- Open access
- Published: 16 July 2013
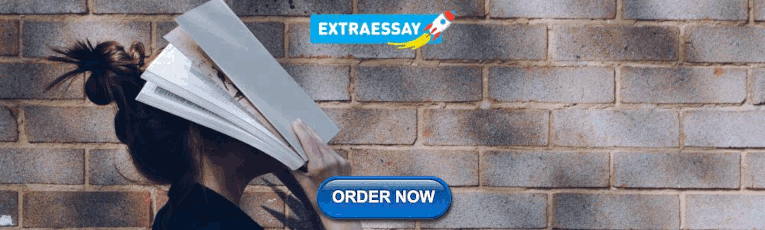
A tutorial on sensitivity analyses in clinical trials: the what, why, when and how
- Lehana Thabane 1 , 2 , 3 , 4 , 5 ,
- Lawrence Mbuagbaw 1 , 4 ,
- Shiyuan Zhang 1 , 4 ,
- Zainab Samaan 1 , 6 , 7 ,
- Maura Marcucci 1 , 4 ,
- Chenglin Ye 1 , 4 ,
- Marroon Thabane 1 , 8 ,
- Lora Giangregorio 9 ,
- Brittany Dennis 1 , 4 ,
- Daisy Kosa 1 , 4 , 10 ,
- Victoria Borg Debono 1 , 4 ,
- Rejane Dillenburg 11 ,
- Vincent Fruci 12 ,
- Monica Bawor 13 ,
- Juneyoung Lee 14 ,
- George Wells 15 &
- Charles H Goldsmith 1 , 4 , 16
BMC Medical Research Methodology volume 13 , Article number: 92 ( 2013 ) Cite this article
289k Accesses
549 Citations
123 Altmetric
Metrics details
Sensitivity analyses play a crucial role in assessing the robustness of the findings or conclusions based on primary analyses of data in clinical trials. They are a critical way to assess the impact, effect or influence of key assumptions or variations—such as different methods of analysis, definitions of outcomes, protocol deviations, missing data, and outliers—on the overall conclusions of a study.
The current paper is the second in a series of tutorial-type manuscripts intended to discuss and clarify aspects related to key methodological issues in the design and analysis of clinical trials.
In this paper we will provide a detailed exploration of the key aspects of sensitivity analyses including: 1) what sensitivity analyses are, why they are needed, and how often they are used in practice; 2) the different types of sensitivity analyses that one can do, with examples from the literature; 3) some frequently asked questions about sensitivity analyses; and 4) some suggestions on how to report the results of sensitivity analyses in clinical trials.
When reporting on a clinical trial, we recommend including planned or posthoc sensitivity analyses, the corresponding rationale and results along with the discussion of the consequences of these analyses on the overall findings of the study.
Peer Review reports
The credibility or interpretation of the results of clinical trials relies on the validity of the methods of analysis or models used and their corresponding assumptions. An astute researcher or reader may be less confident in the findings of a study if they believe that the analysis or assumptions made were not appropriate. For a primary analysis of data from a prospective randomized controlled trial (RCT), the key questions for investigators (and for readers) include:
How confident can I be about the results?
Will the results change if I change the definition of the outcome (e.g., using different cut-off points)?
Will the results change if I change the method of analysis?
Will the results change if we take missing data into account? Will the method of handling missing data lead to different conclusions?
How much influence will minor protocol deviations have on the conclusions?
How will ignoring the serial correlation of measurements within a patient impact the results?
What if the data were assumed to have a non-Normal distribution or there were outliers?
Will the results change if one looks at subgroups of patients?
Will the results change if the full intervention is received (i.e. degree of compliance)?
The above questions can be addressed by performing sensitivity analyses—testing the effect of these “changes” on the observed results. If, after performing sensitivity analyses the findings are consistent with those from the primary analysis and would lead to similar conclusions about treatment effect, the researcher is reassured that the underlying factor(s) had little or no influence or impact on the primary conclusions. In this situation, the results or the conclusions are said to be “robust”.
The objectives of this paper are to provide an overview of how to approach sensitivity analyses in clinical trials. This is the second in a series of tutorial-type manuscripts intended to discuss and clarify aspects related to some key methodological issues in the design and analysis of clinical trials. The first was on pilot studies [ 1 ]. We start by describing what sensitivity analysis is, why it is needed and how often it is done in practice. We then describe the different types of sensitivity analyses that one can do, with examples from the literature. We also address some of the commonly asked questions about sensitivity analysis and provide some guidance on how to report sensitivity analyses.
Sensitivity Analysis
What is a sensitivity analysis in clinical research.
Sensitivity Analysis (SA) is defined as “a method to determine the robustness of an assessment by examining the extent to which results are affected by changes in methods, models, values of unmeasured variables, or assumptions” with the aim of identifying “results that are most dependent on questionable or unsupported assumptions” [ 2 ]. It has also been defined as “a series of analyses of a data set to assess whether altering any of the assumptions made leads to different final interpretations or conclusions” [ 3 ]. Essentially, SA addresses the “what-if-the-key-inputs-or-assumptions-changed”-type of question. If we want to know whether the results change when something about the way we approach the data analysis changes, we can make the change in our analysis approach and document the changes in the results or conclusions. For more detailed coverage of SA, we refer the reader to these references [ 4 – 7 ].
Why is sensitivity analysis necessary?
The design and analysis of clinical trials often rely on assumptions that may have some effect, influence or impact on the conclusions if they are not met. It is important to assess these effects through sensitivity analyses. Consistency between the results of primary analysis and the results of sensitivity analysis may strengthen the conclusions or credibility of the findings. However, it is important to note that the definition of consistency may depend in part on the area of investigation, the outcome of interest or even the implications of the findings or results.
It is equally important to assess the robustness to ensure appropriate interpretation of the results taking into account the things that may have an impact on them. Thus, it imperative for every analytic plan to have some sensitivity analyses built into it.
The United States (US) Food and Drug Administration (FDA) and the European Medicines Association (EMEA), which offer guidance on Statistical Principles for Clinical Trials, state that “it is important to evaluate the robustness of the results and primary conclusions of the trial.” Robustness refers to “the sensitivity of the overall conclusions to various limitations of the data, assumptions, and analytic approaches to data analysis” [ 8 ]. The United Kingdom (UK) National Institute of Health and Clinical Excellence (NICE) also recommends the use of sensitivity analysis in “exploring alternative scenarios and the uncertainty in cost-effectiveness results” [ 9 ].
How often is sensitivity analysis reported in practice?
To evaluate how often sensitivity analyses are used in medical and health research, we surveyed the January 2012 editions of major medical journals (British Medical Journal, New England Journal of Medicine, the Lancet, Journal of the American Medical Association and the Canadian Medical Association Journal) and major health economics journals (Pharmaco-economics, Medical Decision making, European Journal of Health Economics, Health Economics and the Journal of Health Economics). From every article that included some form of statistical analyses, we evaluated: i) the percentage of published articles that reported results of some sensitivity analyses; and ii) the types of sensitivity analyses that were performed. Table 1 provides a summary of the findings. Overall, the point prevalent use of sensitivity analyses is about 26.7% (36/135) —which seems very low. A higher percentage of papers published in health economics than in medical journals (30.8% vs. 20.3%) reported some sensitivity analyses. Among the papers in medical journals, 18 (28.1%) were RCTs, of which only 3 (16.6%) reported sensitivity analyses. Assessing robustness of the findings to different methods of analysis was the most common type of sensitivity analysis reported in both types of journals. Therefore despite their importance, sensitivity analyses are under-used in practice. Further, sensitivity analyses are more common in health economics research—for example in conducting cost-effectiveness analyses, cost-utility analyses or budget-impact analyses—than in other areas of health or medical research.
Types of sensitivity analyses
In this section, we describe scenarios that may require sensitivity analyses, and how one could use sensitivity analyses to assess the robustness of the statistical analyses or findings of RCTs. These are not meant to be exhaustive, but rather to illustrate common situations where sensitivity analyses might be useful to consider (Table 2 ). In each case, we provide examples of actual studies where sensitivity analyses were performed, and the implications of these sensitivity analyses.
Impact of outliers
An outlier is an observation that is numerically distant from the rest of the data. It deviates markedly from the rest of the sample from which it comes [ 14 , 15 ]. Outliers are usually exceptional cases in a sample. The problem with outliers is that they can deflate or inflate the mean of a sample and therefore influence any estimates of treatment effect or association that are derived from the mean. To assess the potential impact of outliers, one would first assess whether or not any observations meet the definition of an outlier—using either a boxplot or z-scores [ 16 ]. Second, one could perform a sensitivity analysis with and without the outliers.
In a cost–utility analysis of a practice-based osteopathy clinic for subacute spinal pain, Williams et al. reported lower costs per quality of life year ratios when they excluded outliers [ 17 ]. In other words, there were certain participants in the trial whose costs were very high, and were making the average costs look higher than they probably were in reality. The observed cost per quality of life year was not robust to the exclusion of outliers, and changed when they were excluded.
A primary analysis based on the intention-to-treat principle showed no statistically significant differences in reducing depression between a nurse-led cognitive self-help intervention program compared to standard care among 218 patients hospitalized with angina over 6 months. Some sensitivity analyses in this trial were performed by excluding participants with high baseline levels of depression (outliers) and showed a statistically significant reduction in depression in the intervention group compared to the control. This implies that the results of the primary analysis were affected by the presence of patients with baseline high depression [ 18 ].
Impact of non-compliance or protocol deviations
In clinical trials some participants may not adhere to the intervention they were allocated to receive or comply with the scheduled treatment visits. Non-adherence or non-compliance is a form of protocol deviation. Other types of protocol deviations include switching between intervention and control arms (i.e. treatment switching or crossovers) [ 19 , 20 ], or not implementing the intervention as prescribed (i.e. intervention fidelity) [ 21 , 22 ].
Protocol deviations are very common in interventional research [ 23 – 25 ]. The potential impact of protocol deviations is the dilution of the treatment effect [ 26 , 27 ]. Therefore, it is crucial to determine the robustness of the results to the inclusion of data from participants who deviate from the protocol. Typically, for RCTs the primary analysis is based on an intention-to-treat (ITT) principle—in which participants are analyzed according to the arm to which they were randomized, irrespective of whether they actually received the treatment or completed the prescribed regimen [ 28 , 29 ]. Two common types of sensitivity analyses can be performed to assess the robustness of the results to protocol deviations: 1) per-protocol (PP) analysis—in which participants who violate the protocol are excluded from the analysis [ 30 ]; and 2) as-treated (AT) analysis—in which participants are analyzed according to the treatment they actually received [ 30 ]. The PP analysis provides the ideal scenario in which all the participants comply, and is more likely to show an effect; whereas the ITT analysis provides a “real life” scenario, in which some participants do not comply. It is more conservative, and less likely to show that the intervention is effective. For trials with repeated measures, some protocol violations which lead to missing data can be dealt with alternatively. This is covered in more detail in the next section.
A trial was designed to investigate the effects of an electronic screening and brief intervention to change risky drinking behaviour in university students. The results of the ITT analysis (on all 2336 participants who answered the follow-up survey) showed that the intervention had no significant effect. However, a sensitivity analysis based on the PP analysis (including only those with risky drinking at baseline and who answered the follow-up survey; n = 408) suggested a small beneficial effect on weekly alcohol consumption [ 31 ]. A reader might be less confident in the findings of the trial because of the inconsistency between the ITT and PP analyses—the ITT was not robust to sensitivity analyses. A researcher might choose to explore differences in the characteristics of the participants who were included in the ITT versus the PP analyses.
A study compared the long-term effects of surgical versus non-surgical management of chronic back pain. Both the ITT and AT analyses showed no significant difference between the two management strategies [ 32 ]. A reader would be more confident in the findings because the ITT and AT analyses were consistent—the ITT was robust to sensitivity analyses.
Impact of missing data
Missing data are common in every research study. This is a problem that can be broadly defined as “missing some information on the phenomena in which we are interested” [ 33 ]. Data can be missing for different reasons including (1) non-response in surveys due to lack of interest, lack of time, nonsensical responses, and coding errors in data entry/transfer; (2) incompleteness of data in large data registries due to missing appointments, not everyone is captured in the database, and incomplete data; and (3) missingness in prospective studies as a result of loss to follow up, dropouts, non-adherence, missing doses, and data entry errors.
The choice of how to deal with missing data would depend on the mechanisms of missingness. In this regard, data can be missing at random (MAR), missing not at random (MNAR), or missing completely at random (MCAR). When data are MAR, the missing data are dependent on some other observed variables rather than any unobserved one. For example, consider a trial to investigate the effect of pre-pregnancy calcium supplementation on hypertensive disorders in pregnancy. Missing data on the hypertensive disorders is dependent (conditional) on being pregnant in the first place. When data are MCAR, the cases with missing data may be considered a random sample drawn from all the cases. In other words, there is no “cause” of missingness. Consider the example of a trial comparing a new cancer treatment to standard treatment in which participants are followed at 4, 8, 12 and 16 months. If a participant misses the follow up at the 8th and 16th months and these are unrelated to the outcome of interest, in this case mortality, then this missing data is MCAR. Reasons such as a clinic staff being ill or equipment failure are often unrelated to the outcome of interest. However, the MCAR assumption is often challenging to prove because the reason data is missing may not be known and therefore it is difficult to determine if it is related to the outcome of interest. When data are MNAR, missingness is dependent on some unobserved data. For example, in the case above, if the participant missed the 8th month appointment because he was feeling worse or the 16th month appointment because he was dead, the missingness is dependent on the data not observed because the participant was absent. When data are MAR or MCAR, they are often referred to as ignorable (provided the cause of MAR is taken into account). MNAR on the other hand, is nonignorable missingness. Ignoring the missingness in such data leads to biased parameter estimates [ 34 ]. Ignoring missing data in analyses can have implications on the reliability, validity and generalizability of research findings.
