- Write my thesis
- Thesis writers
- Buy thesis papers
- Bachelor thesis
- Master's thesis
- Thesis editing services
- Thesis proofreading services
- Buy a thesis online
- Write my dissertation
- Dissertation proposal help
- Pay for dissertation
- Custom dissertation
- Dissertation help online
- Buy dissertation online
- Cheap dissertation
- Dissertation editing services
- Write my research paper
- Buy research paper online
- Pay for research paper
- Research paper help
- Order research paper
- Custom research paper
- Cheap research paper
- Research papers for sale
- Thesis subjects
- How It Works
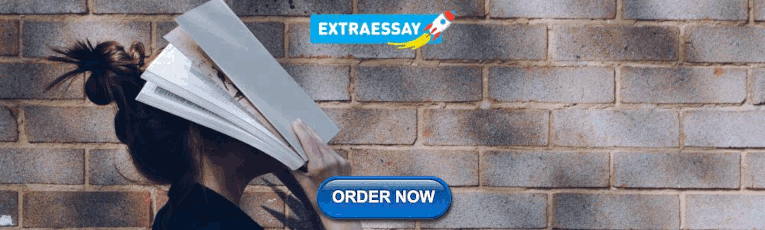
120 Statistical Research Topics: Explore Up-to-date Trends
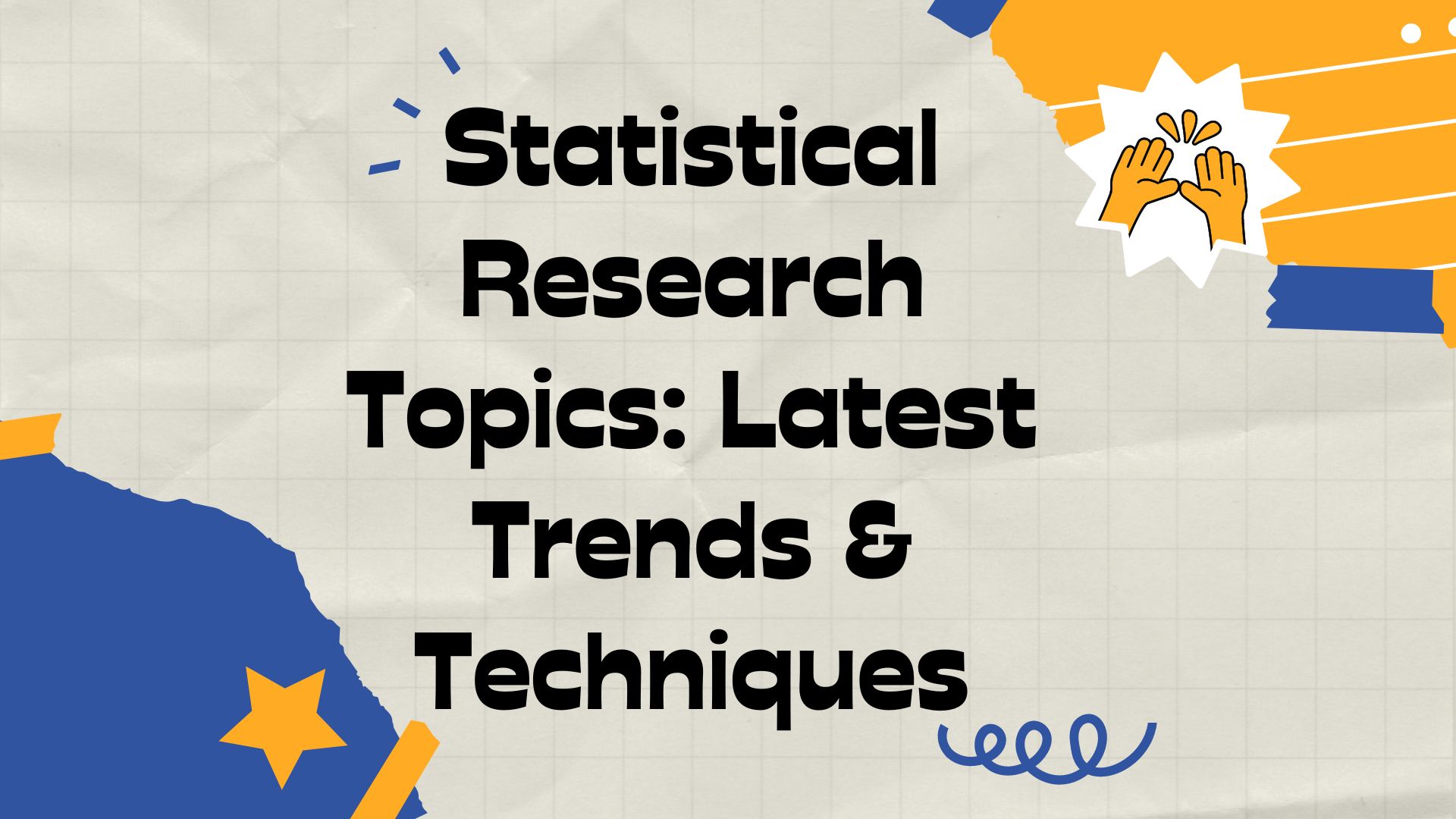
Researchers and statistics teachers are often tasked with writing an article or paper on a given stats project idea. One of the most crucial things in writing an outstanding and well-composed statistics research project, paper, or essay is to come up with a very interesting topic that will captivate your reader’s minds and provoke their thoughts.
What Are the Best Statistical Research Topics Worth Writing On?
Leading statistical research topics for college students that will interest you, project topics in statistics worth considering, the best idea for statistics project you can focus on, good experiments for statistics topics you should be writing on, what are the best ap statistics project ideas that will be of keen interest to you, good statistics project ideas suitable for our modern world, some of the most crucial survey topics for statistics project, statistical projects topics every researcher wants to write on, statistical research topics you can focus your research on.
Students often find it difficult to come up with well-composed statistical research project topics that take the format of argumentative essay topics to pass across their message. In this essay, we will look at some of the most interesting statistics research topics to focus your research on.
Here are some of the best statistical research topics worth writing on:
- Predictive Healthcare Modeling with Machine Learning
- Analyzing Online Education During COVID-19 Epidemic
- Modeling How Climate Change Affects Natural Disasters
- Essential Elements Influencing Personnel Productivity
- Social Media Influence on Customer Choices and Behavior
- Can Geographical Statistics Aid In Analyzing Crime Trends and Patterns?
- Financial Markets and Stock Price Predictions
- Statistical Analysis of Voting-related Behaviors
- An Analysis of Public Transportation Usage Trends in Urban Areas
- How Can Public Health Education Reduce Air Pollution?
- Statistical Analysis of Suicide In Adolescents and Adults
- A Review of Divorce and How It Affects Children
As a college student, here are the best statistical projects for high school students to focus your research on, especially if you need social media research topics .
- Major Factors Influencing College Students’ Academic Performance
- Social Media and How It Defines thee Mental Health of Students
- Evaluation of the Elements Influencing Student Engagement and Retention
- An Examination of Extracurricular Activities On Academic Success
- Does Parental Involvement Determine Academic Achievement of Kids?
- Examining How Technology Affects Improving Educational Performance
- Factors That Motivate Students’ Involvement In Online Learning
- The Impact of Socioeconomic Status On Academic Performance
- Does Criticism Enhance Student Performance?
- Student-Centered Learning and Improved Performance
- A Cursory Look At Students’ Career Goals and Major Life Decisions
- Does Mental Health Impact Academic Achievement?
Are you a student tasked with writing a project but can’t come up with befitting stats research topics? Here are the best ideas for statistical projects worth considering:
- Financial Data And Stock Price Forecasting
- Investigation of Variables Influencing Students’ Grades
- What Causes Traffic Flow and Congestion In Urban Areas?
- How to Guarantee Customer Retention In the Retail Sector
- Using Epidemiological Data to Model the Spread of Infectious Diseases
- Does Direct Advertisement Affect Consumer Preferences and Behavior?
- How to Predict and Adapt to Climate Change
- Using Spatial Statistics to Analyze Trends and Patterns In Crime
- Examination of the Elements Influencing Workplace Morale and Productivity
- Understanding User Behavior and Preferences Through Statistical Analysis of Social Media Data
- How Many Percent Get Married After Their Degree Programs?
- A Comparative Analysis of Different Academic Fee Payments
If you have been confused based on the availability of different statistics project topics to choose from, here are some of the best thesis statement about social media to choose from:
- Analysis of the Variables Affecting A Startup’s Success
- The Valid Connection Between Mental Health and Social Media Use
- Different Teaching Strategies and Academic Performance
- Factors Influencing Employee Satisfaction In Different Work Environments
- The Impact of Public Policy On Different Population Groups
- Reviewing Different Health Outcomes and Incomes
- Different Marketing Tactics for Good Service Promotion
- What Influences Results In Different Sports Competitions?
- Differentiating Elements Affecting Students’ Performance In A Given Subject
- Internal Communication and Building An Effective Workplace
- Does the Use of Business Technologies Boost Workers’ Output?
- The Role of Modern Communication In An Effective Company Management
Are you a student tasked with writing an essay on social issues research topics but having challenges coming up with a topic? Here are some amazing statistical experiments ideas you can center your research on.
- How Global Pandemic Affects Local Businesses
- Investigating the Link Between Income and Health Outcomes In a Demography
- Key Motivators for Student’s Performance In a Particular Academic Program
- Evaluating the Success of a Promotional Plan Over Others
- Continuous Social Media Use and Impact On Mental Health
- Does Culture Impact the Religious Beliefs of Certain Groups?
- Key Indicators of War and How to Manage These Indicators
- An Overview of War As a Money Laundering Scheme
- How Implementations Guarantee Effectiveness of Laws In Rural Areas
- Performance of Students In War-torn Areas
- Key Indicators For Measuring the Success of Your Venture
- How Providing FAQs Can Help a Business Scale
The best AP statistic project ideas every student especially those interested in research topics for STEM students will want to write in include:
- The Most Affected Age Demography By the Covid-19 Pandemic
- The Health Outcomes Peculiar to a Specific Demography
- Unusual Ways to Enhance Student Performance In a Classroom
- How Marketing Efforts Can Determine Promotional Outputs
- Can Mental Health Solutions Be Provided On Social Media?
- Assessing How Certain Species Are Affected By Climate Change.
- What Influences Voter Turnouts In Different Elections?
- How Many People Have Used Physical Exercises to Improve Mental Health
- How Financial Circumstances Can Determine Criminal Activities
- Ways DUI Laws Can Reduce Road Accidents
- Examining the Connection Between Corruption and Underdevelopment In Africa
- What Key Elements Do Top Global Firms Engage for Success?
If you need some of the best economics research paper topics , here are the best statistics experiment ideas you can write research on:
- Retail Client Behaviors and Weather Trends
- The Impact of Marketing Initiatives On Sales and Customer Retention
- How Socioeconomic Factors Determine Crime Rates In Different Locations
- Public and Private School Students: Who Performs Better?
- How Fitness Affects the Mental Health of People In Different Ages
- Focus On the Unbanked Employees Globally
- Does Getting Involve In a Kid’s Life Make Them Better?
- Dietary Decisions and a Healthy Life
- Managing Diabetes and High Blood Pressure of a Specific Group
- How to Engage Different Learning Methods for Effectiveness
- Understudying the Sleeping Habits of Specific Age Groups
- How the Numbers Can Help You Create a Brand Recognition
As a student who needs fresh ideas relating to the topic for a statistics project to write on, here are crucial survey topics for statistics that will interest you.
- Understanding Consumer Spending and Behavior In Different Regions
- Why Some People in Certain Areas Live Longer than Others
- Comparative Analysis of Different Customer Behaviors
- Do Social Media Businesses Benefit More than Physical Businesses?
- Does a Healthy Work Environment Guarantee Productivity?
- The Impact of Ethnicity and Religion On Voting Patterns
- Does Financial Literacy Guarantee Better Money Management?
- Cultural Identities and Behavioral Patterns
- How Religious Orientation Determines Social Media Use
- The Growing Need for Economists Globally
- Getting Started with Businesses On Social Media
- Which Is Better: A 9-5 or An Entrepreneurial Job?
Do you want to write on unique statistical experiment ideas? Here are some topics you do not want to miss out on:
- Consumer Satisfaction-Related Variables on E-Commerce Websites
- Obesity Rates and Socioeconomic Status In Developed Countries
- How Marketing Strategies Can Make or Mar Sales Performance
- The Correlation Between Increased Income and Happiness In Various Nations
- Regression Models and Forecasting Home Prices
- Climate Change Affecting Agricultural Production In Specific Areas
- A Study of Employee Satisfaction In the Healthcare Industry
- Social Media, Marketing Tactics, and Consumer Behavior In the Fashion Industry
- Predicting the Risk of Default Among Credit Card Holders In Different Regions
- Why Crime Rates Are Increasing In Urban Areas than Rural Areas
- Statistical Evaluation of Methamphetamine’s Impact On Drug Users
- Genes and a Child’s Total Immunity
Here are some of the most carefully selected stat research topics you can focus on.
- Social Media’s Effects On Consumer Behavior
- The Correlation Between Urban Crime Rates and Poverty Levels
- Physical Exercise and Mental Health Consequences
- Predictive Modeling In the Financial Markets
- How Minimum Wage Regulations Impact Employment Rates
- Healthcare Outcomes and Access Across Various Socioeconomic Groups
- How High School Students’ Environment Affect Academic Performance
- Automated Technology and Employment Loss
- Environmental Elements and Their Effects On Public Health
- Various Advertising Tactics and How They Influence Customer Behavior
- Political Polarization And Economic Inequality
- Climate Change and Agricultural Productivity
The above statistics final project examples will stimulate your curiosity and test your abilities, and they can even be linked to some biochemistry topics and anatomy research paper topics . Writing about these statistics project ideas helps provide a deeper grasp of the natural and social phenomena that affect our lives and the environment by studying these subjects.
Leave a Reply Cancel reply
- Buy Custom Assignment
- Custom College Papers
- Buy Dissertation
- Buy Research Papers
- Buy Custom Term Papers
- Cheap Custom Term Papers
- Custom Courseworks
- Custom Thesis Papers
- Custom Expository Essays
- Custom Plagiarism Check
- Cheap Custom Essay
- Custom Argumentative Essays
- Custom Case Study
- Custom Annotated Bibliography
- Custom Book Report
- How It Works
- Writing Guides
- +1 (888) 398 0091
- Essay Samples
- Essay Topics
- Research Topics
- Writing Tips
Statistics Research Topics: Ideas & Questions
June 16, 2023
Looking for research topics in statistics? Whether you’re a student working on a class project or a researcher in need of inspiration, finding the right topic can be challenging. With numerous areas to explore in statistics, narrowing down your options can be overwhelming. But with some creativity and research, you can find an interesting and relevant topic. This article offers ideas and examples of statistics research topics to consider, so let’s dive in!
Statistics Research: What It Comprises
The data collected by statistics research can be quantitative (numbers) or qualitative (text). The data can also be presented in tables or graphs for easy understanding by the audience. However, it is not always necessary to present the data in the form of tables or graphs, as sometimes the raw data can be good enough to convey the message from the researcher.
In statistics projects, the researchers usually design experiments to test specific hypotheses about a population’s characteristics or behavior. For example, suppose you want to know whether people who wear glasses will have better eyesight than those who don’t wear glasses. In that case, you need to collect information about their vision before and after wearing glasses (experimental group) and compare their vision with those who do not wear glasses (control group). You would then find out whether there was any difference between these two groups with respect to eyesight improvement due to wearing glasses.
Tips on How to Choose a Statistics Research Topic
Firstly, remember that a good statistics topic should interest you and also have a substantial amount of data available for analysis. Once you have decided on your topic, you can collect data for your study using secondary sources or conducting primary research through surveys or interviews.
You can also use search engines like Google or Yahoo! to find information about your topic of interest. You can use keywords like “income disparity” or “inequality causes” to find relevant websites on which you can find information related to your topic of interest.
Next, consider what types of questions your supervisor would like answered with this data type. For example, if you’re looking at crime rates in your city, maybe they would like to know which areas have higher crime rates than others to plan police patrols accordingly. Or maybe they just want to know whether there’s any correlation between high crime rates and low-income neighborhoods (there probably will be).
Feel free to select any topic and try our free AI essay generator to craft your essay.
Statistics Research Topics in Business
- Understanding the factors that influence consumer purchase decisions in the technology industry
- Advertising and sales revenue: a time-series analysis
- The effectiveness of customer loyalty programs in increasing customer retention and revenue
- The relationship between employee job satisfaction and productivity
- The factors that contribute to employee turnover in the hospitality industry
- Product quality on customer satisfaction and loyalty: a longitudinal study
- The application of social media marketing in increasing brand awareness and customer engagement
- Corporate social responsibility (CSR) initiatives and brand reputation: a meta-analysis
- Understanding the factors that influence customer satisfaction in the restaurant industry
- E-commerce on traditional brick-and-mortar retail sales: a comparative analysis
- The effectiveness of supply chain management strategies in reducing operational costs and improving efficiency
- The relationship between market competition and innovation: a cross-country analysis
- Understanding the factors that influence employee motivation and engagement in the workplace
- Business analytics on strategic decision-making: a case study approach
- The effectiveness of performance-based incentives in increasing employee productivity and job satisfaction
- Organizational performance dependence on employee diversity and organizational performance
- Understanding the factors that contribute to startup success in the technology industry
- The impact of pricing strategies on sales revenue and profitability
- The effectiveness of corporate training programs in improving employee skill development and performance
- The relationship between brand image and customer loyalty
Research Topics in Applied Statistics
- The impact of educational attainment on income level
- The effectiveness of different advertising strategies in increasing sales
- The relationship between socioeconomic status and health outcomes
- The effectiveness of different teaching methods in promoting academic success
- The impact of job training programs on employment rates
- The relationship between crime rates and community demographics
- Different medication dosages in treating a particular condition
- The influence of environmental pollutants on health outcomes
- The interconnection between access to healthcare and health outcomes
- The effectiveness of different weight loss programs in promoting weight loss
- The impact of social support on mental health outcomes
- The relationship between demographic factors and political affiliation
- The effectiveness of different exercise programs in promoting physical fitness
- The influence of parenting styles on child behavior
- The relationship between diet and chronic disease risk
- Different smoking cessation programs for promoting smoking cessation
- The impact of public transportation on urban development
- The relationship between technology usage and social isolation
- The effectiveness of different stress reduction techniques in reducing stress levels
- The influence of climate change on crop
Statistics Research Topics in Psychology
- The correlation between childhood trauma and adult depression
- The effectiveness of cognitive-behavioral therapy in treating anxiety disorders
- The impact of social media on self-esteem and body image in adolescents
- Personality traits and job satisfaction: how are they related?
- The prevalence and predictors of bullying in schools
- The effects of sleep deprivation on cognitive performance
- The role of parenting styles in the development of emotional intelligence
- The effectiveness of mindfulness-based interventions in reducing stress and anxiety
- The impact of childhood abuse on adult relationship satisfaction
- The influence of social support on coping with chronic illness
- The factors that contribute to successful aging
- The prevalence and predictors of addiction relapse
- The impact of cultural factors on mental health diagnosis and treatment
- Exercise and mental health: in which way are they connected?
- The effectiveness of art therapy in treating trauma-related disorders
- The prevalence and predictors of eating disorders in college students
- The influence of attachment styles on romantic relationships
- The effectiveness of group therapy in treating substance abuse disorders
- The prevalence and predictors of postpartum depression
- The impact of childhood socioeconomic
Sports Statistics Research Topics
- The relationship between player performance and team success in the National Football League (NFL)
- Understanding the factors that influence home-field advantage in professional soccer
- The impact of game-day weather conditions on player performance in Major League Baseball (MLB)
- The effectiveness of different training regimens in improving endurance and performance in long-distance running
- The relationship between athlete injury history and future injury risk in professional basketball
- The impact of crowd noise on team performance in college football
- The effectiveness of sports psychology interventions in improving athlete performance and mental health
- The relationship between player height and success in professional basketball: a regression analysis
- Understanding the factors that contribute to the development of youth soccer players in the United States
- The influence of playing surface on injury rates in professional football: a longitudinal study
- The effectiveness of pre-game routines in improving athlete performance in tennis
- The relationship between athletic ability and academic success among college athletes
- Understanding the factors that influence injury risk and recovery time in professional hockey players
- The impact of in-game statistics on coaching decisions in professional basketball
- The effectiveness of different dietary regimens in improving athlete performance in endurance sports
- The relationship between athlete sleep habits and performance: a longitudinal study
- Understanding the factors that influence athlete endorsement deals and sponsorships in professional sports
- The influence of stadium design on crowd noise levels and player performance in college football
- The effectiveness of different strength training regimens in improving athlete performance in track and field events
- The relationship between player salary and team success in professional baseball: a longitudinal analysis
Survey Methods Statistics Research Topics
- Understanding the factors that influence response rates in online surveys
- The effectiveness of different survey question formats in eliciting accurate and reliable responses
- The relationship between survey mode (phone, online, mail) and response quality in political polling
- The impact of incentives on survey response rates and data quality
- Understanding the factors that contribute to respondent satisfaction in surveys
- The effectiveness of different sampling methods in achieving representative samples in survey research
- The relationship between survey item order and response bias: a meta-analysis
- The impact of social desirability bias on survey responses: a longitudinal study
- Understanding the factors that influence survey question wording and response bias
- The effectiveness of different visual aids in improving respondent comprehension and response quality
- The relationship between survey timing and response rate: a comparative analysis
- The impact of interviewer characteristics on survey response quality in face-to-face surveys
- Understanding the factors that contribute to nonresponse bias in survey research
- The effectiveness of different response scales in measuring attitudes and perceptions in surveys
- The relationship between survey length and respondent engagement: a cross-sectional analysis
- The influence of skip patterns on survey response quality and completion rates
- Understanding the factors that influence survey item nonresponse and item refusal rates
- The effectiveness of pre-testing and piloting surveys in improving data quality and reliability
- The relationship between survey administration and response quality: a comparative analysis of phone, online, and in-person surveys
- The impact of survey fatigue on response quality and data completeness: a longitudinal study
As mentioned above, statistics is the science of collecting and analyzing data to draw conclusions and make predictions. To conduct a proper statistical analysis, you must first define your research question, gather data from various sources, analyze the information, and draw conclusions based on the results.
This process can be challenging for many people who do not have an extensive background in statistics. However, it does not have to be so tricky if you use our professional Custom Writing help. Our writers are highly qualified professionals who will work with you to develop a clear understanding of your research problem and then guide you through every step of the process. We will also ensure that your paper follows all academic standards to meet all requirements for originality and quality.
Sociology Research Topics Ideas
Importance of Computer in Nursing Practice Essay
History Research Paper Topics For Students
By clicking “Continue”, you agree to our terms of service and privacy policy. We’ll occasionally send you promo and account related emails.
Latest Articles
Debating in class or composing a persuasive paper is a fruitful intellectual practice. Doing so, participants and writers are expected...
Most students wonder whether it is possible to cite an article in an essay. The answer is “Yes”! Why not...
Let us explain what is what and how it can be used. An anthology is a published collection of poems...
I want to feel as happy, as your customers do, so I'd better order now
We use cookies on our website to give you the most relevant experience by remembering your preferences and repeat visits. By clicking “Accept All”, you consent to the use of ALL the cookies. However, you may visit "Cookie Settings" to provide a controlled consent.
Have a language expert improve your writing
Run a free plagiarism check in 10 minutes, generate accurate citations for free.
- Knowledge Base
The Beginner's Guide to Statistical Analysis | 5 Steps & Examples
Statistical analysis means investigating trends, patterns, and relationships using quantitative data . It is an important research tool used by scientists, governments, businesses, and other organizations.
To draw valid conclusions, statistical analysis requires careful planning from the very start of the research process . You need to specify your hypotheses and make decisions about your research design, sample size, and sampling procedure.
After collecting data from your sample, you can organize and summarize the data using descriptive statistics . Then, you can use inferential statistics to formally test hypotheses and make estimates about the population. Finally, you can interpret and generalize your findings.
This article is a practical introduction to statistical analysis for students and researchers. We’ll walk you through the steps using two research examples. The first investigates a potential cause-and-effect relationship, while the second investigates a potential correlation between variables.
Table of contents
Step 1: write your hypotheses and plan your research design, step 2: collect data from a sample, step 3: summarize your data with descriptive statistics, step 4: test hypotheses or make estimates with inferential statistics, step 5: interpret your results, other interesting articles.
To collect valid data for statistical analysis, you first need to specify your hypotheses and plan out your research design.
Writing statistical hypotheses
The goal of research is often to investigate a relationship between variables within a population . You start with a prediction, and use statistical analysis to test that prediction.
