a theoretical paper vs. empirical paper. Just like the name suggests, a theoretical paper is largely based on theories. On the other hand, a paper whose basis is empirical research is largely based on evidence. Ideally, the features and methodology of empirical research set it apart from the other study approaches. Therefore, researchers must understand what constitutes this study.
An empirical research paper is a write-up that is based on verifiable evidence. Ideally, the conclusion of this paper is arrived at after analyzing verifiable evidence. In simple terms, it’s a paper that solely depends on evidence acquired via scientific methods of gathering data or observation.
Researchers can conduct empirical research using quantitative or qualitative observation methods, based on their data sample. That is non-numerical data or quantifiable data. As hinted, this is what differentiates empirical papers from theoretical papers. Ideally, theoretical papers depend on pre-conceived notions regarding research variables. On the other hand, empirical research entails engaging in a scientific investigation and measuring the experimental probability of those variables.
Perhaps, you’re wondering why you should engage in a scientific investigation to write this paper. Well, empirical research is very common because it’s used to perform systematic investigations. Researchers use this method to validate different research hypotheses in fields like law, anthropology, and medicine. Based on the empirical paper definition, this task is important for the following reasons:
- Authenticating traditional research via observations and experiments
- Providing more authentic and competent findings
- Understanding the dynamic changes that happen over time
- Studying rules, institutions, and procedures to understand their operations
- Testing and validating multiple hypotheses to improve human knowledge
- To study human cultures and behavioral patterns
For your paper to serve its purpose effectively, it should be carefully and thoroughly researched. All information included in your paper should be factual and supported with evidence.
As hinted, the basis of your paper should be verifiable research. Therefore, you must conduct extensive research before you write your paper.
Preparation
Start by preparing to write your paper. This step requires you to do several things as follows.
- Select a topic : Start by choosing a topic for your paper. Pick a topic you’re comfortable researching and writing about. Make sure that your topic is not too broad or too narrow. That’s because you won’t cover a too broad topic exhaustively. And if your topic is too narrow, you won’t meet the specified word count for your paper.
- Research the topic : Gather as much information as possible about the topic. Take your time to analyze the information and understand different aspects of your topic. That way, you will know the best angle to take when writing your paper.
Before you proceed to actual writing, you can find and read an APA empirical paper sample to understand what the educator expects from you.
Actual Writing
Now that you have the topic and information that you need to write the paper, you can proceed to the actual writing step. Here’s a guide on how to write an empirical paper.
- Write the title page : This page should summarize your main point, question, or findings that you will present in the paper. It should also include your institutional affiliations and name. Make the title informative, professional, and interesting. That’s because the title is the first thing your readers will see.
- Write the abstract : In empirical research papers, the abstract can be written after completing the paper. That’s because it’s a paragraph that summarizes the paper for the readers. When writing this section, explain your research question briefly. You can also explain research methods, samples, and conclusions briefly.
- Write the introduction : When writing the introduction of empirical paper, include the research problem and then tell your readers why it’s important. Also, provide background information about your topic. Your introduction should explain specific hypotheses while demonstrating how your chosen research design corresponds with them.
- Methodology : In this section, describe the research methods you used to conduct your research. If writing an APA paper, label subsections like participants, sampling procedures, sample size, and study design. Don’t skip this section because you can easily some of the research methods.
- Results : Perhaps, this is the most important part of an empirical research paper structure. Here, you include statistics and data analysis. Report your findings consistently if you mention them in other sections. That way, your readers can easily follow and understand your findings.
- Discussion : Restate your study findings in this section using plain terms. Also, explain how your research findings relate to your hypotheses. Ideally, interpret results in this section while discussing their implications. And this should include practical and theoretical consequences . If you encountered research limitations, acknowledge them in this section.
- Reference : Use the APA guidelines to compose the reference section of your paper. This is an important part of the paper because it provides additional information on your topic.
The order of parts in empirical paper may vary but these are the most important sections of this write-up.
Proofreading
Once you’ve written your paper, take your time to go through it correcting all typo, spelling, grammatical, and factual errors. That’s because you could have brilliant ideas and findings but minor errors can damage the quality of your paper.
One of the most challenging parts of the process of writing this paper is choosing a topic. That’s because learners want to choose topics they will be comfortable researching and writing about. They also want unique topics that will impress educators to award them good grades. If struggling to find a topic for your paper, consider these ideas.
- An empirical analysis of foreign exchange management in developing economies
- An empirical analysis of liquidity problem in commercial banks
- How capital structure affects profitability- Empirical analysis of selected companies
- An empirical analysis of the effect of multi-national oil companies on an economy
- Conflicts management in financial institutions- Empirical analysis of the staff
- An empirical analysis of the impact of monetary policies on a country’s economy
- Impact of currency depreciation on a country’s economy- Empirical analysis
- An empirical analysis of the effect of political institutions on the development and economic growth
- How religion affects social development- Empirical analysis
- Effects of policies, institutions, and events on a society
You can pick any of these empirical paper topics, research extensively, and analyze your findings to compose a winning paper. Nevertheless, make sure only relevant and evidence-backed information goes into your paper.
Follow these tips and insights to write a brilliant paper:
- Check your educator’s guidelines and follow all the instructions, including word count and formatting.
- Create your empirical research paper outline to come up with a properly organized paper with logically flowing ideas.
- Be concise and clear by including important information only.
- Use headings and subheadings to make your paper easy to read.
- Cite multiple credible sources in your paper.
- Include figures and tables to convey information if you find it hard to discuss using paragraphs.
- Get our thesis writing help if you’re got stuck.
Always remember that your paper should be based on research. And the evidence in your paper should be verifiable. You can gather empirical evidence using qualitative and quantitative research methods. The above guidelines and tips should help you come up with a winning paper. However, follow the instructions provided by your educator when writing this paper. If unable to complete this assignment even with these tips and guidelines, get and read a good empirical research paper example first. That way, you will know the kind of information and presentation the educator expects from you.
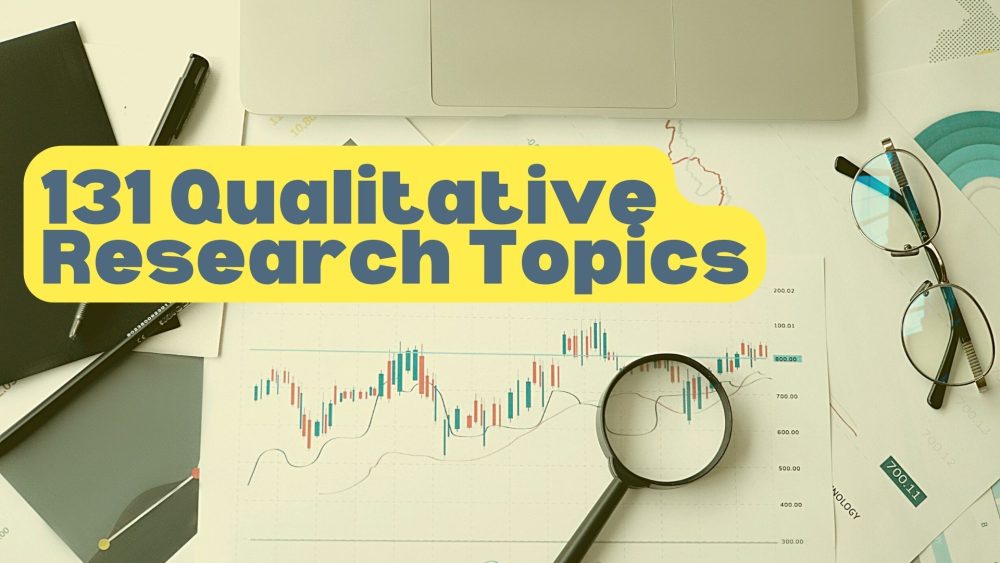
Leave a Reply Cancel reply
Your email address will not be published. Required fields are marked *
Comment * Error message
Name * Error message
Email * Error message
Save my name, email, and website in this browser for the next time I comment.
As Putin continues killing civilians, bombing kindergartens, and threatening WWIII, Ukraine fights for the world's peaceful future.
Ukraine Live Updates
- Skip to main content
- Skip to primary sidebar
- Skip to footer
- QuestionPro

- Solutions Industries Gaming Automotive Sports and events Education Government Travel & Hospitality Financial Services Healthcare Cannabis Technology Use Case NPS+ Communities Audience Contactless surveys Mobile LivePolls Member Experience GDPR Positive People Science 360 Feedback Surveys
- Resources Blog eBooks Survey Templates Case Studies Training Help center

Home Market Research
Empirical Research: Definition, Methods, Types and Examples
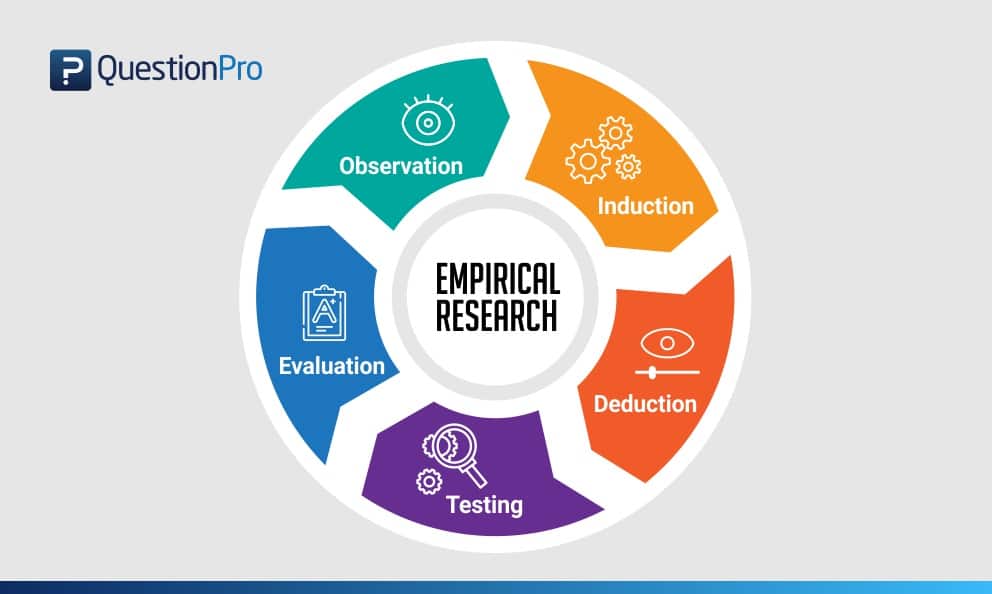
Content Index
Empirical research: Definition
Empirical research: origin, quantitative research methods, qualitative research methods, steps for conducting empirical research, empirical research methodology cycle, advantages of empirical research, disadvantages of empirical research, why is there a need for empirical research.
Empirical research is defined as any research where conclusions of the study is strictly drawn from concretely empirical evidence, and therefore “verifiable” evidence.
This empirical evidence can be gathered using quantitative market research and qualitative market research methods.
For example: A research is being conducted to find out if listening to happy music in the workplace while working may promote creativity? An experiment is conducted by using a music website survey on a set of audience who are exposed to happy music and another set who are not listening to music at all, and the subjects are then observed. The results derived from such a research will give empirical evidence if it does promote creativity or not.
LEARN ABOUT: Behavioral Research
You must have heard the quote” I will not believe it unless I see it”. This came from the ancient empiricists, a fundamental understanding that powered the emergence of medieval science during the renaissance period and laid the foundation of modern science, as we know it today. The word itself has its roots in greek. It is derived from the greek word empeirikos which means “experienced”.
In today’s world, the word empirical refers to collection of data using evidence that is collected through observation or experience or by using calibrated scientific instruments. All of the above origins have one thing in common which is dependence of observation and experiments to collect data and test them to come up with conclusions.
LEARN ABOUT: Causal Research
Types and methodologies of empirical research
Empirical research can be conducted and analysed using qualitative or quantitative methods.
- Quantitative research : Quantitative research methods are used to gather information through numerical data. It is used to quantify opinions, behaviors or other defined variables . These are predetermined and are in a more structured format. Some of the commonly used methods are survey, longitudinal studies, polls, etc
- Qualitative research: Qualitative research methods are used to gather non numerical data. It is used to find meanings, opinions, or the underlying reasons from its subjects. These methods are unstructured or semi structured. The sample size for such a research is usually small and it is a conversational type of method to provide more insight or in-depth information about the problem Some of the most popular forms of methods are focus groups, experiments, interviews, etc.
Data collected from these will need to be analysed. Empirical evidence can also be analysed either quantitatively and qualitatively. Using this, the researcher can answer empirical questions which have to be clearly defined and answerable with the findings he has got. The type of research design used will vary depending on the field in which it is going to be used. Many of them might choose to do a collective research involving quantitative and qualitative method to better answer questions which cannot be studied in a laboratory setting.
LEARN ABOUT: Qualitative Research Questions and Questionnaires
Quantitative research methods aid in analyzing the empirical evidence gathered. By using these a researcher can find out if his hypothesis is supported or not.
- Survey research: Survey research generally involves a large audience to collect a large amount of data. This is a quantitative method having a predetermined set of closed questions which are pretty easy to answer. Because of the simplicity of such a method, high responses are achieved. It is one of the most commonly used methods for all kinds of research in today’s world.
Previously, surveys were taken face to face only with maybe a recorder. However, with advancement in technology and for ease, new mediums such as emails , or social media have emerged.
For example: Depletion of energy resources is a growing concern and hence there is a need for awareness about renewable energy. According to recent studies, fossil fuels still account for around 80% of energy consumption in the United States. Even though there is a rise in the use of green energy every year, there are certain parameters because of which the general population is still not opting for green energy. In order to understand why, a survey can be conducted to gather opinions of the general population about green energy and the factors that influence their choice of switching to renewable energy. Such a survey can help institutions or governing bodies to promote appropriate awareness and incentive schemes to push the use of greener energy.
Learn more: Renewable Energy Survey Template Descriptive Research vs Correlational Research
- Experimental research: In experimental research , an experiment is set up and a hypothesis is tested by creating a situation in which one of the variable is manipulated. This is also used to check cause and effect. It is tested to see what happens to the independent variable if the other one is removed or altered. The process for such a method is usually proposing a hypothesis, experimenting on it, analyzing the findings and reporting the findings to understand if it supports the theory or not.
For example: A particular product company is trying to find what is the reason for them to not be able to capture the market. So the organisation makes changes in each one of the processes like manufacturing, marketing, sales and operations. Through the experiment they understand that sales training directly impacts the market coverage for their product. If the person is trained well, then the product will have better coverage.
- Correlational research: Correlational research is used to find relation between two set of variables . Regression analysis is generally used to predict outcomes of such a method. It can be positive, negative or neutral correlation.
LEARN ABOUT: Level of Analysis
For example: Higher educated individuals will get higher paying jobs. This means higher education enables the individual to high paying job and less education will lead to lower paying jobs.
- Longitudinal study: Longitudinal study is used to understand the traits or behavior of a subject under observation after repeatedly testing the subject over a period of time. Data collected from such a method can be qualitative or quantitative in nature.
For example: A research to find out benefits of exercise. The target is asked to exercise everyday for a particular period of time and the results show higher endurance, stamina, and muscle growth. This supports the fact that exercise benefits an individual body.
- Cross sectional: Cross sectional study is an observational type of method, in which a set of audience is observed at a given point in time. In this type, the set of people are chosen in a fashion which depicts similarity in all the variables except the one which is being researched. This type does not enable the researcher to establish a cause and effect relationship as it is not observed for a continuous time period. It is majorly used by healthcare sector or the retail industry.
For example: A medical study to find the prevalence of under-nutrition disorders in kids of a given population. This will involve looking at a wide range of parameters like age, ethnicity, location, incomes and social backgrounds. If a significant number of kids coming from poor families show under-nutrition disorders, the researcher can further investigate into it. Usually a cross sectional study is followed by a longitudinal study to find out the exact reason.
- Causal-Comparative research : This method is based on comparison. It is mainly used to find out cause-effect relationship between two variables or even multiple variables.
For example: A researcher measured the productivity of employees in a company which gave breaks to the employees during work and compared that to the employees of the company which did not give breaks at all.
LEARN ABOUT: Action Research
Some research questions need to be analysed qualitatively, as quantitative methods are not applicable there. In many cases, in-depth information is needed or a researcher may need to observe a target audience behavior, hence the results needed are in a descriptive analysis form. Qualitative research results will be descriptive rather than predictive. It enables the researcher to build or support theories for future potential quantitative research. In such a situation qualitative research methods are used to derive a conclusion to support the theory or hypothesis being studied.
LEARN ABOUT: Qualitative Interview
- Case study: Case study method is used to find more information through carefully analyzing existing cases. It is very often used for business research or to gather empirical evidence for investigation purpose. It is a method to investigate a problem within its real life context through existing cases. The researcher has to carefully analyse making sure the parameter and variables in the existing case are the same as to the case that is being investigated. Using the findings from the case study, conclusions can be drawn regarding the topic that is being studied.
For example: A report mentioning the solution provided by a company to its client. The challenges they faced during initiation and deployment, the findings of the case and solutions they offered for the problems. Such case studies are used by most companies as it forms an empirical evidence for the company to promote in order to get more business.
- Observational method: Observational method is a process to observe and gather data from its target. Since it is a qualitative method it is time consuming and very personal. It can be said that observational research method is a part of ethnographic research which is also used to gather empirical evidence. This is usually a qualitative form of research, however in some cases it can be quantitative as well depending on what is being studied.
For example: setting up a research to observe a particular animal in the rain-forests of amazon. Such a research usually take a lot of time as observation has to be done for a set amount of time to study patterns or behavior of the subject. Another example used widely nowadays is to observe people shopping in a mall to figure out buying behavior of consumers.
- One-on-one interview: Such a method is purely qualitative and one of the most widely used. The reason being it enables a researcher get precise meaningful data if the right questions are asked. It is a conversational method where in-depth data can be gathered depending on where the conversation leads.
For example: A one-on-one interview with the finance minister to gather data on financial policies of the country and its implications on the public.
- Focus groups: Focus groups are used when a researcher wants to find answers to why, what and how questions. A small group is generally chosen for such a method and it is not necessary to interact with the group in person. A moderator is generally needed in case the group is being addressed in person. This is widely used by product companies to collect data about their brands and the product.
For example: A mobile phone manufacturer wanting to have a feedback on the dimensions of one of their models which is yet to be launched. Such studies help the company meet the demand of the customer and position their model appropriately in the market.
- Text analysis: Text analysis method is a little new compared to the other types. Such a method is used to analyse social life by going through images or words used by the individual. In today’s world, with social media playing a major part of everyone’s life, such a method enables the research to follow the pattern that relates to his study.
For example: A lot of companies ask for feedback from the customer in detail mentioning how satisfied are they with their customer support team. Such data enables the researcher to take appropriate decisions to make their support team better.
Sometimes a combination of the methods is also needed for some questions that cannot be answered using only one type of method especially when a researcher needs to gain a complete understanding of complex subject matter.
We recently published a blog that talks about examples of qualitative data in education ; why don’t you check it out for more ideas?
Learn More: Data Collection Methods: Types & Examples
Since empirical research is based on observation and capturing experiences, it is important to plan the steps to conduct the experiment and how to analyse it. This will enable the researcher to resolve problems or obstacles which can occur during the experiment.
Step #1: Define the purpose of the research
This is the step where the researcher has to answer questions like what exactly do I want to find out? What is the problem statement? Are there any issues in terms of the availability of knowledge, data, time or resources. Will this research be more beneficial than what it will cost.
Before going ahead, a researcher has to clearly define his purpose for the research and set up a plan to carry out further tasks.
Step #2 : Supporting theories and relevant literature
The researcher needs to find out if there are theories which can be linked to his research problem . He has to figure out if any theory can help him support his findings. All kind of relevant literature will help the researcher to find if there are others who have researched this before, or what are the problems faced during this research. The researcher will also have to set up assumptions and also find out if there is any history regarding his research problem
Step #3: Creation of Hypothesis and measurement
Before beginning the actual research he needs to provide himself a working hypothesis or guess what will be the probable result. Researcher has to set up variables, decide the environment for the research and find out how can he relate between the variables.
Researcher will also need to define the units of measurements, tolerable degree for errors, and find out if the measurement chosen will be acceptable by others.
Step #4: Methodology, research design and data collection
In this step, the researcher has to define a strategy for conducting his research. He has to set up experiments to collect data which will enable him to propose the hypothesis. The researcher will decide whether he will need experimental or non experimental method for conducting the research. The type of research design will vary depending on the field in which the research is being conducted. Last but not the least, the researcher will have to find out parameters that will affect the validity of the research design. Data collection will need to be done by choosing appropriate samples depending on the research question. To carry out the research, he can use one of the many sampling techniques. Once data collection is complete, researcher will have empirical data which needs to be analysed.
LEARN ABOUT: Best Data Collection Tools
Step #5: Data Analysis and result
Data analysis can be done in two ways, qualitatively and quantitatively. Researcher will need to find out what qualitative method or quantitative method will be needed or will he need a combination of both. Depending on the unit of analysis of his data, he will know if his hypothesis is supported or rejected. Analyzing this data is the most important part to support his hypothesis.
Step #6: Conclusion
A report will need to be made with the findings of the research. The researcher can give the theories and literature that support his research. He can make suggestions or recommendations for further research on his topic.
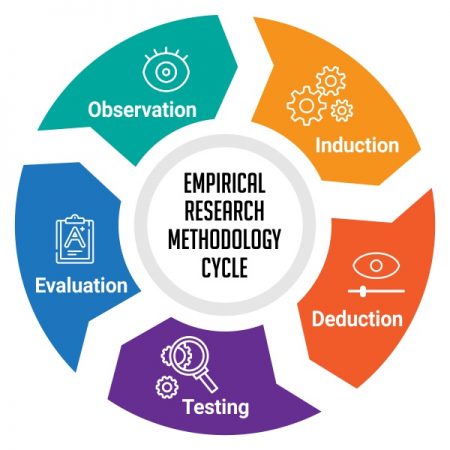
A.D. de Groot, a famous dutch psychologist and a chess expert conducted some of the most notable experiments using chess in the 1940’s. During his study, he came up with a cycle which is consistent and now widely used to conduct empirical research. It consists of 5 phases with each phase being as important as the next one. The empirical cycle captures the process of coming up with hypothesis about how certain subjects work or behave and then testing these hypothesis against empirical data in a systematic and rigorous approach. It can be said that it characterizes the deductive approach to science. Following is the empirical cycle.
- Observation: At this phase an idea is sparked for proposing a hypothesis. During this phase empirical data is gathered using observation. For example: a particular species of flower bloom in a different color only during a specific season.
- Induction: Inductive reasoning is then carried out to form a general conclusion from the data gathered through observation. For example: As stated above it is observed that the species of flower blooms in a different color during a specific season. A researcher may ask a question “does the temperature in the season cause the color change in the flower?” He can assume that is the case, however it is a mere conjecture and hence an experiment needs to be set up to support this hypothesis. So he tags a few set of flowers kept at a different temperature and observes if they still change the color?
- Deduction: This phase helps the researcher to deduce a conclusion out of his experiment. This has to be based on logic and rationality to come up with specific unbiased results.For example: In the experiment, if the tagged flowers in a different temperature environment do not change the color then it can be concluded that temperature plays a role in changing the color of the bloom.
- Testing: This phase involves the researcher to return to empirical methods to put his hypothesis to the test. The researcher now needs to make sense of his data and hence needs to use statistical analysis plans to determine the temperature and bloom color relationship. If the researcher finds out that most flowers bloom a different color when exposed to the certain temperature and the others do not when the temperature is different, he has found support to his hypothesis. Please note this not proof but just a support to his hypothesis.
- Evaluation: This phase is generally forgotten by most but is an important one to keep gaining knowledge. During this phase the researcher puts forth the data he has collected, the support argument and his conclusion. The researcher also states the limitations for the experiment and his hypothesis and suggests tips for others to pick it up and continue a more in-depth research for others in the future. LEARN MORE: Population vs Sample
LEARN MORE: Population vs Sample
There is a reason why empirical research is one of the most widely used method. There are a few advantages associated with it. Following are a few of them.
- It is used to authenticate traditional research through various experiments and observations.
- This research methodology makes the research being conducted more competent and authentic.
- It enables a researcher understand the dynamic changes that can happen and change his strategy accordingly.
- The level of control in such a research is high so the researcher can control multiple variables.
- It plays a vital role in increasing internal validity .
Even though empirical research makes the research more competent and authentic, it does have a few disadvantages. Following are a few of them.
- Such a research needs patience as it can be very time consuming. The researcher has to collect data from multiple sources and the parameters involved are quite a few, which will lead to a time consuming research.
- Most of the time, a researcher will need to conduct research at different locations or in different environments, this can lead to an expensive affair.
- There are a few rules in which experiments can be performed and hence permissions are needed. Many a times, it is very difficult to get certain permissions to carry out different methods of this research.
- Collection of data can be a problem sometimes, as it has to be collected from a variety of sources through different methods.
LEARN ABOUT: Social Communication Questionnaire
Empirical research is important in today’s world because most people believe in something only that they can see, hear or experience. It is used to validate multiple hypothesis and increase human knowledge and continue doing it to keep advancing in various fields.
For example: Pharmaceutical companies use empirical research to try out a specific drug on controlled groups or random groups to study the effect and cause. This way, they prove certain theories they had proposed for the specific drug. Such research is very important as sometimes it can lead to finding a cure for a disease that has existed for many years. It is useful in science and many other fields like history, social sciences, business, etc.
LEARN ABOUT: 12 Best Tools for Researchers
With the advancement in today’s world, empirical research has become critical and a norm in many fields to support their hypothesis and gain more knowledge. The methods mentioned above are very useful for carrying out such research. However, a number of new methods will keep coming up as the nature of new investigative questions keeps getting unique or changing.
Create a single source of real data with a built-for-insights platform. Store past data, add nuggets of insights, and import research data from various sources into a CRM for insights. Build on ever-growing research with a real-time dashboard in a unified research management platform to turn insights into knowledge.
