Thank you for visiting nature.com. You are using a browser version with limited support for CSS. To obtain the best experience, we recommend you use a more up to date browser (or turn off compatibility mode in Internet Explorer). In the meantime, to ensure continued support, we are displaying the site without styles and JavaScript.
- View all journals
- Explore content
- About the journal
- Publish with us
- Sign up for alerts
- Perspective
- Published: 23 December 2021
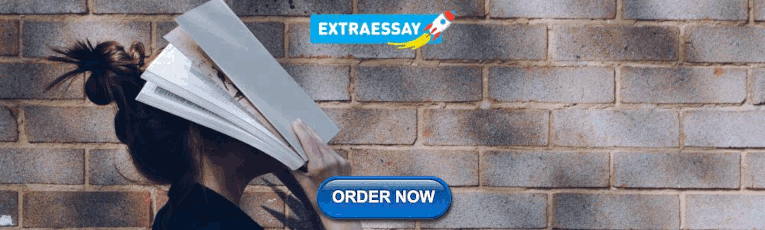
On-Farm Experimentation to transform global agriculture
- Myrtille Lacoste ORCID: orcid.org/0000-0001-6557-1865 1 , 2 ,
- Simon Cook ORCID: orcid.org/0000-0003-0902-1476 1 , 3 ,
- Matthew McNee 4 ,
- Danielle Gale ORCID: orcid.org/0000-0003-3733-025X 1 ,
- Julie Ingram ORCID: orcid.org/0000-0003-0712-4789 5 ,
- Véronique Bellon-Maurel 6 , 7 ,
- Tom MacMillan ORCID: orcid.org/0000-0002-2893-6981 8 ,
- Roger Sylvester-Bradley 9 ,
- Daniel Kindred ORCID: orcid.org/0000-0001-7910-7676 9 ,
- Rob Bramley ORCID: orcid.org/0000-0003-0643-7409 10 ,
- Nicolas Tremblay ORCID: orcid.org/0000-0003-1409-4442 11 ,
- Louis Longchamps ORCID: orcid.org/0000-0002-4761-6094 12 ,
- Laura Thompson ORCID: orcid.org/0000-0001-5751-7869 13 ,
- Julie Ruiz ORCID: orcid.org/0000-0001-5672-2705 14 ,
- Fernando Oscar García ORCID: orcid.org/0000-0001-6681-0135 15 , 16 ,
- Bruce Maxwell 17 ,
- Terry Griffin ORCID: orcid.org/0000-0001-5664-484X 18 ,
- Thomas Oberthür ORCID: orcid.org/0000-0002-6050-9832 19 , 20 ,
- Christian Huyghe 21 ,
- Weifeng Zhang 22 ,
- John McNamara 23 &
- Andrew Hall ORCID: orcid.org/0000-0002-8580-6569 24
Nature Food volume 3 , pages 11–18 ( 2022 ) Cite this article
4000 Accesses
84 Citations
142 Altmetric
Metrics details
- Agriculture
- Science, technology and society
- Scientific community
- Sustainability
Restructuring farmer–researcher relationships and addressing complexity and uncertainty through joint exploration are at the heart of On-Farm Experimentation (OFE). OFE describes new approaches to agricultural research and innovation that are embedded in real-world farm management, and reflects new demands for decentralized and inclusive research that bridges sources of knowledge and fosters open innovation. Here we propose that OFE research could help to transform agriculture globally. We highlight the role of digitalization, which motivates and enables OFE by dramatically increasing scales and complexity when investigating agricultural challenges.
This is a preview of subscription content, access via your institution
Access options
Access Nature and 54 other Nature Portfolio journals
Get Nature+, our best-value online-access subscription
24,99 € / 30 days
cancel any time
Subscribe to this journal
Receive 12 digital issues and online access to articles
111,21 € per year
only 9,27 € per issue
Buy this article
- Purchase on SpringerLink
- Instant access to full article PDF
Prices may be subject to local taxes which are calculated during checkout
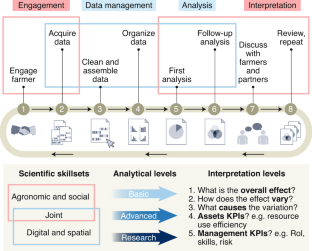
Similar content being viewed by others
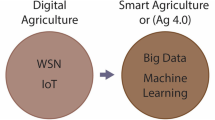
Digital Regenerative Agriculture
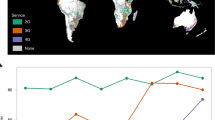
The global divide in data-driven farming
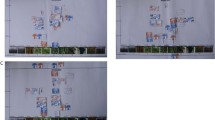
Transdisciplinary participatory-action-research from questions to actionable knowledge for sustainable viticulture development
Data availability.
The authors declare that the data supporting the findings of this study are available within the paper and its Supplementary Information (sources of Figs. 1 – 3 ).
Whitfield, S., Challinor, A. J. & Rees, R. M. Frontiers in climate smart food systems: outlining the research space. Front. Sustain. Food Syst. 2 , https://doi.org/10.3389/fsufs.2018.00002 (2018).
Scoones, I. & Thompson, J. (eds) Farmer First Revisited: Innovation for Agricultural Research and Development 1st edn (Practical Action Publishing, 2009).
Stone, G. D. Towards a general theory of agricultural knowledge production: environmental, social, and didactic learning. Cult. Agric. Food Environ. 38 , 5–17 (2016).
Hansson, S. O. Farmers’ experiments and scientific methodology. Euro. J. Phil. Sci. 9 , 32 (2019).
Maat, H. & Glover, D. in Contested Agronomy: Agricultural Research in a Changing World (eds Sumberg, J. & Thompson, J.) 131–145 (Routledge, 2012).
Šūmane, S. et al. Local and farmers’ knowledge matters! How integrating informal and formal knowledge enhances sustainable and resilient agriculture. J. Rural Stud. 59 , 232–241 (2018).
Article Google Scholar
de Janvry, A., Sadoulet, E. & Rao, M. Adjusting Extension Models to the Way Farmers Learn Policy Brief No. 159 (FERDI, 2016).
Cross, R. & Ampt, P. Exploring agroecological sustainability: unearthing innovators and documenting a community of practice in Southeast Australia. Soc. Nat. Resour. 30 , 585–600 (2016).
Rickards, L., Alexandra, J., Jolley, C., Farhey, K. & Frewer, T. Review of Agricultural Extension (ACIAR, 2019).
MacMillan, T. & Benton, T. G. Engage farmers in research. Nature 509 , 25–27 (2014).
Article ADS CAS Google Scholar
Waters-Bayer, A. et al. Exploring the impact of farmer-led research supported by civil society organisations. Agric. Food Secur. 4 , 4 (2015).
Berthet, E. T. A., Barnaud, C., Girard, N., Labatut, J. & Martin, G. How to foster agroecological innovations? A comparison of participatory design methods. J. Environ. Plan. Manage. 59 , 280–301 (2015).
Cook, S. et al. An on-farm experimental philosophy for farmer-centric digital innovation. In 14th International Conference on Precision Agriculture (ISPA, 2018).
Cook, S. E., Cock, J., Oberthür, T. & Fisher, M. On-farm experimentation. Better Crops 97 , 17–20 (2013).
Google Scholar
Richardson, M. et al. Farmer research networks in principle and practice. Int. J. Agric. Sustain. https://doi.org/10.1080/14735903.2021.1930954 (2021).
Thompson, L. J. et al. Farmers as researchers: in‐depth interviews to discern participant motivation and impact. Agron. J. 111 , 2670–2680 (2019).
Sewell, A. M. et al. Hatching new ideas about herb pastures: learning together in a community of New Zealand farmers and agricultural scientists. Agric. Syst. 125 , 63–73 (2014).
Bramley, R. G. V., Lawes, R. & Cook, S. in Precision Agriculture for Sustainability and Environmental Protection (eds Oliver, M. A., Bishop, T. F. A. & Marchant, B. M.) 205–218 (Routledge, 2013).
Marchant, B. et al. Establishing the precision and robustness of farmers’ crop experiments. Field Crops Res. 230 , 31–45 (2019).
Briggs, J. Indigenous knowledge: a false dawn for development theory and practice? Progr. Dev. Stud. 13 , 231–243 (2013).
Caron, P., Biénabe, E. & Hainzelin, E. Making transition towards ecological intensification of agriculture a reality: the gaps in and the role of scientific knowledge. Curr. Opin. Environ. Sustain. 8 , 44–52 (2014).
Kool, H., Andersson, J. A. & Giller, K. E. Reproducibility and external validity of on-farm experimental research in Africa. Exp. Agric . 56 , 587–607 (2020).
de Roo, N., Andersson, J. A. & Krupnik, T. J. On-farm trials for development impact? The organisation of research and the scaling of agricultural technologies. Exp. Agric. 55 , 163–184 (2019).
Möhring, N. et al. Pathways for advancing pesticide policies. Nat. Food 1 , 535–540 (2020).
Sylvester-Bradley, R. et al. Agronōmics: transforming crop science through digital technologies. Adv. Anim. Biosci. 8 , 728–733 (2017).
Ruiz, J., Dumont, A. & Zingraff, V. in Penser le Gouvernement des Ressources Naturelles (eds Busca, D. & Lew, N.) 293–330 (Presses de l’Université Laval, 2019).
Fabregas, R., Kremer, M. & Schilbach, F. Realizing the potential of digital development: the case of agricultural advice. Science https://doi.org/10.1126/science.aay3038 (2019).
Dowd, A.-M. et al. The role of networks in transforming Australian agriculture. Nat. Clim. Change 4 , 558–563 (2014).
Article ADS Google Scholar
Klerkx, L., van Mierlo, B. & Leeuwis, C. in Farming Systems Research into the 21st Century: The New Dynamic (eds Darnhofer, I., Gibbon, D. & Dedieu, B.) 457–483 (Springer, 2012).
Ingram, J., Gaskell, P., Mills, J. & Dwyer, J. How do we enact co-innovation with stakeholders in agricultural research projects? Managing the complex interplay between contextual and facilitation processes. J. Rural Stud. 78 , 65–77 (2020).
Jackson, L. et al. Biodiversity and agricultural sustainagility: from assessment to adaptive management. Curr. Opin. Environ. Sustain. 2 , 80–87 (2010).
Laurent, A., Kyveryga, P., Makowski, D. & Miguez, F. A framework for visualization and analysis of agronomic field trials from on‐farm research networks. Agron. J. 111 , 2712–2723 (2019).
Kyveryga, P. M. On‐farm research: experimental approaches, analytical frameworks, case studies, and impact. Agron. J. 111 , 2633–2635 (2019).
Tremblay, N. in Precision Agriculture for Sustainability (ed. Stafford, J.) 145–168 (Burleigh Dodds Science Limited, 2019); https://doi.org/10.1201/9781351114592
Bullock, D. S. et al. The data‐intensive farm management project: changing agronomic research through on‐farm precision experimentation. Agron. J. 111 , 2736–2746 (2019).
Wyatt, J., Brown, T. & Carey, S. The next chapter in design for social innovation. Stanford Soc. Innov. Rev. 19 , 40–47 (2021).
