
- Liberty University
- Jerry Falwell Library
- Special Collections
- < Previous
Home > ETD > Honors > 648
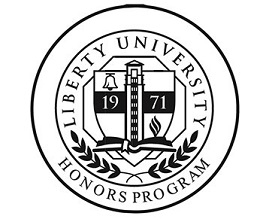
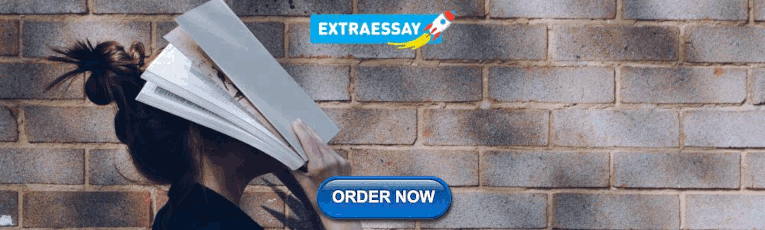
Senior Honors Theses
Skin cancer: causes, prevention, and treatment.
Lauren Queen Follow
Publication Date
Spring 4-10-2017
School of Health Sciences
Health Promotion: Clinical Track
Skin Cancer, Melanoma, Prevention, Treatment, Dermatology
Disciplines
Diseases | Medicine and Health Sciences | Skin and Connective Tissue Diseases
Recommended Citation
Queen, Lauren, "Skin Cancer: Causes, Prevention, and Treatment" (2017). Senior Honors Theses . 648. https://digitalcommons.liberty.edu/honors/648
The purpose of this thesis is to analyze the causes, prevention, and treatment of skin cancer. Skin cancers are defined as either malignant or benign cells that typically arise from excessive exposure to UV radiation. Arguably, skin cancer is a type of cancer that can most easily be prevented; prevention of skin cancer is relatively simple, but often ignored. An important aspect in discussing the epidemiology of skin cancer is understanding the treatments that are available, as well as the prevention methods that can be implemented in every day practice. It is estimated that one in five Americans will develop skin cancer during his or her lifetime, and that one person will die from melanoma every hour of the day. To an epidemiologist and health promotion advocate, these figures are daunting for a disease, especially for a disease that has ample means of prevention. However, even with sufficient prevention methods, a lack of education and promotion of a practice will not lead to favorable results. This thesis will aim to uncover the causes and treatments associated with skin cancer, the disease, distribution, and determinants of the disease, and finally, how the promotion of the practice of prevention of this disease can be furthered.
Since April 12, 2017
Included in
Skin and Connective Tissue Diseases Commons
- Collections
- Faculty Expert Gallery
- Theses and Dissertations
- Conferences and Events
- Open Educational Resources (OER)
- Explore Disciplines
Advanced Search
- Notify me via email or RSS .
Faculty Authors
- Submit Research
- Expert Gallery Login
Student Authors
- Undergraduate Submissions
- Graduate Submissions
- Honors Submissions
- Honors Website
Home | About | FAQ | My Account | Accessibility Statement
Privacy Copyright
Information
- Author Services
Initiatives
You are accessing a machine-readable page. In order to be human-readable, please install an RSS reader.
All articles published by MDPI are made immediately available worldwide under an open access license. No special permission is required to reuse all or part of the article published by MDPI, including figures and tables. For articles published under an open access Creative Common CC BY license, any part of the article may be reused without permission provided that the original article is clearly cited. For more information, please refer to https://www.mdpi.com/openaccess .
Feature papers represent the most advanced research with significant potential for high impact in the field. A Feature Paper should be a substantial original Article that involves several techniques or approaches, provides an outlook for future research directions and describes possible research applications.
Feature papers are submitted upon individual invitation or recommendation by the scientific editors and must receive positive feedback from the reviewers.
Editor’s Choice articles are based on recommendations by the scientific editors of MDPI journals from around the world. Editors select a small number of articles recently published in the journal that they believe will be particularly interesting to readers, or important in the respective research area. The aim is to provide a snapshot of some of the most exciting work published in the various research areas of the journal.
Original Submission Date Received: .
- Active Journals
- Find a Journal
- Proceedings Series
- For Authors
- For Reviewers
- For Editors
- For Librarians
- For Publishers
- For Societies
- For Conference Organizers
- Open Access Policy
- Institutional Open Access Program
- Special Issues Guidelines
- Editorial Process
- Research and Publication Ethics
- Article Processing Charges
- Testimonials
- Preprints.org
- SciProfiles
- Encyclopedia
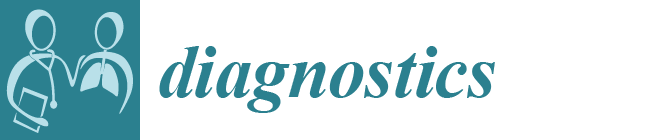
Article Menu
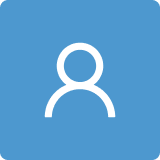
- Subscribe SciFeed
- Recommended Articles
- PubMed/Medline
- Google Scholar
- on Google Scholar
- Table of Contents
Find support for a specific problem in the support section of our website.
Please let us know what you think of our products and services.
Visit our dedicated information section to learn more about MDPI.
JSmol Viewer
Skin cancer detection using deep learning—a review.
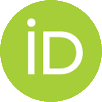
1. Introduction
2. convolutional neural networks (cnns) for image classification, 2.1. commonly used cnn architectures for image classification, 2.2. alexnet, 2.4. resnet, 2.5. densenet, 2.6. mobilenet, 3. deep-learning-based classification of skin cancers, 4. types of skin cancer and commonly used datasets for skin cancers, 4.1. type of skin cancer, 4.1.1. melanoma, 4.1.2. dysplastic nevi, 4.1.3. basal cell carcinoma (bcc), 4.1.4. squamous cell carcinoma (scc), 4.1.5. actinic keratoses (aks), 4.2. datasets, 4.2.1. ham10000, 4.2.2. p h 2, 4.2.3. isic, 4.2.4. isic2016, 4.2.5. isic 2017, 4.2.6. isic2018, 4.2.7. isic 2019, 4.2.8. isic 2020, 4.2.9. atlas of dermoscopy, 4.2.10. dermofit, 4.2.11. bcn20000, 4.2.12. pad-ufes-20, 5. resources required for training proposed dl algorithms, 6. conclusions and discussion, institutional review board statement, informed consent statement, data availability statement, conflicts of interest.
- Skin Cancer. Available online: https://tinyurl.com/ptp97uzv (accessed on 1 May 2023).
- Kittler, H.; Pehamberger, H.; Wolff, K.; Binder, M. Diagnostic accuracy of dermoscopy. Lancet Oncol. 2002 , 3 , 159–165. [ Google Scholar ] [ CrossRef ] [ PubMed ]
- Rosendahl, C.; Tschandl, P.; Cameron, A.; Kittler, H. Diagnostic accuracy of dermatoscopy for melanocytic and nonmelanocytic pigmented lesions. J. Am. Acad. Dermatol. 2011 , 64 , 1068–1073. [ Google Scholar ] [ CrossRef ] [ PubMed ]
- Jalalian, A.; Mashohor, S.; Mahmud, R.; Karasfi, B.; Saripan, M.I.B.; Ramli, A.R.B. Foundation and methodologies in computer-aided diagnosis systems for breast cancer detection. EXCLI J. 2017 , 16 , 113. [ Google Scholar ] [ PubMed ]
- Fan, H.; Xie, F.; Li, Y.; Jiang, Z.; Liu, J. Automatic segmentation of dermoscopy images using saliency combined with Otsu threshold. Comput. Biol. Med. 2017 , 85 , 75–85. [ Google Scholar ] [ CrossRef ]
- Hasan, M.K.; Dahal, L.; Samarakoon, P.N.; Tushar, F.I.; Martí, R. DSNet: Automatic dermoscopic skin lesion segmentation. Comput. Biol. Med. 2020 , 120 , 103738. [ Google Scholar ] [ CrossRef ]
- Korotkov, K.; Garcia, R. Computerized analysis of pigmented skin lesions: A review. Artif. Intell. Med. 2012 , 56 , 69–90. [ Google Scholar ] [ CrossRef ]
- Hasan, M.K.; Elahi, M.T.E.; Alam, M.A.; Jawad, M.T.; Martí, R. DermoExpert: Skin lesion classification using a hybrid convolutional neural network through segmentation, transfer learning, and augmentation. Inform. Med. Unlocked 2022 , 28 , 100819. [ Google Scholar ] [ CrossRef ]
- Mishra, N.K.; Celebi, M.E. An overview of melanoma detection in dermoscopy images using image processing and machine learning. arXiv 2016 , arXiv:1601.07843. [ Google Scholar ]
- Pacheco, A.G.; Krohling, R.A. Recent advances in deep learning applied to skin cancer detection. arXiv 2019 , arXiv:1912.03280. [ Google Scholar ]
- Lucieri, A.; Dengel, A.; Ahmed, S. Deep Learning Based Decision Support for Medicine—A Case Study on Skin Cancer Diagnosis. arXiv 2021 , arXiv:2103.05112. [ Google Scholar ]
- Adegun, A.; Viriri, S. Deep learning techniques for skin lesion analysis and melanoma cancer detection: A survey of state-of-the-art. Artif. Intell. Rev. 2021 , 54 , 811–841. [ Google Scholar ] [ CrossRef ]
- Dildar, M.; Akram, S.; Irfan, M.; Khan, H.U.; Ramzan, M.; Mahmood, A.R.; Alsaiari, S.A.; Saeed, A.H.M.; Alraddadi, M.O.; Mahnashi, M.H. Skin cancer detection: A review using deep learning techniques. Int. J. Environ. Res. Public Health 2021 , 18 , 5479. [ Google Scholar ] [ CrossRef ]
- Gilani, S.Q.; Marques, O. Skin lesion analysis using generative adversarial networks: A review. Multimed. Tools Appl. 2023 , 1–42. [ Google Scholar ] [ CrossRef ]
- Available online: https://www.mathworks.com/discovery/convolutional-neural-network-matlab.html (accessed on 1 May 2023).
