Random Dot Stereogram
Activity length, activity type, demonstration.
In this demonstration, students discover how the structure and placement of the eyes creates depth perception.
Depth perception occurs as your brain compares the pictures received in each eye to figure out how far objects are from you. Each eye sees a slightly different picture because they're in slightly different places.
If you use first one eye then the other to view an object, nearby objects tend to jump back and forth more, whereas faraway objects hardly seem to move at all.
In the first part of the demonstration, one eye sees the object covered by the index finger. The other eye has an unobstructed view. This demonstrates that each eye is getting a different picture of the world.
Single image random dot stereograms are the original versions of the popular 'Magic Eye' pictures in which a 3D image pops out from what appears to be a sheet of random dots. The dots are arranged in repeating pattern, with slight differences in each repetition. Each eye sees a slightly different pattern because of the different angles between the page and each eye. Your brain tries to overlap the two patterns, and creates the virtual 3D object.
If you want to view movies or pictures in 3D, you have to show a different picture to each eye. That's why they give you glasses with 2 lenses of different colours (or let through light of different polarities.)
Describe how depth perception affects how the brain forms an image.
a selection of single image random dot stereograms , printed on either an overhead sheet or on a large sheet of paper overhead projector (optional)
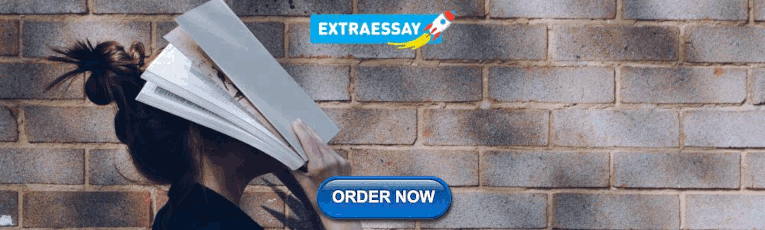
Key Questions
- Do both eyes have the same point of view of the objects before them?
- If you bring your finger closer to your face, do you find it jumps back and forth more or less than when it’s farther away?
- What was your method for “seeing” the image?
Part 1: Depth Perception
- Focus on a person or object.
- Hold up your index finger at arm’s length.
- Close your left eye. Looking only with your right eye, move your finger so it covers the object.
- Now look with just your left eye.
Part 2: Stereograms
- Show random dot stereograms to students.
- Try and see what image is “hidden” in the stereograms.
Note: Stereograms are difficult to make out on computer screens
- How would being blind in one eye affect your depth perception? Why?
- How are random dot stereograms created?
About the sticker
Artist: Jeff Kulak
Jeff is a senior graphic designer at Science World. His illustration work has been published in the Walrus, The National Post, Reader’s Digest and Chickadee Magazine. He loves to make music, ride bikes, and spend time in the forest.
Comet Crisp
T-Rex and Baby
Artist: Michelle Yong
Michelle is a designer with a focus on creating joyful digital experiences! She enjoys exploring the potential forms that an idea can express itself in and helping then take shape.
Buddy the T-Rex
Science Buddies
Artist: Ty Dale
From Canada, Ty was born in Vancouver, British Columbia in 1993. From his chaotic workspace he draws in several different illustrative styles with thick outlines, bold colours and quirky-child like drawings. Ty distils the world around him into its basic geometry, prompting us to look at the mundane in a different way.
Western Dinosaur
Time-Travel T-Rex
Related Resources
It is often said that seeing is believing, but can we really trust what our eyes are telling us in…, related school offerings.
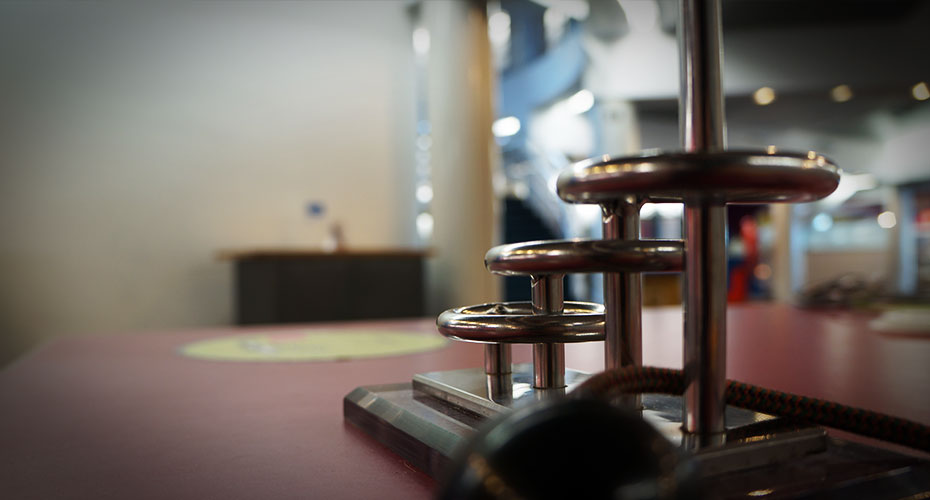
Visit Puzzles and Illusions Gallery

Eyeball Optics
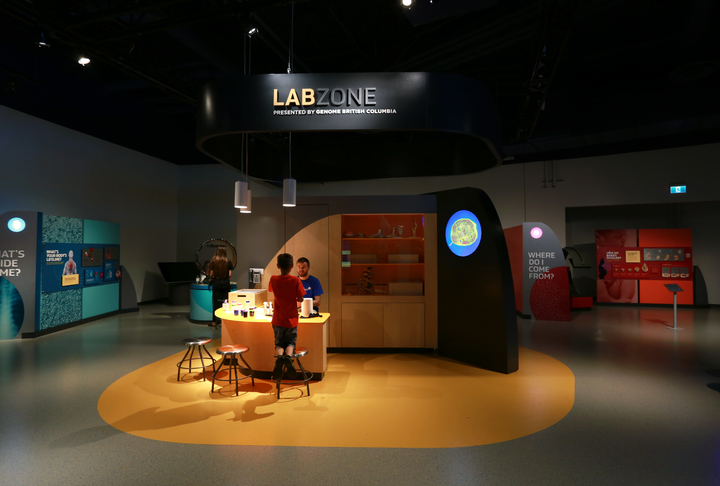
Visit BodyWorks Gallery
We believe that now, more than ever, the world needs people who care about science. help us fund the future and next generation of problem solvers, wonder seekers, world changers and nerds..
Stereogram Tutorial
Julio M. Otuyama
Introduction
Stereograms are illusions of 3D surfaces. This tutorial shows how it works, with a simple "do it by yourself" approach. The random dot stereogram is very easy to understand, although not a trivial concept. Unlike holograms that require laser beams, stereograms can be created, printed and visualized with computers. But it may not be an easy task to see its 3D surfaces. Stereo Pairs / Stereograms Stereo Pairs / stereograms work because the brain can detect small differences between the images seen by each eye. Stereo pairs have been studied for a long time - Leonardo da Vinci almost discovered the concepts of stereo pair visualization. But only Wheatstone correctly described these concepts. Random dot stereograms is a very new discovery, developed by Bela Julezs, during 1970's. Random Dot Stereograms give us a deep impact because there is an invisible hidden surface. Stereo pair random dot stereogram Hidden surface: square Visualization The visualization of a stereogram requires an unusual skill: each eye must be targeted to distinct places on the image. This is unusual to our brains. That is the reason some people hardly (or can not) see stereograms. There are two ways to visualize stereograms: divergent view convergent view The target of each eye (left and right) is shown: regular visualization - no stereogram divergent visualization of a stereogram convergent visualization of a stereogram (cross) Once one gets the divergent or convergent visualization, he/she has a clear view of 3D surfaces. Helper to visualization: divergent: Eyes relax (infinite focus) Transparent media (stereogram on a plastic film) convergent: Reference point between the eyes and stereogram (put a pen in the half way from your eyes to the stereogram)
Random Dot Stereogram
Our brain is able to perceive the 3D information of the environment. The main reason for that are small differences between each eye image. Both images are captured with a small difference (the distance between our eyes). This results in very small shifts on each retina projection image, hard to notice. But although small, those differences give us the 3D sensation. Creating a Random Dot Stereogram The easiest way to learn how a stereogram works is to create one. Next, the recipe to generate a random dot stereogram (adapted from Bela Julesz article). There is also a second approach, based on "ASCII Stereogram". With the ASCII Stereogram, it is easier to understand the disparity shifts. You can see both as stereograms, if you have skill enough. Steps to generate a stereogram: Procedure steps Random Dot Stereogram ASCII Stereogram (a) Create a random dot image ABCDEFGHI JKLMNOPQR STUVWXYZ0 123456789 0ZYXWVUTS RQPONMLKJ IHGFEDCBA (b) Duplicate the image and put side by side ABCDEFGHI ABCDEFGHI JKLMNOPQR JKLMNOPQR STUVWXYZ0 STUVWXYZ0 123456789 123456789 0ZYXWVUTS 0ZYXWVUTS RQPONMLKJ RQPONMLKJ IHGFEDCBA IHGFEDCBA (c) Select a piece of one image ABCDEFGHI ABCDEFGHI JKLMNOPQR JKLMNOPQR STU VWX YZ0 STU VWX YZ0 123 456 789 123 456 789 0ZY XWV UTS 0ZY XWV UTS RQPONMLKJ RQPONMLKJ IHGFEDCBA IHGFEDCBA (d) Horizontal shift of this piece of image ABCDEFGHI ABCDEFGHI JKLMNOPQR JKLMNOPQR STU VWX YZ0 ST VWX XYZ0 123 456 789 12 456 6789 0ZY XWV UTS 0Z XWV VUTS RQPONMLKJ RQPONMLKJ IHGFEDCBA IHGFEDCBA The stereogram is ready! The hidden image is the piece shifted ABCDEFGHI ABCDEFGHI JKLMNOPQR JKLMNOPQR STU VWX YZ0 ST VWX XYZ0 123 456 789 12 456 6789 0ZY XWV UTS 0Z XWV VUTS RQPONMLKJ RQPONMLKJ IHGFEDCBA IHGFEDCBA Convergent/Divergent view and disparity With a divergent view, the shifted square appears as floating (nearer than everything else). With a convergent view the shifted square appears as a hole (farther than everything else). Disparity is the concept that explains why we get the 3D perception of the nearer and the farther distance. This concept can be explained with "ASCII Stereogram". Let's call disparity as the number of letters to to go from a letter in the left pair to its counterpart at the right pair. For instance, from the first letter "A" of the left pair takes 10 letters of disparity to reach its counterpart in the right pair: ABCDEFGHI A ^123456789^ On the (b) step, all the letters have the same disparity (it is only a flat surface). The shift on step (d) changes the disparity. For instance, the letter " V " now takes 9 letters of disparity to reach its counterpart in the right pair: VWX YZ0 ST V ^12345678^ With a divergent view this means that our eyes have to "move a little" to perceive this as the same letter by both eyes. The "movement" required is the right eye to move a little toward the left, just like we were seeing things nearer. That is why this letter is perceived as nearer than the background. As a matter of fact, our eyes don't move, but the image projected on the retina causes this effect, and our brain is able to detect this. Now we can make a relationship of shifts and disparity: Left Pair Right Pair Increase Disparity Shift to Left Shift to Right Decrease Disparity Shift to Right Shift to Left And a relationship of disparity and divergent/convergent view: Smaller Disparity Larger Disparity Divergent View Float Near Float Far Convergent View Float Far Float Near The disparity causes inverse effects with divergent versus convergent views because of the cross in the convergent view. Try it (MS Windows 3.x/95/98/me/NT/2000/XP/2003/Vista/7/8/10)! This lab can be done on your computer in a few moments. No extra software is required. This description is based on MS Windows / IE, but it's easy to adapt to Apple Mac, Linux, etc. Steps to generate a Stereogram: Save this image on your computer: Open the image with an image edition tool (Paint, Paintbrush, Photoshop, etc) Select a small piece of one image with the selection tool (usually the dotted square) Shift the selection (horizontal shift only) The resulting image is a random dot stereogram made by you ! Hint: you can select any kind of shape, even letters ( yes, your name! ). But the shape must be large enough for our brain to detect it.
SIRDS - Single Image Random Dot Stereogram
The Random Dot Stereogram is a scientific proof (see below), but what made this concept widely known was the illustrations called SIRDS (books like Magic Eye, CG Stereogram, etc). To create a SIRDS, you have to put not two, but a repeating pattern of random dots, and make shifts to get the desired effect. Instead of black and white random dot patterns, you can use a colored pattern to fill the repetition. To create complex surfaces there are computer algorithms that copy the pattern side by side based on grayscale disparity maps. Grayscale disparity maps The easiest way to create stereograms with complex surfaces is to use grayscale disparity maps. The grayscale disparity map is a representation of some surface with each gray tone associated with a distance. For instance, you could create a background black disparity map, with a floating square near, represented by a white square. To create a random dot stereogram based on this disparity map, you have to copy (or copy and shift) the random dot pattern based on the corresponding coordinate content color in the disparity map. When the color is black, just copy the pixel to the other image. If the color is white, copy the pixel to the other image and shift it. The figure below illustrates this procedure. This is almost the same random dot stereogram presented previously. Grayscale disparity map (only one cycle) The disparity map associates gray tones to distances: black - background distance gray and white - floating squares at different distances Copy the pixel based on the corresponding coordinate content color of disparity map: copy pixel with no shift if the disparity map content is black copy pixel with small shift if the disparity map content is gray copy pixel with large shift if the disparity map content is white Result: Random Dot Stereogram black: background gray square: small disparity white square: large disparity Creating a SIRDS Now we can create a generic SIRDS with a (not so) complex surface. There are many cycles to compose the SIRDS because one cycle requires the pattern from the previous cycle. That is the reason SIRDS requires computer programs to generate. It is a difficult task to copy the pattern based on the disparity map, and this copy requires data only available after the computation of each cycle. Sometimes there are holes with no pattern after cycle copy, that requires artificial filling with extra patterns. Sometimes the same pattern is copied many times at very close pixels, creating an (undesirable) visual clue of the hidden surface. As a matter of fact, a computer program doesn't need to implement the cycles, just copy a non-transparent pixel to another pixel as many times as is required. The cycle is just a consequence of the procedure. This procedure shows a black (no shift) and white (shift) copy. With a general grayscale disparity map, the shift can be small or big, based on how black or white the gray tone is. Usually the image is processed with higher resolution than the original, to achieve a sub pixel shift accuracy. Grayscale disparity map (many cycles) Disparity Map Cycle 1 Cycle 2 Cycle 3 Cycle 4 Result: SIRDS Someone could try to move the whole white selection with just one shift, avoiding the cycles. It wouldn't work because the disparity of the background and the whole selection is the same. There is another approach to generate SIRDS, with an algorithm based on ray tracing concepts. The result is almost the same, with no visible differences, but it takes a lot more computer resources. Yes, I did implement a ray tracing algorithm to create stereograms - because at that time there was no information of how stereograms worked, and I had no idea who developed those illustrations (there was no stereogram books yet; I could only get a copy from an anonymous copy (of a copy) of a one page stereogram illustration). Everybody was amazed with that illusion. I was learning C programming language during my under graduation, so the ray tracing stereogram program was a good exercise. Later I found information about Bela Julesz and random dot stereograms.
An interesting illusion
The random dot stereogram is described in the "Foundations of Cyclopean Perception" article of Bela Julesz. This article is not about entertainment, but a scientific procedure to show how our brain works. In this article Bela Julesz shows that with the random dot stereogram: Random dot stereogram pair conveys information that is lost with only one image presentation. Each image does not have any clue about 3D information (shape from shade, contours, etc); Our brain is able to extract the 3D information from a random dot stereogram (you just need to visualize it). So, this shows that: There is 3D information detection from the horizontal shifts (brain stereopsis skill); This 3D information can not be detected at retina stage; Detection happens somewhere that receives information from both eyes (binocular stage).
Stereogram Gallery
Images: Flag of Brazil , Wine Glass , Rings , USP , Pigeons , Birds , Half Sphere , Helicoid Flash animation: Hanabi Stereogram QR Stereo Code HTML5: Generate SIRDS online
External Links
Foundations of Cyclopean Perception - Bela Julesz Book Eye, Brain and Vision (EBV) from David Hubel . That is a good introduction to human vision from a neuro-scientist. It is a scientific book, but it is very easy to read. Stereogram , Autostereogram , Christopher Tyler
Back to home page