Griffin, T. W., Fitzgerald, G. J., Lowenberg‐DeBoer, J. & Barnes, E. M. Modeling local and global spatial correlation in field‐scale experiments. Agron. J . https://doi.org/10.1002/agj2.20266 (2020).
Coudel, E., Tonneau, J.-P. & Rey-Valette, H. Diverse approaches to learning in rural and development studies: review of the literature from the perspective of action learning. Knowl. Manage. Res. Pract. 9 , 120–135 (2017).
Browning, D. M. et al. Emerging technological and cultural shifts advancing drylands research and management. Front. Ecol. Environ. 13 , 52–60 (2015).
Maxwell, B. et al. Can optimization associated with on-farm experimentation using site-specific technologies improve producer management decisions? In 14th International Conference on Precision Agriculture (2018).
Kindred, D. et al. Supporting and analysing on-farm nitrogen tramline trials so farmers, industry, agronomists and scientists can learn together. In 14th International Conference on Precision Agriculture (2018).
Oberthür, T. et al. Plantation intelligence applied oil palm operations: unlocking value by analysing commercial data. Planter 93 , 339–351 (2017).
Jin, H., Shuvo Bakar, K., Henderson, B. L., Bramley, R. G. V. & Gobbett, D. L. An efficient geostatistical analysis tool for on-farm experiments targeted at localised treatment. Biosys. Eng. 205 , 121–136 (2021).
Berthet, E. T., Hickey, G. M. & Klerkx, L. Opening design and innovation processes in agriculture: insights from design and management sciences and future directions. Agric. Syst. 165 , 111–115 (2018).
Curley, M. Twelve principles for open innovation 2.0. Nature 533 , 315–316 (2016).
Ryan, S. F. et al. The role of citizen science in addressing grand challenges in food and agriculture research. Proc. Biol. Sci . 285 , 20181977 (2018).
Herrero, M. et al. Innovation can accelerate the transition towards a sustainable food system. Nat. Food 1 , 266–272 (2020).
Fielke, S. J. et al. Conceptualising the DAIS: implications of the ‘digitalisation of agricultural innovation systems’ on technology and policy at multiple levels. NJAS 90–91 , 100296 (2019).
Cook, S., Jackson, E. L., Fisher, M. J., Baker, D. & Diepeveen, D. Embedding digital agriculture into sustainable Australian food systems: pathways and pitfalls to value creation. Int. J. Agric. Sustain. https://doi.org/10.1080/14735903.2021.1937881 (2021).
van Etten, J. et al. Crop variety management for climate adaptation supported by citizen science. Proc. Natl Acad. Sci. USA 116 , 4194–4199 (2019).
Ingram, J. & Maye, D. What are the implications of digitalisation for agricultural knowledge? Front. Sustain. Food Syst. 4 , https://doi.org/10.3389/fsufs.2020.00066 (2020).
McNee, M. Government Support for Farmer-Based Research in the Falkland Islands AAC Agenda 07.11.2019, Item 10 (Agricultural Advisory Committee, Falkland Islands Government, 2019).
Zhang, W. et al. Closing yield gaps in China by empowering smallholder farmers. Nature 537 , 671–674 (2016).
Lechenet, M., Dessaint, F., Py, G., Makowski, D. & Munier-Jolain, N. Reducing pesticide use while preserving crop productivity and profitability on arable farms. Nat. Plants 3 , 17008 (2017).
García, F. et al. La Red de Nutrición de la Región Crea Sur de Santa Fe: Resultados y Conclusiones de los Primeros Diez Años 2000-2009 (AACREA, 2010).
Posner, S. M., McKenzie, E. & Ricketts, T. H. Policy impacts of ecosystem services knowledge. Proc. Natl. Acad Sci. USA 113 , 1760–1765 (2016).
Moore, M.-L., Riddell, D. & Vocisano, D. Scaling out, scaling up, scaling deep. Strategies of non-profits in advancing systemic social innovation. J. Corp. Citizenship 58 , 67–84 (2015).
Payan, J.‐C. & Pichon, L. ApeX‐Vigne, Version 2020: Une Application Mobile Gratuite pour Faciliter le Suivi de la Croissance des Vignes et Estimer la Contrainte Hydrique (Institut Français de la Vigne et du Vin and Institut Agro, Montpellier SupAgro, 2020).
Samberg, L. H. A collaboration worth its weight in grain. Nature 537 , 624–625 (2016).
Download references
Acknowledgements
This study was funded by the Premier’s Agriculture and Food Fellowship Program of Western Australia. This Fellowship is a collaboration between Curtin and Murdoch Universities and the State Government. The Fellowship is the centrepiece of the Science and Agribusiness Connect initiative, made possible by the State Government’s Royalties for Regions program. Additional support was provided by the MAK’IT-FIAS Fellowship programme (Montpellier Advanced Knowledge Institute on Transitions – French Institutes for Advanced Study) co-funded by the University of Montpellier and the European Union’s Horizon 2020 Marie Skłodowska-Curie Actions (co-fund grant agreement no. 945408), the Digital Agriculture Convergence Lab #DigitAg (grant no. ANR-16-CONV-0004) supported by ANR/PIA, and the Elizabeth Creak Charitable Trust. Contributions toward enabling workshops were made by the USDA (USDA AFRI FACT Los Angeles 2017), the International Society for Precision Agriculture (ICPA Montreal 2018 OFE-C, On-Farm Experimentation Community), the National Key Research and Development Program of China (2016YFD0201303) and ADAS (Cambridge 2018), the European Conference for Precision Agriculture (ECPA Montpellier 2019) and the OECD Co-operative Research Program for ‘Biological resource management for sustainable agricultural systems – Transformational technologies and innovation’ towards ‘#OFE2021, the first Conference on farmer-centric On-Farm Experimentation – Digital Tools for a Scalable Transformative Pathway’. L. Tresh assisted with the design and preparation of Figs. 2 and 3. Members of the #OFE2021 Working Groups also contributed their experiences and insights.
Author information
Authors and affiliations.
Centre for Digital Agriculture, Curtin University, Perth, Western Australia, Australia
Myrtille Lacoste, Simon Cook & Danielle Gale
Montpellier Advanced Knowledge Institute on Transitions (MAK’IT), University of Montpellier, Montpellier, France
Myrtille Lacoste
Centre for Digital Agriculture, Murdoch University, Perth, Western Australia, Australia
Department of Agriculture, Falkland Islands Government, Stanley, Falkland Islands
Matthew McNee
Countryside and Community Research Institute, University of Gloucestershire, Cheltenham, UK
Julie Ingram
Technologies and methods for the agricultures of tomorrow (ITAP), University of Montpellier–National Research Institute for Agriculture, Food and Environment (INRAE)–L’Institut Agro, Montpellier, France
Véronique Bellon-Maurel
Digital Agriculture Convergence Lab (#DigitAg), National Research Institute for Agriculture, Food and Environment (INRAE), Montpellier, France
Centre for Effective Innovation in Agriculture, Royal Agricultural University, Cirencester, UK
Tom MacMillan
ADAS, Cambridge, UK
Roger Sylvester-Bradley & Daniel Kindred
Commonwealth Scientific and Industrial Research Organisation (CSIRO), Adelaide, South Australia, Australia
Rob Bramley
Saint-Jean-sur-Richelieu Research and Development Centre, Agriculture and Agri-Food Canada (AAFC), St-Jean-sur-Richelieu, Quebec, Canada
Nicolas Tremblay
School of Integrative Plant Science, Cornell University, Ithaca, NY, USA
Louis Longchamps
Institute of Agriculture and Natural Resources, University of Nebraska-Lincoln, Falls City, NE, USA
Laura Thompson
Watershed and Aquatic Ecosystem Interactions Research Centre (RIVE), Université du Québec à Trois-Rivières, Trois-Rivières, Québec, Canada
Latin America Southern Cone Group, International Plant Nutrition Institute (IPNI), Buenos Aires, Argentina
Fernando Oscar García
Faculty of Agricultural Sciences, National University of Mar del Plata, Balcarce, Argentina
Montana Institute on Ecosystems, Montana State University, Bozeman, MT, USA
Bruce Maxwell
Department of Agricultural Economics, Kansas State University, Manhattan, KS, USA
Terry Griffin
Southeast Asia Group, International Plant Nutrition Institute (IPNI), Penang, Malaysia
Thomas Oberthür
Business and Partnership Development, African Plant Nutrition Institute (APNI), Benguérir, Morocco
Scientific Direction of Agriculture, National Research Institute for Agriculture, Food and Environment (INRAE), Paris, France
Christian Huyghe
College of Resources and Environmental Sciences and National Academy of Agriculture Green Development, China Agricultural University, Beijing, China
Weifeng Zhang
National Animal Nutrition Program (NANP), United States Department of Agriculture (USDA), Pullman, WA, USA
John McNamara
Commonwealth Scientific and Industrial Research Organisation (CSIRO), Canberra, Australian Capital Territory, Australia
Andrew Hall
You can also search for this author in PubMed Google Scholar
Contributions
M.L. and S.C. developed the study concept. M.M., D.G., J.I., V.B.-M., T.M., R.S.-B. and A.H. contributed additional concept development. M.L. and D.G. obtained the data and prepared the results. M.L., M.M., L.T., D.K., F.O.G., B.M., V.B.-M., J.R., C.H. and W.Z. contributed data. M.L. wrote the manuscript with input from all other authors.
Corresponding author
Correspondence to Myrtille Lacoste .
Ethics declarations
Competing interests.
The authors declare no competing interests.
Additional information
Peer review information Nature Food thanks Carol Shennan, Petro Kyveryga, Nicolas Martin and the other, anonymous, reviewer(s) for their contribution to the peer review of this work.
Publisher’s note Springer Nature remains neutral with regard to jurisdictional claims in published maps and institutional affiliations.
Supplementary information
Supplementary information.
Sources for Figs. 1–3.
Rights and permissions
Reprints and permissions
About this article
Cite this article.
Lacoste, M., Cook, S., McNee, M. et al. On-Farm Experimentation to transform global agriculture. Nat Food 3 , 11–18 (2022). https://doi.org/10.1038/s43016-021-00424-4
Download citation
Received : 13 August 2020
Accepted : 05 November 2021
Published : 23 December 2021
Issue Date : January 2022
DOI : https://doi.org/10.1038/s43016-021-00424-4
Share this article
Anyone you share the following link with will be able to read this content:
Sorry, a shareable link is not currently available for this article.
Provided by the Springer Nature SharedIt content-sharing initiative
This article is cited by
On-farm evaluation of a crop forecast-based approach for season-specific nitrogen application in winter wheat.
- Manschadi A.M.
Precision Agriculture (2024)
Adaptation rather than adoption: a case study of cropping system change in West Africa
- Anne Périnelle
- Eric Scopel
- Jean-Marc Meynard
Agronomy for Sustainable Development (2024)
Can machine learning models provide accurate fertilizer recommendations?