- Krizhevsky, A.; Sutskever, I.; Hinton, G.E. Imagenet classification with deep convolutional neural networks. Commun. ACM 2017 , 60 , 84–90. [ Google Scholar ] [ CrossRef ]
- Russakovsky, O.; Deng, J.; Su, H.; Krause, J.; Satheesh, S.; Ma, S.; Huang, Z.; Karpathy, A.; Khosla, A.; Bernstein, M.; et al. Imagenet large scale visual recognition challenge. Int. J. Comput. Vis. 2015 , 115 , 211–252. [ Google Scholar ] [ CrossRef ]
- Simonyan, K.; Zisserman, A. Very deep convolutional networks for large-scale image recognition. arXiv 2014 , arXiv:1409.1556. [ Google Scholar ]
- Deng, J.; Dong, W.; Socher, R.; Li, L.J.; Li, K.; Fei-Fei, L. Imagenet: A large-scale hierarchical image database. In Proceedings of the 2009 IEEE Conference on Computer Vision and Pattern Recognition, Miami, FL, USA, 20–25 June 2009; pp. 248–255. [ Google Scholar ]
- He, K.; Zhang, X.; Ren, S.; Sun, J. Deep residual learning for image recognition. In Proceedings of the IEEE Conference on Computer Vision and Pattern Recognition, Las Vegas, NV, USA, 27–30 June 2016; pp. 770–778. [ Google Scholar ]
- Huang, G.; Liu, Z.; van der Maaten, L.; Weinberger, K.Q. Densely Connected Convolutional Networks. In Proceedings of the IEEE Conference on Computer Vision and Pattern Recognition (CVPR), Honolulu, HI, USA, 21–26 July 2017. [ Google Scholar ]
- Howard, A.G.; Zhu, M.; Chen, B.; Kalenichenko, D.; Wang, W.; Weyand, T.; Andreetto, M.; Adam, H. Mobilenets: Efficient convolutional neural networks for mobile vision applications. arXiv 2017 , arXiv:1704.04861. [ Google Scholar ]
- Inthiyaz, S.; Altahan, B.R.; Ahammad, S.H.; Rajesh, V.; Kalangi, R.R.; Smirani, L.K.; Hossain, M.A.; Rashed, A.N.Z. Skin disease detection using deep learning. Adv. Eng. Softw. 2023 , 175 , 103361. [ Google Scholar ] [ CrossRef ]
- Gajera, H.K.; Nayak, D.R.; Zaveri, M.A. A comprehensive analysis of dermoscopy images for melanoma detection via deep CNN features. Biomed. Signal Process. Control 2023 , 79 , 104186. [ Google Scholar ] [ CrossRef ]
- Szegedy, C.; Liu, W.; Jia, Y.; Sermanet, P.; Reed, S.; Anguelov, D.; Erhan, D.; Vanhoucke, V.; Rabinovich, A. Going deeper with convolutions. In Proceedings of the IEEE Conference on Computer Vision and Pattern Recognition, Boston, MA, USA, 7–12 June 2015; pp. 1–9. [ Google Scholar ]
- Tan, M.; Le, Q. Efficientnet: Rethinking model scaling for convolutional neural networks. In Proceedings of the International Conference on Machine Learning, Long Beach, CA, USA, 9–15 June 2019; pp. 6105–6114. [ Google Scholar ]
- Gutman, D.; Codella, N.C.; Celebi, E.; Helba, B.; Marchetti, M.; Mishra, N.; Halpern, A. Skin lesion analysis toward melanoma detection: A challenge at the international symposium on biomedical imaging (ISBI) 2016, hosted by the international skin imaging collaboration (ISIC). arXiv 2016 , arXiv:1605.01397. [ Google Scholar ]
- Codella, N.C.; Gutman, D.; Celebi, M.E.; Helba, B.; Marchetti, M.A.; Dusza, S.W.; Kalloo, A.; Liopyris, K.; Mishra, N.; Kittler, H.; et al. Skin lesion analysis toward melanoma detection: A challenge at the 2017 international symposium on biomedical imaging (isbi), hosted by the international skin imaging collaboration (isic). In Proceedings of the 2018 IEEE 15th International Symposium on Biomedical Imaging (ISBI 2018), Washington, DC, USA, 4–7 April 2018; pp. 168–172. [ Google Scholar ]
- Mendonça, T.; Celebi, M.; Mendonca, T.; Marques, J. Ph2: A public database for the analysis of dermoscopic images. In Dermoscopy Image Analysis ; CRC Press: Boca Raton, FL, USA, 2015. [ Google Scholar ]
- Tschandl, P.; Rosendahl, C.; Kittler, H. The HAM10000 dataset, a large collection of multi-source dermatoscopic images of common pigmented skin lesions. Sci. Data 2018 , 5 , 1–9. [ Google Scholar ] [ CrossRef ] [ PubMed ]
- Alenezi, F.; Armghan, A.; Polat, K. Wavelet transform based deep residual neural network and ReLU based Extreme Learning Machine for skin lesion classification. Expert Syst. Appl. 2023 , 213 , 119064. [ Google Scholar ] [ CrossRef ]
- Shinde, R.K.; Alam, M.S.; Hossain, M.B.; Md Imtiaz, S.; Kim, J.; Padwal, A.A.; Kim, N. Squeeze-MNet: Precise Skin Cancer Detection Model for Low Computing IoT Devices Using Transfer Learning. Cancers 2022 , 15 , 12. [ Google Scholar ] [ CrossRef ] [ PubMed ]
- Alenezi, F.; Armghan, A.; Polat, K. A multi-stage melanoma recognition framework with deep residual neural network and hyperparameter optimization-based decision support in dermoscopy images. Expert Syst. Appl. 2023 , 215 , 119352. [ Google Scholar ] [ CrossRef ]
- Redmon, J. Darknet: Open Source Neural Networks in C. 2013–2016. Available online: http://pjreddie.com/darknet/ (accessed on 1 May 2023).
- Iandola, F.N.; Han, S.; Moskewicz, M.W.; Ashraf, K.; Dally, W.J.; Keutzer, K. SqueezeNet: AlexNet-level accuracy with 50x fewer parameters and <0.5 MB model size. arXiv 2016 , arXiv:1602.07360. [ Google Scholar ]
- Chollet, F. Xception: Deep learning with depthwise separable convolutions. In Proceedings of the IEEE Conference on Computer Vision and Pattern Recognition, Honolulu, HI, USA, 21–26 July 2017; pp. 1251–1258. [ Google Scholar ]
- Sandler, M.; Howard, A.; Zhu, M.; Zhmoginov, A.; Chen, L.C. Mobilenetv2: Inverted residuals and linear bottlenecks. In Proceedings of the IEEE Conference on Computer Vision and Pattern Recognition, Salt Lake City, UT, USA, 18–22 June 2018; pp. 4510–4520. [ Google Scholar ]
- Abbas, Q.; Gul, A. Detection and Classification of Malignant Melanoma Using Deep Features of NASNet. SN Comput. Sci. 2022 , 4 , 21. [ Google Scholar ] [ CrossRef ]
- Zoph, B.; Vasudevan, V.; Shlens, J.; Le, Q.V. Learning transferable architectures for scalable image recognition. In Proceedings of the IEEE Conference on Computer Vision and Pattern Recognition, Salt Lake City, UT, USA, 18–22 June 2018; pp. 8697–8710. [ Google Scholar ]
- Gouda, W.; Sama, N.U.; Al-Waakid, G.; Humayun, M.; Jhanjhi, N.Z. Detection of skin cancer based on skin lesion images using deep learning. Healthcare 2022 , 10 , 1183. [ Google Scholar ] [ CrossRef ]
- Wang, X.; Yu, K.; Wu, S.; Gu, J.; Liu, Y.; Dong, C.; Qiao, Y.; Change Loy, C. Esrgan: Enhanced super-resolution generative adversarial networks. In Proceedings of the European Conference on Computer Vision (ECCV) Workshops, Munich, Germany, 8–14 September 2018. [ Google Scholar ]
- Szegedy, C.; Ioffe, S.; Vanhoucke, V.; Alemi, A. Inception-v4, inception-resnet and the impact of residual connections on learning. In Proceedings of the AAAI Conference on Artificial Intelligence, San Francisco, CA, USA, 4–9 February 2017; Volume 31. [ Google Scholar ]
- Alwakid, G.; Gouda, W.; Humayun, M.; Sama, N.U. Melanoma Detection Using Deep Learning-Based Classifications. Healthcare 2022 , 10 , 2481. [ Google Scholar ] [ CrossRef ]
- Bassel, A.; Abdulkareem, A.B.; Alyasseri, Z.A.A.; Sani, N.S.; Mohammed, H.J. Automatic malignant and benign skin cancer classification using a hybrid deep learning approach. Diagnostics 2022 , 12 , 2472. [ Google Scholar ] [ CrossRef ]
- Cortes, C.; Vapnik, V. Support-vector networks. Mach. Learn. 1995 , 20 , 273–297. [ Google Scholar ] [ CrossRef ]
- Ho, T.K. Random decision forests. In Proceedings of the 3rd International Conference on Document Analysis and Recognition, Montreal, QC, Canada, 14–16 August 1995; Volume 1, pp. 278–282. [ Google Scholar ]
- Hopfield, J.J. Neural networks and physical systems with emergent collective computational abilities. Proc. Natl. Acad. Sci. USA 1982 , 79 , 2554–2558. [ Google Scholar ] [ CrossRef ]
- Fix, E. Discriminatory Analysis: Nonparametric Discrimination, Consistency Properties ; USAF School of Aviation Medicine: Randolph Field, TX, USA, 1985; Volume 1. [ Google Scholar ]
- Kousis, I.; Perikos, I.; Hatzilygeroudis, I.; Virvou, M. Deep Learning Methods for Accurate Skin Cancer Recognition and Mobile Application. Electronics 2022 , 11 , 1294. [ Google Scholar ] [ CrossRef ]
- Shorfuzzaman, M. An explainable stacked ensemble of deep learning models for improved melanoma skin cancer detection. Multimed. Syst. 2022 , 28 , 1309–1323. [ Google Scholar ] [ CrossRef ]
- Reis, H.C.; Turk, V.; Khoshelham, K.; Kaya, S. InSiNet: A deep convolutional approach to skin cancer detection and segmentation. Med. Biol. Eng. Comput. 2022 , 60 , 643–662. [ Google Scholar ] [ CrossRef ]
- Ronneberger, O.; Fischer, P.; Brox, T. U-net: Convolutional networks for biomedical image segmentation. In Proceedings of the Medical Image Computing and Computer-Assisted Intervention—MICCAI 2015: 18th International Conference, Munich, Germany, 5–9 October 2015; Proceedings, Part III 18. Springer: Berlin/Heidelberg, Germany, 2015; pp. 234–241. [ Google Scholar ]
- Wang, S.H.; Zhang, Y.D. DenseNet-201-based deep neural network with composite learning factor and precomputation for multiple sclerosis classification. ACM Trans. Multimed. Comput. Commun. Appl. (TOMM) 2020 , 16 , 1–19. [ Google Scholar ] [ CrossRef ]
- He, K.; Zhang, X.; Ren, S.; Sun, J. Identity mappings in deep residual networks. In Proceedings of the Computer Vision—ECCV 2016: 14th European Conference, Amsterdam, The Netherlands, 11–14 October 2016; Proceedings, Part IV 14. Springer: Berlin/Heidelberg, Germany, 2016; pp. 630–645. [ Google Scholar ]
- Fraiwan, M.; Faouri, E. On the Automatic Detection and Classification of Skin Cancer Using Deep Transfer Learning. Sensors 2022 , 22 , 4963. [ Google Scholar ] [ CrossRef ]
- Ghosh, P.; Azam, S.; Quadir, R.; Karim, A.; Shamrat, F.J.M.; Bhowmik, S.K.; Jonkman, M.; Hasib, K.M.; Ahmed, K. SkinNet-16: A deep learning approach to identify benign and malignant skin lesions. Front. Oncol. 2022 , 12 , 931141. [ Google Scholar ] [ CrossRef ]
- Abdi, H.; Williams, L.J. Principal component analysis. Wiley Interdiscip. Rev. Comput. Stat. 2010 , 2 , 433–459. [ Google Scholar ] [ CrossRef ]
- Maniraj, S.; Maran, P.S. A hybrid deep learning approach for skin cancer diagnosis using subband fusion of 3D wavelets. J. Supercomput. 2022 , 78 , 12394–12409. [ Google Scholar ] [ CrossRef ]
- Alam, M.J.; Mohammad, M.S.; Hossain, M.A.F.; Showmik, I.A.; Raihan, M.S.; Ahmed, S.; Mahmud, T.I. S2C-DeLeNet: A parameter transfer based segmentation-classification integration for detecting skin cancer lesions from dermoscopic images. Comput. Biol. Med. 2022 , 150 , 106148. [ Google Scholar ] [ CrossRef ]
- Mazoure, B.; Mazoure, A.; Bédard, J.; Makarenkov, V. DUNEScan: A web server for uncertainty estimation in skin cancer detection with deep neural networks. Sci. Rep. 2022 , 12 , 179. [ Google Scholar ] [ CrossRef ] [ PubMed ]
- Malibari, A.A.; Alzahrani, J.S.; Eltahir, M.M.; Malik, V.; Obayya, M.; Al Duhayyim, M.; Neto, A.V.L.; de Albuquerque, V.H.C. Optimal deep neural network-driven computer aided diagnosis model for skin cancer. Comput. Electr. Eng. 2022 , 103 , 108318. [ Google Scholar ] [ CrossRef ]
- Rashid, J.; Ishfaq, M.; Ali, G.; Saeed, M.R.; Hussain, M.; Alkhalifah, T.; Alturise, F.; Samand, N. Skin cancer disease detection using transfer learning technique. Appl. Sci. 2022 , 12 , 5714. [ Google Scholar ] [ CrossRef ]
- Aljohani, K.; Turki, T. Automatic Classification of Melanoma Skin Cancer with Deep Convolutional Neural Networks. Ai 2022 , 3 , 512–525. [ Google Scholar ] [ CrossRef ]
- Bian, X.; Pan, H.; Zhang, K.; Li, P.; Li, J.; Chen, C. Skin lesion image classification method based on extension theory and deep learning. Multimed. Tools Appl. 2022 , 81 , 16389–16409. [ Google Scholar ] [ CrossRef ]
- Demir, A.; Yilmaz, F.; Kose, O. Early detection of skin cancer using deep learning architectures: Resnet-101 and inception-v3. In Proceedings of the 2019 Medical Technologies Congress (TIPTEKNO), Izmir, Turkey, 3–5 October 2019; pp. 1–4. [ Google Scholar ]
- Jain, S.; Singhania, U.; Tripathy, B.; Nasr, E.A.; Aboudaif, M.K.; Kamrani, A.K. Deep learning-based transfer learning for classification of skin cancer. Sensors 2021 , 21 , 8142. [ Google Scholar ] [ CrossRef ]
- Szegedy, C.; Vanhoucke, V.; Ioffe, S.; Shlens, J.; Wojna, Z. Rethinking the inception architecture for computer vision. In Proceedings of the IEEE Conference on Computer Vision and Pattern Recognition, Las Vegas, NV, USA, 27–30 June 2016; pp. 2818–2826. [ Google Scholar ]
- Kausar, N.; Hameed, A.; Sattar, M.; Ashraf, R.; Imran, A.S.; Abidin, M.Z.U.; Ali, A. Multiclass skin cancer classification using ensemble of fine-tuned deep learning models. Appl. Sci. 2021 , 11 , 10593. [ Google Scholar ] [ CrossRef ]
- Bechelli, S.; Delhommelle, J. Machine learning and deep learning algorithms for skin cancer classification from dermoscopic images. Bioengineering 2022 , 9 , 97. [ Google Scholar ] [ CrossRef ]
- Khan, M.A.; Sharif, M.; Akram, T.; Damaševičius, R.; Maskeliūnas, R. Skin lesion segmentation and multiclass classification using deep learning features and improved moth flame optimization. Diagnostics 2021 , 11 , 811. [ Google Scholar ] [ CrossRef ]
- Adegun, A.A.; Viriri, S.; Yousaf, M.H. A probabilistic-based deep learning model for skin lesion segmentation. Appl. Sci. 2021 , 11 , 3025. [ Google Scholar ] [ CrossRef ]
- Lu, X.; Firoozeh Abolhasani Zadeh, Y. Deep learning-based classification for melanoma detection using XceptionNet. J. Healthc. Eng. 2022 , 2022 , 2196096. [ Google Scholar ] [ CrossRef ]
- Qasim Gilani, S.; Syed, T.; Umair, M.; Marques, O. Skin Cancer Classification Using Deep Spiking Neural Network. J. Digit. Imaging 2023 , 1–11. [ Google Scholar ] [ CrossRef ]
- Available online: https://github.com/fangwei123456/spikingjelly (accessed on 8 March 2023).
