Have a language expert improve your writing
Run a free plagiarism check in 10 minutes, generate accurate citations for free.
- Knowledge Base
Methodology
- Survey Research | Definition, Examples & Methods
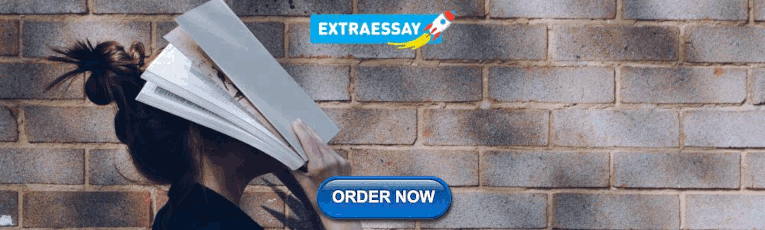
Survey Research | Definition, Examples & Methods
Published on August 20, 2019 by Shona McCombes . Revised on June 22, 2023.
Survey research means collecting information about a group of people by asking them questions and analyzing the results. To conduct an effective survey, follow these six steps:
- Determine who will participate in the survey
- Decide the type of survey (mail, online, or in-person)
- Design the survey questions and layout
- Distribute the survey
- Analyze the responses
- Write up the results
Surveys are a flexible method of data collection that can be used in many different types of research .
Table of contents
What are surveys used for, step 1: define the population and sample, step 2: decide on the type of survey, step 3: design the survey questions, step 4: distribute the survey and collect responses, step 5: analyze the survey results, step 6: write up the survey results, other interesting articles, frequently asked questions about surveys.
Surveys are used as a method of gathering data in many different fields. They are a good choice when you want to find out about the characteristics, preferences, opinions, or beliefs of a group of people.
Common uses of survey research include:
- Social research : investigating the experiences and characteristics of different social groups
- Market research : finding out what customers think about products, services, and companies
- Health research : collecting data from patients about symptoms and treatments
- Politics : measuring public opinion about parties and policies
- Psychology : researching personality traits, preferences and behaviours
Surveys can be used in both cross-sectional studies , where you collect data just once, and in longitudinal studies , where you survey the same sample several times over an extended period.
Here's why students love Scribbr's proofreading services
Discover proofreading & editing
Before you start conducting survey research, you should already have a clear research question that defines what you want to find out. Based on this question, you need to determine exactly who you will target to participate in the survey.
Populations
The target population is the specific group of people that you want to find out about. This group can be very broad or relatively narrow. For example:
- The population of Brazil
- US college students
- Second-generation immigrants in the Netherlands
- Customers of a specific company aged 18-24
- British transgender women over the age of 50
Your survey should aim to produce results that can be generalized to the whole population. That means you need to carefully define exactly who you want to draw conclusions about.
Several common research biases can arise if your survey is not generalizable, particularly sampling bias and selection bias . The presence of these biases have serious repercussions for the validity of your results.
It’s rarely possible to survey the entire population of your research – it would be very difficult to get a response from every person in Brazil or every college student in the US. Instead, you will usually survey a sample from the population.
The sample size depends on how big the population is. You can use an online sample calculator to work out how many responses you need.
There are many sampling methods that allow you to generalize to broad populations. In general, though, the sample should aim to be representative of the population as a whole. The larger and more representative your sample, the more valid your conclusions. Again, beware of various types of sampling bias as you design your sample, particularly self-selection bias , nonresponse bias , undercoverage bias , and survivorship bias .
There are two main types of survey:
- A questionnaire , where a list of questions is distributed by mail, online or in person, and respondents fill it out themselves.
- An interview , where the researcher asks a set of questions by phone or in person and records the responses.
Which type you choose depends on the sample size and location, as well as the focus of the research.
Questionnaires
Sending out a paper survey by mail is a common method of gathering demographic information (for example, in a government census of the population).
- You can easily access a large sample.
- You have some control over who is included in the sample (e.g. residents of a specific region).
- The response rate is often low, and at risk for biases like self-selection bias .
Online surveys are a popular choice for students doing dissertation research , due to the low cost and flexibility of this method. There are many online tools available for constructing surveys, such as SurveyMonkey and Google Forms .
- You can quickly access a large sample without constraints on time or location.
- The data is easy to process and analyze.
- The anonymity and accessibility of online surveys mean you have less control over who responds, which can lead to biases like self-selection bias .
If your research focuses on a specific location, you can distribute a written questionnaire to be completed by respondents on the spot. For example, you could approach the customers of a shopping mall or ask all students to complete a questionnaire at the end of a class.
- You can screen respondents to make sure only people in the target population are included in the sample.
- You can collect time- and location-specific data (e.g. the opinions of a store’s weekday customers).
- The sample size will be smaller, so this method is less suitable for collecting data on broad populations and is at risk for sampling bias .
Oral interviews are a useful method for smaller sample sizes. They allow you to gather more in-depth information on people’s opinions and preferences. You can conduct interviews by phone or in person.
- You have personal contact with respondents, so you know exactly who will be included in the sample in advance.
- You can clarify questions and ask for follow-up information when necessary.
- The lack of anonymity may cause respondents to answer less honestly, and there is more risk of researcher bias.
Like questionnaires, interviews can be used to collect quantitative data: the researcher records each response as a category or rating and statistically analyzes the results. But they are more commonly used to collect qualitative data : the interviewees’ full responses are transcribed and analyzed individually to gain a richer understanding of their opinions and feelings.
Next, you need to decide which questions you will ask and how you will ask them. It’s important to consider:
- The type of questions
- The content of the questions
- The phrasing of the questions
- The ordering and layout of the survey
Open-ended vs closed-ended questions
There are two main forms of survey questions: open-ended and closed-ended. Many surveys use a combination of both.
Closed-ended questions give the respondent a predetermined set of answers to choose from. A closed-ended question can include:
- A binary answer (e.g. yes/no or agree/disagree )
- A scale (e.g. a Likert scale with five points ranging from strongly agree to strongly disagree )
- A list of options with a single answer possible (e.g. age categories)
- A list of options with multiple answers possible (e.g. leisure interests)
Closed-ended questions are best for quantitative research . They provide you with numerical data that can be statistically analyzed to find patterns, trends, and correlations .
Open-ended questions are best for qualitative research. This type of question has no predetermined answers to choose from. Instead, the respondent answers in their own words.
Open questions are most common in interviews, but you can also use them in questionnaires. They are often useful as follow-up questions to ask for more detailed explanations of responses to the closed questions.
The content of the survey questions
To ensure the validity and reliability of your results, you need to carefully consider each question in the survey. All questions should be narrowly focused with enough context for the respondent to answer accurately. Avoid questions that are not directly relevant to the survey’s purpose.
When constructing closed-ended questions, ensure that the options cover all possibilities. If you include a list of options that isn’t exhaustive, you can add an “other” field.
Phrasing the survey questions
In terms of language, the survey questions should be as clear and precise as possible. Tailor the questions to your target population, keeping in mind their level of knowledge of the topic. Avoid jargon or industry-specific terminology.
Survey questions are at risk for biases like social desirability bias , the Hawthorne effect , or demand characteristics . It’s critical to use language that respondents will easily understand, and avoid words with vague or ambiguous meanings. Make sure your questions are phrased neutrally, with no indication that you’d prefer a particular answer or emotion.