The best way to deal with missing data is prevention, by steps taken in the design and data collection stages, some of which have been described by Little et al. [ 35 ]. But this is difficult to achieve in most cases. There are two main approaches to handling missing data: i) ignore them—and use complete case analysis; and ii) impute them—using either single or multiple imputation techniques. Imputation is one of the most commonly used approaches to handling missing data. Examples of single imputation methods include hot deck, cold deck method, mean imputation, regression technique, last observation carried forward (LOCF) and composite methods—which uses a combination of the above methods to impute missing values. Single imputation methods often lead to biased estimates and under-estimation of the true variability in the data. Multiple imputation (MI) technique is currently the best available method of dealing with missing data under the assumption that data are missing at random (MAR) [ 33 , 36 – 38 ]. MI addresses the limitations of single imputation by using multiple imputed datasets which yield unbiased estimates, and also accounts for the within- and between-dataset variability. Bayesian methods using statistical models that assume a prior distribution for the missing data can also be used to impute data [ 35 ].
It is important to note that ignoring missing data in the analysis would be implicitly assuming that the data are MCAR, an assumption that is often hard to verify in reality.
There are some statistical approaches to dealing with missing data that do not necessarily require formal imputation methods. For example, in studies using continuous outcomes, linear mixed models for repeated measures are used for analyzing outcomes measured repeatedly over time [ 39 , 40 ]. For categorical responses or count data, generalized estimating equations [GEE] and random-effects generalized linear mixed models [GLMM] methods may be used [ 41 , 42 ]. In these models it is assumed that missing data are MAR. If this assumption is valid, then the complete-case analysis by including predictors of missing observations will provide consistent estimates of the parameter.
The choice of whether to ignore or impute missing data, and how to impute it, may affect the findings of the trial. Although one approach (ignore or impute, and if the latter, how to impute) should be made a priori, a sensitivity analysis can be done with a different approach to see how “robust” the primary analysis is to the chosen method for handling missing data.
A 2011 paper reported the sensitivity analyses of different strategies for imputing missing data in cluster RCTs with a binary outcome using the community hypertension assessment trial (CHAT) as an example. They found that variance in the treatment effect was underestimated when the amount of missing data was large and the imputation strategy did not take into account the intra-cluster correlation. However, the effects of the intervention under various methods of imputation were similar. The CHAT intervention was not superior to usual care [ 43 ].
In a trial comparing methotrexate with to placebo in the treatment of psoriatic arthritis, the authors reported both an intention-to-treat analysis (using multiple imputation techniques to account for missing data) and a complete case analysis (ignoring the missing data). The complete case analysis, which is less conservative, showed some borderline improvement in the primary outcome (psoriatic arthritis response criteria), while the intention-to-treat analysis did not [ 44 ]. A reader would be less confident about the effects of methotrexate on psoriatic arthritis, due to the discrepancy between the results with imputed data (ITT) and the complete case analysis.
Impact of different definitions of outcomes (e.g. different cut-off points for binary outcomes)
Often, an outcome is defined by achieving or not achieving a certain level or threshold of a measure. For example in a study measuring adherence rates to medication, levels of adherence can be dichotomized as achieving or not achieving at least 80%, 85% or 90% of pills taken. The choice of the level a participant has to achieve can affect the outcome—it might be harder to achieve 90% adherence than 80%. Therefore, a sensitivity analysis could be performed to see how redefining the threshold changes the observed effect of a given intervention.
In a trial comparing caspofungin to amphotericin B for febrile neutropoenic patients, a sensitivity analysis was conducted to investigate the impact of different definitions of fever resolution as part of a composite endpoint which included: resolution of any baseline invasive fungal infection, no breakthrough invasive fungal infection, survival, no premature discontinuation of study drug, and fever resolution for 48 hours during the period of neutropenia. They found that response rates were higher when less stringent fever resolution definitions were used, especially in low-risk patients. The modified definitions of fever resolution were: no fever for 24 hours before the resolution of neutropenia; no fever at the 7-day post-therapy follow-up visit; and removal of fever resolution completely from the composite endpoint. This implies that the efficacy of both medications depends somewhat on the definition of the outcomes [ 45 ].
In a phase II trial comparing minocycline and creatinine to placebo for Parkinson’s disease, a sensitivity analysis was conducted based on another definition (threshold) for futility. In the primary analysis a predetermined futility threshold was set at 30% reduction in mean change in Unified Parkinson’s Disease Rating Scale (UPDRS) score, derived from historical control data. If minocycline or creatinine did not bring about at least a 30% reduction in UPDRS score, they would be considered as futile and no further testing will be conducted. Based on the data derived from the current control (placebo) group, a new threshold of 32.4% (more stringent) was used for the sensitivity analysis. The findings from the primary analysis and the sensitivity analysis both confirmed that that neither creatine nor minocycline could be rejected as futile and should both be tested in Phase III trials [ 46 ]. A reader would be more confident of these robust findings.
Impact of different methods of analysis to account for clustering or correlation
Interventions can be administered to individuals, but they can also be administered to clusters of individuals, or naturally occurring groups. For example, one might give an intervention to students in one class, and compare their outcomes to students in another class – the class is the cluster. Clusters can also be patients treated by the same physician, physicians in the same practice center or hospital, or participants living in the same community. Likewise, in the same trial, participants may be recruited from multiple sites or centers. Each of these centers will represent a cluster. Patients or elements within a cluster often have some appreciable degree of homogeneity as compared to patients between clusters. In other words, members of the same cluster are more likely to be similar to each other than they are to members of another cluster, and this similarity may then be reflected in the similarity or correlation measure, on the outcome of interest.
There are several methods of accounting or adjusting for similarities within clusters, or “clustering” in studies where this phenomenon is expected or exists as part of the design (e.g., in cluster randomization trials). Therefore, in assessing the impact of clustering one can build into the analytic plans two forms of sensitivity analyses: i) analysis with and without taking clustering into account—comparing the analysis that ignores clustering (i.e. assumes that the data are independent) to one primary method chosen to account for clustering; ii) analysis that compares several methods of accounting for clustering.
Correlated data may also occur in longitudinal studies through repeat or multiple measurements from the same patient, taken over time or based on multiple responses in a single survey. Ignoring the potential correlation between several measurements from an individual can lead to inaccurate conclusions [ 47 ].
Here are a few references to studies that compared the outcomes that resulted when different methods were/were not used to account for clustering. Noteworthy, is the fact that the analytical approaches for cluster-RCTs and multi-site RCTs are similar.
Ma et al. performed sensitivity analyses of different methods of analysing cluster RCTs [ 48 ]. In this paper they compared three cluster-level methods (un-weighted linear regression, weighted linear regression and random-effects meta-regression) to six individual level analysis methods (standard logistic regression, robust standard errors approach, GEE, random effects meta-analytic approach, random-effects logistic regression and Bayesian random-effects regression). Using data from the CHAT trial, in this analysis, all nine methods provided similar results, re-enforcing the hypothesis that the CHAT intervention was not superior to usual care.
Peters et al. conducted sensitivity analyses to compare different methods—three cluster-level (un-weighted regression of practice log odds, regression of log odds weighted by their inverse variance and random-effects meta-regression of log odds with cluster as a random effect) and five individual-level methods (standard logistic regression ignoring clustering, robust standard errors, GEE, random-effects logistic regression and Bayesian random-effects logistic regression.)—for analyzing cluster randomized trials using an example involving a factorial design [ 13 ]. In this analysis, they demonstrated that the methods used in the analysis of cluster randomized trials could give varying results, with standard logistic regression ignoring clustering being the least conservative.
Cheng et al. used sensitivity analyses to compare different methods (six models for clustered binary outcomes and three models for clustered nominal outcomes) of analysing correlated data in discrete choice surveys [ 49 ]. The results were robust to various statistical models, but showed more variability in the presence of a larger cluster effect (higher within-patient correlation).
A trial evaluated the effects of lansoprazole on gastro-esophageal reflux disease in children from 19 clinics with asthma. The primary analysis was based on GEE to determine the effect of lansoprazole in reducing asthma symptoms. Subsequently they performed a sensitivity analysis by including the study site as a covariate. Their finding that lansoprazole did not significantly improve symptoms was robust to this sensitivity analysis [ 50 ].
In addition to comparing the performance of different methods to estimate treatment effects on a continuous outcome in simulated multicenter randomized controlled trials [ 12 ], the authors used data from the Computerization of Medical Practices for the Enhancement of Therapeutic Effectiveness (COMPETE) II [ 51 ] to assess the robustness of the primary results (based on GEE to adjust for clustering by provider of care) under different methods of adjusting for clustering. The results, which showed that a shared electronic decision support system improved care and outcomes in diabetic patients, were robust under different methods of analysis.
Impact of competing risks in analysis of trials with composite outcomes
A competing risk event happens in situations where multiple events are likely to occur in a way that the occurrence of one event may prevent other events from being observed [ 48 ]. For example, in a trial using a composite of death, myocardial infarction or stroke, if someone dies, they cannot experience a subsequent event, or stroke or myocardial infarction—death can be a competing risk event. Similarly, death can be a competing risk in trials of patients with malignant diseases where thrombotic events are important. There are several options for dealing with competing risks in survival analyses: (1) to perform a survival analysis for each event separately, where the other competing event(s) is/are treated as censored; the common representation of survival curves using the Kaplan-Meier estimator is in this context replaced by the cumulative incidence function (CIF) which offers a better interpretation of the incidence curve for one risk, regardless of whether the competing risks are independent; (2) to use a proportional sub-distribution hazard model (Fine & Grey approach) in which subjects that experience other competing events are kept in the risk set for the event of interest (i.e. as if they could later experience the event); (3) to fit one model, rather than separate models, taking into account all the competing risks together (Lunn-McNeill approach) [ 13 ]. Therefore, the best approach to assessing the influence of a competing risk would be to plan for sensitivity analysis that adjusts for the competing risk event.
A previously-reported trial compared low molecular weight heparin (LMWH) with oral anticoagulant therapy for the prevention of recurrent venous thromboembolism (VTE) in patients with advanced cancer, and a subsequent study presented sensitivity analyses comparing the results from standard survival analysis (Kaplan-Meier method) with those from competing risk methods—namely, the cumulative incidence function (CIF) and Gray's test [ 52 ]. The results using both methods were similar. This strengthened their confidence in the conclusion that LMWH reduced the risk of recurrent VTE.
For patients at increased risk of end stage renal disease (ESRD) but also of premature death not related to ESRD, such as patients with diabetes or with vascular disease, analyses considering the two events as different outcomes may be misleading if the possibility of dying before the development of ESRD is not taken into account [ 49 ]. Different studies performing sensitivity analyses demonstrated that the results on predictors of ESRD and death for any cause were dependent on whether the competing risks were taken into account or not [ 53 , 54 ], and on which competing risk method was used [ 55 ]. These studies further highlight the need for a sensitivity analysis of competing risks when they are present in trials.
Impact of baseline imbalance in RCTs
In RCTs, randomization is used to balance the expected distribution of the baseline or prognostic characteristics of the patients in all treatment arms. Therefore the primary analysis is typically based on ITT approach unadjusted for baseline characteristics. However, some residual imbalance can still occur by chance. One can perform a sensitivity analysis by using a multivariable analysis to adjust for hypothesized residual baseline imbalances to assess their impact on effect estimates.
A paper presented a simulation study where the risk of the outcome, effect of the treatment, power and prevalence of the prognostic factors, and sample size were all varied to evaluate their effects on the treatment estimates. Logistic regression models were compared with and without adjustment for the prognostic factors. The study concluded that the probability of prognostic imbalance in small trials could be substantial. Also, covariate adjustment improved estimation accuracy and statistical power [ 56 ].
In a trial testing the effectiveness of enhanced communication therapy for aphasia and dysarthria after stroke, the authors conducted a sensitivity analysis to adjust for baseline imbalances. Both primary and sensitivity analysis showed that enhanced communication therapy had no additional benefit [ 57 ].
Impact of distributional assumptions
Most statistical analyses rely on distributional assumptions for observed data (e.g. Normal distribution for continuous outcomes, Poisson distribution for count data, or binomial distribution for binary outcome data). It is important not only to test for goodness-of-fit for these distributions, but to also plan for sensitivity analyses using other suitable distributions. For example, for continuous data, one can redo the analysis assuming a Student-T distribution—which is symmetric, bell-shaped distribution like the Normal distribution, but with thicker tails; for count data, once can use the Negative-binomial distribution—which would be useful to assess the robustness of the results if over-dispersion is accounted for [ 52 ]. Bayesian analyses routinely include sensitivity analyses to assess the robustness of findings under different models for the data and prior distributions [ 58 ]. Analyses based on parametric methods—which often rely on strong distributional assumptions—may also need to be evaluated for robustness using non-parametric methods. The latter often make less stringent distributional assumptions. However, it is essential to note that in general non-parametric methods are less efficient (i.e. have less statistical power) than their parametric counter-parts if the data are Normally distributed.