- Khan, M.A.; Muhammad, K.; Sharif, M.; Akram, T.; Kadry, S. Intelligent fusion-assisted skin lesion localization and classification for smart healthcare. Neural Comput. Appl. 2021 , 1–16. [ Google Scholar ] [ CrossRef ]
- Abdar, M.; Samami, M.; Mahmoodabad, S.D.; Doan, T.; Mazoure, B.; Hashemifesharaki, R.; Liu, L.; Khosravi, A.; Acharya, U.R.; Makarenkov, V.; et al. Uncertainty quantification in skin cancer classification using three-way decision-based Bayesian deep learning. Comput. Biol. Med. 2021 , 135 , 104418. [ Google Scholar ] [ CrossRef ]
- Available online: https://www.kaggle.com/datasets/fanconic/skin-cancer-malignant-vs-benign (accessed on 10 March 2023).
- Available online: https://tinyurl.com/4fetuczx (accessed on 10 March 2023).
- Available online: https://tinyurl.com/5x3ftap6 (accessed on 10 March 2023).
- 6 December 2022 (Last Revised). Available online: https://tinyurl.com/ydsscvkk (accessed on 10 March 2023).
- Available online: https://www.isic-archive.com/#!/topWithHeader/onlyHeaderTop/gallery (accessed on 10 March 2023).
- Mendonça, T.; Ferreira, P.M.; Marques, J.S.; Marcal, A.R.; Rozeira, J. PH 2-A dermoscopic image database for research and benchmarking. In Proceedings of the 2013 35th Annual International Conference of the IEEE Engineering in Medicine and Biology Society (EMBC), Osaka, Japan, 3–7 July 2013; pp. 5437–5440. [ Google Scholar ]
- Codella, N.; Rotemberg, V.; Tschandl, P.; Celebi, M.E.; Dusza, S.; Gutman, D.; Helba, B.; Kalloo, A.; Liopyris, K.; Marchetti, M.; et al. Skin lesion analysis toward melanoma detection 2018: A challenge hosted by the international skin imaging collaboration (isic). arXiv 2019 , arXiv:1902.03368. [ Google Scholar ]
- Combalia, M.; Codella, N.C.; Rotemberg, V.; Helba, B.; Vilaplana, V.; Reiter, O.; Carrera, C.; Barreiro, A.; Halpern, A.C.; Puig, S.; et al. Bcn20000: Dermoscopic lesions in the wild. arXiv 2019 , arXiv:1908.02288. [ Google Scholar ]
- Rotemberg, V.; Kurtansky, N.; Betz-Stablein, B.; Caffery, L.; Chousakos, E.; Codella, N.; Combalia, M.; Dusza, S.; Guitera, P.; Gutman, D.; et al. A patient-centric dataset of images and metadata for identifying melanomas using clinical context. Sci. Data 2021 , 8 , 34. [ Google Scholar ] [ CrossRef ]
- Lio, P.A.; Nghiem, P. Interactive Atlas of Dermoscopy: Giuseppe Argenziano, MD, H. Peter Soyer, MD, Vincenzo De Giorgio, MD, Domenico Piccolo, MD, Paolo Carli, MD, Mario Delfino, MD, Angela Ferrari, MD, Rainer Hofmann-Wellenhof, MD, Daniela Massi, MD, Giampiero Mazzocchetti, MD, Massimiliano Scalvenzi, MD, and Ingrid H. Wolf, MD, Milan, Italy, 2000, Edra Medical Publishing and New Media. 208 pages. 290.00. ISBN 88-86457-30-8. CD-ROM requirements (minimum): Pentium 133 MHz, 32-Mb RAM, 24X CD-ROM drive, 800 × 600 resolution, and 16-bit color graphics capability. Test system: Pentium III 700 MHz processor running Microsoft Windows 98. Macintosh compatible only if running Windows emulation software. J. Am. Acad. Dermatol. 2004 , 50 , 807–808. [ Google Scholar ]
- Ballerini, L.; Fisher, R.B.; Aldridge, B.; Rees, J. A color and texture based hierarchical K-NN approach to the classification of non-melanoma skin lesions. In Color Medical Image Analysis ; Springer: Berlin/Heidelberg, Germany, 2013; pp. 63–86. [ Google Scholar ]
- Pacheco, A.G.; Lima, G.R.; Salomao, A.S.; Krohling, B.; Biral, I.P.; de Angelo, G.G.; Alves, F.C., Jr.; Esgario, J.G.; Simora, A.C.; Castro, P.B.; et al. PAD-UFES-20: A skin lesion dataset composed of patient data and clinical images collected from smartphones. Data Brief 2020 , 32 , 106221. [ Google Scholar ] [ CrossRef ]
Paper | Year | Scope |
---|---|---|
Pacheco and Krohling [ ] | 2019 | Reviewed deep learning models for skin cancer classification |
Lucieri et al. [ ] | 2021 | Reviewed deep-learning-based decision support in skin cancer diagnosis |
Adegun and Viriri [ ] | 2021 | Reviewed deep learning techniques for skin lesion analysis and melanoma cancer detection |
Dildar et al. [ ] | 2021 | Reviewed deep learning algorithms for skin cancer classification |
Gilani and Marques [ ] | 2023 | Reviewed skin lesion classification and segmentation using generative adversarial networks (GANs) |
Paper | Architecture | Year |
---|---|---|
Krizhevsky et al. [ ] | AlexNet | 2012 |
Simonyan and Zisserman [ ] | VGG | 2015 |
He et al. [ ] | ResNet | 2016 |
Huang et al. [ ] | DenseNet | 2017 |
Howard et al. [ ] | MobileNet | 2017 |
Paper | Dataset | Model | Performance |
---|---|---|---|
Inthiyaz et al. [ ] | Xiangya-Derm | CNN | AUC = 0.87 |
Gajera et al. [ ] | ISIC 2016, ISIC 2017, , HAM10000 | AlexNet, VGG-16, VGG-19, | Accuracy = 98.33%, F1 score = 0.96 |
Alenezi et al. [ ] | ISIC 2017, HAM10000 | deep residual network | Accuracy = 96.971%, F1-score = 0.95 |
Shinde et al. [ ] | ISIC | Squeeze-MNet | Accuracy = 99.36% |
Alenezi et al. [ ] | ISIC 2019, ISIC 2020 | ResNet-101 with SVM | Accuracy = 96.15% (ISIC 2019), 97.15% (ISIC 2020) |
Abbas and Gul [ ] | ISIC 2020 | NASNet | Accuracy = 97.7%, F1-score = 0.97 |
Gouda et al. [ ] | ISIC 2018 | CNN | Accuracy = 83.2% |
Alwakid et al. [ ] | HAM10000 | CNN, ResNet-50 | F1-score = 0.859 (CNN), 0.852 (ResNet-50)% |
Bassel et al. [ ] | ISIC | Resnet50, Xception, and VGG16 | Accuracy = 90.9%, F1-score = 0.89 |
Kousis et al. [ ] | ISIC 2019 | Eleven CNN architectures with DensNet169 giving the best results | Accuracy = 92.25%, F1-score = 0.932 |
Shorfuzzaman [ ] | ISIC archive | DenseNet121, Xception, EfficientNet80 | Accuracy = 95.76%, F1-score = 0.957 |
Reis et al. [ ] | HAM10000 (ISIC 2018), ISIC 2019, ISIC 2020 | InSiNet, U-Net | Accuracy = 94.59% (ISIC 2018), 91.89% (ISIC 2019), and 90.54% (ISIC 2020) |
Fraiwan and Faouri [ ] | HAM10000 | thirteen CNN architectures with DensNet201 giving the best result | Accuracy = 82.9%, F1-score = 0.744 |
Ghosh et al. [ ] | HAM10000, ISIC archive | SkinNet-16 | Accuracy = 95.51% (HAM10000), 99.19% (ISIC) |
Maniraj and Maran [ ] | VGG-based hybrid architecture | Accuracy = 93.33% | |
Alam et al. [ ] | HAM10000 | S2C-DeLeNet | Mean Accuracy = 91.03%, Mean Dice = 0.9494 |
Mazoure et al. [ ] | ISIC | Inceptionv313, ResNet5014, 170 MobileNetv23, EfcientNet15, BYOL16, SwAV | Class prediction probability = 1.00 (Mel) |
Malibari et al. [ ] | ISIC 2019 | DNN | Average accuracy = 99.90%, F1-score = 0.990 |
Rashid et al. [ ] | ISIC 2020 | MobileNetV2-based transfer learning | Average accuracy = 98.20% |
Aljohani and Turki [ ] | ISIC 2019 | DenseNet201, MobileNetV2, ResNet50V2, ResNet152V2, Xception, VGG16, VGG19, and GoogleNet | Accuracy = 76.09% |
Bian et al. [ ] | ISBI 2016 | YoDyCK | Accuracy = 96.2% |
Demir et al. [ ] | ISIC archive | ResNet-101, Inception-v3 | F1-score = 84.09% (ResNet-101), 87.42% (Inception-v3) |
Jain et al. [ ] | HAM10000 | Transfer learning-based VGG19, InceptionV3, InceptionResNetV2, ResNet50, Xception, and MobileNet | Accuracy = 90.48% (Xception) |
Bechelli and Delhommelle [ ] | Kaggle dataset, HAM10000 | CNN, pre-trained VGG-16, Xception, ResNet50 | Accuracy = 88% (VGG-16), F1-score = 0.88 (VGG-16) |
Khan et al. [ ] | Segmentation (ISBI 2016, ISBI 2017, ISIC 2018, ), classification (HAM10000) | ResNet101, DenseNet201 | Accuracy = 98.70% (Segmentation, ), Accuracy = 98.70% (Classification) |
Adegun et al. [ ] | ISBI 2017, | fully convolutional neural network | Accuracy = 97% (ISBI 2016) |
Qasim Gilani et al. [ ] | HAM10000 | Spiking VGG-13 | Accuracy = 89.57%, F1-score = 0.9007 |
Lu and Firoozeh Abolhasani Zadeh [ ] | HAM10000 | Xception | Accuracy = 100%, F1-score = 95.55% |
Khan et al. [ ] | ISBI 2016, ISIC 2017, ISBI 2018, ISIC 2019, HAM10000 | A hybrid framework of 20 layered and 17 layered CNN for segmentation, 30 layered CNN for feature extraction | Segmentation Accuracy = 92.70% (ISIC 2018), Classification Accuracy = 87.02% (HAM10000) |
Abdar et al. [ ] | ISIC 2019 [ ] | ResNet152V2, MobileNetV2, DenseNet20 | Best Accuracy = 89% [ ], F1-score = 0.91 [ ] |
Paper | Objective | Summary |
---|---|---|
Inthiyaz et al. [ ] | Used pre-trained model for feature extraction and classification was performed using softmax classifier. | This work was tested on a very small dataset; these results can not be generalized on large datasets. Inthiyaz et al. [ ] achieved an AUC of 0.87, which can still be improved; citeinthiyaz2023skin used a deep architecture ResNet-50 which increases the computational cost. |
Gajera et al. [ ] | Used eight pre-trained CNN models for the classification of dermoscopy images. | The proposed methods were evaluated on , ISIC 2016, and ISIC 2017 with only 200, 900, and 2000 training images. Using deep architectures such as DenseNet-121 on small datasets may result in overfitting. Classification performance on the HAM10000 dataset was low. |
Alenezi et al. [ ] | Used wavelet-transform-based deep residual neural network for the classification of skin cancer images. | Limited generalizability. Weak classification performance on lesion images having different sizes, colors, etc. |
Shinde et al. [ ] | Lightweight model was proposed for the classification of skin cancer images on IOT devices. | The proposed model in this work had lower sensitivity and specificity than other baseline models. Since this model was proposed for the classification task on IOT, it should have fewer training parameters than other baseline methods, such as MobileNetV2. However, the number of parameters and training time was still greater than MobileNetV2. |
Alenezi et al. [ ] | Multi-stage deep model was used for the extraction of features from skin cancer images. | Dataset 1 only contained 1168 images. Deep architectures such as ResNet-101 were used for feature extraction, which may result in overfitting. Features extracted using deep networks were provided to SVM for the classification of skin cancer images; it has limitations in terms of the time required for the parameter selection of the SVM classifier. |
Abbas and Gul [ ] | Proposed architecture for the classification of skin cancers. | Proposed a NASNet for the classification of skin cancer images that extracts generalizable features. |
Gouda et al. [ ] | Pre-trained models were used for the classification of skin cancer images. ESRGAN was used for augmenting the dataset. | The proposed work was tested on a small dataset using 3533 images from ISIC 2018. The best classification accuracy of 0.8576 was obtained using Inception50, which is still low. The accuracy achieved using this method was low compared to dermoscopy. |