A statistical hypothesis is a formal way of writing a prediction about a population. Every research prediction is rephrased into null and alternative hypotheses that can be tested using sample data.
While the null hypothesis always predicts no effect or no relationship between variables, the alternative hypothesis states your research prediction of an effect or relationship.
- Null hypothesis: A 5-minute meditation exercise will have no effect on math test scores in teenagers.
- Alternative hypothesis: A 5-minute meditation exercise will improve math test scores in teenagers.
- Null hypothesis: Parental income and GPA have no relationship with each other in college students.
- Alternative hypothesis: Parental income and GPA are positively correlated in college students.
Planning your research design
A research design is your overall strategy for data collection and analysis. It determines the statistical tests you can use to test your hypothesis later on.
First, decide whether your research will use a descriptive, correlational, or experimental design. Experiments directly influence variables, whereas descriptive and correlational studies only measure variables.
- In an experimental design , you can assess a cause-and-effect relationship (e.g., the effect of meditation on test scores) using statistical tests of comparison or regression.
- In a correlational design , you can explore relationships between variables (e.g., parental income and GPA) without any assumption of causality using correlation coefficients and significance tests.
- In a descriptive design , you can study the characteristics of a population or phenomenon (e.g., the prevalence of anxiety in U.S. college students) using statistical tests to draw inferences from sample data.
Your research design also concerns whether you’ll compare participants at the group level or individual level, or both.
- In a between-subjects design , you compare the group-level outcomes of participants who have been exposed to different treatments (e.g., those who performed a meditation exercise vs those who didn’t).
- In a within-subjects design , you compare repeated measures from participants who have participated in all treatments of a study (e.g., scores from before and after performing a meditation exercise).
- In a mixed (factorial) design , one variable is altered between subjects and another is altered within subjects (e.g., pretest and posttest scores from participants who either did or didn’t do a meditation exercise).
- Experimental
- Correlational
First, you’ll take baseline test scores from participants. Then, your participants will undergo a 5-minute meditation exercise. Finally, you’ll record participants’ scores from a second math test.
In this experiment, the independent variable is the 5-minute meditation exercise, and the dependent variable is the math test score from before and after the intervention. Example: Correlational research design In a correlational study, you test whether there is a relationship between parental income and GPA in graduating college students. To collect your data, you will ask participants to fill in a survey and self-report their parents’ incomes and their own GPA.
Measuring variables
When planning a research design, you should operationalize your variables and decide exactly how you will measure them.
For statistical analysis, it’s important to consider the level of measurement of your variables, which tells you what kind of data they contain:
- Categorical data represents groupings. These may be nominal (e.g., gender) or ordinal (e.g. level of language ability).
- Quantitative data represents amounts. These may be on an interval scale (e.g. test score) or a ratio scale (e.g. age).
Many variables can be measured at different levels of precision. For example, age data can be quantitative (8 years old) or categorical (young). If a variable is coded numerically (e.g., level of agreement from 1–5), it doesn’t automatically mean that it’s quantitative instead of categorical.
Identifying the measurement level is important for choosing appropriate statistics and hypothesis tests. For example, you can calculate a mean score with quantitative data, but not with categorical data.
In a research study, along with measures of your variables of interest, you’ll often collect data on relevant participant characteristics.
Variable | Type of data |
---|---|
Age | Quantitative (ratio) |
Gender | Categorical (nominal) |
Race or ethnicity | Categorical (nominal) |
Baseline test scores | Quantitative (interval) |
Final test scores | Quantitative (interval) |
Parental income | Quantitative (ratio) |
---|---|
GPA | Quantitative (interval) |
Prevent plagiarism. Run a free check.
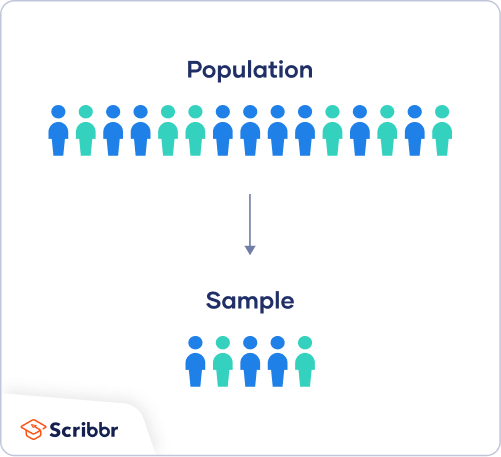
In most cases, it’s too difficult or expensive to collect data from every member of the population you’re interested in studying. Instead, you’ll collect data from a sample.
Statistical analysis allows you to apply your findings beyond your own sample as long as you use appropriate sampling procedures . You should aim for a sample that is representative of the population.
Sampling for statistical analysis
There are two main approaches to selecting a sample.
- Probability sampling: every member of the population has a chance of being selected for the study through random selection.
- Non-probability sampling: some members of the population are more likely than others to be selected for the study because of criteria such as convenience or voluntary self-selection.
In theory, for highly generalizable findings, you should use a probability sampling method. Random selection reduces several types of research bias , like sampling bias , and ensures that data from your sample is actually typical of the population. Parametric tests can be used to make strong statistical inferences when data are collected using probability sampling.
But in practice, it’s rarely possible to gather the ideal sample. While non-probability samples are more likely to at risk for biases like self-selection bias , they are much easier to recruit and collect data from. Non-parametric tests are more appropriate for non-probability samples, but they result in weaker inferences about the population.
If you want to use parametric tests for non-probability samples, you have to make the case that:
- your sample is representative of the population you’re generalizing your findings to.
- your sample lacks systematic bias.
Keep in mind that external validity means that you can only generalize your conclusions to others who share the characteristics of your sample. For instance, results from Western, Educated, Industrialized, Rich and Democratic samples (e.g., college students in the US) aren’t automatically applicable to all non-WEIRD populations.
If you apply parametric tests to data from non-probability samples, be sure to elaborate on the limitations of how far your results can be generalized in your discussion section .
Create an appropriate sampling procedure
Based on the resources available for your research, decide on how you’ll recruit participants.
- Will you have resources to advertise your study widely, including outside of your university setting?
- Will you have the means to recruit a diverse sample that represents a broad population?
- Do you have time to contact and follow up with members of hard-to-reach groups?
Your participants are self-selected by their schools. Although you’re using a non-probability sample, you aim for a diverse and representative sample. Example: Sampling (correlational study) Your main population of interest is male college students in the US. Using social media advertising, you recruit senior-year male college students from a smaller subpopulation: seven universities in the Boston area.
Calculate sufficient sample size
Before recruiting participants, decide on your sample size either by looking at other studies in your field or using statistics. A sample that’s too small may be unrepresentative of the sample, while a sample that’s too large will be more costly than necessary.
There are many sample size calculators online. Different formulas are used depending on whether you have subgroups or how rigorous your study should be (e.g., in clinical research). As a rule of thumb, a minimum of 30 units or more per subgroup is necessary.
To use these calculators, you have to understand and input these key components:
- Significance level (alpha): the risk of rejecting a true null hypothesis that you are willing to take, usually set at 5%.
- Statistical power : the probability of your study detecting an effect of a certain size if there is one, usually 80% or higher.
- Expected effect size : a standardized indication of how large the expected result of your study will be, usually based on other similar studies.
- Population standard deviation: an estimate of the population parameter based on a previous study or a pilot study of your own.
Once you’ve collected all of your data, you can inspect them and calculate descriptive statistics that summarize them.
Inspect your data
There are various ways to inspect your data, including the following:
- Organizing data from each variable in frequency distribution tables .
- Displaying data from a key variable in a bar chart to view the distribution of responses.
- Visualizing the relationship between two variables using a scatter plot .
By visualizing your data in tables and graphs, you can assess whether your data follow a skewed or normal distribution and whether there are any outliers or missing data.
A normal distribution means that your data are symmetrically distributed around a center where most values lie, with the values tapering off at the tail ends.
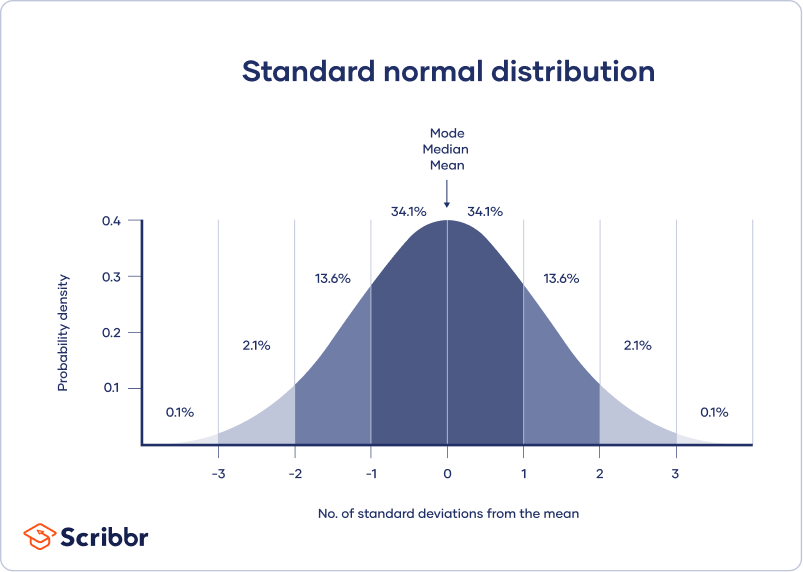
In contrast, a skewed distribution is asymmetric and has more values on one end than the other. The shape of the distribution is important to keep in mind because only some descriptive statistics should be used with skewed distributions.
Extreme outliers can also produce misleading statistics, so you may need a systematic approach to dealing with these values.
Calculate measures of central tendency
Measures of central tendency describe where most of the values in a data set lie. Three main measures of central tendency are often reported:
- Mode : the most popular response or value in the data set.
- Median : the value in the exact middle of the data set when ordered from low to high.
- Mean : the sum of all values divided by the number of values.
However, depending on the shape of the distribution and level of measurement, only one or two of these measures may be appropriate. For example, many demographic characteristics can only be described using the mode or proportions, while a variable like reaction time may not have a mode at all.
Calculate measures of variability
Measures of variability tell you how spread out the values in a data set are. Four main measures of variability are often reported:
- Range : the highest value minus the lowest value of the data set.
- Interquartile range : the range of the middle half of the data set.
- Standard deviation : the average distance between each value in your data set and the mean.
- Variance : the square of the standard deviation.
Once again, the shape of the distribution and level of measurement should guide your choice of variability statistics. The interquartile range is the best measure for skewed distributions, while standard deviation and variance provide the best information for normal distributions.
Using your table, you should check whether the units of the descriptive statistics are comparable for pretest and posttest scores. For example, are the variance levels similar across the groups? Are there any extreme values? If there are, you may need to identify and remove extreme outliers in your data set or transform your data before performing a statistical test.
Pretest scores | Posttest scores | |
---|---|---|
Mean | 68.44 | 75.25 |
Standard deviation | 9.43 | 9.88 |
Variance | 88.96 | 97.96 |
Range | 36.25 | 45.12 |
30 |
From this table, we can see that the mean score increased after the meditation exercise, and the variances of the two scores are comparable. Next, we can perform a statistical test to find out if this improvement in test scores is statistically significant in the population. Example: Descriptive statistics (correlational study) After collecting data from 653 students, you tabulate descriptive statistics for annual parental income and GPA.
It’s important to check whether you have a broad range of data points. If you don’t, your data may be skewed towards some groups more than others (e.g., high academic achievers), and only limited inferences can be made about a relationship.
Parental income (USD) | GPA | |
---|---|---|
Mean | 62,100 | 3.12 |
Standard deviation | 15,000 | 0.45 |
Variance | 225,000,000 | 0.16 |
Range | 8,000–378,000 | 2.64–4.00 |
653 |
A number that describes a sample is called a statistic , while a number describing a population is called a parameter . Using inferential statistics , you can make conclusions about population parameters based on sample statistics.
Researchers often use two main methods (simultaneously) to make inferences in statistics.
- Estimation: calculating population parameters based on sample statistics.
- Hypothesis testing: a formal process for testing research predictions about the population using samples.
You can make two types of estimates of population parameters from sample statistics:
- A point estimate : a value that represents your best guess of the exact parameter.
- An interval estimate : a range of values that represent your best guess of where the parameter lies.
If your aim is to infer and report population characteristics from sample data, it’s best to use both point and interval estimates in your paper.
You can consider a sample statistic a point estimate for the population parameter when you have a representative sample (e.g., in a wide public opinion poll, the proportion of a sample that supports the current government is taken as the population proportion of government supporters).
There’s always error involved in estimation, so you should also provide a confidence interval as an interval estimate to show the variability around a point estimate.
A confidence interval uses the standard error and the z score from the standard normal distribution to convey where you’d generally expect to find the population parameter most of the time.
Hypothesis testing
Using data from a sample, you can test hypotheses about relationships between variables in the population. Hypothesis testing starts with the assumption that the null hypothesis is true in the population, and you use statistical tests to assess whether the null hypothesis can be rejected or not.
Statistical tests determine where your sample data would lie on an expected distribution of sample data if the null hypothesis were true. These tests give two main outputs:
- A test statistic tells you how much your data differs from the null hypothesis of the test.
- A p value tells you the likelihood of obtaining your results if the null hypothesis is actually true in the population.
Statistical tests come in three main varieties:
- Comparison tests assess group differences in outcomes.
- Regression tests assess cause-and-effect relationships between variables.
- Correlation tests assess relationships between variables without assuming causation.
Your choice of statistical test depends on your research questions, research design, sampling method, and data characteristics.
Parametric tests
Parametric tests make powerful inferences about the population based on sample data. But to use them, some assumptions must be met, and only some types of variables can be used. If your data violate these assumptions, you can perform appropriate data transformations or use alternative non-parametric tests instead.
A regression models the extent to which changes in a predictor variable results in changes in outcome variable(s).
- A simple linear regression includes one predictor variable and one outcome variable.
- A multiple linear regression includes two or more predictor variables and one outcome variable.
Comparison tests usually compare the means of groups. These may be the means of different groups within a sample (e.g., a treatment and control group), the means of one sample group taken at different times (e.g., pretest and posttest scores), or a sample mean and a population mean.
- A t test is for exactly 1 or 2 groups when the sample is small (30 or less).
- A z test is for exactly 1 or 2 groups when the sample is large.
- An ANOVA is for 3 or more groups.
The z and t tests have subtypes based on the number and types of samples and the hypotheses:
- If you have only one sample that you want to compare to a population mean, use a one-sample test .
- If you have paired measurements (within-subjects design), use a dependent (paired) samples test .
- If you have completely separate measurements from two unmatched groups (between-subjects design), use an independent (unpaired) samples test .
- If you expect a difference between groups in a specific direction, use a one-tailed test .
- If you don’t have any expectations for the direction of a difference between groups, use a two-tailed test .
The only parametric correlation test is Pearson’s r . The correlation coefficient ( r ) tells you the strength of a linear relationship between two quantitative variables.
However, to test whether the correlation in the sample is strong enough to be important in the population, you also need to perform a significance test of the correlation coefficient, usually a t test, to obtain a p value. This test uses your sample size to calculate how much the correlation coefficient differs from zero in the population.
You use a dependent-samples, one-tailed t test to assess whether the meditation exercise significantly improved math test scores. The test gives you:
- a t value (test statistic) of 3.00
- a p value of 0.0028
Although Pearson’s r is a test statistic, it doesn’t tell you anything about how significant the correlation is in the population. You also need to test whether this sample correlation coefficient is large enough to demonstrate a correlation in the population.
A t test can also determine how significantly a correlation coefficient differs from zero based on sample size. Since you expect a positive correlation between parental income and GPA, you use a one-sample, one-tailed t test. The t test gives you:
- a t value of 3.08
- a p value of 0.001
Receive feedback on language, structure, and formatting
Professional editors proofread and edit your paper by focusing on:
- Academic style
- Vague sentences
- Style consistency
See an example
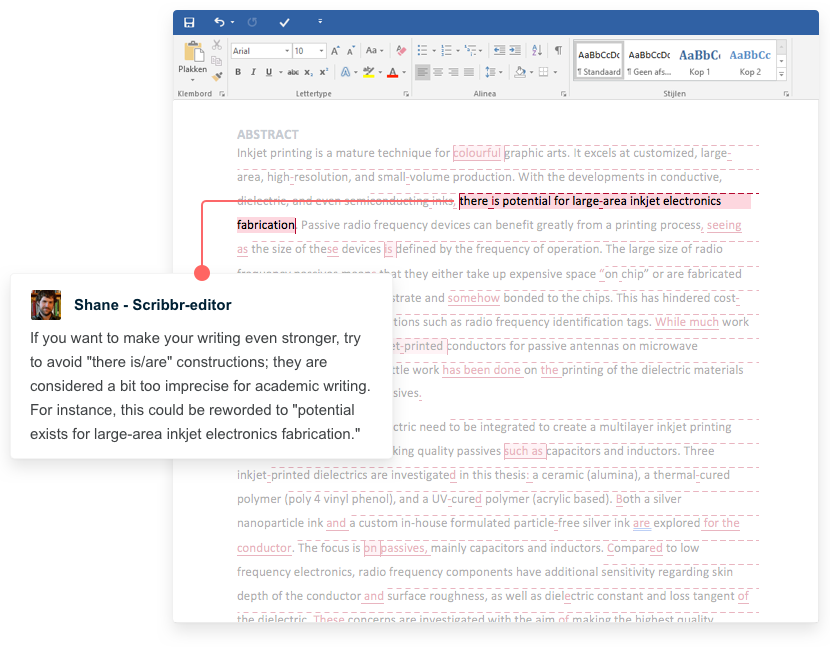
The final step of statistical analysis is interpreting your results.
Statistical significance
In hypothesis testing, statistical significance is the main criterion for forming conclusions. You compare your p value to a set significance level (usually 0.05) to decide whether your results are statistically significant or non-significant.
Statistically significant results are considered unlikely to have arisen solely due to chance. There is only a very low chance of such a result occurring if the null hypothesis is true in the population.
This means that you believe the meditation intervention, rather than random factors, directly caused the increase in test scores. Example: Interpret your results (correlational study) You compare your p value of 0.001 to your significance threshold of 0.05. With a p value under this threshold, you can reject the null hypothesis. This indicates a statistically significant correlation between parental income and GPA in male college students.
Note that correlation doesn’t always mean causation, because there are often many underlying factors contributing to a complex variable like GPA. Even if one variable is related to another, this may be because of a third variable influencing both of them, or indirect links between the two variables.
Effect size
A statistically significant result doesn’t necessarily mean that there are important real life applications or clinical outcomes for a finding.
In contrast, the effect size indicates the practical significance of your results. It’s important to report effect sizes along with your inferential statistics for a complete picture of your results. You should also report interval estimates of effect sizes if you’re writing an APA style paper .
With a Cohen’s d of 0.72, there’s medium to high practical significance to your finding that the meditation exercise improved test scores. Example: Effect size (correlational study) To determine the effect size of the correlation coefficient, you compare your Pearson’s r value to Cohen’s effect size criteria.
Decision errors
Type I and Type II errors are mistakes made in research conclusions. A Type I error means rejecting the null hypothesis when it’s actually true, while a Type II error means failing to reject the null hypothesis when it’s false.
You can aim to minimize the risk of these errors by selecting an optimal significance level and ensuring high power . However, there’s a trade-off between the two errors, so a fine balance is necessary.
Frequentist versus Bayesian statistics
Traditionally, frequentist statistics emphasizes null hypothesis significance testing and always starts with the assumption of a true null hypothesis.
However, Bayesian statistics has grown in popularity as an alternative approach in the last few decades. In this approach, you use previous research to continually update your hypotheses based on your expectations and observations.
Bayes factor compares the relative strength of evidence for the null versus the alternative hypothesis rather than making a conclusion about rejecting the null hypothesis or not.
If you want to know more about statistics , methodology , or research bias , make sure to check out some of our other articles with explanations and examples.
- Student’s t -distribution
- Normal distribution
- Null and Alternative Hypotheses
- Chi square tests
- Confidence interval
Methodology
- Cluster sampling
- Stratified sampling
- Data cleansing
- Reproducibility vs Replicability
- Peer review
- Likert scale
Research bias
- Implicit bias
- Framing effect
- Cognitive bias
- Placebo effect
- Hawthorne effect
- Hostile attribution bias
- Affect heuristic
Is this article helpful?
Other students also liked.
- Descriptive Statistics | Definitions, Types, Examples
- Inferential Statistics | An Easy Introduction & Examples
- Choosing the Right Statistical Test | Types & Examples
More interesting articles
- Akaike Information Criterion | When & How to Use It (Example)
- An Easy Introduction to Statistical Significance (With Examples)
- An Introduction to t Tests | Definitions, Formula and Examples
- ANOVA in R | A Complete Step-by-Step Guide with Examples
- Central Limit Theorem | Formula, Definition & Examples
- Central Tendency | Understanding the Mean, Median & Mode
- Chi-Square (Χ²) Distributions | Definition & Examples
- Chi-Square (Χ²) Table | Examples & Downloadable Table
- Chi-Square (Χ²) Tests | Types, Formula & Examples
- Chi-Square Goodness of Fit Test | Formula, Guide & Examples
- Chi-Square Test of Independence | Formula, Guide & Examples
- Coefficient of Determination (R²) | Calculation & Interpretation
- Correlation Coefficient | Types, Formulas & Examples
- Frequency Distribution | Tables, Types & Examples
- How to Calculate Standard Deviation (Guide) | Calculator & Examples
- How to Calculate Variance | Calculator, Analysis & Examples
- How to Find Degrees of Freedom | Definition & Formula
- How to Find Interquartile Range (IQR) | Calculator & Examples
- How to Find Outliers | 4 Ways with Examples & Explanation
- How to Find the Geometric Mean | Calculator & Formula
- How to Find the Mean | Definition, Examples & Calculator
- How to Find the Median | Definition, Examples & Calculator
- How to Find the Mode | Definition, Examples & Calculator
- How to Find the Range of a Data Set | Calculator & Formula
- Hypothesis Testing | A Step-by-Step Guide with Easy Examples
- Interval Data and How to Analyze It | Definitions & Examples
- Levels of Measurement | Nominal, Ordinal, Interval and Ratio
- Linear Regression in R | A Step-by-Step Guide & Examples
- Missing Data | Types, Explanation, & Imputation
- Multiple Linear Regression | A Quick Guide (Examples)
- Nominal Data | Definition, Examples, Data Collection & Analysis
- Normal Distribution | Examples, Formulas, & Uses
- Null and Alternative Hypotheses | Definitions & Examples
- One-way ANOVA | When and How to Use It (With Examples)
- Ordinal Data | Definition, Examples, Data Collection & Analysis
- Parameter vs Statistic | Definitions, Differences & Examples
- Pearson Correlation Coefficient (r) | Guide & Examples
- Poisson Distributions | Definition, Formula & Examples
- Probability Distribution | Formula, Types, & Examples
- Quartiles & Quantiles | Calculation, Definition & Interpretation
- Ratio Scales | Definition, Examples, & Data Analysis
- Simple Linear Regression | An Easy Introduction & Examples
- Skewness | Definition, Examples & Formula
- Statistical Power and Why It Matters | A Simple Introduction
- Student's t Table (Free Download) | Guide & Examples
- T-distribution: What it is and how to use it
- Test statistics | Definition, Interpretation, and Examples
- The Standard Normal Distribution | Calculator, Examples & Uses
- Two-Way ANOVA | Examples & When To Use It
- Type I & Type II Errors | Differences, Examples, Visualizations
- Understanding Confidence Intervals | Easy Examples & Formulas
- Understanding P values | Definition and Examples
- Variability | Calculating Range, IQR, Variance, Standard Deviation
- What is Effect Size and Why Does It Matter? (Examples)
- What Is Kurtosis? | Definition, Examples & Formula
- What Is Standard Error? | How to Calculate (Guide with Examples)
What is your plagiarism score?
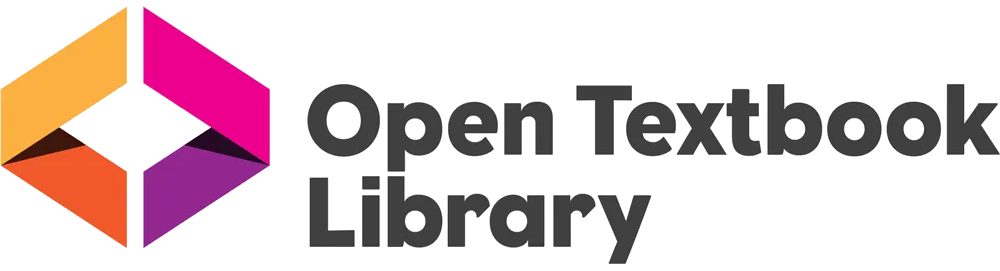
Statistics for Research Students
(2 reviews)