Instructions
- Illustration
The correspondence problem is how the visual system determines which object’s image in one eye matches the same object’s image in the other eye. Here we explain how stereograms can be used to study the correspondence problem and potentially derive some solutions. How the visual system is able to solve the correspondence problem is quite complex computationally. The specifics of this computation were the subject of a debate that took place about the nature of stereopsis. On one side was the view that the visual system must bring to bear knowledge of objects and use that knowledge to match images. The other view was that the visual system matches the left and right images before bringing knowledge to bear on the situation.
In order to distinguish these hypotheses, Hungarian American vision researcher Béla Julesz invented randomdot stereograms (Julesz, 1971). Random-dot stereograms are stereograms in which the images consist of a randomly arranged set of black and white dots. The lefteye and right-eye image are arranged identically, except that a portion of the dots is moved to the left or the right in one of the images to create either a crossed or an uncrossed disparity. This creates the experience that part of the image is either in front of or behind the rest of the dots.
In this activity, you can experience random dot stereogram, manipuate the disparity of a small square and the backgroun, make the square move, and even have the dots change constantly.
Full Screen Mode
To see the illustration in full screen, which is recommended, press the Full Screen button, which appears at the top of the page.
Illustration Tab
On the Illustration tab, you can start, stop and alter the motion of squares to experience optic flow.
Below is a list of the ways that you can alter the illustration. The settings include the following:
Type of Anaglyph : Controls the way the anaglyph is being created. You can choose from Red-Cyan, Red-Blue, and Red-Green. The first word is the color for the left eye. The default is Red-Cyan which are the type of anaglyphs used in the book. Square Disparity : The disparity of the dots in the square in number of pixels on the screen. Positive values refer to crossed disparity. Background Disparity : The disparity of the background dots in number of pixels on the screen. Negative values refer to uncrossed disparity. Size of Dots : Make the dots bigger and larger. Update Stereogram : Static refers to the dots do not change. Dyanimc refers to the dots constantly changing with new random patters always being presented. You get a faster update with larger dots. Move : Have the square move randomly over the background. Center : Redraw the small square in the center of the screen. Speed of Square : How fast the square moves when moving.
Pressing this button restores the settings to their default values.
Proceed to Quiz
- Subscriber Services
- For Authors
- Publications
- Archaeology
- Art & Architecture
- Bilingual dictionaries
- Classical studies
- Encyclopedias
- English Dictionaries and Thesauri
- Language reference
- Linguistics
- Media studies
- Medicine and health
- Names studies
- Performing arts
- Science and technology
- Social sciences
- Society and culture
- Overview Pages
- Subject Reference
- English Dictionaries
- Bilingual Dictionaries
Recently viewed (0)
- Save Search
- Share This Facebook LinkedIn Twitter
Related Content
Related overviews.
visual illusion
More Like This
Show all results sharing these subjects:
random-dot stereogram
Quick reference.
A type of anaglyph for studying binocular depth perception invented by the Hungarian-born US radar engineer and psychologist Bela Julesz (1928–2003) at Bell Telephone Laboratories in 1960, consisting of two random arrays of dots that when viewed stereoscopically, one array being viewed by each eye, appears to contain a form such as a triangle or square lying in a plane either in front or behind the rest of the dots, bounded by an illusory contour. It is constructed by generating two arrays of randomly placed dots, identical except for a clearly defined region that is slightly shifted sideways in one of the arrays, and it is usually presented for viewing by printing one of the arrays in red and the other in green or cyan (blue-green), with a slight horizontal displacement so that the unshifted dots do not fall exactly on top of one another, and it is viewed with spectacles having one red and one green or cyan lens. Also called a Julesz stereogram . See also Cyclopean perception, stereopsis, visual illusion. Compare random-dot kinematogram. [From Greek stereos solid + gramme a line]
From: random-dot stereogram in A Dictionary of Psychology »
Subjects: Science and technology — Psychology
Related content in Oxford Reference
Reference entries, random-dot stereogram n..
View all related items in Oxford Reference »
Search for: 'random-dot stereogram' in Oxford Reference »
- Oxford University Press
PRINTED FROM OXFORD REFERENCE (www.oxfordreference.com). (c) Copyright Oxford University Press, 2023. All Rights Reserved. Under the terms of the licence agreement, an individual user may print out a PDF of a single entry from a reference work in OR for personal use (for details see Privacy Policy and Legal Notice ).
date: 10 August 2024
- Cookie Policy
- Privacy Policy
- Legal Notice
- Accessibility
- [185.126.86.119]
- 185.126.86.119
Character limit 500 /500
Random Dot Stereogram
Imbedded in the computer generated "random dot matrix" below is the 3-D image of the word: PHOTOREAD. Seeing the 3-D image is a fun way to achieve PhotoFocus, the divergent gaze used for PhotoReading.
Allow your eyes to space out and gaze through the paper. You may watch the two dots at the top of the page become four. Each eye sees two dots independently. Overlap the two dots in the center so that you see three dots. When the two central dots fuse into one, just relax and hold your gaze steady, while observing the rest of the image in your peripheral vision. Hold the fused dots and glance slowly around the page. When you hold the patterns, your brain will develop the 3-D image.
Hold the page so close to your eyes that it touches your nose. Relax your eyes so they are uncrossed and you see a blurry field of dots. Wait long enough to become comfortable, just spacing out and looking through the image. Now, slowly, no more than an inch per second, move the page away from your face. Hold the image level at all times. Observe the patterns on the page but do not look at the page. Notice the two dots appear as four. When the image is several inches away, the four dots will fuse into three. Relax, and you will start to sense depth in the picture. Let your brain develop the picture. If you focus on the page itself, the effect will disappear.
Be patient, and soon the 3-D letters will emerge.

Was this article helpful?
Related Posts
- More on the Photo Focus State
- How to Photo Read - Mind Mapping
- The Photo Reading Whole Mind System
- Whole Mind Syntopic Reading
- Technology - Mind Mapping
Readers' Questions
How to view a stereogram?
A stereogram is a two-dimensional image which, when viewed with proper technique, creates the illusion of three-dimensional depth. To view a stereogram, look at it without moving your eyes and relax them until the two-dimensional patterns start to form patterns. Stare at a single point for a few seconds, then slowly move your eyes around the image. You should notice three-dimensional shapes, objects, or patterns emerging from the image.
How to read stereograms?
A stereogram is an image that looks like a random collection of dots or shapes, but when you look at it in a certain way, a 3D image appears. To read a stereogram, you need to relax your eyes and focus on a spot in the image. Try to let your eyes go out of focus, while keeping your gaze on the spot. When you do this, the image should begin to come into focus and the 3D image will appear.
How to read stereogram?
Stereograms are two-dimensional images that contain a hidden 3D image. To view the 3D image, you need to cross your eyes until the two images overlap and merge into one. When viewed correctly, an image will appear to float above or below the background. This is known as the "magic eye" effect.
Perception Lecture Notes: Depth, Size, and Shape
Professor david heeger, what you should know about this lecture.
- binocular rivalry and the neural correlates of visual awareness
Stereo Vision

Close one eye, and hold up your two index fingers, one fairly close to your face and one as far as you can reach. Fixate the more distance hand, and alternately view the scene with your left eye and then your right. As you can see, the distance between the two fingers is different in your left than in your right eye; their relative positions in the two retinae are disparate .

Binocular disparity is defined as the difference in the location of a feature between the right eye's and left eye's image. The amount of disparity depends on the depth (i.e., the difference in distance to the two object and the distance to the point of fixation), and hence it is a cue that the visual system uses to infer depth. Wheatstone (1838) was first to figure this out. Before that, people were confused, thought that having two eyes posed a problem because couldn't figure out how you could see only one image when viewing the world with two eyes. Wheatstone correctly pointed out the advantage of having two eyes to see objects in 3D depth. However, the disparity also depends on the distance to the fixation as well, so that disparities must be further interpreted using estimates of the fixation distance.

Horopter: imaginary 3D surface in the room in front of you that includes the object you are fixating on and all other points in 3D space that project to corresponding positions in the two retinae. The geometric horopter (the set of points with zero disparity) is a circle that includes the fixation point and the optical centers (lenses) of the two eyes.

Uncrossed disparity: An object farther away from you than the horoptor has uncrossed disparities. You'd have to uncross (diverge) your eyes to fixate on it. It lies further to the right from the right eye's viewpoint than from the left eye's viewpoint.
Crossed disparity: An object closer than the horoptor has crossed disparities. You'd have to cross (converge) your eyes to fixate on it. It is further to the left from the right eye's perspective.
Stereoscope: One way to view stereo image pairs is to use a mirror stereoscope. If you put your face in front of a pair of angled mirrors, and put two slightly different pictures off to the sides, your left eye will see the left picture (E') and your right eye will view the right-hand picture (E).

Stereogram: A pair of images (such as E/E' above) that are viewed using a stereoscope (or a red-green anaglyph). The two images in a stereogram are slightly different, with features in one image shifted to slightly different positions in the other image. The shifts mimic differences which ordinarily would exist between the views of genuine 3D objects.
There are lots of ways to make and view stereograms. The basic concept is to present slightly different images to the two eyes. One way is to superimpose two half images, one in red and one in green. Viewed through red-green glasses, one eye sees the red image and the other eye sees the green image.

Stereograms have been part of popular culture in each generation since Wheatstone. Brewster stereoscopes (different design, but same in concept) were popular around 1900 with photographed stereo pairs. 3D movies were viewed with red-green anaglyph glasses in the 1950s. Current 3D movies are usually viewed with polarized glasses instead of red-green so the movies can be in color. Another recent technique is the "magic eye" autostereograms.
Random-dot stereogram: The random-dot stereogram was invented by Bela Julesz, a perceptual psychologist who was very influential over the past 30 years. In the example below, with anaglyph glasses you would see a square-shaped surface floating in depth in front of a background. Both the foreground square and the background have little dots painted on them in random locations.

This has important consequences. It indicates that:

To construct a random-dot stereogram, you first place a bunch of dots randomly in an image. Then make two copies of it. In one copy shift a central square region to the left and in the other copy shift the same central square region to the right. This leaves holes in each of the images (left over from where the square shifted from). Fill the holes with new random dots. Why do you see it in 3D? The shift mimics differences which ordinarily exist between the views of genuine 3D objects. The extra dots (X and Y above) correspond to those parts of the background that one eye can see, but which are occluded from the view of the other eye by the foreground square.
How does the visual system see depth in a random-dot stereogram? One hypothesis is that the visual system matches up features of similar shape, size, contrast, etc. to estimate disparity. But, there can be lots of potential matches. In principle each dot present in one row of one half-image could have a large number of matches in the other half-image.
This problem of resolving this ambiguity is known as the problem of global stereopsis because the brain must find the correct overall (global) set of matches. It can't just try to find a mate for each feature independently. Global stereopsis is not just an issue for random-dot stereograms. Natural scenes (e.g., tree with leaves, carpet, etc.) have similar features. The visual system "solves" the global stereopsis problem by using additional constraints. For example, nearby points in the image are usually at nearby positions in depth, hence have nearly the same disparity.
Autostereogram: The autostereogram is also known as a "magic eye" stimulus. The trick is to display slightly different images to the two eyes. The autostereogram works by having repetitive patterns. To see depth in an autostereogram, you need to either cross or diverge your eyes so that they fixate separately on two different repeats of a repetitive pattern. In this way, you effectively get two different images to the two eyes. A simple example is the wallpaper illusion. If you view vertically striped wallpaper and fixate one eye on one stripe and the other eye on the another stripe, the stripes appear to pop out in depth in front of the wall. This is hard to do because you need to fixate on a point that is effectively in front of the picture while focusing/accomodating on the picture itself. Note that the depth you will perceive will be the opposite if you cross-fuse an autostereogram than if you diverge-fuse it.
Stereoblindness: 10% of people are stereoblind. Some are totally stereoblind, some are blind only to either crossed or uncrossed disparities. Some stereoblindness is caused by strabismus (wandering eye). If not treated/fixed at a very early age (infancy), binocular vision never develops properly. Some people with strabismus end up with amblyopia (sometimes called lazy eye ). Amblyopia is a cortical blindness. Amblyopia is a general term for a visual deficit that has nothing to do with the optics or structure of eye and retina. In amblyopia, the brain basically ignores inputs from one eye. Other people with untreated strabismus end up as alternate fixators who can see with either eye, but never use them both at the same time. That is, they first look at you with their left eye (while the right eye is diverged), then switch and look at you with their right eye (while the left eye is diverged). In either case, there is no binocular vision and no stereopsis.
Stereovision in the brain
If you record with a micro-electrode from a V1 neuron while an animal views oriented lines presented separately to the two eyes and vary the disparity, some neurons are selective for particular disparities.