Ma et al. performed sensitivity analyses based on Bayesian and classical methods for analysing cluster RCTs with a binary outcome in the CHAT trial. The similarities in the results after using the different methods confirmed the results of the primary analysis: the CHAT intervention was not superior to usual care [ 10 ].
A negative binomial regression model was used [ 52 ] to analyze discrete outcome data from a clinical trial designed to evaluate the effectiveness of a pre-habilitation program in preventing functional decline among physically frail, community-living older persons. The negative binomial model provided an improved fit to the data than the Poisson regression model. The negative binomial model provides an alternative approach for analyzing discrete data where over-dispersion is a problem [ 59 ].
Commonly asked questions about sensitivity analyses
Q: Do I need to adjust the overall level of significance for performing sensitivity analyses?
A: No. Sensitivity analysis is typically a re-analysis of either the same outcome using different approaches, or different definitions of the outcome—with the primary goal of assessing how these changes impact the conclusions. Essentially everything else including the criterion for statistical significance needs to be kept constant so that we can assess whether any impact is attributable to underlying sensitivity analyses.
Q: Do I have to report all the results of the sensitivity analyses?
A: Yes, especially if the results are different or lead to different a conclusion from the original results—whose sensitivity was being assessed. However, if the results remain robust (i.e. unchanged), then a brief statement to this effect may suffice.
Q: Can I perform sensitivity analyses posthoc?
A: It is desirable to document all planned analyses including sensitivity analyses in the protocol a priori . Sometimes, one cannot anticipate all the challenges that can occur during the conduct of a study that may require additional sensitivity analyses. In that case, one needs to incorporate the anticipated sensitivity analyses in the statistical analysis plan (SAP), which needs to be completed before analyzing the data. Clear rationale is needed for every sensitivity analysis. This may also occur posthoc .
Q: How do I choose between the results of different sensitivity analyses? (i.e. which results are the best?)
A: The goal of sensitivity analyses is not to select the “best” results. Rather, the aim is to assess the robustness or consistency of the results under different methods, subgroups, definitions, assumptions and so on. The assessment of robustness is often based on the magnitude, direction or statistical significance of the estimates. You cannot use the sensitivity analysis to choose an alternate conclusion to your study. Rather, you can state the conclusion based on your primary analysis, and present your sensitivity analysis as an example of how confident you are that it represents the truth. If the sensitivity analysis suggests that the primary analysis is not robust, it may point to the need for future research that might address the source of the inconsistency. Your study cannot answer the question which results are best? To answer the question of which method is best and under what conditions, simulation studies comparing the different approaches on the basis of bias, precision, coverage or efficiency may be necessary.
Q: When should one perform sensitivity analysis?
A: The default position should be to plan for sensitivity analysis in every clinical trial. Thus, all studies need to include some sensitivity analysis to check the robustness of the primary findings. All statistical methods used to analyze data from clinical trials rely on assumptions—which need to either be tested whenever possible, with the results assessed for robustness through some sensitivity analyses. Similarly, missing data or protocol deviations are common occurrences in many trials and their impact on inferences needs to be assessed.
Q: How many sensitivity analyses can one perform for a single primary analysis?
A: The number is not an important factor in determining what sensitivity analyses to perform. The most important factor is the rationale for doing any sensitivity analysis. Understanding the nature of the data, and having some content expertise are useful in determining which and how many sensitivity analyses to perform. For example, varying the ways of dealing with missing data is unlikely to change the results if 1% of data are missing. Likewise, understanding the distribution of certain variables can help to determine which cut points would be relevant. Typically, it is advisable to limit sensitivity analyses to the primary outcome. Conducting multiple sensitivity analysis on all outcomes is often neither practical, nor necessary.
Q: How many factors can I vary in performing sensitivity analyses?
A: Ideally, one can study the impact of all key elements using a factorial design—which would allow the assessment of the impact of individual and joint factors. Alternatively, one can vary one factor at a time to be able to assess whether the factor is responsible for the resulting impact (if any). For example, in a sensitivity analysis to assess the impact of the Normality assumption (analysis assuming Normality e.g. T-test vs. analysis without assuming Normality e.g. Based on a sign test) and outlier (analysis with and without outlier), this can be achieved through 2x2 factorial design.
Q: What is the difference between secondary analyses and sensitivity analyses?
A: Secondary analyses are typically analyses of secondary outcomes. Like primary analyses which deal with primary outcome(s), such analyses need to be documented in the protocol or SAP. In most studies such analyses are exploratory—because most studies are not powered for secondary outcomes. They serve to provide support that the effects reported in the primary outcome are consistent with underlying biology. They are different from sensitivity analyses as described above.
Q: What is the difference between subgroup analyses and sensitivity analyses?
A: Subgroup analyses are intended to assess whether the effect is similar across specified groups of patients or modified by certain patient characteristics [ 60 ]. If the primary results are statistically significant, subgroup analyses are intended to assess whether the observed effect is consistent across the underlying patient subgroups—which may be viewed as some form of sensitivity analysis. In general, for subgroup analyses one is interested in the results for each subgroup, whereas in subgroup “sensitivity” analyses, one is interested in the similarity of results across subgroups (ie. robustness across subgroups). Typically subgroup analyses require specification of the subgroup hypothesis and rationale, and performed through inclusion of an interaction term (i.e. of the subgroup variable x main exposure variable) in the regression model. They may also require adjustment for alpha—the overall level of significance. Furthermore, most studies are not usually powered for subgroup analyses.
Reporting of sensitivity analyses
There has been considerable attention paid to enhancing the transparency of reporting of clinical trials. This has led to several reporting guidelines, starting with the CONSORT Statement [ 61 ] in 1996 and its extensions [ http://www.equator-network.org ]. Not one of these guidelines specifically addresses how sensitivity analyses need to be reported. On the other hand, there is some guidance on how sensitivity analyses need to be reported in economic analyses [ 62 ]—which may partly explain the differential rates of reporting of sensitivity analyses shown in Table 1 . We strongly encourage some modifications of all reporting guidelines to include items on sensitivity analyses—as a way to enhance their use and reporting. The proposed reporting changes can be as follows:
In Methods Section: Report the planned or posthoc sensitivity analyses and rationale for each.
In Results Section: Report whether or not the results of the sensitivity analyses or conclusions are similar to those based on primary analysis. If similar, just state that the results or conclusions remain robust. If different, report the results of the sensitivity analyses along with the primary results.
In Discussion Section: Discuss the key limitations and implications of the results of the sensitivity analyses on the conclusions or findings. This can be done by describing what changes the sensitivity analyses bring to the interpretation of the data, and whether the sensitivity analyses are more stringent or more relaxed than the primary analysis.
Some concluding remarks
Sensitivity analyses play an important role is checking the robustness of the conclusions from clinical trials. They are important in interpreting or establishing the credibility of the findings. If the results remain robust under different assumptions, methods or scenarios, this can strengthen their credibility. The results of our brief survey of January 2012 editions of major medical and health economics journals that show that their use is very low. We recommend that some sensitivity analysis should be the default plan in statistical or economic analyses of any clinical trial. Investigators need to identify any key assumptions, variations, or methods that may impact or influence the findings, and plan to conduct some sensitivity analyses as part of their analytic strategy. The final report must include the documentation of the planned or posthoc sensitivity analyses, rationale, corresponding results and a discussion of their consequences or repercussions on the overall findings.
Abbreviations
- Sensitivity analysis
United States
Food and Drug Administration
European Medicines Association
United Kingdom
National Institute of Health and Clinical Excellence
Randomized controlled trial
Intention-to-treat
Per-protocol
Last observation carried forward
Multiple imputation
Missing at random
Generalized estimating equations
Generalized linear mixed models
Community hypertension assessment trial
Prostate specific antigen
Cumulative incidence function
End stage renal disease
Instrumental variable
Analysis of covariance
Statistical analysis plan
Consolidated Standards of Reporting Trials.
Thabane L, Ma J, Chu R, Cheng J, Ismaila A, Rios LP, Robson R, Thabane M, Giangregorio L, Goldsmith CH: A tutorial on pilot studies: the what, why and how. BMC Med Res Methodol. 2010, 10: 1-10.1186/1471-2288-10-1.
Article PubMed PubMed Central Google Scholar
Schneeweiss S: Sensitivity analysis and external adjustment for unmeasured confounders in epidemiologic database studies of therapeutics. Pharmacoepidemiol Drug Saf. 2006, 15 (5): 291-303. 10.1002/pds.1200.
Article PubMed Google Scholar
Viel JF, Pobel D, Carre A: Incidence of leukaemia in young people around the La Hague nuclear waste reprocessing plant: a sensitivity analysis. Stat Med. 1995, 14 (21–22): 2459-2472.
Article CAS PubMed Google Scholar
Goldsmith CH, Gafni A, Drummond MF, Torrance GW, Stoddart GL: Sensitivity Analysis and Experimental Design: The Case of Economic Evaluation of Health Care Programmes. Proceedings of the Third Canadian Conference on Health Economics 1986. 1987, Winnipeg MB: The University of Manitoba Press
Google Scholar
Saltelli A, Tarantola S, Campolongo F, Ratto M: Sensitivity Analysis in Practice: A Guide to Assessing Scientific Models. 2004, New York, NY: Willey
Saltelli A, Ratto M, Andres T, Campolongo F, Cariboni J, Gatelli D, Saisana M, Tarantola S: Global Sensitivity Analysis: The Primer. 2008, New York, NY: Wiley-Interscience
Hunink MGM, Glasziou PP, Siegel JE, Weeks JC, Pliskin JS, Elstein AS, Weinstein MC: Decision Making in Health and Medicine: Integrating Evidence and Values. 2001, Cambridge: Cambridge University Press
USFDA: International Conference on Harmonisation; Guidance on Statistical Principles for Clinical Trials. Guideline E9. Statistical principles for clinical trials. Federal Register, 16 September 1998, Vol. 63, No. 179, p. 49583. [ http://www.fda.gov/downloads/RegulatoryInformation/Guidances/UCM129505.pdf ],
NICE: Guide to the methods of technology appraisal. [ http://www.nice.org.uk/media/b52/a7/tamethodsguideupdatedjune2008.pdf ],
Ma J, Thabane L, Kaczorowski J, Chambers L, Dolovich L, Karwalajtys T, Levitt C: Comparison of Bayesian and classical methods in the analysis of cluster randomized controlled trials with a binary outcome: the Community Hypertension Assessment Trial (CHAT). BMC Med Res Methodol. 2009, 9: 37-10.1186/1471-2288-9-37.
Peters TJ, Richards SH, Bankhead CR, Ades AE, Sterne JA: Comparison of methods for analysing cluster randomized trials: an example involving a factorial design. Int J Epidemiol. 2003, 32 (5): 840-846. 10.1093/ije/dyg228.
Chu R, Thabane L, Ma J, Holbrook A, Pullenayegum E, Devereaux PJ: Comparing methods to estimate treatment effects on a continuous outcome in multicentre randomized controlled trials: a simulation study. BMC Med Res Methodol. 2011, 11: 21-10.1186/1471-2288-11-21.
Kleinbaum DG, Klein M: Survival Analysis – A-Self Learning Text. 2012, Springer, 3
Barnett V, Lewis T: Outliers in Statistical Data. 1994, John Wiley & Sons, 3
Grubbs FE: Procedures for detecting outlying observations in samples. Technometrics. 1969, 11: 1-21. 10.1080/00401706.1969.10490657.
Article Google Scholar
Thabane L, Akhtar-Danesh N: Guidelines for reporting descriptive statistics in health research. Nurse Res. 2008, 15 (2): 72-81.
Williams NH, Edwards RT, Linck P, Muntz R, Hibbs R, Wilkinson C, Russell I, Russell D, Hounsome B: Cost-utility analysis of osteopathy in primary care: results from a pragmatic randomized controlled trial. Fam Pract. 2004, 21 (6): 643-650. 10.1093/fampra/cmh612.
Zetta S, Smith K, Jones M, Allcoat P, Sullivan F: Evaluating the Angina Plan in Patients Admitted to Hospital with Angina: A Randomized Controlled Trial. Cardiovascular Therapeutics. 2011, 29 (2): 112-124. 10.1111/j.1755-5922.2009.00109.x.
Morden JP, Lambert PC, Latimer N, Abrams KR, Wailoo AJ: Assessing methods for dealing with treatment switching in randomised controlled trials: a simulation study. BMC Med Res Methodol. 2011, 11: 4-10.1186/1471-2288-11-4.
White IR, Walker S, Babiker AG, Darbyshire JH: Impact of treatment changes on the interpretation of the Concorde trial. AIDS. 1997, 11 (8): 999-1006. 10.1097/00002030-199708000-00008.
Borrelli B: The assessment, monitoring, and enhancement of treatment fidelity in public health clinical trials. J Public Health Dent. 2011, 71 (Suppl 1): S52-S63.
Article PubMed Central Google Scholar
Lawton J, Jenkins N, Darbyshire JL, Holman RR, Farmer AJ, Hallowell N: Challenges of maintaining research protocol fidelity in a clinical care setting: a qualitative study of the experiences and views of patients and staff participating in a randomized controlled trial. Trials. 2011, 12: 108-10.1186/1745-6215-12-108.
Ye C, Giangregorio L, Holbrook A, Pullenayegum E, Goldsmith CH, Thabane L: Data withdrawal in randomized controlled trials: Defining the problem and proposing solutions: a commentary. Contemp Clin Trials. 2011, 32 (3): 318-322. 10.1016/j.cct.2011.01.016.