Ordering the survey questions
The questions should be arranged in a logical order. Start with easy, non-sensitive, closed-ended questions that will encourage the respondent to continue.
If the survey covers several different topics or themes, group together related questions. You can divide a questionnaire into sections to help respondents understand what is being asked in each part.
If a question refers back to or depends on the answer to a previous question, they should be placed directly next to one another.
Before you start, create a clear plan for where, when, how, and with whom you will conduct the survey. Determine in advance how many responses you require and how you will gain access to the sample.
When you are satisfied that you have created a strong research design suitable for answering your research questions, you can conduct the survey through your method of choice – by mail, online, or in person.
There are many methods of analyzing the results of your survey. First you have to process the data, usually with the help of a computer program to sort all the responses. You should also clean the data by removing incomplete or incorrectly completed responses.
If you asked open-ended questions, you will have to code the responses by assigning labels to each response and organizing them into categories or themes. You can also use more qualitative methods, such as thematic analysis , which is especially suitable for analyzing interviews.
Statistical analysis is usually conducted using programs like SPSS or Stata. The same set of survey data can be subject to many analyses.
Finally, when you have collected and analyzed all the necessary data, you will write it up as part of your thesis, dissertation , or research paper .
In the methodology section, you describe exactly how you conducted the survey. You should explain the types of questions you used, the sampling method, when and where the survey took place, and the response rate. You can include the full questionnaire as an appendix and refer to it in the text if relevant.
Then introduce the analysis by describing how you prepared the data and the statistical methods you used to analyze it. In the results section, you summarize the key results from your analysis.
In the discussion and conclusion , you give your explanations and interpretations of these results, answer your research question, and reflect on the implications and limitations of the research.
If you want to know more about statistics , methodology , or research bias , make sure to check out some of our other articles with explanations and examples.
- Student’s t -distribution
- Normal distribution
- Null and Alternative Hypotheses
- Chi square tests
- Confidence interval
- Quartiles & Quantiles
- Cluster sampling
- Stratified sampling
- Data cleansing
- Reproducibility vs Replicability
- Peer review
- Prospective cohort study
Research bias
- Implicit bias
- Cognitive bias
- Placebo effect
- Hawthorne effect
- Hindsight bias
- Affect heuristic
- Social desirability bias
A questionnaire is a data collection tool or instrument, while a survey is an overarching research method that involves collecting and analyzing data from people using questionnaires.
A Likert scale is a rating scale that quantitatively assesses opinions, attitudes, or behaviors. It is made up of 4 or more questions that measure a single attitude or trait when response scores are combined.
To use a Likert scale in a survey , you present participants with Likert-type questions or statements, and a continuum of items, usually with 5 or 7 possible responses, to capture their degree of agreement.
Individual Likert-type questions are generally considered ordinal data , because the items have clear rank order, but don’t have an even distribution.
Overall Likert scale scores are sometimes treated as interval data. These scores are considered to have directionality and even spacing between them.
The type of data determines what statistical tests you should use to analyze your data.
The priorities of a research design can vary depending on the field, but you usually have to specify:
- Your research questions and/or hypotheses
- Your overall approach (e.g., qualitative or quantitative )
- The type of design you’re using (e.g., a survey , experiment , or case study )
- Your sampling methods or criteria for selecting subjects
- Your data collection methods (e.g., questionnaires , observations)
- Your data collection procedures (e.g., operationalization , timing and data management)
- Your data analysis methods (e.g., statistical tests or thematic analysis )
Cite this Scribbr article
If you want to cite this source, you can copy and paste the citation or click the “Cite this Scribbr article” button to automatically add the citation to our free Citation Generator.
McCombes, S. (2023, June 22). Survey Research | Definition, Examples & Methods. Scribbr. Retrieved August 7, 2024, from https://www.scribbr.com/methodology/survey-research/
Is this article helpful?
Shona McCombes
Other students also liked, qualitative vs. quantitative research | differences, examples & methods, questionnaire design | methods, question types & examples, what is a likert scale | guide & examples, what is your plagiarism score.
What is survey sampling: Understanding methodology and sampling techniques
The importance of survey sampling, cost-effectiveness, increased efficiency, generalizability, practicality, reducing bias, flexibility, ethical considerations, feasibility of analysis, population and sampling frame, sample size, simple random sampling, stratified sampling, systematic sampling, cluster sampling, convenience sampling, use surveyplanet to start survey sampling.
Surveys are crucial to the collection of valuable data and insights for research, market analysis, and decision-making. However, surveying an entire population is impractical and too costly.
This is why there is survey sampling. What is survey sampling? It is a statistical method that allows researchers to study a smaller population subset to draw meaningful conclusions about the entire group. Now we’ll cover its importance, fundamentals, and the various sampling techniques that researchers use in survey sampling.
Imagine a scenario where you want to study the preferences of coffee drinkers across an entire country. Interviewing every coffee lover would be impossible. Survey sampling allows researchers to select a representative group that can provide insights applicable to the entire population of coffee enthusiasts.
Properly conducted survey sampling yields reliable results while being cost and time-efficient.
The benefits of survey sampling
Survey sampling offers several benefits that make it a valuable research technique. Some of the key advantages include:
Surveying an entire population can be time-consuming, expensive, and sometimes impractical. Sampling helps researchers gather data from a population subset, significantly reducing costs, time, and effort—while still providing valuable insights.
By focusing on a smaller, representative sample, researchers can efficiently gather the necessary information without having to collect data from every individual in the population. This increased efficiency is particularly useful for large or geographically dispersed populations.
When done correctly, survey sampling provides results that are representative of the entire population. This means that the findings obtained from the sample can be generalized to the larger group, allowing researchers to draw conclusions and make inferences about the population as a whole.
Surveys are versatile research tools that can be applied to various research questions and objectives. Sampling makes it feasible to study complex or hard-to-reach populations for which conducting a census might not be feasible.
When a well-designed sampling method is used, it can help reduce potential bias in the data. Bias occurs when the sample needs to accurately represent the characteristics of the population, leading to accurate conclusions.
Proper sampling techniques, such as random sampling or stratified sampling, aim to minimize bias and improve the reliability of the findings. Learn how to avoid biased survey questions with our tips and tricks and successfully avoid gathering inaccurate data.
Surveys can be adapted to different data collection methods, such as face-to-face interviews, telephone surveys, online questionnaires, or mail surveys. This flexibility allows researchers to choose the most suitable method for their specific research context.
In some cases, conducting a census or surveying an entire population may raise ethical concerns, especially if the study involves sensitive or personal information. Sampling can protect the privacy and confidentiality of individuals since only a subset of the population is involved.
Read our article about the most common types of errors in surveying respondents and concise guidelines on how to avoid them.
Smaller sample sizes are more manageable for data analysis, especially when dealing with large datasets. Researchers can process the collected data more easily, leading to quicker results and insights.
Understanding survey sampling methodology
Survey sampling methods are essential techniques used in statistics and research to gather data from a subset of a larger population. The objective is to obtain accurate and reliable information about the entire population while minimizing costs and time.
We’ll now cover some common survey sampling methods. If you want to learn more about survey methodology, read our ultimate guide to survey data collection with methods, examples, and analysis .