This neuron does not respond at all when a line is shown to one eye at a time. To get a response, the line must be presented simultaneously to both eyes, it must have the correct orientation, direction of motion and the correct binocular disparity, in this case a disparity of about 1/2 deg of visual angle.

You have two eyes, and hence two visual fields. A big question in the early 19th century (before Wheatstone): we have two eyes, why don't we alway see two views of the world? Answer: the two images are combined in the brain to yield a single unified perceptual experience.

Panun's fusional area is the range of disparities, or equivalently the range of depths in 3D space on either side of the horoptor, over which the visual system can successfully fuse the two views. If disparity is small enough, within Panum's fusional area, then the visual system suceeds in fusing the two views. If disparity is too large then the neurons in the brain cannot cope with it to create single vision and you either get: diplopia or suppression or binocular rivalry .
Diplopia (double vision): Look at a distant object with boths eyes open. While fixating that object, put your index finger about 6 inches in front of your face. You will see two index fingers (one from the left eye's image and one from the right).
Suppression: This is what normally happens when the retinal disparity is too big (outside of Panum's fusional area). One eye's view dominates. That one is perceived. And the other eye's view is suppressed from awareness.
Binocular rivalry: This is a phenomenon we experience when the two eyes' views are very different from one another. One eye's view dominates for several seconds and is then replaced by that of the other eye. For example, if a horizontal grating presented to one eye and a vertical grating in the other eye, in the percept one might first see the horizontal for a few seconds, then a mixture, then the vertical for a few seconds, etc. The phenomenon of binocular rivalry is of particular interest in studying consciousness/visual awareness because the physical stimuli (the two gratings) do not change, yet the conscious percept changes dramatically over time. Moreover, we have no conscious control over the percept; you cannot by force of will cause the percept to switch from one to the other.

Summary: There are two ways to have single vision. (1) Small disparity yields fusion and stereopsis. (2) Large disparity often causes one eye's view to be suppressed. Binocular rivalry is a special case of suppression in which the suppression switches back and forth between the two views. Note that you can have stereopsis in part of the visual field, diplopia in another part of the visual field and rivalry/suppression in yet another part of the visual field, all simultaneously.
Binocular rivalry in the brain
Neural correlates of conscious perception can be measured experimentally using perceptual illusions in which the percept is dissociated from the physical stimulus. Binocular rivalry is an example of such perceptual illusion that has been used for research on the neural correlates of consciousness. Binocular rivalry occurs when different visual stimuli are presented simultaneously to each eye. Typically, awareness of one or the other stimulus is suppressed so that we are consciously aware of one stimulus at the time, never both. One eye’s view dominates consciousness for several seconds, only to be replaced by the other eye’s view. What makes binocular rivalry so remarkable is that the perceptual experience fluctuates while the physical stimulus remains constant. Because of this dissociation, binocular rivalry presents a unique opportunity for studying the neural correlates of consciousness.
According to one idea, binocular rivalry occurs because neurons in the early stages in visual processing respond to the physical stimulus of each eye, whereas neurons in later stages of visual processing are switched on and off and cause the perceptual alternations. Somewhere between these early and later stages the neuronal signals conveying one of the two stimuli are suppressed, as if there was a “gate” to visual consciousness. V1 is the first place in the visual processing pathways of the brain where this "gate" could be located, because it is the first place where the signals from the two eyes come together.

Does such a gate exist? If so, what neurons in the brain have this gating function? Are the neurons localized in particular brain areas? Are they a particular cell type? Does the gating occur through modulation of the cells’ firing rate or some other component of their responses (e.g., spike timing, synchronous firing)? What are the neural circuits and neural computations that support the competition between the two stimuli?
Although we do not yet have firm answers to these questions, we have begun to study this in my lab with fMRI. We capitalized on an interesting aspect of the perceptual phenomenon; during a perceptual alternation, one typically perceives a traveling wave in which the dominance of one pattern emerges initially at one location and expands progressively as it renders the other pattern invisible. Note again that there is no physical change in the stimulus while this conscious perceptual change is taking place it is all “in your head”. This experiment established that waves of activity in primary visual cortex (V1) accompanied the perceptual changes during binocular rivalry. By relying on the fact that visual cortex is retinotopically organized (neurons at nearby locations in visual cortex respond to nearby locations in the visually field), we showed that neural activity propagated over subregions of visual cortex in a manner that correlated with the dynamic perceptual changes experienced during binocular rivalry.
This movie shows a demonstration of our results. Right, Example of the temporal sequence of an observer’s perceptual experience. Left, fMRI responses. Gray scale, anatomical image passing through the posterior occipital lobe, roughly perpendicular to the Calcarine sulcus. Yellow highlights, V1 gray matter regions exceeding 75% of the peak response. Pay attention to the left hemisphere. The lower lip of the Calcarine sulcus responds first followed by the upper lip of the sulcus. Then the activity in the lower lip of the sulcus subsides before the activity in the upper lip does so. This activity in the brain (left) corresponds nicely to the dynamics of the perceptual changes (right), given the retinotopic organization of V1 (lower lip of left-hemisphere Calcarine sulcus corresponds to upper-right quadrant of visual field and upper lip of left-hemisphere Calcarine sulcus corresponds to lower-right quadrant of visual field). The same would be evident in the right hemisphere, but in a different slice through the brain. Red curve, fMRI responses measured from a subregion of V1 (lower lip of the left hemisphere Calcarine sulcus) corresponding retinotopically to the upper-right quadrant of the stimulus annulus. Green curve, fMRI responses measured from a subregion of V1 (upper lip of the Calcarine sulcus) corresponding retinotopically to the lower-right quadrant of the stimulus annulus. Yellow again indicates when the responses in each subregion exceeded 75% of their respective peaks. The green curve is delayed in time and larger in amplitude than the red curve, as expected from a travelling wave.
Indeed, activity in a number of brain areas correlates with the perceptual alternations of binocular rivalry, including not only primary visual cortex but also higher-order visual cortical areas in the inferior temporal lobe, and areas in parietal and prefrontal cortex. It is likely that these different cortical areas play different roles in visual perception during binocular rivalry.
Pictorial Depth Cues

What does the visual system do to deal with this ambiguity? Your visual system relies on multiple cues for estimating/inferring distance, depth and 3D shape.There are a large set of such cues: relative size, occlusion, cast shadows, shading, dynamic shadows (shadow motion), aerial perspective, linear perspective, texture perspective, and height within the image. Most of these are based on the concept illustrated above: the size of the retinal image of an object is proportional to the object's size, but inversely proportional to the distance to the object.

Texture provides 3 cues about shape/distance:
- texture elements become more dense with distance.
- texture elements become smaller with distance
- foreshortening (circles become ovals) when the surface is tilted away.

Brightness of a surface depends on its orientation with respect to the light source. The visual system assumes that the light comes from above. Brighter patches appear to be tilted up facing the light.

The interpretation of shape from shading interacts with the interpretation of shape from contours. These two images have the same shading, but different bounding contours, and you see different shapes.

The 3 white squares are identical to one another and they are placed in exactly the same way with respect to the checkerboard grid underneath. Only the shadows differ, giving the impression that the square on the right is floating higher above the checkerboard.

Linear perspective is another monocular depth cue. The distance between the rails is constant in the 3D scene but gets smaller and smaller in the image. This is a cue for distance. The visual system uses this to compare the sizes of objects. The two lines are the same length but the one on top appears bigger because it is seen as being further away and the visual system is compensating for the perspective. This compensation for distance in interpreting size is known as "size constancy".

Analogous to brightness constancy and brightness contrast, or color constancy and color contrast, we also experience size constancy and size contrast. The size of an object is interpreted relative to the objects around it and in the context of the other cues (e.g., linear perspective) for size and distance. The man in the pictures above is physically the same size in both photos (measure him) and he appears normal in size on the left but tiny on the right. The center circles in the drawings below are the same size but the one on the left looks bigger because it is surrounded by small circles and the one on the right looks smaller because it is surrounded by large circles.

A central principle of object perception is that we see objects in a three-dimensional world. If there is an opportunity to interpret a drawing or an image as a three-dimensional object, we do. The visual system compensates for perspective in making judgements about size. It is striking that we are so unaware of this. We have a tendency to interpret shape and size in 3D - often unaware of 2D size. The two table tops above have precisely the same two-dimensional shape on the page, except for a rigid rotation. Nobody believes this when they first look at the illusion. The illusion shows that we don't see the two-dimensional shape drawn on the page, but instead we see the three-dimensional shape of the object in space.
Monocular Physiological Cues
When we fixate an object, we typically accommodate to the object, i.e., change the power of the lens in our eyes to bring that object into focus. The accommodative effort is a weak cue to depth. Once we've accommodated to that distance, objects that are much closer or further from us than that distance are out of focus on our retina. Thus, blur is a cue that objects are at a different distance than the accommodative distance, although the cue is ambiguous as to whether the objects are closer or more distant. Even weaker still as depth cues (although theoretically useful) are the image distortions resulting from astigmatism (the cornea isn't a perfect sphere) and chromatic aberration (when yellow light is in focus, blue light is out of focus, from a given distance to the object).
Movement Cues
The next set of cues involve movement on the retinal image. There are two cases. If the observer moves through a stationary environment, the resulting movement is called motion parallax . Objects will move at different speeds on your retina (for a particular speed of observer movement and choice of fixation object) depending on their distance from the observer.
Motion parallax movie
In the second case, the observer is stationary but the object is in motion (e.g., it is rotating and/or moving in a straight path relative to the observer). The resulting retinal velocities will depend on the relative distance of each object feature from the observer resulting in the kinetic depth effect (the calculation of depth here is called structure-from-motion ).

- Keep it simple - don't use too many different parameters.
- Example: (diode OR solid-state) AND laser [search contains "diode" or "solid-state" and laser]
- Example: (photons AND downconversion) - pump [search contains both "photons" and "downconversion" but not "pump"]
- Improve efficiency in your search by using wildcards.
- Asterisk ( * ) -- Example: "elect*" retrieves documents containing "electron," "electronic," and "electricity"
- Question mark (?) -- Example: "gr?y" retrieves documents containing "grey" or "gray"
- Use quotation marks " " around specific phrases where you want the entire phrase only.
- For best results, use the separate Authors field to search for author names.
- Use these formats for best results: Smith or J Smith
- Use a comma to separate multiple people: J Smith, RL Jones, Macarthur
- Note: Author names will be searched in the keywords field, also, but that may find papers where the person is mentioned, rather than papers they authored.
- Annual Meeting Optical Society of America
- Technical Digest Series (Optica Publishing Group, 1988),
- paper THEE4
- • https://doi.org/10.1364/OAM.1988.THEE4
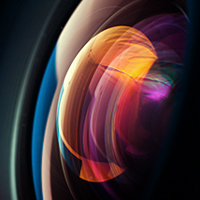
What we learn to see in random-dot stereograms
Alice O’Toole and Daniel Kersten
Author Affiliations
Brown U., Psychology Department, 89 Waterman St., Providence, RI 02912.
- Santa Clara, California United States
- 30 October–4 November 1988
From the session Vision: In-Depth (THEE)
- Annual Meeting Optical Society of America , Technical Digest Series (Optica Publishing Group, 1988), paper THEE4.%0Ahttps://opg.optica.org/abstract.cfm?URI=OAM-1988-THEE4%0A---------------------------------------------------------------------------%0AThis is sent to you as an email notification feature from Optica Publishing Group: https://opg.optica.org"> Email
- Share with Facebook
- X Share on X
- Post on reddit
- Share with LinkedIn
- Add to Mendeley

- Share with WeChat
- Endnote (RIS)
- Citation alert
- Save article
- Back to Top
In six experiments, we examined some properties of the learning effects reported for random-dot stereograms. The first three experiments offer a controlled replication of the retinal position-specific learning found by Ramachandran (1976) and a reexamination of the issue of random-dot pattern learning. Experiment 1 replicated the position-specific learning effect. Experiments 2 and 3 offered evidence that observers learn the monocular random-dot patterns and that this learning facilitates the depth percept. This result indicates that the traditional claim that random-dot stereograms are devoid of monocularly recognizable or useful forms should be reconsidered.
© 1988 Optical Society of America
A. I. Cogan and A. J. Lomakin MAA4 OSA Annual Meeting (FIO) 1992
G. Pellegrini WA1 Optical Fiber Communication Conference (OFC) 1983
Miguel Gonzalez-Herraez, Maria R. Fernandez-Ruiz, Hugo F. Martins, Carlos Becerril, Sonia Martin-Lopez, Ethan Williams, Zhongwen Zhan, Mariona Claret, Jose Lluis Pepegrí, Arantza Ugalde, Antonio Villaseñor, Cesar Ranero, Jorn Callies, and Kraig Winters SM1D.1 Optical Sensors (Sensors) 2023
Gislin Dagnelie IM3A.1 Imaging Systems and Applications (IS) 2015
Rafael G. González-Acuña and S. Thibault 1207803 International Optical Design Conference (IODC) 2021
OSA Annual Meeting 1988
- From the session Vision: In-Depth (THEE)
Confirm Citation Alert
Field error.
- Publishing Home
- Conferences
- Preprints (Optica Open)
- Information for
- Open Access Information
- Open Access Statement and Policy
- Terms for Journal Article Reuse
- Other Resources
- Optica Open
- Optica Publishing Group Bookshelf
- Optics ImageBank
- Optics & Photonics News
- Spotlight on Optics
- Optica Home
- About Optica Publishing Group
- About My Account
- Sign up for Alerts
- Send Us Feedback
- Go to My Account
- Login to access favorites
- Recent Pages
Login or Create Account
Academia.edu no longer supports Internet Explorer.
To browse Academia.edu and the wider internet faster and more securely, please take a few seconds to upgrade your browser .
Enter the email address you signed up with and we'll email you a reset link.
- We're Hiring!
- Help Center
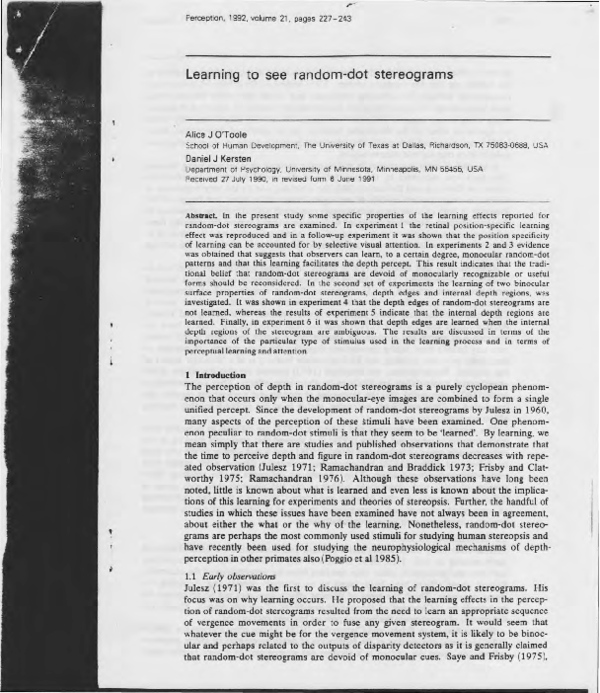
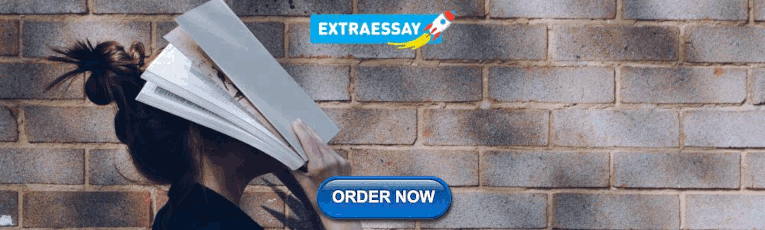
Learning to see random-dot stereograms