- Takashi S. T. Tanaka
- Gerard B. M. Heuvelink
- David S. Bullock
Digital strategies for nitrogen management in grain production systems: lessons from multi-method assessment using on-farm experimentation
- A. F. Colaço
- B. M. Whelan
- R. A. Lawes
Fully Bayesian economically optimal design for a spatially varying coefficient linear stochastic plateau model over multiple years
- Davood Poursina
- B. Wade Brorsen
Stochastic Environmental Research and Risk Assessment (2024)
Quick links
- Explore articles by subject
- Guide to authors
- Editorial policies
Sign up for the Nature Briefing: Anthropocene newsletter — what matters in anthropocene research, free to your inbox weekly.

Experimental Agriculture
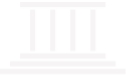
Subject Area and Category
- Agronomy and Crop Science
Cambridge University Press
Publication type
00144797, 14694441
Information
How to publish in this journal
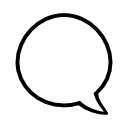
The set of journals have been ranked according to their SJR and divided into four equal groups, four quartiles. Q1 (green) comprises the quarter of the journals with the highest values, Q2 (yellow) the second highest values, Q3 (orange) the third highest values and Q4 (red) the lowest values.
Category | Year | Quartile |
---|---|---|
Agronomy and Crop Science | 1999 | Q2 |
Agronomy and Crop Science | 2000 | Q2 |
Agronomy and Crop Science | 2001 | Q2 |
Agronomy and Crop Science | 2002 | Q2 |
Agronomy and Crop Science | 2003 | Q3 |
Agronomy and Crop Science | 2004 | Q2 |
Agronomy and Crop Science | 2005 | Q3 |
Agronomy and Crop Science | 2006 | Q2 |
Agronomy and Crop Science | 2007 | Q2 |
Agronomy and Crop Science | 2008 | Q2 |
Agronomy and Crop Science | 2009 | Q3 |
Agronomy and Crop Science | 2010 | Q2 |
Agronomy and Crop Science | 2011 | Q2 |
Agronomy and Crop Science | 2012 | Q2 |
Agronomy and Crop Science | 2013 | Q2 |
Agronomy and Crop Science | 2014 | Q1 |
Agronomy and Crop Science | 2015 | Q2 |
Agronomy and Crop Science | 2016 | Q2 |
Agronomy and Crop Science | 2017 | Q2 |
Agronomy and Crop Science | 2018 | Q2 |
Agronomy and Crop Science | 2019 | Q2 |
Agronomy and Crop Science | 2020 | Q2 |
Agronomy and Crop Science | 2021 | Q2 |
Agronomy and Crop Science | 2022 | Q2 |
Agronomy and Crop Science | 2023 | Q2 |
The SJR is a size-independent prestige indicator that ranks journals by their 'average prestige per article'. It is based on the idea that 'all citations are not created equal'. SJR is a measure of scientific influence of journals that accounts for both the number of citations received by a journal and the importance or prestige of the journals where such citations come from It measures the scientific influence of the average article in a journal, it expresses how central to the global scientific discussion an average article of the journal is.
Year | SJR |
---|---|
1999 | 0.493 |
2000 | 0.485 |
2001 | 0.544 |
2002 | 0.410 |
2003 | 0.321 |
2004 | 0.422 |
2005 | 0.347 |
2006 | 0.438 |
2007 | 0.397 |
2008 | 0.544 |
2009 | 0.266 |
2010 | 0.459 |
2011 | 0.474 |
2012 | 0.505 |
2013 | 0.636 |
2014 | 0.701 |
2015 | 0.488 |
2016 | 0.411 |
2017 | 0.542 |
2018 | 0.624 |
2019 | 0.493 |
2020 | 0.585 |
2021 | 0.498 |
2022 | 0.524 |
2023 | 0.362 |
Evolution of the number of published documents. All types of documents are considered, including citable and non citable documents.
Year | Documents |
---|---|
1999 | 37 |
2000 | 38 |
2001 | 31 |
2002 | 32 |
2003 | 32 |
2004 | 33 |
2005 | 29 |
2006 | 30 |
2007 | 31 |
2008 | 37 |
2009 | 34 |
2010 | 33 |
2011 | 49 |
2012 | 37 |
2013 | 38 |
2014 | 37 |
2015 | 38 |
2016 | 39 |
2017 | 41 |
2018 | 66 |
2019 | 107 |
2020 | 43 |
2021 | 21 |
2022 | 51 |
2023 | 23 |
This indicator counts the number of citations received by documents from a journal and divides them by the total number of documents published in that journal. The chart shows the evolution of the average number of times documents published in a journal in the past two, three and four years have been cited in the current year. The two years line is equivalent to journal impact factor ™ (Thomson Reuters) metric.
Cites per document | Year | Value |
---|---|---|
Cites / Doc. (4 years) | 1999 | 0.586 |
Cites / Doc. (4 years) | 2000 | 0.514 |
Cites / Doc. (4 years) | 2001 | 0.507 |
Cites / Doc. (4 years) | 2002 | 0.698 |
Cites / Doc. (4 years) | 2003 | 0.514 |
Cites / Doc. (4 years) | 2004 | 0.534 |
Cites / Doc. (4 years) | 2005 | 0.602 |
Cites / Doc. (4 years) | 2006 | 0.770 |
Cites / Doc. (4 years) | 2007 | 1.016 |
Cites / Doc. (4 years) | 2008 | 1.089 |
Cites / Doc. (4 years) | 2009 | 0.945 |
Cites / Doc. (4 years) | 2010 | 1.008 |
Cites / Doc. (4 years) | 2011 | 1.274 |
Cites / Doc. (4 years) | 2012 | 1.405 |
Cites / Doc. (4 years) | 2013 | 1.288 |
Cites / Doc. (4 years) | 2014 | 1.567 |
Cites / Doc. (4 years) | 2015 | 1.646 |
Cites / Doc. (4 years) | 2016 | 1.233 |
Cites / Doc. (4 years) | 2017 | 1.401 |
Cites / Doc. (4 years) | 2018 | 1.542 |
Cites / Doc. (4 years) | 2019 | 1.674 |
Cites / Doc. (4 years) | 2020 | 2.008 |
Cites / Doc. (4 years) | 2021 | 2.078 |
Cites / Doc. (4 years) | 2022 | 2.228 |
Cites / Doc. (4 years) | 2023 | 2.005 |
Cites / Doc. (3 years) | 1999 | 0.586 |
Cites / Doc. (3 years) | 2000 | 0.391 |
Cites / Doc. (3 years) | 2001 | 0.472 |
Cites / Doc. (3 years) | 2002 | 0.613 |
Cites / Doc. (3 years) | 2003 | 0.465 |
Cites / Doc. (3 years) | 2004 | 0.579 |
Cites / Doc. (3 years) | 2005 | 0.474 |
Cites / Doc. (3 years) | 2006 | 0.713 |
Cites / Doc. (3 years) | 2007 | 0.913 |
Cites / Doc. (3 years) | 2008 | 0.978 |
Cites / Doc. (3 years) | 2009 | 0.837 |
Cites / Doc. (3 years) | 2010 | 1.078 |
Cites / Doc. (3 years) | 2011 | 1.212 |
Cites / Doc. (3 years) | 2012 | 1.422 |
Cites / Doc. (3 years) | 2013 | 1.345 |
Cites / Doc. (3 years) | 2014 | 1.605 |
Cites / Doc. (3 years) | 2015 | 1.348 |
Cites / Doc. (3 years) | 2016 | 1.221 |
Cites / Doc. (3 years) | 2017 | 1.404 |
Cites / Doc. (3 years) | 2018 | 1.619 |
Cites / Doc. (3 years) | 2019 | 1.568 |
Cites / Doc. (3 years) | 2020 | 1.860 |
Cites / Doc. (3 years) | 2021 | 2.023 |
Cites / Doc. (3 years) | 2022 | 2.228 |
Cites / Doc. (3 years) | 2023 | 1.461 |
Cites / Doc. (2 years) | 1999 | 0.438 |
Cites / Doc. (2 years) | 2000 | 0.400 |
Cites / Doc. (2 years) | 2001 | 0.360 |
Cites / Doc. (2 years) | 2002 | 0.478 |
Cites / Doc. (2 years) | 2003 | 0.524 |
Cites / Doc. (2 years) | 2004 | 0.453 |
Cites / Doc. (2 years) | 2005 | 0.492 |
Cites / Doc. (2 years) | 2006 | 0.694 |
Cites / Doc. (2 years) | 2007 | 0.847 |
Cites / Doc. (2 years) | 2008 | 0.869 |
Cites / Doc. (2 years) | 2009 | 0.824 |
Cites / Doc. (2 years) | 2010 | 1.070 |
Cites / Doc. (2 years) | 2011 | 1.090 |
Cites / Doc. (2 years) | 2012 | 1.451 |
Cites / Doc. (2 years) | 2013 | 1.314 |
Cites / Doc. (2 years) | 2014 | 1.213 |
Cites / Doc. (2 years) | 2015 | 1.147 |
Cites / Doc. (2 years) | 2016 | 1.133 |
Cites / Doc. (2 years) | 2017 | 1.519 |
Cites / Doc. (2 years) | 2018 | 1.488 |
Cites / Doc. (2 years) | 2019 | 1.393 |
Cites / Doc. (2 years) | 2020 | 1.832 |
Cites / Doc. (2 years) | 2021 | 2.087 |
Cites / Doc. (2 years) | 2022 | 1.578 |
Cites / Doc. (2 years) | 2023 | 1.042 |
Evolution of the total number of citations and journal's self-citations received by a journal's published documents during the three previous years. Journal Self-citation is defined as the number of citation from a journal citing article to articles published by the same journal.
Cites | Year | Value |
---|---|---|
Self Cites | 1999 | 18 |
Self Cites | 2000 | 6 |
Self Cites | 2001 | 12 |
Self Cites | 2002 | 9 |
Self Cites | 2003 | 4 |
Self Cites | 2004 | 9 |
Self Cites | 2005 | 11 |
Self Cites | 2006 | 13 |
Self Cites | 2007 | 6 |
Self Cites | 2008 | 19 |
Self Cites | 2009 | 3 |
Self Cites | 2010 | 16 |
Self Cites | 2011 | 16 |
Self Cites | 2012 | 21 |
Self Cites | 2013 | 22 |
Self Cites | 2014 | 15 |
Self Cites | 2015 | 3 |
Self Cites | 2016 | 6 |
Self Cites | 2017 | 6 |
Self Cites | 2018 | 9 |
Self Cites | 2019 | 17 |
Self Cites | 2020 | 15 |
Self Cites | 2021 | 6 |
Self Cites | 2022 | 5 |
Self Cites | 2023 | 1 |
Total Cites | 1999 | 65 |
Total Cites | 2000 | 43 |
Total Cites | 2001 | 51 |
Total Cites | 2002 | 65 |
Total Cites | 2003 | 47 |
Total Cites | 2004 | 55 |
Total Cites | 2005 | 46 |
Total Cites | 2006 | 67 |
Total Cites | 2007 | 84 |
Total Cites | 2008 | 88 |
Total Cites | 2009 | 82 |
Total Cites | 2010 | 110 |
Total Cites | 2011 | 126 |
Total Cites | 2012 | 165 |
Total Cites | 2013 | 160 |
Total Cites | 2014 | 199 |
Total Cites | 2015 | 151 |
Total Cites | 2016 | 138 |
Total Cites | 2017 | 160 |
Total Cites | 2018 | 191 |
Total Cites | 2019 | 229 |
Total Cites | 2020 | 398 |
Total Cites | 2021 | 437 |
Total Cites | 2022 | 381 |
Total Cites | 2023 | 168 |
Evolution of the number of total citation per document and external citation per document (i.e. journal self-citations removed) received by a journal's published documents during the three previous years. External citations are calculated by subtracting the number of self-citations from the total number of citations received by the journal’s documents.