The population is the entire group of individuals, items, or events that researchers aim to study. It represents the target of interest for the research.
Defining the population accurately is crucial because the conclusions drawn from the sample will be generalized back to this larger group. For example, if a company wants to understand the preferences of smartphone users in a country, the population would be all smartphone users within that country.
The sampling frame is a list or representation of all elements within the population. It acts as the basis for selecting the sample. It is essential to ensure that the sampling frame is comprehensive and up-to-date, as any omissions or inaccuracies may introduce bias into the sample.
For instance, in the smartphone user survey, the sampling frame ideally includes an updated list of all smartphone users or phone numbers within the country.
Determining a good survey sample size is one of the most important steps in survey sampling. The sample size directly impacts the precision of the estimates derived from the survey. A larger sample size generally results in more accurate findings, as it reduces the margin of error and increases the statistical power of the study. However, larger samples may also incur higher costs and require more time to collect and analyze the data.
The sample size calculation involves considering factors such as the desired confidence level, margin of error, population variability, and precision level required. Researchers often use statistical formulas to determine the optimal sample size that balances cost, time, and accuracy.
Explore our survey sample size calculator that can help you determine how many people you need to survey for data to be statistically significant.
Simple random sampling is one of the most straightforward and commonly used sampling techniques. It involves randomly selecting individuals from the sampling frame with each member of it having an equal probability of being chosen. This method ensures that the sample is representative of the population and reduces the risk of bias. Random number generators or drawing lots are often used to implement this technique.
Simple random sampling is particularly useful when the population is relatively homogeneous and there is no specific pattern or structure within the data. It is widely used in various research fields and surveys.
Stratified sampling is employed when the population can be divided into subgroups or strata based on specific characteristics or attributes. These characteristics can be demographic (age, gender), socioeconomic (income, education), geographic (region), or any other relevant factor.
Researchers then take random samples from each stratum. The sample size is proportional to the size of each stratum within the population. This method ensures that each subgroup is well represented in the final sample, making it ideal for studying the variations between different population segments.
For example, a study examining consumer preferences for a new product might use stratified sampling to ensure representation from different age groups and income levels.
Systematic sampling is a convenient and straightforward technique. It involves selecting every “k-th” element from the sampling frame after randomly choosing a starting point. The value of “k” is calculated by dividing the population size by the sample size. For instance, if the population size is 1000 and the desired sample size is 100, “k” would be 1000/100 = 10. Every 10th individual in the sampling frame would be selected for the sample.
Easy to implement and often more efficient than simple random sampling, systematic sampling is especially helpful when no specific order or pattern exists within the data. However, it may introduce bias if there is a periodicity or pattern in the sampling frame that aligns with the value of “k.”
Cluster sampling is employed when the population is geographically or organizationally clustered. Instead of sampling individuals directly from the sampling frame, researchers first divide the population into clusters or groups (e.g., neighborhoods, schools, companies).
Random samples of clusters are selected, with all individuals within the chosen clusters surveyed. Cluster sampling is useful when it is impractical to sample individuals directly. It also has the advantage of reducing costs and logistical challenges.
However, cluster sampling can introduce cluster-level biases and the sample may not be as diverse as other sampling techniques. Careful consideration of the clusters’ representativeness is essential to ensure valid conclusions.
Convenience sampling involves selecting individuals who are easily accessible or readily available to the researcher. This sampling method is often used when time and resources are limited, making it convenient to gather data quickly.
While convenience sampling is easy to implement, it can introduce significant bias into the results. The individuals selected may not be similar to the larger population, leading to potential inaccuracies in the findings. It is essential to interpret the results from convenience sampling with caution and recognize its limitations.
Survey sampling is a powerful tool that allows researchers to draw meaningful conclusions about a larger population by studying a carefully selected subset. Understanding the various sampling techniques and their appropriate use is crucial for obtaining reliable and valid results.
By applying survey sampling methodologies effectively, researchers can gain valuable insights and make better-informed decisions. The next time you come across survey data, consider the sampling technique used—it greatly impacts the credibility of the findings.
Sign up at SurveyPlanet to use hundreds of features that will help you create the perfect survey and then draw the most accurate conclusions about any subject of interest.
An overview of sampling methods
Last updated
27 February 2023
Reviewed by
Cathy Heath
When researching perceptions or attributes of a product, service, or people, you have two options:
Survey every person in your chosen group (the target market, or population), collate your responses, and reach your conclusions.
Select a smaller group from within your target market and use their answers to represent everyone. This option is sampling .
Sampling saves you time and money. When you use the sampling method, the whole population being studied is called the sampling frame .
The sample you choose should represent your target market, or the sampling frame, well enough to do one of the following:
Generalize your findings across the sampling frame and use them as though you had surveyed everyone
Use the findings to decide on your next step, which might involve more in-depth sampling
Make research less tedious
Dovetail streamlines research to help you uncover and share actionable insights
How was sampling developed?
Valery Glivenko and Francesco Cantelli, two mathematicians studying probability theory in the early 1900s, devised the sampling method. Their research showed that a properly chosen sample of people would reflect the larger group’s status, opinions, decisions, and decision-making steps.
They proved you don't need to survey the entire target market, thereby saving the rest of us a lot of time and money.
- Why is sampling important?
We’ve already touched on the fact that sampling saves you time and money. When you get reliable results quickly, you can act on them sooner. And the money you save can pay for something else.
It’s often easier to survey a sample than a whole population. Sample inferences can be more reliable than those you get from a very large group because you can choose your samples carefully and scientifically.
Sampling is also useful because it is often impossible to survey the entire population. You probably have no choice but to collect only a sample in the first place.
Because you’re working with fewer people, you can collect richer data, which makes your research more accurate. You can:
Ask more questions
Go into more detail
Seek opinions instead of just collecting facts
Observe user behaviors
Double-check your findings if you need to
In short, sampling works! Let's take a look at the most common sampling methods.
- Types of sampling methods
There are two main sampling methods: probability sampling and non-probability sampling. These can be further refined, which we'll cover shortly. You can then decide which approach best suits your research project.
Probability sampling method
Probability sampling is used in quantitative research , so it provides data on the survey topic in terms of numbers. Probability relates to mathematics, hence the name ‘quantitative research’. Subjects are asked questions like:
How many boxes of candy do you buy at one time?
How often do you shop for candy?
How much would you pay for a box of candy?
This method is also called random sampling because everyone in the target market has an equal chance of being chosen for the survey. It is designed to reduce sampling error for the most important variables. You should, therefore, get results that fairly reflect the larger population.
Non-probability sampling method
In this method, not everyone has an equal chance of being part of the sample. It's usually easier (and cheaper) to select people for the sample group. You choose people who are more likely to be involved in or know more about the topic you’re researching.
Non-probability sampling is used for qualitative research. Qualitative data is generated by questions like:
Where do you usually shop for candy (supermarket, gas station, etc.?)
Which candy brand do you usually buy?
Why do you like that brand?
- Probability sampling methods
Here are five ways of doing probability sampling:
Simple random sampling (basic probability sampling)
Systematic sampling
Stratified sampling.
Cluster sampling
Multi-stage sampling
Simple random sampling.