LEARN MORE FREE TRIAL
MORE LIKE THIS
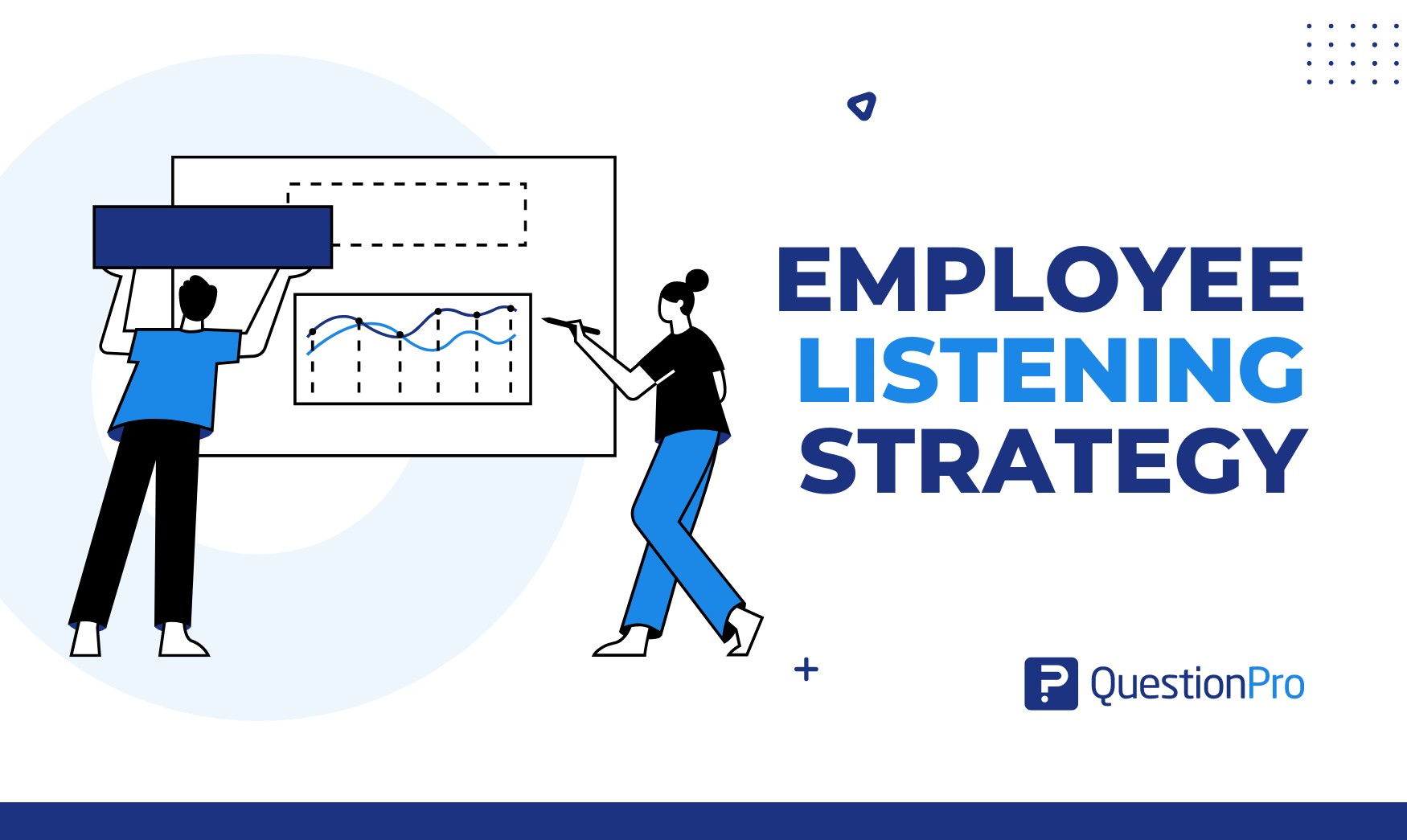
Employee Listening Strategy: What it is & How to Build One
Jul 17, 2024
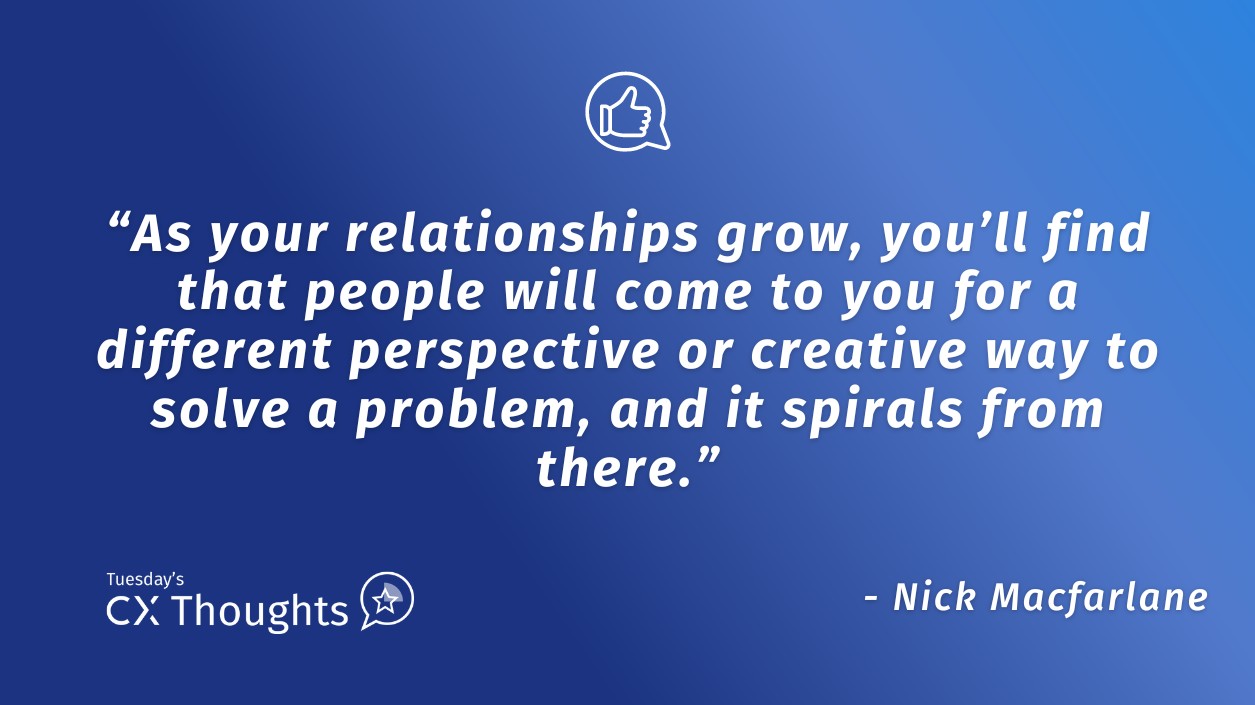
Winning the Internal CX Battles — Tuesday CX Thoughts
Jul 16, 2024
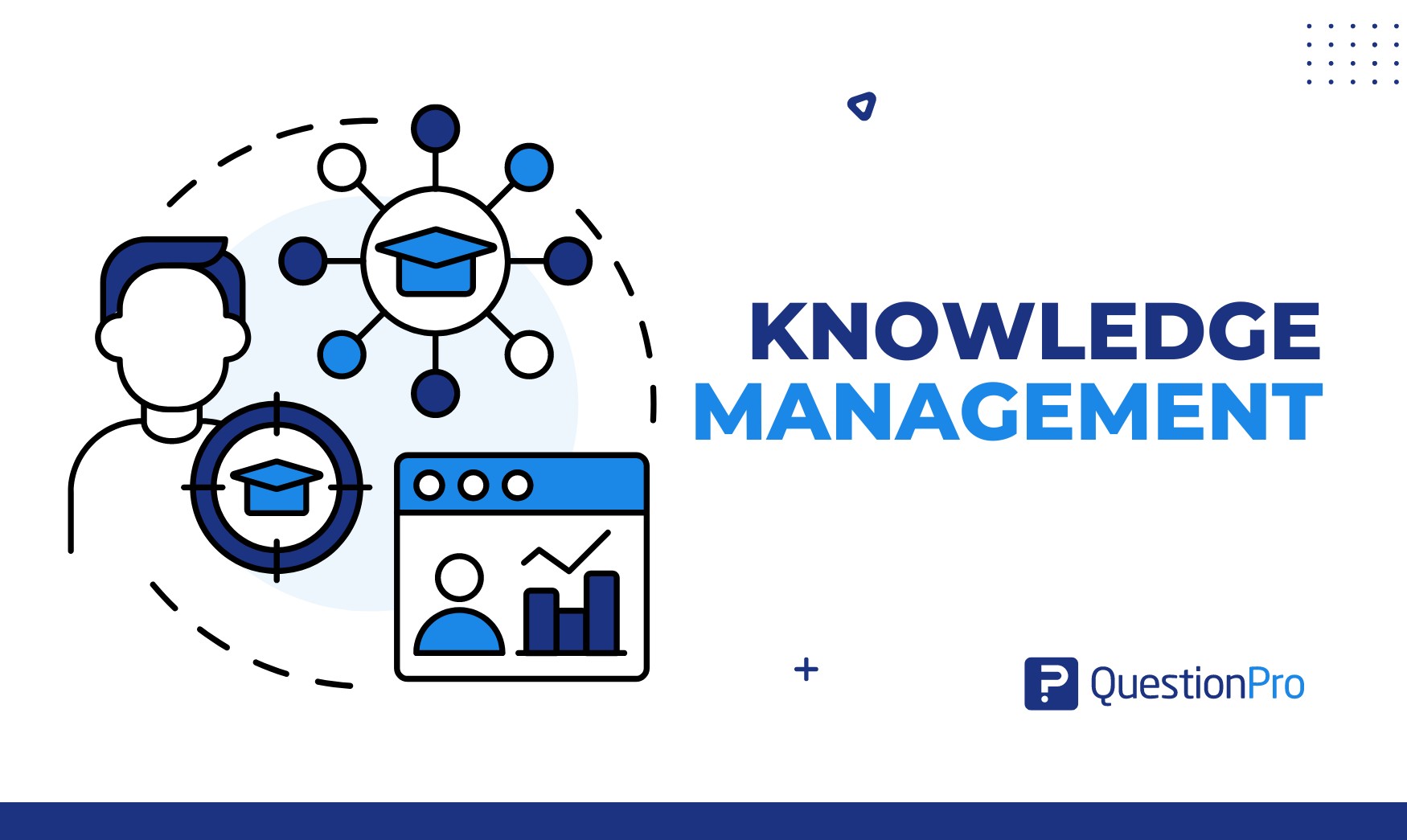
Knowledge Management: What it is, Types, and Use Cases
Jul 12, 2024
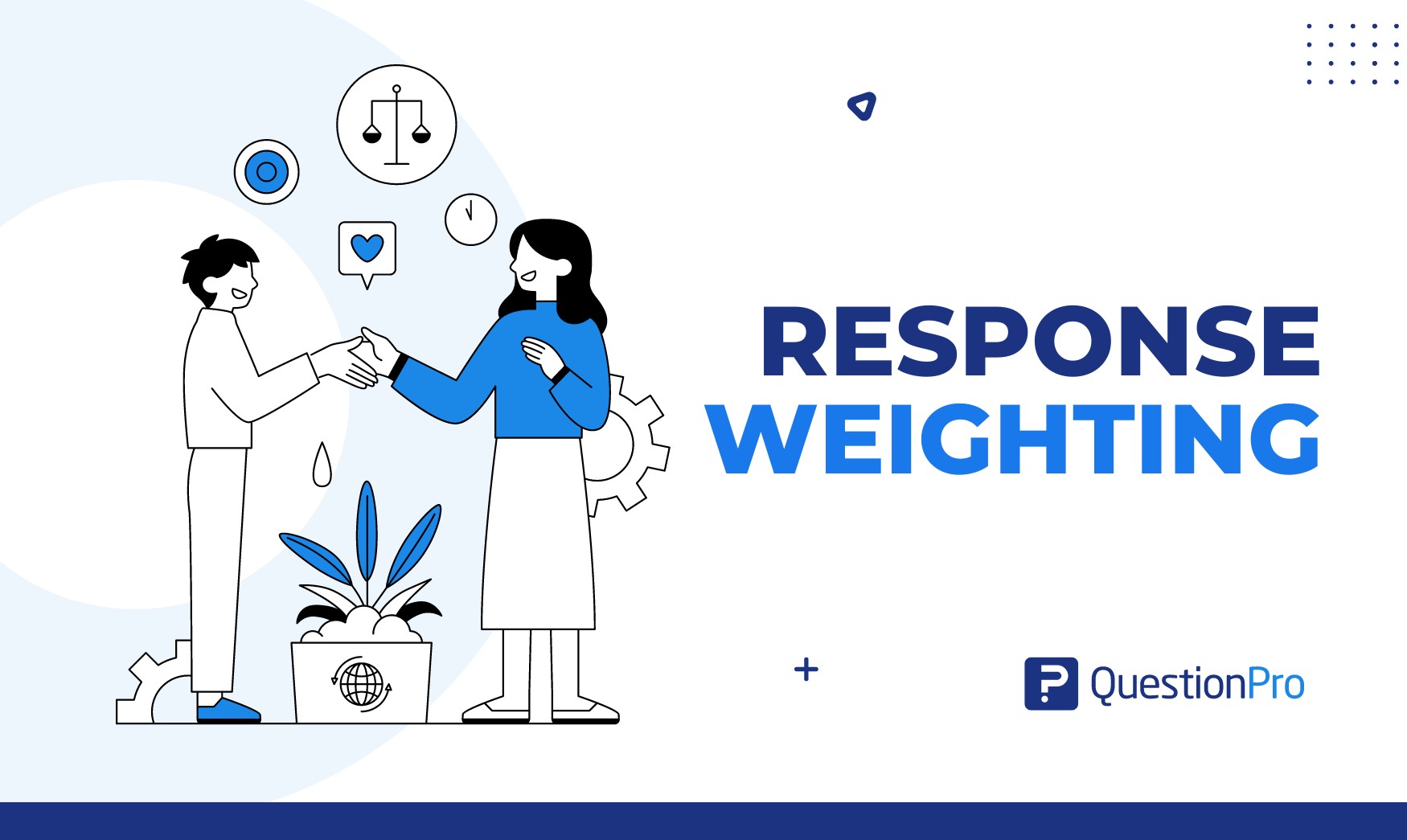
Response Weighting: Enhancing Accuracy in Your Surveys
Jul 11, 2024
Other categories
- Academic Research
- Artificial Intelligence
- Assessments
- Brand Awareness
- Case Studies
- Communities
- Consumer Insights
- Customer effort score
- Customer Engagement
- Customer Experience
- Customer Loyalty
- Customer Research
- Customer Satisfaction
- Employee Benefits
- Employee Engagement
- Employee Retention
- Friday Five
- General Data Protection Regulation
- Insights Hub
- Life@QuestionPro
- Market Research
- Mobile diaries
- Mobile Surveys
- New Features
- Online Communities
- Question Types
- Questionnaire
- QuestionPro Products
- Release Notes
- Research Tools and Apps
- Revenue at Risk
- Survey Templates
- Training Tips
- Tuesday CX Thoughts (TCXT)
- Uncategorized
- What’s Coming Up
- Workforce Intelligence

Identifying Empirical Research Articles
Identifying empirical articles.
- Searching for Empirical Research Articles
What is Empirical Research?
An empirical research article reports the results of a study that uses data derived from actual observation or experimentation. Empirical research articles are examples of primary research. To learn more about the differences between primary and secondary research, see our related guide:
- Primary and Secondary Sources
By the end of this guide, you will be able to:
- Identify common elements of an empirical article
- Use a variety of search strategies to search for empirical articles within the library collection
Look for the IMRaD layout in the article to help identify empirical research. Sometimes the sections will be labeled differently, but the content will be similar.
- I ntroduction: why the article was written, research question or questions, hypothesis, literature review
- M ethods: the overall research design and implementation, description of sample, instruments used, how the authors measured their experiment
- R esults: output of the author's measurements, usually includes statistics of the author's findings
- D iscussion: the author's interpretation and conclusions about the results, limitations of study, suggestions for further research
Parts of an Empirical Research Article
Parts of an empirical article.
The screenshots below identify the basic IMRaD structure of an empirical research article.
Introduction
The introduction contains a literature review and the study's research hypothesis.

The method section outlines the research design, participants, and measures used.

Results
The results section contains statistical data (charts, graphs, tables, etc.) and research participant quotes.

The discussion section includes impacts, limitations, future considerations, and research.

Learn the IMRaD Layout: How to Identify an Empirical Article
This short video overviews the IMRaD method for identifying empirical research.
- Next: Searching for Empirical Research Articles >>
- Last Updated: Nov 16, 2023 8:24 AM
CityU Home - CityU Catalog


- University of La Verne
- Subject Guides
Identify Empirical Research Articles
- Interactive Tutorial
- Literature Matrix
- Guide to the Successful Thesis and Dissertation: A Handbook for Students and Faculty
- Practical Guide to the Qualitative Dissertation
- Guide to Writing Empirical Papers, Theses, and Dissertations
What is a Literature Review--YouTube
Literature Review Guides
- How to write a literature review
- The Literature Review:a few steps on conducting it Permission granted from Writing at the University of Toronto
- Six steps for writing a literature review This blog, written by Tanya Golash-Bozal PhD an Associate Professor of Sociology at the University of California at Merced, offers a very nice and simple advice on how to write a literature review from the point of view of an experience professional.
- The Writing Center, University of North Carolina at Chapel Hill Permission granted to use this guide.
- Writing Center University of North Carolina
- Literature Reviews Otago Polytechnic in New Zealand produced this guide and in my opinion, it is one of the best. NOTE: Except where otherwise noted, content on this site is licensed under a Creative Commons Attribution-NonCommercial-ShareAlike 4.0 International License. However, all images and Otago Polytechnic videos are copyrighted.
What Are Empirical Articles?
As a student at the University of La Verne, faculty may instruct you to read and analyze empirical articles when writing a research paper, a senior or master's project, or a doctoral dissertation. How can you recognize an empirical article in an academic discipline? An empirical research article is an article which reports research based on actual observations or experiments. The research may use quantitative research methods, which generate numerical data and seek to establish causal relationships between two or more variables.(1) Empirical research articles may use qualitative research methods, which objectively and critically analyze behaviors, beliefs, feelings, or values with few or no numerical data available for analysis.(2)
How can I determine if I have found an empirical article?
When looking at an article or the abstract of an article, here are some guidelines to use to decide if an article is an empirical article.
- Is the article published in an academic, scholarly, or professional journal? Popular magazines such as Business Week or Newsweek do not publish empirical research articles; academic journals such as Business Communication Quarterly or Journal of Psychology may publish empirical articles. Some professional journals, such as JAMA: Journal of the American Medical Association publish empirical research. Other professional journals, such as Coach & Athletic Director publish articles of professional interest, but they do not publish research articles.
- Does the abstract of the article mention a study, an observation, an analysis or a number of participants or subjects? Was data collected, a survey or questionnaire administered, an assessment or measurement used, an interview conducted? All of these terms indicate possible methodologies used in empirical research.
- Introduction -The introduction provides a very brief summary of the research.
- Methodology -The method section describes how the research was conducted, including who the participants were, the design of the study, what the participants did, and what measures were used.
- Results -The results section describes the outcomes of the measures of the study.
- Discussion -The discussion section contains the interpretations and implications of the study.
- Conclusion -
- References -A reference section contains information about the articles and books cited in the report and should be substantial.
- How long is the article? An empirical article is usually substantial; it is normally seven or more pages long.
When in doubt if an article is an empirical research article, share the article citation and abstract with your professor or a librarian so that we can help you become better at recognizing the differences between empirical research and other types of scholarly articles.
How can I search for empirical research articles using the electronic databases available through Wilson Library?
- A quick and somewhat superficial way to look for empirical research is to type your search terms into the database's search boxes, then type STUDY OR STUDIES in the final search box to look for studies on your topic area. Be certain to use the ability to limit your search to scholarly/professional journals if that is available on the database. Evaluate the results of your search using the guidelines above to determine if any of the articles are empirical research articles.
- In EbscoHost databases, such as Education Source , on the Advanced Search page you should see a PUBLICATION TYPE field; highlight the appropriate entry. Empirical research may not be the term used; look for a term that may be a synonym for empirical research. ERIC uses REPORTS-RESEARCH. Also find the field for INTENDED AUDIENCE and highlight RESEARCHER. PsycArticles and Psycinfo include a field for METHODOLOGY where you can highlight EMPIRICAL STUDY. National Criminal Justice Reference Service Abstracts has a field for DOCUMENT TYPE; highlight STUDIES/RESEARCH REPORTS. Then evaluate the articles you find using the guidelines above to determine if an article is empirical.
- In ProQuest databases, such as ProQuest Psychology Journals , on the Advanced Search page look under MORE SEARCH OPTIONS and click on the pull down menu for DOCUMENT TYPE and highlight an appropriate type, such as REPORT or EVIDENCE BASED. Also look for the SOURCE TYPE field and highlight SCHOLARLY JOURNALS. Evaluate the search results using the guidelines to determine if an article is empirical.
- Pub Med Central , Sage Premier , Science Direct , Wiley Interscience , and Wiley Interscience Humanities and Social Sciences consist of scholarly and professional journals which publish primarily empirical articles. After conducting a subject search in these databases, evaluate the items you find by using the guidelines above for deciding if an article is empirical.
- "Quantitative research" A Dictionary of Nursing. Oxford University Press, 2008. Oxford Reference Online. Oxford University Press. University of La Verne. 25 August 2009
- "Qualitative analysis" A Dictionary of Public Health. Ed. John M. Last, Oxford University Press, 2007. Oxford Reference Online . Oxford University Press. University of La Verne. 25 August 2009
Empirical Articles:Tips on Database Searching
- Identifying Empirical Articles
- Next: Interactive Tutorial >>
- Last Updated: Mar 5, 2024 4:49 PM
- URL: https://laverne.libguides.com/empirical-articles
Have a language expert improve your writing
Run a free plagiarism check in 10 minutes, generate accurate citations for free.
- Knowledge Base
- Research paper
Writing a Research Paper Conclusion | Step-by-Step Guide
Published on October 30, 2022 by Jack Caulfield . Revised on April 13, 2023.
- Restate the problem statement addressed in the paper
- Summarize your overall arguments or findings
- Suggest the key takeaways from your paper
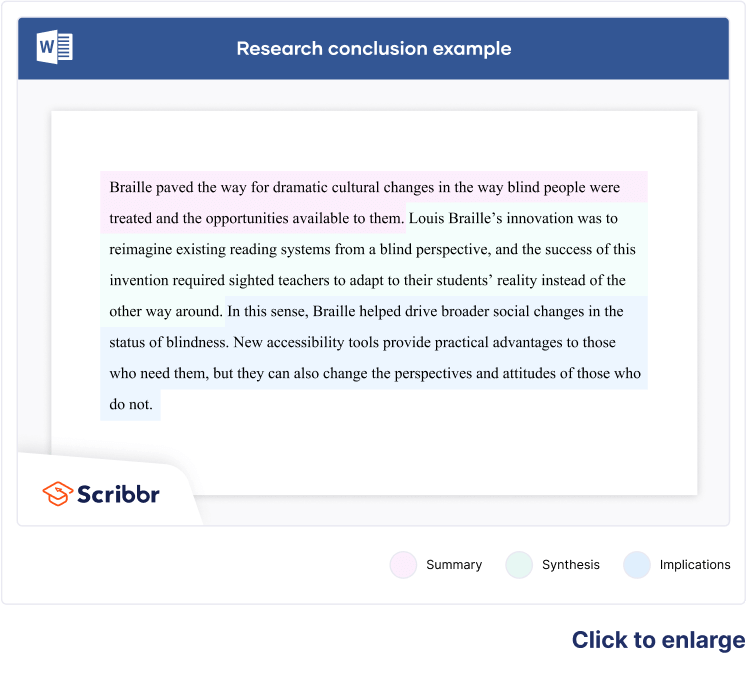
The content of the conclusion varies depending on whether your paper presents the results of original empirical research or constructs an argument through engagement with sources .
Instantly correct all language mistakes in your text
Upload your document to correct all your mistakes in minutes
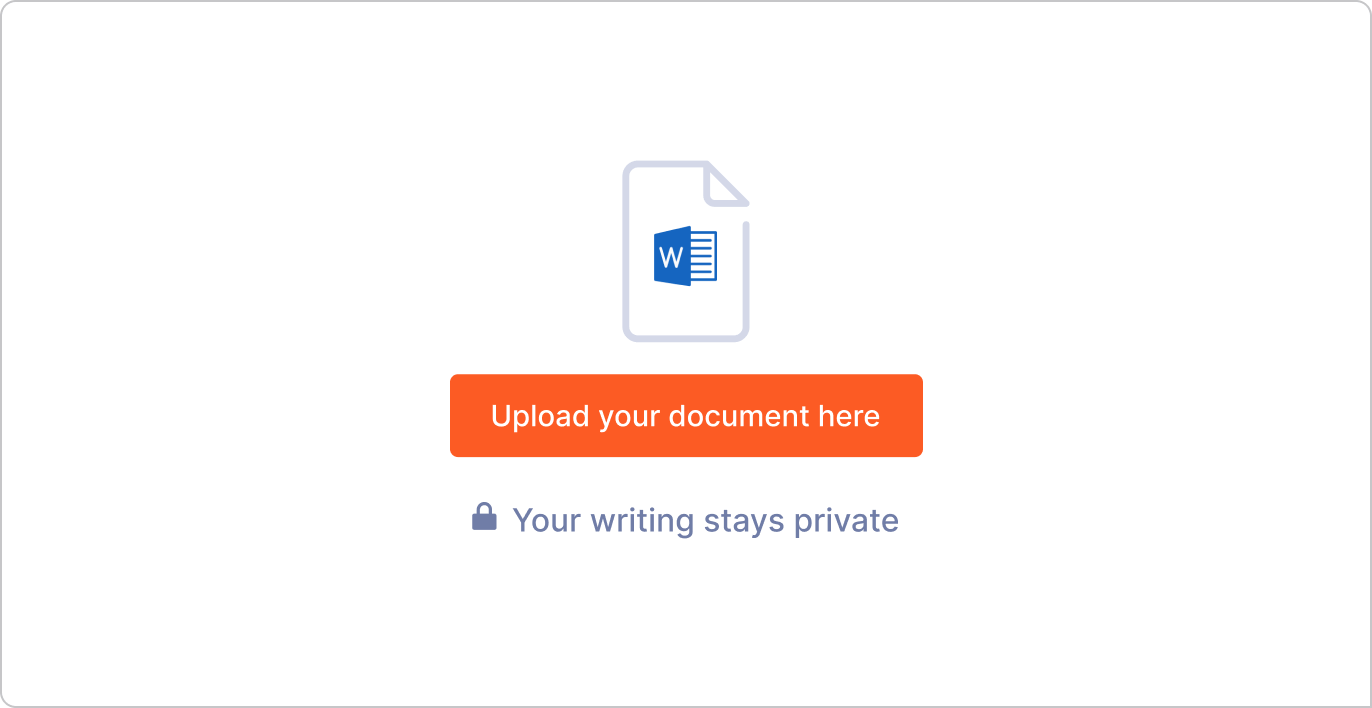
Table of contents
Step 1: restate the problem, step 2: sum up the paper, step 3: discuss the implications, research paper conclusion examples, frequently asked questions about research paper conclusions.