1992, Perception
In the present study some specific properties of the learning effects reported for random-dot stereograms are examined. In experiment 1 the retinal position-specific learning effect was reproduced and in a follow-up experiment it was shown that the position specificity of learning can be accounted for by selective visual attention. In experiments 2 and 3 evidence was obtained that suggests that observers can learn, to a certain degree, monocular random-dot patterns and that this learning facilitates the depth percept. This result indicates that the traditional belief that random-dot stereograms are devoid of monocularly recognizable or useful forms should be reconsidered. In the second set of experiments the learning of two binocular surface properties of random-dot stereograms, depth edges and internal depth regions, was investigated. It was shown in experiment 4 that the depth edges of random-dot stereograms are not learned, whereas the results of experiment 5 indicate that the in...
Related Papers
Saumil Patel
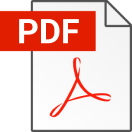
Vision research
Srividhya Vilupuru
The proposed influence of objects that are visible to both eyes on the perceived direction of an object that is seen by only one eye is known as the "capture of binocular visual direction". The purpose of this study was to evaluate whether stereoscopic depth perception is necessary for the "capture of binocular visual direction" to occur. In one pair of experiments, perceived alignment between two nearby monocular lines changed systematically with the magnitude and direction of horizontal but not vertical disparity. In four of the five observers, the effect of horizontal disparity on perceived alignment depended on which eye viewed the monocular lines. In additional experiments, the perceived alignment between the monocular lines changed systematically with the magnitude and direction of both horizontal and vertical disparities when the monocular line separation was increased from 1.1 degrees to 3.3 degrees . These results indicate that binocular capture depends ...
Journal of Clinical and Experimental Optometry, 95: 146–152
José Antonio Aznar-Casanova
Background: Single image random dot stereograms (SIRDS) have been used to study diverse visual parameters and skills. The aim of the present study was to identify the main optometric factors involved in the perception of SIRDS and to obtain a discriminant model to categorise our participants in terms of their skill in perceiving SIRDS. Methods: Response time was determined to assess the ability of 69 participants to perceive the hidden three-dimensional shape in an auto-stereogram presented under controlled conditions, whereupon three skill level groups were defined. The same participants were administered a battery of optometric tests to evaluate various aspects of accommodation and convergence, as well as stereopsis and phoria. Linear discriminant analysis, which served to examine the relationship between response times and the evaluated visual parameters and skills, provided a set of discriminant functions (or model), thus allowing for the categorisation of participants according to their skill to perceive SIRDS. Results: Two discriminant functions were obtained, which allowed for an overall predictive accuracy of 66.67 per cent (p = 0.024), with a higher predictive accuracy for groups 1 (minimum time less than 10 seconds, 78.26 per cent) and 2 (minimum time greater than 10 seconds, 75.86 per cent) than for group 3 (SIRDS not perceived, 35.29 per cent). Stereoacuity, negative relative convergence, phoria at near and, to a lesser extent, the accommodative convergence and accommodation ratio were found to be the most relevant discriminant variables, although between-group statistically significant differences were only disclosed for stereoacuity (p = 0.001) and negative relative convergence (p = 0.003). Conclusion: The ability to perceive SIRDS was related to many visual parameters and skills, including, but not limited to, stereoacuity and negative relative convergence. It is uncertain whether SIRDS might be considered a useful tool in clinical practice.
International Journal of Man-Machine Studies
Parvati Dev
Vision Research
Casper J Erkelens
The dynamic properties of the version and vergence system were studied in relation to stereopsis for movements of the whole visual scene. Large random-dot stereograms (30 × 30deg arc), moving laterally, were viewed dichoptically by human subjects without a fixed visual frame of reference. Sinusoidal movements in counterphase of the two half-images constituting the stereogram induced sinusoidal ocular vergence movements. The gain of vergence depended on the frequency as well as the amplitude of stimulus movement, while the phase lag depended only on the frequency. Fusion and stereopsis were retained up to a maximal velocity of change in relative position of the two half-images between 6 and 13.5 deg/sec. Sinusoidal movement of one half-image while the other one remained stationary induced sinusoidal ocular version as well as vergence movements. For version gains were higher and phase lags were smaller than for vergence. At the retinal level, residual overall binocular disparities between the two half-images up to 2 deg arc were tolerated without loss of stereopsis. The presence of sinusoidally varying overall binocular disparities and ocular vergence movements without perception of motion in depth suggests that these variables are not adequate cues for perception of (change in) depth.
Barbara Gillam
Paul B Hibbard
Frederick Kingdom
Alan Cobo-Lewis
Lawrence K. Cormack
Loading Preview
Sorry, preview is currently unavailable. You can download the paper by clicking the button above.
RELATED PAPERS
Stephanie Shorter
Casper J Erkelens , Elena Gheorghiu
Optometry and Vision Science
O-Ree Sanri Sidabutar
Perception & Psychophysics
Richard Herring
David Simmons
Bart Farell
Journal of the Optical Society of America A
Alfred Bruckstein
Alice O'Toole
Journal of Vision
Ichiro Fujita
Nature Neuroscience
Heinrich Bülthoff
Perception & psychophysics
Joachim Meyer , David Leiser
Susumu Tachi
Julian Fernandez
Suzanne McKee
Keith White
Hongjing Lu
Journal of Optical Technology
Gunta Krumina
JOSE LUIS VAZQUEZ JIMENEZ
Neuropsychologia
Anna Grabowska
Marco Bertamini
RELATED TOPICS
- We're Hiring!
- Help Center
- Find new research papers in:
- Health Sciences
- Earth Sciences
- Cognitive Science
- Mathematics
- Computer Science
- Academia ©2024
An experiment was performed to determine whether knowledge of the form of the embedded image affected the time required for subjects to fuse the images. One group of subjects (group NV) received either no information or just verbal information about the shape of the embedded object. A second group (group VV) received both verbal information and visual information (e.g., a drawing of the object).
The investigators performed the usual two-sample pooled-variance t-test, giving t(76) = 1.9395, p-value = 0.0562, and concluded that, at the 0.05 significance level, no difference between the groups was demonstrated. However, analysis of these data using the unequal-variance t-test reveals a marginally significant result (p-value=0.0453). Note that the test of equality of variances gives a highly significant result.
Boxplots of the raw data show that the time values are positively skewed, longer fusion time in the NV group is accompanied by greater variability, and there is one very large potential outlier in the NV group.
These defects are all cured by transforming the data to log(time).

An official website of the United States government
The .gov means it’s official. Federal government websites often end in .gov or .mil. Before sharing sensitive information, make sure you’re on a federal government site.
The site is secure. The https:// ensures that you are connecting to the official website and that any information you provide is encrypted and transmitted securely.
- Publications
- Account settings
Preview improvements coming to the PMC website in October 2024. Learn More or Try it out now .
- Advanced Search
- Journal List
- v.20(4); 2020 Apr
Calibration of random dot stereograms and correlograms free of monocular cues
János radó.
Institute of Physiology, Medical School, University of Pécs, Pécs, Hungary
Szentágothai Research Center, University of Pécs, Pécs, Hungary
Centre for Neuroscience, University of Pécs, Pécs, Hungary
Zoltán Sári
Department of Technical Informatics, Faculty of Engineering and Information Technology, University of Pécs, Pécs, Hungary
Péter Buzás
Gábor jandó.
Email: [email protected].
Address: Institute of Physiology, Medical School, University of Pécs, Pécs, Hungary.
Associated Data
Dynamic random dot stereograms (DRDSs) and correlograms (DRDCs) are cyclopean stimuli containing binocular depth cues that are ideally, invisible by one eye alone. Thus, they are important tools in assessing stereoscopic function in experimental or ophthalmological diagnostic settings. However, widely used filter-based three-dimensional display technologies often cannot guarantee complete separation of the images intended for the two eyes. Without proper calibration, this may result in unwanted monocular cues in DRDSs and DRDCs, which may bias scientific or diagnostic results. Here, we use a simple mathematical model describing the relationship of digital video values and average luminance and dot contrast in the two eyes. We present an optimization algorithm that provides the set of digital video values that achieve minimal crosstalk at user-defined average luminance and dot contrast for both eyes based on photometric characteristics of a given display. We demonstrated in a psychophysical experiment with color normal participants that this solution is optimal because monocular cues were not detectable at either the calculated or the experimentally measured optima. We also explored the error by which a range of luminance and contrast combinations can be implemented. Although we used a specific monitor and red-green glasses as an example, our method can be easily applied for other filter based three-dimensional systems. This approach is useful for designing psychophysical experiments using cyclopean stimuli for a specific display.
Introduction
Three dimensional display technology is widely used in vision research as well as in medicine, engineering and entertainment. In order to create the illusion of a three-dimensional visual scene on flat displays, dichoptic stimulation is needed, that is, the two eyes have to be presented with different but clearly separated images. There is a wide variety of technical solutions, each of them involving certain compromises against the natural viewing situation. The most perfect image separation can be provided if the images of two separate displays are fused with the help of an optical system. Mirror stereoscopes ( Wheatstone, 1838 ) and recently, virtual reality goggles use this technique. Fusion is still difficult for some viewers (children for instance) due to the unnatural viewing situation [discussed by Markó et al. (2009) ]. Techniques using shutters to present the two images in an alternating fashion are prone to flicker, as well as ghosting, the intrusion of the image intended for one eye into the other eye.
The most economical and thus, wide-spread solution to these problems is to present the two images simultaneously and to separate them based on their spectral composition using colored filters (anaglyphic technology) or taking advantage of light polarization and using polarizing filter goggles. Ideally, the filters would guarantee (1) complete separation of the lights intended for each eye (no crosstalk); (2) that the luminance of transmitted light is equal through both filters (equal light attenuation). Because of imperfection of physical filters though, the separation of eye channels is never complete. Furthermore, the luminance of light reaching the two eyes may be different due to differences between the light sources intended for each eye as well as due to unequal transmittance of the filters.
When viewing a conventional stereogram such as in 3D cinema, uncompensated filter crosstalk results in image ghosts where each eye also receives the shifted image intended for the other eye. A certain amount of crosstalk can be neglected in entertainment applications but in vision research, it can severely bias scientific results and conclusions.
Besides ghosting, stereopsis is also sensitive to interocular differences in luminance as well as contrast. A well-known example of the effect of interocular luminance difference is the Pulfrich-effect ( Pulfrich, 1922 ), which is due to luminance dependent differences in processing time in the visual pathways ( Froehlich & Kaufman, 1991 ; Kurita-Tashima, Tobimatsu, Nakayama-Hiromatsu, & Kato, 1992 ; Markó, Mikó-Baráth, Kiss, Török, & Jandó, 2012 ). The same effect can deteriorate the depth percept in stereo kinematograms. Interocular contrast differences on the other hand, rapidly deteriorate binocular fusion, whereas equalizing interocular contrast restores it. This phenomenon is often referred to as contrast paradox in stereopsis ( Halpern & Blake, 1988 ; Legge & Gu, 1989 ; Stevenson & Cormack, 2000 ). Also, it is often desirable in research applications that the average luminance and contrast of stereoscopic displays be precisely controlled. Finally, it is important to know the extent to which this complex set of requirements can be fulfilled by the specific displays and filters used.
Dynamic random dot stereograms (DRDSs) ( Julesz, 1960 ; Julesz & Miller, 1962 ) and correlograms (DRDCs) ( Julesz, Kropfl, & Petrig, 1980 ) are visual stimuli originally invented by Béla Julesz to selectively activate low-level disparity sensitive mechanisms of the visual system. A strict requirement for the utility of DRDSs is that the three-dimensional (3D) pattern be only visible by binocular viewing and functional stereoscopic vision whereas monocular viewing or dysfunctional stereopsis would result in a meaningless random dot pattern for either eye ( Figure 1 ). Monocular cues (also called monocular artefacts in the current context) on the other hand, can result in responses from monocular perceptual mechanisms essentially based on luminance contrast ( Figure 2 ). In such cases, the responses, whether behavioral or neurophysiological, could be confounded with responses of the stereoscopic mechanism. Although the problem is generally recognized by researchers ( Baker, Kaestner, & Gouws, 2016 ), yet the techniques of proper calibration are often not published. A notable exception is a study by Mulligan (1986) who presented a mathematical model of perceived luminances of the dots in DRDS displays.

Top, a single frame of the anaglyphic dynamic random dot correlogram stimulus with an embedded Snellen E optotype. The middle row shows the monocular views of the stimulus assuming optimal calibration of the anaglyphic colors and viewing through corresponding red-green glasses. The bottom part of the figure is a map of correlation state (correlated or anticorrelated) between the left and right images. Observers with proper stereoscopic vision perceive the correlated and anticorrelated regions different when viewing the stimulus at the top through red-green glasses.