Erich C Fein, Toowoomba, Australia
John Gilmour, Toowoomba, Australia
Tayna Machin, Toowoomba, Australia
Liam Hendry, Toowoomba, Australia
Copyright Year: 2022
ISBN 13: 9780645326109
Publisher: University of Southern Queensland
Language: English
Formats Available
Conditions of use.

Learn more about reviews.
Reviewed by Sojib Bin Zaman, Assistant Professor, James Madison University on 3/18/24
From exploring data in Chapter One to learning advanced methodologies such as moderation and mediation in Chapter Seven, the reader is guided through the entire process of statistical methodology. With each chapter covering a different statistical... read more
Comprehensiveness rating: 5 see less
From exploring data in Chapter One to learning advanced methodologies such as moderation and mediation in Chapter Seven, the reader is guided through the entire process of statistical methodology. With each chapter covering a different statistical technique and methodology, students gain a comprehensive understanding of statistical research techniques.
Content Accuracy rating: 5
During my review of the textbook, I did not find any notable errors or omissions. In my opinion, the material was comprehensive, resulting in an enjoyable learning experience.
Relevance/Longevity rating: 5
A majority of the textbook's content is aligned with current trends, advancements, and enduring principles in the field of statistics. Several emerging methodologies and technologies are incorporated into this textbook to enhance students' statistical knowledge. It will be a valuable resource in the long run if students and researchers can properly utilize this textbook.
Clarity rating: 5
A clear explanation of complex statistical concepts such as moderation and mediation is provided in the writing style. Examples and problem sets are provided in the textbook in a comprehensive and well-explained manner.
Consistency rating: 5
Each chapter maintains consistent formatting and language, with resources organized consistently. Headings and subheadings worked well.
Modularity rating: 5
The textbook is well-structured, featuring cohesive chapters that flow smoothly from one to another. It is carefully crafted with a focus on defining terms clearly, facilitating understanding, and ensuring logical flow.
Organization/Structure/Flow rating: 5
From basic to advanced concepts, this book provides clarity of progression, logical arranging of sections and chapters, and effective headings and subheadings that guide readers. Further, the organization provides students with a lot of information on complex statistical methodologies.
Interface rating: 5
The available formats included PDFs, online access, and e-books. The e-book interface was particularly appealing to me, as it provided seamless navigation and viewing of content without compromising usability.
Grammatical Errors rating: 5
I found no significant errors in this document, and the overall quality of the writing was commendable. There was a high level of clarity and coherence in the text, which contributed to a positive reading experience.
Cultural Relevance rating: 5
The content of the book, as well as its accompanying examples, demonstrates a dedication to inclusivity by taking into account cultural diversity and a variety of perspectives. Furthermore, the material actively promotes cultural diversity, which enables readers to develop a deeper understanding of various cultural contexts and experiences.
In summary, this textbook provides a comprehensive resource tailored for advanced statistics courses, characterized by meticulous organization and practical supplementary materials. This book also provides valuable insights into the interpretation of computer output that enhance a greater understanding of each concept presented.
Reviewed by Zhuanzhuan Ma, Assistant Professor, University of Texas Rio Grande Valley on 3/7/24
The textbook covers all necessary areas and topics for students who want to conduct research in statistics. It includes foundational concepts, application methods, and advanced statistical techniques relevant to research methodologies. read more
The textbook covers all necessary areas and topics for students who want to conduct research in statistics. It includes foundational concepts, application methods, and advanced statistical techniques relevant to research methodologies.
The textbook presents statistical methods and data accurately, with up-to-date statistical practices and examples.
Relevance/Longevity rating: 4
The textbook's content is relevant to current research practices. The book includes contemporary examples and case studies that are currently prevalent in research communities. One small drawback is that the textbook did not include the example code for conduct data analysis.
The textbook break down complex statistical methods into understandable segments. All the concepts are clearly explained. Authors used diagrams, examples, and all kinds of explanations to facilitate learning for students with varying levels of background knowledge.
The terminology, framework, and presentation style (e.g. concepts, methodologies, and examples) seem consistent throughout the book.
The textbook is well organized that each chapter and section can be used independently without losing the context necessary for understanding. Also, the modular structure allows instructors and students to adapt the materials for different study plans.
The textbook is well-organized and progresses from basic concepts to more complex methods, making it easier for students to follow along. There is a logical flow of the content.
The digital format of the textbook has an interface that includes the design, layout, and navigational features. It is easier to use for readers.
The quality of writing is very high. The well-written texts help both instructors and students to follow the ideas clearly.
The textbook does not perpetuate stereotypes or biases and are inclusive in their examples, language, and perspectives.
Table of Contents
- Acknowledgement of Country
- Accessibility Information
- About the Authors
- Introduction
- I. Chapter One - Exploring Your Data
- II. Chapter Two - Test Statistics, p Values, Confidence Intervals and Effect Sizes
- III. Chapter Three- Comparing Two Group Means
- IV. Chapter Four - Comparing Associations Between Two Variables
- V. Chapter Five- Comparing Associations Between Multiple Variables
- VI. Chapter Six- Comparing Three or More Group Means
- VII. Chapter Seven- Moderation and Mediation Analyses
- VIII. Chapter Eight- Factor Analysis and Scale Reliability
- IX. Chapter Nine- Nonparametric Statistics
Ancillary Material
About the book.
This book aims to help you understand and navigate statistical concepts and the main types of statistical analyses essential for research students.
About the Contributors
Dr Erich C. Fein is an Associate Professor at the University of Southern Queensland. He received substantial training in research methods and statistics during his PhD program at Ohio State University. He currently teaches four courses in research methods and statistics. His research involves leadership, occupational health, and motivation, as well as issues related to research methods such as the following article: “ Safeguarding Access and Safeguarding Meaning as Strategies for Achieving Confidentiality .” Click here to link to his Google Scholar profile.
Dr John Gilmour is a Lecturer at the University of Southern Queensland and a Postdoctoral Research Fellow at the University of Queensland, His research focuses on the locational and temporal analyses of crime, and the evaluation of police training and procedures. John has worked across many different sectors including PTSD, social media, criminology, and medicine.
Dr Tanya Machin is a Senior Lecturer and Associate Dean at the University of Southern Queensland. Her research focuses on social media and technology across the lifespan. Tanya has co-taught Honours research methods with Erich, and is also interested in ethics and qualitative research methods. Tanya has worked across many different sectors including primary schools, financial services, and mental health.
Dr Liam Hendry is a Lecturer at the University of Southern Queensland. His research interests focus on long-term and short-term memory, measurement of human memory, attention, learning & diverse aspects of cognitive psychology.
Contribute to this Page
Innovative Statistics Project Ideas for Insightful Analysis

Table of contents
- 1.1 AP Statistics Topics for Project
- 1.2 Statistics Project Topics for High School Students
- 1.3 Statistical Survey Topics
- 1.4 Statistical Experiment Ideas
- 1.5 Easy Stats Project Ideas
- 1.6 Business Ideas for Statistics Project
- 1.7 Socio-Economic Easy Statistics Project Ideas
- 1.8 Experiment Ideas for Statistics and Analysis
- 2 Conclusion: Navigating the World of Data Through Statistics
Diving into the world of data, statistics presents a unique blend of challenges and opportunities to uncover patterns, test hypotheses, and make informed decisions. It is a fascinating field that offers many opportunities for exploration and discovery. This article is designed to inspire students, educators, and statistics enthusiasts with various project ideas. We will cover:
- Challenging concepts suitable for advanced placement courses.
- Accessible ideas that are engaging and educational for younger students.
- Ideas for conducting surveys and analyzing the results.
- Topics that explore the application of statistics in business and socio-economic areas.
Each category of topics for the statistics project provides unique insights into the world of statistics, offering opportunities for learning and application. Let’s dive into these ideas and explore the exciting world of statistical analysis.
Top Statistics Project Ideas for High School
Statistics is not only about numbers and data; it’s a unique lens for interpreting the world. Ideal for students, educators, or anyone with a curiosity about statistical analysis, these project ideas offer an interactive, hands-on approach to learning. These projects range from fundamental concepts suitable for beginners to more intricate studies for advanced learners. They are designed to ignite interest in statistics by demonstrating its real-world applications, making it accessible and enjoyable for people of all skill levels.
Need help with statistics project? Get your paper written by a professional writer Get Help Reviews.io 4.9/5
AP Statistics Topics for Project
- Analyzing Variance in Climate Data Over Decades.
- The Correlation Between Economic Indicators and Standard of Living.
- Statistical Analysis of Voter Behavior Patterns.
- Probability Models in Sports: Predicting Outcomes.
- The Effectiveness of Different Teaching Methods: A Statistical Study.
- Analysis of Demographic Data in Public Health.
- Time Series Analysis of Stock Market Trends.
- Investigating the Impact of Social Media on Academic Performance.
- Survival Analysis in Clinical Trial Data.
- Regression Analysis on Housing Prices and Market Factors.
Statistics Project Topics for High School Students
- The Mathematics of Personal Finance: Budgeting and Spending Habits.
- Analysis of Class Performance: Test Scores and Study Habits.
- A Statistical Comparison of Local Public Transportation Options.
- Survey on Dietary Habits and Physical Health Among Teenagers.
- Analyzing the Popularity of Various Music Genres in School.
- The Impact of Sleep on Academic Performance: A Statistical Approach.
- Statistical Study on the Use of Technology in Education.
- Comparing Athletic Performance Across Different Sports.
- Trends in Social Media Usage Among High School Students.
- The Effect of Part-Time Jobs on Student Academic Achievement.
Statistical Survey Topics
- Public Opinion on Environmental Conservation Efforts.
- Consumer Preferences in the Fast Food Industry.
- Attitudes Towards Online Learning vs. Traditional Classroom Learning.
- Survey on Workplace Satisfaction and Productivity.
- Public Health: Attitudes Towards Vaccination.
- Trends in Mobile Phone Usage and Preferences.
- Community Response to Local Government Policies.
- Consumer Behavior in Online vs. Offline Shopping.
- Perceptions of Public Safety and Law Enforcement.
- Social Media Influence on Political Opinions.
Statistical Experiment Ideas
- The Effect of Light on Plant Growth.
- Memory Retention: Visual vs. Auditory Information.
- Caffeine Consumption and Cognitive Performance.
- The Impact of Exercise on Stress Levels.
- Testing the Efficacy of Natural vs. Chemical Fertilizers.
- The Influence of Color on Mood and Perception.
- Sleep Patterns: Analyzing Factors Affecting Sleep Quality.
- The Effectiveness of Different Types of Water Filters.
- Analyzing the Impact of Room Temperature on Concentration.
- Testing the Strength of Different Brands of Batteries.
Easy Stats Project Ideas
- Average Daily Screen Time Among Students.
- Analyzing the Most Common Birth Months.
- Favorite School Subjects Among Peers.
- Average Time Spent on Homework Weekly.
- Frequency of Public Transport Usage.
- Comparison of Pet Ownership in the Community.
- Favorite Types of Movies or TV Shows.
- Daily Water Consumption Habits.
- Common Breakfast Choices and Their Nutritional Value.
- Steps Count: A Week-Long Study.
Business Ideas for Statistics Project
- Analyzing Customer Satisfaction in Retail Stores.
- Market Analysis of a New Product Launch.
- Employee Performance Metrics and Organizational Success.
- Sales Data Analysis for E-commerce Websites.
- Impact of Advertising on Consumer Buying Behavior.
- Analysis of Supply Chain Efficiency.
- Customer Loyalty and Retention Strategies.
- Trend Analysis in Social Media Marketing.
- Financial Risk Assessment in Investment Decisions.
- Market Segmentation and Targeting Strategies.
Socio-Economic Easy Statistics Project Ideas
- Income Inequality and Its Impact on Education.
- The Correlation Between Unemployment Rates and Crime Levels.
- Analyzing the Effects of Minimum Wage Changes.
- The Relationship Between Public Health Expenditure and Population Health.
- Demographic Analysis of Housing Affordability.
- The Impact of Immigration on Local Economies.
- Analysis of Gender Pay Gap in Different Industries.
- Statistical Study of Homelessness Causes and Solutions.
- Education Levels and Their Impact on Job Opportunities.
- Analyzing Trends in Government Social Spending.
Experiment Ideas for Statistics and Analysis
- Multivariate Analysis of Global Climate Change Data.
- Time-Series Analysis in Predicting Economic Recessions.
- Logistic Regression in Medical Outcome Prediction.
- Machine Learning Applications in Statistical Modeling.
- Network Analysis in Social Media Data.
- Bayesian Analysis of Scientific Research Data.
- The Use of Factor Analysis in Psychology Studies.
- Spatial Data Analysis in Geographic Information Systems (GIS).
- Predictive Analysis in Customer Relationship Management (CRM).
- Cluster Analysis in Market Research.
Conclusion: Navigating the World of Data Through Statistics
In this exploration of good statistics project ideas, we’ve ventured through various topics, from the straightforward to the complex, from personal finance to global climate change. These ideas are gateways to understanding the world of data and statistics, and platforms for cultivating critical thinking and analytical skills. Whether you’re a high school student, a college student, or a professional, engaging in these projects can deepen your appreciation of how statistics shapes our understanding of the world around us. These projects encourage exploration, inquiry, and a deeper engagement with the world of numbers, trends, and patterns – the essence of statistics.
Readers also enjoyed
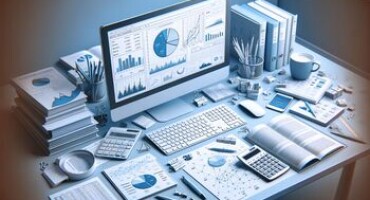
WHY WAIT? PLACE AN ORDER RIGHT NOW!
Just fill out the form, press the button, and have no worries!
We use cookies to give you the best experience possible. By continuing we’ll assume you board with our cookie policy.