The first task of your conclusion is to remind the reader of your research problem . You will have discussed this problem in depth throughout the body, but now the point is to zoom back out from the details to the bigger picture.
While you are restating a problem you’ve already introduced, you should avoid phrasing it identically to how it appeared in the introduction . Ideally, you’ll find a novel way to circle back to the problem from the more detailed ideas discussed in the body.
For example, an argumentative paper advocating new measures to reduce the environmental impact of agriculture might restate its problem as follows:
Meanwhile, an empirical paper studying the relationship of Instagram use with body image issues might present its problem like this:
“In conclusion …”
Avoid starting your conclusion with phrases like “In conclusion” or “To conclude,” as this can come across as too obvious and make your writing seem unsophisticated. The content and placement of your conclusion should make its function clear without the need for additional signposting.
Receive feedback on language, structure, and formatting
Professional editors proofread and edit your paper by focusing on:
- Academic style
- Vague sentences
- Style consistency
See an example
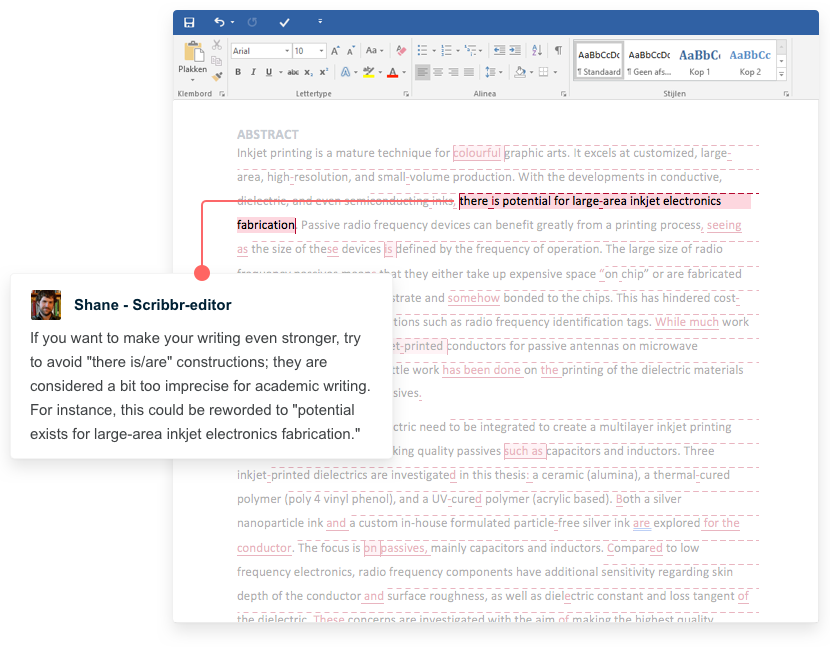
Having zoomed back in on the problem, it’s time to summarize how the body of the paper went about addressing it, and what conclusions this approach led to.
Depending on the nature of your research paper, this might mean restating your thesis and arguments, or summarizing your overall findings.
Argumentative paper: Restate your thesis and arguments
In an argumentative paper, you will have presented a thesis statement in your introduction, expressing the overall claim your paper argues for. In the conclusion, you should restate the thesis and show how it has been developed through the body of the paper.
Briefly summarize the key arguments made in the body, showing how each of them contributes to proving your thesis. You may also mention any counterarguments you addressed, emphasizing why your thesis holds up against them, particularly if your argument is a controversial one.
Don’t go into the details of your evidence or present new ideas; focus on outlining in broad strokes the argument you have made.
Empirical paper: Summarize your findings
In an empirical paper, this is the time to summarize your key findings. Don’t go into great detail here (you will have presented your in-depth results and discussion already), but do clearly express the answers to the research questions you investigated.
Describe your main findings, even if they weren’t necessarily the ones you expected or hoped for, and explain the overall conclusion they led you to.
Having summed up your key arguments or findings, the conclusion ends by considering the broader implications of your research. This means expressing the key takeaways, practical or theoretical, from your paper—often in the form of a call for action or suggestions for future research.
Argumentative paper: Strong closing statement
An argumentative paper generally ends with a strong closing statement. In the case of a practical argument, make a call for action: What actions do you think should be taken by the people or organizations concerned in response to your argument?
If your topic is more theoretical and unsuitable for a call for action, your closing statement should express the significance of your argument—for example, in proposing a new understanding of a topic or laying the groundwork for future research.
Empirical paper: Future research directions
In a more empirical paper, you can close by either making recommendations for practice (for example, in clinical or policy papers), or suggesting directions for future research.
Whatever the scope of your own research, there will always be room for further investigation of related topics, and you’ll often discover new questions and problems during the research process .
Finish your paper on a forward-looking note by suggesting how you or other researchers might build on this topic in the future and address any limitations of the current paper.
Full examples of research paper conclusions are shown in the tabs below: one for an argumentative paper, the other for an empirical paper.
- Argumentative paper
- Empirical paper
While the role of cattle in climate change is by now common knowledge, countries like the Netherlands continually fail to confront this issue with the urgency it deserves. The evidence is clear: To create a truly futureproof agricultural sector, Dutch farmers must be incentivized to transition from livestock farming to sustainable vegetable farming. As well as dramatically lowering emissions, plant-based agriculture, if approached in the right way, can produce more food with less land, providing opportunities for nature regeneration areas that will themselves contribute to climate targets. Although this approach would have economic ramifications, from a long-term perspective, it would represent a significant step towards a more sustainable and resilient national economy. Transitioning to sustainable vegetable farming will make the Netherlands greener and healthier, setting an example for other European governments. Farmers, policymakers, and consumers must focus on the future, not just on their own short-term interests, and work to implement this transition now.
As social media becomes increasingly central to young people’s everyday lives, it is important to understand how different platforms affect their developing self-conception. By testing the effect of daily Instagram use among teenage girls, this study established that highly visual social media does indeed have a significant effect on body image concerns, with a strong correlation between the amount of time spent on the platform and participants’ self-reported dissatisfaction with their appearance. However, the strength of this effect was moderated by pre-test self-esteem ratings: Participants with higher self-esteem were less likely to experience an increase in body image concerns after using Instagram. This suggests that, while Instagram does impact body image, it is also important to consider the wider social and psychological context in which this usage occurs: Teenagers who are already predisposed to self-esteem issues may be at greater risk of experiencing negative effects. Future research into Instagram and other highly visual social media should focus on establishing a clearer picture of how self-esteem and related constructs influence young people’s experiences of these platforms. Furthermore, while this experiment measured Instagram usage in terms of time spent on the platform, observational studies are required to gain more insight into different patterns of usage—to investigate, for instance, whether active posting is associated with different effects than passive consumption of social media content.
If you’re unsure about the conclusion, it can be helpful to ask a friend or fellow student to read your conclusion and summarize the main takeaways.
- Do they understand from your conclusion what your research was about?
- Are they able to summarize the implications of your findings?
- Can they answer your research question based on your conclusion?
You can also get an expert to proofread and feedback your paper with a paper editing service .
The conclusion of a research paper has several key elements you should make sure to include:
- A restatement of the research problem
- A summary of your key arguments and/or findings
- A short discussion of the implications of your research
No, it’s not appropriate to present new arguments or evidence in the conclusion . While you might be tempted to save a striking argument for last, research papers follow a more formal structure than this.
All your findings and arguments should be presented in the body of the text (more specifically in the results and discussion sections if you are following a scientific structure). The conclusion is meant to summarize and reflect on the evidence and arguments you have already presented, not introduce new ones.
Cite this Scribbr article
If you want to cite this source, you can copy and paste the citation or click the “Cite this Scribbr article” button to automatically add the citation to our free Citation Generator.
Caulfield, J. (2023, April 13). Writing a Research Paper Conclusion | Step-by-Step Guide. Scribbr. Retrieved July 21, 2024, from https://www.scribbr.com/research-paper/research-paper-conclusion/
Is this article helpful?
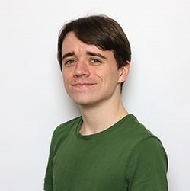
Jack Caulfield
Other students also liked, writing a research paper introduction | step-by-step guide, how to create a structured research paper outline | example, checklist: writing a great research paper, what is your plagiarism score.

The Research Process: Parts of the Research Article
- Search Strategies
- Creating a Research Question
- Search Terms
- Finding e-Books
- Finding Articles and Images
- Journal Articles
- Books & Other Sources
Parts of the Research Article
- Reading and Understanding Research
- Data and Datasets
- Literature Reviews
- Recording Your Research
- Annotated Bibliographies
When you write a research paper, many instructors will ask you to find scholarly research articles as a basis for your research. A scholarly research article presents original research and is written and reviewed by experts in a field. A typical scholarly research article has several sections that can include the:
Introduction
Bibliography or reference list, definitions.
Each part of the research article above has a specific purpose for the reader.
The abstract is a succinct summary of a research article, usually only a paragraph long. The abstract should tell readers whether an article will be useful for their research.
The introduction gives background information on the topic or problems that the research article addresses. The introduction usually includes a problem statement, which describes a specific problem that the researchers are trying to solve. The introduction can also include a literature review, intended to critically evaluate material that has been published on a certain topic or research problem. A literature review is often included as a separate section within a research article.
In the Methods section of a research article, the researchers describe the design of their study and how it was carried out. This may include a description of any instrument used to collect quantitative or qualitative data, the sample or participants, how data was gathered, and any statistical analysis of data.
The Results section of a research article reports the findings of the study, whether they come from quantitative or qualitative measures. In this section the findings are presented to the reader, but it does not include an interpretation of the findings
In the Discussion section, researchers interpret the findings of the study and comment on whether the study answered the problem/s it was attempting to solve. Researchers may also discuss the limitations of the study and suggestions for further research.
The bibliography or references section is a list of all the resources cited within the body of the article.
Other Resources
- Parts of the Research Article: Marymount University Library
- OpenMichigan: Major Content Sections of a Research Report and Related Critiquing Guidelines
- Components of a Research Paper: Center for Innovation in Research and Teaching
- Writing the Empirical Research Article: Book Chapter by D.J. Bem
- << Previous: Books & Other Sources
- Next: Reading and Understanding Research >>
- Last Updated: Feb 9, 2024 2:22 PM
- URL: https://alvernia.libguides.com/researchprocess
- DOI: 10.33168/jsms.2024.0803
- Corpus ID: 270501952
An Empirical Study on the Impact of Organizational Culture on Organizational Citizenship Behavior with Affective Commitment as a Mediator
- Published in Journal of system and… 10 June 2024
- Business, Psychology
Related Papers
Showing 1 through 3 of 0 Related Papers
External shocks and dynamics of resource use patterns: empirical evidence from the Indian manufacturing sector
- Open access
- Published: 19 July 2024
- Volume 5 , article number 159 , ( 2024 )
Cite this article
You have full access to this open access article
- Tiyasa Mishra ORCID: orcid.org/0009-0000-5012-507X 1 &
- Bhagirath Behera ORCID: orcid.org/0000-0001-9413-5675 1
114 Accesses
1 Altmetric
Explore all metrics
While promoting manufacturing growth is imperative to boost economic growth, potential adverse consequences on natural resources and ecology have created sustainability-related concerns and challenges. In India, with the reform processes deepening further and the business environment becoming more market-oriented over the years, the adoption of appropriate measures for sustainable manufacturing growth under such conditions would require a deeper investigation of the underlying dynamics, particularly to address the impacts of external shocks. This is especially true when external shocks alter market dynamics and, hence, input is used significantly in the manufacturing sector. Given this backdrop, the present paper examines how the major external shocks to the Indian economy during in the post-reform era have altered the resource use patterns in selected manufacturing industries. The paper uses secondary data collected from the KLEMS database of the Reserve Bank of India for the period from 1980–81 to 2019–20 and applies descriptive statistics and econometric techniques to address the research objective. It is found that energy intensity, vertical integration, capital intensity, and rate of growth of total factor productivity decreased over the decades, whereas labour productivity experienced an increasing trend in most industries. However, the trends are mixed in the case of material intensity. Further, the regression results indicate significant effects of external shocks on resource use, factor productivity, and structural changes. There are also industry-specific differences in the nature and extent of such changes. Hence, a deeper investigation of the underlying factors and impacts at a disaggregate industry level is necessary to draw more robust insights.
Similar content being viewed by others
Baseline analysis of productivity changes with and without considering carbon dioxide emissions in the major manufacturing sector of indonesia, analysing energy intensity trends and decoupling of growth from energy use in indian manufacturing industries during 1973–1974 to 2011–2012.
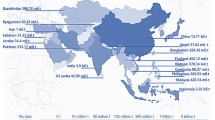
Assessing green energy production and industrial excellence in Asian emerging economies in the context of industrial transformation and sustainable development
Avoid common mistakes on your manuscript.
1 Introduction
While promoting manufacturing growth is imperative to strengthen agriculture-industry linkages and boost overall economic growth, potential adverse consequences on natural resources and the ecology have created sustainability-related concerns and challenges. In particular, rapid technological progress has caused two opposite effects on the manufacturing sector [ 1 ]. On the one hand, process innovations have improved production processes and enhanced resource use efficiency [ 2 ]. On the other hand, product innovations have shortened life cycles considerably in many industries [ 3 ]. In India, with the reform processes deepening further and the business environment becoming more market-oriented over the years, the adoption of appropriate measures for sustainable manufacturing growth under such conditions would require a deeper investigation of the underlying dynamics, particularly to address the impacts of external shocks and maintain the balance between process and product innovations [ 2 , 3 ]. This is especially true when external shocks alter market dynamics and, hence, input is used significantly in the manufacturing sector [ 4 ].
Such changes in the manufacturing sector through external shocks are imperative as the process of economic reforms has made a marked departure with a focus on attracting foreign investments, restructuring public sector enterprises, facilitating international trade, and enhancing market competition [ 5 , 6 ]. For example, the simplification of the trade policies and removal of quantitative restrictions and tariffs have led to favourable changes in the value, composition, and direction of Indian exports [ 7 ]. Further, there has been an acceleration in factor productivity growth, primarily due to technical efficiency and progress improvements [ 8 ]. In addition, macroeconomic policies have significantly helped improve productivity [ 9 ]. While all such changes are expected to promote the growth of different industries, there are challenges with respect to enhancing demand, profit margins, product quality, and technological progress vis-à-vis the global competitors [ 1 , 10 ] and encountering the adverse impacts of external shocks. In particular, with economic reforms making the business environment more market-oriented and external shocks potentially affecting the market dynamics, changes in the use of inputs in manufacturing processes are very likely to occur in the upcoming years.
Besides the effects of external shocks, factors such as rising energy prices [ 11 ] can also cause major challenges to global competitiveness. This is crucial when a weak institutional structure of a developing country impedes the domestic production system [ 12 ]. On the contrary, emerging markets, changing customer choices, global competition, and emphasis on the reliability and safety of products cause additional pressures on firms [ 13 ]. The issues have been emphasized widely in recent years, with Indian firms focussing more on sustainable production processes using cleaner technologies, sustainable raw materials, adoption of renewable energy sources, and other environmental measures. Footnote 1
The paper has six sections. The next section reviews the related literature, whereas Sect. 3 presents the conceptual framework used in the study. The data sources used and the tools and techniques applied are discussed in Sect. 4. The subsequent section presents and analyses the findings. The last section makes the conclusion and policy implications by summarizing the major findings and their implications, along with the limitations of the study and the scope of further research.
2 Review of related literature
The literature review has been divided into different sub-sections to focus on the studies which have considered variables such as labour productivity, energy intensity, material intensity, capital intensity, total factor productivity and vertical integration (considered in the present study) and to understand the challenges faced by the industries. The literature review aims to identify the research gaps and emerging issues from the existing studies to proceed with the present study that can help overcome such gaps.
The performance of the Indian manufacturing industries based on the growth of the value-added, capital intensity, labour productivity and total factor productivity suggests that the productivity growth and efficiency level have not progressed in the post-reform period, as per expectations [ 14 ]. The differences in nature and fund requirements of the industries contribute to the differences in their capital structure [ 15 ]. Existing studies show that domestic firms are more export-intensive as compared to foreign firms, whereas private firms are more export-intensive than public firms [ 16 ]. Accordingly, the export intensity of firms across industries has increased considerably during the post-reform which signals improved global competitiveness of Indian manufacturing firms following economic reforms [ 6 ]. The Indian manufacturing industries have observed an increase in the capital-labour ratio due to higher employment of capital as well as displacement of workers [ 17 ]. High emission intensive industries are capital intensive whereas low emission intensive industries are labour intensive [ 18 ]. Although one advantage of the manufacturing sector is the falling capital-output ratio and labour-capital ratio, however, the falling return on capital can turn out to be a bane for the sector [ 18 , 19 , 20 ].
The increasing level of total factor productivity is related to a lower level of energy per unit. Compared to technological change, technical efficiency can affect the productivity growth of Indian manufacturing industries [ 21 ]. The growth path of total factor productivity growth can vary since it is highly sensitive to the methodology adopted for its estimation [ 22 ]. Due to the economic reforms of 1991, manufacturing industries located in most of the Indian states have experienced faster productivity growth in the post-reform period. However, manufacturing industries located in a few other Indian states, such as Puducherry, Delhi, Bihar, Goa, and Madhya Pradesh, have either faced a slowdown in productivity growth or even a productivity decline in some cases [ 23 ].
Moreover, energy efficiency can improve due to better labour productivity and capacity utilization [ 24 ]. Energy intensity in Indian manufacturing industries depends upon the transformational shift from a less efficient technology or fuel to a more efficient one and also the efficiency gains from the newer technology or fuel adopted technology [ 25 ]. Energy efficiency spillover effects from foreign to local firms have been observed during the post-reform period [ 14 ]. Results show that firm size and raw material intensity help to determine labour productivity at the firm level [ 13 ]. Vertical integration is associated with declining marginal costs and rising mark-ups at the product level. High productivity means a higher level of R&D and investment associated with the manufacturing sector [ 26 ].
Consumer goods and intermediate-producing goods firms largely determine the structure of the manufacturing industry [ 25 ]. Moreover, foreign technology has given dynamic productivity gains in the manufacturing sector [ 27 ]. There have been diverse industry-based technical changes [ 8 ]. Some states have performed better than others in terms of value-added growth. For instance, the manufacturing sector of Gujarat (due to physical infrastructure development) has been more efficient, as compared to all India averages in the post-reform period [ 28 ].
Inefficient resource use, on the one hand, can lead to resource depletion, which is considered an adverse environmental consequence, and, on the other hand, can lead to a reduction in the economic efficiency of the Indian manufacturing industries [ 1 , 29 ]. These industries should also focus on high-grade resource use which can be more productive in the long run. Selection of the appropriate resources becomes crucial during the production process of the manufacturing industries. This can give greater input with lesser energy consumption.
There have been instances of automobile industries being successful due to the adoption of green logistics and green power [ 30 ]. Green manufacturing (based on green and circular economy principles) has become popular over the years [ 31 ]. However, if not properly matched with individual and aggregate perspectives, green manufacturing can result in challenges such as future legislation, public pressure, peer pressure and top management commitment [ 32 ]. India’s challenge is to achieve low carbon-emitting industrial growth. The already-taken research and development initiatives are expected to boost industrial growth, especially for the MSMEs in the coming years [ 33 ].
The existing studies have not focussed on the changes in resource use patterns and productivity due to the impact of different external shocks on the Indian manufacturing sector. They have also neglected the impact on macroeconomic and ecological indicators as a result of external shocks in the economy. In this context, this study examines how the external shocks to the Indian economy during the post-reform period have altered the trends and patterns of resource use and productivity in selected manufacturing industries. Here, the changes in resource use are examined in terms of energy and raw materials use intensity. It is hypothesized that external shocks coupled with structural changes through vertical integration and higher capital intensity would enhance factor productivity, leading to resource use efficiency with respect to both raw materials and energies in manufacturing industries. Hence, the findings of the paper are expected to provide useful insights into encountering the adverse implications of external shocks and making the manufacturing processes sustainable. The rationale behind this study is to focus on the economic changes in the Indian manufacturing sector following the fluctuations in resource use patterns and productivity due to the effect of sudden external shocks. The environmental outcomes as a result of these changes will help to undertake industry-specific policies and regulations in this regard.
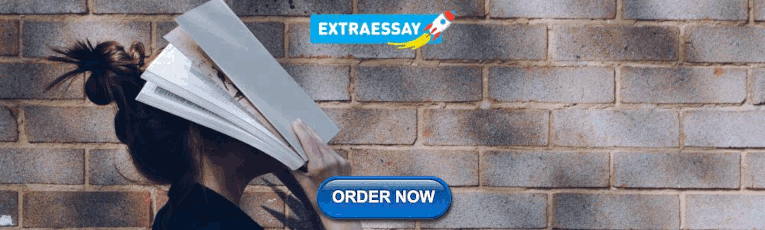
3 Conceptual framework for the study
The conceptual framework of the study is based on the structure-conduct-performance paradigm of Bain (1959) and its subsequent development by [ 34 ]. This framework can potentially deal with the complex relationships amongst market structure, firms’ strategic responses, their performance, and the changes in policies and regulations. Since the present study aims to understand firms’ responses to the changing market dynamics and external shocks, the SCP framework appears appropriate for analysing the resource use patterns in firms of different industries. Figure 1 shows the interlinkages among the different variables used in the study. It is hypothesized that the need for the adoption of a sustainable and cleaner production system coupled with the changes in market dynamics and external shocks have altered the production process through resource use patterns and efficiency in the Indian manufacturing industries. Nevertheless, firms’ responses with respect to resource use and technological and production efficiency differ across industries, given that the competitive business environment and external shocks force the adoption of better and cleaner technologies and business management systems.
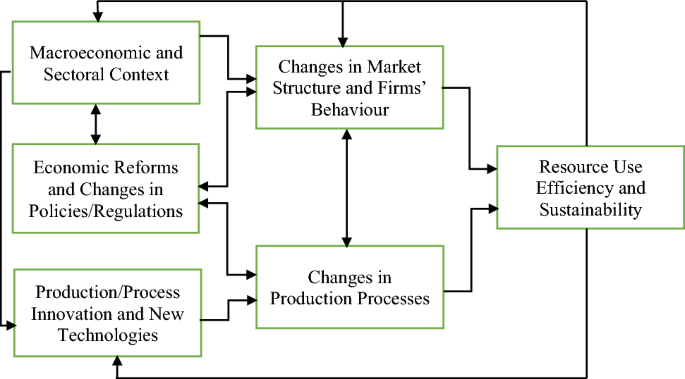
Source: Author’s Design based on Literature Review and Preliminary Analysis
Conceptual framework of the study.
Here, technical progress (although results in a shorter product life cycle) is assumed to be beneficial for the firms through changes in factor productivity and resource use efficiency observed by the Indian manufacturing sector. This is crucial given that economic reforms have led to changes in policies and regulations along with facilitating the adoption of better technologies through FDI inflows and in-house R&D. Further, economic reforms and other external shocks can also potentially alter the market dynamics and other structural aspects in the long run. The conceptual framework portrayed above can potentially explore such interrelationships. Given such interrelationships, the present study uses six indicators to examine the changes following external shocks. Here, the impact on resource use is examined in terms of energy and material intensity, whereas changes in vertical integration and capital intensity are examined to understand the implications for structural aspects. Further, labour productivity and total factor productivity growth are used to examine the efficiency-related implications.
4 Data sources and methodology
The study uses secondary data collected from the KLEMS database of the Reserve Bank of India for the period from 1980–81 to 2019–20 and applies descriptive statistics and econometric techniques to address the research objective. The entire timeframe is divided into pre- (1980–81 to 1990–91) and post-reform period (1992–1993 to 2019–20) for comparative analysis. Notably, considering the abnormal situations, the years 1991–92 and 2009–10 are dropped from the dataset. Further, the post-reform period is divided into three sub-periods with breaks in 1999–2000 and 2010–11 to capture the changes following the South-East Asian crisis Footnote 2 and global economic slowdown respectively. Decadal averages are calculated to understand the trends, patterns, and fluctuations in resource use and other aspects in major industries of the Indian manufacturing sector. Eleven major industries are considered here, and all the variables are measured at constant 2011–12 prices. Table 1 shows the variables along with their measurements. Both correlation and regression analysis have been carried out to understand the degree of association and statistical significance of the changes. Notably, the years 1991–92 and 2009–10 are dropped due to adjustment issues for the base intercept in relation to economic reforms and global economic slowdown respectively.
Panel data models have been estimated separately for material intensity, energy intensity, labour productivity, total factor productivity growth, vertical integration, and capital intensity as dependent variables. More specifically, least-squares dummy variable (LSDV) models have been estimated for each of the variables to examine their industry-specific differences. In addition, the regression models include dummy variables for economic reforms, the South-East Asian crisis, and the global economic slowdown to examine their impacts. These dummy variables are included to examine the impact of these external shocks.
The following least-squares dummy variable model (LSDV) has been estimated:
Here, the dummy variable D 2i = 1 for Textiles, Textile Products, Leather and Footwear industry, 0 otherwise; D 3i = 1 for Wood and Products of wood industry, 0 otherwise; D 4i = 1 for Pulp, Paper, Paper products, Printing and Publishing industry, 0 otherwise; D 5i = 1 for Coke, Refined Petroleum Products and Nuclear fuel industry, 0 otherwise; D 6i = 1 for Chemicals and Chemical Products industry, 0 otherwise; D 7i = 1 for Rubber and Plastic Products industry, 0 otherwise; D 8i = 1 for Basic Metals and Fabricated Metal Products industry, 0 otherwise; D 9i = 1 for Machinery industry, 0 otherwise; D 10i = 1 for Electrical and Optical Equipment industry, 0 otherwise; D 11i = 1 for Transport Equipment industry, 0 otherwise and so on. Further, the dummy variables ER, SEAC and GFC stand for the economic reforms, South East Asian crisis and global financial crisis respectively, with ER = 1 for the period from 1992–93 to 2019–20 as 1 and 0 otherwise, SEAC = 1 for the period from 1999–00 to 2008–09 as 1 and 0 otherwise, and GFC = 1 for the period from 2010–11 to 2019–20 as 1 and 0 otherwise.