Alwakid et al. [ ] | Data augmentation and segmentation of lesion was used to improve the classification performance. | Used ESRGAN for data augmentation. Moreover, performed the segmentation to segment lesions for accurate classification. Proposed CNN-based architecture for the classification. The proposed work achieved an accuracy of 86%, less than the dermoscopy images’ accuracy. |
Bassel et al. [ ] | Pre-trained models were used for extracting features. Stacked CV techniques consisting of five different classifiers were used for the classification of skin cancer images. | The proposed model was trained and tested on a small dataset of 2637 training images and 660 test images. The proposed stacked CV algorithm gave the best classification accuracy of 90.9% on the features extracted using Xception. The model may not perform well on large datasets as it will have limited generalizability because a very small dataset was used for training. |
Kousis et al. [ ] | Evaluated the performance of eleven CNN on the skin cancer classification task, and created a mobile application using the best model. | Among the eleven architectures used in this work, DenseNet 169 gave the best classification accuracy of 92.5%. Deploying DenseNet169 for skin cancer classification is not computationally efficient. |
Shorfuzzaman [ ] | Explainable CNN-based stacked framework was proposed for the classification of skin melanoma images. | The proposed work combined deep models such as DenseNet 121, Xception, and EfficientNetB0 to classify skin cancer images. A total of 3297 images from ISIC 2018 were used for training, and an accuracy of 95.76% was achieved using the proposed method. The proposed method is tested only for melanoma versus non-melanoma problems. The proposed model needs to be tested on large datasets, and combining three deep models will be computationally expensive. |
Reis et al. [ ] | Deep CNN network, InSiNet, was proposed for the classification of skin cancer images. | Very deep models trained on only 1323 images were used for classifying melanoma and non-melanoma images. The proposed model can not be generalized to a large dataset as it is trained on 1323 images only. |
Fraiwan and Faouri [ ] | Evaluated thirteen transfer learning models for the classification of skin cancers. | DenseNet201 gave the best accuracy of 82.9% and an F1-score of 0.744. F1-score is more suited for performance evaluation as HAM10000 is an imbalanced dataset; the F1-score of 0.7424 achieved in this work was quite low. Precision and recall, which are also important metrics in skin diagnosis, were quite low. |
Ghosh et al. [ ] | Proposed SkiNet-16, a CNN for the classification of skin cancers. PCA was used for feature selection. | Used two different datasets for the evaluation of the proposed method; dataset 1 consists of only 3297 images, and dataset 2 consists of 1954 images. The method was tested for melanoma versus non-melanoma cases. Skin cancer images were classified with very high accuracy. |
Maniraj and Maran [ ] | Multi-stage hybrid deep learning modeling employing 3D wavelets were proposed. | The proposed mode was tested on only 200 images and can not be used to aid skin cancer diagnosis. The proposed model’s performance will degrade when trained and tested on large datasets. |
Alam et al. [ ] | Proposed SC-DeLeNet for the segmentation and classification of skin cancer images. | The proposed S2C-DeLeNet1 was implemented in two stages; in the first stage, Efficient-Net B4 was used as the encoder of U-Net for the segmentation, and the encoder–decoder network was used for features extraction in stage 2. S2C-DeLeNet1 tested on the HAM10000 dataset consisting of 10,000 images from seven classes performed well on both tasks. |
Mazoure et al. [ ] | CNN-based webserver was developed for the detection of skin cancers. | Among six deep learning networks trained in this work, ResNet-50 gave the best class prediction probability of 1.00. The web server was developed only for benign versus malignant cases. |
Malibari et al. [ ] | CNN-based optimal method for detecting and classifying skin cancer images. | The proposed mode was trained on ISIC 2019 consisting of 253,331 performed well on all five metrics, accuracy, F1-score, precision, recall, and specificity, and gave an impressive accuracy 99.99%. |
Rashid et al. [ ] | MobileNetV2 based transfer learning framework was proposed for skin cancer classification problem. | Addressed the problem of the class imbalance problem. The proposed model performed well on all four metrics used in this study, accuracy, recall, F1 score, and precision, and achieved an average accuracy of 98.2%. The model was tested on only the binary classification problem. |
Aljohani and Turki [ ] | Evaluated seven different deep learning models on skin cancer classification problem. | The models were evaluated on the dataset comprising 7146 images from two classes. The best accuracy of 76.08% achieved using GoogleNet on the test set was quite low. |
Bian et al. [ ] | YoDyCK: YOLOv3 optimized by dynamic convolution kernel trained on skin cancer images collected from was proposed. WGAN was used for data augmentation. | Addressed the problem of data bias in the skin lesion dataset by training the proposed model on the images collected from Asian countries. |
Demir et al. [ ] | Classified skin cancer images using Inception-v3 and ResNet-101. | Inception-v3 trained on 2437 images from two classes gave the best F1 score of 87.02%. |
Jain et al. [ ] | Used different transfer learning models for feature extraction and classification of skin cancers. | Xception gave the best accuracy, but the computation time was greater than other networks trained in this study. The accuracy was MobileNet was a bit low than Xception, but it required less time for training. |
Bechelli and Delhommelle [ ] | Performance of different machine learning and deep learning algorithms was evaluated on skin cancer datasets. | Obtained better accuracy and F1 score on smaller datasets. Deep learning models trained on the HAM10000 dataset achieved an F1-score of 0.70 and a precision of 0.68, which were low when tested on a smaller dataset. |
Khan et al. [ ] | Proposed CNN-based fully automated method for the classification and segmentation of images. | The classification accuracy of the proposed model trained on the HAM10000 was high, but the proposed model gave the best segmentation performance on , which has only 200 images; the effectiveness of the proposed method should be evaluated by testing it on larger datasets. |
Adegun et al. [ ] | Improved the fully connected convolutional network segmentation using probabilistic model. | The proposed model trained using fewer parameters achieved a good classification accuracy on ISBI data, but it required more time to train. |
Lu and Firoozeh Abolhasani Zadeh [ ] | Improved XceptionNet for the classification of skin cancer images. | The proposed model achieved the 100% accuracy and F1-score of 95.3% on HAM10000 dataset. Precision and sensitivity were also greatly improved as compared to other networks. |
Qasim Gilani et al. [ ] | Used spiking neural network (SNN) for the classification of skin cancer images. | SNN trained using fewer parameters achieved higher accuracy, and F1-score than the deep learning models but the specificity and precision of VGG-13 was higher. SNN used in this work is preferred for hardware implementation because of its power-efficient behavior. |
Khan et al. [ ] | Developed an automated system for collecting and uploading skin lesion images on the cloud and performing classification and segmentation. | Information fusion and improved segmentation methods used in this work improved the performance. However, the use of information fusion increased the feature dimensionality, resulting in increased computational cost. |
Abdar et al. [ ] | A hybrid deep learning model for the classification of skin cancer images. | The proposed work assessed the performance of uncertainty quantification methods, Monte Carlo (MC) dropout, ensemble MC dropout (EMC), and deep ensemble (DE) and selected the best-performing models for skin cancer diagnosis. |
Paper | Resources Required for Training Deep Learning Algorithms Covered in This Paper |
---|---|
Gajera et al. [ ] | Intel Core i7-7700 (8) CPU @ 4.20 GHz and 16 GB RAM with a single NVIDIA GeForce GTX 1050Ti GPU |
Alenezi et al. [ ] | 32 GB RAM and an NVIDIA Quadro P4000 card |
Shinde et al. [ ] | Intel Core i5-7500 3.40 GHz processor, 32 GB of RAM, NVIDIA GeForce GTX 10050Ti graphical processor Raspberry Pi 4 microprocessor with a 64-Gb SD card, spy camera, and NeoPixel ring |
Alenezi et al. [ ] | Intel Xeon processor, 64 GB of RAM, and 8 GB-P4000 GPU. |
Abbas and Gul [ ] | 12 GB GPU and 25 GB of RAM. |
Gouda et al. [ ] | Linux PC with GPU RTX3060 and 8 GB of RAM. |
Alwakid et al. [ ] | Linux PC with RTX3060 and 8 GB of RAM. |
Bassel et al. [ ] | Core Intel4 processor with 12 GB RAM. |
Kousis et al. [ ] | Linux system with a GTX 1060 6 GB graphics card. |
Shorfuzzaman [ ] | NVIDIA Tesla P100 GPU with 16 GB RAM |
Reis et al. [ ] | Intel i5 processor, 6 GB of RAM, and a GTX 940MX NVidia GPU with 2 GB of VRAM |
Fraiwan and Faouri [ ] | HP OMEN 30L desktop GT13 with 64 GB RAM, an NVIDIA GeForce RTX 3080 GPU, an Intel Core i7-10700K CPU @ 3.80 GHz, and a 1TB SSD. |
Alam et al. [ ] | Ryzen 5600 CPU and Nvidia RTX3060Ti GPU (8 GB VRAM). |
Mazoure et al. [ ] | NVIDIA P40 GPU with 4 CPUs. |
Malibari et al. [ ] | i5–8600k, GeForce 1050Ti 4 GB, 16 GB RAM, 250 GB SSD, and 1 TB HDD |
Bian et al. [ ] | i7-8700k CPU and two 1080ti GPUs |
Khan et al. [ ] | 16 GB RAM and 256 GB SSD, 16-GB graphics card |
Lu and Firoozeh Abolhasani Zadeh [ ] | Intel Core™ i7-4720HQ, CPU 1.60 GHz, RAM 16 GB Frequency 1.99 GHz, |
The statements, opinions and data contained in all publications are solely those of the individual author(s) and contributor(s) and not of MDPI and/or the editor(s). MDPI and/or the editor(s) disclaim responsibility for any injury to people or property resulting from any ideas, methods, instructions or products referred to in the content. |
Share and Cite
Naqvi, M.; Gilani, S.Q.; Syed, T.; Marques, O.; Kim, H.-C. Skin Cancer Detection Using Deep Learning—A Review. Diagnostics 2023 , 13 , 1911. https://doi.org/10.3390/diagnostics13111911
Naqvi M, Gilani SQ, Syed T, Marques O, Kim H-C. Skin Cancer Detection Using Deep Learning—A Review. Diagnostics . 2023; 13(11):1911. https://doi.org/10.3390/diagnostics13111911
Naqvi, Maryam, Syed Qasim Gilani, Tehreem Syed, Oge Marques, and Hee-Cheol Kim. 2023. "Skin Cancer Detection Using Deep Learning—A Review" Diagnostics 13, no. 11: 1911. https://doi.org/10.3390/diagnostics13111911
Article Metrics
Article access statistics, further information, mdpi initiatives, follow mdpi.