Best stats research topics in 2023: Innovations in Statistical Analysis
Are you searching for the best stats research topics in 2023? If yes, then have a close look at some of the best stats research topics in 2023.
Statistics is the branch of mathematics that deals with the collection, analysis, interpretation, presentation, and organization of data. It is an essential tool for understanding and making sense of complex data sets and is used in a wide range of fields, including business, healthcare, social sciences, and engineering.
Research in statistics is critical for developing new statistical methods, improving existing techniques, and applying statistical analysis to solve real-world problems. This paper will provide an overview of some of the key research topics in statistics, including data analysis, experimental design, statistical modeling, machine learning, and data visualization.
Each section will define the topic, provide an overview of the current state of research, and discuss future directions for research in that area. By providing an overview of these key topics, this paper aims to highlight the importance of ongoing research in statistics and to inspire future research in this critical field.
Importance of Statistics Research
Table of Contents
Have a close look at the importance of statistics research.
Helps in making informed decisions
Statistics research helps individuals, businesses, and governments make informed decisions based on data-driven analysis. For example, businesses can use statistical analysis to identify consumer trends, predict demand for products, and make decisions about marketing strategies. Governments can use statistical analysis to evaluate the effectiveness of policies, allocate resources, and make decisions about public services.
Improves accuracy
Statistics research helps to improve the accuracy of predictions, forecasts, and estimations. By analyzing large datasets, researchers can identify patterns and trends that may not be immediately visible. This can lead to more accurate predictions about future outcomes, which can be useful in many fields, from finance to healthcare.
Provides insights
Statistics research can provide insights into the relationships between variables and the factors that drive certain outcomes. For example, by analyzing data on consumer behavior, researchers can identify which factors influence purchasing decisions, and use this information to develop more effective marketing strategies.
Validates hypotheses
Statistics research can be used to test hypotheses and validate theories in various fields. For example, in the field of psychology, researchers can use statistical analysis to test the effectiveness of different therapy techniques and validate theories about human behavior.
Enables evidence-based policymaking
Statistics research can provide evidence to support policymaking and guide public policy decisions. For example, by analyzing data on crime rates, policymakers can identify areas where crime is most prevalent and develop policies to address the issue.
Assists in risk management
Statistics research can be used to assess risks and identify potential threats in various contexts. For example, in the field of finance, statistical analysis can be used to assess the risk associated with different investment strategies.
Enhances research and development
Statistics research can help to enhance research and development efforts by providing valuable insights and feedback. For example, in the field of medicine, statistical analysis can be used to evaluate the effectiveness of new drugs and treatments.
Supports quality improvement
Statistics research can support quality improvement efforts by identifying areas of improvement and measuring the effectiveness of interventions. For example, in the field of education, statistical analysis can be used to evaluate the effectiveness of different teaching methods and identify areas where improvements can be made.
Facilitates performance measurement
Statistics research can be used to measure performance in various contexts, such as business, healthcare, and education. For example, in the field of business, statistical analysis can be used to measure employee performance and identify areas where improvements can be made.
Helps in predicting future trends
Statistics research can be used to analyze past trends and make predictions about future trends and outcomes. For example, in the field of finance, statistical analysis can be used to make predictions about stock market trends and identify investment opportunities.
Stats Research Topics
Have a close look at stats research topics.
Health and Medicine
Correlation between diet and health outcomes.
The study of the correlation between diet and health outcomes is an important topic in health and medicine statistics research. With the rise of chronic diseases such as obesity, diabetes, and heart disease, there is a growing need to understand the relationship between diet and health outcomes. Researchers can use statistics to analyze large datasets and identify patterns and correlations between dietary habits and health outcomes. This analysis can lead to the development of effective interventions and policies to improve dietary habits and prevent chronic diseases.
Effectiveness of various medications
The effectiveness of various medications is another important topic in health and medicine statistics research. With new medications constantly being developed, it is important to evaluate their effectiveness in treating various conditions. Researchers can use statistics to analyze clinical trial data to determine the effectiveness of different medications, and to identify any side effects or risks associated with their use. This analysis can lead to the development of better medications and improved treatment protocols.
Analysis of vaccination rates and their impact on public health
Vaccinations are an important tool in preventing the spread of infectious diseases, but there is often controversy surrounding their use. Statistics research can be used to analyze vaccination rates and their impact on public health, including the reduction of disease outbreaks and healthcare costs associated with treating these diseases. This analysis can lead to the development of effective vaccination policies and programs to improve public health outcomes.
Study of healthcare utilization and costs
The study of healthcare utilization and costs is another important topic in health and medicine statistics research. Researchers can use statistics to analyze healthcare utilization patterns, including hospital admissions, emergency department visits, and physician visits. This analysis can help identify areas where healthcare resources are being overused or underused, and can inform the development of policies and interventions to improve healthcare utilization and reduce costs.
Analysis of health disparities
Health disparities refer to differences in health outcomes between different groups of people. Statistics research can be used to analyze health disparities and identify the factors that contribute to them. This analysis can help inform the development of interventions and policies to reduce health disparities and improve health outcomes for all populations.
Study of environmental health
Environmental health is an important topic in health and medicine statistics research. Researchers can use statistics to analyze the relationship between environmental exposures and health outcomes. This analysis can help inform the development of policies and interventions to reduce environmental exposures and improve public health outcomes.
Overall, statistics research plays a crucial role in the field of health and medicine, allowing researchers to better understand the relationships between diet, medications, vaccinations, healthcare utilization, health disparities, and environmental health. By using statistical methods to analyze large datasets, researchers can identify patterns and correlations that can lead to improved healthcare practices and better public health outcomes.
Social Sciences
Social sciences deal with the study of human society and relationships between individuals and groups. Here are some potential research topics related to social sciences:
Here are some potential statistics research topics in the field of social sciences:
Study of crime rates and factors that contribute to criminal behavior
Researchers can use statistical methods to analyze crime rates and identify the factors that contribute to criminal behavior, such as poverty, unemployment, and education levels. This information can help policymakers develop effective strategies to prevent crime and improve public safety.
Analysis of income inequality and its effects on society
Income inequality is a pressing social issue that can have far-reaching impacts on society, such as increased crime rates and decreased social mobility. Researchers can use statistical methods to analyze income inequality trends and their impacts on various aspects of society, such as healthcare, education, and employment.
Impact of various forms of media on social attitudes and behaviors
Social media, television, and other forms of media have the power to shape social attitudes and behaviors. Researchers can use statistical methods to analyze the effects of different forms of media on issues such as political polarization, racial attitudes, and mental health.
Study of the effects of education on income and social mobility
Education is often seen as a key factor in promoting social mobility and reducing income inequality. Researchers can use statistical methods to analyze the relationship between education levels and income, and to identify the factors that influence this relationship.
Analysis of the effects of immigration on society
Immigration is a complex issue that can have significant impacts on society, such as changes in demographics, economic growth, and cultural norms. Researchers can use statistical methods to analyze the effects of immigration on various aspects of society, such as crime rates, healthcare, and labor markets.
Study of the effects of social policies on vulnerable populations: Social policies such as welfare programs and healthcare reforms are designed to help vulnerable populations, but their effectiveness can vary widely. Researchers can use statistical methods to analyze the impacts of social policies on different populations, such as low-income families, the elderly, and individuals with disabilities.
Here are some potential statistics research topics in economics:
Economic impacts of COVID-19 pandemic
The COVID-19 pandemic has had significant impacts on the global economy, with wide-ranging effects on different industries, countries, and demographic groups. Statistics research can be used to analyze the economic impacts of the pandemic, including changes in employment rates, consumer spending, and GDP.
Analysis of stock market trends and investment strategies
The stock market is a complex and dynamic system that can be difficult to predict. Statistics research can be used to analyze stock market trends and identify potential investment strategies, such as diversification, value investing, and growth investing.
Relationship between minimum wage and economic growth
The minimum wage is a controversial topic in economics, with proponents arguing that it can stimulate economic growth by increasing consumer spending and reducing poverty, while opponents argue that it can lead to job losses and inflation. Statistics research can be used to analyze the relationship between minimum wage and economic growth, including the effects on employment rates, inflation, and GDP.
Analysis of international trade patterns
International trade is a critical component of the global economy, with significant impacts on different countries and industries. Statistics research can be used to analyze international trade patterns, including the factors that drive trade flows, the effects on economic growth and development, and the implications for global economic governance.
Evaluation of economic policies
Governments and international organizations often implement economic policies aimed at promoting economic growth, reducing inequality, and mitigating economic crises. Statistics research can be used to evaluate the effectiveness of these policies, including the impacts on different sectors of the economy and the distributional effects on different demographic groups.
Analysis of income inequality
Income inequality is a growing concern in many countries, with significant implications for social welfare and economic development. Statistics research can be used to analyze income inequality patterns, including the factors that contribute to income disparities, the effects on different demographic groups, and the implications for economic growth and development.
Overall, statistics research can be a powerful tool for analyzing complex economic phenomena and developing evidence-based policies and strategies to promote economic growth, reduce inequality, and mitigate economic crises.
: |
Environment and Sustainability
Here are some potential statistics research topics in the field of Environment and Sustainability:
Analysis of climate change impacts on agriculture
Climate change can have significant impacts on agricultural productivity, including changes in temperature, rainfall patterns, and extreme weather events. Researchers can use statistical analysis to understand the relationships between climate variables and agricultural outcomes, and to develop strategies to adapt to and mitigate the impacts of climate change on food systems.
Evaluation of the effectiveness of carbon pricing policies
Carbon pricing policies such as carbon taxes and emissions trading systems are increasingly being implemented as a means of reducing greenhouse gas emissions. Researchers can use statistical methods to evaluate the effectiveness of these policies in reducing emissions and achieving other environmental goals, such as promoting the transition to renewable energy sources .
Analysis of water resource management strategies
Water is a critical resource for human well-being and ecosystem health, and effective management is essential for sustainability. Researchers can use statistical analysis to evaluate the effectiveness of different water resource management strategies, such as water conservation programs and watershed management plans, and to identify areas for improvement.
Assessment of the environmental impacts of transportation systems
Transportation is a significant contributor to greenhouse gas emissions and air pollution. Researchers can use statistical methods to analyze the environmental impacts of different transportation modes, such as cars, buses, trains, and airplanes, and to evaluate the effectiveness of policies to promote sustainable transportation.
Evaluation of sustainable land use practices
Land use change is a major driver of biodiversity loss, deforestation, and soil degradation. Researchers can use statistical analysis to evaluate the effectiveness of different sustainable land use practices, such as agroforestry, conservation agriculture, and reforestation, in promoting biodiversity conservation and ecosystem health.
Overall, statistics research is essential for understanding the complex relationships between human activities and the natural environment, and for developing effective strategies for promoting sustainability and mitigating environmental impacts.
Technology-related statistics research
Technology-related statistics research is an important field of study that involves analyzing data related to technological advancements and their impact on society. Some potential research topics in this field include:
Analysis of cybersecurity threats
With the increasing reliance on technology in various industries, cybersecurity threats have become a major concern. Statistics research can be used to analyze patterns and trends in cyber attacks, and to develop strategies to mitigate the risks associated with them.
Evaluation of technology adoption rates
The adoption of new technologies can have a significant impact on businesses and society as a whole. Statistics research can be used to analyze adoption rates of new technologies, and to identify factors that influence their adoption.
Study of the impact of technology on employment
Advances in technology have led to significant changes in the job market, with some jobs becoming obsolete and new jobs emerging. Statistics research can be used to analyze the impact of technology on employment, and to develop strategies to mitigate the negative effects.
Analysis of social media trends
Social media has become an integral part of modern society, with billions of users around the world. Statistics research can be used to analyze social media trends and behaviors, and to develop strategies for using social media effectively.
Study of the impact of artificial intelligence (AI)
AI is becoming increasingly prevalent in various industries, from healthcare to finance. Statistics research can be used to analyze the impact of AI on these industries, and to identify potential risks and benefits.
Evaluation of technology-related policies
Governments around the world have implemented various policies related to technology, such as net neutrality and data privacy regulations. Statistics research can be used to evaluate the effectiveness of these policies and to identify areas for improvement.
Overall, technology-related statistics research is an important field of study that can help us better understand the impact of technology on society and develop strategies for using technology effectively and responsibly.
In conclusion, statistics research plays a crucial role in various fields such as health, social sciences, economics, and the environment. The potential research topics in each of these fields are vast, ranging from the correlation between diet and health outcomes to the analysis of climate change and its effects on ecosystems. The findings of statistics research can have significant implications for decision-makers in various industries, policymakers, and society as a whole. Therefore, it is vital to continue exploring and studying statistics research to gain a deeper understanding of the world around us.
Frequently Asked Questions
What is statistics research.
Statistics research involves the collection, analysis, and interpretation of numerical data to derive insights and make informed decisions. It is used in various fields to study trends, patterns, and relationships in data.
Why is statistics research important?
Statistics research helps us make informed decisions based on data, rather than relying on assumptions or guesswork. It allows us to study complex phenomena, identify patterns and trends, and test hypotheses. It is used in many fields, including healthcare, social sciences, economics, and environmental studies.
What are some common statistical methods used in research?
Some common statistical methods used in research include regression analysis, hypothesis testing, data visualization, and time series analysis. The choice of method depends on the research question and the type of data being analyzed.
How can statistics research benefit society?
Statistics research can benefit society in many ways, such as identifying factors that contribute to public health issues, evaluating the effectiveness of social policies, and predicting economic trends. It can also inform decision-making in industries such as healthcare, education, and environmental conservation.
What are some potential limitations of statistics research?
Some potential limitations of statistics research include the possibility of sampling bias, errors in data collection, and confounding variables that may affect the results. It is important to carefully design studies and use appropriate statistical methods to minimize these limitations.
Similar Articles

13 Best Tips To Write An Assignment
Whenever the new semester starts, you will get a lot of assignment writing tasks. Now you enter the new academic…

How To Do Homework Fast – 11 Tips To Do Homework Fast
Homework is one of the most important parts that have to be done by students. It has been around for…
Leave a Comment Cancel Reply
Your email address will not be published. Required fields are marked *
This site uses Akismet to reduce spam. Learn how your comment data is processed .
- Privacy Policy

Home » 500+ Quantitative Research Titles and Topics
500+ Quantitative Research Titles and Topics
Table of Contents

Quantitative research involves collecting and analyzing numerical data to identify patterns, trends, and relationships among variables. This method is widely used in social sciences, psychology , economics , and other fields where researchers aim to understand human behavior and phenomena through statistical analysis. If you are looking for a quantitative research topic, there are numerous areas to explore, from analyzing data on a specific population to studying the effects of a particular intervention or treatment. In this post, we will provide some ideas for quantitative research topics that may inspire you and help you narrow down your interests.
Quantitative Research Titles
Quantitative Research Titles are as follows:
Business and Economics
- “Statistical Analysis of Supply Chain Disruptions on Retail Sales”
- “Quantitative Examination of Consumer Loyalty Programs in the Fast Food Industry”
- “Predicting Stock Market Trends Using Machine Learning Algorithms”
- “Influence of Workplace Environment on Employee Productivity: A Quantitative Study”
- “Impact of Economic Policies on Small Businesses: A Regression Analysis”
- “Customer Satisfaction and Profit Margins: A Quantitative Correlation Study”
- “Analyzing the Role of Marketing in Brand Recognition: A Statistical Overview”
- “Quantitative Effects of Corporate Social Responsibility on Consumer Trust”
- “Price Elasticity of Demand for Luxury Goods: A Case Study”
- “The Relationship Between Fiscal Policy and Inflation Rates: A Time-Series Analysis”
- “Factors Influencing E-commerce Conversion Rates: A Quantitative Exploration”
- “Examining the Correlation Between Interest Rates and Consumer Spending”
- “Standardized Testing and Academic Performance: A Quantitative Evaluation”
- “Teaching Strategies and Student Learning Outcomes in Secondary Schools: A Quantitative Study”
- “The Relationship Between Extracurricular Activities and Academic Success”
- “Influence of Parental Involvement on Children’s Educational Achievements”
- “Digital Literacy in Primary Schools: A Quantitative Assessment”
- “Learning Outcomes in Blended vs. Traditional Classrooms: A Comparative Analysis”
- “Correlation Between Teacher Experience and Student Success Rates”
- “Analyzing the Impact of Classroom Technology on Reading Comprehension”
- “Gender Differences in STEM Fields: A Quantitative Analysis of Enrollment Data”
- “The Relationship Between Homework Load and Academic Burnout”
- “Assessment of Special Education Programs in Public Schools”
- “Role of Peer Tutoring in Improving Academic Performance: A Quantitative Study”
Medicine and Health Sciences
- “The Impact of Sleep Duration on Cardiovascular Health: A Cross-sectional Study”
- “Analyzing the Efficacy of Various Antidepressants: A Meta-Analysis”
- “Patient Satisfaction in Telehealth Services: A Quantitative Assessment”
- “Dietary Habits and Incidence of Heart Disease: A Quantitative Review”
- “Correlations Between Stress Levels and Immune System Functioning”
- “Smoking and Lung Function: A Quantitative Analysis”
- “Influence of Physical Activity on Mental Health in Older Adults”
- “Antibiotic Resistance Patterns in Community Hospitals: A Quantitative Study”
- “The Efficacy of Vaccination Programs in Controlling Disease Spread: A Time-Series Analysis”
- “Role of Social Determinants in Health Outcomes: A Quantitative Exploration”
- “Impact of Hospital Design on Patient Recovery Rates”
- “Quantitative Analysis of Dietary Choices and Obesity Rates in Children”
Social Sciences
- “Examining Social Inequality through Wage Distribution: A Quantitative Study”
- “Impact of Parental Divorce on Child Development: A Longitudinal Study”
- “Social Media and its Effect on Political Polarization: A Quantitative Analysis”
- “The Relationship Between Religion and Social Attitudes: A Statistical Overview”
- “Influence of Socioeconomic Status on Educational Achievement”
- “Quantifying the Effects of Community Programs on Crime Reduction”
- “Public Opinion and Immigration Policies: A Quantitative Exploration”
- “Analyzing the Gender Representation in Political Offices: A Quantitative Study”
- “Impact of Mass Media on Public Opinion: A Regression Analysis”
- “Influence of Urban Design on Social Interactions in Communities”
- “The Role of Social Support in Mental Health Outcomes: A Quantitative Analysis”
- “Examining the Relationship Between Substance Abuse and Employment Status”
Engineering and Technology
- “Performance Evaluation of Different Machine Learning Algorithms in Autonomous Vehicles”
- “Material Science: A Quantitative Analysis of Stress-Strain Properties in Various Alloys”
- “Impacts of Data Center Cooling Solutions on Energy Consumption”
- “Analyzing the Reliability of Renewable Energy Sources in Grid Management”
- “Optimization of 5G Network Performance: A Quantitative Assessment”
- “Quantifying the Effects of Aerodynamics on Fuel Efficiency in Commercial Airplanes”
- “The Relationship Between Software Complexity and Bug Frequency”
- “Machine Learning in Predictive Maintenance: A Quantitative Analysis”
- “Wearable Technologies and their Impact on Healthcare Monitoring”
- “Quantitative Assessment of Cybersecurity Measures in Financial Institutions”
- “Analysis of Noise Pollution from Urban Transportation Systems”
- “The Influence of Architectural Design on Energy Efficiency in Buildings”
Quantitative Research Topics
Quantitative Research Topics are as follows:
- The effects of social media on self-esteem among teenagers.
- A comparative study of academic achievement among students of single-sex and co-educational schools.
- The impact of gender on leadership styles in the workplace.
- The correlation between parental involvement and academic performance of students.
- The effect of mindfulness meditation on stress levels in college students.
- The relationship between employee motivation and job satisfaction.
- The effectiveness of online learning compared to traditional classroom learning.
- The correlation between sleep duration and academic performance among college students.
- The impact of exercise on mental health among adults.
- The relationship between social support and psychological well-being among cancer patients.
- The effect of caffeine consumption on sleep quality.
- A comparative study of the effectiveness of cognitive-behavioral therapy and pharmacotherapy in treating depression.
- The relationship between physical attractiveness and job opportunities.
- The correlation between smartphone addiction and academic performance among high school students.
- The impact of music on memory recall among adults.
- The effectiveness of parental control software in limiting children’s online activity.
- The relationship between social media use and body image dissatisfaction among young adults.
- The correlation between academic achievement and parental involvement among minority students.
- The impact of early childhood education on academic performance in later years.
- The effectiveness of employee training and development programs in improving organizational performance.
- The relationship between socioeconomic status and access to healthcare services.
- The correlation between social support and academic achievement among college students.
- The impact of technology on communication skills among children.
- The effectiveness of mindfulness-based stress reduction programs in reducing symptoms of anxiety and depression.
- The relationship between employee turnover and organizational culture.
- The correlation between job satisfaction and employee engagement.
- The impact of video game violence on aggressive behavior among children.
- The effectiveness of nutritional education in promoting healthy eating habits among adolescents.
- The relationship between bullying and academic performance among middle school students.
- The correlation between teacher expectations and student achievement.
- The impact of gender stereotypes on career choices among high school students.
- The effectiveness of anger management programs in reducing violent behavior.
- The relationship between social support and recovery from substance abuse.
- The correlation between parent-child communication and adolescent drug use.
- The impact of technology on family relationships.
- The effectiveness of smoking cessation programs in promoting long-term abstinence.
- The relationship between personality traits and academic achievement.
- The correlation between stress and job performance among healthcare professionals.
- The impact of online privacy concerns on social media use.
- The effectiveness of cognitive-behavioral therapy in treating anxiety disorders.
- The relationship between teacher feedback and student motivation.
- The correlation between physical activity and academic performance among elementary school students.
- The impact of parental divorce on academic achievement among children.
- The effectiveness of diversity training in improving workplace relationships.
- The relationship between childhood trauma and adult mental health.
- The correlation between parental involvement and substance abuse among adolescents.
- The impact of social media use on romantic relationships among young adults.
- The effectiveness of assertiveness training in improving communication skills.
- The relationship between parental expectations and academic achievement among high school students.
- The correlation between sleep quality and mood among adults.
- The impact of video game addiction on academic performance among college students.
- The effectiveness of group therapy in treating eating disorders.
- The relationship between job stress and job performance among teachers.
- The correlation between mindfulness and emotional regulation.
- The impact of social media use on self-esteem among college students.
- The effectiveness of parent-teacher communication in promoting academic achievement among elementary school students.
- The impact of renewable energy policies on carbon emissions
- The relationship between employee motivation and job performance
- The effectiveness of psychotherapy in treating eating disorders
- The correlation between physical activity and cognitive function in older adults
- The effect of childhood poverty on adult health outcomes
- The impact of urbanization on biodiversity conservation
- The relationship between work-life balance and employee job satisfaction
- The effectiveness of eye movement desensitization and reprocessing (EMDR) in treating trauma
- The correlation between parenting styles and child behavior
- The effect of social media on political polarization
- The impact of foreign aid on economic development
- The relationship between workplace diversity and organizational performance
- The effectiveness of dialectical behavior therapy in treating borderline personality disorder
- The correlation between childhood abuse and adult mental health outcomes
- The effect of sleep deprivation on cognitive function
- The impact of trade policies on international trade and economic growth
- The relationship between employee engagement and organizational commitment
- The effectiveness of cognitive therapy in treating postpartum depression
- The correlation between family meals and child obesity rates
- The effect of parental involvement in sports on child athletic performance
- The impact of social entrepreneurship on sustainable development
- The relationship between emotional labor and job burnout
- The effectiveness of art therapy in treating dementia
- The correlation between social media use and academic procrastination
- The effect of poverty on childhood educational attainment
- The impact of urban green spaces on mental health
- The relationship between job insecurity and employee well-being
- The effectiveness of virtual reality exposure therapy in treating anxiety disorders
- The correlation between childhood trauma and substance abuse
- The effect of screen time on children’s social skills
- The impact of trade unions on employee job satisfaction
- The relationship between cultural intelligence and cross-cultural communication
- The effectiveness of acceptance and commitment therapy in treating chronic pain
- The correlation between childhood obesity and adult health outcomes
- The effect of gender diversity on corporate performance
- The impact of environmental regulations on industry competitiveness.
- The impact of renewable energy policies on greenhouse gas emissions
- The relationship between workplace diversity and team performance
- The effectiveness of group therapy in treating substance abuse
- The correlation between parental involvement and social skills in early childhood
- The effect of technology use on sleep patterns
- The impact of government regulations on small business growth
- The relationship between job satisfaction and employee turnover
- The effectiveness of virtual reality therapy in treating anxiety disorders
- The correlation between parental involvement and academic motivation in adolescents
- The effect of social media on political engagement
- The impact of urbanization on mental health
- The relationship between corporate social responsibility and consumer trust
- The correlation between early childhood education and social-emotional development
- The effect of screen time on cognitive development in young children
- The impact of trade policies on global economic growth
- The relationship between workplace diversity and innovation
- The effectiveness of family therapy in treating eating disorders
- The correlation between parental involvement and college persistence
- The effect of social media on body image and self-esteem
- The impact of environmental regulations on business competitiveness
- The relationship between job autonomy and job satisfaction
- The effectiveness of virtual reality therapy in treating phobias
- The correlation between parental involvement and academic achievement in college
- The effect of social media on sleep quality
- The impact of immigration policies on social integration
- The relationship between workplace diversity and employee well-being
- The effectiveness of psychodynamic therapy in treating personality disorders
- The correlation between early childhood education and executive function skills
- The effect of parental involvement on STEM education outcomes
- The impact of trade policies on domestic employment rates
- The relationship between job insecurity and mental health
- The effectiveness of exposure therapy in treating PTSD
- The correlation between parental involvement and social mobility
- The effect of social media on intergroup relations
- The impact of urbanization on air pollution and respiratory health.
- The relationship between emotional intelligence and leadership effectiveness
- The effectiveness of cognitive-behavioral therapy in treating depression
- The correlation between early childhood education and language development
- The effect of parental involvement on academic achievement in STEM fields
- The impact of trade policies on income inequality
- The relationship between workplace diversity and customer satisfaction
- The effectiveness of mindfulness-based therapy in treating anxiety disorders
- The correlation between parental involvement and civic engagement in adolescents
- The effect of social media on mental health among teenagers
- The impact of public transportation policies on traffic congestion
- The relationship between job stress and job performance
- The effectiveness of group therapy in treating depression
- The correlation between early childhood education and cognitive development
- The effect of parental involvement on academic motivation in college
- The impact of environmental regulations on energy consumption
- The relationship between workplace diversity and employee engagement
- The effectiveness of art therapy in treating PTSD
- The correlation between parental involvement and academic success in vocational education
- The effect of social media on academic achievement in college
- The impact of tax policies on economic growth
- The relationship between job flexibility and work-life balance
- The effectiveness of acceptance and commitment therapy in treating anxiety disorders
- The correlation between early childhood education and social competence
- The effect of parental involvement on career readiness in high school
- The impact of immigration policies on crime rates
- The relationship between workplace diversity and employee retention
- The effectiveness of play therapy in treating trauma
- The correlation between parental involvement and academic success in online learning
- The effect of social media on body dissatisfaction among women
- The impact of urbanization on public health infrastructure
- The relationship between job satisfaction and job performance
- The effectiveness of eye movement desensitization and reprocessing therapy in treating PTSD
- The correlation between early childhood education and social skills in adolescence
- The effect of parental involvement on academic achievement in the arts
- The impact of trade policies on foreign investment
- The relationship between workplace diversity and decision-making
- The effectiveness of exposure and response prevention therapy in treating OCD
- The correlation between parental involvement and academic success in special education
- The impact of zoning laws on affordable housing
- The relationship between job design and employee motivation
- The effectiveness of cognitive rehabilitation therapy in treating traumatic brain injury
- The correlation between early childhood education and social-emotional learning
- The effect of parental involvement on academic achievement in foreign language learning
- The impact of trade policies on the environment
- The relationship between workplace diversity and creativity
- The effectiveness of emotion-focused therapy in treating relationship problems
- The correlation between parental involvement and academic success in music education
- The effect of social media on interpersonal communication skills
- The impact of public health campaigns on health behaviors
- The relationship between job resources and job stress
- The effectiveness of equine therapy in treating substance abuse
- The correlation between early childhood education and self-regulation
- The effect of parental involvement on academic achievement in physical education
- The impact of immigration policies on cultural assimilation
- The relationship between workplace diversity and conflict resolution
- The effectiveness of schema therapy in treating personality disorders
- The correlation between parental involvement and academic success in career and technical education
- The effect of social media on trust in government institutions
- The impact of urbanization on public transportation systems
- The relationship between job demands and job stress
- The correlation between early childhood education and executive functioning
- The effect of parental involvement on academic achievement in computer science
- The effectiveness of cognitive processing therapy in treating PTSD
- The correlation between parental involvement and academic success in homeschooling
- The effect of social media on cyberbullying behavior
- The impact of urbanization on air quality
- The effectiveness of dance therapy in treating anxiety disorders
- The correlation between early childhood education and math achievement
- The effect of parental involvement on academic achievement in health education
- The impact of global warming on agriculture
- The effectiveness of narrative therapy in treating depression
- The correlation between parental involvement and academic success in character education
- The effect of social media on political participation
- The impact of technology on job displacement
- The relationship between job resources and job satisfaction
- The effectiveness of art therapy in treating addiction
- The correlation between early childhood education and reading comprehension
- The effect of parental involvement on academic achievement in environmental education
- The impact of income inequality on social mobility
- The relationship between workplace diversity and organizational culture
- The effectiveness of solution-focused brief therapy in treating anxiety disorders
- The correlation between parental involvement and academic success in physical therapy education
- The effect of social media on misinformation
- The impact of green energy policies on economic growth
- The relationship between job demands and employee well-being
- The correlation between early childhood education and science achievement
- The effect of parental involvement on academic achievement in religious education
- The impact of gender diversity on corporate governance
- The relationship between workplace diversity and ethical decision-making
- The correlation between parental involvement and academic success in dental hygiene education
- The effect of social media on self-esteem among adolescents
- The impact of renewable energy policies on energy security
- The effect of parental involvement on academic achievement in social studies
- The impact of trade policies on job growth
- The relationship between workplace diversity and leadership styles
- The correlation between parental involvement and academic success in online vocational training
- The effect of social media on self-esteem among men
- The impact of urbanization on air pollution levels
- The effectiveness of music therapy in treating depression
- The correlation between early childhood education and math skills
- The effect of parental involvement on academic achievement in language arts
- The impact of immigration policies on labor market outcomes
- The effectiveness of hypnotherapy in treating phobias
- The effect of social media on political engagement among young adults
- The impact of urbanization on access to green spaces
- The relationship between job crafting and job satisfaction
- The effectiveness of exposure therapy in treating specific phobias
- The correlation between early childhood education and spatial reasoning
- The effect of parental involvement on academic achievement in business education
- The impact of trade policies on economic inequality
- The effectiveness of narrative therapy in treating PTSD
- The correlation between parental involvement and academic success in nursing education
- The effect of social media on sleep quality among adolescents
- The impact of urbanization on crime rates
- The relationship between job insecurity and turnover intentions
- The effectiveness of pet therapy in treating anxiety disorders
- The correlation between early childhood education and STEM skills
- The effect of parental involvement on academic achievement in culinary education
- The impact of immigration policies on housing affordability
- The relationship between workplace diversity and employee satisfaction
- The effectiveness of mindfulness-based stress reduction in treating chronic pain
- The correlation between parental involvement and academic success in art education
- The effect of social media on academic procrastination among college students
- The impact of urbanization on public safety services.
About the author
Muhammad Hassan
Researcher, Academic Writer, Web developer
You may also like