There are three basic steps to simple random sampling:
Choose your sampling frame.
Decide on your sample size. Make sure it is large enough to give you reliable data.
Randomly choose your sample participants.
You could put all their names in a hat, shake the hat to mix the names, and pull out however many names you want in your sample (without looking!)
You could be more scientific by giving each participant a number and then using a random number generator program to choose the numbers.
Instead of choosing names or numbers, you decide beforehand on a selection method. For example, collect all the names in your sampling frame and start at, for example, the fifth person on the list, then choose every fourth name or every tenth name. Alternatively, you could choose everyone whose last name begins with randomly-selected initials, such as A, G, or W.
Choose your system of selecting names, and away you go.
This is a more sophisticated way to choose your sample. You break the sampling frame down into important subgroups or strata . Then, decide how many you want in your sample, and choose an equal number (or a proportionate number) from each subgroup.
For example, you want to survey how many people in a geographic area buy candy, so you compile a list of everyone in that area. You then break that list down into, for example, males and females, then into pre-teens, teenagers, young adults, senior citizens, etc. who are male or female.
So, if there are 1,000 young male adults and 2,000 young female adults in the whole sampling frame, you may want to choose 100 males and 200 females to keep the proportions balanced. You then choose the individual survey participants through the systematic sampling method.
Clustered sampling
This method is used when you want to subdivide a sample into smaller groups or clusters that are geographically or organizationally related.
Let’s say you’re doing quantitative research into candy sales. You could choose your sample participants from urban, suburban, or rural populations. This would give you three geographic clusters from which to select your participants.
This is a more refined way of doing cluster sampling. Let’s say you have your urban cluster, which is your primary sampling unit. You can subdivide this into a secondary sampling unit, say, participants who typically buy their candy in supermarkets. You could then further subdivide this group into your ultimate sampling unit. Finally, you select the actual survey participants from this unit.
- Uses of probability sampling
Probability sampling has three main advantages:
It helps minimizes the likelihood of sampling bias. How you choose your sample determines the quality of your results. Probability sampling gives you an unbiased, randomly selected sample of your target market.
It allows you to create representative samples and subgroups within a sample out of a large or diverse target market.
It lets you use sophisticated statistical methods to select as close to perfect samples as possible.
- Non-probability sampling methods
To recap, with non-probability sampling, you choose people for your sample in a non-random way, so not everyone in your sampling frame has an equal chance of being chosen. Your research findings, therefore, may not be as representative overall as probability sampling, but you may not want them to be.
Sampling bias is not a concern if all potential survey participants share similar traits. For example, you may want to specifically focus on young male adults who spend more than others on candy. In addition, it is usually a cheaper and quicker method because you don't have to work out a complex selection system that represents the entire population in that community.
Researchers do need to be mindful of carefully considering the strengths and limitations of each method before selecting a sampling technique.
Non-probability sampling is best for exploratory research , such as at the beginning of a research project.
There are five main types of non-probability sampling methods:
Convenience sampling
Purposive sampling, voluntary response sampling, snowball sampling, quota sampling.
The strategy of convenience sampling is to choose your sample quickly and efficiently, using the least effort, usually to save money.
Let's say you want to survey the opinions of 100 millennials about a particular topic. You could send out a questionnaire over the social media platforms millennials use. Ask respondents to confirm their birth year at the top of their response sheet and, when you have your 100 responses, begin your analysis. Or you could visit restaurants and bars where millennials spend their evenings and sign people up.
A drawback of convenience sampling is that it may not yield results that apply to a broader population.
This method relies on your judgment to choose the most likely sample to deliver the most useful results. You must know enough about the survey goals and the sampling frame to choose the most appropriate sample respondents.
Your knowledge and experience save you time because you know your ideal sample candidates, so you should get high-quality results.
This method is similar to convenience sampling, but it is based on potential sample members volunteering rather than you looking for people.
You make it known you want to do a survey on a particular topic for a particular reason and wait until enough people volunteer. Then you give them the questionnaire or arrange interviews to ask your questions directly.
Snowball sampling involves asking selected participants to refer others who may qualify for the survey. This method is best used when there is no sampling frame available. It is also useful when the researcher doesn’t know much about the target population.
Let's say you want to research a niche topic that involves people who may be difficult to locate. For our candy example, this could be young males who buy a lot of candy, go rock climbing during the day, and watch adventure movies at night. You ask each participant to name others they know who do the same things, so you can contact them. As you make contact with more people, your sample 'snowballs' until you have all the names you need.
This sampling method involves collecting the specific number of units (quotas) from your predetermined subpopulations. Quota sampling is a way of ensuring that your sample accurately represents the sampling frame.
- Uses of non-probability sampling
You can use non-probability sampling when you:
Want to do a quick test to see if a more detailed and sophisticated survey may be worthwhile
Want to explore an idea to see if it 'has legs'
Launch a pilot study
Do some initial qualitative research
Have little time or money available (half a loaf is better than no bread at all)
Want to see if the initial results will help you justify a longer, more detailed, and more expensive research project
- The main types of sampling bias, and how to avoid them
Sampling bias can fog or limit your research results. This will have an impact when you generalize your results across the whole target market. The two main causes of sampling bias are faulty research design and poor data collection or recording. They can affect probability and non-probability sampling.
Faulty research
If a surveyor chooses participants inappropriately, the results will not reflect the population as a whole.
A famous example is the 1948 presidential race. A telephone survey was conducted to see which candidate had more support. The problem with the research design was that, in 1948, most people with telephones were wealthy, and their opinions were very different from voters as a whole. The research implied Dewey would win, but it was Truman who became president.
Poor data collection or recording
This problem speaks for itself. The survey may be well structured, the sample groups appropriate, the questions clear and easy to understand, and the cluster sizes appropriate. But if surveyors check the wrong boxes when they get an answer or if the entire subgroup results are lost, the survey results will be biased.
How do you minimize bias in sampling?
To get results you can rely on, you must:
Know enough about your target market
Choose one or more sample surveys to cover the whole target market properly
Choose enough people in each sample so your results mirror your target market
Have content validity . This means the content of your questions must be direct and efficiently worded. If it isn’t, the viability of your survey could be questioned. That would also be a waste of time and money, so make the wording of your questions your top focus.
If using probability sampling, make sure your sampling frame includes everyone it should and that your random sampling selection process includes the right proportion of the subgroups
If using non-probability sampling, focus on fairness, equality, and completeness in identifying your samples and subgroups. Then balance those criteria against simple convenience or other relevant factors.
What are the five types of sampling bias?
Self-selection bias. If you mass-mail questionnaires to everyone in the sample, you’re more likely to get results from people with extrovert or activist personalities and not from introverts or pragmatists. So if your convenience sampling focuses on getting your quota responses quickly, it may be skewed.
Non-response bias. Unhappy customers, stressed-out employees, or other sub-groups may not want to cooperate or they may pull out early.
Undercoverage bias. If your survey is done, say, via email or social media platforms, it will miss people without internet access, such as those living in rural areas, the elderly, or lower-income groups.
Survivorship bias. Unsuccessful people are less likely to take part. Another example may be a researcher excluding results that don’t support the overall goal. If the CEO wants to tell the shareholders about a successful product or project at the AGM, some less positive survey results may go “missing” (to take an extreme example.) The result is that your data will reflect an overly optimistic representation of the truth.