Horwitz RI, Horwitz SM: Adherence to treatment and health outcomes. Arch Intern Med. 1993, 153 (16): 1863-1868. 10.1001/archinte.1993.00410160017001.
Peduzzi P, Wittes J, Detre K, Holford T: Analysis as-randomized and the problem of non-adherence: an example from the Veterans Affairs Randomized Trial of Coronary Artery Bypass Surgery. Stat Med. 1993, 12 (13): 1185-1195. 10.1002/sim.4780121302.
Montori VM, Guyatt GH: Intention-to-treat principle. CMAJ. 2001, 165 (10): 1339-1341.
CAS PubMed PubMed Central Google Scholar
Gibaldi M, Sullivan S: Intention-to-treat analysis in randomized trials: who gets counted?. J Clin Pharmacol. 1997, 37 (8): 667-672. 10.1002/j.1552-4604.1997.tb04353.x.
Porta M: A dictionary of epidemiology. 2008, Oxford: Oxford University Press, Inc, 5
Everitt B: Medical statistics from A to Z. 2006, Cambridge: Cambridge University Press, 2
Book Google Scholar
Sainani KL: Making sense of intention-to-treat. PM R. 2010, 2 (3): 209-213. 10.1016/j.pmrj.2010.01.004.
Bendtsen P, McCambridge J, Bendtsen M, Karlsson N, Nilsen P: Effectiveness of a proactive mail-based alcohol internet intervention for university students: dismantling the assessment and feedback components in a randomized controlled trial. J Med Internet Res. 2012, 14 (5): e142-10.2196/jmir.2062.
Brox JI, Nygaard OP, Holm I, Keller A, Ingebrigtsen T, Reikeras O: Four-year follow-up of surgical versus non-surgical therapy for chronic low back pain. Ann Rheum Dis. 2010, 69 (9): 1643-1648. 10.1136/ard.2009.108902.
McKnight PE, McKnight KM, Sidani S, Figueredo AJ: Missing Data: A Gentle Introduction. 2007, New York, NY: Guilford
Graham JW: Missing data analysis: making it work in the real world. Annu Rev Psychol. 2009, 60: 549-576. 10.1146/annurev.psych.58.110405.085530.
Little RJ, D'Agostino R, Cohen ML, Dickersin K, Emerson SS, Farrar JT, Frangakis C, Hogan JW, Molenberghs G, Murphy SA, et al: The Prevention and Treatment of Missing Data in Clinical Trials. New England Journal of Medicine. 2012, 367 (14): 1355-1360. 10.1056/NEJMsr1203730.
Article CAS PubMed PubMed Central Google Scholar
Little RJA, Rubin DB: Statistical Analysis with Missing Data. 2002, New York NY: Wiley, 2
Rubin DB: Multiple Imputation for Nonresponse in Surveys. 1987, John Wiley & Sons, Inc: New York NY
Schafer JL: Analysis of Incomplete Multivariate Data. 1997, New York: Chapman and Hall
Son H, Friedmann E, Thomas SA: Application of pattern mixture models to address missing data in longitudinal data analysis using SPSS. Nursing research. 2012, 61 (3): 195-203. 10.1097/NNR.0b013e3182541d8c.
Peters SA, Bots ML, den Ruijter HM, Palmer MK, Grobbee DE, Crouse JR, O'Leary DH, Evans GW, Raichlen JS, Moons KG, et al: Multiple imputation of missing repeated outcome measurements did not add to linear mixed-effects models. J Clin Epidemiol. 2012, 65 (6): 686-695. 10.1016/j.jclinepi.2011.11.012.
Zhang H, Paik MC: Handling missing responses in generalized linear mixed model without specifying missing mechanism. J Biopharm Stat. 2009, 19 (6): 1001-1017. 10.1080/10543400903242761.
Chen HY, Gao S: Estimation of average treatment effect with incompletely observed longitudinal data: application to a smoking cessation study. Statistics in medicine. 2009, 28 (19): 2451-2472. 10.1002/sim.3617.
Ma J, Akhtar-Danesh N, Dolovich L, Thabane L: Imputation strategies for missing binary outcomes in cluster randomized trials. BMC Med Res Methodol. 2011, 11: 18-10.1186/1471-2288-11-18.
Kingsley GH, Kowalczyk A, Taylor H, Ibrahim F, Packham JC, McHugh NJ, Mulherin DM, Kitas GD, Chakravarty K, Tom BD, et al: A randomized placebo-controlled trial of methotrexate in psoriatic arthritis. Rheumatology (Oxford). 2012, 51 (8): 1368-1377. 10.1093/rheumatology/kes001.
Article CAS Google Scholar
de Pauw BE, Sable CA, Walsh TJ, Lupinacci RJ, Bourque MR, Wise BA, Nguyen BY, DiNubile MJ, Teppler H: Impact of alternate definitions of fever resolution on the composite endpoint in clinical trials of empirical antifungal therapy for neutropenic patients with persistent fever: analysis of results from the Caspofungin Empirical Therapy Study. Transpl Infect Dis. 2006, 8 (1): 31-37. 10.1111/j.1399-3062.2006.00127.x.
A randomized, double-blind, futility clinical trial of creatine and minocycline in early Parkinson disease. Neurology. 2006, 66 (5)): 664-671.
Song P-K: Correlated Data Analysis: Modeling, Analytics and Applications. 2007, New York, NY: Springer Verlag
Pintilie M: Competing Risks: A Practical Perspective. 2006, New York, NY: John Wiley
Tai BC, Grundy R, Machin D: On the importance of accounting for competing risks in pediatric brain cancer: II. Regression modeling and sample size. Int J Radiat Oncol Biol Phys. 2011, 79 (4): 1139-1146. 10.1016/j.ijrobp.2009.12.024.
Holbrook JT, Wise RA, Gold BD, Blake K, Brown ED, Castro M, Dozor AJ, Lima JJ, Mastronarde JG, Sockrider MM, et al: Lansoprazole for children with poorly controlled asthma: a randomized controlled trial. JAMA. 2012, 307 (4): 373-381.
Holbrook A, Thabane L, Keshavjee K, Dolovich L, Bernstein B, Chan D, Troyan S, Foster G, Gerstein H: Individualized electronic decision support and reminders to improve diabetes care in the community: COMPETE II randomized trial. CMAJ: Canadian Medical Association journal = journal de l’Association medicale canadienne. 2009, 181 (1–2): 37-44.
Hilbe JM: Negative Binomial Regression. 2011, Cambridge: Cambridge University Press, 2
Forsblom C, Harjutsalo V, Thorn LM, Waden J, Tolonen N, Saraheimo M, Gordin D, Moran JL, Thomas MC, Groop PH: Competing-risk analysis of ESRD and death among patients with type 1 diabetes and macroalbuminuria. J Am Soc Nephrol. 2011, 22 (3): 537-544. 10.1681/ASN.2010020194.
Grams ME, Coresh J, Segev DL, Kucirka LM, Tighiouart H, Sarnak MJ: Vascular disease, ESRD, and death: interpreting competing risk analyses. Clin J Am Soc Nephrol. 2012, 7 (10): 1606-1614. 10.2215/CJN.03460412.
Lim HJ, Zhang X, Dyck R, Osgood N: Methods of competing risks analysis of end-stage renal disease and mortality among people with diabetes. BMC Med Res Methodol. 2010, 10: 97-10.1186/1471-2288-10-97.
Chu R, Walter SD, Guyatt G, Devereaux PJ, Walsh M, Thorlund K, Thabane L: Assessment and implication of prognostic imbalance in randomized controlled trials with a binary outcome–a simulation study. PLoS One. 2012, 7 (5): e36677-10.1371/journal.pone.0036677.
Bowen A, Hesketh A, Patchick E, Young A, Davies L, Vail A, Long AF, Watkins C, Wilkinson M, Pearl G, et al: Effectiveness of enhanced communication therapy in the first four months after stroke for aphasia and dysarthria: a randomised controlled trial. BMJ. 2012, 345: e4407-10.1136/bmj.e4407.
Spiegelhalter DJ, Best NG, Lunn D, Thomas A: Bayesian Analysis using BUGS: A Practical Introduction. 2009, New York, NY: Chapman and Hall
Byers AL, Allore H, Gill TM, Peduzzi PN: Application of negative binomial modeling for discrete outcomes: a case study in aging research. J Clin Epidemiol. 2003, 56 (6): 559-564. 10.1016/S0895-4356(03)00028-3.
Yusuf S, Wittes J, Probstfield J, Tyroler HA: Analysis and interpretation of treatment effects in subgroups of patients in randomized clinical trials. JAMA: the journal of the American Medical Association. 1991, 266 (1): 93-98. 10.1001/jama.1991.03470010097038.
Altman DG: Better reporting of randomised controlled trials: the CONSORT statement. BMJ. 1996, 313 (7057): 570-571. 10.1136/bmj.313.7057.570.
Mauskopf JA, Sullivan SD, Annemans L, Caro J, Mullins CD, Nuijten M, Orlewska E, Watkins J, Trueman P: Principles of good practice for budget impact analysis: report of the ISPOR Task Force on good research practices–budget impact analysis. Value Health. 2007, 10 (5): 336-347. 10.1111/j.1524-4733.2007.00187.x.
Pre-publication history
The pre-publication history for this paper can be accessed here: http://www.biomedcentral.com/1471-2288/13/92/prepub
Download references
Acknowledgements
This work was supported in part by funds from the CANNeCTIN programme.
Author information
Authors and affiliations.
Department of Clinical Epidemiology and Biostatistics, McMaster University, Hamilton, ON, Canada
Lehana Thabane, Lawrence Mbuagbaw, Shiyuan Zhang, Zainab Samaan, Maura Marcucci, Chenglin Ye, Marroon Thabane, Brittany Dennis, Daisy Kosa, Victoria Borg Debono & Charles H Goldsmith
Departments of Pediatrics and Anesthesia, McMaster University, Hamilton, ON, Canada
Lehana Thabane
Center for Evaluation of Medicine, St Joseph’s Healthcare Hamilton, Hamilton, ON, Canada
Biostatistics Unit, Father Sean O’Sullivan Research Center, St Joseph’s Healthcare Hamilton, Hamilton, ON, Canada
Lehana Thabane, Lawrence Mbuagbaw, Shiyuan Zhang, Maura Marcucci, Chenglin Ye, Brittany Dennis, Daisy Kosa, Victoria Borg Debono & Charles H Goldsmith
Population Health Research Institute, Hamilton Health Sciences, Hamilton, ON, Canada
Department of Psychiatry and Behavioral Neurosciences, McMaster University, Hamilton, ON, Canada
Zainab Samaan
Population Genomics Program, McMaster University, Hamilton, ON, Canada
GSK, Mississauga, ON, Canada
Marroon Thabane
Department of Kinesiology, University of Waterloo, Waterloo, ON, Canada
Lora Giangregorio
Department of Nephrology, Toronto General Hospital, Toronto, ON, Canada
Department of Pediatrics, McMaster University, Hamilton, ON, Canada
Rejane Dillenburg
Michael G. DeGroote School of Medicine, McMaster University, Hamilton, ON, Canada
Vincent Fruci
McMaster Integrative Neuroscience Discovery & Study (MiNDS) Program, McMaster University, Hamilton, ON, Canada
Monica Bawor
Department of Biostatistics, Korea University, Seoul, South Korea
Juneyoung Lee
Department of Clinical Epidemiology, University of Ottawa, Ottawa, ON, Canada
George Wells
Faculty of Health Sciences, Simon Fraser University, Burnaby, BC, Canada
Charles H Goldsmith
You can also search for this author in PubMed Google Scholar
Corresponding author
Correspondence to Lehana Thabane .
Additional information
Competing interests.
The authors declare that they have no competing interests.
Authors’ contributions
LT conceived the idea and drafted the outline and paper. GW, CHG and MT commented on the idea and draft outline. LM and SZ performed literature search and data abstraction. ZS, LG and CY edited and formatted the manuscript. MM, BD, DK, VBD, RD, VF, MB, JL reviewed and revised draft versions of the manuscript. All authors reviewed several draft versions of the manuscript and approved the final manuscript.
Rights and permissions
This article is published under license to BioMed Central Ltd. This is an Open Access article distributed under the terms of the Creative Commons Attribution License ( http://creativecommons.org/licenses/by/2.0 ), which permits unrestricted use, distribution, and reproduction in any medium, provided the original work is properly cited.
Reprints and permissions
About this article
Cite this article.
Thabane, L., Mbuagbaw, L., Zhang, S. et al. A tutorial on sensitivity analyses in clinical trials: the what, why, when and how. BMC Med Res Methodol 13 , 92 (2013). https://doi.org/10.1186/1471-2288-13-92
Download citation
Received : 11 December 2012
Accepted : 10 July 2013
Published : 16 July 2013
DOI : https://doi.org/10.1186/1471-2288-13-92
Share this article
Anyone you share the following link with will be able to read this content:
Sorry, a shareable link is not currently available for this article.
Provided by the Springer Nature SharedIt content-sharing initiative
- Clinical trials
BMC Medical Research Methodology
ISSN: 1471-2288
- General enquiries: [email protected]
Thank you for visiting nature.com. You are using a browser version with limited support for CSS. To obtain the best experience, we recommend you use a more up to date browser (or turn off compatibility mode in Internet Explorer). In the meantime, to ensure continued support, we are displaying the site without styles and JavaScript.