Schematic representation of the monocular views of the anaglyphic DRDC frame shown in Figure 1 if the anaglyphic colors are “spoiled,” i.e., deviate from optimal calibration. Images at the top of each panel show the same stimulus frame. Panel A illustrates that in this case, the Snellen-E target becomes visible if the stimulus is viewed monocularly through the left (red) filter of a red-green goggle. Note that the exact appearance of the background and target regions can be different depending on which of the colors deviate from the optimum and in which direction. Panel B shows the same for viewing through the right (green) filter.
In this article, we present a solution for minimizing monocular artefacts in random dot stereograms and correlograms presented on computer displays by proper compensation of crosstalk while ensuring equal mean luminance and contrast in the two eyes. Using nonlinear optimization, the algorithm finds the intensity weighting of the light sources for each eye channel needed to approximate an expected contrast and mean luminance by minimal error. We also show how the range of displayable contrast and mean luminance combinations on a particular display device can be calculated. Finally, we demonstrate using psychophysical tests, the lack of monocular cues in DRDC test stimuli using the calibrated color settings. Although we present the problem and its solution on the example of red-green anaglyphs, our solution is applicable for any filter-based dichoptic technique including polarizing filters. An earlier version of the numerical solution presented here was briefly described in the appendix in Markó et al. (2009) . An implementation of the numerical solution is provided as Matlab source code along with example data on GitHub ( https://github.com/JanosRado/StereoMonitorCalibration ).
Measurement of monitor and filter properties
From the user's side, the intensity of pixels of a computer display are controlled using digital video values. Our aim is to find digital video values that fulfil certain requirements defined in terms of luminance and luminance contrast. The relationship of digital video values and luminance (termed here as luminance characteristics) must therefore be measured in advance. We recorded the luminance characteristics using an ILT1700 photometer (International Light Technologies, Peabody, MA) equipped with a SED033 detector, Y2 filter and R input optic. An increasing arithmetic progression of digital video values was presented in steps of no more than five units. The presentation of the levels was separated by 7 seconds of mid-gray (RGB = [127, 127, 127]). Each level was presented for 7 seconds and measurement was done during the last second. Each level was measured once. Digitized output of the photometer was read through a USB serial line at three digits' precision.
We needed four different luminance characteristics arising from the combination of two monitor phosphors ( r , g ) with the two color filters ( ρ , γ ). The functions will be referred to as p r ρ (Red Attenuation), p r γ (Red Crosstalk), p g γ (Green Attenuation), and p g ρ (Green Crosstalk).
Luminance values at a specific digital video value can be queried from lookup tables made from the measured characteristics. In order to facilitate nonlinear optimization however, it was necessary to describe this conversion by a continuous monotonic, differentiable function. It is customary to approximate the luminance characteristics of monitors by a power function (the “gamma function”) of the form L = ax γ + b , where L is luminance, x is the digital video value of any of the RGB phosphors and a , b and γ are free parameters. Here, we chose to fit a third-order polynomial L = ax 3 + bx 2 + cx + d instead (with free parameters a , b , c , d ) to approximate the measured lookup tables. Model parameters were estimated using the fit function from the Curve Fitting Toolbox in Matlab (The MathWorks, Natick, MA). In preliminary calculations for various computer monitors, both models resulted in similarly good fits ( Figure 3 ) but an analytical solution (see Results) was only practicable when using the third-order polynomial approximation.

Luminance characteristics of the red and green monitor phosphors of the LG Cinema 3D D2343P (LG Display, South Korea) monitor used in the present study. Data points are measured values; red or green curves are best fit third order polynomials. The best fit gamma functions are plotted in gray but they are mostly covered by the polynomial fits. R 2 values were greater than 0.985 for all fitted curves shown.
Psychophysical testing of the calibration procedure
Altogether 16 participants completed the psychophysical test (seven males, nine females). All participants had a best corrected visual acuity of at least 1.0. All procedures performed in this study involving human participants were in accordance with the ethical standards of the institutional and/or national research committee and with the 1964 Helsinki declaration and its later amendments. This study was approved by the Regional and Local Ethics Committee at the University of Pécs (registration number: 5638). Written informed consent was obtained from all participants.
Dynamic random dot correlogram
We used dynamic random-dot correlograms ( Julesz et al., 1980 ) viewed through red-green color goggles. The DRDC stimulus was constructed of two types of regions ( Figure 1 ). Both regions were random dot patterns made of 50% dark and 50% bright, square-shaped dots, each subtending 9.8′ from the viewing distance of 1 m. Random dot images were updated 60 times per second synchronized to the monitor refresh cycle.
In a correlated region, the contrast polarity of each dot was identical for both eyes (correlation of dot luminances equaled +1, Figure 1 ). Since we used red-green anaglyphic presentation, this was ensured by using dots of black and yellow logical colors. In an anticorrelated region, dots had opposite contrast polarity in the two eyes (correlation of dot luminances equaled −1), which was ensured by using the red and green logical colors.
In the actual stimuli, Snellen E optotypes were shown as an anticorrelated region on a correlated background. The Snellen E targets had a size of 11.5° × 11.5° visual angle and the entire stimulus area had a size of 16° × 16°.
Test stimulus set
Stimuli were presented on a LG Cinema 3D D2343P monitor with a refresh rate of 60 Hz. The screen had a resolution of 1920 × 1080 and subtended 27 × 16 degrees of visual angle. The stimuli were generated using Psychophysics Toolbox version 3 extensions ( Brainard, 1997 ; Kleiner, Brainard, & Pelli, 2007 ; Pelli, 1997 ) written in Matlab. Participants wore red-green color goggles equipped with R26 low-pass (red) and YG09 band-pass (green) gelatin filters (Tobiás Optic, Ltd., Budapest, Hungary) for the left and right eyes Markó et al. (2009) . Transmittance spectra of the filters are shown in Supplementary Figure S1 .
The respective RGB values of the four colors of the anaglyphic stimulus ( Table 1 ) were first determined using the numerical optimization procedure described in the present paper. The required space-averaged luminance was 6 cd/m 2 and the required Michelson contrast of dark and bright dots was 50%. This parameter combination was within the region where the calculated luminance and contrast errors for the specific monitor were negligible ( Table 1 , Figure 6 ).
Optimal values of the anaglyph colors predicted by our numerical optimization procedure for the luminance and dot contrast levels used in the psychophysical tests (required luminance 6 cd/m 2 , required Michelson contrast 50%).
Digital video values | ||||||||||
---|---|---|---|---|---|---|---|---|---|---|
for the phosphors | Luminance (cd/m ) | Contrast | ||||||||
Logical | Left | Right | Left mean | Right | Log luminance | Left | Right | Log contrast | ||
colors | ( ) | ( ) | ( ) | mean ( ) | error E | ( ) | ( ) | error E | ||
Red | 220 | 126 | 8.95 | 2.98 | ||||||
5.9868 | 5.9932 | –2.43 | 0.4954 | 0.5029 | –1.88 | |||||
Green | 130 | 203 | 3.02 | 9.01 | ||||||
Yellow | 216 | 202 | 8.98 | 8.97 | ||||||
5.9860 | 5.9942 | 0.5001 | 0.4970 | |||||||
Black | 138 | 128 | 2.99 | 3.02 |
Note that mean luminances and contrasts are very similar between the eyes as well as between the logical colors. For symbols see Figures 4 and 5 and Equations 11 and 12 .

Contour plots of fractional luminance (A, C, E) and contrast (B, D, F) errors over the range of values achievable on the hardware configuration tested. The three rows show results obtained using the symbolic (A, B) and numeric solutions (C, D) and the channel constancy model (E, F), respectively, each result rounded to the nearest 8-bit integer using the procedure described in the text. Error values calculated according to Equation 11 for luminance and Equation 12 for contrast are represented by color on a logarithmic scale shown on the right. Hotter colors indicate higher fractional deviation from the required luminance or contrast. The white regions correspond to luminance-contrast pairs where a symbolic solution was not possible (A, B, E, F). White broken contour line marks the smallest common area of the 3 valid regions. Error values in Table 2 were calculated from this area.
Each block of stimuli consisted of DRDCs with 41 different combinations of black, yellow, red and green anaglyph colors. One of them corresponded to the optimized RGB values from the model. The other 40 stimuli were intentionally “spoiled” versions of the optimal color combination. These were obtained by increasing or decreasing either the red or the green phosphor luminances in five steps. The n th increment had a luminance 1.04 n times the optimized value while the n th decrement had a luminance 0.96 n times the optimized value. One such series was generated for each of the four anaglyph colors resulting in 40 different stimuli.
The aim of the psychophysical procedure was to assess the detection probability of monocular artefacts. The stimuli were presented in a 4-alternative forced choice procedure with a method of constant stimuli. Specifically, participants had to decide the orientation of the Snellen E (left, right, up, down) and indicate the response by pressing the corresponding arrows on the keyboard. There was no time limit for the presentation and response times and the subsequent stimulus appeared after the response had been made. Testing was carried out in a darkened room.
The 41 stimuli were randomly shuffled in each block and 10 blocks were presented in one session, which lasted about 40 minutes. One of the eyes was covered with an eye patch in each session. Left and right eyes were tested in separate sessions to limit the effect of fatigue. In the session where stimuli were viewed through the left (red) filter, only the red RGB component was varied. In the session, where they viewed through the right (green) filter, only the green component was varied. Thus, a total of 820 responses were obtained from each participant. Nine participants (five males and four females) started with the right eye and the others started with the left eye. We did not systematically investigate the effect of varying the RGB component for which the respective filter was “closed” because a 4% luminance increment or decrement was not possible within the gamut of the monitor.
Fitting of inverted gaussian to the response curve
In order to estimate the empirical optimum of a color value from the psychophysical data, percent correct responses ( y ) were first inverted then fitted by a Gaussian curve (with an additional y-shift) of the following form using the fit function in Matlab:
where x is the R or G value that was varied, µ is the center, σ is the spread. Parameters a and b were also varied during the fit. The center ( µ ) was taken as the empirical optimum digital video value.
Problem statement
The left-hand side of Figure 5 illustrates the problem faced when presenting random-dot stereograms or correlograms using the anaglyphic method. What the observer needs to see is patterns of dark and bright dots in both eyes whereby four combinations of dark and bright dots can occur: dark in both eyes, bright in both eyes, dark left and bright right and vice versa ( Figure 1 ). We term the first two cases correlated dots because the contrast polarity of the dot is equal in both eyes. Dots seen in opposite contrast in the two eyes are termed anticorrelated. Figure 2 shows that unwanted monocular cues arise in RDCs when luminance and or contrast of correlated and anticorrelated regions differ. Therefore, we require that the mean luminance ( L 0 ) and the contrast ( C 0 ) of these types of regions be equal in both eyes (right-hand side of Figure 5 ).

Schematic demonstration of the problem and its solution. Our task is to find the red (r) and green (g) digital video values of a computer monitor that define the four logical colors fulfilling the following criteria. When the four colors are viewed through the red and green filters with the two eyes respectively, they must appear brighter or darker than a predefined average luminance (L 0 ) as shown in Figure 4 . Furthermore, the luminance increments and decrements must be equal so that their contrast (C 0 ) is always equal between the two eyes.
This sort of dichoptic stimulus can be composed by using the red and green primary lights (phosphors) of a computer monitor ( r , g , respectively) in combination with red and green colored filters ( ρ , γ , respectively). The light emitted by the red phosphor mainly can pass through the red filter and light emitted by the green phosphor mainly can pass through the green filter. When viewed without the filter goggles, the display will thus consist of dots with one of four colors, which we term here as logical colors red, green, black and yellow (denoted R, G, B, Y, respectively, Figure 4 45). 5 ). However, if the logical colors would naively, be composed purely of the red and green primary lights driven at full intensity, their mean luminances and contrasts would not be equal as required. This is because of (1) unequal luminance of the phosphors, (2) unequal light attenuation and (3) crosstalk between the filters. These problems can be compensated by adjusting the red and green phosphor components of the logical colors but the effects of these adjustments on the mean luminance and contrast of the display must be regarded together.