500+ Environmental Research Topics

500+ Nursing Research Topic Ideas

500+ Psychology Research Paper Topics

500+ Criminal Justice Research Topics

500+ Qualitative Research Titles and Topics

500+ Business Research Topics
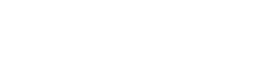
Recent Dissertation Topics
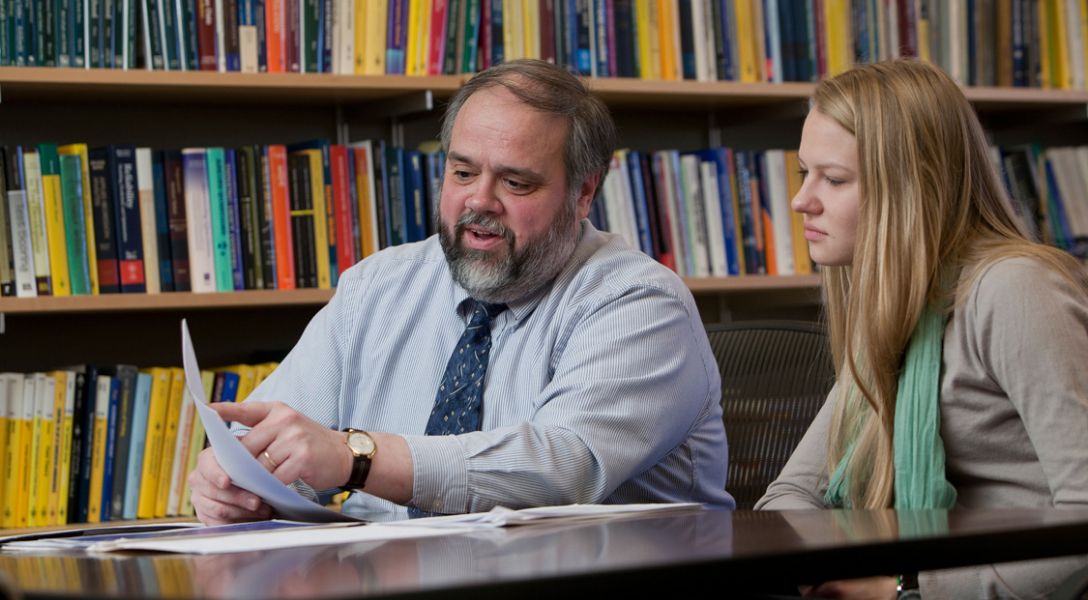
Kerstin Emily Frailey - “PRACTICAL DATA QUALITY FOR MODERN DATA & MODERN USES, WITH APPLICATIONS TO AMERICA’S COVID-19 DATA"
Dissertation Advisor: Martin Wells
Initial job placement: Co-Founder & CEO
David Kent - “Smoothness-Penalized Deconvolution: Rates of Convergence, Choice of Tuning Parameter, and Inference"
Dissertation Advisor: David Ruppert
Initial job placement: VISITING ASSISTANT PROFESSOR - Cornell University
Yuchen Xu - “Dynamic Atomic Column Detection in Transmission Electron Microscopy Videos via Ridge Estimation”
Dissertation Advisor: David Matteson
Initial job placement: Postdoctoral Fellow - UCLA
Siyi Deng - “Optimal and Safe Semi-supervised Estimation and Inference for High-dimensional Linear Regression"
Dissertation Advisor: Yang Ning
Initial job placement: Data Scientist - TikTok
Peter (Haoxuan) Wu - “Advances in adaptive and deep Bayesian state-space models”
Initial job placement: Quantitative Researcher - DRW
Grace Deng - “Generative models and Bayesian spillover graphs for dynamic networks”
Initial job placement: Data Scientist - Research at Google
Samriddha Lahiry - “Some problems of asymptotic quantum statistical inference”
Dissertation Advisor: Michael Nussbaum
Initial job placement: Postdoctoral Fellow - Harvard University
Yaosheng Xu - “WWTA load-balancing for parallel-server systems with heterogeneous servers and multi-scale heavy traffic limits for generalized Jackson networks”
Dissertation Advisor: Jim Dai
Initial job placement: Applied Scientist - Amazon
Seth Strimas-Mackey - “Latent structure in linear prediction and corpora comparison”
Dissertation Advisor: Marten Wegkamp and Florentina Bunea
Initial job placement: Data Scientist at Google
Tao Zhang - “Topics in modern regression modeling”
Dissertation Advisor: David Ruppert and Kengo Kato
Initial job placement: Quantitative Researcher - Point72
Wentian Huang - “Nonparametric and semiparametric approaches to functional data modeling”
Initial job placement: Ernst & Young
Binh Tang - “Deep probabilistic models for sequential prediction”
Initial job placement: Amazon
Yi Su - “Off-policy evaluation and learning for interactive systems"
Dissertation Advisor: Thorsten Joachims
Initial job placement: Berkeley (postdoc)
Ruqi Zhang - “Scalable and reliable inference for probabilistic modeling”
Dissertation Advisor: Christopher De Sa
Jason Sun - “Recent developments on Matrix Completion"
Initial job placement: LinkedIn
Indrayudh Ghosal - “Model combinations and the Infinitesimal Jackknife : how to refine models with boosting and quantify uncertainty”
Dissertation Advisor: Giles Hooker
Benjamin Ryan Baer - “Contributions to fairness and transparency”
Initial job placement: Rochester (postdoc)
Megan Lynne Gelsinger - “Spatial and temporal approaches to analyzing big data”
Dissertation Advisor: David Matteson and Joe Guinness
Initial job placement: Institute for Defense Analysis
Zhengze Zhou - “Statistical inference for machine learning : feature importance, uncertainty quantification and interpretation stability”
Initial job placement: Facebook
Huijie Feng - “Estimation and inference of high-dimensional individualized threshold with binary responses”
Initial job placement: Microsoft
Xiaojie Mao - “Machine learning methods for data-driven decision making : contextual optimization, causal inference, and algorithmic fairness”
Dissertation Advisor: Nathan Kallus and Madeleine Udell
Initial job placement: Tsinghua University, China
Xin Bing - “Structured latent factor models : Identifiability, estimation, inference and prediction”
Initial job placement: Cambridge (postdoc), University of Toronto
Yang Liu - “Nonparametric regression and density estimation on a network"
Dissertation Advisor: David Ruppert and Peter Frazier
Initial job placement: Research Analyst - Cubist Systematic Strategies
Skyler Seto - “Learning from less : improving and understanding model selection in penalized machine learning problems”
Initial job placement: Machine Learning Researcher - Apple
Jiekun Feng - “Markov chain, Markov decision process, and deep reinforcement learning with applications to hospital management and real-time ride-hailing”
Initial job placement:
Wenyu Zhang - “Methods for change point detection in sequential data”
Initial job placement: Research Scientist - Institute for Infocomm Research
Liao Zhu - “The adaptive multi-factor model and the financial market"
Initial job placement: Quantitative Researcher - Two Sigma
Xiaoyun Quan - “Latent Gaussian copula model for high dimensional mixed data, and its applications”
Dissertation Advisor: James Booth and Martin Wells
Praphruetpong (Ben) Athiwaratkun - "Density representations for words and hierarchical data"
Dissertation Advisor: Andrew Wilson
Initial job placement: AI Scientist - AWS AI Labs
Yiming Sun - “High dimensional data analysis with dependency and under limited memory”
Dissertation Advisor: Sumanta Basu and Madeleine Udell
Zi Ye - “Functional single index model and jensen effect"
Dissertation Advisor: Giles Hooker
Initial job placement: Data & Applied Scientist - Microsoft
Hui Fen (Sarah) Tan - “Interpretable approaches to opening up black-box models”
Dissertation Advisor: Giles Hooker and Martin Wells
Daniel E. Gilbert - “Luck, fairness and Bayesian tensor completion”
Yichen zhou - “asymptotics and interpretability of decision trees and decision tree ensemblesg”.
Initial job placement: Data Scientist - Google
Ze Jin - “Measuring statistical dependence and its applications in machine learning”
Initial job placement: Research Scientist, Facebook Integrity Ranking & ML - Facebook
Xiaohan Yan - “Statistical learning for structural patterns with trees”
Dissertation Advisor: Jacob Bien
Initial job placement: Senior Data Scientist - Microsoft
Guo Yu - “High-dimensional structured regression using convex optimization”
Dan kowal - "bayesian methods for functional and time series data".
Dissertation Advisor: David Matteson and David Ruppert
Initial job placement: assistant professor, Department of Statistics, Rice University
Keegan Kang - "Data Dependent Random Projections"
David sinclair - "model selection results for high dimensional graphical models on binary and count data with applications to fmri and genomics", liu, yanning – "statistical issues in the design and analysis of clinical trials".
Dissertation Advisor: Bruce Turnbull
Nicholson, William Bertil – "Tools for Modeling Sparse Vector Autoregressions"
Tupper, laura lindley – "topics in classification and clustering of high-dimensional data", chetelat, didier – "high-dimensional inference by unbiased risk estimation".
Initial Job Placement: Assistant Professor Universite de Montreal, Montreal, Canada
Gaynanova, Irina – "Estimation Of Sparse Low-Dimensional Linear Projections"
Dissertation Advisor: James Booth
Initial Job Placement: Assistant Professor, Texas A&M, College Station, TX
Mentch, Lucas – "Ensemble Trees and CLTS: Statistical Inference in Machine Learning"
Initial Job Placement: Assistant Professor, University of Pittsburgh, Pittsburgh, PA
Risk, Ben – "Topics in Independent Component Analysis, Likelihood Component Analysis, and Spatiotemporal Mixed Modeling"
Dissertation Advisors: David Matteson and David Ruppert
Initial Job Placement: Postdoctoral Fellow, University of North Carolina, Chapel Hill, NC
Zhao, Yue – "Contributions to the Statistical Inference for the Semiparametric Elliptical Copula Model"
Disseration Advisor: Marten Wegkamp
Initial Job Placement: Postoctoral Fellow, McGill University, Montreal, Canada
Chen, Maximillian Gene – "Dimension Reduction and Inferential Procedures for Images"
Dissertation Advisor: Martin Wells
Earls, Cecelia – Bayesian hierarchical Gaussian process models for functional data analysis
Dissertation Advisor: Giles Hooker
Initial Job Placement: Lecturer, Cornell University, Ithaca, NY
Li, James Yi-Wei – "Tensor (Multidimensional Array) Decomposition, Regression, and Software for Statistics and Machine Learning"
Initial Job Placement: Research Scientist, Yahoo Labs
Schneider, Matthew John – "Three Papers on Time Series Forecasting and Data Privacy"
Dissertation Advisor: John Abowd
Initial Job Placement: Assistant Professor, Northwestern University, Evanston, IL
Thorbergsson, Leifur – "Experimental design for partially observed Markov decision processes"
Initial Job Placement: Data Scientist, Memorial Sloan Kettering Cancer Center, New York, NY
Wan, Muting – "Model-Based Classification with Applications to High-Dimensional Data in Bioinformatics"
Initial Job Placement: Senior Associate, 1010 Data, New York, NY
Johnson, Lynn Marie – "Topics in Linear Models: Methods for Clustered, Censored Data and Two-Stage Sampling Designs"
Dissertation Advisor: Robert Strawderman
Initial Job Placement: Statistical Consultant, Cornell, Statistical Consulting Unit, Ithaca, NY
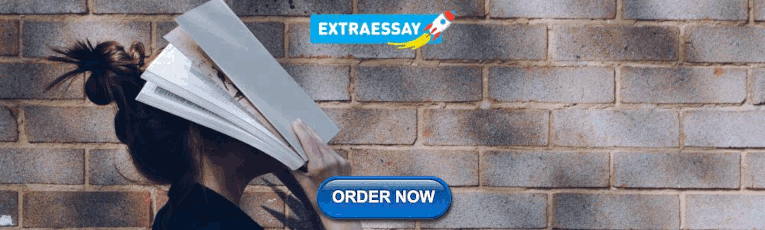
Tecuapetla Gomez, Inder Rafael – "Asymptotic Inference for Locally Stationary Processes"
Initial Job Placement: Postdoctoral Fellow, Georg-August-Universitat Gottigen, Gottigen, Germany.
Bar, Haim – "Parallel Testing, and Variable Selection -- a Mixture-Model Approach with Applications in Biostatistics"
Dissertation Advisor: James Booth
Initial Job Placement: Postdoc, Department of Medicine, Weill Medical Center, New York, NY
Cunningham, Caitlin – "Markov Methods for Identifying ChIP-seq Peaks"
Initial Job Placement: Assistant Professor, Le Moyne College, Syracuse, NY
Ji, Pengsheng – "Selected Topics in Nonparametric Testing and Variable Selection for High Dimensional Data"
Dissertation Advisor: Michael Nussbaum
Initial Job Placement: Assistant Professor, University of Georgia, Athens, GA
Morris, Darcy Steeg – "Methods for Multivariate Longitudinal Count and Duration Models with Applications in Economics"
Dissertation Advisor: Francesca Molinari
Initial Job Placement: Research Mathematical Statistician, Center for Statistical Research and Methodology, U.S. Census Bureau, Washington DC
Narayanan, Rajendran – "Shrinkage Estimation for Penalised Regression, Loss Estimation and Topics on Largest Eigenvalue Distributions"
Initial Job Placement: Visiting Scientist, Indian Statistical Institute, Kolkata, India
Xiao, Luo – "Topics in Bivariate Spline Smoothing"
Dissertation Advisor: David Ruppert
Initial Job Placement: Postdoc, Johns Hopkins University, Baltimore, MD
Zeber, David – "Extremal Properties of Markov Chains and the Conditional Extreme Value Model"
Dissertation Advisor: Sidney Resnick
Initial Job Placement: Data Analyst, Mozilla, San Francisco, CA
Clement, David – "Estimating equation methods for longitudinal and survival data"
Dissertation Advisor: Robert Strawderman
Initial Job Placement: Quantitative Analyst, Smartodds, London UK
Eilertson, Kirsten – "Estimation and inference of random effect models with applications to population genetics and proteomics"
Dissertation Advisor: Carlos Bustamante
Initial Job Placement: Biostatistician, The J. David Gladstone Institutes, San Francisco CA
Grabchak, Michael – "Tempered stable distributions: properties and extensions"
Dissertation Advisor: Gennady Samorodnitsky
Initial Job Placement: Assistant Professor, UNC Charlotte, Charlotte NC
Li, Yingxing – "Aspects of penalized splines"
Initial Job Placement: Assistant Professor, The Wang Yanan Institute for Studies in Economics, Xiamen University
Lopez Oliveros, Luis – "Modeling end-user behavior in data networks"
Dissertation Advisor: Sidney Resnick
Initial Job Placement: Consultant, Murex North America, New York NY
Ma, Xin – "Statistical Methods for Genome Variant Calling and Population Genetic Inference from Next-Generation Sequencing Data"
Initial Job Placement: Postdoc, Stanford University, Stanford CA
Kormaksson, Matthias – "Dynamic path analysis and model based clustering of microarray data"
Dissertation Advisor: James Booth
Initial Job Placement: Postdoc, Department of Public Health, Weill Cornell Medical College, New York NY
Schifano, Elizabeth – "Topics in penalized estimation"
Initial Job Placement: Postdoc, Department of Biostatistics, Harvard University, Boston MA
Hanlon, Bret – "High-dimensional data analysis"
Dissertation Advisor: Anand Vidyashankar
Shaby, Benjamin – "Tools for hard bayesian computations"
Initial Job Placement: Postdoc, SAMSI, Durham NC
Zipunnikov, Vadim – "Topics on generalized linear mixed models"
Initial Job Placement: Postdoc, Department of Biostatistics, Johns Hopkins University, Baltimore MD
Barger, Kathryn Jo-Anne – "Objective bayesian estimation for the number of classes in a population using Jeffreys and reference priors"
Dissertation Advisor: John Bunge
Initial Job Placement: Pfizer Incorporated
Chan, Serena Suewei – "Robust and efficient inference for linear mixed models using skew-normal distributions"
Initial Job Placement: Statistician, Takeda Pharmaceuticles, Deerfield IL
Lin, Haizhi – "Distressed debt prices and recovery rate estimation"
Dissertation Advisor: Martin Wells
Initial Job Placement: Associate, Fixed Income Department, Credit Suisse Securities (USA), New York, NY
Statistical Papers
Statistical Papers is a forum for presentation and critical assessment of statistical methods encouraging the discussion of methodological foundations and potential applications.
- The Journal stresses statistical methods that have broad applications, giving special attention to those relevant to the economic and social sciences.
- Covers all topics of modern data science, such as frequentist and Bayesian design and inference as well as statistical learning.
- Contains original research papers (regular articles), survey articles, short communications, reports on statistical software, and book reviews.
- High author satisfaction with 90% likely to publish in the journal again.
- Werner G. Müller,
- Carsten Jentsch,
- Shuangzhe Liu,
- Ulrike Schneider
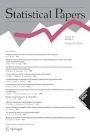
Latest issue
Volume 65, Issue 6
Latest articles
Coherent indexes for shifted count and semicontinuous models.
- Marcelo Bourguignon
- Célestin C. Kokonendji
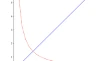
Exceedance statistics based on bottom- \(k\) -lists
- Halil Tanil
General classes of bivariate distributions for modeling data with common observations
- Na Young Yoo
- Ji Hwan Cha
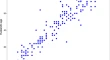
Hotelling \(T^2\) test in high dimensions with application to Wilks outlier method
- Reza Modarres
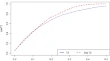
On the functional regression model and its finite-dimensional approximations
- José R. Berrendero
- Alejandro Cholaquidis
- Antonio Cuevas
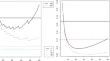
Journal updates
Write & submit: overleaf latex template.
Overleaf LaTeX Template
Journal information
- Australian Business Deans Council (ABDC) Journal Quality List
- Current Index to Statistics
- Google Scholar
- Japanese Science and Technology Agency (JST)
- Mathematical Reviews
- Norwegian Register for Scientific Journals and Series
- OCLC WorldCat Discovery Service
- Research Papers in Economics (RePEc)
- Science Citation Index Expanded (SCIE)
- TD Net Discovery Service
- UGC-CARE List (India)
Rights and permissions
Editorial policies
© Springer-Verlag GmbH Germany, part of Springer Nature
- Find a journal
- Publish with us
- Track your research
Put a stop to deadline pressure, and have your homework done by an expert.
Top 100 Statistics Topics To Research In 2023
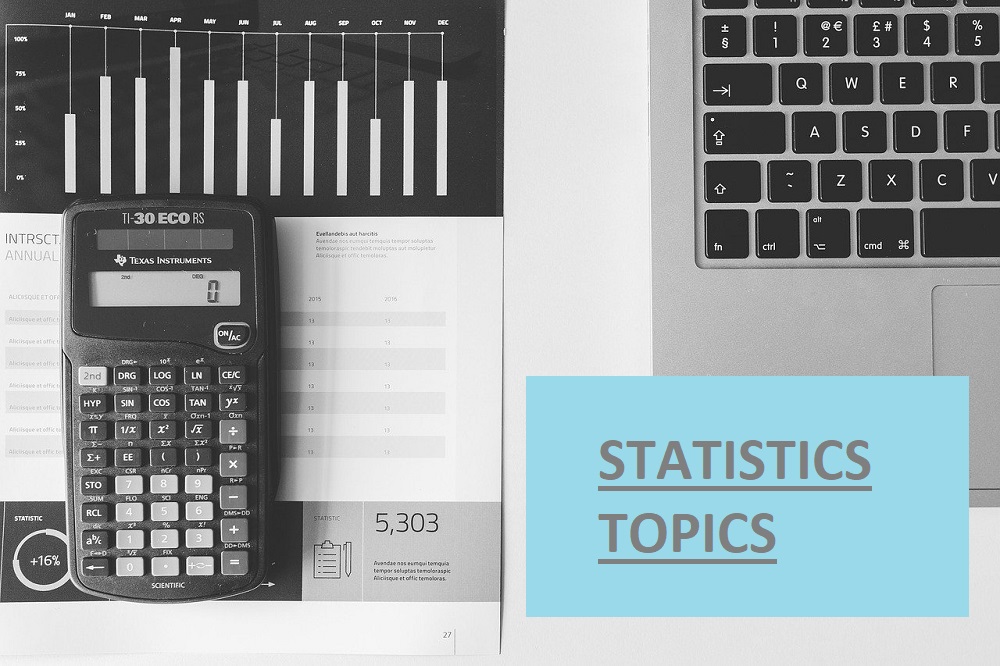
If you are looking for some interesting statistics topics that should work well in 2023, you have arrived at the right place. We have a list of 100 awesome statistics topics that you can use to get the inspiration you need. And did you know that all our statistics topics for project and statistics paper topics are 100% free? You can use them as you like and even reword them.
The Importance of a Good Statistics Topic
Why would you need our statistics project topics list? What makes a good statistics topic so important? The truth is that professors are subjective when it comes to essays and topics. Most of them will award bonus points to students who manage to come up with interesting statistics project topic ideas. After all, a great topic means you’ve invested a lot of time and effort into the paper, studied popular and scholarly sources to write it. We know that original statistics project topics are hard to come by, so we’ve created a list of 100 brand new topics for 2023.
Statistics Projects Topics
Our ENL writers compiled a list of the most common statistics projects topics. You can easily write an essay on these in one or two days because they don’t require much research:
- Using statistics in actuarial science
- Analyze an example of statistical signal processing
- Compare the Smith chart and the Sankey diagram
- Discuss the correlation coefficient
- Practical application of the Metropolis-Hastings algorithm
- Getting ready for a world of robots
Easy Statistics Research Topics
We have a list of easy statistics research topics that you can surely handle all by yourself. Choose one of these topics and start writing:
- Using statistics in epidemiology
- Applications of statistical physics
- Pros and cons of the Stemplot and Radar chart
- Using a Venn diagram correctly
- Child marriages in Africa (statistics)
- Discuss the analysis of variance (ANOVA) process
- Discuss the Box–Jenkins method
Statistical Research Topic for High School
Are you a high school student who needs to find a great statistics idea for an essay? Check out the following statistical research topic for high school:
- Using statistics in chemometrics
- Statistics and business analytics
- Discuss the field of statistical thermodynamics
- Principal component analysis in multivariate statistics
- What is a kernel density estimation?
- Selecting the correct sample for a survey
- What are cross-sectional studies?
Most Interesting Topics in Statistics
We’ve included all of the most interesting topics in statistics in a separate list. You can find the best of the best right here:
- Using statistics in machine learning
- What are statistical finance processes?
- Statistics in quality control in 2023
- Compare and contrast the Skewplot and the Sparkline
- Using Renkonen similarity index in botanic studies
- Calculate the probability of success using the binomial proportion confidence interval
- Statistics as a mathematical science
Hot Topics for Statistics Projects
Some ideas are better than others, especially when it comes to finding a good topic. Here are what we consider to be very hot topics for statistics projects:
- Using statistics in jurimetrics
- What are environmental statistics?
- Compare the curve fitting and smoothing processes
- Analyze 3 GEEs (Generalized estimating equations)
- Discuss the Rule of three in medicine
- The Goodman and Kruskal’s lambda measure
Survey Topics for Statistics
Conducting a survey is not that difficult, we agree. However, finding a good topic for your survey is. Pick one of our survey topics for statistics and start organizing the survey in minutes:
- Gather information about the GPA from 70 students in your university
- Survey how much time students spend doing their homework
- Make a survey on surveys
- Make a survey about the English language in high school
- What is your favorite city survey
- What do you think about our government survey
- Are you satisfied with your life survey
Good Topics for Statistics Projects
This is the list where you can find the topics that are not breathtaking. Check out these good topics for statistics projects and select one today:
- Analyze the Markov Chain central limit theorem
- Discuss the loop-erased random walk model
- Bernoulli matrix vs the Centering matrix in statistics
- Using statistics in psychometrics
- Interpreting the total sum of squares correctly
- Apply Kuder–Richardson’s Formula 20 in psychometrics
AP Statistics Topics
Advanced Placement Statistics is one of the most difficult courses for college students. This is why we want to help you with some very interesting AP statistics topics:
- Getting an adjacency matrix quickly
- What is the orthostochastic matrix?
- Obtaining the transition matrix optimally
- Discuss econometrics and its role
- Analyze the pros of the Probit Model
- Categorical data analysis and the Cochran–Armitage test for trend
- The history of probability
Theoretical Statistics Topics for a Core Course
If you are looking for some nice theoretical statistics topics for a core course, you have arrived at the right place. Here are some of our best ideas:
- Advantages of the Ornstein–Uhlenbeck process
- Discuss the Malliavin stochastic calculus
- Discuss stochastic optimal control
- Discuss homoscedasticity and heteroscedasticity
- Predicting errors using the Akaike information criterion
- The history of statistics
Business Statistics Topics
Would you like to write about business? Our experienced team of writers and editors managed to come up with these original business statistics topics:
- The importance of statistics to business in 2023
- Kinds of data in business statistics
- Measures of central tendency and dispersion
- Discuss inferential statistics
- The process of sampling business data
- Effective uses of statistics in key business decisions
- The effects of probability on business decisions
Good Statistics Projects Topics
We know you want to keep things fresh and get some bonus points for an interesting topic. Here are some very good statistics projects topics that should work great in 2023:
- Statistics and the medical treatment of drug addiction
- How did Nate Silver predict the outcome of the 2008 US election?
- Describe the information theory in statistics
- How does AI use the Fuzzy associative matrix?
- Composing a questionnaire the right way
- Effects of questions on interviewees
- The importance of the order of questions in a survey
Statistical Research Topics for College Students
Of course, we have plenty of statistical research topics for college students. These are more difficult than those for high school students, but they should be manageable:
- Analyze John Tukey’s contribution to statistics
- Florence Nightingale and visual representation in statistics
- Discuss Gertrude Cox’s experimental design in statistics
- How does statistics improve ADHD treatment?
- The Krichevsky–Trofimov estimator in information theory
- The timeline of probability in statistics
- Discuss Pseudorandomness and Quasirandomness
Controversial Topics for Statistics Project
Just like any field, statistics has its fair share of controversial topics. We managed to gather the most intriguing controversial topics for statistics project right here:
- Should we pursue the artificial neural network?
- Using the Attack Rate statistic during an epidemic
- Discuss the ”admissible decision” rule
- The link between statistics and biometrics
- Should we abandon null hypothesis significance testing?
- Is the Bayes theorem incorrect?
Statistics Research Paper Topics for Graduates
We have a list of statistics research paper topics for graduates, of course. You can get some very nice ideas from these examples:
- Discuss Bayesian hierarchical models
- Discuss basic AJD (basic affine jump diffusion)
- A thorough analysis of Lévy’s continuity theorem
- Analyze the Chinese restaurant process
- The Cochran–Mantel–Haenszel test
- A practical analysis of the principle of maximum entropy
- An in-depth look at the Hewitt–Savage Zero–One law
Difficult Statistical Research Topics
If you want to try your hand at a more difficult topic, we can help. Take a quick look at these difficult statistical research topics and choose the one you like:
- Statistics and the science of probability
- Organizing neurobiological time series data
- Analyzing intrinsic fluctuations in biochemical systems
- Effective data mining of neurophysiological biomarkers
- Econometrics and statistics
- Discuss the axioms of probability (Kolmogorov)
Do you think these statistical project topics are not enough to get you a top grade? If you want an awesome statistics project topic, don’t hesitate to contact us. We will think of some unique topics and send them your way right away. Also, we can do much more than just create statistical projects topics. If you need assignment help , editing or proofreading assistance, we are the company to call. We have extensive experience writing essays and term papers for students of all ages. Our PhD writers are ready to spring into action and make sure you turn in an awesome essay – on time!
Get on top of your homework.
Leave a Reply Cancel reply
Your email address will not be published. Required fields are marked *
Statistical Research Questions: Five Examples for Quantitative Analysis
Table of contents, introduction.
How are statistical research questions for quantitative analysis written? This article provides five examples of statistical research questions that will allow statistical analysis to take place.
In quantitative research projects, writing statistical research questions requires a good understanding and the ability to discern the type of data that you will analyze. This knowledge is elemental in framing research questions that shall guide you in identifying the appropriate statistical test to use in your research.
Thus, before writing your statistical research questions and reading the examples in this article, read first the article that enumerates the four types of measurement scales . Knowing the four types of measurement scales will enable you to appreciate the formulation or structuring of research questions.
Five Examples of Statistical Research Questions
In writing the statistical research questions, I provide a topic that shows the variables of the study, the study description, and a link to the original scientific article to give you a glimpse of the real-world examples.
Topic 1: Physical Fitness and Academic Achievement
Statistical research question no. 1.
Is there a significant relationship between physical fitness and academic achievement?
Notice that this study correlated two variables, namely 1) physical fitness, and 2) academic achievement.
On the other hand, the researchers measured academic achievement in terms of a passing score in Mathematics and English. The variable is the number of passing scores in both Mathematics and English.
Most of what I discuss in the statistics articles I wrote came from self-study. It’s easier to understand concepts now as there are a lot of resource materials available online. Videos and ebooks from places like Youtube, Veoh, The Internet Archives, among others, provide free educational materials. Online education will be the norm of the future. I describe this situation in my post about Education 4.0 .
Topic 2: Climate Conditions and Consumption of Bottled Water
Statistical research question no. 2.
Is there a significant relationship between average temperature and amount of bottled water consumed?
Now, it’s easy to identify the statistical test to analyze the relationship between the two variables. You may refer to my previous post titled Parametric Statistics: Four Widely Used Parametric Tests and When to Use Them . Using the figure supplied in that article, the appropriate test to use is, again, Pearson’s Correlation Coefficient.
Topic 3: Nursing Home Staff Size and Number of COVID-19 Cases
Statistical research question no. 3.
Note that this study on COVID-19 looked into three variables, namely 1) number of unique employees working in skilled nursing homes, 2) number of weekly confirmed cases among residents and staff, and 3) number of weekly COVID-19 deaths among residents.
A simple Pearson test may be used to correlate one variable with another variable. But the study used multiple variables. Hence, they produced regression models that show how multiple variables affect the outcome. Some of the variables in the study may be redundant, meaning, those variables may represent the same attribute of a population. Stepwise multiple regression models take care of those redundancies. Using this statistical test requires further study and experience.
Topic 4: Surrounding Greenness, Stress, and Memory
Statistical research question no. 4.
As this article is behind a paywall and we cannot see the full article, we can content ourselves with the knowledge that three major variables were explored in this study. These are 1) exposure to and use of natural environments, 2) stress, and 3) memory performance.
Topic 5: Income and Happiness
This recent finding is an interesting read and is available online. Just click on the link I provide as the source below. The study sought to determine if income plays a role in people’s happiness across three age groups: young (18-30 years), middle (31-64 years), and old (65 or older). The literature review suggests that income has a positive effect on an individual’s sense of happiness. That’s because more money increases opportunities to fulfill dreams and buy more goods and services.
An investigation was conducted to determine if the size of nursing home staff and the number of COVID-19 cases are correlated. Specifically, they looked into the number of unique employees working daily, and the outcomes include weekly counts of confirmed COVID-19 cases among residents and staff and weekly COVID-19 deaths among residents.
Statistical Research Question No. 5
I do hope that upon reaching this part of the article, you are now well familiar on how to write statistical research questions. Practice makes perfect.
References:
Måseide, H. (2021). Income and Happiness: Does the relationship vary with age?
© P. A. Regoniel 12 October 2021 | Updated 08 January 2024
Related Posts
What is the topic sentence, paragraph unity (tspu) writing technique, how to use a mind map to prepare your research proposal, black wasp: 4 amazing facts, about the author, patrick regoniel, simplyeducate.me privacy policy.
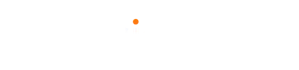
145 Best Statistics Project Ideas and Topics To Consider
Table of Contents
Are you a statistics student hunting for the best research project ideas? If yes, then this blog post is for you. Basically, statistics is a wide academic discipline that studies data and numerical information in almost all fields and various real-life situations that are related to mathematical science. Since the subject is broad, it might be extremely challenging to brainstorm and identify a good statistics research project topic. Therefore, to make your topic selection process easier, here, we have compiled a list of top statistics project ideas for you to consider. Explore them all and pick any idea that meets your requirements.
List of Statistics Project Ideas
For writing an exclusive statistics research paper, you can choose any of the below-mentioned statistics project ideas that justify your knowledge and understanding.
Statistics Project Ideas for School and College Students
- Increasing use of plastics
- Statistical analysis of the road accidents in your local area
- Course cost differentiation in colleges
- Are college students likely to develop drug addictions if given a chance?
- Are online classes helpful?
- Time spent by college students on social media
- Significance of the front seats in the class on success rates
- The effects of sitting in the backseat in a class
- Should mobile phones be allowed in high school or not?
- The ratio of college students getting married after graduation
- Relation between birth order as well as success in academics
- Is being headstrong difficult, or does it make things easy?
- Popular movie genre among students in college
- Web browsing habits of college students
- Caffeine consumption among students as well as its effect on the performance
- Common subjects were chosen by students in college
- What types of music do college students like the most?
- Are e-books better than conventional books?
- Choosing aspects of a subject in college
- Do extra-curricular activities help transform personalities?
- Should stereotypical social issues be highlighted or not?
- Comparison between male as well as female students in college
- Comparative study on the pricing of different clothing store prices in your town.
- Does the race of actors affect the popularity of TV shows among college students?
- Does the experience of a freshman in college with their roommate affect their overall experience at the institution?
- Does the effect of a Teacher who is fresher in University influence the student’s performance?
- Influence of Distinct Subjects on Student’s Performance.
- Significance of Analytics in Studying Statistics.
- Influence of Better Student in Class
- Influence of Backbenchers in their Performance in Class.
- Significance of Medication in Class Performance
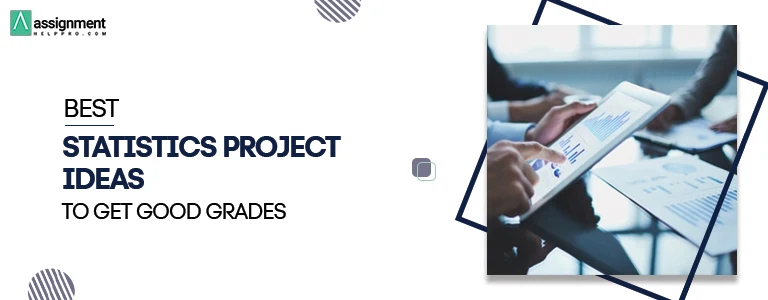
Business Statistics Project Ideas
- Accessibility of businesses to bank benefits
- The influence of social media on business sales
- The effect of social media on the performance of an employee
- Is consumption of alcohol higher among employees with a lower pay scale?
- The impact of cost estimation on business management
- Sexual harassment amongst female employees in the workplace
- Factors contributing to low productivity in a workplace
- Consideration of occupational schedules provided by secretaries
- Trends of death management in business entities
- Relationship between the leaders of a company as well as its employees
- The utilization of modern tools in any organization
- The effect of modern communication on the management of a company
- The importance of internal communication in a workplace
- Can business tools improve the performance of employees?
- The significance of assessment analysis.
- Correlation of business venture investment in the United States.
- Importance of online Business Performance for the impaired workers.
- Impact of the Facebook Marketing on Business Sales.
- Influence of Supervisors on the CEO of Business.
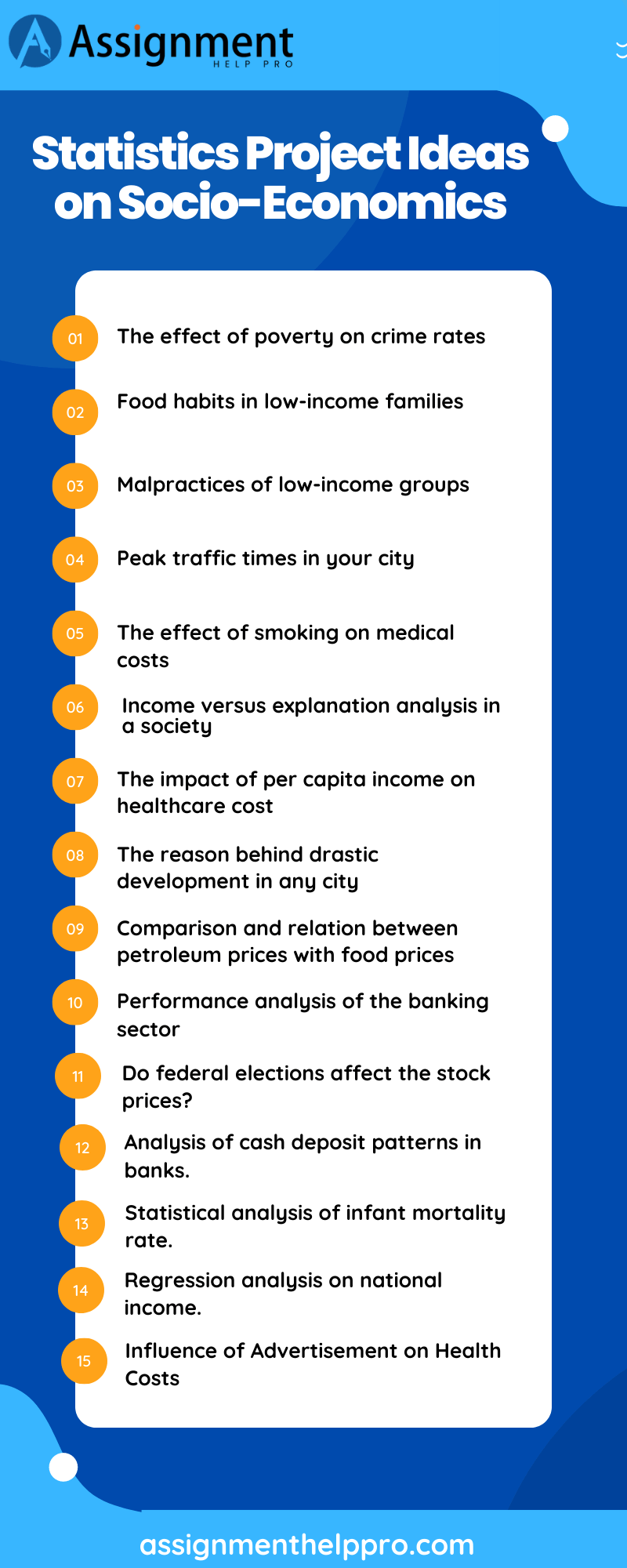
Statistics Project Ideas on Socio-Economics
- Significance of agricultural loans for farmers
- Comparison between criminal offenses in town as well as villages
- The effect of poverty on crime rates
- Food habits in low-income families
- Malpractices of low-income groups
- Income versus explanation analysis in a society
- Peak traffic times in your city
- Analysis of road accidents in the suburb as well as the town area
- The effect of smoking on medical costs
- Analysis of the source of revenue as well as the pattern of expenditure by the local government of the local government
- A relationship between exercises as well as a reduction in overall medical expenses
- The impact of per capita income on healthcare cost
- The reason behind drastic development in any city
- Comparison and relation between petroleum prices with food prices
- Why is it important to train the youth of low-income families?
Statistics Project Topics on Finance and Economics
- Can the growth of an organization in society make a difference in the economy of that community?
- Performance analysis of the banking sector
- Factors affecting financial distress in the banking sector
- Do federal elections affect stock prices?
- Do debt reduction policies of the government also reduce the quality of life?
- Analysis of cash deposit patterns in banks.
- Are computerized budget analysis systems effective?
- Statistical analysis of the impact of birth and death rates on the economy of a country
- Statistical analysis of infant mortality rate.
- Is there a relationship between exercise and a reduction in overall medical costs?
- The effects of poor infrastructural facilities on socio-economic development.
- Are members of certain subpopulations more likely to get the death penalty?
- Analysis of the use of financial reports in assessing the performance of banks.
- Analysis of the sources of revenue and the pattern of expenditure of the local government.
- Regression analysis on national income.
- Income vs Consumption Explanation Study in the Society.
- Influence of Advertisement on Health Costs
- Study of the Worldwide economic growth.
- Influence of Pandemic on Health in the UK
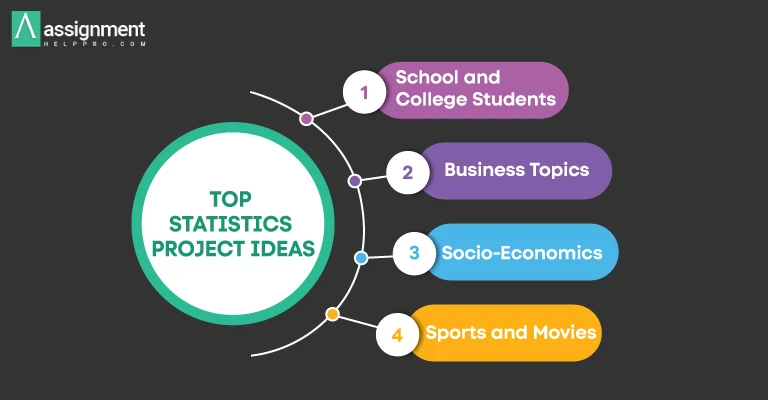
Statistical Analysis Topics on Sports and Movies
- Is there a relation between a basketball player as well as his height?
- Do sports affect the behavior of an individual?
- Do students get lower grades if they are involved in college sports?
- Comparison between hockey as well as basketball?
- Types of shoes worn by basketball players
- Are energy drinks harmful?
- The popularity of baseball as well as football
- Is the involvement of students in sports the reason behind lower grades?
- Statistical analysis of the types of brands endorsed by celebrity sportsmen.
- The revolution of cinema
- Why is there a demand for movie stars?
- Does the cast of a movie influence the interest of people?
- Do people enjoy movies more while eating?
- Do people enjoy movies more when they eat popcorn?
- What are the aspects of a successful movie?
- What are the qualities of a great movie?
- Do People enjoy commercials vs Art Movies?
- Do People in the East like Cricket more than People like Football in the West?
- Is Sports or Movies More Enjoyable?
Interesting Statistics Project Topics
- Examine the connection between mental health outcomes and social media usage.
- Examine the factors that influence voter participation in a particular election or region.
- Determine whether a particular marketing promotion strategy is working.
- Examine a specific species’ response to climate change.
- Analyze the connection between mental health outcomes and exercise.
- Examine the connection between a particular area’s health outcomes and income.
- Determine whether a particular program or intervention is successful in addressing a particular social problem.
- Examine the impact that COVID-19 has had on a particular sector of the economy or industry.
- Research the connection between a region’s economic conditions and crime rates.
- Investigate the factors that influence a student’s success in a specific academic program.
Other Popular Statistical Analysis Topics
- Are taller people considered to be more accurate?
- Analysis of the complexion of humans with their race
- Is mobile surfing helpful?
- Cause of aggression in male
- Are mobile games beneficial for students?
- Does the payroll affect the performance of an employee?
- Significance of health check-ups
- Analysis of people doing regular health check-ups versus those who do not
- Are people similar to the descriptions provided for their star signs?
- Statistical analysis of types of food teenagers consume and its consequences
- Analyze the consequences of cyberbullying
- Should art be given equal importance to science?
- Analyze the percentage of divorce rate in your country.
- Analyze the effects of overpopulation in small countries.
- Does academic success assure success in life?
- Why is there a command for film stars?
- The implication of physical condition checkups
- Examination of persons doing usual physical condition check-ups against those who do not
- Does the quality of a movie power the notice of people?
- Study of skin texture of humans with their race
- What seem to be the elements of a successful movie?
- Is success in sports have an influence on academic success?
Statistics Project Ideas for College Assignments
- Determine whether there is a correlation between having a part-time job and grades.
- Analyze whether there is a correlation between student debt and grades.
- Examine the GPAs of students who are from out of state to those who are inside the state.
- Determine whether there is a correlation between religious affiliation and grades.
- Analyze the academic performance of students who have completed an internship.
- Compare the test scores for students who have to work to support themselves vs. those who do not have to work.
- Analyze the GPAs of students who are married to those who are not married.
- Compare the efficiency of different study methods.
- Compare the test scores of African American students to Caucasian students.
- Investigate the correlation between intelligence and grades.
Out of the wide list of statistics project ideas suggested in this blog post, you can pick the topic of your choice. During the topic selection, keep in mind that the topic you select should have enough data to organize, analyze and interpret. Never pick a project idea that falls out of your interest. In case the topic you have selected is vague, then you can’t write a good hypothesis, and also it may result in low grades. So, spend some time and choose the best statistic project idea that is thought-provoking.
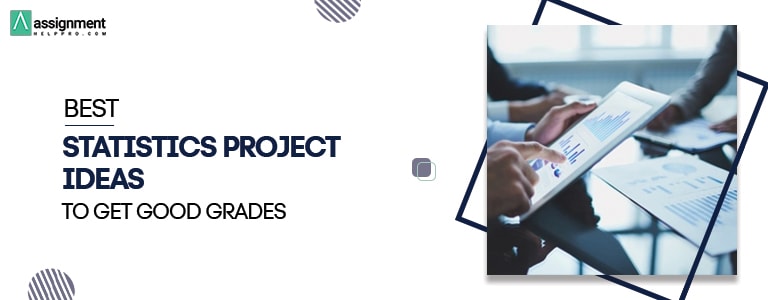
Related Post
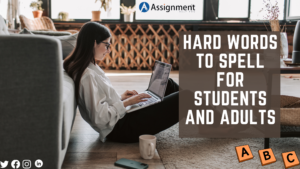
110 Hard Words to Spell for Students and Adults