Cites | Year | Value |
---|---|---|
External Cites per document | 1999 | 0.423 |
External Cites per document | 2000 | 0.336 |
External Cites per document | 2001 | 0.361 |
External Cites per document | 2002 | 0.528 |
External Cites per document | 2003 | 0.426 |
External Cites per document | 2004 | 0.484 |
External Cites per document | 2005 | 0.361 |
External Cites per document | 2006 | 0.574 |
External Cites per document | 2007 | 0.848 |
External Cites per document | 2008 | 0.767 |
External Cites per document | 2009 | 0.806 |
External Cites per document | 2010 | 0.922 |
External Cites per document | 2011 | 1.058 |
External Cites per document | 2012 | 1.241 |
External Cites per document | 2013 | 1.160 |
External Cites per document | 2014 | 1.484 |
External Cites per document | 2015 | 1.321 |
External Cites per document | 2016 | 1.168 |
External Cites per document | 2017 | 1.351 |
External Cites per document | 2018 | 1.542 |
External Cites per document | 2019 | 1.452 |
External Cites per document | 2020 | 1.790 |
External Cites per document | 2021 | 1.995 |
External Cites per document | 2022 | 2.199 |
External Cites per document | 2023 | 1.452 |
Cites per document | 1999 | 0.586 |
Cites per document | 2000 | 0.391 |
Cites per document | 2001 | 0.472 |
Cites per document | 2002 | 0.613 |
Cites per document | 2003 | 0.465 |
Cites per document | 2004 | 0.579 |
Cites per document | 2005 | 0.474 |
Cites per document | 2006 | 0.713 |
Cites per document | 2007 | 0.913 |
Cites per document | 2008 | 0.978 |
Cites per document | 2009 | 0.837 |
Cites per document | 2010 | 1.078 |
Cites per document | 2011 | 1.212 |
Cites per document | 2012 | 1.422 |
Cites per document | 2013 | 1.345 |
Cites per document | 2014 | 1.605 |
Cites per document | 2015 | 1.348 |
Cites per document | 2016 | 1.221 |
Cites per document | 2017 | 1.404 |
Cites per document | 2018 | 1.619 |
Cites per document | 2019 | 1.568 |
Cites per document | 2020 | 1.860 |
Cites per document | 2021 | 2.023 |
Cites per document | 2022 | 2.228 |
Cites per document | 2023 | 1.461 |
International Collaboration accounts for the articles that have been produced by researchers from several countries. The chart shows the ratio of a journal's documents signed by researchers from more than one country; that is including more than one country address.
Year | International Collaboration |
---|---|
1999 | 48.65 |
2000 | 42.11 |
2001 | 70.97 |
2002 | 56.25 |
2003 | 59.38 |
2004 | 51.52 |
2005 | 65.52 |
2006 | 43.33 |
2007 | 54.84 |
2008 | 59.46 |
2009 | 52.94 |
2010 | 30.30 |
2011 | 38.78 |
2012 | 56.76 |
2013 | 26.32 |
2014 | 35.14 |
2015 | 44.74 |
2016 | 38.46 |
2017 | 60.98 |
2018 | 50.00 |
2019 | 56.07 |
2020 | 62.79 |
2021 | 42.86 |
2022 | 45.10 |
2023 | 39.13 |
Not every article in a journal is considered primary research and therefore "citable", this chart shows the ratio of a journal's articles including substantial research (research articles, conference papers and reviews) in three year windows vs. those documents other than research articles, reviews and conference papers.
Documents | Year | Value |
---|---|---|
Non-citable documents | 1999 | 0 |
Non-citable documents | 2000 | 0 |
Non-citable documents | 2001 | 0 |
Non-citable documents | 2002 | 0 |
Non-citable documents | 2003 | 0 |
Non-citable documents | 2004 | 0 |
Non-citable documents | 2005 | 0 |
Non-citable documents | 2006 | 0 |
Non-citable documents | 2007 | 0 |
Non-citable documents | 2008 | 0 |
Non-citable documents | 2009 | 0 |
Non-citable documents | 2010 | 0 |
Non-citable documents | 2011 | 0 |
Non-citable documents | 2012 | 0 |
Non-citable documents | 2013 | 0 |
Non-citable documents | 2014 | 1 |
Non-citable documents | 2015 | 1 |
Non-citable documents | 2016 | 1 |
Non-citable documents | 2017 | 1 |
Non-citable documents | 2018 | 1 |
Non-citable documents | 2019 | 1 |
Non-citable documents | 2020 | 0 |
Non-citable documents | 2021 | 0 |
Non-citable documents | 2022 | 0 |
Non-citable documents | 2023 | 0 |
Citable documents | 1999 | 111 |
Citable documents | 2000 | 110 |
Citable documents | 2001 | 108 |
Citable documents | 2002 | 106 |
Citable documents | 2003 | 101 |
Citable documents | 2004 | 95 |
Citable documents | 2005 | 97 |
Citable documents | 2006 | 94 |
Citable documents | 2007 | 92 |
Citable documents | 2008 | 90 |
Citable documents | 2009 | 98 |
Citable documents | 2010 | 102 |
Citable documents | 2011 | 104 |
Citable documents | 2012 | 116 |
Citable documents | 2013 | 119 |
Citable documents | 2014 | 123 |
Citable documents | 2015 | 111 |
Citable documents | 2016 | 112 |
Citable documents | 2017 | 113 |
Citable documents | 2018 | 117 |
Citable documents | 2019 | 145 |
Citable documents | 2020 | 214 |
Citable documents | 2021 | 216 |
Citable documents | 2022 | 171 |
Citable documents | 2023 | 115 |
Ratio of a journal's items, grouped in three years windows, that have been cited at least once vs. those not cited during the following year.
Documents | Year | Value |
---|---|---|
Uncited documents | 1999 | 71 |
Uncited documents | 2000 | 78 |
Uncited documents | 2001 | 78 |
Uncited documents | 2002 | 63 |
Uncited documents | 2003 | 69 |
Uncited documents | 2004 | 56 |
Uncited documents | 2005 | 62 |
Uncited documents | 2006 | 54 |
Uncited documents | 2007 | 50 |
Uncited documents | 2008 | 41 |
Uncited documents | 2009 | 53 |
Uncited documents | 2010 | 42 |
Uncited documents | 2011 | 51 |
Uncited documents | 2012 | 48 |
Uncited documents | 2013 | 52 |
Uncited documents | 2014 | 43 |
Uncited documents | 2015 | 51 |
Uncited documents | 2016 | 47 |
Uncited documents | 2017 | 46 |
Uncited documents | 2018 | 42 |
Uncited documents | 2019 | 57 |
Uncited documents | 2020 | 74 |
Uncited documents | 2021 | 69 |
Uncited documents | 2022 | 46 |
Uncited documents | 2023 | 49 |
Cited documents | 1999 | 40 |
Cited documents | 2000 | 32 |
Cited documents | 2001 | 30 |
Cited documents | 2002 | 43 |
Cited documents | 2003 | 32 |
Cited documents | 2004 | 39 |
Cited documents | 2005 | 35 |
Cited documents | 2006 | 40 |
Cited documents | 2007 | 42 |
Cited documents | 2008 | 49 |
Cited documents | 2009 | 45 |
Cited documents | 2010 | 60 |
Cited documents | 2011 | 53 |
Cited documents | 2012 | 68 |
Cited documents | 2013 | 67 |
Cited documents | 2014 | 81 |
Cited documents | 2015 | 61 |
Cited documents | 2016 | 66 |
Cited documents | 2017 | 68 |
Cited documents | 2018 | 76 |
Cited documents | 2019 | 89 |
Cited documents | 2020 | 140 |
Cited documents | 2021 | 147 |
Cited documents | 2022 | 125 |
Cited documents | 2023 | 66 |
Evolution of the percentage of female authors.
Year | Female Percent |
---|---|
1999 | 17.39 |
2000 | 15.63 |
2001 | 16.33 |
2002 | 20.83 |
2003 | 15.52 |
2004 | 7.81 |
2005 | 8.06 |
2006 | 17.19 |
2007 | 23.17 |
2008 | 21.59 |
2009 | 17.53 |
2010 | 16.47 |
2011 | 21.09 |
2012 | 18.25 |
2013 | 23.14 |
2014 | 24.60 |
2015 | 26.14 |
2016 | 25.69 |
2017 | 22.16 |
2018 | 21.92 |
2019 | 29.59 |
2020 | 28.65 |
2021 | 27.00 |
2022 | 21.63 |
2023 | 30.93 |
Evolution of the number of documents cited by public policy documents according to Overton database.
Documents | Year | Value |
---|---|---|
Overton | 1999 | 0 |
Overton | 2000 | 0 |
Overton | 2001 | 4 |
Overton | 2002 | 0 |
Overton | 2003 | 0 |
Overton | 2004 | 0 |
Overton | 2005 | 0 |
Overton | 2006 | 0 |
Overton | 2007 | 0 |
Overton | 2008 | 0 |
Overton | 2009 | 0 |
Overton | 2010 | 0 |
Overton | 2011 | 0 |
Overton | 2012 | 0 |
Overton | 2013 | 0 |
Overton | 2014 | 0 |
Overton | 2015 | 0 |
Overton | 2016 | 0 |
Overton | 2017 | 0 |
Overton | 2018 | 0 |
Overton | 2019 | 0 |
Overton | 2020 | 0 |
Overton | 2021 | 0 |
Overton | 2022 | 0 |
Overton | 2023 | 0 |
Evoution of the number of documents related to Sustainable Development Goals defined by United Nations. Available from 2018 onwards.
Documents | Year | Value |
---|---|---|
SDG | 2018 | 37 |
SDG | 2019 | 66 |
SDG | 2020 | 33 |
SDG | 2021 | 15 |
SDG | 2022 | 28 |
SDG | 2023 | 12 |
Leave a comment
Name * Required
Email (will not be published) * Required
* Required Cancel
The users of Scimago Journal & Country Rank have the possibility to dialogue through comments linked to a specific journal. The purpose is to have a forum in which general doubts about the processes of publication in the journal, experiences and other issues derived from the publication of papers are resolved. For topics on particular articles, maintain the dialogue through the usual channels with your editor.