Appearance of the four so-called logical colors (R, Red; G, Green; B, Black; Y, Yellow) comprising a random-dot stereogram or correlogram through the color filters (red (ρ) and green (γ)).
In mathematical terms, the problem to be solved is essentially to minimize the difference between the actual mean luminance and contrast and their required values ( L 0 , C 0 , respectively) for all regions of the display.
Obtaining the luminance values
Here, we begin with calculating the actual mean luminances and contrasts. Let us denote the digital video values defining the intensities of the red ( r ) and green ( g ) phosphors resulting in the four logical colors: red (R), green (G), black (B), and yellow (Y) as follows:
- r R : digital video value of red component of logical red color
- g R : digital video value of green component of logical red color
- r G : digital video value of red component of logical green color
- g G : digital video value of green component of logical green color
- r B : digital video value of red component of logical black color
- g B : digital video value of green component of logical black color
- r Y : digital video value of red component of logical yellow color
- g Y : digital video value of green component of logical yellow color.
Since our requirements are stated in terms of luminance, the conversion between digital video values and luminance of the output device needs to be known. We acquire these luminance characteristics by measurement (see Methods) but in order to facilitate optimization, we approximate them by third-order polynomials ( Figure 3 ). There are four different luminance characteristics referred to as p r ρ (Red Attenuation), p r γ (Red Crosstalk), p g γ (Green Attenuation), and p g ρ (Green Crosstalk). The whole set of luminance values calculated from the measured luminance characteristics is defined as follows
Calculation of average luminance and contrast
First, we calculate the luminance of each logical color as they are seen through each of the color filters. The luminance of the red logical color through the red filter ( L R ρ ) for example is the sum of luminances passed from the red and green phosphors, where the second term accounts for the crosstalk:
Similarly, for the other combinations of logical colors and color filters, we write
The anticorrelated regions of a random dot correlogram are composed of pixels of red and green logical colors and the correlated regions consist of the yellow and black logical colors ( Figure 1 ). In the following, the subscripts RG and YB stand for these regions of the stimulus, respectively. Their average luminances can be calculated from Equations 2 and 3 as
where L ¯ R G ρ and L ¯ R G γ are the average luminances of the anticorrelated region seen through the red and green filters, respectively. Similarly, L ¯ Y B ρ and L ¯ Y B γ are the respective average luminances of the correlated region.
We use Michelson-contrast to quantify the contrast ( C ) of dark and bright dots in each of the regions of the stimulus:
where the subscripts have the same meaning as in Equation 4 .
The goal of the calibration
The goal of calibration is to calculate digital video values corresponding to logical colors for which the average luminance and contrast are equal to pre-defined values. The deviation from these values can be interpreted as an error. The purpose of the various solution approaches is to make this error minimal or in ideal case, zero. Since the error can be calculated separately for the correlated and anti-correlated sets of pixels, the solution of the problem can be also separated into two independent parts corresponding to these regions.
Symbolic solution
Ideally, we require that all average luminances ( Equation 4 ) be equal to the required mean luminance ( L 0 ) and all contrasts ( Equation 5 ) be equal to the required contrast ( C 0 ). For the anticorrelated set of pixels, we can therefore write the following system of equations:
Since we approximated the monitor luminance characteristics by third-order polynomials ( Equation 1 ), the general solution of Equation 6 is rather complicated; yet it can be calculated by a computer algebra system. In this case, the solver finds the algebraic expressions that provide the digital values r R , g R , g G , r G when L 0 , C 0 and the coefficients of the third-order polynomials are known. We solved the equation system consisting of Equations 1 – 6 using Mathematica (Wolfram Research, Champaign, IL). Substituting the known parameters into this expression results in the digital video values satisfying Equation 6 with theoretically, zero error. The precision of the result will nevertheless, depend on two factors; (1) how exactly the third-order polynomials approximate the luminance characteristics and (2) rounding of the real-valued parameters to the integer digital video values (see below).
The same procedure can be carried out for the correlated set of pixels, which should satisfy
Numerical solution
In case of a numerical solution, we seek to minimize an error function E RG for the anticorrelated pixels and an error function E YB for the correlated pixels, which can be written as the Euclidean norms of deviations of average luminance and contrast from their required values:
where the terms e L and e C are fractional luminance and contrast errors for the anticorrelated and correlated pixels as seen through each filter, respectively as follows e L R G ρ = L 0 - L ¯ R G ρ L 0 e Y B ρ = L 0 - L ¯ Y B ρ L 0
When minimizing E RG , the free parameters of the optimization are the digital video values [ r R , g R , g G , r G ]. Similarly, the free parameters [ r B , g B , r Y , g Y ] are optimized when minimizing E YB .
We used the fmincon function of the Optimization Toolbox of Matlab to minimize the error functions. Optional parameters of fmincon were left at their default values (interior-point algorithm; maximum number of iterations 1000; termination tolerances on the function value and on the free parameters were 10 −6 and 10 −10 , respectively). All free parameters (i.e., digital video values) were constrained to the interval [0; 255]. Their starting values were chosen by assuming no crosstalk between the filters. The required digital video values can in this case, easily be obtained from the luminance characteristics for filter attenuation. An implementation of the numerical solution is provided as Matlab source code along with example data on GitHub ( https://github.com/JanosRado/StereoMonitorCalibration ).
Symbolic solution based on the assumption of channel constancy
There is an alternative approach to the problem suggested by Mulligan (1986) , which simplifies the problem in two ways. First, instead of the digital video values, the model predicts the phosphor luminances. Here, we denote the luminances of the red ( r ) and green ( g ) components of the four logical colors ( R , G , Y , B ) by { L Rr , L Rg , L G r , L Gg , L Yr , L Yg , L Br , L Bg }. These can be calculated from the following equation system
The first five equations correspond to Equations 1 – 5 of Mulligan (1986) , where the so-called selectivity indexes S ρ , S γ , S r and S g are defined below. To provide the necessary degrees of freedom however, 3 additional equations have to specify the required mean luminance L 0 , the required luminance contrast C 0 and the equality of luminances in the left and right eyes, respectively.
The second simplification is the assumption of channel constancy meaning that the spectral composition of the primary lights do not change with intensity ( Brainard, Pelli, & Robson, 2002 ). For this reason, we call this approach the “channel constancy model”. The assumption of channel constancy is incorporated in the selectivity indexes. S ρ = T ρ g T ρ r and S γ = T γ r T γ g are the selectivity indexes for filters, S r = T γ r T ρ r and S g = T ρ g T γ g are the selectivity indexes for the phosphors. The T parameters can be interpreted as transmittances; for example, T ρ g represents L ρ g / L g , the fraction of light from the green phosphor transmitted by the red filter. However, if channel constancy of a monitor fails as it is sometimes the case ( Supplementary Figure S2 ), the measured filter selectivity indexes will depend on the intensity of the phosphors instead of being constants. Therefore, to evaluate the channel constancy model for our display device, we used the median values of the T transmittances measured for the entire range of digital video values.
We solved equation system 10 using Mathematica for the luminance values in symbolic form, from which the numeric values of the luminances { L Rr , L Rg , L G r , L Gg , L Yr , L Yg , L Br , L Bg } can be calculated for a given set of T parameters, desired luminance and contrast. Finally, the actual, device specific digital video values of the logical colors can be looked up from the measured luminance characteristics of the r and g phosphors; for example, g R will be the x-value on the luminance curve for the green phosphor at the luminance L Rg , and so on. Note that for this procedure, the luminance curves have to be measured without filters because the filter transmittances have already accounted for by the T parameters.
Minimizing the effect of rounding
Each of the above methods delivers real-valued video values, which must be rounded for usage in a digital computer. Because the solution is an eight-element set and each of them can be rounded up or down, there are 2 8 rounding patterns, each of them having different effect on the overall error. In order to minimize the effect of rounding, we adaptively chose the rounding pattern from these 256 cases that resulted in the smallest overall error calculated as ||(E RG ,E YB )|| from Equations 8 and 9 . The benefit of this method of rounding is most significant when low luminance and contrast are required at the same time (data not shown).
Domain of achievable luminance and contrast values
To investigate the domain of achievable luminance and contrast values for a real display device and pair of color filters, we carried out a parameter sweep of the desired luminance and contrast values for a specific computer monitor and a pair of red-green color goggles (see Methods). We chose the set of desired luminances and contrasts tested to cover the achievable range in 100 equidistant steps. As a goodness measure, we calculated the Euclidean norm of fractional errors in luminance (E L ) and contrast (E C ) separately as follows (using notation introduced above):
Figure 6 shows color coded plots of luminance and contrast errors over the parameter space calculated by each of the three solutions described above. Mean and standard deviation of the plotted values (log transformed) are shown in Table 2 . The achievable region of luminance-contrast combinations is limited by the minimum and maximum luminances that the display device can produce. Therefore the range of achievable mean luminances becomes narrower for increasing contrasts. Another factor limiting the achievable contrast is filter crosstalk, which results in certain amount of light being added to the luminance of a dark pixel if the same pixel needs to be bright in the other eye (anti-correlated case). If the two filters have different spectral selectivities, the one with higher crosstalk will limit the maximum achievable contrast. This also means that if very high contrasts are requested, the numerical solution will converge to the maximum achievable contrast at the expense of increasing error (reddish regions at high contrasts in Figures 6 C and D). For the symbolic method and the channel constancy model, these constraints mean that there is no valid solution for certain high luminances or contrasts (white regions in Figs. 6 A, B, E, F).
Contrast and luminance errors of the three solutions analyzed in the article.
log Luminance error E | log Contrast error E | |||
---|---|---|---|---|
Mean | SD | Mean | SD | |
Symbolic solution rounded to 8 bit integer | −2.0556 | 0.2191 | −1.8669 | 0.4593 |
Numeric solution rounded to 8 bit integer | −2.0556 | 0.2191 | −1.8669 | 0.4593 |
Channel constancy model, rounded to 8 bit integer | −1.3589 | 0.2195 | −1.5140 | 0.2862 |
Symbolic solution rounded to 12 bit integer | −3.2624 | 0.2171 | −3.0675 | 0.4649 |
The table shows means and standard deviations of the log errors calculated for the entire range of values achievable on the hardware configuration tested. Only the range of valid symbolic solutions was taken into account for all methods ( Figure 6 ).
Within the valid range of digital video values, rounding to integer numbers is a common source of error for all three solutions. If required, this sort of quantization error may be reduced by using a display with higher intensity resolution or employing one of the workaround techniques for increasing luminance resolution of a computer monitor (e.g., bit-stealing Tyler, Chan, Liu, McBride, and Kontsevich (1992) ; see Brainard et al. (2002) for an extended list). Assuming a monitor with the same characteristics as the one tested here but 12 bit intensity resolution per channel (an improvement of 4 bits), the error of the solutions would decrease about 2 4 = 16-fold (1.2 log units, Table 2 ).
Apart from rounding errors, the symbolic solution provides the highest possible precision and accuracy. Compared to this benchmark, the numeric optimization gave identical results to at least a precision of 4 digits ( Table 2 ). The most important merit of the numeric solution is, however, its accessibility given that Matlab or Scipy is more widespread in scientific communities than software tools of symbolic mathematics. The channel constancy model provided on average, 3.50% higher error relative to the required luminance and 1.70% higher error relative to the required contrast ( Table 2 ) compared with the symbolic or numeric methods, although for an added benefit of mathematical simplicity. It is important to note that even if the required luminance or contrast cannot be achieved exactly, their actual values can always be obtained from Equations 4 and 5 .
Higher deviation from the required luminance or contrast does not necessarily mean that the cyclopean random-dot stimulus is flawed in the sense that features that should only be detected binocularly would be visible monocularly. As shown in Figure 2 , the presence of monocular cues can be tested using a stimulus consisting of binocularly correlated and anticorrelated regions. If for example, the mean luminances of the correlated (“yellow-black”) and anticorrelated (“red-green”) regions are different in either eye ( L ¯ R G ρ ≠ L ¯ Y B ρ and/or L ¯ R G γ ≠ L ¯ Y B γ ), the two regions could appear different during monocular viewing, thus the pattern could be detected. This source of error can be expressed by the luminance contrast between the two regions. Similarly, if the contrast of dark and bright dots is different within the correlated and anticorrelated regions, they could appear different in one or both eyes ( C RG ρ ≠ C YB ρ and/or C RG γ ≠ C YB γ ) causing monocular artefacts. The strengths of these sources of monocular cues can be summarized in the following metric:
The value of M may be interpreted as contrast between the correlated and anticorrelated regions of the test image, and thus, it is expected to correlate with the likelihood of detectable monocular cues. The calibrated anaglyph colors obtained using the symbolic solution and the numerical approximation both resulted in M = 1.04% ± 0.59% as compared with M = 5.91% ± 0.77% for the channel constancy model ( Figure 7 , Table 3 ).

Estimated strength of monocular cues (M) for a random dot correlogram consisting of correlated and anticorrelated regions such as the one shown in Figure 2 . The symbolic (A) and numerical (B) solutions and the channel constancy model (C) are compared. Hotter colors indicate higher chance of the cyclopean target to be detected monocularly; white regions correspond to non-achievable luminance-contrast pairs. Values are calculated according to Equation 13 for the monitor and filter goggles used for testing (see text).
Mean and standard deviation of the estimated strength of monocular cues across the achievable range of luminances and contrasts shown in Figure 7 .
Estimated strength of monocular cues ( ) | ||
---|---|---|
Mean | SD | |
Symbolic solution rounded to 8 bit integer | 0.0104 | 0.0059 |
Numeric solution rounded to 8 bit integer | 0.0104 | 0.0059 |
Channel constancy model, rounded to 8 bit integer | 0.0591 | 0.0077 |
Symbolic solution rounded to 12 bit integer | 0.00062 | 0.0004 |
Psychophysical test of the stimulus calibration
The Snellen-E optotype embedded in a well-calibrated dynamic random dot correlogram should be binocularly visible because anticorrelated and correlated regions of the DRDC are perceived differently by stereoscopic vision ( Julesz et al., 1980 ). The percept of a correlated background is a noisy surface in the plane of the monitor while in the anti-correlated region of the Snellen-E, an indefinite (“woolly” according to Julesz et al. (1980) ) depth can be perceived. In an optimally calibrated stimulus, the orientation of the target stimulus could be identified only if the observer has intact binocular vision and the image is viewed binocularly. Upon closing either eye, the Snellen-E disappears ( Figure 1 ) and the person cannot detect its orientation.
The aim of our psychophysical test was to identify the minimum of monocular visibility across a range of RGB colors that included the predicted optimum, as well as 40 “spoiled” versions. We expected that for intentionally “spoiled” stimuli, the target stimulus would become monocularly visible to an increasing degree depending on the deviation to any direction from the predicted optimum. The reason for this is that either the contrast between bright and dark dots or the average luminance within the correlated and anticorrelated areas become unequal causing the background and target regions appear visually different ( Figure 2 ). Thus, if our prediction was correct, monocular detectability would show a minimum for the predicted set of RGB values within a reasonable error.
Figure 8 shows percentage of correct responses in a 4-alternative forced choice task where participants had to detect the orientation of a Snellen-E target consisting of anticorrelated dots on a background of correlated dots ( Figures 1 , 2 ). Each color condition was presented 10 times.

Percent correct responses in a psychophysical task measuring the visibility of monocular artefacts in a DRDC. The abscissa shows the digital video values (at 8 bit resolution) with the vertical blue line indicating the values predicted by our model. Each row represents one of the four anaglyphic colors. The left and right columns show results for the two sessions performed with participants viewing through the red (left) and green (right) filters, respectively. We varied only the red component of each color for the red filter and only the green component of each color for the green filter. Gray traces show individual responses of the participants; the red trace is the group average. Horizontal gray line at 60% correct responses indicates the limit of chance level performance for individual participants. Horizontal red line at 32% correct responses indicates the limit of chance level performance when responses of all participants are accumulated. Asterisks show stimulus conditions where the accumulated number of correct responses exceeded the limit of chance level performance.
If the target was invisible by one eye, then an ideal observer would respond by guessing. The probability of giving c or more correct responses out of r trials by chance is given by
where n is the number of alternative choices (i.e., n = 4 orientations). This is the distribution function of a binomial random variable (p. 145 in Ross, 2014 ) with parameter (n − 1)/n. Further explanation of the formula can be found in a previous paper ( Budai et al., 2018 ). We chose to accept a participant's performance as significantly different from chance level if p was less than 0.05, which was given at c ≥ 6 correct responses ( p = 0.01973). This limit is shown by the gray horizontal line in Figure 8 . Clearly, none of the 16 participants could perform above chance if the anaglyph colors were at the predicted optimum but the monocular cues became increasingly visible as either the red or the green component of the anaglyph colors were shifted away from that point ( Figures 8 , 9 ). If the responses of all 16 participants are accumulated, the limit of chance level performance drops to 51/160 ≈ 32% correct responses (red horizontal line in Figure 8 ). As shown by the asterisks, a few RGB units of deviation from the predicted optimum increases the detection of monocular cues above chance level.