Learn How to Avoid Passive Voice in 3 Simple Steps

117 Best Greek Mythology Essay Topics For Students
About author.
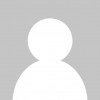
Jacob Smith
I am an Academic Writer and have affection to share my knowledge through posts’. I do not feel tiredness while research and analyzing the things. Sometime, I write down hundred of research topics as per the students requirements. I want to share solution oriented content to the students.
Leave a Reply Cancel reply
You must be logged in to post a comment.
- Featured Posts
140 Unique Geology Research Topics to Focus On
200+ outstanding world history topics and ideas 2023, 190 excellent ap research topics and ideas, 150+ trending group discussion topics and ideas, 170 funny speech topics to blow the minds of audience, who invented exams learn the history of examination, how to focus on reading 15 effective tips for better concentration, what is a rhetorical analysis essay and how to write it, primary school teacher in australia- eligibility, job role, career options, and salary, 4 steps to build a flawless business letter format, get help instantly.
Raise Your Grades with Assignment Help Pro
- Skip to main content
We use cookies
Necessary cookies.
Necessary cookies enable core functionality. The website cannot function properly without these cookies, and can only be disabled by changing your browser preferences.
Analytics cookies
Analytical cookies help us improve our website. We use Google Analytics. All data is anonymised.
Clarity helps us to understand our users’ behaviour by visually representing their clicks, taps and scrolling. All data is anonymised.
Privacy policy
School of Mathematics & Statistics
- Postgraduate research study
- Statistics Thesis Topics
- About the School
- Postgraduate Research Courses
- Mathematics Thesis Topics
Statistics thesis topics
Below are sample topics available for prospective postgraduate research students. These sample topics do not contain every possible project; they are aimed at giving an impression of the breadth of different topics available. Most prospective supervisors would be more than happy to discuss projects not listed below.
Funded projects are projects with project-specific funding. Funding for other projects is usally available on a competitive basis.
Modelling in Space and Time - Example Research Projects
Information about postgraduate research opportunities and how to apply can be found on the Postgraduate Research Study page . Below is a selection of projects that could be undertaken with our group.
Evaluating probabilistic forecasts in high-dimensional settings (PhD)
Supervisors: Jethro Browell Relevant research groups: Modelling in Space and Time , Computational Statistics , Applied Probability and Stochastic Processes
Many decisions are informed by forecasts, and almost all forecasts are uncertain to some degree. Probabilistic forecasts quantify uncertainty to help improve decision-making and are playing an important role in fields including weather forecasting, economics, energy, and public policy. Evaluating the quality of past forecasts is essential to give forecasters and forecast users confidence in their current predictions, and to compare the performance of forecasting systems.
While the principles of probabilistic forecast evaluation have been established over the past 15 years, most notably that of “ sharpness subject to calibration/reliability” , we lack a complete toolkit for applying these principles in many situations, especially those that arise in high-dimensional settings. Furthermore, forecast evaluation must be interpretable by forecast users as well as expert forecasts, and assigning value to marginal improvements in forecast quality remains a challenge in many sectors.
This PhD will develop new statistical methods for probabilistic forecast evaluation considering some of the following issues:
- Verifying probabilistic calibration conditional on relevant covariates
- Skill scores for multivariate probabilistic forecasts where “ideal” performance is unknowable
- Assigning value to marginal forecast improvement though the convolution of utility functions and Murphey Diagrams
- Development of the concept of “anticipated verification” and “predicting the of uncertainty of future forecasts”
- Decomposing forecast misspecification (e.g. into spatial and temporal components)
- Evaluation of Conformal Predictions
Good knowledge of multivariate statistics is essential, prior knowledge of probabilistic forecasting and forecast evaluation would be an advantage.
Adaptive probabilistic forecasting (PhD)
Supervisors: Jethro Browell Relevant research groups: Modelling in Space and Time , Computational Statistics , Applied Probability and Stochastic Processes
Data-driven predictive models depend on the representativeness of data used in model selection and estimation. However, many processes change over time meaning that recent data is more representative than old data. In this situation, predictive models should track these changes, which is the aim of “online” or “adaptive” algorithms. Furthermore, many users of forecasts require probabilistic forecasts, which quantify uncertainty, to inform their decision-making. Existing adaptive methods such as Recursive Least Squares, the Kalman Filter have been very successful for adaptive point forecasting, but adaptive probabilistic forecasting has received little attention. This PhD will develop methods for adaptive probabilistic forecasting from a theoretical perspective and with a view to apply these methods to problems in at least one application area to be determined.
In the context of adaptive probabilistic forecasting, this PhD may consider:
- Online estimation of Generalised Additive Models for Location Scale and Shape
- Online/adaptive (multivariate) time series prediction
- Online aggregation (of experts, or hierarchies)
A good knowledge of methods for time series analysis and regression is essential, familiarity with flexible regression (GAMs) and distributional regression (GAMLSS/quantile regression) would be an advantage.
The evolution of shape (PhD)
Supervisors: Vincent Macaulay Relevant research groups: Bayesian Modelling and Inference , Modelling in Space and Time , Statistical Modelling for Biology, Genetics and *omics
Shapes of objects change in time. Organisms evolve and in the process change form: humans and chimpanzees derive from some common ancestor presumably different from either in shape. Designed objects are no different: an Art Deco tea pot from the 1920s might share some features with one from Ikea in 2010, but they are different. Mathematical models of evolution for certain data types, like the strings of As, Gs , Cs and Ts in our evolving DNA, are quite mature and allow us to learn about the relationships of the objects (their phylogeny or family tree), about the changes that happen to them in time (the evolutionary process) and about the ways objects were configured in the past (the ancestral states), by statistical techniques like phylogenetic analysis. Such techniques for shape data are still in their infancy. This project will develop novel statistical inference approaches (in a Bayesian context) for complex data objects, like functions, surfaces and shapes, using Gaussian-process models, with potential application in fields as diverse as language evolution, morphometrics and industrial design.
New methods for analysis of migratory navigation (PhD)
Supervisors: Janine Illian Relevant research groups: Modelling in Space and Time , Bayesian Modelling and Inference , Computational Statistics , Environmental, Ecological Sciences and Sustainability
Joint project with Dr Urška Demšar (University of St Andrews)
Migratory birds travel annually across vast expanses of oceans and continents to reach their destination with incredible accuracy. How they are able to do this using only locally available cues is still not fully understood. Migratory navigation consists of two processes: birds either identify the direction in which to fly (compass orientation) or the location where they are at a specific moment in time (geographic positioning). One of the possible ways they do this is to use information from the Earth’s magnetic field in the so-called geomagnetic navigation (Mouritsen 2018). While there is substantial evidence (both physiological and behavioural) that they do sense magnetic field (Deutschlander and Beason 2014), we however still do not know exactly which of the components of the field they use for orientation or positioning. We also do not understand how rapid changes in the field affect movement behaviour.
There is a possibility that birds can sense these rapid large changes and that this may affect their navigational process. To study this, we need to link accurate data on Earth’s magnetic field with animal tracking data. This has only become possible very recently through new spatial data science advances: we developed the MagGeo tool, which links contemporaneous geomagnetic data from Swarm satellites of the European Space Agency with animal tracking data (Benitez Paez et al. 2021).
Linking geomagnetic data to animal tracking data however creates a highly-dimensional data set, which is difficult to explore. Typical analyses of contextual environmental information in ecology include representing contextual variables as co-variates in relatively simple statistical models (Brum Bastos et al. 2021), but this is not sufficient for studying detailed navigational behaviour. This project will analyse complex spatio-temporal data using computationally efficient statistical model fitting approches in a Bayesian context.
This project is fully based on open data to support reproducibility and open science. We will test our new methods by annotating publicly available bird tracking data (e.g. from repositories such as Movebank.org), using the open MagGeo tool and implementing our new methods as Free and Open Source Software (R/Python).
Benitez Paez F, Brum Bastos VdS, Beggan CD, Long JA and Demšar U, 2021. Fusion of wildlife tracking and satellite geomagnetic data for the study of animal migration. Movement Ecology , 9:31. https://doi.org/10.1186/s40462-021-00268-4
Brum Bastos VdS, Łos M, Long JA, Nelson T and Demšar U, 2021, Context-aware movement analysis in ecology: a systematic review. International Journal of Geographic Information Science , https://doi.org/10.1080/13658816.2021.1962528
Deutschlander ME and Beason RC, 2014. Avian navigation and geographic positioning. Journal of Field Ornithology , 85(2):111–133. https://doi.org/10.1111/jofo.12055
Integrated spatio-temporal modelling for environmental data (PhD)
Supervisors: Janine Illian Relevant research groups: Modelling in Space and Time , Bayesian Modelling and Inference , Computational Statistics , Environmental, Ecological Sciences and Sustainability
(Jointly supervised by Peter Henrys, CEH)
The last decade has seen a proliferation of environmental data with vast quantities of information available from various sources. This has been due to a number of different factors including: the advent of sensor technologies; the provision of remotely sensed data from both drones and satellites; and the explosion in citizen science initiatives. These data represent a step change in the resolution of available data across space and time - sensors can be streaming data at a resolution of seconds whereas citizen science observations can be in the hundreds of thousands.
Over the same period, the resources available for traditional field surveys have decreased dramatically whilst logistical issues (such as access to sites, ) have increased. This has severely impacted the ability for field survey campaigns to collect data at high spatial and temporal resolutions. It is exactly this sort of information that is required to fit models that can quantify and predict the spread of invasive species, for example.
Whilst we have seen an explosion of data across various sources, there is no single source that provides both the spatial and temporal intensity that may be required when fitting complex spatio-temporal models (cf invasive species example) - each has its own advantages and benefits in terms of information content. There is therefore potentially huge benefit in beginning together data from these different sources within a consistent framework to exploit the benefits each offers and to understand processes at unprecedented resolutions/scales that would be impossible to monitor.
Current approaches to combining data in this way are typically very bespoke and involve complex model structures that are not reusable outside of the particular application area. What is needed is an overarching generic methodological framework and associated software solutions to implement such analyses. Not only would such a framework provide the methodological basis to enable researchers to benefit from this big data revolution, but also the capability to change such analyses from being stand alone research projects in their own right, to more operational, standard analytical routines.
FInally, such dynamic, integrated analyses could feedback into data collection initiatives to ensure optimal allocation of effort for traditional surveys or optimal power management for sensor networks. The major step change being that this optimal allocation of effort is conditional on other data that is available. So, for example, given the coverage and intensity of the citizen science data, where should we optimally send our paid surveyors? The idea is that information is collected at times and locations that provide the greatest benefit in understanding the underpinning stochastic processes. These two major issues - integrated analyses and adaptive sampling - ensure that environmental monitoring is fit for purpose and scientists, policy and industry can benefit from the big data revolution.
This project will develop an integrated statistical modelling strategy that provides a single modelling framework for enabling quantification of ecosystem goods and services while accounting for the fundamental differences in different data streams. Data collected at different spatial resolutions can be used within the same model through projecting it into continuous space and projecting it back into the landscape level of interest. As a result, decisions can be made at the relevant spatial scale and uncertainty is propagated through, facilitating appropriate decision making.
Statistical methodology for assessing the impacts of offshore renewable developments on marine wildlife (PhD)
(jointly supervised by Esther Jones and Adam Butler, BIOSS)
Assessing the impacts of offshore renewable developments on marine wildlife is a critical component of the consenting process. A NERC-funded project, ECOWINGS, will provide a step-change in analysing predator-prey dynamics in the marine environment, collecting data across trophic levels against a backdrop of developing wind farms and climate change. Aerial survey and GPS data from multiple species of seabirds will be collected contemporaneously alongside prey data available over the whole water column from an automated surface vehicle and underwater drone.
These methods of data collection will generate 3D space and time profiles of predators and prey, creating a rich source of information and enormous potential for modelling and interrogation. The data present a unique opportunity for experimental design across a dynamic and changing marine ecosystem, which is heavily influenced by local and global anthropogenic activities. However, these data have complex intrinsic spatio-temporal properties, which are challenging to analyse. Significant statistical methods development could be achieved using this system as a case study, contributing to the scientific knowledge base not only in offshore renewables but more generally in the many circumstances where patchy ecological spatio-temporal data are available.
This PhD project will develop spatio-temporal modelling methodology that will allow user to anaylse these exciting - and complex - data sets and help inform our knowledge on the impact of off-shore renewable on wildlife.
Analysis of spatially correlated functional data objects (PhD)
Supervisors: Surajit Ray Relevant research groups: Modelling in Space and Time , Computational Statistics , Nonparametric and Semi-parametric Statistics , Imaging, Image Processing and Image Analysis
Historically, functional data analysis techniques have widely been used to analyze traditional time series data, albeit from a different perspective. Of late, FDA techniques are increasingly being used in domains such as environmental science, where the data are spatio-temporal in nature and hence is it typical to consider such data as functional data where the functions are correlated in time or space. An example where modeling the dependencies is crucial is in analyzing remotely sensed data observed over a number of years across the surface of the earth, where each year forms a single functional data object. One might be interested in decomposing the overall variation across space and time and attribute it to covariates of interest. Another interesting class of data with dependence structure consists of weather data on several variables collected from balloons where the domain of the functions is a vertical strip in the atmosphere, and the data are spatially correlated. One of the challenges in such type of data is the problem of missingness, to address which one needs develop appropriate spatial smoothing techniques for spatially dependent functional data. There are also interesting design of experiment issues, as well as questions of data calibration to account for the variability in sensing instruments. Inspite of the research initiative in analyzing dependent functional data there are several unresolved problems, which the student will work on:
- robust statistical models for incorporating temporal and spatial dependencies in functional data
- developing reliable prediction and interpolation techniques for dependent functional data
- developing inferential framework for testing hypotheses related to simplified dependent structures
- analysing sparsely observed functional data by borrowing information from neighbours
- visualisation of data summaries associated with dependent functional data
- Clustering of functional data
Estimating the effects of air pollution on human health (PhD)
Supervisors: Duncan Lee Relevant research groups: Modelling in Space and Time , Biostatistics, Epidemiology and Health Applications
The health impact of exposure to air pollution is thought to reduce average life expectancy by six months, with an estimated equivalent health cost of 19 billion each year (from DEFRA). These effects have been estimated using statistical models, which quantify the impact on human health of exposure in both the short and the long term. However, the estimation of such effects is challenging, because individual level measures of health and pollution exposure are not available. Therefore, the majority of studies are conducted at the population level, and the resulting inference can only be made about the effects of pollution on overall population health. However, the data used in such studies are spatially misaligned, as the health data relate to extended areas such as cities or electoral wards, while the pollution concentrations are measured at individual locations. Furthermore, pollution monitors are typically located where concentrations are thought to be highest, known as preferential sampling, which is likely to result in overly high measurements being recorded. This project aims to develop statistical methodology to address these problems, and thus provide a less biased estimate of the effects of pollution on health than are currently produced.
Mapping disease risk in space and time (PhD)
Disease risk varies over space and time, due to similar variation in environmental exposures such as air pollution and risk inducing behaviours such as smoking. Modelling the spatio-temporal pattern in disease risk is known as disease mapping, and the aims are to: quantify the spatial pattern in disease risk to determine the extent of health inequalities, determine whether there has been any increase or reduction in the risk over time, identify the locations of clusters of areas at elevated risk, and quantify the impact of exposures, such as air pollution, on disease risk. I am working on all these related problems at present, and I have PhD projects in all these areas.
Bayesian Mixture Models for Spatio-Temporal Data (PhD)
Supervisors: Craig Anderson Relevant research groups: Modelling in Space and Time , Bayesian Modelling and Inference , Biostatistics, Epidemiology and Health Applications
The prevalence of disease is typically not constant across space – instead the risk tends to vary from one region to another. Some of this variability may be down to environmental conditions, but many of them are driven by socio-economic differences between regions, with poorer regions tending to have worse health than wealthier regions. For example, within the the Greater Glasgow and Clyde region, where the World Health Organisation noted that life expectancy ranges from 54 in Calton to 82 in Lenzie, despite these areas being less than 10 miles apart. There is substantial value to health professionals and policymakers in identifying some of the causes behind these localised health inequalities.
Disease mapping is a field of statistical epidemiology which focuses on estimating the patterns of disease risk across a geographical region. The main goal of such mapping is typically to identify regions of high disease risk so that relevant public health interventions can be made. This project involves the development of statistical models which will enhance our understanding regional differences in the risk of suffering from major diseases by focusing on these localised health inequalities.
Standard Bayesian hierarchical models with a conditional autoregressive prior are frequently used for risk estimation in this context, but these models assume a smooth risk surface which is often not appropriate in practice. In reality, it will often be the case that different regions have vastly different risk profiles and require different data generating functions as a result.
In this work we propose a mixture model based approach which allows different sub-populations to be represented by different underlying statistical distributions within a single modelling framework. By integrating CAR models into mixture models, researchers can simultaneously account for spatial dependencies and identify distinct disease patterns within subpopulations.
Bayesian Modelling and Inference - Example Research Projects
Modelling genetic variation (msc/phd).
Supervisors: Vincent Macaulay Relevant research groups: Bayesian Modelling and Inference , Statistical Modelling for Biology, Genetics and *omics
Variation in the distribution of different DNA sequences across individuals has been shaped by many processes which can be modelled probabilistically, processes such as demographic factors like prehistoric population movements, or natural selection. This project involves developing new techniques for teasing out information on those processes from the wealth of raw data that is now being generated by high-throughput genetic assays, and is likely to involve computationally-intensive sampling techniques to approximate the posterior distribution of parameters of interest. The characterization of the amount of population structure on different geographical scales will influence the design of experiments to identify the genetic variants that increase risk of complex diseases, such as diabetes or heart disease.
The evolution of shape (PhD)
Supervisors: Vincent Macaulay Relevant research groups: Bayesian Modelling and Inference , Modelling in Space and Time , Statistical Modelling for Biology, Genetics and *omics
New methods for analysis of migratory navigation (PhD)
Integrated spatio-temporal modelling for environmental data (phd), statistical methodology for assessing the impacts of offshore renewable developments on marine wildlife (phd).
This PhD project will develop spatio-temporal modelling methodology that will allow user to anaylse these exciting - and complex - data sets and help inform our knowledge on the impact of off-shore renewable on wildlife.
Bayesian variable selection for genetic and genomic studies (PhD)
Supervisors: Mayetri Gupta Relevant research groups: Bayesian Modelling and Inference , Computational Statistics , Statistical Modelling for Biology, Genetics and *omics
An important issue in high-dimensional regression problems is the accurate and efficient estimation of models when, compared to the number of data points, a substantially larger number of potential predictors are present. Further complications arise with correlated predictors, leading to the breakdown of standard statistical models for inference; and the uncertain definition of the outcome variable, which is often a varying composition of several different observable traits. Examples of such problems arise in many scenarios in genomics- in determining expression patterns of genes that may be responsible for a type of cancer; and in determining which genetic mutations lead to higher risks for occurrence of a disease. This project involves developing broad and improved Bayesian methodologies for efficient inference in high-dimensional regression-type problems with complex multivariate outcomes, with a focus on genetic data applications.
The successful candidate should have a strong background in methodological and applied Statistics, expert skills in relevant statistical software or programming languages (such as R, C/C++/Python), and also have a deep interest in developing knowledge in cross-disciplinary topics in genomics. The candidate will be expected to consolidate and master an extensive range of topics in modern Statistical theory and applications during their PhD, including advanced Bayesian modelling and computation, latent variable models, machine learning, and methods for Big Data. The successful candidate will be considered for funding to cover domestic tuition fees, as well as paying a stipend at the Research Council rate for four years.
Bayesian statistical data integration of single-cell and bulk “OMICS” datasets with clinical parameters for accurate prediction of treatment outcomes in Rheumatoid Arthritis (PhD)
Supervisors: Mayetri Gupta Relevant research groups: Bayesian Modelling and Inference , Computational Statistics , Statistical Modelling for Biology, Genetics and *omics , Biostatistics, Epidemiology and Health Applications
In recent years, many different computational methods to analyse biological data have been established: including DNA (Genomics), RNA (Transcriptomics), Proteins (proteomics) and Metabolomics, that captures more dynamic events. These methods were refined by the advent of single cell technology, where it is now possible to capture the transcriptomics profile of single cells, spatial arrangements of cells from flow methods or imaging methods like functional magnetic resonance imaging. At the same time, these OMICS data can be complemented with clinical data – measurement of patients, like age, smoking status, phenotype of disease or drug treatment. It is an interesting and important open statistical question how to combine data from different “modalities” (like transcriptome with clinical data or imaging data) in a statistically valid way, to compare different datasets and make justifiable statistical inferences. This PhD project will be jointly supervised with Dr. Thomas Otto and Prof. Stefan Siebert from the Institute of Infection, Immunity & Inflammation ), you will explore how to combine different datasets using Bayesian latent variable modelling, focusing on clinical datasets from Rheumatoid Arthritis.
Funding Notes
The successful candidate will be considered for funding to cover domestic tuition fees, as well as paying a stipend at the Research Council rate for four years.
Computational Statistics - Example Research Projects
Supervisors: Jethro Browell Relevant research groups: Modelling in Space and Time , Computational Statistics , Applied Probability and Stochastic Processes
Supervisors: Jethro Browell Relevant research groups: Modelling in Space and Time , Computational Statistics , Applied Probability and Stochastic Processes
This project will develop an integrated statistical modelling strategy that provides a single modelling framework for enabling quantification of ecosystem goods and services while accounting for the fundamental differences in different data streams. Data collected at different spatial resolutions can be used within the same model through projecting it into continuous space and projecting it back into the landscape level of interest. As a result, decisions can be made at the relevant spatial scale and uncertainty is propagated through, facilitating appropriate decision making.
Statistical methodology for assessing the impacts of offshore renewable developments on marine wildlife (PhD)
Bayesian variable selection for genetic and genomic studies (phd), bayesian statistical data integration of single-cell and bulk “omics” datasets with clinical parameters for accurate prediction of treatment outcomes in rheumatoid arthritis (phd), analysis of spatially correlated functional data objects (phd), nonparametric and semi-parametric statistics - example research projects, modality of mixtures of distributions (phd).
Supervisors: Surajit Ray Relevant research groups: Nonparametric and Semi-parametric Statistics , Applied Probability and Stochastic Processes , Statistical Modelling for Biology, Genetics and *omics , Biostatistics, Epidemiology and Health Applications
Finite mixtures provide a flexible and powerful tool for fitting univariate and multivariate distributions that cannot be captured by standard statistical distributions. In particular, multivariate mixtures have been widely used to perform modeling and cluster analysis of high-dimensional data in a wide range of applications. Modes of mixture densities have been used with great success for organizing mixture components into homogenous groups. But the results are limited to normal mixtures. Beyond the clustering application existing research in this area has provided fundamental results regarding the upper bound of the number of modes, but they too are limited to normal mixtures. In this project, we wish to explore the modality of non-normal distributions and their application to real life problems.
Applied Probability and Stochastic Processes - Example Research Projects
Modality of mixtures of distributions (phd).
Finite mixtures provide a flexible and powerful tool for fitting univariate and multivariate distributions that cannot be captured by standard statistical distributions. In particular, multivariate mixtures have been widely used to perform modeling and cluster analysis of high-dimensional data in a wide range of applications. Modes of mixture densities have been used with great success for organizing mixture components into homogenous groups. But the results are limited to normal mixtures. Beyond the clustering application existing research in this area has provided fundamental results regarding the upper bound of the number of modes, but they too are limited to normal mixtures. In this project, we wish to explore the modality of non-normal distributions and their application to real life problems.
Machine Learning and AI - Example Research Projects
Medical image segmentation and uncertainty quantification (phd).
Supervisors: Surajit Ray Relevant research groups: Machine Learning and AI , Imaging, Image Processing and Image Analysis
This project focuses on the application of medical imaging and uncertainty quantification for the detection of tumours. The project aims to provide clinicians with accurate, non-invasive methods for detecting and classifying the presence of malignant and benign tumours. It seeks to combine advanced medical imaging technologies such as ultrasound, computed tomography (CT) and magnetic resonance imaging (MRI) with the latest artificial intelligence algorithms. These methods will automate the detection process and may be used for determining malignancy with a high degree of accuracy. Uncertainty quantification (UQ) techniques will help generate a more precise prediction for tumour malignancy by providing a characterisation of the degree of uncertainty associated with the diagnosis. The combination of medical imaging and UQ will significantly decrease the requirement for performing invasive medical procedures such as biopsies. This will improve the accuracy of the tumour detection process and reduce the duration of diagnosis. The project will also benefit from the development of novel image processing algorithms (e.g. deep learning) and machine learning models. These algorithms and models will help improve the accuracy of the tumour detection process and assist clinicians in making the best treatment decisions.
Generating deep fake left ventricles: a step towards personalised heart treatments (PhD)
Supervisors: Andrew Elliott , Vinny Davies , Hao Gao Relevant research groups: Machine Learning and AI , Emulation and Uncertainty Quantification , Biostatistics, Epidemiology and Health Applications , Imaging, Image Processing and Image Analysis
Personalised medicine is an exciting avenue in the field of cardiac healthcare where an understanding of patient-specific mechanisms can lead to improved treatments ( Gao et al., 2017 ). The use of mathematical models to link the underlying properties of the heart with cardiac imaging offers the possibility of obtaining important parameters of heart function non-invasively ( Gao et al., 2015 ). Unfortunately, current estimation methods rely on complex mathematical forward simulations, resulting in a solution taking hours, a time frame not suitable for real-time treatment decisions. To increase the applicability of these methods, statistical emulation methods have been proposed as an efficient way of estimating the parameters ( Davies et al., 2019 ; Noè et al., 2019 ). In this approach, simulations of the mathematical model are run in advance and then machine learning based methods are used to estimate the relationship between the cardiac imaging and the parameters of interest. These methods are, however, limited by our ability to understand the how cardiac geometry varies across patients which is in term limited by the amount of data available ( Romaszko et al., 2019 ). In this project we will look at AI based methods for generating fake cardiac geometries which can be used to increase the amount of data ( Qiao et al., 2023 ). We will explore different types of AI generation, including Generative Adversarial Networks or Variational Autoencoders, to understand how we can generate better 3D and 4D models of the fake left ventricles and create an improved emulation strategy that can make use of them.
Emulation and Uncertainty Quantification - Example Research Projects
Supervisors: Andrew Elliott , Vinny Davies , Hao Gao Relevant research groups: Machine Learning and AI , Emulation and Uncertainty Quantification , Biostatistics, Epidemiology and Health Applications , Imaging, Image Processing and Image Analysis
Environmental, Ecological Sciences and Sustainability - Example Research Projects
There is also the possibility of applying to The Leverhulme Programme for Doctoral Training in Ecological Data Science which is hosted in our school. Information on how to apply can be found on the programme's application page .
Statistical methodology for assessing the impacts of offshore renewable developments on marine wildlife (PhD)
Statistical modelling for biology, genetics and *omics - example research projects, modelling genetic variation (msc/phd).
Variation in the distribution of different DNA sequences across individuals has been shaped by many processes which can be modelled probabilistically, processes such as demographic factors like prehistoric population movements, or natural selection. This project involves developing new techniques for teasing out
Finite mixtures provide a flexible and powerful tool for fitting univariate and multivariate distributions that cannot be captured by standard statistical distributions. In particular, multivariate mixtures have been widely used to perform modeling and cluster analysis of high-dimensional data in a wide range of applications. Modes of mixture densities have been used with great success for organizing mixture components into homogenous groups. But the results are limited to normal mixtures. Beyond the clustering application existing research in this area has provided fundamental results regarding the upper bound of the number of modes, but they too are limited to normal mixtures. In this project, we wish to explore the modality of non-normal distributions and their application to real life problems
Implementing a biology-empowered statistical framework to detect rare varient risk factors for complex diseases in whole genome sequence cohorts (PhD)
Supervisors: Vincent Macaulay , Luísa Pereira (Geneticist, i3s ) Relevant research groups: Statistical Modelling for Biology, Genetics and *omics , Biostatistics, Epidemiology and Health Applications
The traditional genome-wide association studies to detect candidate genetic risk factors for complex diseases/phenotypes (GWAS) recur largely to the microarray technology, genotyping at once thousands or millions of variants regularly spaced across the genome. These microarrays include mostly common variants (minor allele frequency, MAF>5%), missing candidate rare variants which are the more likely to be deleterious [ 1 ]. Currently, the best strategy to genotype low-frequency (1%<MAF<5%) and rare (MAF<1%) variants is through next generation sequencing, and the increasingly availability of whole genome sequences (WGS) places us in the brink of detecting rare variants associated with complex diseases [ 2 ]. Statistically, this detection constitutes a challenge, as the massive number of rare variants in genomes (for example, 64.7M in 150 Iberian WGSs) would imply genotyping millions/billions of individuals to attain statistical power. In the last couple years, several statistical methods have being tested in the context of association of rare variants with complex traits [ 2 , 3 , 4 ], largely testing strategies to aggregate the rare variants. These works have not yet tested the statistical empowerment that can be gained by incorporating reliable biological evidence on the aggregation of rare variants in the most probable functional regions, such as non-coding regulatory regions that control the expression of genes [ 4 ]. In fact, it has been demonstrated that even for common candidate variants, most of these variants (around 88%; [ 5 ]) are located in non-coding regions. If this is true for the common variants detected by the traditional GWAS, it is highly probable to be also true for rare variants.
In this work, we will implement a biology-empowered statistical framework to detect rare variant risk factors for complex diseases in WGS cohorts. We will recur to the 200,000 WGSs from UK Biobank database [ 6 ], that will be available to scientists before the end of 2023. Access to clinical information of these >40 years old UK residents is also provided. We will build our framework around type-2 diabetes (T2D), a common complex disease for which thousands of common variant candidates have been found [ 7 ]. Also, the mapping of regulatory elements is well known for the pancreatic beta cells that play a leading role in T2D [ 8 ]. We will use this mapping in guiding the rare variants’ aggregation and test it against a random aggregation across the genome. Of course, the framework rationale will be appliable to any other complex disease. We will browse literature for aggregation methods available at the beginning of this work, but we already selected the method SKAT (sequence kernel association test; [ 3 ]) to be tested. SKAT fits a random-effects model to the set of variants within a genomic interval or biologically-meaningful region (such as a coding or regulatory region) and computes variant-set level p-values, while permitting correction for covariates (such as the principal components mentioned above that can account for population stratification between cases and controls).
Supervisors: Andrew Elliott , Vinny Davies , Hao Gao Relevant research groups: Machine Learning and AI , Emulation and Uncertainty Quantification , Biostatistics, Epidemiology and Health Applications , Imaging, Image Processing and Image Analysis
Biostatistics, Epidemiology and Health Applications - Example Research Projects
Bayesian statistical data integration of single-cell and bulk “omics” datasets with clinical parameters for accurate prediction of treatment outcomes in rheumatoid arthritis (phd).
Supervisors: Mayetri Gupta Relevant research groups: Bayesian Modelling and Inference , Computational Statistics , Vincent Macaulay , Biostatistics, Epidemiology and Health Applications
Supervisors: Andrew Elliott , Vinny Davies , Hao Gao Relevant research groups: Machine Learning and AI , Emulation and Uncertainty Quantification , Biostatistics, Epidemiology and Health Applications , Statistical Modelling for Biology, Genetics and *omics
Supervisors: Craig Anderson Relevant research groups: Modelling in Space and Time , Bayesian Modelling and Inference , Biostatistics, Epidemiology and Health Applications
Implementing a biology-empowered statistical framework to detect rare varient risk factors for complex diseases in whole genome sequence cohorts (PhD)
Supervisors: Vincent Macaulay , Luísa Pereira (Geneticist, i3s ) Relevant research groups: Statistical Modelling for Biology, Genetics and *omics , Biostatistics, Epidemiology and Health Applications
The traditional genome-wide association studies to detect candidate genetic risk factors for complex diseases/phenotypes (GWAS) recur largely to the microarray technology, genotyping at once thousands or millions of variants regularly spaced across the genome. These microarrays include mostly common variants (minor allele frequency, MAF>5%), missing candidate rare variants which are the more likely to be deleterious [ 1 ]. Currently, the best strategy to genotype low-frequency (1%<MAF<5%) and rare (MAF<1%) variants is through next generation sequencing, and the increasingly availability of whole genome sequences (WGS) places us in the brink of detecting rare variants associated with complex diseases [ 2 ]. Statistically, this detection constitutes a challenge, as the massive number of rare variants in genomes (for example, 64.7M in 150 Iberian WGSs) would imply genotyping millions/billions of individuals to attain statistical power. In the last couple years, several statistical methods have being tested in the context of association of rare variants with complex traits [ 2 , 3 , 4 ], largely testing strategies to aggregate the rare variants. These works have not yet tested the statistical empowerment that can be gained by incorporating reliable biological evidence on the aggregation of rare variants in the most probable functional regions, such as non-coding regulatory regions that control the expression of genes [ 4 ]. In fact, it has been demonstrated that even for common candidate variants, most of these variants (around 88%; [ 5 ]) are located in non-coding regions. If this is true for the common variants detected by the traditional GWAS, it is highly probable to be also true for rare variants.
Social and Urban Studies - Example Research Projects
Our group has an active PhD student community, and every year we admit new PhD students. We welcome applications from across the world. Further information can be found here .
Imaging, Image Processing and Image Analysis - Example Research Projects
Supervisors: Andrew Elliott , Vinny Davies , Hao Gao Relevant research groups: Machine Learning and AI , Emulation and Uncertainty Quantification , Biostatistics, Epidemiology and Health Applications , Imaging, Image Processing and Image Analysis
Statistics in Chemistry/Physics - Example Research Projects
Information about postgraduate research opportunities and how to apply can be found on the Postgraduate Research Study page . Projects will appear below here when they become available.
Statistics and Data Analytics Education - Example Research Projects
Our group has an active PhD student community, and every year we admit new PhD students. We welcome applications from across the world. Further information can be found here .
Thank you for visiting nature.com. You are using a browser version with limited support for CSS. To obtain the best experience, we recommend you use a more up to date browser (or turn off compatibility mode in Internet Explorer). In the meantime, to ensure continued support, we are displaying the site without styles and JavaScript.
- View all journals
- Explore content
- About the journal
- Publish with us
- Sign up for alerts
- Open access
- Published: 15 August 2024
What differentiates clinical trial statistics from preclinical methods and why robust approaches matter
Nature Communications volume 15 , Article number: 7035 ( 2024 ) Cite this article
Metrics details
Clinical trial statistics underlie the central decision-making process for whether a therapeutic approach can enter the clinic, but the nuances of this field may not be widely understood. Furthermore, how the statistics used in clinical trials differ from preclinical approaches and why they differ is not always clear. Here, three experts discuss the intricacies of clinical trial statistical planning and analysis as well as common issues that arise and emerging trends. The experts are Dr Tao Chen (Senior lecturer in Biostatistics at the Liverpool School of Tropical medicine), Professor Li Chao (Professor in Biostatistics at Xi’an Jiaotong University) and Professor Yang Wang (Professor in Biostatistics at the Chinese Academy of Medical Sciences and Peking Union Medical College). They have a diverse range of backgrounds across biostatistics and have been involved in numerous clinical trials of varying types.
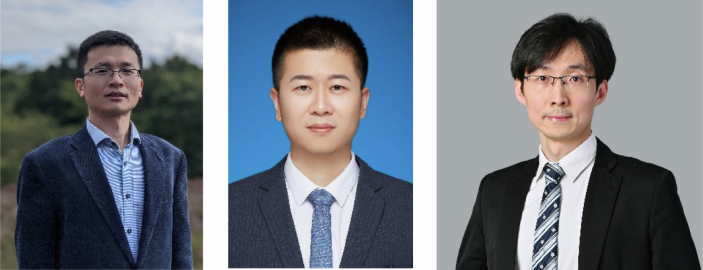
1. Statistics for clinical trials is a speciality in and of itself: could you please start by highlighting what differentiates clinical trial statistical approaches and practises from those applied in other areas of life sciences?
While statistics is a fundamental tool across all areas of life sciences, its application in clinical trials is characterized by the specific challenges and requirements associated with testing medical interventions in human populations. Key considerations that distinguish statistics applied to clinical trials include the incorporation of stringent ethical considerations to establish the balance between benefit and risk from the investigational treatment, the need for meticulous regulatory compliance (e.g., The International Council for Harmonisation of Technical Requirements for Pharmaceuticals for Human Use (ICH)), the systematic use of randomization and blinding to eliminate selection bias and confounding effects, the focus on clinically relevant outcomes directly related to the primary objective of the trial, a predetermined sample size calculation to ensure a study is sufficiently powered to assess the desired outcomes (an underpowered study, with limited sample size, may lead to false negative results), and prespecified statistical analysis plans to avoid selective reporting. Collectively, these elements aim to provide robust evidence regarding the safety and efficacy of medical interventions. Meanwhile, they also shape the statistical approaches in clinical trials, setting them apart from methodologies applied in other areas of life sciences research.
2. Clinical trials are separated into different phases and types, how do the statistics differ between phases or types?
Clinical trials are typically organized into different phases (e.g., Phase I to Phase IV) and types (e.g., exploratory trials looking at new effects and confirmatory trials to support previous results), which form a structured framework for evaluating the safety, efficacy, and effectiveness of medical treatments and guide the progression of treatments from initial testing to regulatory approval and post-market surveillance. As such, the emphasis on statistical aspects including sample size, study design, and choice of statistical methods varies to address the unique objectives and challenges of each phase of clinical trials.
For example, a dose escalation design with a small sample size of healthy volunteers is often employed to descriptively characterise the preliminary safety profile in phase I trials before advancing to subsequent phases of clinical trials. Whereas larger-scale trials with complex designs, may be used to confirm efficacy and safety assessments involving rigorous hypothesis testing - such as the establishment of clear and predefined hypotheses (e.g., testing for superiority or non-inferiority), selection of appropriate statistical test and control of type I error rate—These ultimately ensure the reliability and validity of study findings, leading to more informed decisions in clinical practice and healthcare policy.
3. Statistics play an important role in both the analysis and design of clinical trials, could you please define these different roles?
Statistics is integral to both the analysis and design of clinical trials. In the design phase, statistics is employed to plan and structure the trial to prevent bias to ensure validity, efficiency, and ethical conduct and optimize the chances of obtaining meaningful results. Various types of biases could be incurred if deviations from the protocol occurs. These include attrition bias due to differential loss of participants from different groups (e.g., a higher dropout rate in the control group) leading to biased estimates of treatment effects, ascertainment bias when knowing the treatment allocation prematurely, which inadvertently influence the assessment of outcomes (e.g., giving more attention or ancillary treatments/test for the subjects from intervention group), reporting bias introduced from outcome switching or failure to adjust for the multiplicity which can occur when multiple outcomes are being assessed, and selection bias when there is systematic differences in characteristics between groups being compared in a study(e.g., poor randomisation) as in these situations the probability of obtaining false positive associations increases.
In the analysis phase, the primary goal is to draw valid and reliable conclusions from the collected data, which involves assessing the efficacy and safety of the investigational treatment and making inferences from the trial population to the broader target population but with cautiousness. It remains imperative to consistently consider the prespecified analysis plan throughout the trial design process and ensure alignment with the overall trial design to maintain scientific integrity, prevent data fishing, facilitate interpretation and reproducibility, as well as comply with regulations and uphold ethical standards.
4. Could you please highlight some of the key issues with statistics in the design of trials that authors, reviewers, and editors should be aware of when assessing manuscripts?
Several critical issues related to statistics in the design of trials should be considered during the manuscript assessment process by authors, reviewers, and editors. These issues may include failure to prospectively register the trial, the absence of the pre-specified statistical analysis plan, ethical lapses, poor randomization, unclear allocation concealment (e.g., Interactive web response system to reduce the chance of guessing the randomisation sequence correctly), unjustified sample sizes calculation, inappropriate selection of endpoints that are not clinically relevant and validated, and imbalanced baseline characteristics or confounding factors between groups. Failure to comply with best practice in clinical trials would result in biased, unreliable, or misleading findings, compromising the validity, integrity, and ethical conduct of the research. For example, absence of a predefined analysis plan can lead to publication bias, selective reporting of outcomes, and incomplete dissemination of study results, hindering transparency and misleading conclusions.
5. Specifically, how does trial design influence whether data obtained from a clinical trial is meaningful?
The design exerts influence over various aspects of clinical trials. For instance, meaningful data relies on the careful selection of clinically relevant endpoints that directly measure the outcomes of interest. The use of inappropriate endpoints may lead to results that are inconclusive or even misleading. Similarly, the relevance of the data depends on how well the study population mirrors the target patient population. Excessive restrictions or inappropriate inclusion criteria have the potential to limit the generalizability of the results. Likewise, inadequate sample sizes can result in underpowered studies, diminishing the ability to detect true treatment effects and possibly yielding inconclusive or misleading results. Therefore, a well-thought-out design, aligned with study objectives, ethical standards, and robust statistical methodologies, enhances the validity and relevance of the trial outcomes.
6. It is well known that there are abundant issues with statistical analyses of preclinical studies, could you comment on how these preclinical issues carry over and affect clinical trials?
Issues stemming from preclinical study can significantly influence the planning, execution, and interpretation of subsequent clinical trials. These challenges are multifaceted, including the translatability of preclinical findings, biological variability between animal models and humans, lack of methodological rigors (e.g., randomisation and blinding), potential false positives from multiplicity, hypothesis driven research with small sample size and ethical concerns regarding the applicability of interventions to human subjects. The exploratory nature and challenges inherent in preclinical research findings increase the complexities and uncertainties while designing and analysing in further clinical trials. Therefore, by promoting transparency, judiciously leveraging information from preclinical studies and integrating this prior information into subsequent translational research through rigorous methodologies like Bayesian approaches, and fostering collaborations among researchers to which better align preclinical studies with real-world needs and maximize the translational potential of their findings, we can improve the reliability and translatability of findings throughout the translational process.
7. Could you please highlight some of the key issues with statistics in the analysis of trials that authors, reviewers, and editors should be aware of when assessing manuscripts?
Authors, reviewers, and editors must maintain vigilance to safeguard the credibility, transparency, and reliability of statistical analyses in clinical trial manuscripts. Key concerns over the statistical analysis particularly include the inconsistency between the statistical analysis plan and the reported analyses, departure from the original randomised assignment in the final analysis of a clinical trial, failure to adjust for multiple testing, inadequate handling of missing data, overemphasis on P-values, inappropriate subgroup analyses, outcome switching based on observed result, and change to the hypothesis (e.g., from non-inferiority to superiority) without pre-specification. These issues would introduce the risk of reporting or publication bias and erroneous conclusions. For example, overemphasis on p-values to draw conclusions without considering effect sizes, clinical significance, or context can lead to misinterpretation and undermine the reliability of study results.
Addressing these concerns by collaborating with statisticians at the early stage of the trial and maintaining adherence to methodological standards are essential for establishing robustness and integrity of clinical trial research.
8. Further to their implementation, clear and open reporting is vital for clinical trials: could you please comment on what are some of the major things that should be discussed and reported?
Comprehensive reporting allows researchers, clinicians, and the public to understand the study’s methods and results. Also, clear and open reporting ensures that the scientific community can critically evaluate the study, reproduce the research, and consider its findings in the broader context of existing evidence and the limitations of the trial design. The SPIRIT (Standard Protocol Items: Recommendations for Interventional Trials) guideline serves as a valuable resource for researchers and research teams to ensure that clinical trial protocols are comprehensive, transparent, and adhere to best practices with a set of recommendations, such as prospective trial registration, safety monitoring and study design. Correspondingly, researchers are encouraged to refer to the CONSORT (Consolidated Standards of Reporting Trials) and extensions (for specific types of trials, such as non-pharmacological interventions) for the complete checklist and guidance on reporting randomized controlled trials. Some key elements such as the strategies to control Type I error, maintenance of blindness, randomisation procedures, and justification for the non-inferiority margin-a predefined threshold used in non-inferiority clinical trials to determine whether a new treatment is not unacceptably worse than an active comparator treatment, should always be described in detail to strengthen the completeness and transparency of clinical trial reports.
9. In addition to their use in primary reporting of trials, statistical analysis of post-hoc trials is also important; could you please comment on the value of these analyses and describe how these approaches differ from the original analyses?
While post-hoc analyses can provide valuable insights and generate hypotheses for further exploration, it’s crucial to acknowledge their distinct characteristics and implications compared to the original analyses, necessitating a cautious approach. Generally, post-hoc analyses involve exploring data without predefined hypotheses, rendering them exploratory in nature and may be considered as supplementary or as validation for the original analysis. Additionally, statistical methods for post-hoc analysis may be less stringent, requiring adjustments for multiple comparisons to mitigate the increased risk of false positives. Overall, there is a risk of data-driven analyses, post hoc changes, or selective reporting of statistically significant results, leading to biased or misleading conclusions for post hoc analysis of trial data without a predefined analysis plan. Therefore, transparency regarding the exploratory nature of these analyses, stating them as such in the manuscripts, timing for conducting the analyses, appropriate statistical adjustments, and validation in independent studies are essential to ensure the reliability and robustness of the findings. Researchers should distinctly delineate between pre-specified analyses and post-hoc exploratory analyses when reporting trial results.
10. Finally, as with all fields of academia, statistics is constantly evolving and changing, could you please mention some of the changes that are happening currently which may influence clinical trial statistical design/analysis in the future?
Statistics, encompassing its application in clinical trial design and analysis, is continually evolving with ongoing technological advancements (e.g., artificial intelligence, digital health and telemedicine) and emerging changes (e.g., precision medicine) that are transforming the future of medicine and improving the quality of life for individuals. Several trends and developments are currently shaping the landscape of statistical approaches in clinical trials. Bayesian methodologies offer flexibility by incorporating prior information, updating analyses as data accumulate, and providing more informative posterior distributions after combining information from the observed data and any prior beliefs. Real-world evidence is gaining importance as it complements traditional clinical trial data, offering insights into long-term outcomes, patient perspectives, and treatment effectiveness in real-world settings. The integration of machine learning and artificial intelligence technologies holds promise for identifying intricate data patterns, optimizing patient recruitment strategies, and enhancing predictive modelling for patient outcomes. Precision medicine approaches, guided by genetic information, are advancing, leading to more targeted and personalized treatments. Clinical trial simulations are gaining recognition as an important component of clinical development programs, offering valuable insights to enhance understanding and inform decision-making at various stages of drug development. These trends collectively underscore the dynamic nature of statistical methodologies in clinical trials, driven by technological advancements, shifts in regulatory landscapes, and a commitment to improving the efficiency and ethical conduct of clinical trials.
Rights and permissions
Open Access This article is licensed under a Creative Commons Attribution-NonCommercial-NoDerivatives 4.0 International License, which permits any non-commercial use, sharing, distribution and reproduction in any medium or format, as long as you give appropriate credit to the original author(s) and the source, provide a link to the Creative Commons licence, and indicate if you modified the licensed material. You do not have permission under this licence to share adapted material derived from this article or parts of it. The images or other third party material in this article are included in the article’s Creative Commons licence, unless indicated otherwise in a credit line to the material. If material is not included in the article’s Creative Commons licence and your intended use is not permitted by statutory regulation or exceeds the permitted use, you will need to obtain permission directly from the copyright holder. To view a copy of this licence, visit http://creativecommons.org/licenses/by-nc-nd/4.0/ .
Reprints and permissions
About this article
Cite this article.
What differentiates clinical trial statistics from preclinical methods and why robust approaches matter. Nat Commun 15 , 7035 (2024). https://doi.org/10.1038/s41467-024-51486-4
Download citation
Published : 15 August 2024
DOI : https://doi.org/10.1038/s41467-024-51486-4
Share this article
Anyone you share the following link with will be able to read this content:
Sorry, a shareable link is not currently available for this article.
Provided by the Springer Nature SharedIt content-sharing initiative
Quick links
- Explore articles by subject
- Guide to authors
- Editorial policies
Sign up for the Nature Briefing newsletter — what matters in science, free to your inbox daily.