5 Results and discussions
5.1 trends and patterns in production processes, 5.1.1 changes in energy and material intensities.
While manufacturing firms are highly dependent on high-quality and imported intermediate goods in order to enhance their productivity [ 35 ], Table 2 shows a considerable change in energy use in different industries with most of them experiencing lesser energy intensity during the post-reform period. According to [ 36 ], an increase in crude oil prices has led to a significant reduction in energy intensity in many industries. Besides, foreign-owned firms appear to be more technically efficient and, hence, less energy-intensive [ 37 ]. It is also argued that firms with higher TFPG or returns to scale output are likely to be more energy efficient [ 38 , 39 ]. Although energy intensity has increased in the food and wood industry, possibly due to growing mechanization [ 36 ], the increase has not been substantial.
Table 3 shows a very high material intensity in the fuel-related industry, possibly because a wide range of by-products can be produced therein [ 40 ]. Notably, material intensity has increased in some industries depending on their nature and the type of raw materials used in such industries. In particular, the use of materials has been consistent over the years in the food, machinery, and transport equipment industries. However, some raw materials are energy intensive as well indicating their complementarity [ 37 , 38 , 39 ]. This is important given that the Indian manufacturing sector has a significant increase in the import of raw materials [ 41 ].
5.1.2 Changes in factor productivities
The TFPG influences overall economic growth, including output productivity, human capital, entrepreneurship, competitiveness, technological development, and innovations [ 8 , 42 ]. Generally, TFPG depends on the age of firms (negative but significant relationship), market share (positive relationship), employees’ contribution (U-shaped relationship) and export intensity (inverted U-shaped relationship) [ 43 ]. This is contradictory to the results obtained from Table 4 . Table 4 shows that TFPG remained positive throughout the period in food products, beverages and tobacco, and electrical and optical equipment. However, many of the industries recorded a deceleration in TFPG in the post-reform period.
Although the textiles had a high TFPG in the post-recession period, the overall TFPG in the industry is not very high due to the sluggish growth rate in the past two decades. A similar case is seen in the wood and wood products industry. The electrical and optical equipment industry recorded a high TFPG during 2000–01 to 2008–09, but it could not be maintained afterward. From Tables 2 and 4 , it has been observed that higher TFPG may not always result in lower energy efficiency as shown in [ 38 , 39 ]
Like TFPG, labour productivity has also varied across industries as well as over time (Table 5 ). Further, except for the wood industry, the rest of the industries have experienced an increase in labour productivity in the post-reform period, possibly due to product and process innovations [ 44 ]. In spite of the increasing dependence on updated technology in the global manufacturing sector, the Indian manufacturing sector has equally focussed on labour use due to unskilled labour. While wood is a natural, renewable as well as biodegradable raw material with legal restrictions and hence little attention given [ 45 ], chemicals and transport equipment industries had a considerably high labour productivity during 2010–11 to 2019–20. These industries have emerged as big exporters, possibly because of their improved product quality [ 46 , 47 ].
5.1.3 Changes in vertical integration and capital intensities
While vertical integration is influenced by factors such as transaction costs, demand variability and market power motive [ 48 ], and information externalities due to uncertainties [ 49 ], it can potentially help the firms in better coordination and investment decisions [ 50 ]. In addition, vertical integration also serves as a strategy for proper management and customization of production according to consumer needs [ 48 ]. However, Table 6 shows that vertical integration has decreased for all the industries in the post-reform period, except for fuel and chemicals which shows that most of the manufacturing industries have neglected the process of vertical integration. This is so possibly because vertical integration reduces flexibility in industrial operations. It is also possible that decreasing externalities have reduced the extent of vertical integration in several industries. On the contrary, fuel and chemical industries have greater vertical integration, possibly due to their lower costs and higher economies of scale. Higher vertical integration indicates better productivity and higher profits.
Besides, capital intensity has also decreased in most of the industries, except food products, chemicals, and fuels during the post-reform period (Table 7 ). Although there are fluctuations, the post-reform period has mostly faced a declining trend due to the aftereffects of the global recession in 2008–09. Further, the capital base of an industry also depends on the size of firms. For example, large firms are likely to have higher capital intensity as compared to small and medium enterprises [ 51 ]. However, the results obtained from Table 7 do not give a clear picture of the industry-specific size of the firms. Apart from size, profitability, non-debt tax shield, growth in assets, liquidity and interest coverage also have a linear relationship with the capital structure of an industry [ 52 ].
Thus, there have been diverse natures and extent of changes in resource use, factor productivity, and structural aspects across different industries of the Indian manufacturing sector during the post-reform period [ 42 ]. While most of the industries have recorded decreasing energy and material intensities, showing a shift towards efficient resource use, lower capital share, and vertical integration may be largely because of changing business environments and opportunities. On the other hand, both labour as well as total factor productivity growth have increased over the years, possibly due to the increasing pressure of competition following the economic reforms of 1991.
The pair-wise correlation matrix presented in Table 8 shows the association between resource use (i.e., material intensity and energy intensity) and other aspects of manufacturing (i.e., vertical integration, labour productivity, total factor productivity growth, and capital intensity). It is found that the nature and extent of association varies across industries. Further, in many cases, this association is not statistically significant. Such variations in association across the variables create the necessity of examining their differences statistically, particularly with experiences of major external shocks. The next sub-section attempts for the same.
5.2 Econometric investigation of changes in production processes
Table 9 shows the summary statistics of the estimated as well as the dummy variables included in the models. The regression results are presented in Tables 10 , 11 and 12 . In the estimated models, the food products are taken as the base industry (for base intercept). Here, three dummy variables (for economic reforms, South-Asian crisis and global economic slowdown) are included to specify the panel data for regression models in the econometric analysis. These dummy variables examine if the respective external shocks have any significant impact. Industry-specific differential intercepts are also estimated. These differential intercepts are deviations from the base intercept. In the estimated models, the respective F-statistic confirms their overall significance, except the model on TFPG (which is significant at 14 percent level).
Table 10 shows that external shocks have affected both material and energy intensity. However, while average energy consumption in Indian industries has reduced following different shocks, material use has decreased following economic reforms only. On the contrary, material intensity is found to be higher after other shocks. External shocks affect the terms of trade of countries leading to a higher focus on domestic production rather than expensive imports. In addition, the differential intercepts of material intensity for machinery and electrical equipment industries are not significant, whereas, in the case of energy intensity, it is not significant for textile and petroleum industries. Importantly, the sign of the differential intercepts differs across industries. This indicates that there are industry-specific differences in average energy or material intensity vis-à-vis food products.
Here, the number of observations is less for TFPG as compared to labour productivity because the first observation is used for the computation of the growth rate (Table 11 ). However, while average labour productivity has been higher following economic reforms and the global economic slowdown, external shocks have no significant impact on TFPG. Further, the differential intercepts of labour productivity are significant for petroleum products, chemicals, machinery, and transport equipment, whereas, in the case of TFPG, it is so for wood and petroleum products. However, while the differential intercepts are negative for TFPG, they are positive in the case of labour productivity.
Table 12 shows the significant impact of external shocks on vertical integration and capital intensity of the manufacturing sector. While the impacts of all the shocks are found to be negative on vertical integration, it is positive for economic reforms in the case of capital intensity (though negative for other shocks). Thus, both vertical integration and capital intensity have decreased following the global economic slowdown and the Southeast Asian crisis. However, while vertical integration has been higher during the post-reform period, it is found to be reversed in the case of capital intensity. Importantly, all the differential intercepts are significant and positive, except for petroleum products. In the case of petroleum products, the differential intercept is significant but negative.
Table 13 summarizes the regression results. It is evident that while average energy consumption has decreased and there has been greater vertical disintegration following the external shocks, the impacts vary in the case of other indicators. For example, material use intensity is found to be lower following economic reforms, but higher after other shocks. However, the impact is found to be reversed in the case of capital intensity. Further, none of the shocks has any significant impact on total factor productivity growth. Importantly, statistical significance and signs of the industry-specific intercepts vary. This indicates that industry-specific resource use, factor productivity and structural aspects differ vis-à-vis food products.
6 Conclusion and policy implications
The paper finds that there has been a significant impact of external shocks on resource use in Indian industries. It is evident that while average energy consumption has decreased and there has been greater vertical disintegration following the external shocks, the impacts vary in the case of other indicators. For example, material use intensity is found to be lower following economic reforms, but higher after other shocks. However, the impact is found to be reversed in the case of capital intensity. Further, none of the shocks significantly impact total factor productivity growth. Importantly, statistical significance and signs of the industry-specific intercepts vary. This indicates that industry-specific resource use, factor productivity and structural aspects differ vis-à-vis food products.
Apart from the food products, chemicals and fuel industries, there has been a decrease in the capital intensity in the post-reform period. On the other hand, the food industry has portrayed a decline in TFPG as compared to other industries in the post-reform period. Moreover, most of these industries have shown a decrease in energy intensity over the years. Although, there has been a slight increase in energy intensity in exceptional cases, however, it is negligible as compared to the pre-reform period. Material intensity has decreased for some industries whereas it has increased for some others depending upon whether the raw materials are domestically produced or imported. Vertical integration has been reduced for most of the industries because of its expensive nature. The results also show that, except for the wood industry, every other industry has shown a significant rise in labour productivity due to product and process innovations. The material intensity and energy intensity have a positive relationship between them in the case of most of the Indian manufacturing industries. There has been a high impact of the structural breaks on both material as well as energy intensities.
It is evident that, except for TFPG, all the indicators show the significant impact of external shocks. Further, the nature and significance of the indicators also differ across the industries. The results obtained from the present study highlight the linkage of different macroeconomic factors with the existing literature. However, it has shown contradictory results in some cases. TFPG does not always follow an inverted U-shape and gives a positive relationship with energy efficiency [ 38 , 39 , 43 ]. Capital intensity may not only depend on firm size [ 51 ]. Such differences may be because of the nature of the industries, market dynamics, firms’ business strategies, and industry-specific policies and regulations. Therefore, further analysis of these aspects is necessary for more robust policies and regulations.
The present study has not highlighted the shock-specific impact on resource use patterns and productivity in the Indian manufacturing sector. The reasons behind the trends and patterns of different macroeconomic variables affecting the manufacturing industries have been neglected. Further scope of the study includes policies and regulations that can prioritize individual factors such as labour, capital, land and entrepreneurship rather than total factor productivity of the Indian manufacturing industries. The policies should also focus on the environmental outcomes as a result of changes in different industry-specific macroeconomic variables.
Data availability
The paper uses secondary data collected from the KLEMS database of the Reserve Bank of India for the period from 1980–81 to 2019–20 which is publicly available. The dataset will be shared on request.
Nevertheless, concerns remain for small and medium enterprises that lack resource use efficiency, innovations, and productivity growth.
Although the South East Asian crisis started in Thailand in 1997–98 and spread to other countries subsequently, here the break is introduced in the year 1999–2000 to capture its aftermath affect as well as the implications of formation of a more stable government in India.
Uddin MMM. What are the dynamic links between agriculture and manufacturing growth and environmental degradation? Evidence from different panel income countries. Environ Sustain Indic. 2020;7:100041.
Google Scholar
Rochina-Barrachina ME, Mañez JA, Sanchis-Llopis JA. Process innovations and firm productivity growth. Small Bus Econ. 2010;34:147–66.
Article Google Scholar
Srinivasan S, Pauwels K, Silva-Risso J, Hanssens DM. Product innovations, advertising, and stock returns. J Mark. 2009;73(1):24–43.
Patnaik A. Measuring demand and supply shocks from COVID-19: an industry-level analysis for India. Margin J Appl Econ Res. 2022;16(1):76–105.
Kanda R. Indian manufacturing sector: a review on the problems & declining scenario of Indian industries. Int J Sci Res. 2015;4:1039–42.
Basant R, Mishra P. Trends in strategies and performance of the Indian corporate sector: What has changed in two decades of economic reforms? Ahmedabad: Indian Institute of Management; 2016.
Sahni P. Trends in India’s exports: a comparative study of pre and post reform period. IOSR J Econ Finance. 2014;3(2):8–18.
Ray SC. Did India’s economic reforms improve efficiency and productivity? A nonparametric analysis of the initial evidence from manufacturing. Indian Econ Rev. 2002;37:23–57.
Ghosh S. Do economic reforms matter for manufacturing productivity? Evidence from the Indian experience. Econ Model. 2013;31:723–33.
Mukherjee S. Challenges to Indian micro small scale and medium enterprises in the era of globalization. J Glob Entrep Res. 2018;8:1–19.
Sadath AC, Acharya RH. Effects of energy price rise on investment: firm level evidence from Indian manufacturing sector. Energy Econ. 2015;49:516–22.
Mani S. The role of industrial policy in market-friendly economies case of COVID-19 vaccine R&D and its manufacturing in India and its contrast with the US-II. Econ Polit Wkly. 2022;57:51–8.
Deshmukh J, Pyne PK. Labour productivity and export performance: firm-level evidence from Indian manufacturing industries since 1991. 2013.
Golder B. Energy intensity of Indian manufacturing firms: effect of energy prices, technology and firm characteristics. Sci Technol Soc. 2011;16(3):351–72.
Manjule R. Impact of capital structure in Indian industries. Int J Sci Eng Res. 2014;5(1):2239–49.
Rentala S, Anand B, Shaban M. Technological capabilities and firm resources as determinants of export competitiveness: evidence from Indian pharmaceutical industry using quantile regression approach. J Med Mark. 2014;14(2–3):133–44.
Das P, Sengupta A. Wages, productivity and employment in Indian manufacturing industries: 1998–2010. J Ind Stat. 2015;4(2):208–20.
Ranjan R. Determinants of emission and energy intensities in indian organized manufacturing sector. Artha Vijnana J Gokhale Inst Polit Econ. 2015. https://doi.org/10.21648/arthavij/2015/v57/i3/109158 .
Krishna KL, Goldar B, Erumban AA, Das PC, Aggarwal SC. Sources of India’s post-reform economic growth: an analysis based on India KLEMS database. Econ Pol Wkly. 2022;57(31):36–43.
Basole A, Narayan A. Long-run performance of the organised manufacturing sector in India: an analysis of sub-periods and industry-level trends. Econ Pol Wkly. 2020;55(10):35–44.
Joshi RN, Singh SP. Estimation of total factor productivity in the Indian garment industry. J Fash Mark Manag Int J. 2010;14(1):145–60.
Pradhan G, Barik K. Fluctuating total factor productivity in India: evidence from selected polluting industries. Econ Polit Wkly. 1998;33:M25–30.
Deb AK, Ray SC. Economic reforms and total factor productivity growth of Indian manufacturing: An inter-state analysis (No. 2013–04). University of Connecticut, Department of Economics. 2013.
Haider S, Bhat JA. Does total factor productivity affect the energy efficiency: evidence from the Indian paper industry. Int J Energy Sect Manage. 2020;14(1):108–25.
Dasgupta S, Roy J. Analysing energy intensity trends and decoupling of growth from energy use in Indian manufacturing industries during 1973–1974 to 2011–2012. Energ Effi. 2017;10(4):925–43.
Stiebale J, Vencappa D. Import competition and vertical integration: evidence from India. J Dev Econ. 2022;155:102790.
Rijesh R. Technology import and manufacturing productivity in India: firm level analysis. J Ind Compet Trade. 2015;15(4):411–34.
Unni J, Lalitha N, Rani U. Economic reforms and productivity trends in Indian manufacturing. Econ Polit Wkly. 2001;36:3914–22.
Zhang W, Ma W, Ji Y, Fan M, Oenema O, Zhang F. Efficiency, economics, and environmental implications of phosphorus resource use and the fertilizer industry in China. Nutr Cycl Agroecosyst. 2008;80:131–44.
Karurkar S, Unnikrishnan S, Panda SS. Study of environmental sustainability and green manufacturing practices in the Indian automobile industry. OIDA Int J Sustain Dev. 2018;11(06):49–62.
Toke LK, Kalpande SD. Critical success factors of green manufacturing for achieving sustainability in Indian context. Int J Sustain Eng. 2019;12(6):415–22.
Mittal VK, Sangwan KS. Prioritizing drivers for green manufacturing: environmental, social and economic perspectives. Procedia Cirp. 2014;15:135–40.
Pulicherla KK, Adapa V, Ghosh M, Ingle P. Current efforts on sustainable green growth in the manufacturing sector to complement “make in India” for making “self-reliant India.” Environ Res. 2022;206:112263.
Article CAS Google Scholar
Tirole J. The theory of industrial organization. Cambridge: MIT press; 1988.
Sharma C. Imported intermediate inputs, R&D, and productivity at firm level: evidence from Indian manufacturing industries. Int Trade J. 2014;28(3):246–63.
Reddy BS, Ray BK. Understanding industrial energy use: physical energy intensity changes in Indian manufacturing sector. Energy Policy. 2011;39(11):7234–43.
Sahu S, Narayanan K. Determinants of energy intensity in Indian manufacturing industries: a firm level analysis. 2010; 4(21646)
Sahu SK, Sharma H. Productivity, energy intensity and output: a unit level analysis of the Indian manufacturing sector. J Quant Econ. 2016;14(2):283–300.
Bala Subrahmanya MH. Energy intensity and economic performance in small scale bricks and foundry clusters in India: does energy intensity matter? Energy Policy. 2006;34(4):489–97. https://doi.org/10.1016/j.enpol.2004.06.016 .
Solomon S. Sugarcane by-products based industries in India. Sugar Tech. 2011;13:408–16.
York R, Ergas C, Rosa EA, Dietz T. It’s a material world: trends in material extraction in China, India, Indonesia, and Japan. Nat Cult. 2011. https://doi.org/10.3167/nc.2011.060201 .
Das N, Bera P. Estimation of TFPG from the cost-function for the Indian manufacturing industries: a panel-time series approach. 2021; 11: 175–192
Maiti S, Chakraborty C. Total factor productivity growth of indian fabrics sector: a firm level analysis. 2018; Vol. XXII, 2017-18, ISSN - 0975-8003
Woltjer G, Van Galen M, Logatcheva K. Industrial innovation, labour productivity, sales and employment. Int J Econ Bus. 2021;28(1):89–113.
Mai C, Kües U, Militz H. Biotechnology in the wood industry. Appl Microbiol Biotechnol. 2004;63:477–94.
Kathuria V, Aiyar A. Link between exporting and productivity: firm level analysis for Indian chemical industry. Sci Technol Soc. 2011;16(3):309–31.
Keshari PK, Saggar M. A firm level study of the determinants of export performance in machinery and transport equipment industry of India. 2013; Vol. 36, No. 3 (1989): pp. 36–48.
Bhuyan S. An empirical evaluation of factors determining vertical integration in US food manufacturing industries. Agribus Int J. 2005;21(3):429–45.
Hennessy DA. Information asymmetry as a reason for food industry vertical integration. Am J Agr Econ. 1996;78(4):1034–43.
Liu X. Vertical integration and innovation. Int J Ind Organ. 2016;47:88–120.
Das S, Roy M. Inter-industry differences in capital structure: evidence from India. Finance India. 2007;21(2):517.
Adhegaonkar V, Indi RM. Determinants of capital structure: a case of Indian chemical industry. Int J Mark Technol. 2012;2(10):130–6.
Download references
Not applicable.
Author information
Authors and affiliations.
Department of Humanities and Social Sciences, Indian Institute of Technology Kharagpur, Kharagpur, West Bengal, 721302, India
Tiyasa Mishra & Bhagirath Behera
You can also search for this author in PubMed Google Scholar
Contributions
TM: idea and contextualisation, introduction and literature review, data collection, analysis, results and discussions. BB: idea and contextualisation, objective, conceptual framework, data collection, analysis, results and discussions, limitations and policy recommendations. Both the authors significantly contributed in finalizing the manuscript.
Corresponding author
Correspondence to Tiyasa Mishra .
Ethics declarations
Ethics approval and consent to participate, informed consent, competing interests.
The authors report there are no competing interests to declare.
Additional information
Publisher's note.
Springer Nature remains neutral with regard to jurisdictional claims in published maps and institutional affiliations.
Rights and permissions
Open Access This article is licensed under a Creative Commons Attribution 4.0 International License, which permits use, sharing, adaptation, distribution and reproduction in any medium or format, as long as you give appropriate credit to the original author(s) and the source, provide a link to the Creative Commons licence, and indicate if changes were made. The images or other third party material in this article are included in the article's Creative Commons licence, unless indicated otherwise in a credit line to the material. If material is not included in the article's Creative Commons licence and your intended use is not permitted by statutory regulation or exceeds the permitted use, you will need to obtain permission directly from the copyright holder. To view a copy of this licence, visit http://creativecommons.org/licenses/by/4.0/ .
Reprints and permissions
About this article
Mishra, T., Behera, B. External shocks and dynamics of resource use patterns: empirical evidence from the Indian manufacturing sector. Discov Sustain 5 , 159 (2024). https://doi.org/10.1007/s43621-024-00362-8
Download citation
Received : 23 May 2024
Accepted : 11 July 2024
Published : 19 July 2024
DOI : https://doi.org/10.1007/s43621-024-00362-8
Share this article
Anyone you share the following link with will be able to read this content:
Sorry, a shareable link is not currently available for this article.
Provided by the Springer Nature SharedIt content-sharing initiative
- Production technology
- Productivity
- Resource use
- Green manufacturing
- Ecological sustainability
Advertisement
- Find a journal
- Publish with us
- Track your research
Cookies on GOV.UK
We use some essential cookies to make this website work.
We’d like to set additional cookies to understand how you use GOV.UK, remember your settings and improve government services.
We also use cookies set by other sites to help us deliver content from their services.
You have accepted additional cookies. You can change your cookie settings at any time.
You have rejected additional cookies. You can change your cookie settings at any time.
King's Speech 2024: background briefing notes
Read the briefing notes on the announcements made in the 2024 King’s Speech.
The King's Speech 2024: background briefing notes
PDF , 556 KB , 104 pages
This file may not be suitable for users of assistive technology.
Updates to this page
The attachment on this page has been updated.
First published.
Sign up for emails or print this page
Is this page useful.
- Yes this page is useful
- No this page is not useful
Help us improve GOV.UK
Don’t include personal or financial information like your National Insurance number or credit card details.
To help us improve GOV.UK, we’d like to know more about your visit today. Please fill in this survey (opens in a new tab) .
Thank you for visiting nature.com. You are using a browser version with limited support for CSS. To obtain the best experience, we recommend you use a more up to date browser (or turn off compatibility mode in Internet Explorer). In the meantime, to ensure continued support, we are displaying the site without styles and JavaScript.
- View all journals
- Explore content
- About the journal
- Publish with us
- Sign up for alerts
- Open access
- Published: 12 July 2024
The nature of the last universal common ancestor and its impact on the early Earth system
- Edmund R. R. Moody ORCID: orcid.org/0000-0002-8785-5006 1 ,
- Sandra Álvarez-Carretero ORCID: orcid.org/0000-0002-9508-6286 1 ,
- Tara A. Mahendrarajah ORCID: orcid.org/0000-0001-7032-6581 2 ,
- James W. Clark 3 ,
- Holly C. Betts 1 ,
- Nina Dombrowski ORCID: orcid.org/0000-0003-1917-2577 2 ,
- Lénárd L. Szánthó ORCID: orcid.org/0000-0003-3363-2488 4 , 5 , 6 ,
- Richard A. Boyle 7 ,
- Stuart Daines 7 ,
- Xi Chen ORCID: orcid.org/0000-0001-7098-607X 8 ,
- Nick Lane ORCID: orcid.org/0000-0002-5433-3973 9 ,
- Ziheng Yang ORCID: orcid.org/0000-0003-3351-7981 9 ,
- Graham A. Shields ORCID: orcid.org/0000-0002-7828-3966 8 ,
- Gergely J. Szöllősi 5 , 6 , 10 ,
- Anja Spang ORCID: orcid.org/0000-0002-6518-8556 2 , 11 ,
- Davide Pisani ORCID: orcid.org/0000-0003-0949-6682 1 , 12 ,
- Tom A. Williams ORCID: orcid.org/0000-0003-1072-0223 12 ,
- Timothy M. Lenton ORCID: orcid.org/0000-0002-6725-7498 7 &
- Philip C. J. Donoghue ORCID: orcid.org/0000-0003-3116-7463 1
Nature Ecology & Evolution ( 2024 ) Cite this article
54k Accesses
886 Altmetric
Metrics details
- Microbial genetics
- Molecular evolution
- Phylogenetics
The nature of the last universal common ancestor (LUCA), its age and its impact on the Earth system have been the subject of vigorous debate across diverse disciplines, often based on disparate data and methods. Age estimates for LUCA are usually based on the fossil record, varying with every reinterpretation. The nature of LUCA’s metabolism has proven equally contentious, with some attributing all core metabolisms to LUCA, whereas others reconstruct a simpler life form dependent on geochemistry. Here we infer that LUCA lived ~4.2 Ga (4.09–4.33 Ga) through divergence time analysis of pre-LUCA gene duplicates, calibrated using microbial fossils and isotope records under a new cross-bracing implementation. Phylogenetic reconciliation suggests that LUCA had a genome of at least 2.5 Mb (2.49–2.99 Mb), encoding around 2,600 proteins, comparable to modern prokaryotes. Our results suggest LUCA was a prokaryote-grade anaerobic acetogen that possessed an early immune system. Although LUCA is sometimes perceived as living in isolation, we infer LUCA to have been part of an established ecological system. The metabolism of LUCA would have provided a niche for other microbial community members and hydrogen recycling by atmospheric photochemistry could have supported a modestly productive early ecosystem.