Pre-screening bias. If the researcher, whose experience and knowledge are being used to pre-select respondents in a judgmental sampling, focuses more on convenience than judgment, the results may be compromised.
How do you minimize sampling bias?
Focus on the bullet points in the next section and:
Make survey questionnaires as direct, easy, short, and available as possible, so participants are more likely to complete them accurately and send them back
Follow up with the people who have been selected but have not returned their responses
Ignore any pressure that may produce bias
- How do you decide on the type of sampling to use?
Use the ideas you've gleaned from this article to give yourself a platform, then choose the best method to meet your goals while staying within your time and cost limits.
If it isn't obvious which method you should choose, use this strategy:
Clarify your research goals
Clarify how accurate your research results must be to reach your goals
Evaluate your goals against time and budget
List the two or three most obvious sampling methods that will work for you
Confirm the availability of your resources (researchers, computer time, etc.)
Compare each of the possible methods with your goals, accuracy, precision, resource, time, and cost constraints
Make your decision
- The takeaway
Effective market research is the basis of successful marketing, advertising, and future productivity. By selecting the most appropriate sampling methods, you will collect the most useful market data and make the most effective decisions.
Should you be using a customer insights hub?
Do you want to discover previous research faster?
Do you share your research findings with others?
Do you analyze research data?
Start for free today, add your research, and get to key insights faster
Editor’s picks
Last updated: 18 April 2023
Last updated: 27 February 2023
Last updated: 6 February 2023
Last updated: 5 February 2023
Last updated: 16 April 2023
Last updated: 9 March 2023
Last updated: 30 April 2024
Last updated: 12 December 2023
Last updated: 11 March 2024
Last updated: 4 July 2024
Last updated: 6 March 2024
Last updated: 5 March 2024
Last updated: 13 May 2024
Latest articles
Related topics, .css-je19u9{-webkit-align-items:flex-end;-webkit-box-align:flex-end;-ms-flex-align:flex-end;align-items:flex-end;display:-webkit-box;display:-webkit-flex;display:-ms-flexbox;display:flex;-webkit-flex-direction:row;-ms-flex-direction:row;flex-direction:row;-webkit-box-flex-wrap:wrap;-webkit-flex-wrap:wrap;-ms-flex-wrap:wrap;flex-wrap:wrap;-webkit-box-pack:center;-ms-flex-pack:center;-webkit-justify-content:center;justify-content:center;row-gap:0;text-align:center;max-width:671px;}@media (max-width: 1079px){.css-je19u9{max-width:400px;}.css-je19u9>span{white-space:pre;}}@media (max-width: 799px){.css-je19u9{max-width:400px;}.css-je19u9>span{white-space:pre;}} decide what to .css-1kiodld{max-height:56px;display:-webkit-box;display:-webkit-flex;display:-ms-flexbox;display:flex;-webkit-align-items:center;-webkit-box-align:center;-ms-flex-align:center;align-items:center;}@media (max-width: 1079px){.css-1kiodld{display:none;}} build next, decide what to build next, log in or sign up.
Get started for free
Root out friction in every digital experience, super-charge conversion rates, and optimise digital self-service
Uncover insights from any interaction, deliver AI-powered agent coaching, and reduce cost to serve
Increase revenue and loyalty with real-time insights and recommendations delivered straight to teams on the ground
Know how your people feel and empower managers to improve employee engagement, productivity, and retention
Take action in the moments that matter most along the employee journey and drive bottom line growth
Whatever they’re are saying, wherever they’re saying it, know exactly what’s going on with your people
Get faster, richer insights with qual and quant tools that make powerful market research available to everyone
Run concept tests, pricing studies, prototyping + more with fast, powerful studies designed by UX research experts
Track your brand performance 24/7 and act quickly to respond to opportunities and challenges in your market
Meet the operating system for experience management
- Free Account
- Product Demos
- For Digital
- For Customer Care
- For Human Resources
- For Researchers
- Financial Services
- All Industries
Popular Use Cases
- Customer Experience
- Employee Experience
- Employee Exit Interviews
- Net Promoter Score
- Voice of Customer
- Customer Success Hub
- Product Documentation
- Training & Certification
- XM Institute
- Popular Resources
- Customer Stories
- Artificial Intelligence
- Market Research
- Partnerships
- Marketplace
The annual gathering of the experience leaders at the world’s iconic brands building breakthrough business results.
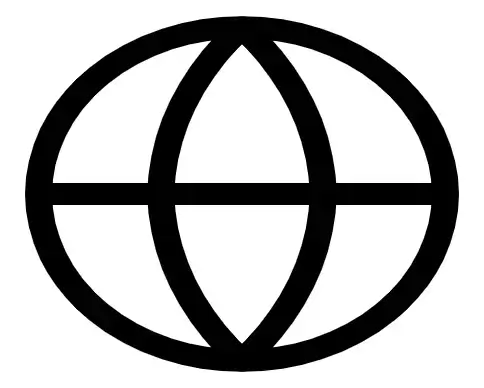
- English/AU & NZ
- Español/Europa
- Español/América Latina
- Português Brasileiro
- REQUEST DEMO
- Experience Management
- Sampling Methods
Try Qualtrics for free
Sampling methods, types & techniques.
15 min read Your comprehensive guide to the different sampling methods available to researchers – and how to know which is right for your research.
What is sampling?
In survey research, sampling is the process of using a subset of a population to represent the whole population. To help illustrate this further, let’s look at data sampling methods with examples below.
Let’s say you wanted to do some research on everyone in North America. To ask every person would be almost impossible. Even if everyone said “yes”, carrying out a survey across different states, in different languages and timezones, and then collecting and processing all the results , would take a long time and be very costly.
Sampling allows large-scale research to be carried out with a more realistic cost and time-frame because it uses a smaller number of individuals in the population with representative characteristics to stand in for the whole.
However, when you decide to sample, you take on a new task. You have to decide who is part of your sample list and how to choose the people who will best represent the whole population. How you go about that is what the practice of sampling is all about.
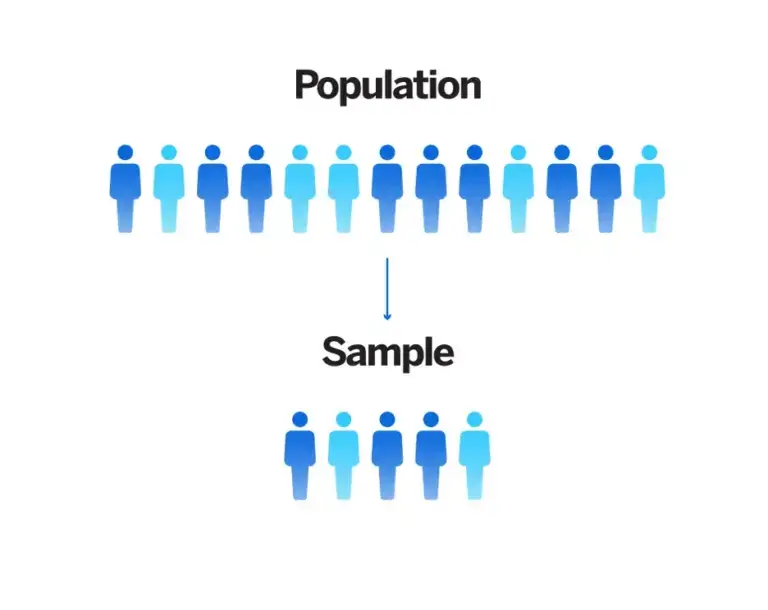
Sampling definitions
- Population: The total number of people or things you are interested in
- Sample: A smaller number within your population that will represent the whole
- Sampling: The process and method of selecting your sample
Free eBook: 2024 Market Research Trends
Why is sampling important?