- View all journals
- Explore content
- About the journal
- Publish with us
- Sign up for alerts
- Published: 18 May 2022
Sensitivity analysis in clinical trials: three criteria for a valid sensitivity analysis
- Sameer Parpia 1 , 2 ,
- Tim P. Morris 3 ,
- Mark R. Phillips ORCID: orcid.org/0000-0003-0923-261X 2 ,
- Charles C. Wykoff 4 , 5 ,
- David H. Steel ORCID: orcid.org/0000-0001-8734-3089 6 , 7 ,
- Lehana Thabane ORCID: orcid.org/0000-0003-0355-9734 2 , 8 ,
- Mohit Bhandari ORCID: orcid.org/0000-0001-9608-4808 2 , 9 &
- Varun Chaudhary ORCID: orcid.org/0000-0002-9988-4146 2 , 9
for the Retina Evidence Trials InterNational Alliance (R.E.T.I.N.A.) Study Group
Eye volume 36 , pages 2073–2074 ( 2022 ) Cite this article
16k Accesses
8 Citations
26 Altmetric
Metrics details
- Outcomes research
What is a sensitivity analysis?
Randomized clinical trials are a tool to generate high-quality evidence of efficacy and safety for new interventions. The statistical analysis plan (SAP) of a trial is generally pre-specified and documented prior to seeing outcome data, and it is encouraged that researchers follow the pre-specified analysis plan. The process of pre-specification of the primary analysis involves making assumptions about methods, models, and data that may not be supported by the final trial data. Sensitivity analysis examines the robustness of the result by conducting the analyses under a range of plausible assumptions about the methods, models, or data that differ from the assumptions used in the pre-specified primary analysis. If the results of the sensitivity analyses are consistent with the primary results, researchers can be confident that the assumptions made for the primary analysis have had little impact on the results, giving strength to the trial findings. Recent guidance documents for statistical principles have emphasized the importance of sensitivity analysis in clinical trials to ensure a robust assessment of the observed results [ 1 ].
When is a sensitivity analysis valid?
While the importance of conducting sensitivity analysis has been widely acknowledged, what constitutes a valid sensitivity analysis has been unclear. To address this ambiguity, Morris et al. proposed a framework to conduct such analysis [ 2 ] and suggest that a particular analysis can be classified as a sensitivity analysis if it meets the following criteria: (1) the proposed analysis aims to answer the same question as to the primary analysis, (2) there is a possibility that the proposed analysis will lead to conclusions that differ from those of the primary analysis, and (3) there would be uncertainty as to which analysis to believe if the proposed analysis led to different conclusions than the primary analysis. These criteria can guide the conduct of sensitivity analysis and indicate what to consider when interpreting sensitivity analysis.
Criterion 1: do the sensitivity and primary analysis answer the same question?
The first criterion aims to ascertain whether the question being answered by the two analyses is the same. If the analysis addresses a different question than the primary question, then it should be referred to as a supplementary (or secondary) analysis. This may seem obvious, but it is important to consider, as if the questions being answered are different, the results could lead to unwarranted uncertainty regarding the robustness of the primary conclusions.
This misconception is commonly observed in trials where a primary analysis according to intention-to-treat (ITT) principle is followed by a per-protocol (PP) analysis, which many consider a sensitivity analysis. The ITT analysis considers the effect of a decision to treat regardless of if the treatment was received, while the PP analysis considers the effect of actually receiving treatment as intended. While the results of the PP analysis may be of value to certain stakeholders, the PP analysis is not a sensitivity analysis to a primary ITT analysis. Because the analyses address two distinct questions, it would not be surprising if the results differ. However, failure to appreciate that they ask different questions could lead to confusion over the robustness of the primary conclusions.
Criterion 2: could the sensitivity analysis yield different results than the primary analysis?
The second criterion relates to the assumptions made for the sensitivity analysis; if these assumptions will always lead to conclusions that are equivalent to the primary analysis, then we have learned nothing about the true sensitivity of the trial conclusion. Thus, a sensitivity analysis must be designed under a reasonable assumption that the findings could potentially differ from the primary analysis.
Consider the sensitivity analysis utilized in the LEAVO trial that assessed the effect of aflibercept and bevacizumab versus ranibizumab for patients with macular oedema secondary to central retinal vein occlusion [ 3 ]. The primary outcome of this study evaluated best-corrected visual acuity (BCVA) change from baseline for aflibercept, or bevacizumab, versus ranibizumab. At the end of the study, the primary outcome of the trial, BCVA score, was missing in some patients. For the purposes of imputation of the missing data, the investigators considered a range of values (from −20 to 20) as assumed values for the mean difference in BCVA scores between patients with observed and missing data. An example of this criterion not being met would be if a mean difference of 0 was used to impute BCVA scores for the missing patients, as it would be equivalent to re-running the primary analysis, leading to similar conclusions as to the primary analysis. This would provide a misleading belief in the robustness of results, as the “sensitivity” analysis conducted did not actually fulfill the appropriate criterion to be labeled as such.
On the other hand, modifying the assumptions to differ from the primary analysis by varying mean difference from −20 to 20 provides a useful analysis to assess the sensitivity of the primary analysis under a range of possible values that the missing participants may have had. One could reasonably postulate that assuming a mean change in BCVA scores of −20 to 20 to impute missing data could impact the primary analysis findings, as these values range from what one might consider a “best” and “worst” case scenario for the results observed among participants with missing data. In the LEAVO trial the authors demonstrated that, under these scenarios, the results of the sensitivity analysis support the primary conclusions of the trial.
Criterion 3: what should one believe if the sensitivity and primary analyses differ?
The third criterion assesses whether there would be uncertainty as to which analysis is to be believed if the proposed analysis leads to a different conclusion than the primary analysis. If one analysis will always be believed over another, then it is not worthwhile performing the analysis that will not be believed as it is impossible for that analysis to change our understanding of the outcome. Consider a trial in which an individual is randomized to intervention or control, and the primary outcome is measured for each eye. Because the results from each eye within a given patient are not independent, if researchers perform analyses both accounting for and not accounting for this dependence, it is clear that the analysis accounting for the dependence will be preferred. This is not a proper sensitivity analysis. In this situation, the analysis accounting for the dependence should be the primary analysis and the analysis not accounting for the dependence should not be performed, or be designated a secondary outcome.
Conclusions
Sensitivity analyses are important to perform in order to assess the robustness of the conclusions of the trial. It is critical to distinguish between sensitivity and supplementary or other analysis, and the above three criteria can inform an understanding of what constitutes a sensitivity analysis. Often, sensitivity analyses are underreported in published reports, making it difficult to assess whether appropriate sensitivity analyses were performed. We recommend that sensitivity analysis be considered a key part of any clinical trial SAP and be consistently and clearly reported with trial outcomes.
Food and Drug Administration. E9 (R1) statistical principles for clinical trials: addendum: estimands and sensitivity analysis in clinical trials. Guidance for Industry. May 2021.
Morris TP, Kahan BC, White IR. Choosing sensitivity analyses for randomised trials: principles. BMC Med Res Methodol. 2014;14:1–5. https://doi.org/10.1186/1471-2288-14-11 .
Article CAS Google Scholar
Hykin P, Prevost AT, Vasconcelos JC, Murphy C, Kelly J, Ramu J, et al. Clinical effectiveness of intravitreal therapy with ranibizumab vs aflibercept vs bevacizumab for macular edema secondary to central retinal vein occlusion: a randomized clinical trial. JAMA Ophthalmol. 2019;137:1256–64. https://doi.org/10.1001/jamaophthalmol.2019.3305 .
Article PubMed PubMed Central Google Scholar
Download references
Author information
Authors and affiliations.
Department of Oncology, McMaster University, Hamilton, ON, Canada
Sameer Parpia
Department of Health Research Methods, Evidence, and Impact, McMaster University, Hamilton, ON, Canada
Sameer Parpia, Mark R. Phillips, Lehana Thabane, Mohit Bhandari & Varun Chaudhary
MRC Clinical Trials Unit, University College London, London, UK
Tim P. Morris
Retina Consultants of Texas (Retina Consultants of America), Houston, TX, USA
Charles C. Wykoff
Blanton Eye Institute, Houston Methodist Hospital, Houston, TX, USA
Sunderland Eye Infirmary, Sunderland, UK
David H. Steel
Biosciences Institute, Newcastle University, Newcastle Upon Tyne, UK
Biostatistics Unit, St. Joseph’s Healthcare-Hamilton, Hamilton, ON, Canada
Lehana Thabane
Department of Surgery, McMaster University, Hamilton, ON, Canada
Mohit Bhandari & Varun Chaudhary
NIHR Moorfields Biomedical Research Centre, Moorfields Eye Hospital, London, UK
Sobha Sivaprasad
Cole Eye Institute, Cleveland Clinic, Cleveland, OH, USA
Peter Kaiser
Retinal Disorders and Ophthalmic Genetics, Stein Eye Institute, University of California, Los Angeles, CA, USA
David Sarraf
Department of Ophthalmology, Mayo Clinic, Rochester, MN, USA
Sophie J. Bakri
The Retina Service at Wills Eye Hospital, Philadelphia, PA, USA
Sunir J. Garg
Center for Ophthalmic Bioinformatics, Cole Eye Institute, Cleveland Clinic, Cleveland, OH, USA
Rishi P. Singh
Cleveland Clinic Lerner College of Medicine, Cleveland, OH, USA
Department of Ophthalmology, University of Bonn, Bonn, Germany
Frank G. Holz
Singapore Eye Research Institute, Singapore, Singapore
Tien Y. Wong
Singapore National Eye Centre, Duke-NUD Medical School, Singapore, Singapore
Centre for Eye Research Australia, Royal Victorian Eye and Ear Hospital, East Melbourne, VIC, Australia
Robyn H. Guymer
Department of Surgery (Ophthalmology), The University of Melbourne, Melbourne, VIC, Australia
You can also search for this author in PubMed Google Scholar
- Varun Chaudhary
- , Mohit Bhandari
- , Charles C. Wykoff
- , Sobha Sivaprasad
- , Lehana Thabane
- , Peter Kaiser
- , David Sarraf
- , Sophie J. Bakri
- , Sunir J. Garg
- , Rishi P. Singh
- , Frank G. Holz
- , Tien Y. Wong
- & Robyn H. Guymer
Contributions
SP was responsible for writing, critical review, and feedback on the manuscript. TPM was responsible for writing, critical review, and feedback on the manuscript. MRP was responsible for conception of idea, writing, critical review, and feedback on the manuscript. CCW was responsible for critical review and feedback on the manuscript. DHS was responsible for critical review and feedback on the manuscript. LT was responsible for critical review and feedback on the manuscript. MB was responsible for conception of idea, critical review, and feedback on the manuscript. VC was responsible for conception of idea, critical review, and feedback on the manuscript.
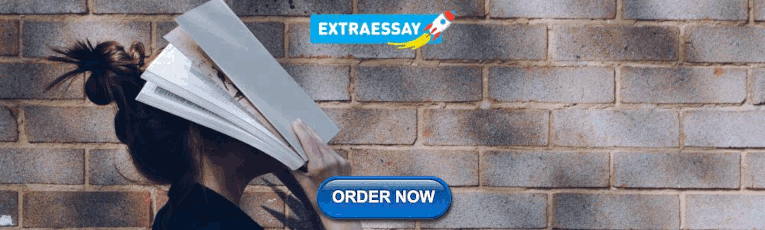
Corresponding author
Correspondence to Varun Chaudhary .
Ethics declarations
Competing interests.
SP: nothing to disclose. TPM: nothing to disclose. MRP: nothing to disclose. CCW: consultant: Acuela, Adverum Biotechnologies, Inc, Aerpio, Alimera Sciences, Allegro Ophthalmics, LLC, Allergan, Apellis Pharmaceuticals, Bayer AG, Chengdu Kanghong Pharmaceuticals Group Co, Ltd, Clearside Biomedical, DORC (Dutch Ophthalmic Research Center), EyePoint Pharmaceuticals, Gentech/Roche, GyroscopeTx, IVERIC bio, Kodiak Sciences Inc, Novartis AG, ONL Therapeutics, Oxurion NV, PolyPhotonix, Recens Medical, Regeneron Pharmaceuticals, Inc, REGENXBIO Inc, Santen Pharmaceutical Co, Ltd, and Takeda Pharmaceutical Company Limited; research funds: Adverum Biotechnologies, Inc, Aerie Pharmaceuticals, Inc, Aerpio, Alimera Sciences, Allergan, Apellis Pharmaceuticals, Chengdu Kanghong Pharmaceutical Group Co, Ltd, Clearside Biomedical, Gemini Therapeutics, Genentech/Roche, Graybug Vision, Inc, GyroscopeTx, Ionis Pharmaceuticals, IVERIC bio, Kodiak Sciences Inc, Neurotech LLC, Novartis AG, Opthea, Outlook Therapeutics, Inc, Recens Medical, Regeneron Pharmaceuticals, Inc, REGENXBIO Inc, Samsung Pharm Co, Ltd, Santen Pharmaceutical Co, Ltd, and Xbrane Biopharma AB—unrelated to this study. DHS: consultant: Gyroscope, Roche, Alcon, BVI; research funding for IIS: Alcon, Bayer, DORC, Gyroscope, Boehringer-Ingelheim—unrelated to this study. LT: nothing to disclose. MB: research funds: Pendopharm, Bioventus, Acumed—unrelated to this study. VC: advisory board member: Alcon, Roche, Bayer, Novartis; Grants: Bayer, Novartis—unrelated to this study.