Similar content being viewed by others
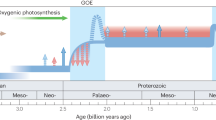
Co‐evolution of early Earth environments and microbial life
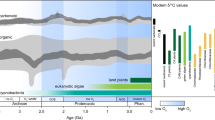
The curious consistency of carbon biosignatures over billions of years of Earth-life coevolution
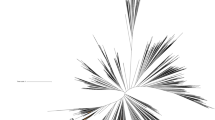
Phanerozoic radiation of ammonia oxidizing bacteria
The common ancestry of all extant cellular life is evidenced by the universal genetic code, machinery for protein synthesis, shared chirality of the almost-universal set of 20 amino acids and use of ATP as a common energy currency 1 . The last universal common ancestor (LUCA) is the node on the tree of life from which the fundamental prokaryotic domains (Archaea and Bacteria) diverge. As such, our understanding of LUCA impacts our understanding of the early evolution of life on Earth. Was LUCA a simple or complex organism? What kind of environment did it inhabit and when? Previous estimates of LUCA are in conflict either due to conceptual disagreement about what LUCA is 2 or as a result of different methodological approaches and data 3 , 4 , 5 , 6 , 7 , 8 , 9 . Published analyses differ in their inferences of LUCA’s genome, from conservative estimates of 80 orthologous proteins 10 up to 1,529 different potential gene families 4 . Interpretations range from little beyond an information-processing and metabolic core 6 through to a prokaryote-grade organism with much of the gene repertoire of modern Archaea and Bacteria 8 , recently reviewed in ref. 7 . Here we use molecular clock methodology, horizontal gene-transfer-aware phylogenetic reconciliation and existing biogeochemical models to address questions about LUCA’s age, gene content, metabolism and impact on the early Earth system.
Estimating the age of LUCA
Life’s evolutionary timescale is typically calibrated to the oldest fossil occurrences. However, the veracity of fossil discoveries from the early Archaean period has been contested 11 , 12 . Relaxed Bayesian node-calibrated molecular clock approaches provide a means of integrating the sparse fossil and geochemical record of early life with the information provided by molecular data; however, constraining LUCA’s age is challenging due to limited prokaryote fossil calibrations and the uncertainty in their placement on the phylogeny. Molecular clock estimates of LUCA 13 , 14 , 15 have relied on conserved universal single-copy marker genes within phylogenies for which LUCA represented the root. Dating the root of a tree is difficult because errors propagate from the tips to the root of the dated phylogeny and information is not available to estimate the rate of evolution for the branch incident on the root node. Therefore, we analysed genes that duplicated before LUCA with two (or more) copies in LUCA’s genome 16 . The root in these gene trees represents this duplication preceding LUCA, whereas LUCA is represented by two descendant nodes. Use of these universal paralogues also has the advantage that the same calibrations can be applied at least twice. After duplication, the same species divergences are represented on both sides of the gene tree 17 , 18 and thus can be assumed to have the same age. This considerably reduces the uncertainty when genetic distance (branch length) is resolved into absolute time and rate. When a shared node is assigned a fossil calibration, such cross-bracing also serves to double the number of calibrations on the phylogeny, improving divergence time estimates. We calibrated our molecular clock analyses using 13 calibrations (see ‘Fossil calibrations’ in Supplementary Information ). The calibration on the root of the tree of life is of particular importance. Some previous studies have placed a younger maximum constraint on the age of LUCA based on the assumption that life could not have survived Late Heavy Bombardment (LHB) (~3.7–3.9 billion years ago (Ga)) 19 . However, the LHB hypothesis is extrapolated and scaled from the Moon’s impact record, the interpretation of which has been questioned in terms of the intensity, duration and even the veracity of an LHB episode 20 , 21 , 22 , 23 . Thus, the LHB hypothesis should not be considered a credible maximum constraint on the age of LUCA. We used soft-uniform bounds, with the maximum-age bound based on the time of the Moon-forming impact (4,510 million years ago (Ma) ± 10 Myr), which would have effectively sterilized Earth’s precursors, Tellus and Theia 13 . Our minimum bound on the age of LUCA is based on low δ 98 Mo isotope values indicative of Mn oxidation compatible with oxygenic photosynthesis and, therefore, total-group Oxyphotobacteria in the Mozaan Group, Pongola Supergroup, South Africa 24 , 25 , dated minimally to 2,954 Ma ± 9 Myr (ref. 26 ).
Our estimates for the age of LUCA are inferred with a concatenated and a partitioned dataset, both consisting of five pre-LUCA paralogues: catalytic and non-catalytic subunits from ATP synthases, elongation factor Tu and G, signal recognition protein and signal recognition particle receptor, tyrosyl-tRNA and tryptophanyl-tRNA synthetases, and leucyl- and valyl-tRNA synthetases 27 . Marginal densities (commonly referred to as effective priors) fall within calibration densities (that is, user-specified priors) when topologically adjacent calibrations do not overlap temporally, but may differ when they overlap, to ensure the relative age relationships between ancestor-descendant nodes. We consider the marginal densities a reasonable interpretation of the calibration evidence given the phylogeny; we are not attempting to test the hypothesis that the fossil record is an accurate temporal archive of evolutionary history because it is not 28 . The duplicated LUCA node age estimates we obtained under the autocorrelated rates (geometric Brownian motion (GBM)) 29 , 30 and independent-rates log-normal (ILN) 31 , 32 relaxed-clock models with our partitioned dataset (GBM, 4.18–4.33 Ga; ILN, 4.09–4.32 Ga; Fig. 1 ) fall within our composite age estimate for LUCA ranging from 3.94 Ga to 4.52 Ga, comparable to previous studies 13 , 18 , 33 . Dating analyses based on single genes, or concatenations that excluded each gene in turn, returned compatible timescales (Extended Data Figs. 1 and 2 and ‘Additional methods’ in Methods ).
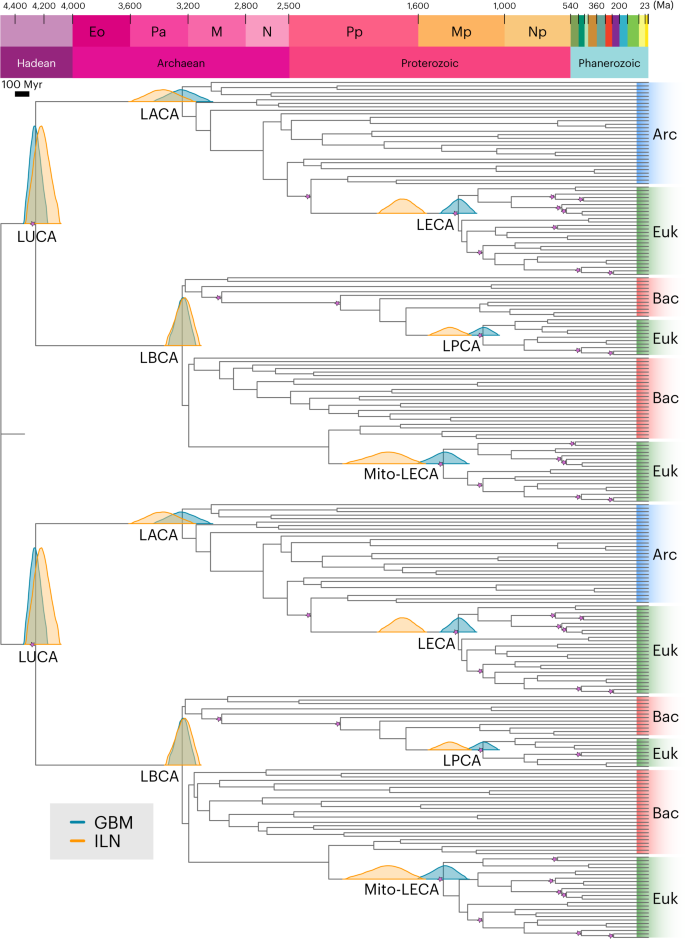
Our results suggest that LUCA lived around 4.2 Ga, with a 95% confidence interval spanning 4.09–4.33 Ga under the ILN relaxed-clock model (orange) and 4.18–4.33 Ga under the GBM relaxed-clock model (teal). Under a cross-bracing approach, nodes corresponding to the same species divergences (that is, mirrored nodes) have the same posterior time densities. This figure shows the corresponding posterior time densities of the mirrored nodes for the last universal, archaeal, bacterial and eukaryotic common ancestors (LUCA, LACA, LBCA and LECA, respectively); the last common ancestor of the mitochondrial lineage (Mito-LECA); and the last plastid-bearing common ancestor (LPCA). Purple stars indicate nodes calibrated with fossils. Arc, Archaea; Bac, Bacteria; Euk, Eukarya.
LUCA’s physiology
To estimate the physiology of LUCA, we first inferred an updated microbial phylogeny from 57 phylogenetic marker genes (see ‘Universal marker genes’ in Methods ) on 700 genomes, comprising 350 Archaea and 350 Bacteria 15 . This tree was in good agreement with recent phylogenies of the archaeal and bacterial domains of life 34 , 35 . For example, the TACK 36 and Asgard clades of Archaea 37 , 38 , 39 and Gracilicutes within Bacteria 40 , 41 were recovered as monophyletic. However, the analysis was equivocal as to the phylogenetic placement of the Patescibacteria (CPR) 42 and DPANN 43 , which are two small-genome lineages that have been difficult to place in trees. Approximately unbiased 44 tests could not distinguish the placement of these clades, neither at the root of their respective domains nor in derived positions, with CPR sister to Chloroflexota (as reported recently in refs. 35 , 41 , 45 ) and DPANN sister to Euryarchaeota. To account for this phylogenetic uncertainty, we performed LUCA reconstructions on two trees: our maximum likelihood (ML) tree (topology 1; Extended Data Fig. 3 ) and a tree in which CPR were placed as the sister of Chloroflexota, with DPANN sister to all other Archaea (topology 2; Extended Data Fig. 4 ). In both cases, the gene families mapped to LUCA were very similar (correlation of LUCA presence probabilities (PP), r = 0.6720275, P < 2.2 × 10 − 16 ). We discuss the results on the tree with topology 2 and discuss the residual differences in Supplementary Information , ‘Topology 1’ (Supplementary Data 1 ).
We used the probabilistic gene- and species-tree reconciliation algorithm ALE 46 to infer the evolution of gene family trees for each sampled entry in the KEGG Orthology (KO) database 47 on our species tree. ALE infers the history of gene duplications, transfers and losses based on a comparison between a distribution of bootstrapped gene trees and the reference species tree, allowing us to estimate the probability that the gene family was present at a node in the tree 35 , 48 , 49 . This reconciliation approach has several advantages for drawing inferences about LUCA. Most gene families have experienced gene transfer since the time of LUCA 50 , 51 and so explicitly modelling transfers enables us to include many more gene families in the analysis than has been possible using previous approaches. As the analysis is probabilistic, we can also account for uncertainty in gene family origins and evolutionary history by averaging over different scenarios using the reconciliation model. Using this approach, we estimated the probability that each KEGG gene family (KO) was present in LUCA and then used the resulting probabilities to construct a hypothetical model of LUCA’s gene content, metabolic potential (Fig. 2 ) and environmental context (Fig. 3 ). Using the KEGG annotation is beneficial because it allows us to connect our inferences to curated functional annotations; however, it has the drawback that some widespread gene families that were likely present in LUCA are divided into multiple KO families that individually appear to be restricted to particular taxonomic groups and inferred to have arisen later. To account for this limitation, we also performed an analysis of COG (Clusters of Orthologous Genes) 52 gene families, which correspond to more coarse-grained functional annotations (Supplementary Data 2 ).
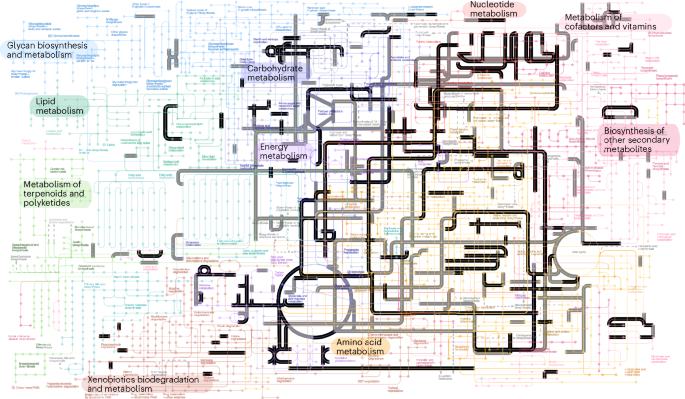
In black: enzymes and metabolic pathways inferred to be present in LUCA with at least PP = 0.75, with sampling in both prokaryotic domains. In grey: those inferred in our least-stringent threshold of PP = 0.50. The analysis supports the presence of a complete WLP and an almost complete TCA cycle across multiple confidence thresholds. Metabolic maps derived from KEGG 47 database through iPath 109 . GPI, glycosylphosphatidylinositol; DDT, 1,1,1-trichloro-2,2-bis(p-chlorophenyl)ethane.
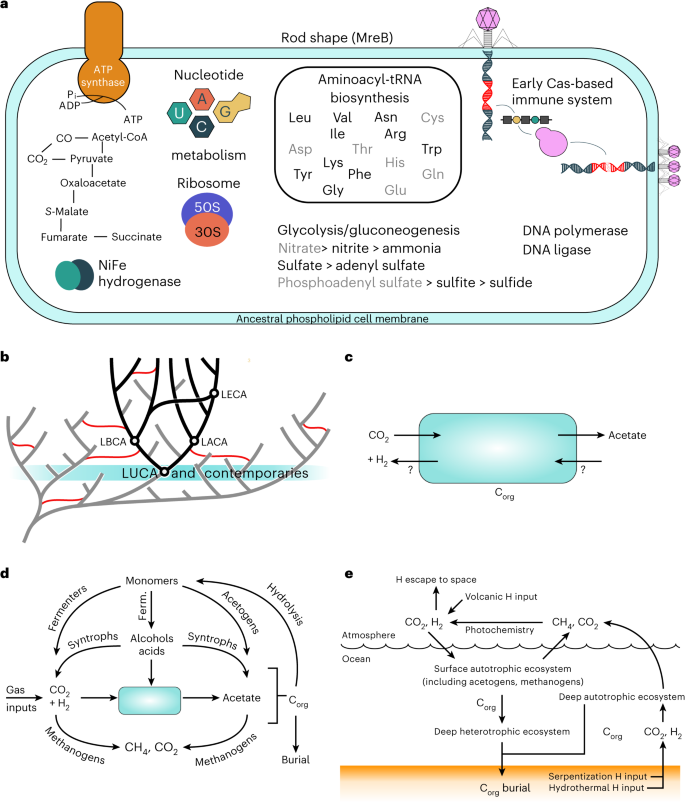
a , A representation of LUCA based on our ancestral gene content reconstruction. Gene names in black have been inferred to be present in LUCA under the most-stringent threshold (PP = 0.75, sampled in both domains); those in grey are present at the least-stringent threshold (PP = 0.50, without a requirement for presence in both domains). b , LUCA in the context of the tree of life. Branches on the tree of life that have left sampled descendants today are coloured black, those that have left no sampled descendants are in grey. As the common ancestor of extant cellular life, LUCA is the oldest node that can be reconstructed using phylogenetic methods. It would have shared the early Earth with other lineages (highlighted in teal) that have left no descendants among sampled cellular life today. However, these lineages may have left a trace in modern organisms by transferring genes into the sampled tree of life (red lines) before their extinction. c , LUCA’s chemoautotrophic metabolism probably relied on gas exchange with the immediate environment to achieve organic carbon (C org ) fixation via acetogenesis and it may also have run the metabolism in reverse. d , LUCA within the context of an early ecosystem. The CO 2 and H 2 that fuelled LUCA’s plausibly acetogenic metabolism could have come from both geochemical and biotic inputs. The organic matter and acetate that LUCA produced could have created a niche for other metabolisms, including ones that recycled CO 2 and H 2 (as in modern sediments). e , LUCA in an Earth system context. Acetogenic LUCA could have been a key part of both surface and deep (chemo)autotrophic ecosystems, powered by H 2 . If methanogens were also present, hydrogen would be released as CH 4 to the atmosphere, converted to H 2 by photochemistry and thus recycled back to the surface ecosystem, boosting its productivity. Ferm., fermentation.
Genome size and cellular features
By using modern prokaryotic genomes as training data, we used a predictive model to estimate the genome size and the number of protein families encoded by LUCA based on the relationship between the number of KEGG gene families and the total number of proteins encoded by modern prokaryote genomes (Extended Data Figs. 5 and 6 ). On the basis of the PPs for KEGG KO gene families, we identified a conservative subset of 399 KOs that were likely to be present in LUCA, with PPs ≥0.75, and found in both Archaea and Bacteria (Supplementary Data 1 ); these families form the basis of our metabolic reconstruction. However, by integrating over the inferred PPs of all KO gene families, including those with low probabilities, we also estimate LUCA’s genome size. Our predictive model estimates a genome size of 2.75 Mb (2.49–2.99 Mb) encoding 2,657 (2,451–2,855) proteins ( Methods ). Although we can estimate the number of genes in LUCA’s genome, it is more difficult to identify the specific gene families that might have already been present in LUCA based on the genomes of modern Archaea and Bacteria. It is likely that the modern version of the pathways would be considered incomplete based on LUCA’s gene content through subsequent evolutionary changes. We should therefore expect reconstructions of metabolic pathways to be incomplete due to this phylogenetic noise and other limitations of the analysis pipeline. For example, when looking at genes and pathways that can uncontroversially be mapped to LUCA, such as the ribosome and aminoacyl-tRNA synthetases for implementing the genetic code, we find that we map many (but not all) of the key components to LUCA (see ‘Notes’ in Supplementary Information ). We interpret this to mean that our reconstruction is probably incomplete but our interpretation of LUCA’s metabolism relies on our inference of pathways, not individual genes.
The inferred gene content of LUCA suggests it was an anaerobe as we do not find support for the presence of terminal oxidases (Supplementary Data 1 ). Instead we identified almost all genes encoding proteins of the archaeal (and most of the bacterial) versions of the Wood–Ljungdahl pathway (WLP) (PP > 0.7), indicating that LUCA had the potential for acetogenic growth and/or carbon fixation 53 , 54 , 55 (Supplementary Data 3 ). LUCA encoded some NiFe hydrogenase subunits ( K06281 , PP = 0.90; K14126 , PP = 0.92), which may have enabled growth on hydrogen (see ‘Notes’ in Supplementary Information ). Complexes involved in methanogenesis such as methyl-coenzyme M reductase and tetrahydromethanopterin S-methyltransferase were inferred to be absent, suggesting that LUCA was unlikely to function as a modern methanogen. We found strong support for some components of the TCA cycle (including subunits of oxoglutarate/2-oxoacid ferredoxin oxidoreductase ( K00175 and K00176 ), succinate dehydrogenase ( K00239 ) and homocitrate synthase ( K02594 )), although some steps are missing. LUCA was probably capable of gluconeogenesis/glycolysis in that we find support for most subunits of enzymes involved in these pathways (Supplementary Data 1 and 3 ). Considering the presence of the WLP, this may indicate that LUCA had the ability to grow organoheterotrophically and potentially also autotrophically. Gluconeogenesis would have been important in linking carbon fixation to nucleotide biosynthesis via the pentose phosphate pathway, most enzymes of which seem to be present in LUCA (see ‘Notes’ in Supplementary Information ). We found no evidence that LUCA was photosynthetic, with low PPs for almost all components of oxygenic and anoxygenic photosystems (Supplementary Data 3 ).
We find strong support for the presence of ATP synthase, specifically, the A ( K02117 , PP = 0.98) and B ( K02118 , PP = 0.94) subunit components of the hydrophilic V/A1 subunit, and the I (subunit a, K02123 , PP = 0.99) and K (subunit c, K02124 , PP = 0.82) subunits of the transmembrane V/A0 subunit. In addition, if we relax the sampling threshold, we also infer the presence of the F1-type β-subunit ( K02112 , PP = 0.94). This is consistent with many previous studies that have mapped ATP synthase subunits to LUCA 6 , 17 , 18 , 56 , 57 .
We obtain moderate support for the presence of pathways for assimilatory nitrate (ferredoxin-nitrate reductase, K00367 , PP = 0.69; ferredoxin-nitrite reductase, K00367 , PP = 0.53) and sulfate reduction (sulfate adenylyltransferase, K00957 , PP = 0.80, and K00958 , PP = 0.73; sulfite reductase, K00392 , PP = 0.82; phosphoadenosine phosphosulfate reductase, K00390 , PP = 0.56), probably to fuel amino acid biosynthesis, for which we inferred the presence of 37 partially complete pathways.
We found support for the presence of 19 class 1 CRISPR–Cas effector protein families in the genome of LUCA, including types I and III (cas3, K07012 , PP = 0.80, and K07475 , PP = 0.74; cas10, K07016 , PP = 0.96, and K19076 , PP = 0.67; and cas7, K07061 , PP = 0.90, K09002 , PP = 0.84, K19075 , PP = 0.97, K19115 , PP = 0.98, and K19140 , PP = 0.80). The absence of Cas1 and Cas2 may suggest LUCA encoded an early Cas system with the means to deliver an RNA-based immune response by cutting (Cas6/Cas3) and binding (CSM/Cas10) RNA, but lacking the full immune-system-site CRISPR. This supports the idea that the effector stage of CRISPR–Cas immunity evolved from RNA sensing for signal transduction, based on the similarities in RNA binding modules of the proteins 58 . This is consistent with the idea that cellular life was already involved in an arms race with viruses at the time of LUCA 59 , 60 . Our results indicate that an early Cas system was an ancestral immune system of extant cellular life.
Altogether, our metabolic reconstructions suggest that LUCA was a relatively complex organism, similar to extant Archaea and Bacteria 6 , 7 . On the basis of ancient duplications of the Sec and ATP synthase genes before LUCA, along with high PPs for key components of those systems, membrane-bound ATP synthase subunits, genes involved in peptidoglycan synthesis ( mraY , K01000 ; murC , K01924 ) and the cytoskeletal actin-like protein, MreB ( K03569 ) (Supplementary Data 3 ), it is highly likely that LUCA possessed the core cellular apparatus of modern prokaryotic life. This might include the basic constituents of a phospholipid membrane, although our analysis did not conclusively establish its composition. In particular, we recovered the following enzymes involved in the synthesis of ether and ester lipids, (alkyldihydroxyacetonephosphate synthase, glycerol 3-phosphate and glycerol 1-phosphate) and components of the mevalonate pathway (mevalonate 5-phosphate dehydratase (PP = 0.84), hydroxymethylglutaryl-CoA reductase (PP = 0.52), mevalonate kinase (PP = 0.51) and hydroxymethylglutaryl-CoA synthase (PP = 0.51)).
Compared with previous estimates of LUCA’s gene content, we find 81 overlapping COG gene families with the consensus dataset of ref. 7 and 69 overlapping KOs with the dataset of ref. 6 . Key points of agreement between previous studies include the presence of signal recognition particle protein, ffh (COG0541, K03106 ) 7 used in the targeting and delivery of proteins for the plasma membrane, a high number of aminoacyl-tRNA synthetases for amino acid synthesis and glycolysis/gluconeogenesis enzymes.
Ref. 6 inferred LUCA to be a thermophilic anaerobic autotroph using the WLP for carbon fixation based on the presence of a single enzyme (CODH), and similarly suggested that LUCA was capable of nitrogen fixation using a nitrogenase. Our reconstruction agrees with ref. 6 that LUCA was an anaerobic autotroph using the WLP for carbon fixation, but we infer the presence of a much more complete WLP than that previously obtained. We did not find strong evidence for nitrogenase or nitrogen fixation, and the reconstruction was not definitive with respect to the optimal growth environment of LUCA.
We used a probabilistic approach to reconstruct LUCA—that is, we estimated the probability with which each gene family was present in LUCA based on a model of how gene families evolve along an overarching species tree. This approach differs from analyses of phylogenetic presence–absence profiles 3 , 4 , 9 or those that used filtering criteria (such as broadly distributed or highly vertically evolving families) to define a high-confidence subset of modern genes that might have been present in LUCA. Our reconstruction maps many more genes to LUCA—albeit each with lower probability—than previous analyses 8 and yields an estimate of LUCA’s genome size that is within the range of modern prokaryotes. The result is an incomplete picture of a cellular organism that was prokaryote grade rather than progenotic 2 and that, similarly to prokaryotes today, probably existed as part of an ecosystem. As the common ancestor of sampled, extant prokaryotic life, LUCA is the oldest node on the species tree that we can reconstruct via phylogenomics but, as Fig. 3 illustrates, it was already the product of a highly innovative period in evolutionary history during which most of the core components of cells were established. By definition, we cannot reconstruct LUCA’s contemporaries using phylogenomics but we can propose hypotheses about their physiologies based on the reconstructed LUCA whose features immediately suggest the potential for interactions with other prokaryotic metabolisms.
LUCA’s environment, ecosystem and Earth system context
The inference that LUCA used the WLP helps constrain the environment and ecology in which it could have lived. Modern acetogens can grow autotrophically on H 2 (and CO 2 ) or heterotrophically on a wide range of alternative electron donors including alcohols, sugars and carboxylic acids 55 . This metabolic flexibility is key to their modern ecological success. Acetogenesis, whether autotrophic or heterotrophic, has a low energy yield and growth efficiency (although use of the reductive acetyl-CoA pathway for both energy production and biosynthesis reduces the energy cost of biosynthesis). This would be consistent with an energy-limited early biosphere 61 .
If LUCA functioned as an organoheterotrophic acetogen, it was necessarily part of an ecosystem containing autotrophs providing a source of organic compounds (because the abiotic source flux of organic molecules was minimal on the early Earth). Alternatively, if LUCA functioned as a chemoautotrophic acetogen it could (in principle) have lived independently off an abiotic source of H 2 (and CO 2 ). However, it is implausible that LUCA would have existed in isolation as the by-products of its chemoautotrophic metabolism would have created a niche for a consortium of other metabolisms (as in modern sediments) (Fig. 3d ). This would include the potential for LUCA itself to grow as an organoheterotroph.
A chemoautotrophic acetogenic LUCA could have occupied two major potential habitats (Fig. 3e ): the first is the deep ocean where hydrothermal vents and serpentinization of sea-floor provided a source of H 2 (ref. 62 ). Consistent with this, we find support for the presence of reverse gyrase (PP = 0.97), a hallmark enzyme of hyperthermophilic prokaryotes 6 , 63 , 64 , 65 , which would not be expected if early life existed at the ocean surface (although the evolution of reverse gyrase is complex 63 ; see ‘Reverse gyrase’ in Supplementary Information ). The second habitat is the ocean surface where the atmosphere would have provided a source of H 2 derived from volcanoes and metamorphism. Indeed, we detected the presence of spore photoproduct lyase (COG1533, K03716 , PP = 0.88) that in extant organisms repairs methylene-bridged thymine dimers occurring in spore DNA as a result of damage induced through ultraviolet (UV) radiation 66 , 67 . However, this gene family also occurs in modern taxa that neither form endospores nor dwell in environments where they are likely to accrue UV damage to their DNA and so is not an exclusive hallmark of environments exposed to UV. Previous studies often favoured a deep-ocean environment for LUCA as early life would have been better protected there from an episode of LHB. However, if the LHB was less intense than initially proposed 20 , 22 , or just a sampling artefact 21 , these arguments weaken. Another possibility may be that LUCA inhabited a shallow hydrothermal vent or a hot spring.