Number of participants where detection of monocular artefacts exceeded the chance level of 60%. The abscissa and the arrangement of panels correspond to those of Figure 8 . For the predicted red and green digital values (vertical blue line), artefact visibility did not exceed chance level for any of the participants.
Figures 8 shows percentage of correct responses in a 4-alternative forced choice task where participants had to detect the orientation of a Snellen-E target consisting of anticorrelated dots on a background of correlated dots ( Figures 1 , ,2). 2 ). Each color condition was presented 10 times.
It is also apparent from Figure 8 that individual participants may have required different color settings for optimal elimination of monocular artefacts. In order to characterize an individual's deviation from the optimum, we determined the minimum of artefact visibility by fitting inverted Gaussian functions to the individual response curves (see Methods). The center of the fitted Gaussian ( µ ) of a participant was taken as the optimum R or G value for the respective anaglyph color. Figure 10 shows that the individual empirical optima (blue tick marks) were indeed often different from the prediction by several RGB units. The medians of the optima were nevertheless within 1.25 RGB units for all anaglyphic color and filter combinations ( Figure 11 ).

. Determining the empirical optimum of R and G values for each of the anaglyphic colors. The coordinate systems and the arrangement of panels correspond to those of Figure 8 . Diamonds indicate the average of percent correct responses also seen in Figure 8 . Black lines show inverted Gaussian curves fitted to these average values and their minima are indicated by the red tick marks. Similar Gaussian curves were fitted to the responses of each participant and their minima are indicated by the blue tick marks. Vertical blue line shows the value predicted by our model.

Boxplots of the difference of empirical optimum R and G digital video values from the predicted optima (shown by the vertical red line at zero). Boxes are bounded by the first and third quartiles, red lines in the boxes show the medians and whiskers show the lowest and highest data points within 1.5 times the interquartile range to the median. The largest deviation of the median empirical optimum from the prediction was −1.250 RGB units (upper right data set). Outliers (7 data points altogether) are not shown but they can be found in Figure 10 . Arrangement of the data sets is the same as in Figure 8 .
Dynamic random dot stereograms and correlograms are indispensable tools in assessing stereoscopic function in experimental or diagnostic settings. Their utility strongly relies on proper adjustment of the luminance and contrast of the comprising dots in the face of technical limitations of a given display device. Here, we present a numeric approximation method that provides the set of digital video values that achieve a given average luminance and dot contrast with minimal error on a given display device. We demonstrate that this solution is optimal because it is indistinguishable from the analytic solution. The analytic solution is possible but less practical. We also compare the numeric approximation with an alternative analytic solution ( Mulligan, 1986 ), which incorporates the constraint of channel constancy Brainard et al. (2002) .
Using a specific monitor and red-green glasses as an example, we explored the error by which a range of luminance and contrast combinations can be implemented. This approach is useful for designing psychophysical experiments using DRDS or DRDC stimuli for a specific hardware.
Finally, we determined by a psychophysical method, the digital video values that result in no visible monocular artefacts and found these to be within 1.5 RGB units (at an intensity resolution of 8 bit per channel) of the optimum values obtained by the numerical solution.
Comparison to previous approaches
The problems of presenting 3D content on computer displays have been studied extensively mainly due to the demands of consumer electronics. Undoubtedly, the most disturbing type of artefact that deteriorates stereoscopic viewing is the appearance of ghosts of the other image due to crosstalk between the eye channels ( Woods, 2012 ). When viewing real-world scenes, ghosting may cause misjudgment of depth ( Tsirlin, Wilcox, & Allison, 2011 , 2012 ), general deterioration of image quality ( Wilcox & Stewart, 2003 ) or eye strain ( Seuntiëns, Meesters, & Ijsselsteijn, 2005 ). Image ghosts are in our terminology, monocular artefacts because they are visible by viewing the display (through the appropriate filters) by one eye.
Methods of crosstalk cancellation have been presented for minimizing image ghosts in 3D displays using passive filter goggles ( Woods, 2012 ) or shutter glasses ( Konrad, Lacotte, & Dubois, 2000 ). The validity of these models is usually tested by subjective rating of the corrected stimuli ( Chang, Kim, Choi, & Yu, 2008 ). Alternatively, a mathematical model of crosstalk magnitude is provided and perceptual optimum is assumed when this quantity is minimized ( Sanftmann & Weiskopf, 2011 ). Our approach is similar insofar as we also minimize a calculated term, namely the error of required mean luminance and contrast but we also performed a rigorous psychophysical test of the results.
The demands of psychophysical studies where cyclopean mechanisms need to be isolated are more specific. Crosstalk does not cause ghosts in DRDSs or DRDCs but it does result in the appearance of monocular cues, which may be confounded with cyclopean perception. This problem is generally recognized by researchers ( Baker et al., 2016 ), yet the techniques of proper calibration are often not published. A notable exception is a study by Mulligan (1986) who presented a mathematical model of perceived luminances of the dots in DRDS displays. The main difference of this model from ours is the assumption of channel constancy ( Brainard et al., 2002 ). We extended the system of equations suggested by Mulligan (1986) with the requirements of a given average luminance and dot contrast in both eyes and calculated solutions for a specific display device and pair of filters. This method resulted in higher errors than our numeric optimization procedure probably because the monitor we used for testing failed to conform to channel constancy ( Supplementary Figure S2 ).
Monocular cues are especially vivid when a pattern containing both correlated and anti-correlated regions is used as test stimulus but they disappear when optimal crosstalk cancellation is achieved. We took advantage of this property of DRDCs to design a sensitive and quantitative psychophysical test of crosstalk cancellation. In addition, this test is also sensitive to variation of contrast between regions of the stimulus, which again, result in monocular cues. Testing for monocular artefacts in DRDC patterns is thus a useful tool in evaluating calibration of 3D displays for demanding psychophysical applications.
Unwanted differences in mean luminance or contrast between the left and right images can only be detected by comparing the two images. Interocular luminance differences result in unequal latencies of evoked neural responses ( Froehlich & Kaufman, 1991 ; Kurita-Tashima et al., 1992 ) and a similar effect caused by interocular contrast differences of matching features has been described recently ( Reynaud & Hess, 2017 ). A well-known perceptual consequence is the Pulfrich effect, the illusory appearance of a pendulum moving in depth when observed through a pair of glasses with unequal optical densities ( Pulfrich, 1922 ). The classical Pulfrich effect (i.e. change in perceived depth) cannot occur in DRDS or DRDC. The reason is that the matching features in a pair of DRDS or DRDC only exist for the duration of a single frame and they change randomly over time. Therefore, the apparent time-shift between the frame sequences of the two eyes that result from unequal luminance causes decorrelation of the left and right eye images and the breakdown of the 3D percept instead of the appearance of illusory depth. Theoretically, the loss of the stereo percept could be used to test for interocular luminance or contrast errors in calibration. In practice however, the interocular luminance differences must be 60% or more for such an effect to occur ( Boydstun, Rogers, Tripp, & Patterson, 2009 ; Diehl, 1991 ) and therefore a calibration error that is worth a few RGB units is unlikely to be detected.
Potential extensions of the method
An important point to note is that the space averaged mean luminance of a stimulus display composed of the calibrated logical colors will optimally correspond to the required mean luminance ( L 0 ) only if the ratio of bright and dark dots is 1:1. This assumption is built in Equation 4 . If the ratio of bright dots in the entire display is n , the final space averaged luminance can be calculated post hoc as L ¯ = n L b r i g h t + ( 1 - n ) L d a r k , where L bright = L 0 (1 + C 0 ) and L dark = L 0 (1 − C 0 ).
Sometimes, stereoscopic displays are composed of bright features on a dark background. Because the total area of the bright features is typically less than 50% in such displays, the above consideration has to be taken into account. Furthermore, the dark background must be brighter than the nominal black produced by the RGB setting of (0; 0; 0). This is because to make crosstalk invisible, the luminance of the dark background pixels has to be increased to the level of the crosstalk caused by bright pixels in the other eye. This issue is discussed in section “Domain and resolution of achievable luminance and contrast values” above.
Another limitation of the present method is that it can only calculate the logical colors if the images presented to each eye are composed of two (dark or bright) states. In an anaglyphic image, this requires the use of 2 2 = 4 logical colors. Where more than two states of pixels are needed, the algorithm must be run multiple times for different combinations of mean luminance and contrast. For instance, if pixels can have three states (e.g., dark, background and bright) and they can be varied independently in the two eyes, we would require 3 2 logical colors. To generate the nine logical colors, the algorithm must be run three times, each time for a combination of two different states. In the 12 resulting logical colors, those where the left and right images are in identical state (e.g., dark in both eyes) will be obtained twice.
In many display systems, separation of the two images is achieved by a combination of polarized light emitting displays and polarizing filters. Although crosstalk and asymmetric light attenuation of the two filters are much smaller than they are for color filters, calibration is still necessary if monocular cues have to be excluded. Our algorithm can be used to achieve this for dichoptic grayscale images. Here, the red and green components of the four logical colors are interpreted as gray-scale values set for the left and right eye-channels, respectively. For example, Yellow logical color of a pixel means that it must be bright for both eyes. For a polarized light system, the gray values of the left and right eye channels must equal to the red and green components of Yellow, respectively.
Conclusions
Unwanted monocular cues are a potential source of spurious results when using random dot correlograms and stereograms to study cyclopean vision. Human observers are surprisingly sensitive to such monocular cues therefore proper calibration of RDS and RDC stimuli is essential. A numerical optimization method described in the present paper can find digital video values for a computer monitor that efficiently avoid monocular cues for color normal observers.
Supplementary Material
Acknowledgments.
We are indebted to András Frigyik and the two Reviewers for critical reading of the manuscript and for helpful suggestions. This study was supported by the following grants to P.B. and G.J.: OTKA K108747, the Hungarian Brain Research Program (KTIA_13_NAP-A-I/11 and 2017-1.2.1.-NKP-2017-00002) and the Higher Education Institutional Excellence Programme of the Ministry for Innovation and Technology in Hungary, within the framework of the 5th thematic programme of the University of Pécs as well as the Kispál Gyula Award of the University of Pécs Medical School to P.B. The publication fee of this paper was paid by the University of Pécs Medical School. The principal investigators in this study were P.B. and G. J.
Commercial relationships: none.
- Baker D.H., Kaestner M., Gouws A.D (2016). Measurement of crosstalk in stereoscopic display systems used for vision research . Journal of Vision, 16 , 14. [ PMC free article ] [ PubMed ] [ Google Scholar ]
- Boydstun A.S., Rogers J.A., Tripp L.M., & Patterson R. (2009). Stereoscopic depth perception survives significant interocular luminance differences . Journal of the Society for Information Display, 17 , 467–471. [ Google Scholar ]
- Brainard D.H. (1997). The Psychophysics Toolbox . Spatial Vision, 10 , 433–436. [ PubMed ] [ Google Scholar ]
- Brainard D.H., Pelli D.G., & Robson T. (2002). Display characterization . In Hornak J.P. (Ed.), Encyclopedia of Imaging Science and Technology (pp. 172–188): Wiley. [ Google Scholar ]
- Budai A., Czigler A., Mikó-Baráth E., Nemes V.A., Horváth G., Pusztai Á., et al.. (2018). Validation of dynamic random dot stereotests in pediatric vision screening . Graefe's Archive for Clinical and Experimental Ophthalmology, 257 , 413–423. [ PubMed ] [ Google Scholar ]
- Chang A.J., Kim H.J., Choi J.W., & Yu K.Y. (2008). Ghosting reduction method for color anaglyphs . Paper presented at the Electronic Imaging.
- Diehl R.R. (1991). Measurement of interocular delays with dynamic random-dot stereograms . European Archives of Psychiatry and Clinical Neuroscience, 241 , 115–118. [ PubMed ] [ Google Scholar ]
- Froehlich J., & Kaufman D.I. (1991). Effect of decreased retinal illumination on simultaneously recorded pattern electroretinograms and visual-evoked potentials . Investigative Ophthalmology & Visual Science, 32 , 310–318. [ PubMed ] [ Google Scholar ]
- Halpern D.L., & Blake R.R. (1988). How contrast affects stereoacuity . Perception, 17 , 483–495. [ PubMed ] [ Google Scholar ]
- Julesz B. (1960). Binocular depth perception of computer-generated patterns . Bell System Technical Journal, 39 , 1125–1162. [ Google Scholar ]
- Julesz B., Kropfl W., & Petrig B. (1980). Large evoked potentials to dynamic random-dot correlograms and stereograms permit quick determination of stereopsis . Proceedings of the National Academy of Sciences of the United States of America, 77 , 2348–2351. [ PMC free article ] [ PubMed ] [ Google Scholar ]
- Julesz B., & Miller J.E. (1962). Automatic steroscopic presentation of functions of two variables . Bell System Technical Journal, 41 , 663–676. [ Google Scholar ]
- Kleiner M., Brainard D., & Pelli D.G. (2007, 27 – 31 August 2007). What's new in Psychtoolbox-3? Paper presented at the Thirtieth European Conference on Visual Perception , Arezzo. [ Google Scholar ]
- Konrad J., Lacotte B., & Dubois E. (2000). Cancellation of image crosstalk in time-sequential displays of stereoscopic video . IEEE Transactions on Image Processing, 9 , 897–908. [ PubMed ] [ Google Scholar ]
- Kurita-Tashima S., Tobimatsu S., Nakayama-Hiromatsu M., & Kato M. (1992). The neurophysiologic significance of frontal negativity in pattern-reversal visual-evoked potentials . Investigative Ophthalmology & Visual Science, 33 , 2423–2428. [ PubMed ] [ Google Scholar ]
- Legge G.E., & Gu Y.C. (1989). Stereopsis and contrast . Vision Research, 29 , 989–1004. [ PubMed ] [ Google Scholar ]
- Markó K., Kiss H.J., Mikó-Baráth E., Bártfai O., Török B., Kovács I., et al.. (2009). Contrast independence of dynamic random dot correlogram evoked VEP amplitude . Journal of Vision, 9 , 8 1–10. [ PubMed ] [ Google Scholar ]
- Markó K., Mikó-Baráth E., Kiss H.J., Török B., & Jandó G. (2012). Effects of luminance on dynamic random-dot correlogram evoked visual potentials . Perception, 41 , 648–660. [ PubMed ] [ Google Scholar ]
- Mulligan J.B. (1986). Optimizing stereo separation in color television anaglyphs . Perception, 15 , 27–36. [ PubMed ] [ Google Scholar ]
- Pelli D.G. (1997). The VideoToolbox software for visual psychophysics: transforming numbers into movies . Spatial Vision, 10 , 437–442. [ PubMed ] [ Google Scholar ]
- Pulfrich C. (1922). Die Stereoskopie im Dienste der isochromen und heterochromen Photometrie . Naturwissenschaften, 10 , 751–761. [ Google Scholar ]
- Reynaud A., & Hess R.F. (2017). Interocular contrast difference drives illusory 3D percept . Scientific Reports, 7 , 5587. [ PMC free article ] [ PubMed ] [ Google Scholar ]
- Ross S. (2014). A first course in probability (9 ed.) Boston, MA: Pearson. [ Google Scholar ]
- Sanftmann H., & Weiskopf D. (2011). Anaglyph stereo without ghosting . Paper presented at the Proceedings of the Twenty-second Eurographics conference on Rendering , 1251–1259.
- Seuntiëns P.J.H., Meesters L.M.J., & Ijsselsteijn W.A. (2005). Perceptual attributes of crosstalk in 3D images . Displays, 26 , 177–183. [ Google Scholar ]
- Stevenson S.B., & Cormack L.K. (2000). A contrast paradox in stereopsis, motion detection, and vernier acuity . Vision Research, 40 , 2881–2884. [ PubMed ] [ Google Scholar ]
- Tsirlin I., Wilcox L.M., & Allison R.S. (2011). The Effect of Crosstalk on the Perceived Depth From Disparity and Monocular Occlusions . IEEE Transactions on Broadcasting, 57 , 445–453. [ Google Scholar ]
- Tsirlin I., Wilcox L.M., Allison R.S (2012). Effect of crosstalk on depth magnitude in thin structures . Journal of Electronic Imaging, 21 , 1–8, 8. [ Google Scholar ]
- Tyler C.W., Chan H., Liu L., McBride B., & Kontsevich L.L. (1992). Bit stealing: How to get 1786 or more gray levels from an 8-bit color monitor. Human vision, visual processing, and digital display III , 1666 , 351–364. Paper presented at the SPIE - The International Society for Optical Engineering . [ Google Scholar ]
- Wheatstone C. (1838). Contributions to the physiology of vision - Part the first. On some remarkable and hitherto unobserved phenomena of binocular vision . Philosophical Transactions of the Royal Society of London, 128 , 371–394. [ Google Scholar ]
- Wilcox L.M., & Stewart J.A.D. (2003). Determinants of perceived image quality: ghosting vs. brightness . Paper presented at the Electronic Imaging 2003 .
- Woods A.J. (2012). Crosstalk in stereoscopic displays: a review . Journal of Electronic Imaging, 21 , 1–22, 22. [ Google Scholar ]