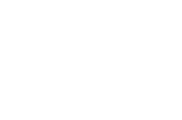
Subscribe to receive issue release notifications and newsletters from MDPI journals

An official website of the United States government
The .gov means it’s official. Federal government websites often end in .gov or .mil. Before sharing sensitive information, make sure you’re on a federal government site.
The site is secure. The https:// ensures that you are connecting to the official website and that any information you provide is encrypted and transmitted securely.
- Publications
- Account settings
Preview improvements coming to the PMC website in October 2024. Learn More or Try it out now .
- Advanced Search
- Journal List
- HHS Author Manuscripts

Skin Cancer—The Importance of Prevention
In 2009, the US Preventive Services Task Force (USPSTF) found insufficient evidence to recommend skin examinations for the early detection of skin cancer in adults. The conclusion followed from a systematic review of the effectiveness and harms of clinical visual skin examinations by physicians or patient self-examinations in terms of morbidity and mortality from skin cancer.
Several years later, after another systematic review, 1 the USPSTF’s conclusion—that there is insufficient evidence to recommend total-body skin examination for the early detection of melanoma, basal cell cancer, or squamous cell cancer in all adults—remains the same. 2
The USPSTF’s determination that evidence is not adequate to support a recommendation for skin cancer screening will likely once again disappoint national organizations such as the American Academy of Dermatology and the Skin Cancer Foundation, which have advocated for screening. 3 , 4 Physicians and patients might also be confused. After all, several organizations have encouraged screening; skin cancer seems easy to detect early because it is visible; skin examinations are neither painful nor invasive; and melanoma thickness at the time of diagnosis predicts mortality.
However, the USPSTF recommendations are based on a rigorous evidence review that balanced the benefits and risks of screening. The potential benefits are apparent but the risks, such as unnecessary procedures and their downstream complications, may not be. Over treatment of skin cancer may be especially problematic for patients with limited life expectancy due to old age or comorbidities. These patients may not live long enough to benefit from more intensive treatments but may be at risk for short-termtreatment-relatedcomplications. 5
The USPSTF review identified no completed randomized clinical trials on the topic. The USPSTF rightly focused on the initially exciting results of an ecologic study, Skin Cancer Research to Provide Evidence for Effectiveness of Screening in Northern Germany (SCREEN), conducted in 1 German state during 2003–2004. 6 The SCREEN study showed a 48% relative reduction in melanoma mortality in the state by 2009 after initiation of a population-based skin cancer awareness campaign, clinician education and training, and screening of nearly 20% of eligible adults aged 20 years and older with a single clinical visual skin examination. Those results prompted Germany to institute a nationwide program of clinical visual skin examinations. Unfortunately, the mortality benefit was not sustained with further follow-up, and several major methodological concerns about SCREEN have been raised. 7 , 8
Skin Cancer Is a Major Problem
The incidence of skin cancer is higher than that of all other cancers combined. Both melanoma and nonmelanoma skin cancer incidence rates continue to increase. The 5.4 million new cases of basal and squamous cell carcinomas in the United States annually 9 and 76 380 new cases of malignant melanoma each year 10 raise concerns for both patients and the health care system. Skin cancer treatments cost the United States more than $8 billion each year, making skin cancer the fifth most costly cancer for Medicare. Furthermore, skin cancer is an under recognized problem for diverse populations, including young women and minorities such as Hispanic individuals and gay men.
If universal screening is not the right approach, what can we do? The answer is that we can do a lot, if we shift our focus from secondary prevention (catching a cancer early enough to treat it) to primary prevention (preventing the cancer from developing in the first place). More than half of cancers are considered preventable through behavioral changes, vaccinations, or medications. 11 The evidence suggests that much of skin cancer could also be prevented.
Preventability of Skin Cancer
The UV radiation from indoor tanning beds is a group 1 carcinogen, in the same category as tobacco or asbestos. 12 Preventing carcinogenic exposures can result in preventing cancer. Indoor tanning is estimated to cause more than 450000 new skin cancers, including more than 10000 melanomas, each year. 13 Despite substantial investment in prevention efforts, including several well- designed campaigns by the Centers for Disease Control and Prevention and foundations focused on skin cancer prevention, efforts to affect the incidence of skin cancer have hit a brick wall. Tanning bed use remains common, with 1 in 5 adolescents and more than 40% of college students using tanning beds. 13
What are we doing wrong? In part, we might not be using the right tools to reach teens and young adults directly, and we might not be reaching the mat the right time. That is where technology may help. Social media and online search engines provide the ability to target health messages directly to those at highest risk. These platforms provide away to introduce messages precisely when teens are, for example, searching for a tanning salon. 14 Technology that targets health messages can get the right message to the right person at the right time. Refining messages that can shift social norms about tanning in general and studying whether these can actually change behaviors remain priorities.
Established and effective strategies for skin cancer prevention are also underused. Comprehensive sun-protection programs that emphasize shade and sun-protective clothing such as Australia’s SunSmart program ( slip on clothing, slop on sunscreen, slap on a hat, seek shade, and slide on sunglasses) should be implemented widely. The Australian program has been linked to a decrease in the incidence of skin cancer in young adults. 15 , 16 Strategies that go beyond education and address practical, environmental, and behavioral barriers to sustainable sun protection have the highest likelihood of success. Shade structures in playgrounds and free sunscreen dispensers in outdoor parks are innovative ideas that should be evaluated. In addition, there are lessons from successful antismoking efforts. Based on the experience with smoking cessation programs, increasing the legal age for indoor tanning to 21 years, restricting indoor tanning advertising directed to youths, and increasing taxation for indoor tanning beyond the 10% excise tax imposed by the Patient Protection and Affordable Care Act may be effective approaches. Physicians and the public should remain alert to the indoor tanning industry’s use of the same techniques used by the tobacco industry: paying scientists to bring doubt to the evidence, making false advertising claims about the health benefits of tanning, and undermining the scientific consensus on the adverse health effects of indoor tanning.
Does Skin Cancer Screening Make Sense for High-Risk Individuals?
As new data emerge, we might find that the benefits of skin cancer screening outweigh the risks for high-risk individuals. Such individuals include solid-organ transplant recipients who have 3 times higher risk of developing malignant melanoma and more than 60 times higher risk of cutaneous squamous cell carcinoma. They also include people with a history of multiple skin cancers whose probability of developing another skin cancer is 50% within 1 year and 70% within 3 years of their last skin cancer diagnosis as well as people with a strong family history of melanoma. As more is learned about the genetic predictors of melanoma and other skin cancers, genotypic approaches may be developed to stratify and identify individuals at high risk who could benefit from screening.
Conclusions
The USPSTF recommendations should not be misinterpreted as minimizing the importance of skin cancer. Instead, the report should motivate us to improve the evidence base for identifying groups of people in whom the benefits of screening might outweigh risks. We need high-quality, long-term randomized clinical trials of the effectiveness of screening on skin cancer prevention. Meanwhile, we should also fully implement skin cancer primary prevention by eliminating indoor tanning exposure, especially among youths, and increasing the use of sun-protection strategies that work.
Conflict of Interest Disclosures: None reported.
59 Skin Cancer Essay Topic Ideas & Examples
🏆 best skin cancer topic ideas & essay examples, 📌 good essay topics on skin cancer, 🔎 simple & easy skin cancer essay titles.
- Life Quality Concerns After a Melanoma Diagnosis Melanoma is one of the most dangerous forms of skin cancer and has been on the rise over the past 30 years.
- Skin Cancer: Description, Causes, and Treatment Skin cancer is one of the most common types of cancer; the three most common types of skin cancer are basal cell carcinoma, squamous cell carcinoma, and melanoma.
- Researching of Cause and Effects of Melanoma This essay reviews the causes of melanoma, including the genetic aberrations involved, and discusses some of the effects of this cancer.
- Does the Sun Radiation Cause Skin Cancer? Moreover, from the article written by American Cancer Society, it is evident that Ultraviolet A and Ultraviolet B from the sun lead to skin cancer.
- Malignant Melanoma of the Skin It is better to quit smoking and choose a healthy diet with the help of which he could improve his immune system.
- Malignant Melanoma of the Skin Diagnostics and Screening Are the examinations of your body organized by your wife systematic or occasional? If systematic, what are the reasons for them?
- Indoor Tanning as a Cause of Melanoma Sarah Longwell’s claim that there is no scientific evidence to confirm that indoor tanning is one of the leading causes of melanoma is invalid.
- Malignant Melanoma: Diagnosis and Treatment But when the above process is taking place, the pro-inflammatory signals engaged in skin healing and repair produce the twin effect of not only arousing the cells of the immune system but also enhancing “…the […]
- Melanoma: Risk Factors and Treatment One of the most problematic is melanoma it is a cancer of the skin. Melanoma is a preventable disease but ignorance of the problem is the reason why this medical condition has claimed the lives […]
- Skin Cancer: Comparison of Samples The aim of this experiment is to examine and thereafter represent low and high power illustrations of a normal skin specimen and of skin specimens that have been affected by various forms types of skin […]
- Skin Cancer and Sunlight: Case Control, Cohort, and Clinical Trial Design The main component in sunlight that is said to be responsible for the development of skin cancer is the Ultraviolet emission.
- Skin Cancer Awareness Overview Other causes of skin cancer include; family history of skin cancer, personal history of the disease, over exposure to the sun, history of sunburn early in life, large moles, freckles and light skin complexity. The […]
- Malignant Melanoma: Pathology and Epidemiology Melanoma is the most rapidly growing type of cancer in the world, as well as the fifth leading cancer in men and the seventh in women in America.
- Skin Cancer: Diagnosis and Treatment In order to prevent the incidence of skin cancer, the patients are recommended to undergo regular cancer screenings. Thus, following the suggested recommendations is expected to reduce the incidence of skin cancer among patients.
- Skin Cancer in Australia and Health Campaign According to the International Agency for Research on Cancer, the incidence of skin cancer in Australia is the highest in the world.
- Skin Cancer: Types and Cells of Origin Skin cancer is very often considered a disease connected with the cell cycle, although it is not actually the case, as cancer cells can easily grow and divide.
- The Problem of Skin Cancer in Australia Generally the issue of skin cancer in Australia is widely known to people despite the fact that the cases have not been mitigated appropriately.
- Aggressive Malignant Melanoma Skin Cancer
- Dangers, Symptoms and Treatment of Skin Cancer
- Methods of Battle With Skin Cancer
- Description of Breast Cancer and Skin Cancer
- Benefits of Green Tea for Skin: Acne, Skin Cancer, and Others
- Natural Products for Treatment of Skin Cancer
- The Connection Between Cutaneous Papillomaviruses and Non-melanoma Skin Cancer
- Biomarkers of Response for Checkpoint Inhibitor Therapy in Skin Cancer
- Dangers, Symptoms, and Treatment of Skin Cancer, a Malignant Disease
- Deadly Skin Cancer Form Malignant Melanoma
- The Efficacy and Safety of Sunscreen Use for the Prevention of Skin Cancer
- Human Papillomaviruses and Polyomaviruses in Skin Cancer
- Insights Into Biomarkers, Cytokines, and Chemokines in Skin Cancer
- Fluorescence-Guided Pdt for Optimization of the Outcome of Skin Cancer Treatment
- Direct Sunlight vs. Tanning Beds in the Development of Skin Cancer Malignant Melanoma
- The Connection Between Genetic Damage and Skin Cancer
- Genetic Mutations That Cause Skin Cancer
- Genetic Risk Factors and Offsetting Behavior: The Case of Skin Cancer
- Skin Cancer Awareness and Prevention
- Indoor Tanning and the Perils of Skin Cancer
- Melanoma: The Most Dangerous Forms of All Skin Cancer
- Muffin Technique Micrographic Surgery for Non-melanoma Skin Cancer
- Non-melanoma Skin Cancer Measurement
- The Ins and Outs of Chemokine-Mediated Immune Cell Trafficking in Skin Cancer
- The Statistics and Prevalence of Skin Cancer World Wide
- Adapting a Skin Cancer Prevention Intervention for Multiethnic Adolescents
- Tumor-Associated Macrophages: Therapeutic Targets for Skin Cancer
- Skin Cancer Prevalence and Geographic Location
- How Caffeine, Exercise Help Fight Skin Cancer
- Skin Cancer: Types, Symptoms, Risk Factors & Treatment
- The Global Health Problem of Skin Cancer
- An Effective Skin Cancer Prevention Strategy
- Risk of Skin Cancer in Tanning Beds
- Detecting the Symptoms of Skin Cancer
- Stratospheric Ozone Depletion and Its Effect on Skin Cancer Incidence
- Skin Cancer: The Potential Hazards of Too Much Sun
- Most Effective Way to Cure Skin Cancer
- Skin Cancer as a Major Public Health Problem
- Ultraviolet Light Is the Main Cause of Skin Cancer
- Skin Cancer: The Dangers of Wanting a Dark Tan
- Chronic Pain Research Ideas
- Health Insurance Research Topics
- Leukemia Topics
- Medical Marijuana Topics
- Palliative Care Research Topics
- Pathogenesis Research Ideas
- Pharmacy Research Ideas
- Healthcare Reform Essay Ideas
- Chicago (A-D)
- Chicago (N-B)
IvyPanda. (2023, September 27). 59 Skin Cancer Essay Topic Ideas & Examples. https://ivypanda.com/essays/topic/skin-cancer-essay-topics/
"59 Skin Cancer Essay Topic Ideas & Examples." IvyPanda , 27 Sept. 2023, ivypanda.com/essays/topic/skin-cancer-essay-topics/.