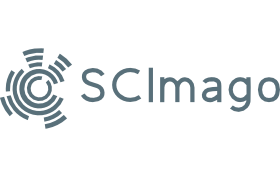
Follow us on @ScimagoJR Scimago Lab , Copyright 2007-2024. Data Source: Scopus®

Cookie settings
Cookie Policy
Legal Notice
Privacy Policy
Experimental methods in agriculture
Andrea Onofri and Dario Sacco
Update: v. 1.1 (2023-12-06), compil. 2023-12-13
Introduction
This is the website for the book “Experimental methods in agriculture”, where we deal with the organisation of experiments and data analyses in agriculture and, more generally, in biology. Experiments are the key element to scientific progress and they need to be designed in a way that reliable data is produced. Once this fundamental requirement has been fulfilled, statistics can be used to summarise and explore the results, making a clear distinction between ‘signal’ and ‘noise’ and, hence, reaching appropriate conclusions.
In this book, we will try to give some essential information to support the adoption of good research practices, with particular reference to field experiments, which are used to compare, e.g., innovative genotypes, agronomic practices, herbicides and other weed control methods. We firmly believe that the advancement of cropping techniques should always be based on the evidence provided by scientifically sound experiments.
We will follow a ‘learn-by-doing’ approach, making use of several examples and case studies, while keeping theory and maths at a minimum level; indeed, we are talking to agronomists and biologists and not to statisticians! However, we will not totally remove theory: we think that being able to do some simple hand-calculations is the best way to master the process of data-analysis.
This website is (and will always be) free to use, and is licensed under the Creative Commons Attribution-NonCommercial-NoDerivs 3.0 License. It is written in RMarkdown with the ‘bookdown’ package and it is rebuilt every now and then, to incorporate corrections and updates. This is necessary, as R is a rapidly evolving language.
This book is not written aiming at completeness, but it is finely tuned for a 6 ECTS introductory course in biometry, for master or PhD students. It is mainly aimed at building solid foundations for starting a job in the research field and, eventually, to be able to tackle more advanced statistical material.
How this book is organised
In the first two Chapters we will deal with the experimental design: we need to be able to distinguish good from bad experiments. One key aspect is that our experimental results are only a sample from a universe of possible results and we can never be totally sure that such a sample fully reflects the characteristics of the whole universe. Hence, uncertainty is an unavoidable component of science, which we need to tackle by ensuring that the experimental methods are as reliable as possible.
In Chapter 3 we will show how we can describe the experimental results, based on some simple stats, such as the mean, median, chi square value and Pearson correlation coefficient. In chapter 4 we will introduce some simple models, which we can use to describe the results of our experiments. Of course, the observed data come as the result of deterministic and stochastic processes and, therefore, we will also describe some stochastic models, with particular reference to the Gaussian Density function.
In Chapters 5 and 6 we will talk about statistical inference and Formal Hypothesis Testing. We will describe the basic concepts of confidence intervals, P-levels and error types and we will introduce t-tests and chi-square tests.
From Chapter 7 to Chapter 12 we will talk about the ANOVA, that is one of the most widely used techniques of data analysis. We will show one-way and two-ways ANOVA models and we will also introduce more complex designs, such as the split-plot and strip-plot. Chapter 13 and 14 will be devoted to describe, respectively, linear and nonlinear regression models. In the Chapters from 7 to 14, we will always start from a motivating example, so that the readers can have an idea of the experimental situation, before diving into the details. In the final chapter 15, we will provide exercises for all book chapters, which should help the readers to practice with what they have learned, while reading the book.
Statistical software
In this book, we will work through all the examples by using the R statistical software, together with the RStudio environment. We selected such software for a number of reasons: first of all we like it very much and we think that it is a pleasure to use it, once the initial difficulties have been overcame! Second, it is freeware, which is fundamental for the students. Third, in recent years the software skills of students in master degree or PhD programmes have notably increased and writing small chunks of code is no longer a problem for most of them. Last, but not least, we have seen that some experience with R is a very often required skill when applying for a job. We should acknowledge that R and RStudio are two wonderful pieces of software and we are very much indebted to the whole community who is working to ensure their wide availability and freeware nature.
R is characterised by a modular structure and its basic functionalities can be widely extended by a set of add-in packages. As this is mainly an introductory course, we decided to stick to the main packages, which come with the basic R installation. However, we could not avoid the use of a few very important packages, which we will indicate later on. Of course, it is necessary to state that many of the tasks we perform in this book could be as well (or even better) performed by using additional packages, such as those included in the relatively new ‘tidyverse’ package. We should also mention that this book was built by using the ‘bookdown’ package and it is hosted on the blog ‘www.statforbiology.com’, which is built by using the ‘blogdown’ package. We will not use these two packages during the course, but we should mention that they are really useful.
We will not assume any prior knowledge, and we will start from the very beginning. In order to help the readers, we also provide a very gentle introduction to R as an appendix.
The authors
Andrea is Associate Professor at the Department of Agricultural, Food and Environmental Science, University of Perugia and he has taught ‘Experimental methods in Agriculture’ since 2000. Dario was Associate Professors at the Department of Agricultural, Forest and Food Sciences, University of Torino; he used to teach ‘Experimental Methods in Agriculture’ until 2020, when he suddenly died, far too early. Unfortunately, he could not see this book completed.
Cornell AES is an indispensable part of the College of Agriculture & Life Sciences , and also serves the Colleges of Human Ecology & Veterinary Medicine .

- Cornell University Agricultural Experiment Station

Cornell AES
Bringing research to life
Improving the health and welfare of people and our planet
Cornell AES is vital to New York state agriculture and food security. We contribute to healthy and resilient communities, and improve the lives and well-being of people by advancing research on agriculture and food systems, the environment, applied economics, and community and individual development.
Cornell AES by the numbers
Researchers supported by Cornell AES funding annually
Research projects on farms and in greenhouses
Research farms across New York state, and 127K square feet of greenhouse space
Working for a sustainable future
The challenges facing our planet and people are daunting. At Cornell AES we are passionate about creating a more resilient future. To us, sustainability is not just a concept, but an intricate part of everything we do.

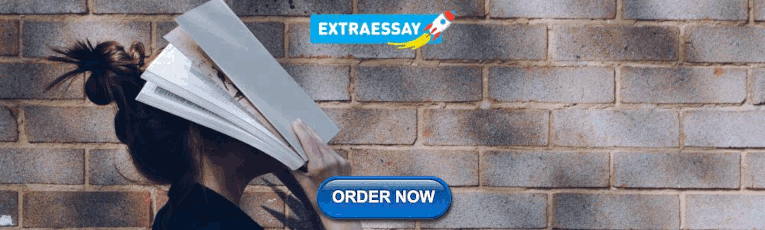
Research farms and facilities
Cornell’s world-class research farms and plant growth facilities that are managed by Cornell AES, provide the crucial support and environments needed to move critical research from concept to commercial application.
Funding research
Cornell AES-managed Federal Capacity Funds provide vital support for important research projects in three Cornell colleges. Learn about the grant programs and the application process.
Research impacts
Find out how research supported by Cornell AES addresses a broad array of real-world problems. Read about innovative science-based solutions and promising research projects important to people, the economy, the environment and our future.
News & updates

- Biological Field Station
- Musgrave Research Farm

- Soil and Crop Sciences Section
- Agriculture

- Food Science
- Synthetic Biology
- Cornell AES events
- More Cornell AES news

- Paper Archives
- Journal Indexing
- Research Conference
- Add Journal
Searching By
- Search More ...

American Journal of Experimental Agriculture (ISSN: 2231-0606)
Publisher SCIENCEDOMAIN international
ISSN-L 2231-0606
ISSN 2231-0606
IF(Impact Factor) 2024 Evaluation Pending
Website http://www.sciencedomain.org
Description
Last modified: 2011-08-16 09:51:52
- No Archives
Advertisement
How to Conduct Research on Your Farm or Ranch
Basics of experimental design.
or call (301) 779-1007 to order.
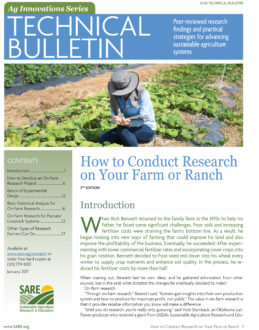
The previous section summarized the 10 steps for developing and implementing an on-farm research project. In steps 1 through 3, you wrote out your research question and objective, developed a hypothesis, and figured out what you will observe and measure in the field. Now you are ready to actually design the experiment. This section provides more detail on step 4 in the process.
Recall from the introduction that on-farm research provides a way of dealing with the problem of field and environmental variability. In comparing the effects of different practices (treatments), you need to know if the effects that you observe in the crop or in the field are simply a product of the natural variation that occurs in every ecological system, or whether those changes are truly a result of the new practices that you have implemented.
Take the simple example of comparing two varieties of tomatoes: a standard variety and a new one that you have just heard about. You could plant half of a field in the standard variety and the other half of the field in the new variety. You plant the tomatoes on exactly the same day, and you manage both halves of the field exactly the same throughout the growing season. Throughout the harvest period, you keep separate records of the yield from each half of the field so that at the end of the season you have the total yield for each variety. Suppose that under this scenario, the new variety had a 15 percent higher yield than your standard variety. Can you say for sure that the new variety outperforms your standard variety? The answer is no, because there may be other factors that led to the difference in yield, including:
- The new variety was planted in a part of the field that had better soil.
- One end of the field was wetter than the other and some of the tomatoes were infected with powdery mildew.
- Soil texture differences resulted in increased soil moisture from one end of the field to the other.
- Part of the field with the standard variety receives afternoon shade from an adjacent line of trees.
- Weed pressure is greater in one part of the field with the standard variety.
- Adjacent forest or wildlands are a source of pests that affect one end of the field more than the other.
With the right experimental design and statistical analysis, you can identify and isolate the effects of natural variation and determine whether the differences between treatments are “real,” within certain levels of probability. This section looks at three basic experimental design methods: the paired comparison, the randomized complete block and the split-plot design. Which one you choose depends largely on the research question that you are asking and the number of treatments in your experiment (Table 2).
The number of treatments in your experiment should be apparent from your research question and hypothesis. If that is not the case, then you will need to go back and refine your research question so that you have more clarity as to what you are testing. As previously noted, when identifying your research question (step 1), remember to keep things simple. Avoid over-complicating your experiment by trying to do too much at once. And, keep in mind that although the randomized complete block and split-plot designs provide more information than the paired comparison, they also require a larger field area, more management and more sophisticated statistics to analyze the data. Table 2 also lists the type of statistical analysis associated with each experimental design method. These statistical techniques are covered in the next section, Basic Statistical Analysis for On-Farm Research . First is a review of some basic experimental design terminology.
TABLE 2: Three Experimental Design Methods
DESIGN METHOD | WHEN TO USE | STATISTICAL ANALYSIS |
Paired comparison | To compare two treatments | t-test |
Randomized complete block | To compare three or more treatments | Analysis of variance (ANOVA) |
Split-plot | To see how different treatments interact | Analysis of variance (ANOVA) |
Treatments: A treatment is the production practice that you are evaluating. Examples of treatments include choice of variety, different fertilizer rates, different fertilizer timing, choice of cover crops, different cover crop management strategies, timing of planting, type of tillage, different pest control methods or different irrigation strategies. For animal operations, treatments might be different feed rations, type of bedding, pasture versus confinement, grazing period, nutritional supplements, or disease/parasite controls. The choices are limitless given the complexity of farming. On-farm research usually compares just two or three practices. In most cases, one of the treatments is the standard practice, or what you usually do, and is known as the “control.”