Hydrogen fluxes in these ecosystems could have been several times higher on the early Earth (with its greater internal heat source) than today. Volcanism today produces ~1 × 10 12 mol H 2 yr −1 and serpentinization produces ~0.4 × 10 12 mol H 2 yr − 1 . With the present H 2 flux and the known scaling of the H 2 escape rate to space, an abiotic atmospheric concentration of H 2 of ~150 ppmv is predicted 68 . Chemoautotrophic acetogens would have locally drawn down the concentration of H 2 (in either surface or deep niche) but their low growth efficiency would ensure H 2 (and CO 2 ) remained available. This and the organic matter and acetate produced would have created niches for other metabolisms, including methanogenesis (Fig. 3d ).
On the basis of thermodynamic considerations, CH 4 and CO 2 are expected to be the eventual metabolic end products of the resulting ecosystem, with a small fraction of the initial hydrogen consumption buried as organic matter. The resulting flux of CH 4 to the atmosphere would fuel photochemical H 2 regeneration and associated productivity in the surface ocean (Fig. 3e ). Existing models suggest the resulting global H 2 recycling system is highly effective, such that the supply flux of H 2 to the surface could have exceeded the volcanic input of H 2 to the atmosphere by at least an order of magnitude, in turn implying that the productivity of such a biosphere was boosted by a comparable factor 69 . Photochemical recycling to CO would also have supported a surface niche for organisms consuming CO (ref. 69 ).
In deep-ocean habitats, there could be some localized recycling of electrons (Fig. 3d ) but a quantitative loss of highly insoluble H 2 and CH 4 to the atmosphere and minimal return after photochemical conversion of CH 4 to H 2 means global recycling to depth would be minimal (Fig. 3e ). Hence the surface environment for LUCA could have become dominant (albeit recycling of the resulting organic matter could be spread through ocean depth; ‘Deep heterotrophic ecosystem’ in Fig. 3e ). The global net primary productivity of an early chemoautotrophic biosphere including acetogenic LUCA and methanogens could have been of order ~1 × 10 12 to 7 × 10 12 mol C yr − 1 (~3 orders of magnitude less than today) 69 .
The nutrient supply (for example, N) required to support such a biosphere would need to balance that lost in the burial flux of organic matter. Earth surface redox balance dictates that hydrogen loss to space and burial of electrons/hydrogen must together balance input of electrons/hydrogen. Considering contemporary H 2 inputs, and the above estimate of net primary productivity, this suggests a maximum burial flux in the order of ~10 12 mol C yr − 1 , which, with contemporary stoichiometry (C:N ratio of ~7) could demand >10 11 mol N yr − 1 . Lightning would have provided a source of nitrite and nitrate 70 , consistent with LUCA’s inferred pathways of nitrite and (possibly) nitrate reduction. However, it would only have been of the order 3 × 10 9 mol N yr − 1 (ref. 71 ). Instead, in a global hydrogen-recycling system, HCN from photochemistry higher in the atmosphere, deposited and hydrolysed to ammonia in water, would have increased available nitrogen supply by orders of magnitude toward ~3 × 10 12 mol N yr − 1 (refs. 71 , 72 ). This HCN pathway is consistent with the anomalously light nitrogen isotopic composition of the earliest plausible biogenic matter of 3.8–3.7 Ga (ref. 73 ), although that considerably postdates our inferred age of LUCA. These considerations suggest that the proposed LUCA biosphere (Fig. 3e ) would have been energy or hydrogen limited not nitrogen limited.
Conclusions
By treating gene presence probabilistically, our reconstruction maps many more genes (2,657) to LUCA than previous analyses and results in an estimate of LUCA’s genome size (2.75 Mb) that is within the range of modern prokaryotes. The result is a picture of a cellular organism that was prokaryote grade rather than progenotic 2 and that probably existed as a component of an ecosystem, using the WLP for acetogenic growth and carbon fixation. We cannot use phylogenetics to reconstruct other members of this early ecosystem but we can infer their physiologies based on the metabolic inputs and outputs of LUCA. How evolution proceeded from the origin of life to early communities at the time of LUCA remains an open question, but the inferred age of LUCA (~4.2 Ga) compared with the origin of the Earth and Moon suggests that the process required a surprisingly short interval of geologic time.
Universal marker genes
A list of 298 markers were identified by creating a non-redundant list of markers used in previous studies on archaeal and bacterial phylogenies 10 , 35 , 38 , 74 , 75 , 76 , 77 , 78 , 79 . These markers were mapped to the corresponding COG, arCOG and TIGRFAM profile to identify which profile is best suited to extract proteins from taxa of interest. To evaluate whether the markers cover all archaeal and bacterial diversity, proteins from a set of 574 archaeal and 3,020 bacterial genomes were searched against the COG, arCOG and TIGRFAM databases using hmmsearch (v.3.1b2; settings, hmmsearch–tblout output–domtblout–notextw) 52 , 80 , 81 , 82 . Only hits with an e-value less than or equal to 1 × 10 −5 were investigated further and for each protein the best hit was determined based on the e-value (expect value) and bit-score. Results from all database searches were merged based on the protein identifiers and the table was subsetted to only include hits against the 298 markers of interest. On the basis of this table we calculated whether the markers occurred in Archaea, Bacteria or both Archaea and Bacteria. Markers were only included if they were present in at least 50% of taxa and contained less than 10% of duplications, leaving a set of 265 markers. Sequences for each marker were aligned using MAFFT L-INS-i v.7.407 (ref. 83 ) for markers with less than 1,000 sequences or MAFFT 84 for those with more than 1,000 sequences (setting, –reorder) 84 and sequences were trimmed using BMGE 85 , set for amino acids, a BLOcks SUbstitution Matrix 30 similarity matrix, with a entropy score of 0.5 (v.1.12; settings, -t AA -m BLOSUM30 -h 0.5). Single gene trees were generated with IQ-TREE 2 (ref. 86 ), using the LG substitution matrix, with ten-profile mixture models, four CPUs, with 1,000 ultrafast bootstraps optimized by nearest neighbour interchange written to a file retaining branch lengths (v.2.1.2; settings, -m LG + C10 + F + R -nt 4 -wbtl -bb 1,000 -bnni). These single gene trees were investigated for archaeal and bacterial monophyly and the presence of paralogues. Markers that failed these tests were not included in further analyses, leaving a set of 59 markers (3 arCOGs, 46 COGs and 10 TIGRFAMs) suited for phylogenies containing both Archaea and Bacteria (Supplementary Data 4 ).
Marker gene sequence selection
To limit selecting distant paralogues and false positives, we used a bidirectional or reciprocal approach to identify the sequences corresponding to the 59 single-copy markers. In the first inspection (query 1), the 350 archaeal and 350 bacterial reference genomes were queried against all arCOG HMM (hidden Markov model) profiles (All_Arcogs_2018.hmm), all COG HMM profiles (NCBI_COGs_Oct2020.hmm) and all TIGRFAM HMM profiles (TIGRFAMs_15.0_HMM.LIB) using a custom script built on hmmsearch: hmmsearchTable <genomes.faa> <database.hmm> -E 1 × 10 −5 >HMMscan_Output_e5 (HMMER v.3.3.2) 87 . HMM profiles corresponding to the 59 single-copy marker genes (Supplementary Data 4 ) were extracted from each query and the best-hit sequences were identified based on the e-value and bit-score. We used the same custom hmmsearchTable script and conditions (see above) in the second inspection (query 2) to query the best-hit sequences identified above against the full COG HMM database (NCBI_COGs_Oct2020.hmm). Results were parsed and the COG family assigned in query 2 was compared with the COG family assigned to sequences based on the marker gene identity (Supplementary Data 4 ). Sequence hits were validated using the matching COG identifier, resulting in 353 mismatches (that is, COG family in query 1 does not match COG family in query 2) that were removed from the working set of marker gene sequences. These sequences were aligned using MAFFT L-INS-i 83 and then trimmed using BMGE 85 with a BLOSUM30 matrix. Individual gene trees were inferred under ML using IQ-TREE 2 (ref. 86 ) with model fitting, including both the default homologous substitution models and the following complex heterogeneous substitution models (LG substitution matrices with 10–60-profile mixture models, with empirical base frequencies and a discrete gamma model with four categories accounting for rate heterogeneity across sites): LG + C60 + F + G, LG + C50 + F + G, LG + C40 + F + G, LG + C30 + F + G, LG + C20 + F + G and LG + C10 + F + G, with 10,000 ultrafast bootstraps and 10 independent runs to avoid local optima. These 59 gene trees were manually inspected and curated over multiple rounds. Any horizontal gene transfer events, paralogous genes or sequences that violated domain monophyly were removed and two genes (arCOG01561, tuf ; COG0442, ProS ) were dropped at this stage due to the high number of transfer events, resulting in 57 single-copy orthologues for further tree inference.
Species-tree inference
These 57 orthologous sequences were concatenated and ML trees were inferred after three independent runs with IQ-TREE 2 (ref. 86 ) using the same model fitting and bootstrap settings as described above. The tree with the highest log-likelihood of the three runs was chosen as the ML species tree (topology 1). To test the effect of removing the CPR bacteria, we removed all CPR bacteria from the alignment before inferring a species tree (same parameters as above). We also performed approximately unbiased 44 tree topology tests (with IQ-TREE 2 (ref. 86 ), using LG + C20 + F + G) when testing the significance of constraining the species-tree topology (ML tree; Supplementary Fig. 1 ) to have a DPANN clade as sister to all other Archaea (same parameters as above but with a minimally constrained topology with monophyletic Archaea and DPANN sister to other Archaea present in a polytomy (Supplementary Fig. 2 )) and testing a constraint of CPR to be sister to Chloroflexi (Supplementary Fig. 3 ), and a combination of both the DPANN and CPR constraints (topology 2); these were tested against the ML topology, both using the normal 20 amino acid alignments and also with Susko–Roger recoding 88 .
Gene families
For the 700 representative species 15 , gene family clustering was performed using EGGNOGMAPPER v.2 (ref. 89 ), with the following parameters: using the DIAMOND 90 search, a query cover of 50% and an e-value threshold of 0.0000001. Gene families were collated using their KEGG 47 identifier, resulting in 9,365 gene families. These gene families were then aligned using MAFFT 84 v.7.5 with default settings and trimmed using BMGE 85 (with the same settings as above). Five independent sets of ML trees were then inferred using IQ-TREE 2 (ref. 86 ), using LG + F + G, with 1,000 ultrafast bootstrap replicates. We also performed a COG-based clustering analysis in which COGs were assigned based on the modal COG identifier annotated for each KEGG gene family based on the results from EGGNOGMAPPER v.2 (ref. 89 ). These gene families were aligned, trimmed and one set of gene trees (with 1,000 ultrafast bootstrap replicates) was inferred using the same parameters as described above for the KEGG gene families.
Reconciliations
The five sets of bootstrap distributions were converted into ALE files, using ALEobserve, and reconciled against topology 1 and topology 2 using ALEml_undated 91 with the fraction missing for each genome included (where available). Gene family root origination rates were optimized for each COG functional category as previously described 35 and families were categorized into four different groups based on the probability of being present in the LUCA node in the tree. The most-stringent category was that with sampling above 1% in both domains and a PP ≥ 0.75, another category was with PP ≥ 0.75 with no sampling requirement, another with PP ≥ 0.5 with the sampling requirement; the least stringent was PP ≥ 0.5 with no sampling requirement. We used the median probability at the root from across the five runs to avoid potential biases from failed runs in the mean and to account for variation across bootstrap distributions (see Supplementary Fig. 4 for distributions of the inferred ratio of duplications, transfers and losses for all gene families across all tips in the species tree; see Supplementary Data 5 for the inferred duplications, transfers and losses ratios for LUCA, the last bacterial common ancestor and the last archaeal common ancestor).
Metabolic pathway analysis
Metabolic pathways for gene families mapped to the LUCA node were inferred using the KEGG 47 website GUI and metabolic completeness for individual modules was estimated with Anvi’o 92 (anvi-estimate-metabolism), with pathwise completeness.
Additional testing
We tested for the effects of model complexity on reconciliation by using posterior mean site frequency LG + C20 + F + G across three independent runs in comparison with 3 LG + F + G independent runs. We also performed a 10% subsampling of the species trees and gene family alignments across two independent runs for two different subsamples, one with and one without the presence of Asgard archaea. We also tested the likelihood of the gene families under a bacterial root (between Terrabacteria and Gracilicutes) using reconciliations of the gene families under a species-tree topology rooted as such.
Fossil calibrations
On the basis of well-established geological events and the fossil record, we modelled 13 uniform densities to constrain the maximum and minimum ages of various nodes in our phylogeny. We constrained the bounds of the uniform densities to be either hard (no tail probability is allowed after the age constraint) or soft (a 2.5% tail probability is allowed after the age constraint) depending on the interpretation of the fossil record ( Supplementary Information ). Nodes that refer to the same duplication event are identified by MCMCtree as cross-braced (that is, one is chosen as the ‘driver’ node, the rest are ‘mirrored’ nodes). In other words, the sampling during the Markov chain Monte Carlo (MCMC) for cross-braced nodes is not independent: the same posterior time density is inferred for matching mirror–driver nodes (see ‘Additional methods’ for details on our cross-bracing approach).
Timetree inference analyses
Timetree inference with the program MCMCtree (PAML v.4.10.7 (ref. 93 )) proceeded under both the GBM and ILN relaxed-clock models. We specified a vague rate prior with the shape parameter equal to 2 and the scale parameter equal to 2.5: Γ(2, 2.5). This gamma distribution is meant to account for the uncertainty on our estimate for the mean evolutionary rate, ~0.81 substitutions per site per time unit, which we calculated by dividing the tree height of our best-scoring ML tree ( Supplementary Information ) into the estimated mean root age of our phylogeny (that is, 4.520 Ga, time unit = 10 9 years; see ‘Fossil calibrations’ in Supplementary Information for justifications on used calibrations). Given that we are estimating very deep divergences, the molecular clock may be seriously violated. Therefore, we applied a very diffuse gamma prior on the rate variation parameter ( σ 2 ), Γ(1, 10), so that it is centred around σ 2 = 0.1. To incorporate our uncertainty regarding the tree shape, we specified a uniform kernel density for the birth–death sampling process by setting the birth and death processes to 1, λ (per-lineage birth rate) = μ (per-lineage death rate) = 1, and the sampling frequency to ρ (sampling fraction) = 0.1. Our main analysis consisted of inferring the timetree for the partitioned dataset under both the GBM and the ILN relaxed-clock models in which nodes that correspond to the same divergences are cross-braced (that is, hereby referred to as cross-bracing A). In addition, we ran 10 additional inference analyses to benchmark the effect that partitioning, cross-bracing and relaxed-clock models can have on species divergence time estimation: (1) GBM + concatenated alignment + cross-bracing A, (2) GBM + concatenated alignment + cross-bracing B (only nodes that correspond to the same divergences for which there are fossil constraints are cross-braced), (3) GBM + concatenated alignment + without cross-bracing, (4) GBM + partitioned alignment + cross-bracing B, (5) GBM + partitioned alignment + without cross-bracing, (6) ILN + concatenated alignment + cross-bracing A, (7) ILN + concatenated alignment + cross-bracing B, (8) ILN + concatenated alignment + without cross-bracing, (9) ILN + partitioned alignment + cross-bracing B, and (10) ILN + partitioned alignment + without cross-bracing. Lastly, we used (1) individual gene alignments, (2) a leave-one-out strategy (rate prior changed for alignments without ATP and Leu , Γ(2, 2.2), and without Tyr , Γ(2, 2.3), but was Γ(2, 2.5) for the rest; see ‘Additional methods’), and (3) a more complex substitution model 94 to assess their impact on timetree inference. Refer to ‘Additional methods’ for details on how we parsed the dataset we used for timetree inference analyses, ran PAML programs CODEML and MCMCtree to approximate the likelihood calculation 95 , and carried out the MCMC diagnostics for the results obtained under each of the previously mentioned scenarios.
We simulated 100 samples of ‘KEGG genomes’ based on the probabilities of each of the (7,467) gene families being present in LUCA using the random.rand function in numpy 96 . The mean number of KEGG gene families was 1,298.25, the 95% HPD (highest posterior density) minimum was 1,255 and the maximum was 1,340. To infer the relationship between the number of KEGG KO gene families encoded by a genome, the number of proteins and the genome size, we used LOESS (locally estimated scatter-plot smoothing) regression to estimate the relationship between the number of KOs and (1) the number of protein-coding genes and (2) the genome size for the 700 prokaryotic genomes used in the LUCA reconstruction. To ensure that our inference of genome size is robust to uncertainty in the number of paralogues that can be expected to have been present in LUCA, we used the presence of probability for each of these KEGG KO gene families rather than the estimated copy number. We used the predict function to estimate the protein-coding genes and genome size of LUCA using these models and the simulated gene contents encoded with 95% confidence intervals.
Additional methods
Cross-bracing approach implemented in mcmctree.
The PAML program MCMCtree was implemented to allow for the analysis of duplicated genes or proteins so that some nodes in the tree corresponding to the same speciation events in different paralogues share the same age. We used the tree topology depicted in Supplementary Fig. 5 to explain how users can label driver or mirror nodes (more on these terms below) so that the program identifies them as sharing the same speciation events. The tree topology shown in Supplementary Fig. 5 can be written in Newick format as:
(((A1,A2),A3),((B1,B2),B3));
In this example, A and B are paralogues and the corresponding tips labelled as A1–A3 and B1–B3 represent different species. Node r represents a duplication event, whereas other nodes are speciation events. If we want to constrain the same speciation events to have the same age (that is, Supplementary Fig. 5 , see labels a and b (that is, A1–A2 ancestor and B1–B2 ancestor, respectively) and labels v and b (that is, A1–A2–A3 ancestor and B1–B2–B3 ancestor, respectively), we use node labels in the format #1, #2, and so on to identify such nodes:
(((A1, A2) #1, A3) #2, ((B1, B2) [#1 B{0.2, 0.4}], B3) #2) 'B(0.9,1.1)';
Node a and node b are assigned the same label (#1) and so they share the same age ( t ): t a = t b . Similarly, node u and node v have the same age: t u = t v . The former nodes are further constrained by a soft-bound calibration based on the fossil record or geological evidence: 0.2 < t a = t b < 0.4. The latter, however, does not have fossil constraints and thus the only restriction imposed is that both t u and t v are equal. Finally, there is another soft-bound calibration on the root age: 0.9 < t r < 1.1.
Among the nodes on the tree with the same label (for example, those nodes labelled with #1 and those with #2 in our example), one is chosen as the driver node, whereas the others are mirror nodes. If calibration information is provided on one of the shared nodes (for example, nodes a and b in Supplementary Fig. 5 ), the same information therefore applies to all shared nodes. If calibration information is provided on multiple shared nodes, that information has to be the same (for example, you could not constrain node a with a different calibration used to constrain node b in Supplementary Fig. 5 ). The time prior (or the prior on all node ages on the tree) is constructed by using a density at the root of the tree, which is specified by the user (for example, 'B(0.9,1.1)' in our example, which has a minimum of 0.9 and a maximum of 1.1). The ages of all non-calibrated nodes are given by the uniform density. This time prior is similar to that used by ref. 29 . The parameters in the birth–death sampling process ( λ , μ , ρ ; specified using the option BDparas in the control file that executes MCMCtree) are ignored. It is noteworthy that more than two nodes can have the same label but one node cannot have two or more labels. In addition, the prior on rates does not distinguish between speciation and duplication events. The implemented cross-bracing approach can only be enabled if option duplication = 1 is included in the control file. By default, this option is set to 0 and users are not required to include it in the control file (that is, the default option is duplication = 0 ).
Timetree inference
Data parsing.
Eight paralogues were initially selected based on previous work showing a likely duplication event before LUCA: the amino- and carboxy-terminal regions from carbamoyl phosphate synthetase, aspartate and ornithine transcarbamoylases, histidine biosynthesis genes A and F , catalytic and non-catalytic subunits from ATP synthase ( ATP ), elongation factor Tu and G ( EF ), signal recognition protein and signal recognition particle receptor ( SRP ), tyrosyl-tRNA and tryptophanyl-tRNA synthetases ( Tyr ), and leucyl- and valyl-tRNA synthetases ( Leu ) 27 . Gene families were identified using BLASTp 97 . Sequences were downloaded from NCBI 98 , aligned with MUSCLE 99 and trimmed with TrimAl 100 (-strict). Individual gene trees were inferred under the LG + C20 + F + G substitution model implemented in IQ-TREE 2 (ref. 86 ). These trees were manually inspected and curated to remove non-homologous sequences, horizontal gene transfers, exceptionally short or long sequences and extremely long branches. Recent paralogues or taxa of inconsistent and/or uncertain placement inferred with RogueNaRok 101 were also removed. Independent verification of an archaeal or bacterial deep split was achieved using minimal ancestor deviation 102 . This filtering process resulted in the five pairs of paralogous gene families 27 ( ATP , EF , SRP , Tyr and Leu ) that we used to estimate the origination time of LUCA. The alignment used for timetree inference consisted of 246 species, with the majority of taxa having at least two copies (for some eukaryotes, they may be represented by plastid, mitochondrial and nuclear sequences).
To assess the impact that partitioning can have on divergence time estimates, we ran our inference analyses with both a concatenated and a partitioned alignment (that is, gene partitioning scheme). We used PAML v.4.10.7 (programs CODEML and MCMCtree) for all divergence time estimation analyses. Given that a fixed tree topology is required for timetree inference with MCMCtree, we inferred the best-scoring ML tree with IQ-TREE 2 under the LG + C20 + F + G4 (ref. 103 ) model following our previous phylogenetic analyses. We then modified the resulting inferred tree topology following consensus views of species-level relationships 34 , 35 , 104 , which we calibrated with the available fossil calibrations (see below). In addition, we ran three sensitivity tests: timetree inference (1) with each gene alignment separately, (2) under a leave-one-out strategy in which each gene alignment was iteratively removed from the concatenated dataset (for example, remove gene ATP but keep genes EF , Leu , SRP and Tyr concatenated in a unique alignment block; apply the same procedure for each gene family), and (3) using the vector of branch lengths, the gradient vector and the Hessian matrix estimated under a complex substitution model (bsinBV method described in ref. 94 ) with the concatenated dataset used for our core analyses. Four of the gene alignments generated for the leave-one-out strategy had gap-only sequences, these were removed when re-inferring the branch lengths under the LG + C20 + F + G4 model (that is, without ATP , 241 species; without EF , 236 species; without Leu , 243 species; without Tyr , 244 species). We used these trees to set the rate prior used for timetree inference for those alignments not including ATP , EF , Leu or Tyr , respectively. The β value (scale parameter) for the rate prior used when analysing alignments without ATP , Leu and Tyr changed minimally but we updated the corresponding rate priors accordingly (see above). When not including SRP , the alignment did not have any sequences removed (that is, 246 species). All alignments were analysed with the same rate prior, Γ(2, 2.5), except for the three previously mentioned alignments.
Approximating the likelihood calculation during timetree inference using PAML programs
Before timetree inference, we ran the CODEML program to infer the branch lengths of the fixed tree topology, the gradient (first derivative of the likelihood function) and the Hessian matrix (second derivative of the likelihood function); the vectors and matrix are required to approximate the likelihood function in the dating program MCMCtree 95 , an approach that substantially reduces computational time 105 . Given that CODEML does not implement the CAT (Bayesian mixture model for across-site heterogeneity) model, we ran our analyses under the closest available substitution model: LG + F + G4 (model = 3). We calculated the aforementioned vectors and matrix for each of the five gene alignments (that is, required for the partitioned alignment), for the concatenated alignment and for the concatenated alignments used for the leave-one-out strategy; the resulting values are written out in an output file called rst2. We appended the rst2 files generated for each of the five individual alignments in the same order the alignment blocks appear in the partitioned alignment file (for example, the first alignment block corresponds to the ATP gene alignment, and thus the first rst2 block will be the one generated when analysing the ATP gene alignment with CODEML). We named this file in_5parts.BV. There is only one rst2 output file for the concatenated alignments, which we renamed in.BV (main concatenated alignment and concatenated alignments under leave-one-out strategy). When analysing each gene alignment separately, we renamed the rst2 files generated for each gene alignment as in.BV.
MCMC diagnostics
All the chains that we ran with MCMCtree for each type of analysis underwent a protocol of MCMC diagnostics consisting of the following steps: (1) flagging and removal of problematic chains; (2) generating convergence plots before and after chain filtering; (3) using the samples collected by those chains that passed the filters (that is, assumed to have converged to the same target distribution) to summarize the results; (4) assessing chain efficiency and convergence by calculating statistics such as R-hat, tail-ESS and bulk-ESS (in-house wrapper function calling Rstan functions, Rstan v.2.21.7; https://mc-stan.org/rstan/ ); and (5) generating the timetrees for each type of analysis with confidence intervals and high-posterior densities to show the uncertainty surrounding the estimated divergence times. Tail-ESS is a diagnostic tool that we used to assess the sampling efficiency in the tails of the posterior distributions of all estimated divergence times, which corresponds to the minimum of the effective sample sizes for quantiles 2.5% and 97.5%. To assess the sampling efficiency in the bulk of the posterior distributions of all estimated divergence, we used bulk-ESS, which uses rank-normalized draws. Note that if tail-ESS and bulk-ESS values are larger than 100, the chains are assumed to have been efficient and reliable parameter estimates (that is, divergence times in our case). R-hat is a convergence diagnostic measure that we used to compare between- and within-chain divergence time estimates to assess chain mixing. If R-hat values are larger than 1.05, between- and within-chain estimates do not agree and thus mixing has been poor. Lastly, we assessed the impact that truncation may have on the estimated divergence times by running MCMCtree when sampling from the prior (that is, the same settings specified above but without using sequence data, which set the prior distribution to be the target distribution during the MCMC). To summarize the samples collected during this analysis, we carried out the same MCMC diagnostics procedure previously mentioned. Supplementary Fig. 6 shows our calibration densities (commonly referred to as user-specified priors, see justifications for used calibrations above) versus the marginal densities (also known as effective priors) that MCMCtree infers when building the joint prior (that is, a prior built without sequence data that considers age constraints specified by the user, the birth–death with sampling process to infer the time densities for the uncalibrated nodes, the rate priors, and so on). We provide all our results for these quality-control checks in our GitHub repository ( https://github.com/sabifo4/LUCA-divtimes ) and in Extended Data Fig. 1 , Supplementary Figs. 7 – 10 and Supplementary Data 6 . Data, figures and tables used and/or generated following a step-by-step tutorial are detailed in the GitHub repository for each inference analysis.