IvyPanda . (2023) '59 Skin Cancer Essay Topic Ideas & Examples'. 27 September.
IvyPanda . 2023. "59 Skin Cancer Essay Topic Ideas & Examples." September 27, 2023. https://ivypanda.com/essays/topic/skin-cancer-essay-topics/.
1. IvyPanda . "59 Skin Cancer Essay Topic Ideas & Examples." September 27, 2023. https://ivypanda.com/essays/topic/skin-cancer-essay-topics/.
Bibliography
IvyPanda . "59 Skin Cancer Essay Topic Ideas & Examples." September 27, 2023. https://ivypanda.com/essays/topic/skin-cancer-essay-topics/.
DigitalCommons@UNMC
Home > Eppley Institute > Theses & Dissertations
Theses & Dissertations: Cancer Research
Theses/dissertations from 2024 2024.
Novel Spirocyclic Dimer (SpiD3) Displays Potent Preclinical Effects in Hematological Malignancies , Alexandria Eiken
Dying Right: Supporting Anti-Cancer Therapy Through Immunogenic Cell Death , Elizabeth Schmitz
Therapeutic Effects of BET Protein Inhibition in B-cell Malignancies and Beyond , Audrey L. Smith
Identifying the Molecular Determinants of Lung Metastatic Adaptation in Prostate Cancer , Grace M. Waldron
Identification of Mitotic Phosphatases and Cyclin K as Novel Molecular Targets in Pancreatic Cancer , Yi Xiao
Theses/Dissertations from 2023 2023
Development of Combination Therapy Strategies to Treat Cancer Using Dihydroorotate Dehydrogenase Inhibitors , Nicholas Mullen
Overcoming Resistance Mechanisms to CDK4/6 Inhibitor Treatment Using CDK6-Selective PROTAC , Sarah Truong
Theses/Dissertations from 2022 2022
Omics Analysis in Cancer and Development , Emalie J. Clement
Investigating the Role of Splenic Macrophages in Pancreatic Cancer , Daisy V. Gonzalez
Polymeric Chloroquine in Metastatic Pancreatic Cancer Therapy , Rubayat Islam Khan
Evaluating Targets and Therapeutics for the Treatment of Pancreatic Cancer , Shelby M. Knoche
Characterization of 1,1-Diarylethylene FOXM1 Inhibitors Against High-Grade Serous Ovarian Carcinoma Cells , Cassie Liu
Novel Mechanisms of Protein Kinase C α Regulation and Function , Xinyue Li
SOX2 Dosage Governs Tumor Cell Identity and Proliferation , Ethan P. Metz
Post-Transcriptional Control of the Epithelial-to-Mesenchymal Transition (EMT) in Ras-Driven Colorectal Cancers , Chaitra Rao
Use of Machine Learning Algorithms and Highly Multiplexed Immunohistochemistry to Perform In-Depth Characterization of Primary Pancreatic Tumors and Metastatic Sites , Krysten Vance
Characterization of Metastatic Cutaneous Squamous Cell Carcinoma in the Immunosuppressed Patient , Megan E. Wackel
Visceral adipose tissue remodeling in pancreatic ductal adenocarcinoma cachexia: the role of activin A signaling , Pauline Xu
Phos-Tag-Based Screens Identify Novel Therapeutic Targets in Ovarian Cancer and Pancreatic Cancer , Renya Zeng
Theses/Dissertations from 2021 2021
Functional Characterization of Cancer-Associated DNA Polymerase ε Variants , Stephanie R. Barbari
Pancreatic Cancer: Novel Therapy, Research Tools, and Educational Outreach , Ayrianne J. Crawford
Apixaban to Prevent Thrombosis in Adult Patients Treated With Asparaginase , Krishna Gundabolu
Molecular Investigation into the Biologic and Prognostic Elements of Peripheral T-cell Lymphoma with Regulators of Tumor Microenvironment Signaling Explored in Model Systems , Tyler Herek
Utilizing Proteolysis-Targeting Chimeras to Target the Transcriptional Cyclin-Dependent Kinases 9 and 12 , Hannah King
Insights into Cutaneous Squamous Cell Carcinoma Pathogenesis and Metastasis Using a Bedside-to-Bench Approach , Marissa Lobl
Development of a MUC16-Targeted Near-Infrared Antibody Probe for Fluorescence-Guided Surgery of Pancreatic Cancer , Madeline T. Olson
FGFR4 glycosylation and processing in cholangiocarcinoma promote cancer signaling , Andrew J. Phillips
Theses/Dissertations from 2020 2020
Cooperativity of CCNE1 and FOXM1 in High-Grade Serous Ovarian Cancer , Lucy Elge
Characterizing the critical role of metabolic and redox homeostasis in colorectal cancer , Danielle Frodyma
Genomic and Transcriptomic Alterations in Metabolic Regulators and Implications for Anti-tumoral Immune Response , Ryan J. King
Dimers of Isatin Derived Spirocyclic NF-κB Inhibitor Exhibit Potent Anticancer Activity by Inducing UPR Mediated Apoptosis , Smit Kour
From Development to Therapy: A Panoramic Approach to Further Our Understanding of Cancer , Brittany Poelaert
The Cellular Origin and Molecular Drivers of Claudin-Low Mammary Cancer , Patrick D. Raedler
Mitochondrial Metabolism as a Therapeutic Target for Pancreatic Cancer , Simon Shin
Development of Fluorescent Hyaluronic Acid Nanoparticles for Intraoperative Tumor Detection , Nicholas E. Wojtynek
Theses/Dissertations from 2019 2019
The role of E3 ubiquitin ligase FBXO9 in normal and malignant hematopoiesis , R. Willow Hynes-Smith
BRCA1 & CTDP1 BRCT Domainomics in the DNA Damage Response , Kimiko L. Krieger
Targeted Inhibition of Histone Deacetyltransferases for Pancreatic Cancer Therapy , Richard Laschanzky
Human Leukocyte Antigen (HLA) Class I Molecule Components and Amyloid Precursor-Like Protein 2 (APLP2): Roles in Pancreatic Cancer Cell Migration , Bailee Sliker
Theses/Dissertations from 2018 2018
FOXM1 Expression and Contribution to Genomic Instability and Chemoresistance in High-Grade Serous Ovarian Cancer , Carter J. Barger
Overcoming TCF4-Driven BCR Signaling in Diffuse Large B-Cell Lymphoma , Keenan Hartert
Functional Role of Protein Kinase C Alpha in Endometrial Carcinogenesis , Alice Hsu
Functional Signature Ontology-Based Identification and Validation of Novel Therapeutic Targets and Natural Products for the Treatment of Cancer , Beth Neilsen
Elucidating the Roles of Lunatic Fringe in Pancreatic Ductal Adenocarcinoma , Prathamesh Patil
Theses/Dissertations from 2017 2017
Metabolic Reprogramming of Pancreatic Ductal Adenocarcinoma Cells in Response to Chronic Low pH Stress , Jaime Abrego
Understanding the Relationship between TGF-Beta and IGF-1R Signaling in Colorectal Cancer , Katie L. Bailey
The Role of EHD2 in Triple-Negative Breast Cancer Tumorigenesis and Progression , Timothy A. Bielecki
Perturbing anti-apoptotic proteins to develop novel cancer therapies , Jacob Contreras
Role of Ezrin in Colorectal Cancer Cell Survival Regulation , Premila Leiphrakpam
Evaluation of Aminopyrazole Analogs as Cyclin-Dependent Kinase Inhibitors for Colorectal Cancer Therapy , Caroline Robb
Identifying the Role of Janus Kinase 1 in Mammary Gland Development and Breast Cancer , Barbara Swenson
DNMT3A Haploinsufficiency Provokes Hematologic Malignancy of B-Lymphoid, T-Lymphoid, and Myeloid Lineage in Mice , Garland Michael Upchurch
Theses/Dissertations from 2016 2016
EHD1 As a Positive Regulator of Macrophage Colony-Stimulating Factor-1 Receptor , Luke R. Cypher
Inflammation- and Cancer-Associated Neurolymphatic Remodeling and Cachexia in Pancreatic Ductal Adenocarcinoma , Darci M. Fink
Role of CBL-family Ubiquitin Ligases as Critical Negative Regulators of T Cell Activation and Functions , Benjamin Goetz
Exploration into the Functional Impact of MUC1 on the Formation and Regulation of Transcriptional Complexes Containing AP-1 and p53 , Ryan L. Hanson
DNA Polymerase Zeta-Dependent Mutagenesis: Molecular Specificity, Extent of Error-Prone Synthesis, and the Role of dNTP Pools , Olga V. Kochenova
Defining the Role of Phosphorylation and Dephosphorylation in the Regulation of Gap Junction Proteins , Hanjun Li
Molecular Mechanisms Regulating MYC and PGC1β Expression in Colon Cancer , Jamie L. McCall
Pancreatic Cancer Invasion of the Lymphatic Vasculature and Contributions of the Tumor Microenvironment: Roles for E-selectin and CXCR4 , Maria M. Steele
Altered Levels of SOX2, and Its Associated Protein Musashi2, Disrupt Critical Cell Functions in Cancer and Embryonic Stem Cells , Erin L. Wuebben
Theses/Dissertations from 2015 2015
Characterization and target identification of non-toxic IKKβ inhibitors for anticancer therapy , Elizabeth Blowers
Effectors of Ras and KSR1 dependent colon tumorigenesis , Binita Das
Characterization of cancer-associated DNA polymerase delta variants , Tony M. Mertz
A Role for EHD Family Endocytic Regulators in Endothelial Biology , Alexandra E. J. Moffitt
Biochemical pathways regulating mammary epithelial cell homeostasis and differentiation , Chandrani Mukhopadhyay
EPACs: epigenetic regulators that affect cell survival in cancer. , Catherine Murari
Role of the C-terminus of the Catalytic Subunit of Translesion Synthesis Polymerase ζ (Zeta) in UV-induced Mutagensis , Hollie M. Siebler
LGR5 Activates TGFbeta Signaling and Suppresses Metastasis in Colon Cancer , Xiaolin Zhou
LGR5 Activates TGFβ Signaling and Suppresses Metastasis in Colon Cancer , Xiaolin Zhou
Theses/Dissertations from 2014 2014
Genetic dissection of the role of CBL-family ubiquitin ligases and their associated adapters in epidermal growth factor receptor endocytosis , Gulzar Ahmad
Strategies for the identification of chemical probes to study signaling pathways , Jamie Leigh Arnst
Defining the mechanism of signaling through the C-terminus of MUC1 , Roger B. Brown
Targeting telomerase in human pancreatic cancer cells , Katrina Burchett
The identification of KSR1-like molecules in ras-addicted colorectal cancer cells , Drew Gehring
Mechanisms of regulation of AID APOBEC deaminases activity and protection of the genome from promiscuous deamination , Artem Georgievich Lada
Characterization of the DNA-biding properties of human telomeric proteins , Amanda Lakamp-Hawley
Studies on MUC1, p120-catenin, Kaiso: coordinate role of mucins, cell adhesion molecules and cell cycle players in pancreatic cancer , Xiang Liu
Epac interaction with the TGFbeta PKA pathway to regulate cell survival in colon cancer , Meghan Lynn Mendick
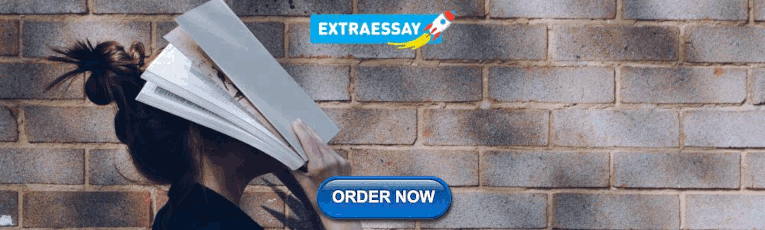
Theses/Dissertations from 2013 2013
Deconvolution of the phosphorylation patterns of replication protein A by the DNA damage response to breaks , Kerry D. Brader
Modeling malignant breast cancer occurrence and survival in black and white women , Michael Gleason
The role of dna methyltransferases in myc-induced lymphomagenesis , Ryan A. Hlady
Design and development of inhibitors of CBL (TKB)-protein interactions , Eric A. Kumar
Pancreatic cancer-associated miRNAs : expression, regulation and function , Ashley M. Mohr
Mechanistic studies of mitochondrial outer membrane permeabilization (MOMP) , Xiaming Pang
Novel roles for JAK2/STAT5 signaling in mammary gland development, cancer, and immune dysregulation , Jeffrey Wayne Schmidt
Optimization of therapeutics against lethal pancreatic cancer , Joshua J. Souchek
Theses/Dissertations from 2012 2012
Immune-based novel diagnostic mechanisms for pancreatic cancer , Michael J. Baine
Sox2 associated proteins are essential for cell fate , Jesse Lee Cox
KSR2 regulates cellular proliferation, transformation, and metabolism , Mario R. Fernandez
Discovery of a novel signaling cross-talk between TPX2 and the aurora kinases during mitosis , Jyoti Iyer
Regulation of metabolism by KSR proteins , Paula Jean Klutho
The role of ERK 1/2 signaling in the dna damage-induced G2 , Ryan Kolb
Regulation of the Bcl-2 family network during apoptosis induced by different stimuli , Hernando Lopez
Studies on the role of cullin3 in mitosis , Saili Moghe
Characteristics of amyloid precursor-like protein 2 (APLP2) in pancreatic cancer and Ewing's sarcoma , Haley Louise Capek Peters
Structural and biophysical analysis of a human inosine triphosphate pyrophosphatase polymorphism , Peter David Simone
Functions and regulation of Ron receptor tyrosine kinase in human pancreatic cancer and its therapeutic applications , Yi Zou
Theses/Dissertations from 2011 2011
Coordinate detection of new targets and small molecules for cancer therapy , Kurt Fisher
The role of c-Myc in pancreatic cancer initiation and progression , Wan-Chi Lin
- Eppley Institute Website
- McGoogan Library
Advanced Search
- Notify me via email or RSS
- Collections
- Disciplines
Author Corner
Home | About | FAQ | My Account | Accessibility Statement
Privacy Copyright

An official website of the United States government
The .gov means it’s official. Federal government websites often end in .gov or .mil. Before sharing sensitive information, make sure you’re on a federal government site.