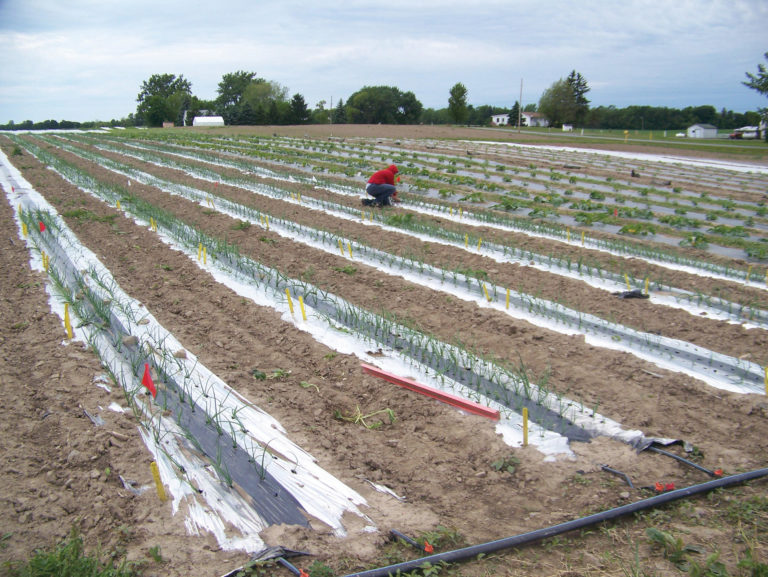
Small-scale intensive onion production on plastic in Interlaken, NY. Cornell extension vegetable specialist Christine Hoepting found growers could improve yields and reduce bacteria incidence by using alternatives to black plastic mulch, and by increasing planting density. Courtesy Cornell University Cooperative Extension
Variable: In statistics, a variable is any property or characteristic that can be manipulated, measured or counted. In on-farm research, the independent variable is the different treatments (practices) you are applying, and the dependent variable is the effect or outcome you are measuring. What you measure in your particular experiment depends on what treatments you apply. Examples include crop yield, weed density, milk production or animal weight gain.
Plot: Plots are the basic units of a field research project—the specific-sized areas in which each treatment is applied. Replication: Replication means repeating individual treatment plots within the field research area. If you set up an experiment comparing two treatments, instead of setting out just one plot of Treatment A and one plot of Treatment B, you repeat the plots within the field multiple times. Replications reduce experimental error and increase the power of the statistics used to analyze data.
Block: It is usually not possible to find a perfectly uniform field in which to conduct the experiment, and some sources of variation simply cannot be controlled (e.g., slope or soil texture gradients). In order to address the problem of field variability, divide your field of interest into sections that have common slope and soil characteristics. Within each section—typically known as blocks—field conditions should be as uniform as possible. Taken together, however, all of your blocks should encompass the variability that exists across the research area. After delineating the areas for your blocks, make sure you include each treatment inside each block; that way, your blocks can serve as replications. In most on-farm research studies, four to six blocks are sufficient to provide a good level of confidence in the results. Figure 2 provides examples of how to use blocking to address field variability due to slope or soil type.
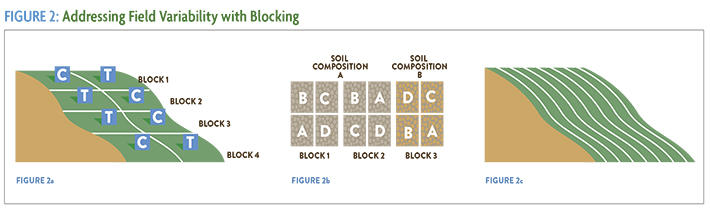
Agricultural research should usually be blocked because of field variability. If your field has a known gradient, such as a fertility or moisture gradient, it is best to place blocks to that conditions are as uniform as possible within each block. Figure 2a: On a slope, for example, each whole block should occupy about the same elevation. Treatments are randomized and run across the slope within each block. Figure 2b: Place whole blocks within different soil types. Figure 2c: If blocks cannot be used to account for variability, then each treatment should run across the whole gradient, as in all the way down the slope or all the way across the field. This arrangement can also be used for a completely randomized design (see Figure 3).
Randomization: In addition to replication, randomization is also important for addressing the problem of field variability, reducing experimental error and determining the true effect of the treatments you are comparing. Replications should be arranged randomly within the field. Or in the case of a blocked experimental design, treatment plots must be arranged randomly within each block. If you have three treatments, for example, you cannot place those treatments in the same left-to right sequence within each block. They must be arranged in a random order. This can be done using the flip of a coin, drawing numbers from a hat or using a random number generator for each block.
American Journal of Experimental Agriculture Latest Publications
Total documents, published by sciencedomain international.
- Latest Documents
- Most Cited Documents
- Contributed Authors
- Related Sources
- Related Keywords
Estimation of the Degree of Diversity for Some Iraqi Wheat Varieties through ISSR, SRAP and RAPD Markers
Assessment of genetic diversity in pepper (capsicum sp.) landraces from ghana using agro-morphological characters, evaluation of ugandan cowpea germplasm for yield and resistance to scab disease, an evaluation of profit margin of sawyers of wood products in the forestry zone surrounding kumba (cameroon), response and economic indices of broilers on low crude protein diets fortified with lysine, inclusion of monosex tilapia (oreochromis niloticus) with freshwater prawn (macrobrachium rosenbergii) in polyculture systems in the ponds of coastal region: impact of stocking density of tilapia on production and profitability, yield and yield attributes of tomato as influenced by organic fertilizer, effects of missing elements (nps) on chemical properties of soil and nutrient uptake of rice var. brri dhan29, impact of cropping ratios of maize (zea mays)-egusi melon (colocynthis citrullus) and npk fertilizer on soil productivity rating of an ultisol of edo state in nigeria, green manure source affects growth and vegetative yield of fluted pumpkin, export citation format, share document.
Journal of Experimental Biology and Agricultural Sciences
About the Journal
Journal of Experimental Biology and Agricultural Sciences (JEBAS) is an online platform for the advancement and rapid dissemination of scientific knowledge generated by highly motivated researchers in the field of agricultural and biological sciences. The journal also welcomes articles of inter-disciplinary nature work. JEBAS publishes high-quality original research and critical up-to-date review articles covering all the aspects of biological, agricultural, veterinary and animal sciences. Journal also published theme-based special issues. The main criteria for acceptance of the articles are novelty, clarity, and significance as relevant to a better understanding of the agricultural and biological sciences. Every year, it publishes six issues in February, April, June, August, October, and December.
Announcements
Naas score 2024.
NAAS Score 2024 - 5.62 [Details]
New Revamped Website of JEBAS
Current issue.
- Vol. 12 No. 3 (2024)
REVIEW ARTICLES
Unleashing the future: exploring the transformative prospects of artificial intelligence in veterinary science, biosynthesis of secondary metabolites in aromatic and medicinal plants in response to abiotic stresses: a review, research articles, plant growth promotion activities of bacillus spp. isolated from jakrem hot water spring of meghalaya, north east india, role of probiotic microorganisms in the brain plasticity development, hematite nanoparticle mediated enhancement of chlorella minutissima lipid productivity for sustainable biodiesel production, isolation and characterization of polygalacturonase producing thermophilic aspergillus niger isolated from decayed tomato fruits, germination of senegalia mellifera seeds in response to presowing treatments, effect of preparation and drying techniques on the physicochemical, functional and nutritional properties of products from beetroot (beta vulgaris l.) varieties, clonal propagated 'ek pothi lehsun' as a potential antifungal agent against candida sp., principal component analysis of morpho-floral traits in oryza sativa × oryza longistaminata advanced backcross lines of rice, exploring intra-allelic and inter-allelic gene interactions influencing seed yield and its components in inter-varietal crosses of mungbean (vigna radiata (l.) wilczek), inheritance pattern of qualitative traits, genetic analysis and association of yield attributes in f2 populations of rice (oryza sativa), proceeding of bionext-2023_review articles, crispr driven cyanobacterial metabolic engineering and its role in metabolite production, the underlying factors of occurrence of mucormycosis in post-covid-19 patients – a meta-analysis of case histories, effect of stress during exam time on immunity - a survey based study, proceeding of bionext-2023_research articles, investigating the antimicrobial activity of neem and clove extract on biofilm-producing oral microflora, online issn, editorial board.
Editor-in-Chief Prof Y. Norma-Rashid (University of Malaya, Kuala Lumpur) [email protected]
Co-Editor in Chief Dr. Kuldeep Dhama, M.V.Sc., Ph.D. NAAS Associate, Principal Scientist, IVRI, Izatnagar India - 243 122 [email protected]
Managing - Editor Kamal K Chaudhary (India) [email protected]
- Scopus [Details]
- Index Copernicus
- UGC-CARE Indexed Journal list Group II Journal SCOPUS [Details]
- NAAS Score 2024 - 5.62 [ Details ]
- AGRICOLA DATABASE (NAL-USDA, United Stats)
- CABI – (UK)
Scopus Cite Score
Scimago journal rank.
HORIZON PUBLISHER INDIA [HPI]
Memberships and Affiliations

Information
- For Readers
- For Authors
- For Librarians
Page Visitors
Make a Submission
©Journal of Experimental Biology and Agricultural Sciences
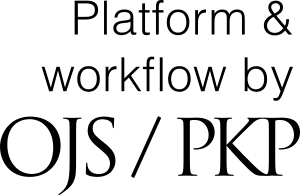
Information
- Author Services
Initiatives
You are accessing a machine-readable page. In order to be human-readable, please install an RSS reader.
All articles published by MDPI are made immediately available worldwide under an open access license. No special permission is required to reuse all or part of the article published by MDPI, including figures and tables. For articles published under an open access Creative Common CC BY license, any part of the article may be reused without permission provided that the original article is clearly cited. For more information, please refer to https://www.mdpi.com/openaccess .
Feature papers represent the most advanced research with significant potential for high impact in the field. A Feature Paper should be a substantial original Article that involves several techniques or approaches, provides an outlook for future research directions and describes possible research applications.
Feature papers are submitted upon individual invitation or recommendation by the scientific editors and must receive positive feedback from the reviewers.
Editor’s Choice articles are based on recommendations by the scientific editors of MDPI journals from around the world. Editors select a small number of articles recently published in the journal that they believe will be particularly interesting to readers, or important in the respective research area. The aim is to provide a snapshot of some of the most exciting work published in the various research areas of the journal.
Original Submission Date Received: .