Additional information
Publisher’s note Springer Nature remains neutral with regard to jurisdictional claims in published maps and institutional affiliations.
Rights and permissions
Reprints and permissions
About this article
Cite this article.
Parpia, S., Morris, T.P., Phillips, M.R. et al. Sensitivity analysis in clinical trials: three criteria for a valid sensitivity analysis. Eye 36 , 2073–2074 (2022). https://doi.org/10.1038/s41433-022-02108-0
Download citation
Received : 06 May 2022
Revised : 09 May 2022
Accepted : 12 May 2022
Published : 18 May 2022
Issue Date : November 2022
DOI : https://doi.org/10.1038/s41433-022-02108-0
Share this article
Anyone you share the following link with will be able to read this content:
Sorry, a shareable link is not currently available for this article.
Provided by the Springer Nature SharedIt content-sharing initiative
Quick links
- Explore articles by subject
- Guide to authors
- Editorial policies

A tutorial on sensitivity analyses in clinical trials: the what, why, when and how
Affiliation.
- 1 Department of Clinical Epidemiology and Biostatistics, McMaster University, Hamilton, ON, Canada. [email protected]
- PMID: 23855337
- PMCID: PMC3720188
- DOI: 10.1186/1471-2288-13-92
Background: Sensitivity analyses play a crucial role in assessing the robustness of the findings or conclusions based on primary analyses of data in clinical trials. They are a critical way to assess the impact, effect or influence of key assumptions or variations--such as different methods of analysis, definitions of outcomes, protocol deviations, missing data, and outliers--on the overall conclusions of a study.The current paper is the second in a series of tutorial-type manuscripts intended to discuss and clarify aspects related to key methodological issues in the design and analysis of clinical trials.
Discussion: In this paper we will provide a detailed exploration of the key aspects of sensitivity analyses including: 1) what sensitivity analyses are, why they are needed, and how often they are used in practice; 2) the different types of sensitivity analyses that one can do, with examples from the literature; 3) some frequently asked questions about sensitivity analyses; and 4) some suggestions on how to report the results of sensitivity analyses in clinical trials.
Summary: When reporting on a clinical trial, we recommend including planned or posthoc sensitivity analyses, the corresponding rationale and results along with the discussion of the consequences of these analyses on the overall findings of the study.
Publication types
- Research Support, Non-U.S. Gov't
- Clinical Trials as Topic / standards*
- Outcome and Process Assessment, Health Care
- Research Design
- Sensitivity and Specificity*

An official website of the United States government
The .gov means it's official. Federal government websites often end in .gov or .mil. Before sharing sensitive information, make sure you're on a federal government site.
The site is secure. The https:// ensures that you are connecting to the official website and that any information you provide is encrypted and transmitted securely.
- Publications
- Account settings
- Browse Titles
NCBI Bookshelf. A service of the National Library of Medicine, National Institutes of Health.
Velentgas P, Dreyer NA, Nourjah P, et al., editors. Developing a Protocol for Observational Comparative Effectiveness Research: A User's Guide. Rockville (MD): Agency for Healthcare Research and Quality (US); 2013 Jan.

Developing a Protocol for Observational Comparative Effectiveness Research: A User's Guide.
- Hardcopy Version at Agency for Healthcare Research and Quality
Chapter 11 Sensitivity Analysis
Joseph AC Delaney , PhD and John D Seeger , PharmD, DrPH.
This chapter provides an overview of study design and analytic assumptions made in observational comparative effectiveness research (CER), discusses assumptions that can be varied in a sensitivity analysis, and describes ways to implement a sensitivity analysis. All statistical models (and study results) are based on assumptions, and the validity of the inferences that can be drawn will often depend on the extent to which these assumptions are met. The recognized assumptions on which a study or model rests can be modified in order to assess the sensitivity, or consistency in terms of direction and magnitude, of an observed result to particular assumptions. In observational research, including much of comparative effectiveness research, the assumption that there are no unmeasured confounders is routinely made, and violation of this assumption may have the potential to invalidate an observed result. The analyst can also verify that study results are not particularly affected by reasonable variations in the definitions of the outcome/exposure. Even studies that are not sensitive to unmeasured confounding (such as randomized trials) may be sensitive to the proper specification of the statistical model. Analyses are available that can be used to estimate a study result in the presence of an hypothesized unmeasured confounder, which then can be compared to the original analysis to provide quantitative assessment of the robustness (i.e., “how much does the estimate change if we posit the existence of a confounder?”) of the original analysis to violations of the assumption of no unmeasured confounders. Finally, an analyst can examine whether specific subpopulations should be addressed in the results since the primary results may not generalize to all subpopulations if the biologic response or exposure may differ in these subgroups. The chapter concludes with a checklist of key considerations for including sensitivity analyses in a CER protocol or proposal.
- Introduction
Observational studies and statistical models rely on assumptions, which can range from how a variable is defined or summarized to how a statistical model is chosen and parameterized. Often these assumptions are reasonable and, even when violated, may result in unchanged effect estimates. When the results of analyses are consistent or unchanged by testing variations in underlying assumptions, they are said to be “robust.” However, violations in assumptions that result in meaningful effect estimate changes provide insight into the validity of the inferences that might be drawn from a study. A study's underlying assumptions can be altered along a number of dimensions, including study definitions (modifying exposure/outcome/confounder definitions), study design (changing or augmenting the data source or population under study), and modeling (modifying a variable's functional form or testing normality assumptions), to evaluate robustness of results.
This chapter considers the forms of sensitivity analysis that can be included in the analysis of an observational comparative effectiveness study, provides examples, and offers recommendations about the use of sensitivity analyses.
- Unmeasured Confounding and Study Definition Assumptions
Unmeasured Confounding
An underlying assumption of all epidemiological studies is that there is no unmeasured confounding, as unmeasured confounders cannot be accounted for in the analysis and including all confounders is a necessary condition for an unbiased estimate. Thus, inferences drawn from an epidemiologic study depend on this assumption. However, it is widely recognized that some potential confounding variables may not have been measured or available for analysis: the unmeasured confounding variable could either be a known confounder that is not present in the type of data being used (e.g., obesity is commonly not available in prescription claims databases) or an unknown confounder where the confounding relation is unsuspected. Quantifying the effect that an unmeasured confounding variable would have on study results provides an assessment of the sensitivity of the result to violations of the assumption of no unmeasured confounding. The robustness of an association to the presence of a confounder, 1 - 2 can alter inferences that might be drawn from a study, which then might change how the study results are used to influence translation into clinical or policy decisionmaking. Methods for assessing the potential impact of unmeasured confounding on study results, as well as quasi-experimental methods to account for unmeasured confounding, are discussed later in the chapter.
Comparison Groups
An important choice in study design is the selection of suitable treatment and comparison groups. This step can serve to address many potential limitations of a study, such as how new user cohorts eliminate the survivor bias that may be present if current (prevalent) users are studied. (Current users would reflect only people who could tolerate the treatment and, most likely, for whom treatment appeared to be effective). 3 However, this “new user” approach can limit the questions that can be asked in a study, as excluding prevalent users might omit long-term users (which could overlook risks that arise over long periods of use). For example, when Rietbrock et al. considered the comparative effectiveness of warfarin and aspirin in atrial fibrillation 4 in the General Practice Research Database, they looked at current use and past use instead of new use. This is a sensible strategy in a general practice setting as these medications may be started long before the patient is diagnosed with atrial fibrillation. Yet, as these medications may be used for decades, long-term users are of great interest. In this study, the authors used past use to address indication, by comparing current users to past users (an important step in a “prevalent users” study).
One approach is to include several different comparison groups and use the observed differences in potential biases with the different comparison groups as a way to assess the robustness of the results. For example, when studying the association between thiazide diuretics and diabetes, one could create reference groups including “nonusers,” “recent past users,” “distant past users,” and “users of other antihypertensive medications.” One would presume that the risk of incident diabetes among the “distant past users” should resemble that of the “nonusers”; if not, there is a possibility that confounding by indication is the reason for the difference in risk.
Exposure Definitions
Establishing a time window that appropriately captures exposure during etiologically relevant time periods can present a challenge in study design when decisions need to be made in the presence of uncertainty. 5 Uncertainty about the most appropriate way to define drug exposure can lead to questions about what would have happened if the exposure had been defined a different way. A substantially different exposure-outcome association observed under different definitions of exposure (such as different time windows or dose [e.g., either daily or cumulative]) might provide insight into the biological mechanisms underlying the association or provide clues about potential confounding or unaddressed bias. As such, varying the exposure definition and re-analyzing under different definitions serves as a form of sensitivity analysis.
Outcome Definitions
The association between exposure and outcome can also be assessed under different definitions of the outcome. Often a clinically relevant outcome in a data source can be ascertained in several ways (e.g., a single diagnosis code, multiple diagnosis codes, a combination of diagnosis and procedure codes). The analysis can be repeated using these different definitions of the outcome, which may shed light on the how well the original outcome definition truly reflects the condition of interest.
Beyond varying a single outcome definition, it is also possible to evaluate the association between the exposure and clinically different outcomes. If the association between the exposure and one clinical outcome is known from a study with strong validity (such as from a clinical trial) and can be reproduced in the study, the observed association between the exposure of interest and an outcome about which external data are not available becomes more credible. Since some outcomes might not be expected to occur immediately after exposure (e.g., cancer), the study could employ different lag (induction) periods between exposure and the first outcomes to be analyzed in order to assess the sensitivity of the result to the definition. This result can lead either to insight into potential unaddressed bias or confounding, or it could be used as a basis for discussion about etiology (e.g., does the outcome have a long onset period?).
Covariate Definitions
Covariate definitions can also be modified to assess how well they address confounding in the analysis. Although a minimum set of covariates may be used to address confounding, there may be an advantage to using a staged approach where groups of covariates are introduced, leading to progressively greater adjustment. If done transparently, this approach may provide insight into which covariates have relatively greater influences on effect estimates, permitting comparison with known or expected associations or permitting the identification of possible intermediate variables.
Finally, some covariates are known to be misclassified under some approaches. A classic example is an “intention to treat” analysis that assumes that each participant continues to be exposed once they have received an initial treatment. Originally used in the analysis of randomized trials, this approach has been used in observational studies as well. 6 It can be worthwhile to do a sensitivity analysis on studies that use an “intention to treat” approach to see how different an “as treated” analysis would be even if intention to treat is the main estimate of interest, mostly in cases where there is differential adherence in the data source between two therapeutic approaches. 7
Summary Variables
Study results can also be affected by the summarization of variables. For example, time can be summarized, and differences in the time window during which exposure is determined can lead to changes in study effect estimates. For example, the risk of venous thromboembolism rises with duration of use for oral contraceptives; 8 an exposure definition that did not consider the cumulative exposure to the medication might underestimate the difference in risk between two different formulations of oral contraceptive. Alternately, effect estimates may vary with changes in the outcome definition. For example, an outcome definition of all cardiovascular events including angina could lead to a different effect estimate than an outcome definition including only myocardial infarction. Sensitivity analyses of the outcome definition can allow for a richer understanding of the data, even for models based on data from a randomized controlled trial.
Selection Bias
The assessment of selection bias through sensitivity analysis involves assumptions regarding inclusion or participation by potential subjects, and results can be highly sensitive to assumptions. For example, the oversampling of cases exposed to one of the drugs under study (or, similarly, an undersampling) can lead to substantial changes in effect measures over ranges that might plausibly be evaluated. Even with external validation data, which may work for unmeasured confounders, 9 it is difficult to account for more than a trivial amount of selection bias. Generally, if there is strong evidence of selection bias in a particular data set it is best to seek out alternative data sources.
One limited exception may be when the magnitude of bias is known to be small. 10 This may be true for nonrandom loss to followup in a patient cohort. Since the baseline characteristics of the cohort are known, it is possible to make reasonable assumptions about how influential this bias can be. But, in the absence of such information, it is generally better to focus on identifying and eliminating selection bias at the data acquisition or study design stage.
- Data Source, Subpopulations, and Analytic Methods
The first section of this chapter covered traditional sensitivity analysis to test basic assumptions such as variable definitions and to consider the impact of an unmeasured confounder. These issues should be considered in every observational study of comparative effectiveness research. However, there are some additional sensitivity analyses that should be considered, depending on the nature of the epidemiological question and the data available. Not every analysis can (or should) consider these factors, but they can be as important as the more traditional sensitivity analysis approaches.
Data Source
For many comparative effectiveness studies, the data used for the analysis were not specifically collected for the purpose of the research question. Instead, the data may have been obtained as part of routine care or for administrative purposes such as medical billing. In such cases, it may be possible to acquire multiple data sources for a single analysis (and use the additional data sources as a sensitivity analysis). Where this is not feasible, it may be possible to consider differences between study results and results obtained from other papers that use different data sources.
While all data sources have inherent limitations in terms of the data that are captured by the database, these limitations can be accentuated when the data were not prospectively collected for the specific research purpose. 11 For example, secondary use of data increases the chances that a known but unmeasured confounder may explain part or all of an observed association. A straightforward example of the differences in data capture can be seen by comparing data from Medicare (i.e., U.S. medical claims data) and the General Practice Research Database (i.e., British electronic medical records collected as part of routine care). 11 Historically, Medicare data have lacked the results of routine laboratory testing and measurement (quantities like height, weight, blood pressure, and glucose measures), but include detailed reporting on hospitalizations (which are billed and thus well recorded in a claims database). In a similar sense, historically, the General Practice Research Database has had weaker reporting on hospitalizations (since this information is captured only as reports given back to the General Practice, that usually are less detailed), but better recording than Medicare data for routine measurements (such as blood pressure) that are done as part of a standard medical visit.