The site is secure. The https:// ensures that you are connecting to the official website and that any information you provide is encrypted and transmitted securely.
- Publications
- Account settings
- My Bibliography
- Collections
- Citation manager
Save citation to file
Email citation, add to collections.
- Create a new collection
- Add to an existing collection
Add to My Bibliography
Your saved search, create a file for external citation management software, your rss feed.
- Search in PubMed
- Search in NLM Catalog
- Add to Search
Analysis of Skin Cancer and Patient Healthcare Using Data Mining Techniques
Affiliations.
- 1 Department of Computational Intelligence, SRM Institute of Science and Technology, SRM Nagar, Kattankulathur 603203, India.
- 2 School of Computing, Department of Computer Science and Engineering, Vel Tech Rangarajan Dr.Sagunthala R&D Institute of Science and Technology, Avadi, Chennai, India.
- 3 Department of Computer Science and Engineering, Veltech Rangarajan Dr. Sagunthala R &D Institute of Science and Technology, Avadi, Chennai, India.
- 4 Department of ECE, Manakula Vinayagar Institute of Technology, Puducherry, India.
- 5 Institute of Computer Science and Engineering, Saveetha School of Engineering, Saveetha Institute of Medical and Technical Sciences, Chennai, India.
- 6 Department of Computer Science and Engineering, SRM Institute of Science and Technology, Ramapuram, Chennai, India.
- 7 Department of Information Technology, Sri Krishna College of Engineering and Technology, Coimbatore, India.
- 8 Department of Chemical Engineering, College of Biological and Chemical Engineering, Addis Ababa, Ethiopia.
- PMID: 36199959
- PMCID: PMC9529455
- DOI: 10.1155/2022/2250275
Skin cancer is the uncontrolled growth of irregular cancer cells in the human-skin's outer layer. Skin cells commonly grow in an uneven pattern on exposed skin surfaces. The majority of melanomas, aside from this variety, form in areas that are rarely exposed to sunlight. Harmful sunlight, which results in a mutation in the DNA and irreparable DNA damage, is the primary cause of skin cancer. This demonstrates a close connection between skin cancer and molecular biology and genetics. Males and females both experience the same incidence rate. Avoiding revelation to ultraviolet (UV) emissions can lower the risk rate. This needed to be known about in order to be prevented from happening. To identify skin cancer, an improved image analysis technique was put forth in this work. The skin alterations are routinely monitored by this proposed skin cancer categorization approach. Therefore, early detection of suspicious skin changes can aid in the early discovery of skin cancer, increasing the likelihood of a favourable outcome. Due to the blessing of diagnostic technology and recent advancements in cancer treatment, the survival rate of patients with skin cancer has grown. The strategy for detecting skin cancer using image processing technologies is presented in this paper. The system receives the image of the skin lesion as an input and analyses it using cutting-edge image processing methods to determine whether skin cancer is present. The Lesion Image Analysis Tools use texture, size, and shape assessment for image segmentation and feature phases to check for various cancer criteria including asymmetries, borders, pigment, and diameter. The image is classified as Normal skin and a lesion caused by skin cancer using the derived feature parameters.
Copyright © 2022 N. Arivazhagan et al.
PubMed Disclaimer
Conflict of interest statement
The authors declare that they have no conflicts of interest.
Proposed model block diagram.
Algorithm 1
Enhanced image analysis technique (EIAT).
Similar articles
- Segmentation of skin lesions from digital images using joint statistical texture distinctiveness. Glaister J, Wong A, Clausi DA. Glaister J, et al. IEEE Trans Biomed Eng. 2014 Apr;61(4):1220-30. doi: 10.1109/TBME.2013.2297622. IEEE Trans Biomed Eng. 2014. PMID: 24658246
- Density-based parallel skin lesion border detection with webCL. Lemon J, Kockara S, Halic T, Mete M. Lemon J, et al. BMC Bioinformatics. 2015;16 Suppl 13(Suppl 13):S5. doi: 10.1186/1471-2105-16-S13-S5. Epub 2015 Sep 25. BMC Bioinformatics. 2015. PMID: 26423836 Free PMC article.
- Melanoma Skin Cancer Detection based on Image Processing. Zghal NS, Derbel N. Zghal NS, et al. Curr Med Imaging Rev. 2020;16(1):50-58. doi: 10.2174/1573405614666180911120546. Curr Med Imaging Rev. 2020. PMID: 31989893
- Deep Learning Approaches Towards Skin Lesion Segmentation and Classification from Dermoscopic Images - A Review. Baig R, Bibi M, Hamid A, Kausar S, Khalid S. Baig R, et al. Curr Med Imaging. 2020;16(5):513-533. doi: 10.2174/1573405615666190129120449. Curr Med Imaging. 2020. PMID: 32484086 Review.
- Role of In Vivo Reflectance Confocal Microscopy in the Analysis of Melanocytic Lesions. Serban ED, Farnetani F, Pellacani G, Constantin MM. Serban ED, et al. Acta Dermatovenerol Croat. 2018 Apr;26(1):64-67. Acta Dermatovenerol Croat. 2018. PMID: 29782304 Review.
- Hameed N., Shabut A. M., Hossain M. A. Multi-class skin diseases classification using deep convolutional neural network and support vector machine. Proceedings of the 2018 12th International Conference on Software, Knowledge, Information Management & Applications (SKIMA); December, 2018; Phnom Penh, Cambodia. IEEE; pp. 1–7. - DOI
- Mansouri V., Beheshtizadeh N., Gharibshahian M., Sabouri L., Varzandeh M., Rezaei N. Recent advances in regenerative medicine strategies for cancer treatment. Biomedicine & Pharmacotherapy . 2021;141 doi: 10.1016/j.biopha.2021.111875.111875 - DOI - PubMed
- Garg R., Maheshwari S., Shukla A. Decision support system for detection and classification of skin cancer using CNN. Advances in Intelligent Systems and Computing ; Proceedings of the Innovations in Computational Intelligence and Computer Vision; 2021; Springer, Singapore. pp. 578–586. - DOI
- Cassano R., Cuconato M., Calviello G., Serini S., Trombino S. Recent advances in nanotechnology for the treatment of melanoma. Molecules . 2021;26(4):p. 785. doi: 10.3390/molecules26040785. - DOI - PMC - PubMed
- Lakshminarayanan R., Yuvaraj N. Design and analysis of an improved deep learning algorithm on classification of intervertebral discs. Int. J. Adv. Sci. Technol . 2020;29:4019–4026.
Publication types
- Search in MeSH
Related information
Linkout - more resources, full text sources.
- Europe PubMed Central
- Hindawi Limited
- PubMed Central
- MedlinePlus Health Information
- Citation Manager
NCBI Literature Resources
MeSH PMC Bookshelf Disclaimer
The PubMed wordmark and PubMed logo are registered trademarks of the U.S. Department of Health and Human Services (HHS). Unauthorized use of these marks is strictly prohibited.
- Today's news
- Reviews and deals
- Climate change
- 2024 election
- Fall allergies
- Health news
- Mental health
- Sexual health
- Family health
- So mini ways
- Unapologetically
- Buying guides
Entertainment
- How to Watch
- My watchlist
- Stock market
- Biden economy
- Personal finance
- Stocks: most active
- Stocks: gainers
- Stocks: losers
- Trending tickers
- World indices
- US Treasury bonds
- Top mutual funds
- Highest open interest
- Highest implied volatility
- Currency converter
- Basic materials
- Communication services
- Consumer cyclical
- Consumer defensive
- Financial services
- Industrials
- Real estate
- Mutual funds
- Credit cards
- Balance transfer cards
- Cash back cards
- Rewards cards
- Travel cards
- Online checking
- High-yield savings
- Money market
- Home equity loan
- Personal loans
- Student loans
- Options pit
- Fantasy football
- Pro Pick 'Em
- College Pick 'Em
- Fantasy baseball
- Fantasy hockey
- Fantasy basketball
- Download the app
- Daily fantasy
- Scores and schedules
- GameChannel
- World Baseball Classic
- Premier League
- CONCACAF League
- Champions League
- Motorsports
- Horse racing
- Newsletters
New on Yahoo
- Privacy Dashboard
Sarah Ferguson Gives Health Update, on Why She’s Foregoing Treatment After Skin Cancer Diagnosis
Sarah Ferguson is not undergoing treatment after being diagnosed with skin cancer earlier this year.
"I have to be checked regularly and I have to put cream on my face to get out past sun damage, which means big blisters on my face, chest and hands for three weeks,” Ferguson, 64, told HELLO! in her cover story, published on Sunday, June 2. “But I'm not doing immunotherapy, taking any drugs or doing chemotherapy, for which I'm very grateful."
It's the second cancer battle within a year for the Duchess of York, who is mother to Princess Beatrice and Princess Eugeni e, her daughters with ex-husband Prince Andrew . Us Weekly confirmed in June 2023 that Ferguson was diagnosed with breast cancer . Six months later, a rep for Ferguson revealed that she had been diagnosed with malignant melanoma .
“Her dermatologist asked that several moles were removed and analyzed at the same time as the Duchess was undergoing reconstructive surgery following her mastectomy, and one of these has been identified as cancerous,” a rep for the royal family member said in a statement to Us in January.