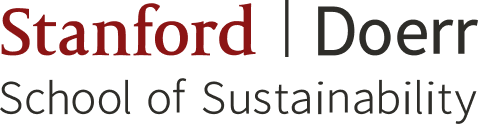
- Biodiversity
- Cities & society
- Land & water
- All research news
- All research topics
- Learning experiences
- Programs & partnerships
- All school news
- All school news topics
- In the media
- For journalists
New climate and sustainability research efforts will focus on eight ‘Solution Areas’
The Stanford Doerr School of Sustainability will establish new research initiatives under topics including climate, water, energy, food, nature, and cities.
The Stanford Doerr School of Sustainability has selected eight interconnected Solution Areas to focus its research efforts over the next decade. This new research plan amplifies the school’s ability to translate Stanford research into large-scale solutions and inform key decision makers in policy and business.
Selected based on extensive faculty input and assessment of where Stanford can make the most meaningful impact, the eight areas are: climate; water; energy; food; risk, resilience, and adaptation; nature; cities; and platforms and tools for monitoring and decision making.
“Solution Areas identify and leverage the critical junctions between the most pressing global sustainability challenges and the areas where Stanford has the talent and expertise to find solutions,” said Dean Arun Majumdar. “This collaborative all-campus approach expands and strengthens our commitment to using all the power we have – the knowledge, the education, the talent, the innovation, the resources, the influence – to build a thriving planet for future generations.”
‘Integrative Projects’ and ‘Flagship Destinations’
In each Solution Area, the school plans to build two types of research initiatives. One type, called Integrative Projects, will be managed by the school’s institutes, including the Stanford Woods Institute for the Environment , the Precourt Institute for Energy , and a planned Sustainable Societies Institute.
Integrative Projects will be organized around decade-long research themes and dedicated to creating solutions through interdisciplinary collaboration, engagement with partners beyond Stanford, identifying significant knowledge gaps, and understanding systems.
According to Chris Field , the Perry L. McCarty Director of the Stanford Woods Institute for the Environment and a professor in the Stanford Doerr School of Sustainability and the School of Humanities and Sciences , the new commitment to these areas “will provide both resources and coordination that expand Stanford faculty’s capacity to deliver sustainability solutions at scale.”
A second type of research initiative, called Flagship Destinations, is managed by Stanford’s Sustainability Accelerator . Flagship Destinations are targets for the pace and scale of work to address challenges facing Earth, climate, and society. For example, the school’s first Flagship Destination, announced in 2023 , calls for enabling the removal of billions of tons of planet-warming gases annually from Earth’s atmosphere by the middle of this century. By working backward from sustainability targets in consultation with faculty and external experts, this initiative seeks to rapidly translate Stanford research into policy and technology solutions. Additional Flagship Destinations will be announced later this week.
Whereas Integrative Projects are designed to produce knowledge and evidence that can eventually lead to solutions, Flagship Destination projects are intended to help verify and demonstrate that well-studied solutions can succeed at large scale so they can be launched out of Stanford and implemented for the benefit of humanity and our planet. Scalable solutions nurtured and launched through these projects could take the form of policy frameworks, open-source platforms, nonprofit organizations, new for-profit companies, and ongoing collaborations all committed to addressing pressing sustainability challenges.
“By working together in these Solution Areas across disciplines and with collaborators beyond the university, we maximize our ability to have positive impacts on the timeframe and scale needed for the planet and humanity,” said Scott Fendorf , senior associate dean for integrative initiatives and the Terry Huffington Professor in the Stanford Doerr School of Sustainability.
Workshops will be held with faculty and external experts to develop research strategies for each Solution Area on a rolling basis. Strategy workshops, opportunities to provide input on future Integrative Projects, and requests for proposals (open to all Stanford faculty) will be announced in the coming months.
Related message from leadership: Read a letter to faculty about the new Solution Areas from Dean Majumdar with Precourt Institute for Energy director William Chueh; Stanford Woods Institute for the Environment director Chris Field; Accelerator faculty director Yi Cui and executive director Charlotte Pera; and Integrative Initiatives associate dean Jenna Davis and senior associate dean Scott Fendorf.
Media Contacts
Josie garthwaite, explore more.

Stanford’s Sustainability Accelerator adds new targets
The Sustainability Accelerator in the Stanford Doerr School of Sustainability will support work in new areas including energy, climate adaptation, industry, and more.
- School planning

Solution Areas and research funding
A message from school leadership announcing solutions-oriented and scale-focused research funding opportunities to address pressing sustainability challenges.

Forecasting climate’s impact on a debilitating disease
In Brazil, climate and other human-made environmental changes threaten efforts to fight schistosomiasis, a widespread and debilitating parasitic disease. Stanford and Brazilian researchers have now developed models that can predict how disease risk will shift in response to environmental changes.
- Health and wellbeing

75+ Realistic Statistics Project Ideas For Students To Score A+
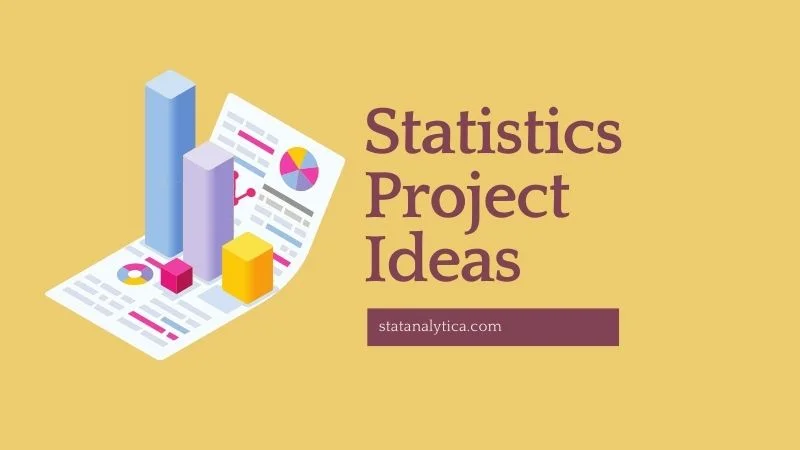
Statistics is one of the major subjects for every student, even in high school or college. These days almost every student is searching for the best, and more practical statistics project ideas. Even if you are a humanities, science or commerce student, you should have a good command of it.
Statistics has many sub-topics such as normal curves, regression, correlation, statistical inference, and many more. But keep in mind that the difficulty level of statistics varies from your study level. It means that statistics concepts can be more difficult for college students than for school students. It implies that statistical project topics would be different for college students and school students. On the other hand, if you are looking for statistics assignment help , then you can get the best assignment help from us.
But before we unveil these good statistics project ideas. Let’s understand what a statistical project is.
What is a Statistical Project?
Table of Contents
A statistical project is the best process of answering the research questions using statistical terminologies and techniques. It also helps us to present the work written in the given report. In statistical projects, the research could be on scientific or generic fields such as advertising, nutrition, and lots more. Therefore the difficulty level of statistical projects varies with research topics. And the statistics concepts also differ from one case to another. You can also visit statanalytica blogs to get assistance for statistical projects assignment idea.
What are Statistics Topics?
There are tons of topics in statistics. The most common statistics topics are normal curves, binomials, regression, correlation, permutation and combinations, statistical inference, and more. And all the statics topics are applicable in our daily life. Whether it is the tech or entertainment industry, everyone uses statistics topics.
Tips for finding easy statistics project ideas
Finding the best and easiest statistics project is not an easy task. But here are some of the best tips that will help you to find easy statistics project ideas:-
- Deeply analyze the data presented by the research
- Do you have an affirmative statement of the problems that have initiated the research?
- Study summary based on your research
- Have a deep discussion of the students’ design to clarify the problem.
All these steps will help you to find the best statistics project ideas. The next step is to write down the essential component of the statistics paper, i.e.:-
- Data analysis (by understanding the importance of data analytics projects )
- Statement of the problem
- Summary and conclusion
- Research design
Although if you follow these steps precisely, you will surely find the best project on statistics. But we are here to make it easy for you; let’s have a look at
Statistics Project Ideas for High School
Let’s find out the best statistics project ideas for high school that will help you to score good grades and showcase your skills:-
- Categorize the researched raw data into qualitative or quantitative
- Evaluate the published reports and graphs based on the analyzed data and conclude.
- Use dice to evaluate the bias and effect of completing data.
- Discuss the factors that can affect the result of the given survey data.
- Increasing use of plastic.
- Are e-books better than conventional books?
- Do extra-curricular activities help transform personalities?
- Should stereotypical social issues be highlighted or not?
- Should mobile phones be allowed in high schools or not?
- The Significance of Medication in Class Performance.
- Does the effect of a teacher who is a fresher at university influence the student’s performance?
- Influence of Distinct Subjects on Students’ Performance.
- Caffeine consumption among students as well as its effect on performance.
- Are online classes helpful?
- Influence of better students in class.
- The significance of the front seats in the class on success rates. Does an online brochure creator reduce marketing costs?
Additional statistics project examples:
The use of mobile phones in the classroom is always a debatable topic. Therefore, it is always a good statistics project idea to write statistics about how many students and teachers are in favor of using mobile phones in the classroom.
Small Business Statistics Project Topics
- The impact of the pandemic on small business survival rates.
- Analysis of the most profitable industries for small businesses.
- Small business failure rates by region and industry.
- The relationship between access to funding and small business success rates.
- The impact of social media marketing (SMM) on small business growth.
- The role of e-commerce in small business growth.
- The impact of government regulations on small business success rates.
- The gender gap in small business ownership and success rates.
- The impact of employee retention on small business growth and success rates.
- The relationship between small business growth and community development.
- The impact of the gig economy on small business growth.
- Analysis of the most common reasons for small business failure.
- The role of technology in small business growth and success rates.
- The impact of competition on small business survival rates.
- The relationship between small business ownership and educational attainment.
Statistics Project Ideas on Socio-Economics
- Income versus explanation analysis in society.
- Peak traffic times in your city.
- The significance of agricultural loans for farmers.
- Food habits in low-income families.
- Malpractices of low-income groups.
- Analysis of road accidents in the suburb and the town area.
- The effect of smoking on medical costs.
- Regression analysis on national income.
- Income vs Consumption Explanation Study in Society.
- A Study of the Worldwide Economic Growth
- The Influence of the Pandemic on Health in the UK
- Influence of Advertisement on Health Costs
- The effect of poverty on crime rates.
- Do federal elections affect stock prices?
Statistics Project Ideas for University Students (2023)
- Analyzing the impact of COVID-19 on a particular industry or economic sector.
- Examining the relationship between income and health outcomes in a particular population or geographic area.
- Investigate the factors influencing student success in a particular course or academic program.
- Analyzing the effectiveness of a specific marketing campaign or promotional strategy.
- Evaluating the relationship between social media usage and mental health outcomes.
- Examining the impact of climate change on a particular ecosystem or species.
- Investigating the factors influencing voter turnout in a particular election or geographic area.
- Analyzing the relationship between exercise and mental health outcomes.
- Evaluating the effectiveness of a particular intervention or program in addressing a specific social issue, such as poverty or homelessness.
- Examining the relationship between crime rates and economic conditions in a particular area.
Statistics Survey Project Ideas
Let’s find out some of the best statistics survey project ideas. Here we go:-
- Have a deep statistics analysis on the pollution level across various cities worldwide.
- Find out the most selling smartphones globally and used by college students.
- Do the behavioral survey of Omicron variant patients across the world.
- Conduct a survey about the global warming world.
Sometimes conducting a survey is itself a headache for you. That is why it is better to get easy statistics to project ideas. A survey report on E-books vs Textbooks is a good idea for students to conduct a survey and write down all useful insights collected from the survey report.
Statistics Project Ideas Hypothesis Testing
Statistics project ideas for hypothesis testing are not for everyone. But have a look at some of the best statistic project examples for hypothesis testing:-
- Peppermint essential oil affects the pangs of anxiety
- Immunity during winter for students who take more vitamin C than those who don’t.
- The productivity level of young boys as compared with the young girls.
- Obesity level of children whose parents are obese.
Hypothesis testing plays an important role in concluding the most estimated result of the experiment. That is why we always suggest students conduct the hypothesis test for the present situation. Like you consider the students’ choice regarding the subjects. And write the statistical factors, like whether students select their subject based on the industry’s stability or as per their liking.
AP Statistics Project Ideas
Let’s have a look at some of the AP statistics project ideas. If statistics are your primary subject, these projects will impact your grades.
- Find out the impact of school jobs and activities on the student’s overall grades.
- Who influences the children more on religious views, either the month or the father?
- Are age and sleeping related to each other, i.e., adult people tend to sleep less than kids and old-age citizens?
- Does plastic surgery change the perspective towards you the people?
To show the study of AP statistics project ideas, you need to offer arguments based on the evidence, perform research, and analyze the issues. You can write a statistics project based on alcohol advertisements and their effect on younger people of these ads.
Statistics Final Project Ideas
A massive number of students look for statistics and final project ideas. Have a look at some of the best final projects in statistics:-
- Do high heel sandals harm the body posture of the lady?
- Does the patient’s intelligence also affect the brilliance of the child?
- Is there any relation to eating hotdogs while watching a baseball match in the stadium?
- Does an opinion poll change the initially perceived election results?
If you are a final-year student looking for exciting project ideas, write a statistical report on the regression analysis. The analysis can be done on the national income, and you can put all the ins-outs on this topic with a detailed report.
Two variable statistics project Ideas
Have a look at the two-variable statistics project where one variable affects the other one:-
- Are electric cars a good choice to have control over global warming?
- Investing in FDIs can help the country to grow its GDP.
- Is lockdown the best solution to stop the spread of Coronavirus?
- Investing in cryptocurrency can have a significant impact on your future.
Statistics Project Ideas for College Students
There are tons of college statistics project examples. But we will share the best ideas for statistics projects for the college. As we have already discussed, college statistics project ideas are pretty complex compared with school-level projects. Let’s have a look at the best statistics project ideas for college:-
- Excessive use of the internet reduces the creativity and innovation skills of the students.
- The use of social media has bypassed studying in the students’ free time.
- Can college students develop drug habits if given a chance?
- Does a college freshman’s experience with their roommate affect their overall experience at the institution?
- A comparative study on the pricing of different clothing stores in your town.
- College students’ Web browsing habits.
- Comparison between male and female students in college.
- Statistical analysis of the highway accidents in your local neighborhood.
- Students in college choose common subjects.
- Choosing aspects of a subject in college.
- Course price differentiation in colleges.
- There is less interest in the students in humanities subjects as compared with science and technology.
- Relationship between birth order as well as academic success.
- Is being headstrong difficult, or does it make things easier?
- Popular movie genre among students in college.
- What kinds of music do college students like the most?
- Difference between the male and female population in a city based on their age.
- The Significance of Analytics in Studying Statistics
- Influence of backbenchers on their performance in class.
Fun Statistics Project Ideas
Have a look at some of the statistics projects examples:-
- Most of the volleyball players are tall compared with a few short ones.
- Men tend to have more interest in cricket as compared with females.
- Shorter and chubby girls are more friendly than tall and skinny girls.
- Aggression between students is based on the environment where they grew up.
- Students involved in co-curricular activities tend to have lower grades than those who don’t.
- Highly pressured employees consume more alcohol than those who do repetitive tasks jobs.
The Point With Statistics Projects Ideas
To write an impressive statistical project, you need to follow some points. Let’s have a look at these points:-
- Always work with organized information. If you get unorganized data, try to organize it first and then start working.
- Start with an outline, and it will help you to organize the final data of your statistics project. For this, you can also look at previous statistics project examples.
- Always write for the beginner’s audience. Don’t expect that your audience already knows everything. For this, be brief, simple, and to the point.
- Don’t miss the citation because it always helps showcase your projects’ authenticity. And keep the citation in the given format.
- The outcome of your statistical test should refer to the hypothesis being tested.
- If you have spent lots of time researching your project, you can take the help of statistics project writing services. For this, you can approach statistics homework help experts, and they will offer you the best statistics projects on your researched idea.
- Don’t get anxious while doing your statistics projects. Because most of the time, the professors give the research questions to the students. And the students need to collect, analyze, and interpret the information to provide the most suitable answer or conclusion to the question using statistical methods and techniques.
There are plenty of tons or even thousands of statistics project ideas to work on. But in this blog, I have mentioned some of the best and more realistic statistics project ideas. If you work on any of these ideas, you will not just get good grades but will also enjoy your project while working on it. As the quote said, “Do what you love, love what you do.”
Also, follow the steps mentioned at the end of the blog to finish up with the best-in-class statistics project. We have covered these ideas for almost every student. But still, if you are not able to find the best project for you, you should get in touch with our experts. Our team of experts will instantly get in touch with you and help you find the most suitable statistics project ideas for you.
Q1. What is meant by statistical project?
Statistics projects are a paper used to present the comprehension analysis of gathering statistical data. It contains the statistical data for the collected statistical data. In other words, it brings the significant results of a specific research question.
Q2. What are some practical uses for statistics in everyday life?
Many people use statistics to make decisions in budgeting and financial planning. On the other hand, most banks use statistics to lower the risk of lending operations, predict the impact of economic crises, and analyze activity in the financial market.
Related Posts
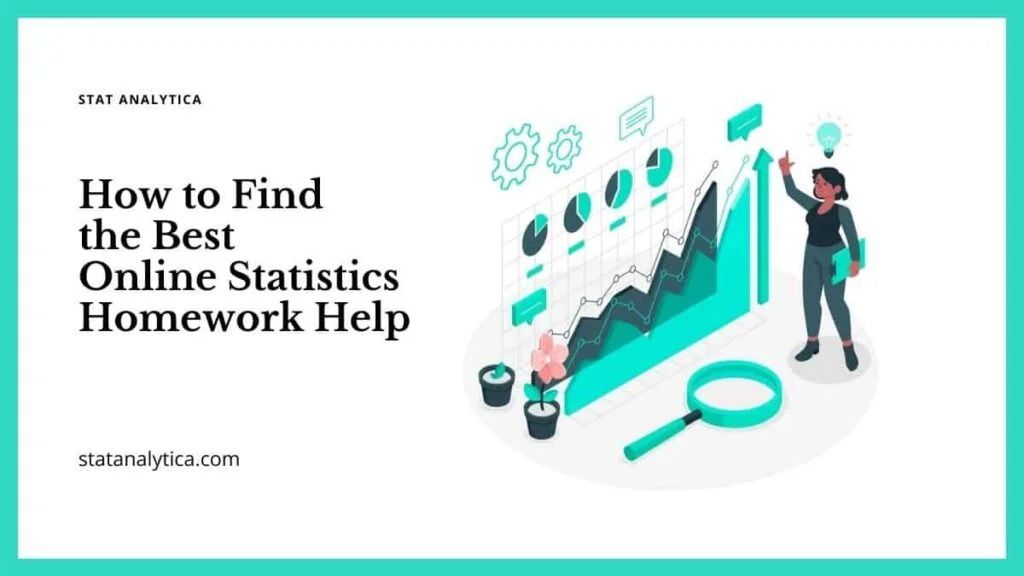
How to Find the Best Online Statistics Homework Help
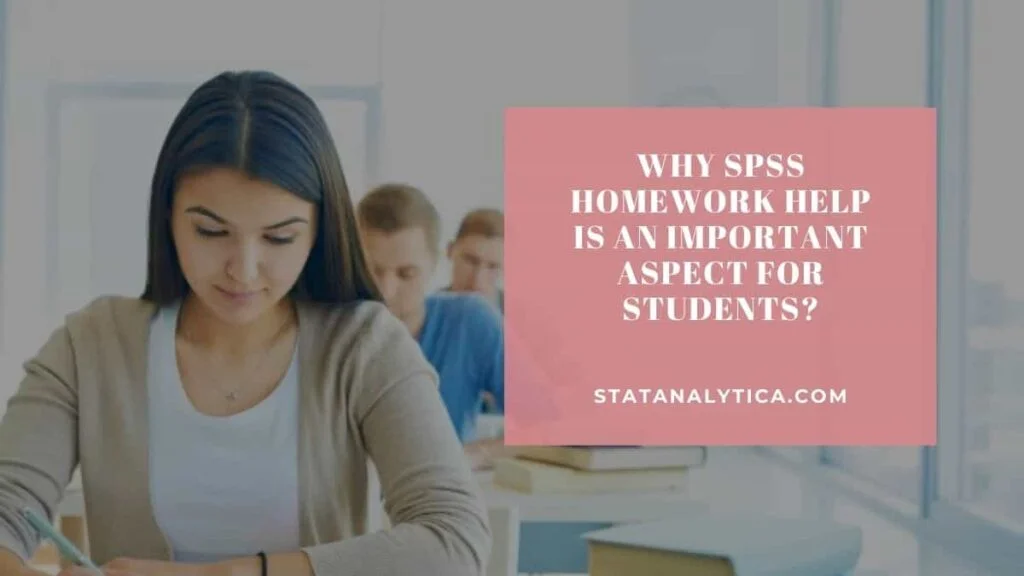
Why SPSS Homework Help Is An Important aspect for Students?
Numbers, Facts and Trends Shaping Your World
Read our research on:
Full Topic List
Regions & Countries
- Publications
- Our Methods
- Short Reads
- Tools & Resources
Read Our Research On:
Harris Energizes Democrats in Transformed Presidential Race
1. the presidential matchup: harris, trump, kennedy, table of contents.
- Other findings: Both Harris and Trump are viewed more favorably than a few months ago
- Voting preferences among demographic groups
- How have voters shifted their preferences since July?
- Harris’ supporters back her more strongly than Biden’s did last month
- Large gap in motivation to vote emerges between the candidates’ younger supporters
- Harris and Trump have gained ground with their own coalitions
- Share of ‘double negatives’ drops significantly with change in presidential candidates
- Views of Biden have changed little since his withdrawal from the 2024 presidential race
- Acknowledgments
- The American Trends Panel survey methodology
Nationally, Vice President Kamala Harris and former President Donald Trump are essentially tied among registered voters in the current snapshot of the presidential race: 46% prefer Harris, 45% prefer Trump and 7% prefer Robert F. Kennedy Jr.
Following Biden’s exit from the race, Trump’s support among voters has remained largely steady (44% backed him in July against Biden, while 45% back him against Harris today). However, Harris’ support is 6 percentage points higher than Biden’s was in July . In addition to holding on to the support of those who backed Biden in July, Harris’ bump has largely come from those who had previously said they supported or leaned toward Kennedy.
Harris performs best among the same demographic groups as Biden. But this coalition of voters is now much more likely to say they strongly support her: In July, 43% of Biden’s supporters characterized their support as strong – today, 62% of Harris’ do.