- Active Journals
- Find a Journal
- Proceedings Series
- For Authors
- For Reviewers
- For Editors
- For Librarians
- For Publishers
- For Societies
- For Conference Organizers
- Open Access Policy
- Institutional Open Access Program
- Special Issues Guidelines
- Editorial Process
- Research and Publication Ethics
- Article Processing Charges
- Testimonials
- Preprints.org
- SciProfiles
- Encyclopedia
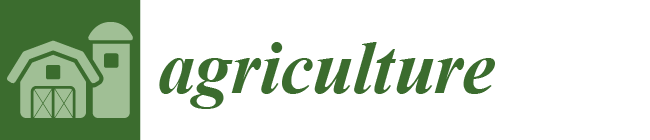
Article Menu
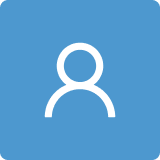
- Subscribe SciFeed
- Recommended Articles
- Google Scholar
- on Google Scholar
- Table of Contents
Find support for a specific problem in the support section of our website.
Please let us know what you think of our products and services.
Visit our dedicated information section to learn more about MDPI.
JSmol Viewer
Experimental research on breakage characteristics of feed pellets under different loading methods.
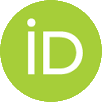
1. Introduction
2. materials and methods, 2.1. samples and preparation, 2.2. experimental equipment, 2.3. experimental methods, 2.3.1. repeated compression, 2.3.2. repeated impacts, 2.4. evaluation of breakage characteristics, 2.4.1. size distribution function, 2.4.2. pulverization rate, 2.4.3. mass-specific energy, 2.4.4. fitting model, 3. results and discussion, 3.1. breakage behaviors of feed pellets, 3.2. particle size distribution, 3.3. energy and pulverization rate, 3.3.1. the influence of loading cycles, 3.3.2. relationship between energy and pulverization rate, 4. conclusions, supplementary materials, author contributions, institutional review board statement, data availability statement, conflicts of interest.
- Halstensen, M.; Ihunegbo, F.N.; Ratnayake, C.; Sveinsvold, K. Online acoustic chemometric monitoring of fish feed pellet velocity in a pneumatic conveying system. Powder Technol. 2014 , 263 , 104–111. [ Google Scholar ] [ CrossRef ]
- Thomas, M.; van der Poel, A.F.B. Physical quality of pelleted animal feed 1. Criteria for pellet quality. Anim. Feed Sci. Technol. 1996 , 61 , 89–112. [ Google Scholar ] [ CrossRef ]
- Chang, C.M.; Fang, W.; Jao, R.C.; Shyu, C.Z.; Liao, I.C. Development of an intelligent feeding controller for indoor intensive culturing of eel. Aquac. Eng. 2005 , 32 , 343–353. [ Google Scholar ] [ CrossRef ]
- Vukmirovic, D.; Colovic, R.; Rakita, S.; Brlek, T.; Duragic, O.; Sola-Oriol, D. Importance of feed structure (particle size) and feed form (mash vs. pellets) in pig nutrition—A review. Anim. Feed Sci. Technol. 2017 , 233 , 133–144. [ Google Scholar ] [ CrossRef ]
- Ardiaca Garcia, M.; Montesinos Barcelo, A.; Bonvehi Nadeu, C.; Jekl, V. Respiratory Diseases in Guinea Pigs, Chinchillas and Degus. Vet. Clin. N. Am. Exot. Anim. Pract. 2021 , 24 , 419–457. [ Google Scholar ] [ CrossRef ]
- Briggs, J.L.; Maier, D.E.; Watkins, B.A.; Behnke, K.C. Effect of ingredients and processing parameters on pellet quality. Poult. Sci. 1999 , 78 , 1464–1471. [ Google Scholar ] [ CrossRef ]
- Amerah, A.M.; Ravindran, V.; Lentle, R.G.; Thomas, D.G. Feed particle size: Implications on the digestion and performance of poultry. Worlds Poult. Sci. J. 2007 , 63 , 439–455. [ Google Scholar ] [ CrossRef ]
- Kaliyan, N.; Morey, R.V. Factors affecting strength and durability of densified biomass products. Biomass Bioenergy 2009 , 33 , 337–359. [ Google Scholar ] [ CrossRef ]
- Aarseth, K.A.; Prestlokken, E. Mechanical properties of feed pellets: Weibull analysis. Biosyst. Eng. 2003 , 84 , 349–361. [ Google Scholar ] [ CrossRef ]
- Aarseth, K.A. Attrition of feed pellets during pneumatic conveying: The influence of velocity and bend radius. Biosyst. Eng. 2004 , 89 , 197–213. [ Google Scholar ] [ CrossRef ]
- Aarseth, K.A.; Perez, V.; Boe, J.K.; Jeksrud, W.K. Reliable pneumatic conveying of fish feed. Aquac. Eng. 2006 , 35 , 14–25. [ Google Scholar ] [ CrossRef ]
- Aas, T.S.; Oehme, M.; Sorensen, M.; He, G.; Lygren, I.; Asgard, T. Analysis of pellet degradation of extruded high energy fish feeds with different physical qualities in a pneumatic feeding system. Aquac. Eng. 2011 , 44 , 25–34. [ Google Scholar ] [ CrossRef ]
- Kong, X.; Liu, J.; Yang, T.; Su, Y.; Geng, J.; Niu, Z. Numerical simulation of feed pellet breakage in pneumatic conveying. Biosyst. Eng. 2022 , 218 , 31–42. [ Google Scholar ] [ CrossRef ]
- Kong, X.; Cao, Q.; Zhao, Z.; Niu, Z.; Liu, J. Breakage probability of feed pellet under repeated compression and impacts. Granul. Matter 2023 , 25 , 47. [ Google Scholar ] [ CrossRef ]
- Haubjerg, A.F.; Veje, C.T.; Jorgensen, B.N.; Simonsen, B.; Lovgreen, S. Structural properties and mechanical durability of extruded fish feed. J. Food Process Eng. 2015 , 38 , 621–631. [ Google Scholar ] [ CrossRef ]
- Haubjerg, A.F.; Veje, C.T. An experimental approach towards increasing mechanical durability of extruded fish feed in the drying process. Dry. Technol. 2019 , 37 , 1418–1426. [ Google Scholar ] [ CrossRef ]
- Wolska, J.; Holst, O.; Adlercreutz, P.; Jonkers, J. Standardized method for the Holmen pellet tester (NHP100) as a tool for evaluating the physical quality of fish feed. Aquac. Res. 2016 , 47 , 1790–1796. [ Google Scholar ] [ CrossRef ]
- Han, Y.; Zhao, D.; Chu, Y.; Zhen, J.; Li, G.; Zhao, H.; Jia, F. Breakage behaviour of single rice particles under compression and impact. Adv. Powder Technol. 2021 , 32 , 4635–4650. [ Google Scholar ] [ CrossRef ]
- Shen, S.; Han, Y.; Hao, X.; Chen, P.; Li, A.; Wang, Y.; Zhang, J.; Feng, W.; Fei, J.; Jia, F. Analysis of the breakage characteristics of rice particle beds under confined compression tests. Powder Technol. 2023 , 418 , 118319. [ Google Scholar ] [ CrossRef ]
- Antonyuk, S.; Tomas, E.; Heinrich, S.; Morl, L. Breakage behaviour of spherical granulates by compression. Chem. Eng. Sci. 2005 , 60 , 4031–4044. [ Google Scholar ] [ CrossRef ]
- Lin, S.S.; Chung, Y.C.; Lin, C.K.; Chen, Y.C. DEM simulation and experimental validation for mechanical response of ellipsoidal particles under confined compression. Adv. Powder Technol. 2018 , 29 , 1292–1305. [ Google Scholar ] [ CrossRef ]
- Orefice, L.; Khinast, J.G. Deformable and breakable DEM particle clusters for modelling compression of plastic and brittle porous materials—Model and structure properties. Powder Technol. 2020 , 368 , 90–104. [ Google Scholar ] [ CrossRef ]
- Saiedirad, M.H.; Tabatabaeefar, A.; Borghei, A.; Mirsalehi, M.; Badii, F.; Varnamkhasti, M.G. Effects of moisture content, seed size, loading rate and seed orientation on force and energy required for fracturing cumin seed ( Cuminum cyminum Linn.) under quasi-static loading. J. Food Eng. 2008 , 86 , 565–572. [ Google Scholar ] [ CrossRef ]
- Baslar, M.; Kalkan, F.; Kara, M.; Ertugay, M.F. Correlation between the protein content and mechanical properties of wheat. Turk. J. Agric. For. 2012 , 36 , 601–607. [ Google Scholar ]
- Ramaj, I.; Romuli, S.; Schock, S.; Mueller, J. Discrete element modelling of bulk behaviour of wheat ( Triticum aestivum L.) cv. ‘Pionier’ during compressive loading. Biosyst. Eng. 2024 , 242 , 123–139. [ Google Scholar ]
- Guerra, M.V.; Harshe, Y.M.; Fries, L.; Rothberg, S.; Palzer, S.; Heinrich, S. Influence of particle size distribution on espresso extraction via packed bed compression. J. Food Eng. 2023 , 340 , 111301. [ Google Scholar ] [ CrossRef ]
- Liu, X.; Shi, Z.; Zhang, Y.; Li, H.; Pei, H.; Yang, H. Characteristics of Damage to Brown Rice Kernels under Single and Continuous Mechanical Compression Conditions. Foods 2024 , 13 , 1069. [ Google Scholar ] [ CrossRef ]
- Chen, Z.P.; Wassgren, C.; Ambrose, R.P.K. Development and validation of a DEM model for predicting impact damage of maize kernels. Biosyst. Eng. 2022 , 224 , 16–33. [ Google Scholar ] [ CrossRef ]
- Chen, Z.P.; Wassgren, C.; Ambrose, R.P.K. Development and validation of a DEM model for predicting compression damage of maize kernels. Biosyst. Eng. 2023 , 230 , 480–496. [ Google Scholar ] [ CrossRef ]
- Mandelbrot, B.B.; Wheeler, J.A. The fractal geometry of nature. Am. J. Phys. 1984 , 51 , 286–287. [ Google Scholar ] [ CrossRef ]
- Xu, Y. The fractal evolution of particle fragmentation under different fracture energy. Powder Technol. 2018 , 323 , 337–345. [ Google Scholar ] [ CrossRef ]
- Weibull, W. A statistical theory of the strength of materials. J. Appl. Mech. 1939 , 18 , 293–297. [ Google Scholar ] [ CrossRef ]
- Yu, M.; Wu, M.; Yang, X.; Lou, R.; Wang, F.; Li, H.; Wang, L. Effect of temperature on the evolution and distribution for particle size of loose broken coal during the uniaxial confined compression process. Fuel 2022 , 318. [ Google Scholar ] [ CrossRef ]
- Kruszelnicka, W.; Leda, P.; Tomporowski, A.; Ambrose, K. Breakage behavior of corn kernels subjected to repeated loadings. Powder Technol. 2024 , 435 , 119372. [ Google Scholar ] [ CrossRef ]
- Abouzeid, A.Z.M.A.; Seifelnassr, A.A.S.; Zain, G.; Mustafa, Y.S. Breakage Behavior of Quartz Under Compression in a Piston Die. Min. Metall. Explor. 2019 , 36 , 173–180. [ Google Scholar ] [ CrossRef ]
- Rozenblat, Y.; Grant, E.; Levy, A.; Kalman, H.; Tomas, J. Selection and breakage functions of particles under impact loads. Chem. Eng. Sci. 2012 , 71 , 56–66. [ Google Scholar ] [ CrossRef ]
- ANSI/ASAE S319.4 ; Method of Determining and Expressing Fineness of Feed Materials by Sieving. American Society of Agricultural and Biological Engineers: St Joseph, MI, USA, 2008.