Additional sensitivity analyses
We compared the divergence times we estimated with the concatenated dataset under the calibration strategy cross-bracing A with those inferred (1) for each gene, (2) for gene alignments analysed under a leave-one-out strategy, and (3) for the main concatenated dataset but when using the vector of branch lengths, the gradient vector and the Hessian matrix estimated under a more complex substitution model 94 . The results are summarized in Extended Data Fig. 2 and Supplementary Data 7 and 8 . The same pattern regarding the calibration densities and marginal densities when the tree topology was pruned (that is, see above for details on the leave-one-out strategy) was observed, and thus no additional figures have been generated. As for our main analyses, the results for these additional sensitivity analyses can be found on our GitHub repository ( https://github.com/sabifo4/LUCA-divtimes ).
Reporting summary
Further information on research design is available in the Nature Portfolio Reporting Summary linked to this article.
Data availability
All data required to interpret, verify and extend the research in this article can be found at our figshare repository at https://doi.org/10.6084/m9.figshare.24428659 (ref. 106 ) for the reconciliation and phylogenomic analyses and GitHub at https://github.com/sabifo4/LUCA-divtimes (ref. 107 ) for the molecular clock analyses. Additional data are available at the University of Bristol data repository, data.bris, at https://doi.org/10.5523/bris.405xnm7ei36d2cj65nrirg3ip (ref. 108 ).
Code availability
All code relating to the dating analysis can be found on GitHub at https://github.com/sabifo4/LUCA-divtimes (ref. 107 ), and other custom scripts can be found in our figshare repository at https://doi.org/10.6084/m9.figshare.24428659 (ref. 106 ).
Theobald, D. L. A formal test of the theory of universal common ancestry. Nature 465 , 219–222 (2010).
Article CAS PubMed Google Scholar
Woese, C. R. & Fox, G. E. The concept of cellular evolution. J. Mol. Evol. 10 , 1–6 (1977).
Mirkin, B. G., Fenner, T. I., Galperin, M. Y. & Koonin, E. V. Algorithms for computing parsimonious evolutionary scenarios for genome evolution, the last universal common ancestor and dominance of horizontal gene transfer in the evolution of prokaryotes. BMC Evol. Biol. 3 , 2 (2003).
Article PubMed PubMed Central Google Scholar
Ouzounis, C. A., Kunin, V., Darzentas, N. & Goldovsky, L. A minimal estimate for the gene content of the last universal common ancestor—exobiology from a terrestrial perspective. Res. Microbiol. 157 , 57–68 (2006).
Gogarten, J. P. & Deamer, D. Is LUCA a thermophilic progenote? Nat. Microbiol 1 , 16229 (2016).
Weiss, M. C. et al. The physiology and habitat of the last universal common ancestor. Nat. Microbiol 1 , 16116 (2016).
Crapitto, A. J., Campbell, A., Harris, A. J. & Goldman, A. D. A consensus view of the proteome of the last universal common ancestor. Ecol. Evol. 12 , e8930 (2022).
Kyrpides, N., Overbeek, R. & Ouzounis, C. Universal protein families and the functional content of the last universal common ancestor. J. Mol. Evol. 49 , 413–423 (1999).
Koonin, E. V. Comparative genomics, minimal gene-sets and the last universal common ancestor. Nat. Rev. Microbiol. 1 , 127–136 (2003).
Harris, J. K., Kelley, S. T., Spiegelman, G. B. & Pace, N. R. The genetic core of the universal ancestor. Genome Res. 13 , 407–412 (2003).
Article CAS PubMed PubMed Central Google Scholar
Javaux, E. J. Challenges in evidencing the earliest traces of life. Nature 572 , 451–460 (2019).
Lepot, K. Signatures of early microbial life from the Archean (4 to 2.5 Ga) eon. Earth Sci. Rev. 209 , 103296 (2020).
Article CAS Google Scholar
Betts, H. C. et al. Integrated genomic and fossil evidence illuminates life’s early evolution and eukaryote origin. Nat. Ecol. Evol. 2 , 1556–1562 (2018).
Zhu, Q. et al. Phylogenomics of 10,575 genomes reveals evolutionary proximity between domains Bacteria and Archaea. Nat. Commun. 10 , 5477 (2019).
Moody, E. R. R. et al. An estimate of the deepest branches of the tree of life from ancient vertically evolving genes. eLife 11 , e66695 (2022).
Schwartz, R. M. & Dayhoff, M. O. Origins of prokaryotes, eukaryotes, mitochondria, and chloroplasts. Science 199 , 395–403 (1978).
Shih, P. M. & Matzke, N. J. Primary endosymbiosis events date to the later Proterozoic 994 with cross-calibrated phylogenetic dating of duplicated ATPase proteins. Proc. Natl Acad. Sci. USA 110 , 996 (2013).
Article Google Scholar
Mahendrarajah, T. A. et al. ATP synthase evolution on a cross-braced dated tree of life. Nat. Commun. 14 , 7456 (2023).
Bottke, W. F. & Norman, M. D. The Late Heavy Bombardment. Annu. Rev. Earth Planet. Sci. 45 , 619–647 (2017).
Reimink, J. et al. Quantifying the effect of late bombardment on terrestrial zircons. Earth Planet. Sci. Lett. 604 , 118007 (2023).
Boehnke, P. & Harrison, T. M. Illusory Late Heavy Bombardments. Proc. Natl Acad. Sci. USA 113 , 10802–10806 (2016).
Ryder, G. Mass flux in the ancient Earth–Moon system and benign implications for the origin of life on Earth. J. Geophys. Res. 107 , 6-1–6-13 (2002).
Google Scholar
Hartmann, W. K. History of the terminal cataclysm paradigm: epistemology of a planetary bombardment that never (?) happened. Geosciences 9 , 285 (2019).
Planavsky, N. J. et al. Evidence for oxygenic photosynthesis half a billion years before the great oxidation event. Nat. Geosci. 7 , 283–286 (2014).
Ossa, F. O. et al. Limited oxygen production in the Mesoarchean ocean. Proc. Natl Acad. Sci. USA 116 , 6647–6652 (2019).
Mukasa, S. B., Wilson, A. H. & Young, K. R. Geochronological constraints on the magmatic and tectonic development of the Pongola Supergroup (Central Region), South Africa. Precambrian Res. 224 , 268–286 (2013).
Zhaxybayeva, O., Lapierre, P. & Gogarten, J. P. Ancient gene duplications and the root(s) of the tree of life. Protoplasma 227 , 53–64 (2005).
Article PubMed Google Scholar
Donoghue, P. C. J. & Yang, Z. The evolution of methods for establishing evolutionary timescales. Philos. Trans. R. Soc. Lond. B Biol. Sci. 371 , 3006–3010 (2016).
Thorne, J. L., Kishino, H. & Painter, I. S. Estimating the rate of evolution of the rate of molecular evolution. Mol. Biol. Evol. 15 , 1647–1657 (1998).
Yang, Z. & Rannala, B. Bayesian estimation of species divergence times under a molecular clock using multiple fossil calibrations with soft bounds. Mol. Biol. Evol. 23 , 212–226 (2006).
Rannala, B. & Yang, Z. Inferring speciation times under an episodic molecular clock. Syst. Biol. 56 , 453–466 (2007).
Lemey, P., Rambaut, A., Welch, J. J. & Suchard, M. A. Phylogeography takes a relaxed random walk in continuous space and time. Mol. Biol. Evol. 27 , 1877–1885 (2010).
Craig, J. M., Kumar, S. & Hedges, S. B. The origin of eukaryotes and rise in complexity were synchronous with the rise in oxygen. Front. Bioinform. 3 , 1233281 (2023).
Aouad, M. et al. A divide-and-conquer phylogenomic approach based on character supermatrices resolves early steps in the evolution of the Archaea. BMC Ecol. Evol. 22 , 1 (2022).
Coleman, G. A. et al. A rooted phylogeny resolves early bacterial evolution. Science 372 , eabe0511 (2021).
Guy, L. & Ettema, T. J. G. The archaeal ‘TACK’ superphylum and the origin of eukaryotes. Trends Microbiol. 19 , 580–587 (2011).
Spang, A. et al. Complex Archaea that bridge the gap between prokaryotes and eukaryotes. Nature 521 , 173–179 (2015).
Zaremba-Niedzwiedzka, K. et al. Asgard Archaea illuminate the origin of eukaryotic cellular complexity. Nature 541 , 353–358 (2017).
Eme, L. et al. Inference and reconstruction of the heimdallarchaeial ancestry of eukaryotes. Nature 618 , 992–999 (2023).
Raymann, K., Brochier-Armanet, C. & Gribaldo, S. The two-domain tree of life is linked to a new root for the Archaea. Proc. Natl Acad. Sci. USA 112 , 6670–6675 (2015).
Megrian, D., Taib, N., Jaffe, A. L., Banfield, J. F. & Gribaldo, S. Ancient origin and constrained evolution of the division and cell wall gene cluster in Bacteria. Nat. Microbiol. 7 , 2114–2127 (2022).
Brown, C. T. et al. Unusual biology across a group comprising more than 15% of domain Bacteria. Nature 523 , 208–211 (2015).
Rinke, C. et al. Insights into the phylogeny and coding potential of microbial dark matter. Nature 499 , 431–437 (2013).
Shimodaira, H. An approximately unbiased test of phylogenetic tree selection. Syst. Biol. 51 , 492–508 (2002).
Taib, N. et al. Genome-wide analysis of the Firmicutes illuminates the diderm/monoderm transition. Nat. Ecol. Evol. 4 , 1661–1672 (2020).
Szöllõsi, G. J., Rosikiewicz, W., Boussau, B., Tannier, E. & Daubin, V. Efficient exploration of the space of reconciled gene trees. Syst. Biol. 62 , 901–912 (2013).
Kanehisa, M. & Goto, S. KEGG: kyoto encyclopedia of genes and genomes. Nucleic Acids Res. 28 , 27–30 (2000).
Williams, T. A. et al. Integrative modeling of gene and genome evolution roots the archaeal tree of life. Proc. Natl Acad. Sci. USA 114 , E4602–E4611 (2017).
Dharamshi, J. E. et al. Gene gain facilitated endosymbiotic evolution of Chlamydiae. Nat. Microbiol. 8 , 40–54 (2023).
Doolittle, W. F. Phylogenetic classification and the universal tree. Science 284 , 2124–2128 (1999).
Dagan, T. & Martin, W. The tree of one percent. Genome Biol. 7 , 118 (2006).
Tatusov, R. L. et al. The COG database: an updated version includes eukaryotes. BMC Bioinf. 4 , 41 (2003).
Ragsdale, S. W. & Pierce, E. Acetogenesis and the Wood–Ljungdahl pathway of CO 2 fixation. Biochim. Biophys. Acta 1784 , 1873–1898 (2008).
Schuchmann, K. & Müller, V. Autotrophy at the thermodynamic limit of life: a model for energy conservation in acetogenic bacteria. Nat. Rev. Microbiol. 12 , 809–821 (2014).
Schuchmann, K. & Müller, V. Energetics and application of heterotrophy in acetogenic bacteria. Appl. Environ. Microbiol. 82 , 4056–4069 (2016).
Iwabe, N., Kuma, K., Hasegawa, M., Osawa, S. & Miyata, T. Evolutionary relationship of archaebacteria, eubacteria, and eukaryotes inferred from phylogenetic trees of duplicated genes. Proc. Natl Acad. Sci. USA 86 , 9355–9359 (1989).
Gogarten, J. P. et al. Evolution of the vacuolar H + -ATPase: implications for the origin of eukaryotes. Proc. Natl Acad. Sci. USA 86 , 6661–6665 (1989).
Koonin, E. V. & Makarova, K. S. Origins and evolution of CRISPR–Cas systems. Phil. Trans. R. Soc. Lond. B Biol. Sci. 374 , 20180087 (2019).
Krupovic, M., Dolja, V. V. & Koonin, E. V. The LUCA and its complex virome. Nat. Rev. Microbiol. 18 , 661–670 (2020).
Koonin, E. V., Dolja, V. V. & Krupovic, M. The logic of virus evolution. Cell Host Microbe 30 , 917–929 (2022).
Lever, M. A. Acetogenesis in the energy-starved deep biosphere—a paradox? Front. Microbiol. 2 , 284 (2011).
PubMed Google Scholar
Martin, W. & Russell, M. J. On the origin of biochemistry at an alkaline hydrothermal vent. Phil. Trans. R. Soc. Lond. B Biol. Sci. 362 , 1887–1925 (2007).
Catchpole, R. J. & Forterre, P. The evolution of reverse gyrase suggests a nonhyperthermophilic last universal common ancestor. Mol. Biol. Evol. 36 , 2737–2747 (2019).
Groussin, M., Boussau, B., Charles, S., Blanquart, S. & Gouy, M. The molecular signal for the adaptation to cold temperature during early life on Earth. Biol. Lett. 9 , 20130608 (2013).
Boussau, B., Blanquart, S., Necsulea, A., Lartillot, N. & Gouy, M. Parallel adaptations to high temperatures in the Archaean eon. Nature 456 , 942–945 (2008).
Chandor, A. et al. Dinucleotide spore photoproduct, a minimal substrate of the DNA repair spore photoproduct lyase enzyme from Bacillus subtilis. J. Biol. Chem. 281 , 26922–26931 (2006).
Chandra, T. et al. Spore photoproduct lyase catalyzes specific repair of the 5R but not the 5S spore photoproduct. J. Am. Chem. Soc. 131 , 2420–2421 (2009).
Kasting, J. F. The evolution of the prebiotic atmosphere. Orig. Life 14 , 75–82 (1984).
Kharecha, P. A. A Coupled Atmosphere–Ecosystem Model of the Early Archean Biosphere . PhD thesis, Pennsylvania State Univ. (2005).
Barth, P. et al. Isotopic constraints on lightning as a source of fixed nitrogen in Earth’s early biosphere. Nat. Geosci. 16 , 478–484 (2023).
Tian, F., Kasting, J. F. & Zahnle, K. Revisiting HCN formation in Earth’s early atmosphere. Earth Planet. Sci. Lett. 308 , 417–423 (2011).
Zahnle, K. J. Photochemistry of methane and the formation of hydrocyanic acid (HCN) in the Earth’s early atmosphere. J. Geophys. Res. 91 , 2819–2834 (1986).
Stüeken, E. E., Boocock, T., Szilas, K., Mikhail, S. & Gardiner, N. J. Reconstructing nitrogen sources to Earth’s earliest biosphere at 3.7 Ga. Front. Earth Sci. 9 , 675726 (2021).
Ciccarelli, F. D. et al. Toward automatic reconstruction of a highly resolved tree of life. Science 311 , 1283–1287 (2006).
Yutin, N., Makarova, K. S., Mekhedov, S. L., Wolf, Y. I. & Koonin, E. V. The deep archaeal roots of eukaryotes. Mol. Biol. Evol. 25 , 1619–1630 (2008).
Petitjean, C., Deschamps, P., López-García, P. & Moreira, D. Rooting the domain Archaea by phylogenomic analysis supports the foundation of the new kingdom Proteoarchaeota. Genome Biol. Evol. 7 , 191–204 (2014).
Williams, T. A., Cox, C. J., Foster, P. G., Szöllősi, G. J. & Embley, T. M. Phylogenomics provides robust support for a two-domains tree of life. Nat. Ecol. Evol. 4 , 138–147 (2020).
Rinke, C. et al. A standardized archaeal taxonomy for the Genome Taxonomy Database. Nat. Microbiol. 6 , 946–959 (2021).
Parks, D. H. et al. Selection of representative genomes for 24,706 bacterial and archaeal species clusters provide a complete genome-based taxonomy. Preprint at bioRxiv https://doi.org/10.1101/771964 (2019).
Finn, R. D., Clements, J. & Eddy, S. R. HMMER web server: interactive sequence similarity searching. Nucleic Acids Res. 39 , W29–W37 (2011).
Makarova, K. S., Wolf, Y. I. & Koonin, E. V. Archaeal Clusters of Orthologous Genes (arCOGs): an update and application for analysis of shared features between Thermococcales, Methanococcales, and Methanobacteriales. Life 5 , 818–840 (2015).
Haft, D. H., Selengut, J. D. & White, O. The TIGRFAMs database of protein families. Nucleic Acids Res. 31 , 371–373 (2003).
Katoh, K., Kuma, K.-I., Toh, H. & Miyata, T. MAFFT version 5: improvement in accuracy of multiple sequence alignment. Nucleic Acids Res. 33 , 511–518 (2005).
Katoh, K., Misawa, K., Kuma, K.-I. & Miyata, T. MAFFT: a novel method for rapid multiple sequence alignment based on fast Fourier transform. Nucleic Acids Res. 30 , 3059–3066 (2002).
Criscuolo, A. & Gribaldo, S. BMGE (Block Mapping and Gathering with Entropy): a new software for selection of phylogenetic informative regions from multiple sequence alignments. BMC Evol. Biol. 10 , 210 (2010).
Minh, B. Q. et al. IQ-TREE 2: new models and efficient methods for phylogenetic inference in the genomic era. Mol. Biol. Evol. 37 , 1530–1534 (2020).
Eddy, S. R. Accelerated profile HMM searches. PLoS Comput. Biol. 7 , e1002195 (2011).
Susko, E. & Roger, A. J. On reduced amino acid alphabets for phylogenetic inference. Mol. Biol. Evol. 24 , 2139–2150 (2007).
Cantalapiedra, C. P., Hernández-Plaza, A., Letunic, I., Bork, P. & Huerta-Cepas, J. eggNOG-mapper v2: functional annotation, orthology assignments, and domain prediction at the metagenomic scale. Mol. Biol. Evol. 38 , 5825–5829 (2021).
Buchfink, B., Reuter, K. & Drost, H.-G. Sensitive protein alignments at tree-of-life scale using DIAMOND. Nat. Methods 18 , 366–368 (2021).
Szöllősi, G. J., Davín, A. A., Tannier, E., Daubin, V. & Boussau, B. Genome-scale phylogenetic analysis finds extensive gene transfer among fungi. Phil. Trans. R. Soc. Lond. B Biol. Sci. 370 , 20140335 (2015).
Eren, A. M. et al. Community-led, integrated, reproducible multi-omics with anvi’o. Nat. Microbiol. 6 , 3–6 (2021).
Yang, Z. PAML 4: phylogenetic analysis by maximum likelihood. Mol. Biol. Evol. 24 , 1586–1591 (2007).
Wang, S. & Luo, H. Dating the bacterial tree of life based on ancient symbiosis. Preprint at bioRxiv https://doi.org/10.1101/2023.06.18.545440 (2023).
dos Reis, M. & Yang, Z. Approximate likelihood calculation on a phylogeny for Bayesian estimation of divergence times. Mol. Biol. Evol. 28 , 2161–2172 (2011).
Harris et al. Array programming with NumPy. Nature 585 , 357–362 (2020).
Altschul, S. F., Gish, W., Miller, W., Myers, E. W. & Lipman, D. J. Basic local alignment search tool. J. Mol. Biol. 215 , 403–410 (1990).
Sayers, E. W. et al. Database resources of the National Center for Biotechnology Information. Nucleic Acids Res. 39 , D38–D51 (2011).
Edgar, R. C. MUSCLE: multiple sequence alignment with high accuracy and high throughput. Nucleic Acids Res. 32 , 1792–1797 (2004).
Capella-Gutiérrez, S., Silla-Martínez, J. M. & Gabaldón, T. trimAl: a tool for automated alignment trimming in large-scale phylogenetic analyses. Bioinformatics 25 , 1972–1973 (2009).
Aberer, A. J., Krompaß, D. & Stamatakis, A. RogueNaRok: An efficient and exact algorithm for rogue taxon identification. Exelixis-RRDR-2011–10 (Heidelberg Institute for Theoretical Studies, 2011).
Tria, F. D. K., Landan, G. & Dagan, T. Phylogenetic rooting using minimal ancestor deviation. Nat. Ecol. Evol. 1 , 193 (2017).
Hoang, D. T., Chernomor, O., von Haeseler, A., Minh, B. Q. & Vinh, L. S. UFBoot2: improving the ultrafast bootstrap approximation. Mol. Biol. Evol. 35 , 518–522 (2018).
Burki, F., Roger, A. J., Brown, M. W. & Simpson, A. G. B. The new tree of eukaryotes. Trends Ecol. Evol. 35 , 43–55 (2020).
Battistuzzi, F. U., Billing-Ross, P., Paliwal, A. & Kumar, S. Fast and slow implementations of relaxed-clock methods show similar patterns of accuracy in estimating divergence times. Mol. Biol. Evol. 28 , 2439–2442 (2011).
Moody, E. R. R. The nature of the last universal common ancestor and its impact on the early Earth system. figshare https://doi.org/10.6084/m9.figshare.24428659 (2024).
Álvarez-Carretero, S. The nature of the last universal common ancestor and its impact on the early Earth system—timetree inference analyses. Zenodo https://doi.org/10.5281/zenodo.11260523 (2024).
Moody, E. R. R. et al. The nature of the Last Universal Common Ancestor and its impact on the early Earth system. Nat. Ecol. Evol. https://doi.org/10.5523/bris.405xnm7ei36d2cj65nrirg3ip (2024).
Darzi, Y., Letunic, I., Bork, P. & Yamada, T. iPath3.0: interactive pathways explorer v3. Nucleic Acids Res. 46 , W510–W513 (2018).
Download references
Acknowledgements
Our research is funded by the John Templeton Foundation (62220 to P.C.J.D., N.L., T.M.L., D.P., G.A.S., T.A.W. and Z.Y.; the opinions expressed in this publication are those of the authors and do not necessarily reflect the views of the John Templeton Foundation), Biotechnology and Biological Sciences Research Council (BB/T012773/1 to P.C.J.D. and Z.Y.; BB/T012951/1 to Z.Y.), by the European Research Council under the European Union’s Horizon 2020 research and innovation programme (947317 ASymbEL to A.S.; 714774, GENECLOCKS to G.J.S.), Leverhulme Trust (RF-2022-167 to P.C.J.D.), Gordon and Betty Moore Foundation (GBMF9741 to T.A.W., D.P., P.C.J.D., A.S. and G.J.S.; GBMF9346 to A.S.), Royal Society (University Research Fellowship (URF) to T.A.W.), the Simons Foundation (735929LPI to A.S.) and the University of Bristol (University Research Fellowship (URF) to D.P.).
Author information
Authors and affiliations.
Bristol Palaeobiology Group, School of Earth Sciences, University of Bristol, Bristol, UK
Edmund R. R. Moody, Sandra Álvarez-Carretero, Holly C. Betts, Davide Pisani & Philip C. J. Donoghue
Department of Marine Microbiology and Biogeochemistry, NIOZ, Royal Netherlands Institute for Sea Research, Den Burg, The Netherlands
Tara A. Mahendrarajah, Nina Dombrowski & Anja Spang
Milner Centre for Evolution, Department of Life Sciences, University of Bath, Bath, UK
James W. Clark
Department of Biological Physics, Eötvös University, Budapest, Hungary
Lénárd L. Szánthó
MTA-ELTE ‘Lendulet’ Evolutionary Genomics Research Group, Budapest, Hungary
Lénárd L. Szánthó & Gergely J. Szöllősi
Institute of Evolution, HUN-REN Center for Ecological Research, Budapest, Hungary
Global Systems Institute, University of Exeter, Exeter, UK
Richard A. Boyle, Stuart Daines & Timothy M. Lenton
Department of Earth Sciences, University College London, London, UK
Xi Chen & Graham A. Shields
Department of Genetics, Evolution and Environment, University College London, London, UK
Nick Lane & Ziheng Yang
Model-Based Evolutionary Genomics Unit, Okinawa Institute of Science and Technology Graduate University, Okinawa, Japan
Gergely J. Szöllősi
Department of Evolutionary & Population Biology, Institute for Biodiversity and Ecosystem Dynamics (IBED), University of Amsterdam, Amsterdam, The Netherlands
Bristol Palaeobiology Group, School of Biological Sciences, University of Bristol, Bristol, UK
Davide Pisani & Tom A. Williams
You can also search for this author in PubMed Google Scholar
Contributions
The project was conceived and designed by P.C.J.D., T.M.L., D.P., G.J.S., A.S. and T.A.W. Dating analyses were performed by H.C.B., J.W.C., S.Á.-C., P.J.C.D. and E.R.R.M. T.A.M., N.D. and E.R.R.M. performed single-copy orthologue analysis for species-tree inference. L.L.S., G.J.S., T.A.W. and E.R.R.M. performed reconciliation analysis. E.R.R.M. performed homologous gene family annotation, sequence, alignment, gene tree inference and sensitivity tests. E.R.R.M., A.S. and T.A.W. performed metabolic analysis and interpretation. T.M.L., S.D. and R.A.B. provided biogeochemical interpretation. E.R.R.M., T.M.L., A.S., T.A.W., D.P. and P.J.C.D. drafted the article to which all authors (including X.C., N.L., Z.Y. and G.A.S.) contributed.
Corresponding authors
Correspondence to Edmund R. R. Moody , Davide Pisani , Tom A. Williams , Timothy M. Lenton or Philip C. J. Donoghue .
Ethics declarations
Competing interests.
The authors declare no competing interests.
Peer review
Peer review information.
Nature Ecology & Evolution thanks Aaron Goldman and the other, anonymous, reviewer(s) for their contribution to the peer review of this work. Peer reviewer reports are available.
Additional information
Publisher’s note Springer Nature remains neutral with regard to jurisdictional claims in published maps and institutional affiliations.
Extended data
Extended data fig. 1 comparison of the mean divergence times and confidence intervals estimated for the two duplicates of luca under each timetree inference analysis..