Although the idea of sampling is easiest to understand when you think about a very large population, it makes sense to use sampling methods in research studies of all types and sizes. After all, if you can reduce the effort and cost of doing a study, why wouldn’t you? And because sampling allows you to research larger target populations using the same resources as you would smaller ones, it dramatically opens up the possibilities for research.
Sampling is a little like having gears on a car or bicycle. Instead of always turning a set of wheels of a specific size and being constrained by their physical properties, it allows you to translate your effort to the wheels via the different gears, so you’re effectively choosing bigger or smaller wheels depending on the terrain you’re on and how much work you’re able to do.
Sampling allows you to “gear” your research so you’re less limited by the constraints of cost, time, and complexity that come with different population sizes.
It allows us to do things like carrying out exit polls during elections, map the spread and effects rates of epidemics across geographical areas, and carry out nationwide census research that provides a snapshot of society and culture.
Types of sampling
Sampling strategies in research vary widely across different disciplines and research areas, and from study to study.
There are two major types of sampling methods: probability and non-probability sampling.
- Probability sampling , also known as random sampling , is a kind of sample selection where randomisation is used instead of deliberate choice. Each member of the population has a known, non-zero chance of being selected.
- Non-probability sampling techniques are where the researcher deliberately picks items or individuals for the sample based on non-random factors such as convenience, geographic availability, or costs.
As we delve into these categories, it’s essential to understand the nuances and applications of each method to ensure that the chosen sampling strategy aligns with the research goals.
Probability sampling methods
There’s a wide range of probability sampling methods to explore and consider. Here are some of the best-known options.
1. Simple random sampling
With simple random sampling , every element in the population has an equal chance of being selected as part of the sample. It’s something like picking a name out of a hat. Simple random sampling can be done by anonymising the population – e.g. by assigning each item or person in the population a number and then picking numbers at random.
Pros: Simple random sampling is easy to do and cheap. Designed to ensure that every member of the population has an equal chance of being selected, it reduces the risk of bias compared to non-random sampling.
Cons: It offers no control for the researcher and may lead to unrepresentative groupings being picked by chance.
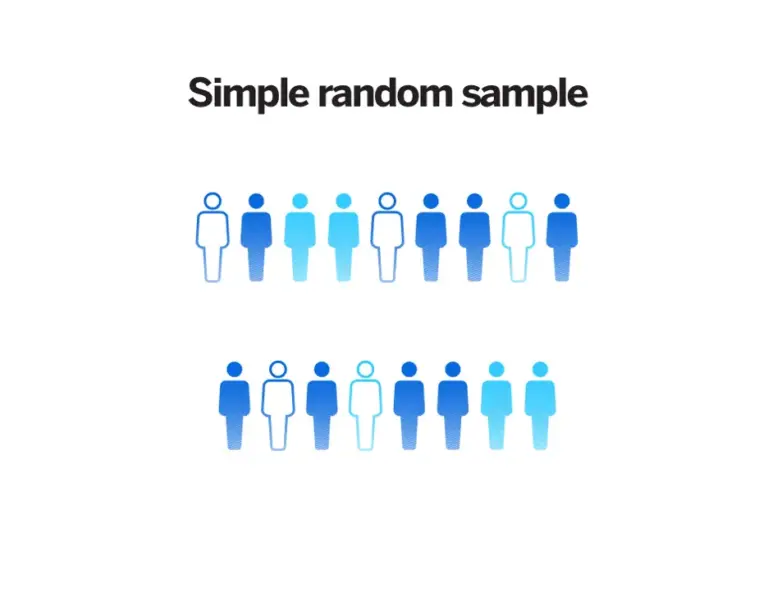
2. Systematic sampling
With systematic sampling the random selection only applies to the first item chosen. A rule then applies so that every nth item or person after that is picked.
Best practice is to sort your list in a random way to ensure that selections won’t be accidentally clustered together. This is commonly achieved using a random number generator. If that’s not available you might order your list alphabetically by first name and then pick every fifth name to eliminate bias, for example.
Next, you need to decide your sampling interval – for example, if your sample will be 10% of your full list, your sampling interval is one in 10 – and pick a random start between one and 10 – for example three. This means you would start with person number three on your list and pick every tenth person.
Pros: Systematic sampling is efficient and straightforward, especially when dealing with populations that have a clear order. It ensures a uniform selection across the population.
Cons: There’s a potential risk of introducing bias if there’s an unrecognised pattern in the population that aligns with the sampling interval.
3. Stratified sampling
Stratified sampling involves random selection within predefined groups. It’s a useful method for researchers wanting to determine what aspects of a sample are highly correlated with what’s being measured. They can then decide how to subdivide (stratify) it in a way that makes sense for the research.
For example, you want to measure the height of students at a college where 80% of students are female and 20% are male. We know that gender is highly correlated with height, and if we took a simple random sample of 200 students (out of the 2,000 who attend the college), we could by chance get 200 females and not one male. This would bias our results and we would underestimate the height of students overall. Instead, we could stratify by gender and make sure that 20% of our sample (40 students) are male and 80% (160 students) are female.
Pros: Stratified sampling enhances the representation of all identified subgroups within a population, leading to more accurate results in heterogeneous populations.
Cons: This method requires accurate knowledge about the population’s stratification, and its design and execution can be more intricate than other methods.
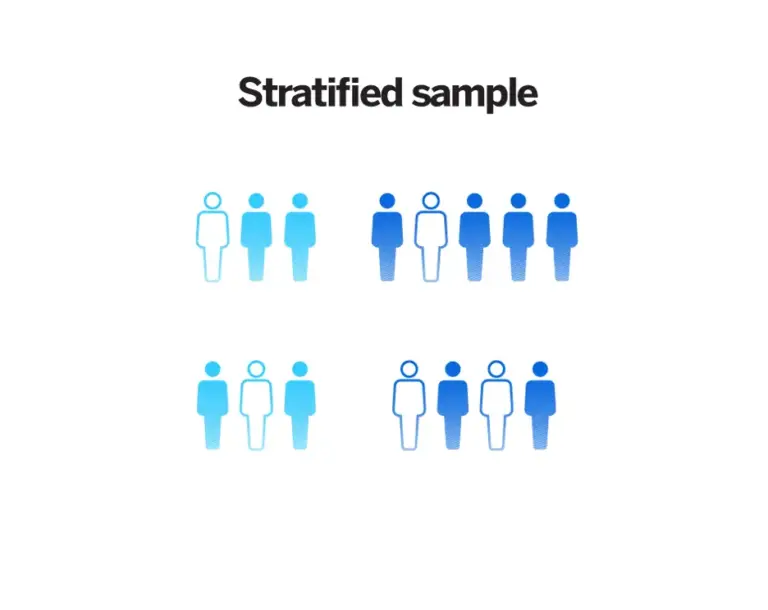
4. Cluster sampling
With cluster sampling, groups rather than individual units of the target population are selected at random for the sample. These might be pre-existing groups, such as people in certain zip codes or students belonging to an academic year.