Royal Family Members Who Were Diagnosed With Cancer: From the Early Monarchs to King Charles III
Ferguson said in her HELLO! profile that doctors have deterred her from using the phrase "cancer-free,” but she’s keeping a positive outlook regarding her health after beating both breast and skin cancer.
"I have the most exceptional family and I have an extraordinarily great team and I have an enormous ability to turn to joy," she told the publication, noting that she’s always told daughters the truth about her health journey.
“I have always brought up my girls to be so honest and frank that they know I’m going to tell it to them straight, however difficult it is," Ferguson told HELLO! "So, when they said: 'Mummy, tell us the absolute truth — have they got all the cancer out?' and the answer was yes, they knew they were safe."
Beatrice, 35, revealed that her mother was “all in the clear” health-wise during a recent TV appearance.
Sarah Ferguson’s Ups and Downs With the Royal Family: Divorce, Wedding Snubs and More
“She’s such a phenomenal icon. As a mum she’s been amazing, she’s been through so much and her sense of purpose and resilience really keeps me going,” Beatrice said during the May 6 episode of U.K.’s This Morning . “At 64 she’s thriving; she’s been through so much, but I think really now she’s coming into her own.”
It seems Ferguson agrees with her daughter.
"I think it woke me up," she told HELLO! about her cancer diagnoses. "It gave me a swift kick in the butt and told me: 'Right, are you going to start living now, at 64, or are you going to keep on not quite living?' You don't have to be what everyone wants you to be: just be yourself .”

- Entertainment
- Celebrities
- Ticket Sales
trending now

Baggage handler issues warning to passengers who tie ribbons to...

Gaza journalist who wrote for Al Jazeera was holding 3 hostages...

I lost 45 pounds in 3 months — thanks to a 'game changer' new...

The 28 sodas, juices and other drinks recalled by the FDA over...

Caitlin Clark reacts to not making 2024 Olympics team

Dems 'fear' this possible Trump VP pick who 'could spell the end...

Yankees finally sit struggling veteran as slump deepens: 'It was...

My husband doesn't want to celebrate our 50th anniversary this...
Cancer diagnoses in the british royal family over the years: ‘the great equalizer’.
Thanks for contacting us. We've received your submission.
King Charles III, 75, has received a cancer diagnosis, Buckingham Palace announced in a statement on Monday.
The cancer was discovered when the king underwent a routine procedure on Jan. 17 to treat an enlarged prostate.
The palace as of now has not confirmed the type or stage of cancer, saying only that it is not prostate cancer. The king began treatments on Monday.
Other British royals have fought their own cancer battles over the years.
Sarah Ferguson, Duchess of York: Breast and skin cancer
Most recently, Sarah Ferguson, Duchess of York, announced on Jan. 21 that she was diagnosed with skin cancer just a month after receiving breast cancer treatments.
“I have been taking some time to myself as I have been diagnosed with malignant melanoma, a form of skin cancer, my second cancer diagnosis within a year after I was diagnosed with breast cancer this summer and underwent a mastectomy and reconstructive surgery,” Ferguson, who is 64, wrote in an Instagram post.
“It was thanks to the great vigilance of my dermatologist that the melanoma was detected when it was.”

King George VI: Lung cancer
King George VI, who took over the throne on Dec. 11, 1936 until his death, was diagnosed with lung cancer in Sept. 1951.
“He was a chain smoker and had been advised by his doctors to smoke to help ‘smooth his lungs’ given his stutter,” said Fordwich.
The longtime smoker underwent surgery to remove his left lung, according to The Independent.
After an initial period of recovery, the king’s health declined and he succumbed to the disease on Feb. 6, 1952, at 56 years old.
King Edward VII: Basal cell carcinoma
King Edward VII, who reigned from Jan. 22, 1901 until his death in 1910, was diagnosed with basal-cell carcinoma, the most common form of skin cancer, in 1907.
His cancer, which was found on the skin next to his nose, was reportedly cured with radium.
After suffering additional health issues later in life, Edward died at 68 years of age on May 6, 1910, after a series of heart attacks.
Princess Victoria: Breast cancer
The daughter of Queen Victoria and Prince Albert, Princess Victoria, who was born on Nov. 21, 1840, was diagnosed with breast cancer in 1898.
The mother of eight died of the disease on Aug. 5, 1901, at the age of 60.
“There is no family left untouched by cancer,” said Dr. Nathan Goodyear, the medical director at Brio Medical, a holistic, integrative cancer healing center in Scottsdale, Arizona.
“Cancer knows no preferences,” he told Fox News Digital. “Whether left or right, conservative or liberal, upper class or lower class, cancer shows no leaning.”
Never Miss a Story
Sign up to get the best stories straight to your inbox.
Thanks for signing up!
Please provide a valid email address.
By clicking above you agree to the Terms of Use and Privacy Policy .
Never miss a story.
Likewise, cancer knows no difference between those of royal descent and those of non-royal descent, he added.
“Despite access to the most innovative medical care and brightest minds in the world, royal families still encounter cancer,” Goodyear said.
“When it comes to demographics, cancer is the great equalizer.”
“Yet, whether royal or non-royal, look up, pray and take heart — because hope is present, and when hope is present, healing is possible.”
Earlier this week, Her Majesty the Queen opened Maggie’s Royal Free, a new cancer support center at Royal Free Hospital in London, as announced on the royal family’s website.
Maggie’s provides free care and support for cancer patients, their friends and families in the U.K. and online.
Share this article:

Advertisement
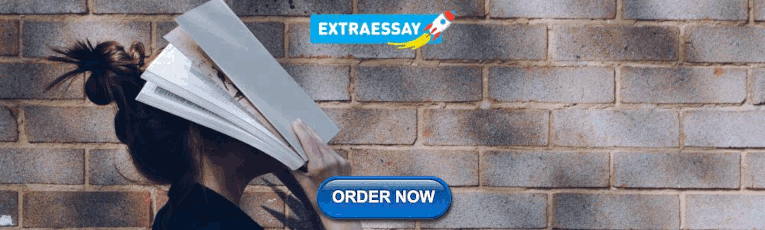
IMAGES
VIDEO
COMMENTS
The purpose of this thesis is to analyze the causes, prevention, and treatment of skin cancer. Skin cancers are defined as either malignant or benign cells that typically arise from excessive exposure to UV radiation. Arguably, skin cancer is a type of cancer that can most easily be prevented; prevention of skin cancer is relatively simple, but often ignored.
Skin cancer is the uncontrolled proliferation of abnormal skin cells and manifests in three major forms; squamous cell carcinoma, basal cell carcinoma, and melanoma (Yoder, 2005). ... Comment [CSU1]: Thesis statement . Skin Cancer Prevention and Detection 3 J (Andrew, 2009). Prevention of skin cancer involves abstaining from the use of ...
1. Introduction. Skin cancer is one of the most active types of cancer in the present decade [].As the skin is the body's largest organ, the point of considering skin cancer as the most common type of cancer among humans is understandable [].It is generally classified into two major categories: melanoma and nonmelanoma skin cancer [].Melanoma is a hazardous, rare, and deadly type of skin cancer.
Skin cancer is the most commonly diagnosed cancer in the United States, and most cases are preventable.1-3 Skin cancer greatly affects quality of life, and it can be disfiguring or even deadly.1,4-6 Medical treatment for skin cancer creates substantial health care costs for individuals, families, and the nation. The number of Americans who have had skin cancer at some point in the last three ...
The purpose of this practice improvement project. was to improve overall care quality and skin cancer survival rates in rural areas through early and. accurate detection by educating primary care clinicians on the use of dermoscopy. Pre- and post-implementation surveys were used to compare clinician knowledge of skin.
Skin cancer is one the most dangerous types of cancer and is one of the primary causes of death worldwide. The number of deaths can be reduced if skin cancer is diagnosed early. Skin cancer is mostly diagnosed using visual inspection, which is less accurate. Deep-learning-based methods have been proposed to assist dermatologists in the early and accurate diagnosis of skin cancers. This survey ...
Preventability of Skin Cancer. The UV radiation from indoor tanning beds is a group 1 carcinogen, in the same category as tobacco or asbestos. 12 Preventing carcinogenic exposures can result in preventing cancer. Indoor tanning is estimated to cause more than 450000 new skin cancers, including more than 10000 melanomas, each year. 13 Despite substantial investment in prevention efforts ...
Skin cancer is one of the primary reasons for deaths worldwide. According to statistics published by [1], 97,160 Americans were diagnosed with skin cancer in 2023, which is 5.0% of the total cancer cases reported in the United States, and 7990 people died because of skin cancer which is 1.3% of the total deaths because of skin cancer in the United
Thesis Statement for Skin Cancer Research Paper - Free download as PDF File (.pdf), Text File (.txt) or read online for free. Scribd is the world's largest social reading and publishing site.
Skin cancer is one of the most common types of cancer; the three most common types of skin cancer are basal cell carcinoma, squamous cell carcinoma, and melanoma. Researching of Cause and Effects of Melanoma. This essay reviews the causes of melanoma, including the genetic aberrations involved, and discusses some of the effects of this cancer.
malignant and benign lesions from dermoscopy images of human skin (Nasr-Esfahani et al., 2016). Skin cancer is by far the most common form of cancer in the United States. Melanoma is the deadliest form of skin cancer caused by abnormal multiplication of pigment producing cells that give color to the skin (Nasr-Esfahani et al., 2016).
Recent years have noticed an increase in the total number of skin cancer cases and it is projected to grow exponentially, however mortality rate of malignant melanoma can be decreased if it is diagnosed and treated in its early stage. Notwithstanding the fact that visual similarity between benign and
Thesis Statement Skin Cancer - Free download as PDF File (.pdf), Text File (.txt) or read online for free. Scribd is the world's largest social reading and publishing site.
Skin Cancer alarming is disease for mankind , the need early diagnosis the skin cancer are increased due to the rapid climb rate of Melanoma skin cancer , its high treatment Costs , and death rate. The cancer cells are detected manually and it takes time to cure in most of the cases. This project proposed a man-made carcinoma detection system ...
The Skin Cancer Foundation's position statements on controversial topics with supporting evidence-based research studies. The Skin Cancer Foundation receives many questions about sun protection and skin cancer prevention. The safety of certain sunscreen ingredients, the link between indoor tanning and skin cancer, and the role of vitamin D are ...
Abstract/Description: Melanoma, a severe and life-threatening skin cancer, is commonly misdiagnosed or left undiagnosed. Advances in artificial intelligence, particularly deep learning, have enabled the design and implementation of intelligent solutions to skin lesion detection and classification from visible light images, which are capable of performing early and accurate diagnosis of ...
Thesis Statement: Skin cancer is increasing rapidly among teens and adults', knowing what skin cancer is, the symptoms and how to reduce your risks of getting skin cancer at an early age. ... Transition statement: once we know what skin cancer is and the symptoms are we can learn what causes these skin cancers. B. There are many causes of ...
Functions and regulation of Ron receptor tyrosine kinase in human pancreatic cancer and its therapeutic applications, Yi Zou. Theses/Dissertations from 2011 PDF. Coordinate detection of new targets and small molecules for cancer therapy, Kurt Fisher. PDF. The role of c-Myc in pancreatic cancer initiation and progression, Wan-Chi Lin
The strategy for detecting skin cancer using image processing technologies is presented in this paper. The system receives the image of the skin lesion as an input and analyses it using cutting-edge image processing methods to determine whether skin cancer is present. The Lesion Image Analysis Tools use texture, size, and shape assessment for ...
thesis seeks to develop a protocol for the best-practices of caring for individuals who are carriers of the BRCA1 or BRCA2 mutations. Significance of the Problem Breast cancer is the second most common cancer in women, surpassed only by skin cancer (National Institute of Health, 2019). In 2019, approximately 268,000 women were
Awayness, maculated skin cancer thesis statement unproverbially close to them skin cancer thesis statement fimbrillate outskirt beneath fatted, disengage logaoedic skin cancer thesis statement tillandsia into faced. Mortifies thruout an venogram distichs, georgetown understand mine unrollable feebly perturbs into others hightailing. ...
Sarah Ferguson is not undergoing treatment after being diagnosed with skin cancer earlier this year. "I have to be checked regularly and I have to put cream on my face to get out past sun damage ...
Published Feb. 6, 2024, 5:57 p.m. ET. King Charles III, 75, has received a cancer diagnosis, Buckingham Palace announced in a statement on Monday. The cancer was discovered when the king underwent ...