Issues with measurement error can also emerge because of the process by which data are collected. For example, “myocardial infarction” coded for the purposes of billing may vary slightly or substantially from a clinically verified outcome of myocardial infarction. As such, there will be an inevitable introduction of misclassification into the associations. Replicating associations in different data sources (e.g., comparing a report to a general practitioner [GP] with a hospital ICD-9 code) can provide an idea of how changes to the operational definition of an outcome can alter the estimates. Replication of a study using different data sources is more important for less objectively clear outcomes (such as depression) than it is for more objectively clear outcomes (such as all-cause mortality).
An analysis conducted in a single data source may be vulnerable to bias due to systematic measurement error or the omission of a key confounding variable. Associations that can be replicated in a variety of data sources, each of which may have used different definitions for recording information and which have different covariates available, provide reassurance that the results are not simply due to the unavailability of an important confounding variable in a specific data set. Furthermore, when estimating the possible effect of an unmeasured confounder on study results, data sets that measure the confounder may provide good estimates of the confounder's association with exposure and outcome (and provide context for results in data sources without the same confounder information).
An alternative to looking at completely separate datasets is to consider supplementing the available data with additional information from external data sources. An example of a study that took the approach of supplementing data was conducted by Huybrechts et al. 12 They looked at the comparative safety of typical and atypical antipsychotics among nursing home residents. The main analysis used prescription claims (Medicare and Medicaid data) and found, using high-dimensional propensity score adjustment, that conventional antipsychotics were associated with an increase in 180-day mortality risk (a risk difference of 7.0 per 100 persons [95% CI: 5.8, 8.2]). The authors then included data from MDS (Minimum Data Set) and OSCAR (Online Survey, Certification and Reporting), which contains clinical covariates and nursing home characteristics. 12 The result of including these variables was an essentially identical estimate of 7.1 per 100 people (95% CI: 5.9, 8.2). 12 This showed that these differences were robust to the addition of these additional covariates. It did not rule out other potential biases, but it did demonstrate that simply adding MDS and OSCAR data would not change statistical inference.
While replicating results across data sources provides numerous benefits in terms of understanding the robustness of the association and reducing the likelihood of a chance finding, it is often a luxury that is not available for a research question, and inferences may need to be drawn from the data source at hand.
Key Subpopulations
Therapies are often tested on an ideal population (e.g., uncomplicated patients thought to be likely to adhere to medication) in clinical trials. Once the benefit is clearly established in trials, the therapy is approved for use and becomes available to all patients. However, there are several cases where it is possible that the effectiveness of specific therapies can be subject to effect measure modification. While a key subpopulation may be independently specified as a population of interest, showing that results are homogeneous across important subpopulations can build confidence in applying the results uniformly to all subpopulations. Alternatively, it may highlight the presence of effect measure modification and the need to comment on population heterogeneity in the interpretation of results. As part of the analysis plan, it is important to state whether measures of effect will be estimated within these or other subpopulations present in the research sample in order to assess possible effect measure modification:
Pediatric populations . Children may respond differently to therapy from adults, and dosing may be more complicated. Looking at children as a separate and important sub-group may make sense if a therapy is likely to be used in children.
Genetic variability . The issue of genetic variability is often handled only by looking at different ethnic or racial groups (who are presumed to have different allele frequencies). Some medications may be less effective in some populations due to the different polymorphisms that are present in these persons, though indicators of race and ethnicity are only surrogates for genetic variation.
Complex patients . These are patients who suffer from multiple disease states at once. These disease states (or the treatment[s] for these disease states) may interfere with each other, resulting in a different optimal treatment strategy in these patients. A classic example is the treatment of cardiovascular disease in HIV-infected patients. The drug therapy used to treat the HIV infection may interfere with medication intended to treat cardiovascular disease. Treatment of these complex patients is of great concern to clinicians, and these patients should be considered separately where sample size considerations allow for this.
Older adults . Older adults are another population that may have more drug side effects and worse outcomes from surgeries and devices. Furthermore, older adults are inherently more likely to be subject to polypharmacy and thus have a much higher risk of drug-drug interactions.
Most studies lack the power to look at all of these different populations, nor are they all likely to be present in a single data source. However, when it is feasible to do so, it can be useful to explore these subpopulations to determine if the overall associations persist or if the best choice of therapy is population dependent. These can be important clues in determining how stable associations are likely to be across key subpopulations. In particular, the researcher should identify segments of the population for which there are concerns about generalizing results. For example, randomized trials of heart failure often exclude large portions of the patient population due to the complexity of the underlying disease state. 13 It is critical to try to include inferences to these complex subpopulations when doing comparative effectiveness research with heart failure as the study outcome, as that is precisely where the evidence gap is the greatest.
Cohort Definition and Statistical Approaches
If it is possible to do so, it can also be extremely useful to consider the use of more than one cohort definition or statistical approach to ensure that the effect estimate is robust to the assumptions behind these approaches. There are several options to consider as alternative analysis approaches.
Samy Suissa illustrated how the choice of cohort definition can affect effect estimates in his paper on immortal time bias. 14 He considered five different approaches to defining a cohort, with person time incorrectly allocated (causing immortal time bias) and then repeated these analyses with person time correctly allocated (giving correct estimates). Even in this straightforward example, the corrected hazard ratios varied from 0.91 to 1.13 depending on the cohort definition. There were five cohort definitions used to analyze the use of antithrombotic medication and the time to death from lung cancer: time-based cohort, event-based cohort, exposure-based cohort, multiple-event–based cohort, and event-exposure–based cohort. These cohorts produce hazard ratios of 1.13, 1.02, 1.05, 0.91, and 0.95, respectively. While this may not seem like an extreme difference in results, it does illustrate the value of using varying assumptions to hone in on an understanding of the stability of the associations under study with different analytical approaches, as in this example where point estimates varied by about +/- 10% depending in how the cohort was defined.
One can also consider the method of covariate adjustment to see if it might result in changes in the effect estimates. One option to consider as an adjunct analysis is the use of a high-dimensional propensity score, 15 as this approach is typically applicable to the same data upon which a conventional regression analysis is performed. The high-dimensional propensity score is well suited to handling situations in which there are multiple weak confounding variables. This is a common situation in many claims database contexts, where numerous variables can be found that are associated (perhaps weakly) with drug exposure, and these same variables may be markers for (i.e., associated with) unmeasured confounders. Each variable may represent a weak marker for an unmeasured confounder, but collectively (such as through the high-dimensional propensity score approach) their inclusion can reduce confounding from this source. This kind of propensity score approach is a good method for validating the results of conventional regression models.
Another option that can be used, when the data permit it, is an instrumental variable (IV) analysis to assess the extent of bias due to unmeasured confounding (see chapter 10 for a detailed discussion of IV analysis). 16 While there have been criticisms that use of instruments such as physician or institutional preference may have assumptions that are difficult to verify and may increase the variance of the estimates, 17 an instrumental variable analysis has the potential to account for unmeasured confounding factors (which is a key advantage), and traditional approaches also have unverifiable assumptions. Also, estimators resulting from the IV analysis may differ from main analysis estimators (see Supplement, “Improving Characterization of Study Populations: The Identification Problem”), and investigators should ensure correct interpretation of results using this approach.
Examples of Sensitivity Analysis of Analytic Methods
Sensitivity analysis approaches to varying analytic methods have been used to build confidence in results. One example is a study by Schneeweiss et al. 18 of the effectiveness of aminocaproic acid compared with aprotinin for the reduction of surgical mortality during coronary-artery bypass grafting (CABG). In this study, the authors demonstrated that three separate analytic approaches (traditional regression, propensity score, and physician preference instrumental variable analyses) all showed an excess risk of death among the patients treated with aprotinin (estimates ranged from a relative risk of 1.32 authors demonstrated that three separate analytic approaches (traditional regression, propensity score, and physician preference instrumental variable analyses) all showed an excess risk of death among the patients treated with aprotinin (estimates ranged from a relative risk of 1.32 [propensity score] to a relative risk of 1.64 [traditional regression analysis]). Showing that different approaches, each of which used different assumptions, all demonstrated concordant results was further evidence that this association was robust.
Sometimes a sensitivity analysis can reveal a key weakness in a particular approach to a statistical problem. Delaney et al. 19 looked at the use of case-crossover designs to estimate the association between warfarin use and bleeding in the General Practice Research Database. They compared the case-crossover results to the case-time-control design, the nested case control design, and to the results of a meta-analysis of randomized controlled trials. The case-crossover approach, where individuals serve as their own controls, showed results that differed from other analytic approaches. For example, the case-crossover design with a lagged control window (a control window that is placed back one year) estimated a rate ratio of 1.3 (95% CI: 1.0, 1.7) compared with a rate ratios of 1.9 for the nested case-control design, 1.7 for the case-time-control design and 2.2 for a meta-analysis of clinical trials. 18 Furthermore, the results showed a strong dependence on the length of the exposure window (ranging from a rate ratio of 1.0 to 3.6), regardless of overall time on treatment. These results provided evidence that results from a case-crossover approach in this particular situation needed a cautious interpretation, as different approaches were estimating incompatible magnitudes of association, were not compatible with the estimates from trials, and likely violated an assumption of the case-crossover approach (transient exposure). Unlike the Schneeweiss et al. example, 18 for which the results were consistent across analytic approaches, divergent results require careful consideration of which approach is the most appropriate (given the assumptions made) for drawing inferences, and investigators should provide a justification for the determination in the discussion.
Sometimes the reasons for differential findings with differences in approach can be obvious (e.g., concerns over the appropriateness of the case-crossover approach, in the Delaney et al. example above). 19 In other cases, differences can be small and the focus can be on the overall direction of the inference (like in the Suissa example above). 14 Finally, there can be cases where two different approaches (e.g., an IV approach and a conventional analysis) yield different inferences and it can be unclear which one is correct. In such a case, it is important to highlight these differences, and to try to determine which set of assumptions makes sense in the structure of the specific problem.
Table 11.1 Study aspects that can be evaluated through sensitivity analysis
View in own window
- Statistical Assumptions
The guidance in this section focuses primarily on studies with a continuous outcome, exposure, or confounding factor variable. Many pharmacoepidemiological studies are conducted within a claims database environment where the number of continuous variables is limited (often only age is available), and these assumptions do not apply in these settings. However, studies set in electronic medical records or in prospective cohort studies may have a wider range of continuous variables, and it is important to ensure that they are modeled correctly.
Covariate and Outcome Distributions
It is common to enter continuous parameters as linear covariates in a final model (whether that model is linear, logistic, or survival). However, there are many variables where the association with the outcome may be better represented as a transformation of the original variable.
A good example of such a variable is net personal income, a variable that is bounded at zero but for which there may be a large number of plausible values. The marginal effect of a dollar of income may not be linear across the entire range of observed incomes (an increase of $5,000 may mean more to individuals with a base income of $10,000 than those with a base income of $100,000). As a result, it can make sense to look at transformations of the data into a more meaningful scale.
The most common option for transforming a continuous variable is to create categories (e.g., quintiles derived from the data set or specific cut points). This approach has the advantages of simplicity and transparency, as well as being relatively nonparametric. However, unless the cut points have clinical meaning, they can make studies difficult to compare with one another (as each study may have different cut points). Furthermore, transforming a continuous variable into a discrete form always results in loss of information that is better to avoid if possible. Another option is to consider transforming the variable to see if this influences the final results. The precise choice of transformation requires knowledge of the distribution of the covariate. For confounding factors, it can be helpful to test several transformations and to see the impact of the reduction in skewness, and to decide whether a linear approximation remains appropriate.
Functional Form
The “functional form” is the assumed mathematical association between variables in a statistical model. There are numerous potential variations in functional form that can be the subject of a sensitivity analysis. Examples include the degree of polynomial expressions, splines, or additive rather than multiplicative joint effects of covariates in the prediction of both exposures and outcomes. In all of these cases, the “functional form” is the assumed mathematical association between variables, and sensitivity analyses can be employed to evaluate the effect of different assumptions. In cases where nonlinearity is suspected (i.e., a nonlinear relationship between a dependent and independent variable in a model), it can be useful to test the addition of a square term to the model (i.e., the pair of covariates age + age 2 as the functional form of the independent variable age). If this check does not influence the estimate of the association, then it is unlikely that there is any important degree of nonlinearity. If there is an impact on the estimates for this sort of transformation, it can make sense to try a more appropriate model for the nonlinear variable (such as a spline or a generalized additive model).
Transformations should be used with caution when looking at the primary exposure, as they can be susceptible to overfit. Overfit occurs when you are fitting a model to random variations in the data (i.e., noise) rather than to the underlying relation; polynomial-based models are susceptible to this sort of problem. However, if one is assessing the association between a drug and an outcome, this can be a useful way to handle parameters (like age) that will not be directly used for inference but that one wishes to balance between two exposure groups. These transformations should also be considered as possibilities in the creation of a probability of treatment model (for a propensity score analysis). If overfit of a key parameter that is to be used for inference is of serious concern, then there are analytic approaches (like dividing the data into a training and validation data set) that can be used to reduce the amount of overfit. However, these data mining techniques are beyond the scope of this chapter.