Cluster sampling can be done by selecting the entire cluster, or in the case of two-stage cluster sampling, by randomly selecting the cluster itself, then selecting at random again within the cluster.
Pros: Cluster sampling is economically beneficial and logistically easier when dealing with vast and geographically dispersed populations.
Cons: Due to potential similarities within clusters, this method can introduce a greater sampling error compared to other methods.
Non-probability sampling methods
The non-probability sampling methodology doesn’t offer the same bias-removal benefits as probability sampling, but there are times when these types of sampling are chosen for expediency or simplicity. Here are some forms of non-probability sampling and how they work.
1. Convenience sampling
People or elements in a sample are selected on the basis of their accessibility and availability. If you are doing a research survey and you work at a university, for example, a convenience sample might consist of students or co-workers who happen to be on campus with open schedules who are willing to take your questionnaire .
This kind of sample can have value, especially if it’s done as an early or preliminary step, but significant bias will be introduced.
Pros: Convenience sampling is the most straightforward method, requiring minimal planning, making it quick to implement.
Cons: Due to its non-random nature, the method is highly susceptible to biases, and the results are often lacking in their application to the real world.
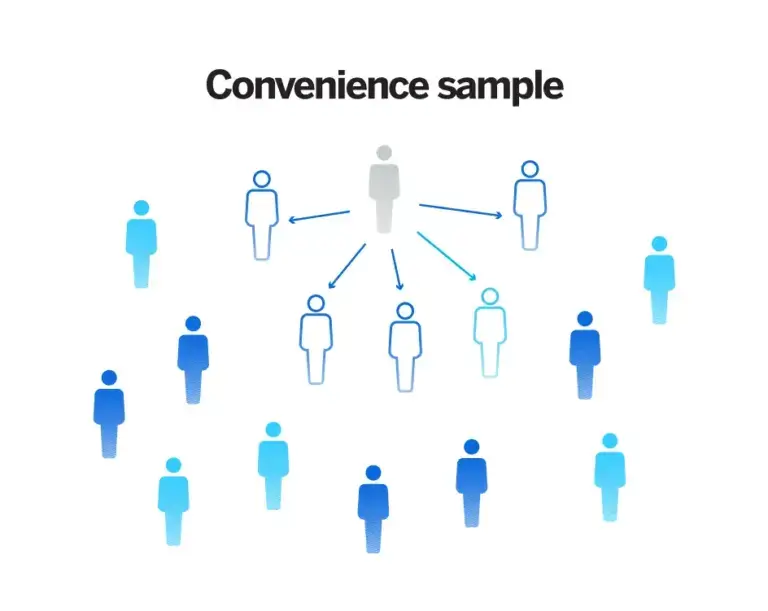
2. Quota sampling
Like the probability-based stratified sampling method, this approach aims to achieve a spread across the target population by specifying who should be recruited for a survey according to certain groups or criteria.
For example, your quota might include a certain number of males and a certain number of females. Alternatively, you might want your samples to be at a specific income level or in certain age brackets or ethnic groups.
Pros: Quota sampling ensures certain subgroups are adequately represented, making it great for when random sampling isn’t feasible but representation is necessary.
Cons: The selection within each quota is non-random and researchers’ discretion can influence the representation, which both strongly increase the risk of bias.
3. Purposive sampling
Participants for the sample are chosen consciously by researchers based on their knowledge and understanding of the research question at hand or their goals.
Also known as judgment sampling, this technique is unlikely to result in a representative sample , but it is a quick and fairly easy way to get a range of results or responses.
Pros: Purposive sampling targets specific criteria or characteristics, making it ideal for studies that require specialised participants or specific conditions.
Cons: It’s highly subjective and based on researchers’ judgment, which can introduce biases and limit the study’s real-world application.
4. Snowball or referral sampling
With this approach, people recruited to be part of a sample are asked to invite those they know to take part, who are then asked to invite their friends and family and so on. The participation radiates through a community of connected individuals like a snowball rolling downhill.
Pros: Especially useful for hard-to-reach or secretive populations, snowball sampling is effective for certain niche studies.
Cons: The method can introduce bias due to the reliance on participant referrals, and the choice of initial seeds can significantly influence the final sample.
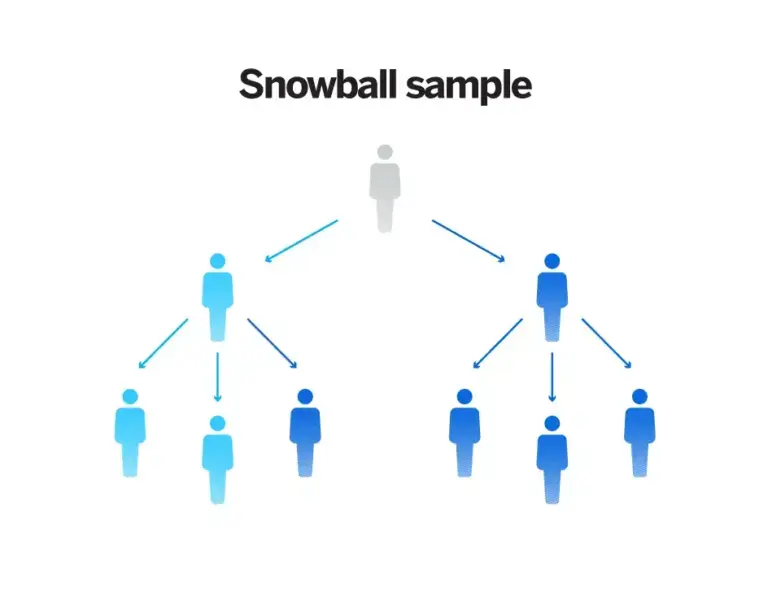
What type of sampling should I use?
Choosing the right sampling method is a pivotal aspect of any research process, but it can be a stumbling block for many.
Here’s a structured approach to guide your decision.
1) Define your research goals
If you aim to get a general sense of a larger group, simple random or stratified sampling could be your best bet. For focused insights or studying unique communities, snowball or purposive sampling might be more suitable.
2) Assess the nature of your population
The nature of the group you’re studying can guide your method. For a diverse group with different categories, stratified sampling can ensure all segments are covered. If they’re widely spread geographically , cluster sampling becomes useful. If they’re arranged in a certain sequence or order, systematic sampling might be effective.
3) Consider your constraints
Your available time, budget and ease of accessing participants matter. Convenience or quota sampling can be practical for quicker studies, but they come with some trade-offs. If reaching everyone in your desired group is challenging, snowball or purposive sampling can be more feasible.
4) Determine the reach of your findings
Decide if you want your findings to represent a much broader group. For a wider representation, methods that include everyone fairly (like probability sampling ) are a good option. For specialised insights into specific groups, non-probability sampling methods can be more suitable.
5) Get feedback
Before fully committing, discuss your chosen method with others in your field and consider a test run.