An official website of the United States government
The .gov means it’s official. Federal government websites often end in .gov or .mil. Before sharing sensitive information, make sure you’re on a federal government site.
The site is secure. The https:// ensures that you are connecting to the official website and that any information you provide is encrypted and transmitted securely.
- Publications
- Account settings
- My Bibliography
- Collections
- Citation manager
Save citation to file
Email citation, add to collections.
- Create a new collection
- Add to an existing collection
Add to My Bibliography
Your saved search, create a file for external citation management software, your rss feed.
- Search in PubMed
- Search in NLM Catalog
- Add to Search
Enhancing Motion-In-Depth Perception of Random-Dot Stereograms
Affiliations.
- 1 School of Science, Faculty of Science and Technology, Communication University of China, Beijing, China; Optics Department, IMT Atlantique, Brest, France.
- 2 Optics Department, IMT Atlantique, Brest, France.
- PMID: 29914316
- DOI: 10.1177/0301006618775026
Random-dot stereograms have been widely used to explore the neural mechanisms underlying binocular vision. Although they are a powerful tool to stimulate motion-in-depth (MID) perception, published results report some difficulties in the capacity to perceive MID generated by random-dot stereograms. The purpose of this study was to investigate whether the performance of MID perception could be improved using an appropriate stimulus design. Sixteen inexperienced observers participated in the experiment. A training session was carried out to improve the accuracy of MID detection before the experiment. Four aspects of stimulus design were investigated: presence of a static reference, background texture, relative disparity, and stimulus contrast. Participants' performance in MID direction discrimination was recorded and compared to evaluate whether varying these factors helped MID perception. Results showed that only the presence of background texture had a significant effect on MID direction perception. This study provides suggestions for the design of 3D stimuli in order to facilitate MID perception.
Keywords: 3D perception; motion-in-depth; random-dot stereograms; stereo vision.
PubMed Disclaimer
Similar articles
- Properties of cyclopean motion perception. Patterson R, Ricker C, McGary J, Rose D. Patterson R, et al. Vision Res. 1992 Jan;32(1):149-56. doi: 10.1016/0042-6989(92)90122-y. Vision Res. 1992. PMID: 1502800
- A new method for assessing motion-in-depth perception in strabismic patients. Watanabe Y, Kezuka T, Harasawa K, Usui M, Yaguchi H, Shioiri S. Watanabe Y, et al. Br J Ophthalmol. 2008 Jan;92(1):47-50. doi: 10.1136/bjo.2007.117507. Epub 2007 Jun 27. Br J Ophthalmol. 2008. PMID: 17596334
- dmax for stereopsis and motion in random dot displays. Glennerster A. Glennerster A. Vision Res. 1998 Mar;38(6):925-35. doi: 10.1016/s0042-6989(97)00213-7. Vision Res. 1998. PMID: 9624441
- Electrophysiological correlates of human texture segregation, an overview. Bach M, Meigen T. Bach M, et al. Doc Ophthalmol. 1998-1999;95(3-4):335-47. doi: 10.1023/a:1001864625557. Doc Ophthalmol. 1998. PMID: 10532414 Review.
- Revealing the Concealed: Alternatives to Random Dots for Stereograms. Wade NJ. Wade NJ. Vision (Basel). 2023 Dec 17;7(4):78. doi: 10.3390/vision7040078. Vision (Basel). 2023. PMID: 38133481 Free PMC article. Review.
- Search in MeSH
LinkOut - more resources
Full text sources, other literature sources.
- scite Smart Citations
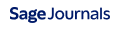
- Citation Manager
NCBI Literature Resources
MeSH PMC Bookshelf Disclaimer
The PubMed wordmark and PubMed logo are registered trademarks of the U.S. Department of Health and Human Services (HHS). Unauthorized use of these marks is strictly prohibited.
random-dot stereogram
On Thu, Oct 2, 2008 at 12:26 PM, Mark J. Pearrow < mjp@... > wrote: There is a decent template for you to work from here: http://www.mathworks.com/matlabcentral/fileexchange/loadFile.do?objectId=14859&objectType=File It's a matlab m-file mjp On Oct 2, 2008, at 12:13 PM, Adam Woods wrote: Hi all, I am attempting to write an experiment using random-dot stereograms. I was wondering if anyone has code for an experiment that they would be willing to share. I am trying to write it for a Windows XP machine. Thanks, Adam Woods
Related Topics
Topic | Replies | Views | Activity | |
---|---|---|---|---|
0 | 160 | January 3, 2003 | ||
0 | 153 | February 10, 2002 | ||
0 | 157 | April 9, 2002 | ||
2 | 704 | September 6, 2013 | ||
0 | 137 | March 22, 2005 |
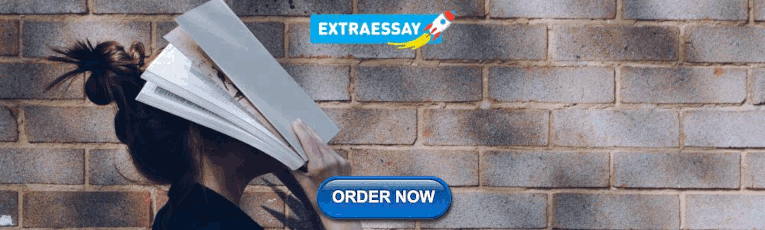
IMAGES
VIDEO
COMMENTS
A random-dot stereogram (RDS) is stereo pair of images of random dots that, when viewed with the aid of a stereoscope, or with the eyes focused on a point in front of or behind the images, produces a sensation of depth due to stereopsis, with objects appearing to be in front of or behind the display level.. The random-dot stereogram technique, known since 1919, was elaborated on by Béla ...
Single image random dot stereograms are the original versions of the popular 'Magic Eye' pictures in which a 3D image pops out from what appears to be a sheet of random dots. The dots are arranged in repeating pattern, with slight differences in each repetition. Each eye sees a slightly different pattern because of the different angles between ...
Introduction. Stereograms are illusions of 3D surfaces. This tutorial shows how it works, with a simple "do it by yourself" approach. The random dot stereogram is very easy to understand, although not a trivial concept. Unlike holograms that require laser beams, stereograms can be created, printed and visualized with computers.
The first three experiments offer a replication of the retinal position-specific learning found by Ramachandran (1976) and a reexamination and extension of the issue of random-dot pattern learning. We develop a three-part method for studying learning in random-dot stereograms that incorporates controls, for some potential difficulties in
dot stereogram was invented by Dr. Bela Julesz in 1959 as an experiment to test stereopsis, the ability to see in 3D. Read more about the history of stereograms here LINKLINKLINK. By using a random dot pattern, Julesz eliminated the depth cues that are inherent in recognizable images. Consider this example.
Random-dot stereograms are stereograms in which the images consist of a randomly arranged set of black and white dots. The lefteye and right-eye image are arranged identically, except that a portion of the dots is moved to the left or the right in one of the images to create either a crossed or an uncrossed disparity. This creates the ...
random-dot stereogram n. A type of anaglyph for studying binocular depth perception invented by the Hungarian-born US radar engineer and psychologist Bela Julesz (1928-2003) at Bell Telephone Laboratories in 1960, consisting of two random arrays of dots that when viewed stereoscopically, one array being viewed by each eye, appears to contain ...
The principles of random dot stereograms were first invented by Prof. B. Julesz in early 1960s who made the dual image random dot stereograms. In 1989, Prof. C. W. Tyler made a significant improvement to produce single image random dot stereogra (RDS) which are now found in many popular 3D pictures books around the world (see TIME (1994) Oct.3 ...
Abstract. In the present study some specific properties of the learning effects reported for random-dot stereograms are examined. In experiment 1 the retinal position-specific learning effect was reproduced and in a follow-up experiment it was shown that the position specificity of learning can be accounted for by selective visual attention.
The introduction of such random dot stereograms made it possible to examine stereovision objectively and quantitatively in isolation, and the computerized production of such stimuli stirred many quantitative experimental investigations on visual processing. ... As the flow of publications reporting on experiments employing computerized random ...
A stereogram is an image that looks like a random collection of dots or shapes, but when you look at it in a certain way, a 3D image appears. To read a stereogram, you need to relax your eyes and focus on a spot in the image. Try to let your eyes go out of focus, while keeping your gaze on the spot.
Random-dot stereogram: The random-dot stereogram was invented by Bela Julesz, a perceptual psychologist who was very influential over the past 30 years. In the example below, with anaglyph glasses you would see a square-shaped surface floating in depth in front of a background. ... This experiment established that waves of activity in primary ...
In six experiments, we examined some properties of the learning effects reported for random-dot stereograms. The first three experiments offer a controlled replication of the retinal position-specific learning found by Ramachandran (1976) and a reexamination of the issue of random-dot pattern learning. Experiment 1 replicated the position-specific learning effect. Experiments 2 and 3 offered ...
This experiment sought to determine whether giving someone information about the embedded image can help speed up how long it takes to view this image. ... (1975) Learning to see complex random-dot stereograms, Perception, 4, 173-178. This page titled 20.11: Stereograms is shared under a Public Domain license and was authored, remixed, and/or ...
Therefore, there is a need to exercise caution in generalizing the results of experiments with random-dot stereograms to normal stereo processes. • Learning to see random-dot stereograms 243 Acknowledgements. This work was supported by grants NSF BNS-8708532 and BRSG PHS2 RR 07085 to Daniel J Kersten and by grants NSF BNS85-18675 and ONR ...
Story Name: Fusion Times for Random Dot Stereograms. Abstract: This dataset contains results from an experiment in visual perception using random dot sterograms, such as that shown below. Both images appear to be composed entirely of random dots. However, they are constructed so that a 3D image (of a diamond) will be seen, if the images are ...
The first RDS (random dot stereogram) was invented by Dr. Bela Julesz in 1959 as an experiment to test stereopsis, the ability to see in 3D. By using a random dot pattern, Julesz eliminated the depth cues that are inherent in recognizable images. In 1960, Julesz tested binocular depth perception using computer generated patterns.
Single Image Random Dot Stereograms Harold W. Thimbleby,† Ian H. Witten,§ and Stuart Inglis‡ Revised 23 September 1993 Abstract This paper describes how to generate a single image which, when viewed in the appropriate way, appears to the brain as a 3D scene. The image is a stereogram composed of seemingly random dots.
Dynamic random dot stereograms (DRDSs) ( Julesz, 1960; Julesz & Miller, 1962) and correlograms (DRDCs) ( Julesz, Kropfl, & Petrig, 1980) are visual stimuli originally invented by Béla Julesz to selectively activate low-level disparity sensitive mechanisms of the visual system. A strict requirement for the utility of DRDSs is that the three ...
Download scientific diagram | A random dot stereogram depicting an example surface used in Experiment 1. from publication: The visual perception of rigid motion from constant flow fields | Four ...
Random-dot stereograms have been widely used to explore the neural mechanisms underlying binocular vision. Although they are a powerful tool to stimulate motion-in-depth (MID) perception, published results report some difficulties in the capacity to perceive MID generated by random-dot stereograms. The purpose of this study was to investigate ...
Hi all, I am attempting to write an experiment using random-dot stereograms. I was wondering if anyone has code for an experiment that they would be willing to share. I am trying to write it for a Windows XP machine. Thanks, Adam Woods. woodsaj13 October 3, 2008, 1:47pm 2. Thanks Mark, As I understand it, you need to create a vector (A) of data ...
The top and bottom images produce a dent or projection depending on whether viewed with cross- () or wall- () eyed vergence. An autostereogram is a two-dimensional (2D) image that can create the optical illusion of a three-dimensional (3D) scene. Autostereograms use only one image to accomplish the effect while normal stereograms require two. The 3D scene in an autostereogram is often ...