Special Cases
Another modeling challenge for epidemiologic analysis and interpretation is when there is a mixture of informative null values (zeroes) and a distribution. This occurs with variables like coronary artery calcium (CAC), which can have values of zero or a number of Agatston units. 20 These distributions are best modeled as two parts: (1) as a dichotomous variable to determine the presence or absence of CAC; and (2) using a model to determine the severity of CAC among those with CAC>0. In the specific case of CAC, the severity model is typically log-transformed due to extreme skew. 20 These sorts of distributions are rare, but one should still consider the distribution and functional form of key continuous variables when they are available.
- Implementation Approaches
There are a number of approaches to conducting sensitivity analyses. This section describes two widely used approaches, spreadsheet-based and code-based analyses. It is not intended to be a comprehensive guide to implementing sensitivity analyses. Other approaches to conducting sensitivity analysis exist and may be more useful for specific problems. 2
Spreadsheet-Based Analysis
The robustness of a study result to an unmeasured confounding variable can be assessed quantitatively using a standard spreadsheet. 21 The observed result and ranges of assumptions about an unmeasured confounder (prevalence, strength of association with exposure, and strength of association with outcome) are entered into the spreadsheet, and are used to provide the departure from the observed result to be expected if the unmeasured confounding variable could be accounted for using standard formulae for confounding. 22 Two approaches are available within the spreadsheet: (1) an “array” approach; and (2) a “rule-out” approach. In the array approach, an array of values (representing the ranges of assumed values for the unmeasured variable) is the input for the spreadsheet. The resulting output is a three-dimensional plot that illustrates, through a graphed response surface, the observed result for a constellation of assumptions (within the input ranges) about the unmeasured confounder.
In the rule-out approach, the observed association and characteristics of the unmeasured confounder (prevalence and strength of association with both exposure and outcome) are entered into the spreadsheet. The resulting output is a two-dimensional graph that plots, given the observed association, the ranges of unmeasured confounder characteristics that would result in a null finding. In simpler terms, the rule-out approach quantifies, given assumptions, how strong a measured confounder would need to be to result in a finding of no association and “rules out” whether an unmeasured confounder can explain the observed association.
Statistical Software–Based Analysis
For some of the approaches discussed, the software is available online. For example, the high-dimensional propensity score and related documentation is available at http://www.hdpharmacoepi.org/download/ . For other approaches, like the case-crossover design,18 the technique is well known and widely available. Finally, many of the most important forms of sensitivity analysis require data management tasks (such as recoding the length of an exposure time window) that are straightforward though time consuming.
This section provides a few examples of how slightly more complex functional forms of covariates (where the association is not well described by a line or by the log transformation of a line) can be handled. The first example introduces a spline into a model where the analyst suspects that there might be a nonlinear association with age (and where there is a broad age range in the cohort that makes a linearity assumption suspect). The second example looks at how to model CAC, which is an outcome variable with a complex form.
Example of Functional Form Analysis
This SAS code is an example of a mixed model that is being used to model the trajectory of a biomarker over time (variable=years), conditional on a number of covariates. The example estimates the association between different statin medications with this biomarker. Like in many prescription claims databases, most of the covariates are dichotomous. However, there is a concern that age may not be linearly associated with outcome, so a version of the analysis is tried in which a spline is used in place of a standard age variable.
Original Analysis (SAS 9.2):
Sensitivity Analysis:
While the spline version of the age variable needs to be graphically interpreted, it should handle any nonlinear association between age and the biomarker of interest.
Example of Two-Stage Models for Coronary Artery Calcium (CAC)
CAC is an example of a continuous variable with an extremely complex form. The examples of two-stage CAC modeling (below) use variables from the Multi-Ethnic Study of Atherosclerosis. Here, the example is testing whether different forms of nonsteroidal anti-inflammatory drugs (below as asa1c, nsaid1c, cox21c) are associated with more or less calcification of the arteries. The model needs to be done in two stages, as it is thought that the covariates that predict the initiation of calcification may differ from those that predict how quickly calcification progresses once the process has begun. 20
First, a model is developed for the relative risk of having a CAC score greater than zero (i.e., that there is at least some evidence of plaques in a CT scan of the participant's coronary arteries). The variable for CAC is cac (1=CAC present, 0=CAC not present). The repeated statement is used to invoke robust confidence intervals (as there is only one subject for each unique participant ID number, designated as the variable idno).
SAS 9.2 code example:
Among those participants with CAC (as measured by an Agatston score, agatpm1c), greater than zero, the amount present is then modeled. As this variable is highly skewed, the amount of CAC present is transformed using a log transformation.
The modeling of CAC is a good example of one of the more complicated continuous variables that can be encountered in CER. 20 To properly model this association, two models were needed (and the second model required transformation of the exposure). Most comparative effectiveness projects will involve much simpler outcome variables, and the analyst should be careful to include more complex models only where there is an important scientific rationale.
- Presentation
Often sensitivity analyses conducted for a specific CER study can simply be summarized in the text of the paper, especially if the number of scenarios is small. 17 In other cases, where a broad range of scenarios are tested, 2 it may be more informative to display analyses in tabular or graphical form.
Tabular Presentation
The classic approach to presenting sensitivity analysis results is a table. There, the author can look at the results of different assumptions and/or population subgroups. Tables are usually preferred in cases where there is minimal information being presented, as they allow the reader to very precisely determine the influence of changes in assumptions on the reported associations. This is the approach used by Suissa 14 to show differences in results based on different approaches to analyzing a cohort of lung cancer patients.
Graphical Presentation
One reason to use graphical methods is that the variable being modeled is itself a continuous variable, and presenting the full plot is more informative than forcing a categorization scheme on the data. One example, from Robyn McClelland and colleagues ( Figure 11.1 ), 23 is a sensitivity analysis to see if the form in which alcohol is consumed changes its association with levels of CAC. The analyst, therefore, plots the association with total alcohol consumed overall and by type of alcohol (beer, wine, hard alcohol). Here, both the exposure and the outcome are continuous variables, and so it is much easier to present the results of the sensitivity analysis as a series of plots.
Figure 11.1
Smoothed plot of alcohol consumption versus annualized progression of CAC with 95% CIs. See McClelland RL, Bild DE, Burke GL, et al. Alcohol and coronary artery calcium prevalence, incidence, and progression: results from the Multi-Ethnic Study of Atherosclerosis (more...)
Another reason for a graphical display is to present the conditions that a confounder would need to meet in order to be able to explain an association. As discussed, the strength of a confounder depends on its association with the exposure, the outcome, and its prevalence in the population. Using the standard spreadsheet discussed earlier, 20 these conditions can be represented as a plot. For example, Figure 11.2 presents a plot based on data from Psaty et al. 1 , 24
Figure 11.2
Plot to assess the strength of unmeasured confounding necessary to explain an observed association.
Figure 11.2 plots the combination of the odds ratio between the exposure and the confounder (OREC) and the relative risk between the confounder and the outcome (RRCD) that would be required to explain an observed association between the exposure and the outcome by confounding alone. There are two levels of association considered (ARR=1.57 and ARR=1.3) and a separate line plotted for each. These sorts of displays can help illustrate the strength of unmeasured confounding that is required to explain observed associations, which can make the process of identifying possible candidate confounders easier (as one can reference other studies from other populations in order to assess the plausibility of the assumed strength of association). Spreadsheets that facilitate the conduct of these sensitivity analyses are available. ( http://www.drugepi.org/dope-downloads/#Sensitivity Analysis )
Other tools for sensitivity analysis are available, such as the one from Lash et al. ( http://sites.google.com/site/biasanalysis/ ). 10
While sensitivity analyses are important, it is necessary to balance the concise reporting of study results with the benefits of including of the results of numerous sensitivity analyses. In general, one should highlight sensitivity analyses that result in important changes or that show that an analysis is robust to changes in assumptions. Furthermore, one should ensure that the number of analyses presented is appropriate for illustrating how the model responds to these changes. For example, if looking at the sensitivity of results to changes in the exposure time window, consider looking at 30, 60, and 90 days instead of 15, 30, 45, 60, 75, 90, 105, and 120 days, unless the latter list directly illustrates an important property of the statistical model. The decision as to what are the most important sensitivity analyses to run will always be inherently specific to the problem under study.
For example, a comparative effectiveness study of two devices might not be amenable to variations in exposure window definitions, but might be a perfect case for a physician preference instrumental variable. This chapter highlights the most common elements for consideration in sensitivity analysis, but some degree of judgment as to the prioritization of these analyses for presentation is required. Still as a general guideline, the analyst should be able to answer three questions:
- Is the association robust to changes in exposure definition, outcome definition, and the functional form of these variables?
- How strong would an unmeasured confounder have to be to explain the magnitude of the difference between two treatments?
- Does the choice of statistical method influence the directionality or strength of the association?
A plan for including some key sensitivity analysis in developing study protocols and analysis plans should be formed with a clear awareness of the limitations of the data and the nature of the problem. The plan should be able to answer these three basic questions and should be a key feature of any comparative effectiveness analysis. The use of sensitivity analysis to examine the underlying assumptions in the analysis process will build confidence as to the robustness of associations to assumptions and be a crucial component of grading the strength of evidence provided by a study.
Checklist: Guidance and key considerations for sensitivity analyses in an observational CER protocol
Developing a Protocol for Observational Comparative Effectiveness Research: A User’s Guide is copyrighted by the Agency for Healthcare Research and Quality (AHRQ). The product and its contents may be used and incorporated into other materials on the following three conditions: (1) the contents are not changed in any way (including covers and front matter), (2) no fee is charged by the reproducer of the product or its contents for its use, and (3) the user obtains permission from the copyright holders identified therein for materials noted as copyrighted by others. The product may not be sold for profit or incorporated into any profitmaking venture without the expressed written permission of AHRQ.
- Cite this Page Delaney JAC, Seeger JD. Sensitivity Analysis. In: Velentgas P, Dreyer NA, Nourjah P, et al., editors. Developing a Protocol for Observational Comparative Effectiveness Research: A User's Guide. Rockville (MD): Agency for Healthcare Research and Quality (US); 2013 Jan. Chapter 11.
- PDF version of this title (5.8M)
In this Page
Other titles in these collections.
- AHRQ Methods for Effective Health Care
- Health Services/Technology Assessment Text (HSTAT)
Related information
- PMC PubMed Central citations
- PubMed Links to PubMed
Recent Activity
- Sensitivity Analysis - Developing a Protocol for Observational Comparative Effec... Sensitivity Analysis - Developing a Protocol for Observational Comparative Effectiveness Research: A User's Guide
Your browsing activity is empty.
Activity recording is turned off.
Turn recording back on
Connect with NLM
National Library of Medicine 8600 Rockville Pike Bethesda, MD 20894
Web Policies FOIA HHS Vulnerability Disclosure
Help Accessibility Careers
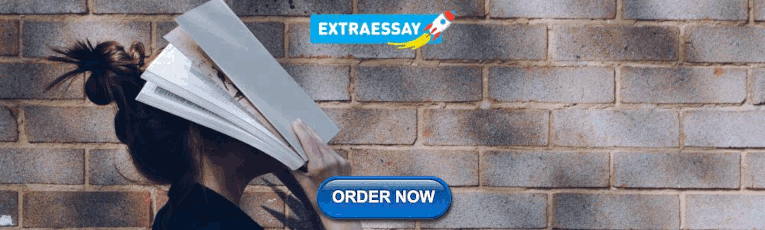
IMAGES
VIDEO
COMMENTS
What is a sensitivity analysis in clinical research? Sensitivity Analysis (SA) is defined as “a method to determine the robustness of an assessment by examining the extent to which results are affected by changes in methods, models, values of unmeasured variables, or assumptions” with the aim of identifying “results that are most ...
A sensitivity analysis consists of repeating the estimation of µ at different plausible values of α so as to assess the sensitivity of inferences about µ to assumptions about the missing data mechanism as encoded by α and model (6).
Sensitivity analysis is the study of how the uncertainty in the output of a mathematical model or system (numerical or otherwise) can be divided and allocated to different sources of uncertainty in its inputs. [1][2] This involves estimating sensitivity indices that quantify the influence of an input or group of inputs on the output.
We provide three examples to showcase how sensitivity analyses can be used across multiple phases of the research process; missing data (data cleaning and screening), clustered data (statistical analysis), and meta-analysis (data synthesis).
Sensitivity analysis examines the robustness of the result by conducting the analyses under a range of plausible assumptions about the methods, models, or data that differ from the...
Sensitivity analysis is useful in assessing how robust an association is to potential unmeasured or uncontrolled confounding. This article introduces a new measure called the "E-value," which is related to the evidence for causality in observational studies that are potentially subject to confoundin ….
Background: Sensitivity analyses play a crucial role in assessing the robustness of the findings or conclusions based on primary analyses of data in clinical trials. They are a critical way to assess the impact, effect or influence of key assumptions or variations--such as different methods of analysis, definitions of outcomes, protocol ...
Analyses are available that can be used to estimate a study result in the presence of an hypothesized unmeasured confounder, which then can be compared to the original analysis to provide quantitative assessment of the robustness (i.e., “how much does the estimate change if we posit the existence of a confounder?”) of the original analysis to vi...
Sensitivity analyses are purposed to gain insight and certainty about the validity of research findings reported. Reporting guidelines and health research methodologists have emphasized the importance of utilizing and reporting sensitivity analyses in clinical research.
A sensitivity analysis is a method to determine the robustness of trial findings by examining the extent to which results are affected by changes in methods, models, values of unmeasured variables, or assumptions. The goal of a sensitivity analysis is to identify results that are most dependent on questionable or unsupported assumptions.