Black dots refer to estimated mean divergence times for analyses without cross-bracing, stars are used to identify those under cross-bracing and triangles for estimated upper and lower confidence intervals. Straight lines are used to link mean divergence time estimates across the various inference analyses we carried out, while dashed lines are used to link the estimated confidence intervals. The node label for the driver node is “248”, while it is “368” for the mirror node, as shown in the title of each graph. Coloured stars and triangles are used to identify which LUCA time estimates were inferred under the same cross-braced analysis for the driver-mirror nodes (that is, equal time and CI estimates). Black dots and triangles are used to identify those inferred when cross-bracing was not enabled (that is, different time and CI estimates). -Abbreviations. “GBM”: Geometric Brownian motion relaxed-clock model; “ILN”: Independent-rate log-normal relaxed-clock model; “conc, cb” dots/triangles: results under cross-bracing A when the concatenated dataset was analysed under GBM (red) and ILN (blue); “conc, fosscb”: results under cross-bracing B when the concatenated dataset was analysed under GBM (orange) and ILN (cyan); “part, cb” dots/triangles: results under cross-bracing A when the partitioned dataset was analysed under GBM (pink) and ILN (purple); “part, fosscb”: results under cross-bracing B when the concatenated dataset was analysed under GBM (light green) and ILN (grey); black dots and triangles: results when cross-bracing was not enabled for both concatenated and partitioned datasets.
Extended Data Fig. 2 Comparison of the posterior time estimates and confidence intervals for the two duplicates of LUCA inferred under the main calibration strategy cross-bracing A with the concatenated dataset and with the datasets for the three additional sensitivity analyses.
Dots refer to estimated mean divergence times and triangles to estimated 2.5% and 97.5% quantiles. Straight lines are used to link the mean divergence times estimated in the same analysis under the two different relaxed-clock models (GBM and ILN). Labels in the x axis are informative about the clock model under which the analysis ran and the type of analysis we carried (see abbreviations below). Coloured dots are used to identify which time estimates were inferred when using the same dataset and strategy under GBM and ILN, while triangles refer to the corresponding upper and lower quantiles for the 95% confidence interval. -Abbreviations. “GBM”: Geometric Brownian motion relaxed-clock model; “ILN”: Independent-rate log-normal relaxed-clock model; “main-conc”: results obtained with the concatenated dataset analysed in our main analyses under cross-bracing A; “ATP/EF/Leu/SRP/Tyr”: results obtained when using each gene alignment separately; “noATP/noEF/noLeu/noSRP/noTyr”: results obtained when using concatenated alignments without the gene alignment mentioned in the label as per the “leave-one-out” strategy; “main-bsinbv”: results obtained with the concatenated dataset analysed in our main analyses when using branch lengths, Hessian, and gradient calculated under a more complex substitution model to infer divergence times.
Extended Data Fig. 3 Maximum Likelihood species tree.
The Maximum Likelihood tree inferred across three independent runs, under the best fitting model (according to BIC: LG + F + G + C60) from a concatenation of 57 orthologous proteins, support values are from 10,000 ultrafast bootstraps. Referred to as topology I in the main text. Tips coloured according to taxonomy: Euryarchaeota (teal), DPANN (purple), Asgardarchaeota (cyan), TACK (blue), Gracilicutes (orange), Terrabacteria (red), DST (brown), CPR (green).
Extended Data Fig. 4 Maximum Likelihood tree for focal reconciliation analysis.
Maximum Likelihood tree (topology II in the main text), where DPANN is constrained to be sister to all other Archaea, and CPR is sister to Chloroflexi. Tips coloured according to taxonomy: Euryarchaeota (teal), DPANN (purple), Asgardarchaeota (cyan), TACK (blue), Gracilicutes (orange), Terrabacteria (red), DST (brown), CPR (green). AU topology test, P = 0.517, this is a one-sided statistical test.
Extended Data Fig. 5 The relationship between the number of KO gene families encoded on a genome and its size.
LOESS regression of the number of KOs per sampled genome against the genome size in megabases. We used the inferred relationship for modern prokaryotes to estimate LUCA’s genome size based on reconstructed KO gene family content, as described in the main text. Shaded area represents the 95% confidence interval.
Extended Data Fig. 6 The relationship between the number of KO gene families encoded on a genome and the total number of protein-coding genes.
LOESS regression of the number of KOs per sampled genome against the number of proteins encoded for per sampled genome. We used the inferred relationship for modern prokaryotes to estimate the total number of protein-coding genes encoded by LUCA based on reconstructed KO gene family content, as described in the main text. Shaded area represents the 95% confidence interval.
Supplementary information
Supplementary information.
Supplementary Notes and Figs. 1–10.
Reporting Summary
Peer review file, supplementary data 1.
This table contains the results of the reconciliations for each gene family. KEGG_ko is the KEGG orthology ID; arc_domain_prop is the proportion of the sampled Archaea; bac_domain_prop is the proportion of the sampled bacteria; gene refers to gene name, description and enzyme code; map refers to the different KEGG maps of which this KEGG gene family is a component; pathway is a text description of the metabolic pathways of which these genes are a component; alignment_length refers to the length of the alignment in amino acids; highest_COG_cat refers to the number of sequences placed in the most frequent COG category; difference_1st_and_2nd is the difference between the most frequent COG category and the second most frequent COG category; categories is the number of different COG categories assigned to this KEGG gene family; COG_freq is the proportion of the sequences placed in the most frequent COG category; COG_cat is the most frequent COG functional category; Archaea is the number of archaeal sequences sampled in the gene family; Bacteria is the number of bacterial sequences sampled in the gene family; alternative_COGs is the number of alternative COG gene families assigned across this KEGG orthologous gene family; COG_perc is the proportion of the most frequent COG ID assigned to this KEGG gene family; COG is the COG ID of the most frequenty COG assigned to this gene family; COG_NAME is the description of the most frequent COG ID assigned to this gene family; COG_TAG is the symbol associated with the most frequent COG gene familiy; sequences is the total number of sequences assigned to this gene family; Arc_prop is the proportion of Archaea that make up this gene family; Bac_prop is the proportion of Bacteria that make up this gene family; constrained_median is the median probability (PP) that this gene was present in LUCA from our reconciliation under the focal constrained tree search across the 5 independent bootstrap distribution reconciliations; ML_median is the median PP of the gene family being present in LUCA with gene tree bootstrap distributions against the ML species-tree topology across the 15 independent bootstrap distribution reconciliations; MEAN_OF_MEDIANS is the mean value across the constrained and ML PP results; RANGE_OF_MEDIANS is the range of the PPs for the constrained and ML topology PPs for LUCA; Probable_and_sampling_threshold_met is our most stringent category of gene families inferred in LUCA with 0.75 + PP and a sampling requirement of 1% met in both Archaea and Bacteria; Possible_and_sampling_threshold_met is a threshold of 0.50 + PP and sampling both domains; probable is simply 0.75 + PP; and possible is 0.50 + PP.
Supplementary Data 2
PP for COGs. This table contains the results for the reconciliations of COG-based gene family clustering against the constrained focal species-tree topology. Columns are named similarly to Supplementary Data 1 but each row is a different COG family. The column Modal_KEGG_ko refers to the most frequent KEGG gene family in which a given COG is found; sequences_in_modal_KEGG refers to the number of sequences in the most frequent KEGG gene family.
Supplementary Data 3
Module completeness. Estimated pathway completeness for KEGG metabolic modules (with a completeness greater than zero in at least one confidence threshold) using Anvi’o’s stepwise pathway completeness 48 . Module_name is the name of the module; module_category is the broader category into which the module falls; module_subcategory is a more specific category; possible_anvio includes the gene families with a median PP ≥ 0.50; probable_anvio related to gene families PP ≥ 0.75; and _ws refers to the sampling requirement being met (presence in at least 1% of the sampled Archaea and Bacteria).
Supplementary Data 4
Marker gene metadata for all markers checked during marker gene curation, including the initial 59 single-copy marker genes used in species-tree inference (see Methods ). Data include marker gene set provenance, marker gene name, marker gene description, presence in different marker gene sets 49 , 50 , 51 , 52 , 53 , 54 , 55 , 56 , 57 , 58 , and presence in Archaea and Bacteria. When available, marker genes are matched with their arCOG, TIGR, and COG ID and their respective occurrence across different taxonomic sets is quantified.
Supplementary Data 5
The ratio of duplications, transfers and losses in relation to the total number of copies for the deep ancestral nodes: the LUCA, archaeal (LACA) and bacterial (LBCA) common ancestors, and the average (mean) and 95th percentile.
Supplementary Data 6
Spreadsheet containing a list of the estimated divergence times for all timetree inferences carried out and the corresponding results of the MCMC diagnostics. Tabs Divtimes_GBM-allnodes and Divtimes_ILN-allnodes represent a list of the estimated divergence times (Ma) for all nodes under the 12 inference analyses we ran under GBM and ILN, respectively. Tabs Divtimes_GBM-highlighted and Divtimes_ILN-highlighted represent a list of the estimated divergence times (Ma) for selected nodes ordered according to their mirrored nodes under the 12 inference analyses we ran under GBM and ILN, respectively. Each of the tabs MCMCdiagn_prior, MCMCdiagn_postGBM and MCMCdiagn_postILN contains the statistical results of the MCMC diagnostics we ran for each inference analysis. Note that, despite the analyses carried out when sampling from the prior could have only been done three times (that is, data are not used, and thus only once under each calibration strategy was enough), we repeated them with each dataset regardless. In other words, results for (1) ‘concatenated + cross-bracing A’ and ‘partitioned + cross-bracing A’; (2) ‘concatenated + without cross-bracing’ and ‘partitioned + without cross-bracing’; and (3) ‘concatenated + cross-bracing B’ and ‘partitioned + cross-bracing B’ would be equivalent, respectively. For tabs 1–4, part represents partitioned dataset; conc, concatenated dataset; cb, cross-bracing A; notcb, without cross-bracing; fosscb, cross-bracing B; mean_t, mean posterior time estimate; 2.5%q, 2.5% quantile of the posterior time density for a given node; and 97.5%q, 97.5% quantile of the posterior time density for a given node. For tabs 5–7, med. num. samples collected per chain represents median of the total amount of samples collected per chain; min. num. samples collected per chain, minimum number of samples collected per chain; max. num. samples collected per chain, minimum number of samples collected per chain; num. samples used to calculate stats, number of samples collected by all chains that passed the filters that were used to calculate the tail-ESS, bulk-ESS and R-hat values. For tail-ESS, we report the median, minimum, and maximum tail-ESS values; all larger than 100 as required for assuming reliable parameter estimates. For bulk-ESS, we report the median, minimum and maximum bulk-ESS values; all larger than 100 as required for assuming reliable parameter estimates. For R-hat, minimum and maximum values reported, all smaller than 1.05 as required to assume good mixing.
Supplementary Data 7
Spreadsheet containing a list of the posterior time estimates for LUCA obtained under the main calibration strategy cross-bracing A with the concatenated dataset and with the datasets for the three additional sensitivity analyses. The first column ‘label’ contains the node number for both the driver and mirror nodes for LUCA (the latter includes the term -dup in the label). Columns mean_t, 2.5%q, and 97.5%q refer to the estimated mean divergence times, and the 2.5%/97.5% quantiles of the posterior time density for the corresponding node. Main-conc, refers to results obtained with the concatenated dataset analysed in our main analyses under cross-bracing A; ATP/EF/Leu/SRP/Tyr, results obtained when using each gene alignment separately; noATP/noEF/noLeu/noSRP/noTyr, results obtained when using concatenated alignments without the gene alignment mentioned in the label as per the leave-one-out strategy; main-bsinbv, results obtained with the concatenated dataset analysed in our main analyses when using branch lengths, Hessian and gradient calculated under a more complex substitution model to infer divergence times.
Supplementary Data 8
Spreadsheet containing a list of the estimated divergence times for all timetree inferences carried out for the sensitivity analyses and the corresponding results for the MCMC diagnostics. Tabs Divtimes_GBM-allnodes and Divtimes_ILN-allnodes represent a list of the estimated divergence times (Ma) for all nodes under the 11 inference analyses we ran under GBM and ILN when testing the impact on divergence times estimation when (1) analysing each gene alignment individually, (2) following a leave-one-out strategy, and (3) using the branch lengths, Hessian and gradient estimated under a more complex model for timetree inference (bsinBV approach). Tabs Divtimes_GBM-highlighted and Divtimes_ILN-highlighted represent a list of the estimated divergence times (Ma) for selected nodes ordered according to their mirrored nodes we ran under GBM and ILN for the sensitivity analyses (we also included the results with the main concatenated dataset for reference). Each of tabs MCMCdiagn_prior, MCMCdiagn_postGBM and MCMCdiagn_postILN contains the statistical results of the MCMC diagnostics we ran for the sensitivity analyses. Note that, despite the analyses carried out when sampling from the prior could have only been done once for each different tree topology (that is, data are not used, only topological changes may affect the resulting marginal densities), we ran them with each dataset regardless as part of our pipeline. For tabs 1–4, main-conc represents results obtained with the concatenated dataset analysed in our main analyses under cross-bracing A; ATP/EF/Leu/SRP/Tyr, results obtained when using each gene alignment separately; noATP/noEF/noLeu/noSRP/noTyr, results obtained when using concatenated alignments without the gene alignment mentioned in the label as per the leave-one-out strategy; main-bsinbv, results obtained with the concatenated dataset analysed in our main analyses when using branch lengths, Hessian and gradient calculated under a more complex substitution model to infer divergence times; mean_t, mean posterior time estimate; 2.5%q, 2.5% quantile of the posterior time density for a given node; and 97.5%q, 97.5% quantile of the posterior time density for a given node. For tabs 5–7, med. num. samples collected per chain represents the median of the total amount of samples collected per chain; min. num. samples collected per chain, minimum number of samples collected per chain; max. num. samples collected per chain, minimum number of samples collected per chain; num. samples used to calculate stats, number of samples collected by all chains that passed the filters that were used to calculate the tail-ESS, bulk-ESS and R-hat values. For tail-ESS, we report the median, minimum and maximum tail-ESS values; all larger than 100 as required for assuming reliable parameter estimates. For bulk-ESS, we report the median, minimum and maximum bulk-ESS values; all larger than 100 as required for assuming reliable parameter estimates. For R-hat, minimum and maximum values are reported, all smaller than 1.05 as required to assume good mixing.
Rights and permissions
Open Access This article is licensed under a Creative Commons Attribution 4.0 International License, which permits use, sharing, adaptation, distribution and reproduction in any medium or format, as long as you give appropriate credit to the original author(s) and the source, provide a link to the Creative Commons licence, and indicate if changes were made. The images or other third party material in this article are included in the article’s Creative Commons licence, unless indicated otherwise in a credit line to the material. If material is not included in the article’s Creative Commons licence and your intended use is not permitted by statutory regulation or exceeds the permitted use, you will need to obtain permission directly from the copyright holder. To view a copy of this licence, visit http://creativecommons.org/licenses/by/4.0/ .
Reprints and permissions
About this article
Cite this article.
Moody, E.R.R., Álvarez-Carretero, S., Mahendrarajah, T.A. et al. The nature of the last universal common ancestor and its impact on the early Earth system. Nat Ecol Evol (2024). https://doi.org/10.1038/s41559-024-02461-1
Download citation
Received : 19 January 2024
Accepted : 04 June 2024
Published : 12 July 2024
DOI : https://doi.org/10.1038/s41559-024-02461-1
Share this article
Anyone you share the following link with will be able to read this content:
Sorry, a shareable link is not currently available for this article.
Provided by the Springer Nature SharedIt content-sharing initiative
Quick links
- Explore articles by subject
- Guide to authors
- Editorial policies
Sign up for the Nature Briefing: Microbiology newsletter — what matters in microbiology research, free to your inbox weekly.

Advertisement
A Visual Timeline of the Trump Rally Shooting
By Leanne Abraham , June Kim , Elena Shao , Julie Walton Shaver , Anjali Singhvi , Christiaan Triebert , Karen Yourish , Lazaro Gamio and Amy Schoenfeld Walker
The Associated Press; Photograph by Doug Mills/The New York Times
Former President Donald J. Trump was whisked off the stage at his rally in Pennsylvania on Saturday after gunshots were fired toward the area where he was speaking. Mr. Trump could be seen bleeding from his right ear, and officials said that the shooting was being investigated as an assassination attempt.
Site of Trump rally the morning after shots were fired
Location of law
enforcement
snipers on roof
Location of gunman’s body
Location of a
wounded rally
Stage where
Trump was speaking
Butler Farm
Show Grounds
Pennsylvania
Location of
gunman’s body
By The New York Times; Aerial image by Doral Chenoweth/USA TODAY NETWORK, via Reuters
The rally took place on the grounds of the Butler Farm Show in western Pennsylvania, about 35 miles north of Pittsburgh. On Saturday afternoon, tens of thousands of rally attendees started trickling in after the doors opened at 1 p.m.
Mr. Trump was set to begin speaking at 5 p.m., but didn’t appear onstage until about an hour later. Here’s how the next 11 minutes unfolded based on footage of the rally.
6:05 p.m. As the song concludes, Mr. Trump approaches the lectern and begins speaking. He spends the first six minutes talking about President Biden and the state of the country before focusing on immigration.

Eric Lee/The New York Times
TMX via Associated Press
Seconds later A gunshot is heard, and Mr. Trump stops midsentence and flinches. He reaches for his right ear, as another two shots are quickly fired, and ducks behind the lectern. One male Secret Service agent is heard yelling, “Get down, get down, get down, get down!”
Photo appears to
capture the path
of a projectile
Blood on Trump’s
hand after he
touches his ear
Photo appears
to capture the
path of a projectile
Photos by Doug Mills/The New York Times
Secret Service agents surround Mr. Trump as a burst of five more shots is fired. Members of the crowd are panicking, screaming and crouching down. More security personnel run onto the stage, including several heavily armed law enforcement agents.
About 42 seconds after shooting began Agents stay crouched over Mr. Trump until an agent can be heard saying, “Shooter down.” The Secret Service confirmed later in a statement that its “personnel neutralized the shooter.”

Doug Mills/The New York Times

A spectator who had been standing just outside the grounds said in an interview with the BBC that a few minutes into Mr. Trump’s speech, he noticed that someone was “bear-crawling up the roof,” clearly armed with a rifle, and that he tried to notify the police. Law enforcement officials later said that the gunman had opened fire from an elevated position outside the rally’s security perimeter.
After the shooting, the gunman’s body was seen on the rooftop of one of the buildings to Mr. Trump’s right. An AR-15-style semiautomatic rifle was recovered at the scene, according to law enforcement officials.
“I was shot with a bullet that pierced the upper part of my right ear,” Mr. Trump said in a post on Truth Social, his social media platform. “I knew immediately that something was wrong in that I heard a whizzing sound, shots, and immediately felt the bullet ripping through the skin.”
Shortly after the shooting, a spokesman for Mr. Trump’s campaign said that the former president was “fine.” Mr. Trump will still attend the Republican National Convention this week, his advisers said in a statement.
One spectator at the rally, Corey Comperatore , a 50-year-old firefighter, was shot and killed. Two others were critically injured.
Scenes from after the shooting

Photographs by Eric Lee and Doug Mills/The New York Times
- Share full article
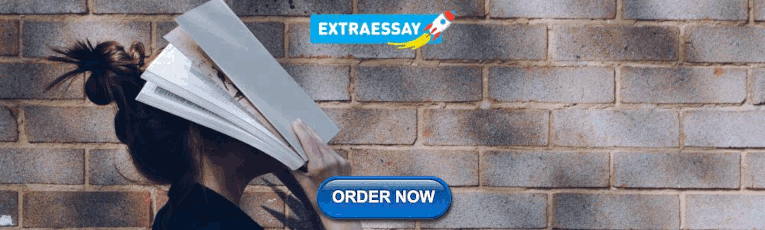
IMAGES
VIDEO
COMMENTS
The introduction section is where you introduce the background and nature of your research question, justify the importance of your research, state your hypotheses, and how your research will contribute to scientific knowledge.. Begin with some opening statements to help situate the reader. Do not immediately dive into the highly technical terminology or the specifics of your research question.
Writing Empirical Papers: Instructions for Beginners Connie Wolfe Muhlenberg College. Note: This guide is intended for students new to writing empirical papers. It is based on conventions used in social psychology; different sub-disciplines have additional or different requirements. The emphasis of the guide is on writing process and content.
Writing an Empirical Paper in APA Style A lab report is a writeup of an experiment and has the same components as a published research study. This handout provides general tips on how to write a psychology lab report. Course standards vary, so check with your instructor if you are not sure what is required. Using APA Style
Literature Review. This section should basically consist of two parts (both of which should be brief). The first section should discuss previous research that is directly relevant to your paper (not every single paper written on the topic). The review need not only be topical, but can include research that employs the same methods you are using ...
Empirical research papers are used to express the results of quantitative (and sometimes qualitative) scientific data on real world phenomena. It is therefore important ... This part of the empirical paper should also list all measures used. If you are giving your participants an IQ test, then you tell the reader which IQ test you ...
Empirical researchers observe, measure, record, and analyze data with the goal of generating knowledge. Empirical research may explore, describe, or explain behaviors or phenomena in humans, animals, or the natural world. It may use any number of quantitative or qualitative methods, ranging from laboratory experiments to surveys to artifact ...
Strategies for Empirical Research in Writing is a particularly accessible approach to both qualitative and quantitative empirical research methods, helping novices appreciate the value of empirical research in writing while easing their fears about the research process. This comprehensive book covers research methods ranging from traditional ...
Empirical research employs rigorous methods to test out theories and hypotheses (expectations) using real data instead of hunches or anecdotal observations. This type of research is easily identifiable as it always consists of the following pieces of information: This Guide will serve to offer a basic understanding on how to approach empirical ...
The research question is the question you want to answer in an empirical research paper. Present your research question clearly and directly, with a minimum of discussion at this point. ... Step 5: Map out your paper. The final part of the introduction is often dedicated to a brief overview of the rest of the paper.
This chapter covers the crucial parts of an empirical research paper common across any type of methodological approach. It discusses a closer examination of these differences and then focuses on the types of empirical research paper common in most social science classes. When it is part of an empirical research paper, the literature review ...
Empirical articles will include charts, graphs, or statistical analysis. Empirical research articles are usually substantial, maybe from 8-30 pages long. There is always a bibliography found at the end of the article. Type of publications that publish empirical studies: Empirical research articles are published in scholarly or academic journals.
Definition: Research Paper is a written document that presents the author's original research, analysis, and interpretation of a specific topic or issue. It is typically based on Empirical Evidence, and may involve qualitative or quantitative research methods, or a combination of both. The purpose of a research paper is to contribute new ...
An annotated Method section and other empirical research paper resources are available here. What is the purpose of the Method section in an empirical research paper? The Method section (also sometimes called Methods, Materials and Methods, or Research Design and Methods) describes the data collection and analysis procedures for a research project.
The Results (also sometimes called Findings) section in an empirical research paper describes what the researcher(s) found when they analyzed their data. Its primary purpose is to use the data collected to answer the research question(s) posed in the introduction, even if the findings challenge the hypothesis.
An empirical research paper is a write-up that is based on verifiable evidence. Ideally, the conclusion of this paper is arrived at after analyzing verifiable evidence. ... Results: Perhaps, this is the most important part of an empirical research paper structure. Here, you include statistics and data analysis. Report your findings consistently ...
Some important tools to have on-hand to make reading an article so much easier:. A dictionary or an encyclopedia will allow you to understand any unclear terms or theories you encounter.; A textbook or an introductory-level book provide some background information written in a way that is easy to understand; Access to a database such as Web of Science which will allow you to quickly access ...
In such cases, a combination of both qualitative and quantitative methods is necessary. Also, papers can also make use of both primary and secondary research methods. ... The observational method is a part of the ethnographic research design, e.g., archival research, survey, etc. ... Empirical Research Cycle: Consists of observation, induction ...
Types and methodologies of empirical research. Empirical research can be conducted and analysed using qualitative or quantitative methods. Quantitative research: Quantitative research methods are used to gather information through numerical data. It is used to quantify opinions, behaviors or other defined variables.
Identifying Empirical Research Articles. Look for the IMRaD layout in the article to help identify empirical research.Sometimes the sections will be labeled differently, but the content will be similar. Introduction: why the article was written, research question or questions, hypothesis, literature review; Methods: the overall research design and implementation, description of sample ...
An empirical research article is an article which reports research based on actual observations or experiments. The research may use quantitative research methods, which generate numerical data and seek to establish causal relationships between two or more variables. (1) Empirical research articles may use qualitative research methods, which ...
Table of contents. Step 1: Restate the problem. Step 2: Sum up the paper. Step 3: Discuss the implications. Research paper conclusion examples. Frequently asked questions about research paper conclusions.
A scientist gathering data for her research. Empirical research is research using empirical evidence.It is also a way of gaining knowledge by means of direct and indirect observation or experience. Empiricism values some research more than other kinds. Empirical evidence (the record of one's direct observations or experiences) can be analyzed quantitatively or qualitatively.
When you write a research paper, many instructors will ask you to find scholarly research articles as a basis for your research. A scholarly research article presents original research and is written and reviewed by experts in a field. A typical scholarly research article has several sections that can include the: Abstract. Introduction. Method.
Another group of papers used resilience mostly in framing the study and interpreting results but did not connect that discussion explicitly to the empirical part of the study. This was common for papers mentioning resilience in the context of other concepts such as vulnerability, adaptive capacity or recovery. Papers without definition were ...
Search 219,793,080 papers from all fields of science. Search. ... Corpus ID: 270501952; An Empirical Study on the Impact of Organizational Culture on Organizational Citizenship Behavior with Affective Commitment as a Mediator @article{2024AnES, title={An Empirical Study on the Impact of Organizational Culture on Organizational Citizenship ...
The paper uses secondary data collected from the KLEMS database of the Reserve Bank of India for the period from 1980-81 to 2019-20 and applies descriptive statistics and econometric techniques to address the research objective. It is found that energy intensity, vertical integration, capital intensity, and rate of growth of total factor ...
Research and statistics. Reports, analysis and official statistics. Policy papers and consultations. Consultations and strategy. Transparency. Data, Freedom of Information releases and corporate ...
Integration of phylogenetics, comparative genomics and palaeobiological approaches suggests that the last universal common ancestor lived about 4.2 billion years ago and was a complex prokaryote ...
Shots rang out as former President Donald J. Trump spoke at a campaign event in Butler, Pa., on Saturday. Maps, photos and video show how the shooting unfolded.