Overall, many of the same voting patterns that were evident in the Biden-Trump matchup from July continue to be seen today. Harris fares better than Trump among younger voters, Black voters, Asian voters and voters with college degrees. By comparison, the former president does better among older voters, White voters and voters without a college degree.
But Harris performs better than Biden across many of these groups – making the race tighter than it was just a few weeks ago.
- In July, women’s presidential preferences were split: 40% backed Biden, 40% preferred Trump and 17% favored Kennedy. With Harris at the top of the ticket, 49% of women voters now support her, while 42% favor Trump and 7% back Kennedy.
- Among men, Trump draws a similar level of support as he did in the race against Biden (49% today, compared with 48% in July). But the share of men who now say they support Harris has grown (to 44% today, up from 38% last month). As a result, Trump’s 10-point lead among men has narrowed to a 5-point lead today.
Race and ethnicity
Harris has gained substantial ground over Biden’s position in July among Black, Hispanic and Asian voters. Most of this movement is attributable to declining shares of support for Kennedy. Trump performs similarly among these groups as he did in July.
- 77% of Black voters support or lean toward Harris. This compares with 64% of Black voters who said they backed Biden a few weeks ago. Trump’s support is unchanged (13% then vs. 13% today). And while 21% of Black voters supported Kennedy in July, this has dropped to 7% in the latest survey.
- Hispanic voters now favor Harris over Trump by a 17-point margin (52% to 35%). In July, Biden and Trump were tied among Hispanic voters with 36% each.
- By about two-to-one, Asian voters support Harris (62%) over Trump (28%). Trump’s support among this group is essentially unchanged since July, but the share of Asian voters backing Harris is 15 points higher than the share who backed Biden in July.
- On balance, White voters continue to back Trump (52% Trump, 41% Harris), though that margin is somewhat narrower than it was in the July matchup against Biden (50% Trump, 36% Biden).
While the age patterns present in the Harris-Trump matchup remain broadly the same as those in the Biden-Trump matchup in July, Harris performs better across age groups than Biden did last month. That improvement is somewhat more pronounced among voters under 50 than among older voters.
- Today, 57% of voters under 30 say they support Harris, while 29% support Trump and 12% prefer Kennedy. In July, 48% of these voters said they backed Biden. Trump’s support among this group is essentially unchanged. And 12% now back Kennedy, down from 22% in July.
- Voters ages 30 to 49 are now about evenly split (45% Harris, 43% Trump). This is a shift from a narrow Trump lead among this group in July.
- Voters ages 50 and older continue to tilt toward Trump (50% Trump vs. 44% Harris).
With Harris now at the top of the Democratic ticket, the race has become tighter.
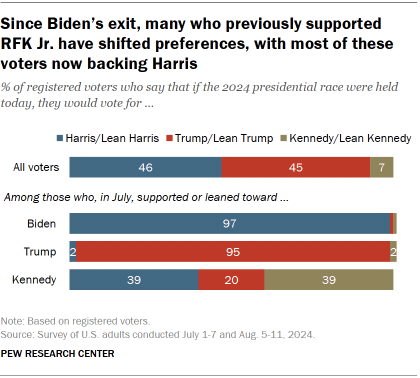
Much of this is the result of shifting preferences among registered voters who, in July, said they favored Kennedy over Trump or Biden.
Among the same group of voters surveyed in July and early August, 97% of those who backed Biden a few weeks ago say they support or lean toward Harris today. Similarly, Trump holds on to 95% of those who supported him a few weeks ago.
But there has been far more movement among voters who previously expressed support for Kennedy. While Kennedy holds on to 39% of those who backed him in July, the majority of these supporters now prefer one of the two major party candidates: By about two-to-one, those voters are more likely to have moved to Harris (39%) than Trump (20%). This pattern is evident across most voting subgroups.
In July, Trump’s voters were far more likely than Biden’s voters to characterize their support for their candidate as “strong” (63% vs. 43%). But that gap is no longer present in the Harris-Trump matchup.
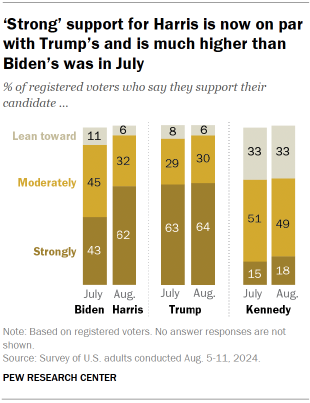
Today, 62% of Harris voters say they strongly support her, while about a third (32%) say they moderately support her. Trump’s voters are just about as likely to say they strongly back him today as they were in July (64% today, 63% then).
Kennedy’s voters make up a smaller share of voters today than a month ago – and just 18% of his voters say they strongly support him, similar to the 15% who said the same in July.
Across demographic groups, strong support for Harris is higher than it was for Biden
Among women voters who supported Biden in July, 45% said they did so strongly. That has grown to 65% today among women voters who support Harris.
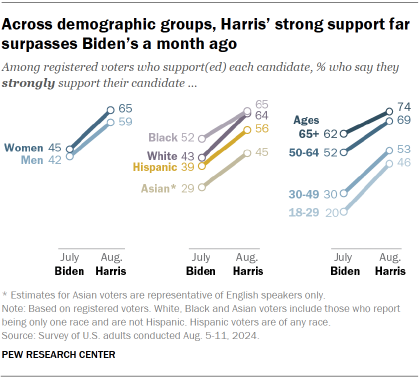
Increased intensity of support is similar among men voters who back the Democratic candidate: In July, 42% of men voters who supported Biden said they did so strongly. This has since grown to 59% of Harris’ voters who are men.
Across racial and ethnic groups, Harris’ supporters are more likely than Biden’s were to say they back their candidates strongly.
Among White voters, 43% who supported Biden in July did so strongly. Today, Harris’ strong support among White voters sits at 64%.
A near identical share of Harris’ Black supporters (65%) characterize their support for her as strong today. This is up from the 52% of Biden’s Black supporters who strongly backed him in July. Among Harris’ Hispanic supporters, 56% support her strongly, while 45% of Asian Harris voters feel the same. Strong support for Harris among these voters is also higher than it was for Biden in July.
Across all age groups, Harris’ strength of support is higher than Biden’s was. But the shift from Biden is less pronounced among older Democratic supporters than among younger groups.
Still, older Harris voters are more likely than younger Harris voters to describe their support as strong. For instance, 51% of Harris’ voters under 50 say they strongly support her, while 71% of Harris supporters ages 50 and older characterize their support as strong.
Today, about seven-in-ten of both Trump supporters (72%) and Harris supporters (70%) say they are extremely motivated to vote.
Motivation to vote is higher in both the Democratic and Republican coalitions than it was in July .
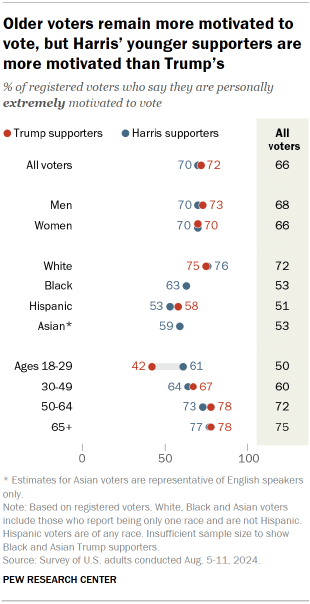
These shifts have occurred across groups but are more pronounced among younger voters.
Today, half of voters under 30 say they are extremely motivated to vote, up 16 points since July. Motivation is up 11 points among voters ages 30 to 49 and 50 to 64, and up 6 points among those ages 65 and older.
Among the youngest voters, the increased motivation to vote is nearly all driven by shifts among Democratic supporters.
- In July, 38% of 18- to 29-year-old Trump voters said they were extremely motivated to vote. Today, a similar share of his voters (42%) report that level of motivation.
- But 18- to 29-year-old Harris supporters are far more likely to say they are extremely motivated to vote than Biden’s supporters in this age group were about a month ago. Today, 61% of Harris’ voters under 30 say this. In July, 42% of voters under 30 who supported Biden said they were extremely motivated to vote.
Sign up for our weekly newsletter
Fresh data delivery Saturday mornings
Sign up for The Briefing
Weekly updates on the world of news & information
- Donald Trump
- Election 2024
- Kamala Harris
- More Leaders
- Political & Civic Engagement
Many Americans are confident the 2024 election will be conducted fairly, but wide partisan differences remain
Joe biden, public opinion and his withdrawal from the 2024 race, amid doubts about biden’s mental sharpness, trump leads presidential race, americans’ views of government’s role: persistent divisions and areas of agreement, cultural issues and the 2024 election, most popular, report materials.
- August 2024 Presidential Preference Detailed Tables
- Questionnaire
901 E St. NW, Suite 300 Washington, DC 20004 USA (+1) 202-419-4300 | Main (+1) 202-857-8562 | Fax (+1) 202-419-4372 | Media Inquiries
Research Topics
- Email Newsletters
ABOUT PEW RESEARCH CENTER Pew Research Center is a nonpartisan fact tank that informs the public about the issues, attitudes and trends shaping the world. It conducts public opinion polling, demographic research, media content analysis and other empirical social science research. Pew Research Center does not take policy positions. It is a subsidiary of The Pew Charitable Trusts .
© 2024 Pew Research Center
POV: Sexual Pleasure Should Be Integrated into Scientific Research on Sexual Health
Aside from disease prevention, elements of sexual health, including pleasure and orgasm, don’t receive much research funding, limiting what’s known.

Photo via iStock/Prostock-Studio
Julia Bond (SPH’24)
In a splashy piece in 2022, the New York Times detailed what many doctors and medical researchers know, but rarely talk about: we really do not know enough about the clitoris. This component of female anatomy has been routinely overlooked and ignored by the medical establishment, despite its substantial role in sexual pleasure for many people. When asked why, the medical experts interviewed in the piece shared the same sentiment: female sexual pleasure has long been viewed as secondary to male sexual pleasure. Unnecessary, even frivolous. Why, then, would this organ, perceived by many to play no role beyond orgasm, be suitable for serious scientific research? I believe the deep-rooted ignorance of the clitoris is a good example of the consequences of a larger, pervasive problem in the field of medical research: the routine dismissal of sexual pleasure as something worth studying.
This year, the National Institutes of Health (NIH), the largest funder of scientific research in the United States, will allocate an estimated $388 million towards the study of sexually transmitted diseases. This is a critical effort, particularly as cases of some sexually transmitted infections have increased in recent years. But the risk of sexually transmitted infections is just one component of sexual health. In 2022, the World Health Organization published an updated definition of sexual health, defining it as more than just the absence of disease. Their definition includes the “possibility of having pleasurable and safe sexual experiences.” Elements of sexual health beyond disease prevention, including pleasure, orgasm, or even pain, do not receive much research funding, which limits what is known.
In addition to little funding, sexual health research is also curtailed by scientists themselves. Researchers are impacted by cultural influences. Across different cultures, it is common for sex and sexual pleasure to be considered a taboo topic. Researchers’ discomfort with topics related to sexual pleasure poses real barriers to the meaningful study of sexual health. If someone is uncomfortable even saying the word “orgasm,” how can they effectively research the full range of human sexual experience?
The lack of scientific research focused on the positive elements of sexual behavior, including pleasure and orgasm, has real consequences. Beyond the ignorance of female sexual anatomy, research also suggests that public health programs that incorporate sexual pleasure might work better. Downplaying or ignoring pleasure may result in less successful efforts to promote sexual health. A narrow research focus on the risk of sexually transmitted infections can also obscure other important issues that are deeply relevant to people, including sexual dysfunction, which is estimated to affect 40 percent of women and 30 percent of men in the United States. The stigma against discussing sexual pleasure and pain also pervades medical care. Research shows that people experiencing sexual health issues often do not discuss them with their providers and providers do not routinely ask about them. This contributes to the often years-long wait that many people experiencing chronic pelvic pain conditions, like endometriosis, experience before receiving a diagnosis.
I believe that scientific and medical research that aims to improve sexual health should incorporate a sex-positive lens. By this, I mean taking into consideration the many reasons people may have sex, including interpersonal connection, pleasure, and joy. This is the focus of a recent commentary I published in the American Journal of Epidemiology , alongside Dr. Jessie Ford of Columbia Mailman School of Public Health. We emphasize that sexual pleasure is important to many people, and therefore should be integrated into scientific research on sexual health. This could help public health research be more aligned with people’s lived experiences, in which pleasure and connection are often central to sexual experiences. In an era where humans have gone to the moon, the intricacies of the clitoris should not be a mystery. Scientific researchers have the potential, and, I would argue, the responsibility, to help bring sexual pleasure out of the darkness, and into the light.
Julia Bond (SPH’24), a research associate in epidemiology at Boston Medical Center, can be reached at [email protected] .
“POV” is an opinion page that provides timely commentaries from students, faculty, and staff on a variety of issues: on-campus, local, state, national, or international. Anyone interested in submitting a piece, which should be about 700 words long, should contact John O’Rourke at [email protected] . BU Today reserves the right to reject or edit submissions. The views expressed are solely those of the author and are not intended to represent the views of Boston University.
Explore Related Topics:
- Public Health
- Sexual Health
- Share this story
- 2 Comments Add
Julia Bond (SPH’24) Profile
Julia Bond (SPH’24), a research associate in epidemiology at Boston Medical Center, can be reached at [email protected].
Comments & Discussion
Boston University moderates comments to facilitate an informed, substantive, civil conversation. Abusive, profane, self-promotional, misleading, incoherent or off-topic comments will be rejected. Moderators are staffed during regular business hours (EST) and can only accept comments written in English. Statistics or facts must include a citation or a link to the citation.
There are 2 comments on POV: Sexual Pleasure Should Be Integrated into Scientific Research on Sexual Health
I heartedly and fully agree with you.
Yes, yes, yes! Really nice piece, Julia!
Post a comment. Cancel reply
Your email address will not be published. Required fields are marked *
Latest from BU Today
10 memes to describe the first week of school, should health researchers ask if you’re a democrat or a republican, to do today: 14th annual african festival of boston, will the new massachusetts law on ghost guns, assault weapons, and gun-carrying pass legal muster, after historic year for bu athletics, what comes next, to do today: copley square farmers market, to do today: yappier hour at the liberty hotel, double chocolate banana pudding pie, to do today: river fit summer fitness series, university makes new contract offer to graduate students, to do today: get your groove on with the tito puente latin music series, questrom student helps women artisans in india become entrepreneurs, bu white coat ceremony welcomes 141 students to the profession of medicine, to do today: clue: a walking mystery, bu experts on boeing’s legal and technical troubles, what does tim walz bring to kamala harris’ ticket, to do today: boston parks movie nights, does it matter if boston mayor michelle wu doesn’t take maternity leave, to do today: explore the boston lights show at the franklin park zoo.
Physical Sciences Division
Gene mazenko, uchicago physicist and leading theorist in statistical mechanics, 1945–2024.
August 14, 2024
Remembered for humor, love of sports, and patient but rigorous mentorship
Gene Mazenko, Professor Emeritus in UChicago’s Department of Physics, the James Franck Institute, and the College, who focused on phase transitions and hydrodynamics of magnets, fluids, liquid crystals, and glasses, died in Antioch, CA, on July 7. He was 79.
Mazenko spent his career working with classical field theory techniques, studying tough problems in nonequilibrium statistical mechanics. The throughline of his work was understanding how fluctuations and disorder led to unexpected physics phenomena.
“He pioneered a powerful theoretical way of approaching a series of questions that had a great impact not only in his subfield of condensed matter physics but more generally to other areas of physics,” said Sidney Nagel, the Stein-Freiler Distinguished Service Professor in UChicago’s Department of Physics.
“Gene had a great instinct for knowing where the exciting problems lay among the many physical systems and models in the world of time-dependent statistical mechanics,” said Sriram Ramaswamy, PhD’83, Honorary Professor of Physics at the Indian Institute of Science and one of Mazenko’s former students. “He wasn’t given to quick hand-waving arguments though; he loved technique and hard calculational detail.”
“A born leader”
Mazenko was born July 5, 1945, in the small coal-mining town of Coalport, PA, and moved with his family to a small city east of Los Angeles when he was eight. In high school, he excelled equally in academics, where he was a member of the Math Club, and in sports, playing baseball (third base) and football (quarterback), becoming captain of both teams.
Mazenko received his BS from Stanford University in 1967 and his PhD from MIT in 1971, where he applied classical field theory techniques to the problem of sound attenuation in a dilute gas. As a postdoctoral research associate at Brandeis in 1971–72, he developed Fully Renormalized Kinetic Theory or FRKT, a modern approach to kinetic theory using operator methods. He then collaborated with researchers at Harvard and MIT to further develop these techniques in 1972–73 before returning to Stanford for a final postdoctoral fellowship to study the critical dynamics of isotropic ferromagnets.
Mazenko arrived at the University of Chicago in December 1974, where he applied the techniques developed earlier in his career to study the dynamics of glassy systems, nonlinear hydrodynamics, vortices, and liquid crystals.
“Gene Mazenko was a born leader—both in his physics and administrative capacity,” said Nagel . “Gene, more than anyone else, helped rebuild the condensed matter effort at UChicago. Both as a member of this group and as the Director of the James Franck Institute for six years, he was instrumental in hiring so many of our colleagues who are still here today.”
His leadership expanded beyond the Physical Sciences Division. From 1992 to 1995, Mazenko served as Special Assistant to the Provost and then as Associate Provost. During that time, he oversaw the University library system, campus-wide computing resources, and research administration.
When Mazenko returned to teaching and research, he focused on the theory of the growth of order in quenched systems and the kinetics of fluid systems. In the early 2000s, he wrote a series of graduate-level textbooks: Equilibrium Statistical Mechanics , Nonequilibrium Statistical Mechanics , and Fluctuations, Order and Defects . This work led him to develop a new fundamental theory of strongly interacting particle systems, which he applied to liquid-to-glass transitions. He retired as Professor Emeritus in 2015.
Independent thinkers
Mazenko trained many graduate students and postdocs who went on to positions worldwide and who continue to use the skills and knowledge they learned under his mentorship.
Robert Wickham, PhD’97, an associate professor in physics at the University of Guelph, highlights Mazenko’s significant impact on his career: “Gene took seriously the idea at Chicago that the thesis work should be the student’s alone.” He appreciated Mazenko’s hands-off approach for students’ final PhD years, which fostered independent research skills.
David McCowan, PhD’14, a laboratory instructor in UChicago’s Physics Department, recalls that “Gene was no-nonsense. He spoke his mind and would be forceful when needed.” Initially intimidated by Gene’s seriousness, McCowan soon discovered a patient, kind, and warm mentor, with “a dry sense of humor and a deadpan delivery that was so subtle you could miss the joke until it hit you a few minutes later.”
Shankar Prasad Das, PhD’86, professor of physics at Jawaharlal Nehru University, emphasizes Mazenko’s commitment to doing science on his own terms. “He took utmost care in this process, spared no effort, and lacked no energy to fully grasp what was being said,” said Das. “After going through that, when he took a stand, he would stand firm. And he was never hesitant to stand alone!”
“Gene expected the best from his colleagues and students,” said Woowon Kang, UChicago professor of physics. “But while he held himself and others to high standards, he was practical enough to make compromises when necessary.”
Lifelong pursuits
While Mazenko was distinctly dedicated to his academic career, his interests beyond science are well remembered. Mazenko was a long-distance runner, competing in the Chicago Marathon, and a dutiful fan of the Chicago Bears and Bulls, as well as the Celtics, from when he lived near Boston. Das recalls attending the 2015 symposium in honor of Mazenko’s retirement, when his former mentor told Das’s son, “Unlike your father, I hope you take an interest in sports,” before discussing cricket.
He loved movies and a broad range of music types, including classical and rock, specifically the Beach Boys and Steely Dan, according to his sister. Wickham recalls Mazenko listening to Enya in his office.
“Gene spent his life doing what he loved,” said nephew Mark Mazenko. “I hope the same can be said of me and of us all.”
Mazenko was preceded in death by his parents, Edward Andrew Stanislaus Mazenko and Margaret Dawn (Jeffries) Mazenko, and his wife, Judith Oakley Mazenko. He is survived by siblings Donald “Edward” Mazenko, Darrel John Mazenko, and Deborah Ann (Mazenko) Jeffries; 10 nieces and nephews; and 74 first cousins.
Related News
Faculty , Newsclips
News Topics
- PSD Spotlights
- Student Awards
- Faculty Awards
- PSD in Practice
News Archive
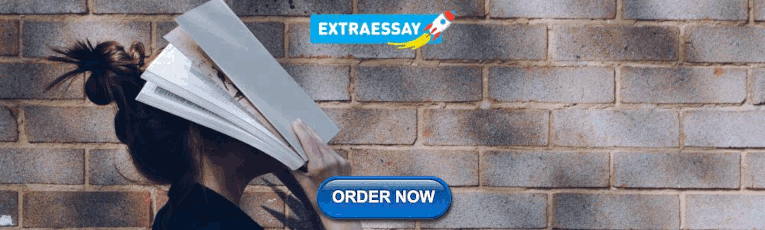
IMAGES
COMMENTS
If we talk about the interesting research topics in statistics, it can vary from student to student. But here are the key topics that are quite interesting for almost every student:-. Literacy rate in a city. Abortion and pregnancy rate in the USA. Eating disorders in the citizens.
500+ Statistics Research Topics. March 25, 2024. by Muhammad Hassan. Statistics is a branch of mathematics that deals with the collection, analysis, interpretation, presentation, and organization of data. It is a fundamental tool used in various fields such as business, social sciences, engineering, healthcare, and many more.
Here are some of the best statistical research topics worth writing on: Predictive Healthcare Modeling with Machine Learning. Analyzing Online Education During COVID-19 Epidemic. Modeling How Climate Change Affects Natural Disasters. Essential Elements Influencing Personnel Productivity. Social Media Influence on Customer Choices and Behavior.
Statistics is the application of mathematical concepts to understanding and analysing large collections of data. A central tenet of statistics is to describe the variations in a data set or ...
Statistics Research Topics in Business. Understanding the factors that influence consumer purchase decisions in the technology industry. Advertising and sales revenue: a time-series analysis. The effectiveness of customer loyalty programs in increasing customer retention and revenue.
Table of contents. Step 1: Write your hypotheses and plan your research design. Step 2: Collect data from a sample. Step 3: Summarize your data with descriptive statistics. Step 4: Test hypotheses or make estimates with inferential statistics.
Applied Statistics Research Topic. - Does education have an impact on financial success of individual? - The financial wealth gap between rich & poor - Statistics analysis to know multi-agent model impact.
This book aims to help you understand and navigate statistical concepts and the main types of statistical analyses essential for research students. ... The textbook covers all necessary areas and topics for students who want to conduct research in statistics. It includes foundational concepts, application methods, and advanced statistical ...
1. Introduction. Statistics and data science are more popular than ever in this era of data explosion and technological advances. Decades ago, John Tukey (Brillinger, 2014) said, "The best thing about being a statistician is that you get to play in everyone's backyard."More recently, Xiao-Li Meng (2009) said, "We no longer simply enjoy the privilege of playing in or cleaning up everyone ...
1.2 Statistics Project Topics for High School Students. 1.3 Statistical Survey Topics. 1.4 Statistical Experiment Ideas. 1.5 Easy Stats Project Ideas. 1.6 Business Ideas for Statistics Project. 1.7 Socio-Economic Easy Statistics Project Ideas. 1.8 Experiment Ideas for Statistics and Analysis. 2 Conclusion: Navigating the World of Data Through ...
Here are some potential statistics research topics in the field of social sciences: Study of crime rates and factors that contribute to criminal behavior. Researchers can use statistical methods to analyze crime rates and identify the factors that contribute to criminal behavior, such as poverty, unemployment, and education levels.
Quantitative research involves collecting and analyzing numerical data to identify patterns, trends, and relationships among variables. This method is widely used in social sciences, psychology, economics, and other fields where researchers aim to understand human behavior and phenomena through statistical analysis. If you are looking for a quantitative research topic, there are numerous areas ...
Finally, we can connect research in statistical methods to trends in the application of statistics within science and engineering. An entire series of articles could be written just on this topic. Here, we mention one such area, the replication crisis or reproducibility revolution in biology, psychology, economics, and other sciences.
2019. - "Density representations for words and hierarchical data". - "High dimensional data analysis with dependency and under limited memory". Dissertation Advisor: Sumanta Basu and Madeleine Udell. - "Functional single index model and jensen effect". - "Interpretable approaches to opening up black-box models".
Top 99+ Trending Statistics Research Topics for Students; See also 10 Different Options for Extracting Data from a PDF File: ... How to Find Statistical Topics for your Paper. Statistics is a discipline that involves collecting, analyzing, organizing, presenting, and interpreting data. If you are looking for the right topic for your work, here ...
Covers all topics of modern data science, such as frequentist and Bayesian design and inference as well as statistical learning. Contains original research papers (regular articles), survey articles, short communications, reports on statistical software, and book reviews. High author satisfaction with 90% likely to publish in the journal again.
Statistical Research Topics for College Students. Of course, we have plenty of statistical research topics for college students. These are more difficult than those for high school students, but they should be manageable: Analyze John Tukey's contribution to statistics; Florence Nightingale and visual representation in statistics
Five Examples of Statistical Research Questions. In writing the statistical research questions, I provide a topic that shows the variables of the study, the study description, and a link to the original scientific article to give you a glimpse of the real-world examples. Topic 1: Physical Fitness and Academic Achievement
Statistics Project Ideas on Socio-Economics. Significance of agricultural loans for farmers. Comparison between criminal offenses in town as well as villages. The effect of poverty on crime rates. Food habits in low-income families. Malpractices of low-income groups. Income versus explanation analysis in a society.
Statistics thesis topics. Below are sample topics available for prospective postgraduate research students. These sample topics do not contain every possible project; they are aim
Big Idea 1: Aggregation; Big Idea 2: Variation; Big Idea 3: Modelling; Big Idea 4: Uncertainty; Big Idea 5: Sampling from a Population; Now that you've understood some of the problems we usually come across when conducting statistics and interpreting the results, let's look at the main ideas that cut through nearly all aspects of statistical thinking.
Clinical trial statistics underlie the central decision-making process for whether a therapeutic approach can enter the clinic, but the nuances of this field may not be widely understood.
Research shows statistical analysis can detect when ChatGPT is used to cheat on multiple-choice chemistry exams. ScienceDaily . Retrieved August 16, 2024 from www.sciencedaily.com / releases ...
The Stanford Doerr School of Sustainability has selected eight interconnected Solution Areas to focus its research efforts over the next decade. This new research plan amplifies the school's ability to translate Stanford research into large-scale solutions and inform key decision makers in policy and business.
It also helps us to present the work written in the given report. In statistical projects, the research could be on scientific or generic fields such as advertising, nutrition, and lots more. Therefore the difficulty level of statistical projects varies with research topics. And the statistics concepts also differ from one case to another.
The survey explores how families are doing by asking about the health and development of their child, access to childcare, and other topics. The Child Wellness Survey (CWS) is a survey for families with children aged 6 months to 11 years across Washington state that started in 2024.
Nationally, Vice President Kamala Harris and former President Donald Trump are essentially tied among registered voters in the current snapshot of the presidential race: 46% prefer Harris, 45% prefer Trump and 7% prefer Robert F. Kennedy Jr. Following Biden's exit from the race, Trump's support ...
Epidemiologist Julia Bond argues that definitions of sexual health—and research funding—should be expanded to include more than just the absence of STDs. ... it is common for sex and sexual pleasure to be considered a taboo topic. Researchers' discomfort with topics related to sexual pleasure poses real barriers to the meaningful study of ...
When Mazenko returned to teaching and research, he focused on the theory of the growth of order in quenched systems and the kinetics of fluid systems. In the early 2000s, he wrote a series of graduate-level textbooks: Equilibrium Statistical Mechanics, Nonequilibrium Statistical Mechanics, and Fluctuations, Order and Defects.
Topics. Introduction to Transportation Statistics; ... Bureau of Transportation Statistics. 1200 NEW JERSEY AVENUE, SE. WASHINGTON, DC 20590. 800-853-1351. Subscribe to email updates. About. About BTS; Contact Us; FAQs; Jobs and Internships; DOT Research and Technology (OST-R) Resources. Ask a Librarian; BTS Open Data Catalog; National ...