- ASAE S269.5 ; Densified Products for Bulk Handling—Definitions and Method. American Society of Agricultural and Biological Engineers: St Joseph, MI, USA, 2021.
- Vogel, L.; Peukert, W. Breakage behaviour of different materials—Construction of a mastercurve for the breakage probability. Powder Technol. 2003 , 129 , 101–110. [ Google Scholar ] [ CrossRef ]
- Chen, Z.; Wassgren, C.; Ambrose, R.P.K. Measured damage resistance of corn and wheat kernels to compression, friction, and repeated impacts. Powder Technol. 2021 , 380 , 638–648. [ Google Scholar ] [ CrossRef ]
- Zeng, Y.; Mao, B.; Jia, F.; Han, Y.; Li, G. Modelling of grain breakage of in a vertical rice mill based on DEM simulation combining particle replacement model. Biosyst. Eng. 2022 , 215 , 32–48. [ Google Scholar ] [ CrossRef ]
- Djordjevic, N.; Morrison, R. Exploratory modelling of grinding pressure within a compressed particle bed. Miner. Eng. 2006 , 19 , 995–1004. [ Google Scholar ] [ CrossRef ]
- Zhou, Q.; Guo, Q.; Pan, Y.; Zhu, C.; Wei, Y. Experimental research on energy-size distribution model of coal particle bed comminution. Physicochem. Probl. Miner. Process. 2020 , 56 , 772–783. [ Google Scholar ] [ CrossRef ]
- Zhang, Z.T.; Gao, W.H.; Zhang, Z.M.; Tang, X.Y.; Wu, J. Evolution of particle disintegration of red sandstone using Weibull distribution. Rock Soil Mech. 2020 , 41 , 877–885. [ Google Scholar ]
- Kang, G.; Ning, Y.J.; Liu, R.; Chen, P.W.; Pang, S.P. Simulation of force chains and particle breakage of granular material by numerical manifold method. Powder Technol. 2021 , 390 , 464–472. [ Google Scholar ] [ CrossRef ]
- Salman; Russell, A.; Aman, S.; Tomas, J. Breakage probability of granules during repeated loading. Powder Technol. 2015 , 269 , 541–547. [ Google Scholar ] [ CrossRef ]
Click here to enlarge figure
Chemical Compositions | Content (%) |
---|---|
Crude protein | 15.00 |
Water content | 11.72 |
Crude ash | 8.00 |
Crude fibre | 7.00 |
Calcium | 0.90 |
Phosphorus | 0.50 |
Parameter | f (kg J ) | E , (J/kg) | α | R |
---|---|---|---|---|
Repeated compression | 9.7449 × 10 | 19.1904 | 0.8833 | 0.9485 |
Repeated impacts | 0.0020 | 5.0086 | 1.7334 | 0.9733 |
The statements, opinions and data contained in all publications are solely those of the individual author(s) and contributor(s) and not of MDPI and/or the editor(s). MDPI and/or the editor(s) disclaim responsibility for any injury to people or property resulting from any ideas, methods, instructions or products referred to in the content. |
Share and Cite
Kong, X.; Cao, Q.; Niu, Z. Experimental Research on Breakage Characteristics of Feed Pellets under Different Loading Methods. Agriculture 2024 , 14 , 1401. https://doi.org/10.3390/agriculture14081401
Kong X, Cao Q, Niu Z. Experimental Research on Breakage Characteristics of Feed Pellets under Different Loading Methods. Agriculture . 2024; 14(8):1401. https://doi.org/10.3390/agriculture14081401
Kong, Xianrui, Qing Cao, and Zhiyou Niu. 2024. "Experimental Research on Breakage Characteristics of Feed Pellets under Different Loading Methods" Agriculture 14, no. 8: 1401. https://doi.org/10.3390/agriculture14081401
Article Metrics
Supplementary material.
ZIP-Document (ZIP, 107 KiB)
Further Information
Mdpi initiatives, follow mdpi.
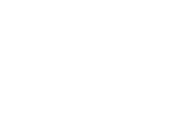
Subscribe to receive issue release notifications and newsletters from MDPI journals
Grab your spot at the free arXiv Accessibility Forum
Help | Advanced Search
Computer Science > Computer Vision and Pattern Recognition
Title: kan you see it kans and sentinel for effective and explainable crop field segmentation.
Abstract: Segmentation of crop fields is essential for enhancing agricultural productivity, monitoring crop health, and promoting sustainable practices. Deep learning models adopted for this task must ensure accurate and reliable predictions to avoid economic losses and environmental impact. The newly proposed Kolmogorov-Arnold networks (KANs) offer promising advancements in the performance of neural networks. This paper analyzes the integration of KAN layers into the U-Net architecture (U-KAN) to segment crop fields using Sentinel-2 and Sentinel-1 satellite images and provides an analysis of the performance and explainability of these networks. Our findings indicate a 2\% improvement in IoU compared to the traditional full-convolutional U-Net model in fewer GFLOPs. Furthermore, gradient-based explanation techniques show that U-KAN predictions are highly plausible and that the network has a very high ability to focus on the boundaries of cultivated areas rather than on the areas themselves. The per-channel relevance analysis also reveals that some channels are irrelevant to this task.
Comments: | Accepted at ECCV 2024 CVPPA Workshop |
Subjects: | Computer Vision and Pattern Recognition (cs.CV); Artificial Intelligence (cs.AI) |
Cite as: | [cs.CV] |
(or [cs.CV] for this version) | |
Focus to learn more arXiv-issued DOI via DataCite |
Submission history
Access paper:.
- HTML (experimental)
- Other Formats

References & Citations
- Google Scholar
- Semantic Scholar
BibTeX formatted citation

Bibliographic and Citation Tools
Code, data and media associated with this article, recommenders and search tools.
- Institution
arXivLabs: experimental projects with community collaborators
arXivLabs is a framework that allows collaborators to develop and share new arXiv features directly on our website.
Both individuals and organizations that work with arXivLabs have embraced and accepted our values of openness, community, excellence, and user data privacy. arXiv is committed to these values and only works with partners that adhere to them.
Have an idea for a project that will add value for arXiv's community? Learn more about arXivLabs .
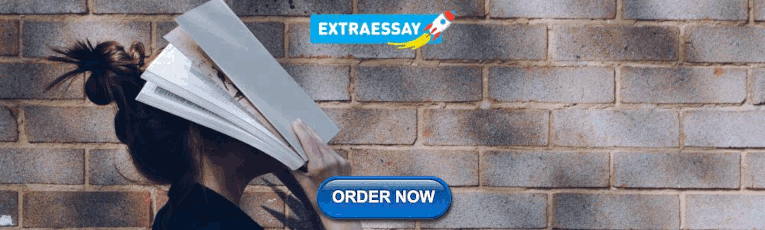
IMAGES
COMMENTS
Building productive relationships between farmers and scientists is critical to developing new innovation pathways to solve the challenges of contemporary agriculture. On-farm experimentation (OFE ...
Experimental Agriculture is a UK-based journal that publishes original research on crops, farming systems, and agricultural issues in tropical and sub-tropical regions. It has a high SJR of 54 and covers the Agronomy and Crop Science subject category.
The article reviews how experimental research in agriculture has moved from lab to field, with examples of new technologies, information, institutions, and transformations. It also discusses the methodological challenges and adaptations of field experiments in agriculture.
Experimental Agriculture. [...] Over a period of seven years farmers participated in trials in which common vetch (Vicia sativa (V)) or chickling (Lathyrus sativus (C)) replaced the fallow in a ...
This is the website for the book "Experimental methods in agriculture", where we deal with the organisation of experiments and data analyses in agriculture and, more generally, in biology. Experiments are the key element to scientific progress and they need to be designed in a way that reliable data is produced.
Experimental Agriculture publishes original research on field, plantation and herbage crops grown for food, feed or industrial purposes, and on farming systems. It also covers methods, issues and reviews of agricultural research, with a focus on the tropics and sub-tropical regions.
The agricultural value of long-term experiments: testing sustainability Our only realistic assessment of the sustainability of an agricultural system is the yields of the crops grown, and for those yields to be maintained or increased there must be a match between the crop, soil, climate and management of the system.
Cornell AES is vital to New York state agriculture and food security. We contribute to healthy and resilient communities, and improve the lives and well-being of people by advancing research on agriculture and food systems, the environment, applied economics, and community and individual development.
Parallel to organizational changes in agricultural experiments, researchers concerned with the validity of their trials developed new experimental methods for agriculture.
Description American Journal of Experimental Agriculture (ISSN: 2231-0606) is a multidisciplinary journal in the field of agriculture and biology. The journal publishes original scientific papers, short communications, review articles and case studies. Subject matters include all areas of agricultural and biological science viz., agronomy, agricultural chemistry, entomology, plant pathology ...
Basics of Experimental Design. or call (301) 779-1007 to order. The previous section summarized the 10 steps for developing and implementing an on-farm research project. In steps 1 through 3, you wrote out your research question and objective, developed a hypothesis, and figured out what you will observe and measure in the field.
Journal of Experimental Agriculture International is a multidisciplinary journal in the field of agriculture and biology. The journal publishes original scientific papers, short communications, review articles and case studies.
A peer-reviewed journal that publishes original research articles on various aspects of agricultural sciences. Browse the latest publications, most cited documents, and related sources on topics such as genetic diversity, crop production, and soil quality.
About the Journal Journal of Experimental Biology and Agricultural Sciences (JEBAS) is an online platform for the advancement and rapid dissemination of scientific knowledge generated by highly motivated researchers in the field of agricultural and biological sciences. The journal also welcomes articles of inter-disciplinary nature work. JEBAS publishes high-quality original research and ...
An agricultural experiment station ( AES) or agricultural research station ( ARS) is a scientific research center that investigates difficulties and potential improvements to food production and agribusiness. Experiment station scientists work with farmers, ranchers, suppliers, processors, and others involved in food production and agriculture .
This article aims to provide a comprehensive guide on experimental design and analysis specifically tailored for agricultural science. By integrating theoretical insights with practical examples ...
Particle breakage is a common phenomenon during the processes of production, storage, and transportation. Because of the requirements for pellet integrity in poultry farming, research on the breakage characteristics of feed pellets is necessary. In this paper, repeated compression tests under different loading forces and repeated impact tests under different air pressures were carried out with ...
Center for Agriculture, Food, and the Environment Center for Agriculture, Food, and the Environment Stockbridge Hall, 80 Campus Center Way University of Massachusetts Amherst Amherst, MA 01003-9246 Phone: (413) 545-4800 Fax: (413) 545-6555 [email protected] Civil Rights and Non-Discrimination Information. Our Covid-19 Response & Resources
View PDF HTML (experimental) Abstract: Segmentation of crop fields is essential for enhancing agricultural productivity, monitoring crop health, and promoting sustainable practices. Deep learning models adopted for this task must ensure accurate and reliable predictions to avoid economic losses and environmental impact.