Avoid or reduce sampling errors and bias
Using a sample is a kind of short-cut. If you could ask every single person in a population to take part in your study and have each of them reply, you’d have a highly accurate (and very labor-intensive) project on your hands.
But since that’s not realistic, sampling offers a “good-enough” solution that sacrifices some accuracy for the sake of practicality and ease. How much accuracy you lose out on depends on how well you control for sampling error, non-sampling error, and bias in your survey design . Our blog post helps you to steer clear of some of these issues.
How to choose the correct sample size
Finding the best sample size for your target population is something you’ll need to do again and again, as it’s different for every study.
To make life easier, we’ve provided a sample size calculator . To use it, you need to know your:
- Population size
- Confidence level
- Margin of error (confidence interval)
If any of those terms are unfamiliar, have a look at our blog post on determining sample size for details of what they mean and how to find them.
Unlock the insights of yesterday to shape tomorrow
In the ever-evolving business landscape, relying on the most recent market research is paramount. Reflecting on 2022, brands and businesses can harness crucial insights to outmaneuver challenges and seize opportunities.
Equip yourself with this knowledge by exploring Qualtrics’ ‘2022 Market Research Global Trends’ report.
Delve into this comprehensive study to unearth:
- How businesses made sense of tricky situations in 2022
- Tips that really helped improve research results
- Steps to take your findings and put them into action
Find out how Qualtrics XM can help you conduct world-class research
Related resources
Sampling and non-sampling errors 10 min read, how to determine sample size 16 min read, convenience sampling 15 min read, non-probability sampling 17 min read, probability sampling 8 min read, stratified random sampling 13 min read, simple random sampling 10 min read, request demo.
Ready to learn more about Qualtrics?
Teach yourself statistics
Survey Sampling Methods
Sampling method refers to the way that observations are selected from a population to be in the sample for a sample survey .
Note: Your browser does not support HTML5 video. If you view this web page on a different browser (e.g., a recent version of Edge, Chrome, Firefox, or Opera), you can watch a video treatment of this lesson.
Population Parameter vs. Sample Statistic
The reason for conducting a sample survey is to estimate the value of some attribute of a population.
- Population parameter . A population parameter is the true value of a population attribute.
- Sample statistic . A sample statistic is an estimate, based on sample data, of a population parameter.
Consider this example. A public opinion pollster wants to know the percentage of voters that favor a flat-rate income tax. The actual percentage of all the voters is a population parameter. The estimate of that percentage, based on sample data, is a sample statistic.
The quality of a sample statistic (i.e., accuracy, precision, representativeness) is strongly affected by the way that sample observations are chosen; that is., by the sampling method.
Probability vs. Non-Probability Samples
As a group, sampling methods fall into one of two categories.
- Probability samples . With probability sampling methods, each population element has a known (non-zero) chance of being chosen for the sample.
- Non-probability samples . With non-probability sampling methods, we do not know the probability that each population element will be chosen, and/or we cannot be sure that each population element has a non-zero chance of being chosen.
Non-probability sampling methods offer two potential advantages - convenience and cost. The main disadvantage is that non-probability sampling methods do not allow you to estimate the extent to which sample statistics are likely to differ from population parameters. Only probability sampling methods permit that kind of analysis.
Non-Probability Sampling Methods
Two of the main types of non-probability sampling methods are voluntary samples and convenience samples.
Suppose, for example, that a news show asks viewers to participate in an online poll. This would be a voluntary sample. The sample is chosen by the viewers, not by the survey administrator.
Consider the following example. A pollster interviews shoppers at a local mall. If the mall was chosen because it was a convenient site from which to solicit survey participants and/or because it was close to the pollster's home or business, this would be a convenience sample.
Probability Sampling Methods
Some common types of probability sampling methods are simple random sampling, stratified sampling, cluster sampling, multistage sampling, and systematic random sampling. The key benefit of probability sampling methods is that they guarantee that the sample chosen is representative of the population. This ensures that the statistical conclusions will be valid.
- The population consists of N objects.
- The sample consists of n objects.
- If all possible samples of n objects are equally likely to occur, the sampling method is called simple random sampling.
There are many ways to obtain a simple random sample. One way would be the lottery method. Each of the N population members is assigned a unique number. The numbers are placed in a bowl and thoroughly mixed. Then, a blind-folded researcher selects n numbers. Population members having the selected numbers are included in the sample.
As a example, suppose we conduct a national survey. We might divide the population into groups or strata, based on geography - north, east, south, and west. Then, within each stratum, we might randomly select survey subjects.
Note the difference between cluster sampling and stratified sampling. With stratified sampling, the sample includes elements from each stratum. With cluster sampling, in contrast, the sample includes elements only from sampled clusters.
For example, in Stage 1, we might use cluster sampling to choose clusters from a population. Then, in Stage 2, we might use simple random sampling to select a subset of elements from each chosen cluster for the final sample.
This method is different from simple random sampling since every possible sample of n elements is not equally likely.
Test Your Understanding
An auto analyst is conducting a satisfaction survey, sampling from a list of 10,000 new car buyers. The list includes 2,500 Ford buyers, 2,500 GM buyers, 2,500 Honda buyers, and 2,500 Toyota buyers. The analyst selects a sample of 400 car buyers, by randomly sampling 100 buyers of each brand.
Is this an example of a simple random sample?
(A) Yes, because each buyer in the sample was randomly sampled. (B) Yes, because each buyer in the sample had an equal chance of being sampled. (C) Yes, because car buyers of every brand were equally represented in the sample. (D) No, because every possible 400-buyer sample did not have an equal chance of being chosen. (E) No, because the population consisted of purchasers of four different brands of car.
The correct answer is (D). A simple random sample requires that every sample of size n (in this problem, n is equal to 400) has an equal chance of being selected. In this problem, there was a 100 percent chance that the sample would include 100 purchasers of each brand of car. There was zero percent chance that the sample would include, for example, 99 Ford buyers, 101 Honda buyers, 100 Toyota buyers, and 100 GM buyers. Thus, all possible samples of size 400 did not have an equal chance of being selected; so this cannot be a simple random sample.
The fact that each buyer in the sample was randomly sampled is a necessary condition for a simple random sample, but it is not sufficient. Similarly, the fact that each buyer in the sample had an equal chance of being selected is characteristic of a simple random sample, but it is not sufficient. The sampling method in this problem used random sampling and gave each buyer an equal chance of being selected; but the sampling method was actually stratified random sampling .
The fact that car buyers of every brand were equally represented in the sample is irrelevant to whether the sampling method was simple random sampling. Similarly, the fact that population consisted of buyers of different car brands is irrelevant.

An official website of the United States government
The .gov means it’s official. Federal government websites often end in .gov or .mil. Before sharing sensitive information, make sure you’re on a federal government site.
The site is secure. The https:// ensures that you are connecting to the official website and that any information you provide is encrypted and transmitted securely.
- Publications
- Account settings
Preview improvements coming to the PMC website in October 2024. Learn More or Try it out now .
- Advanced Search
- Journal List
- J Adv Pract Oncol
- v.6(2); Mar-Apr 2015

Understanding and Evaluating Survey Research
A variety of methodologic approaches exist for individuals interested in conducting research. Selection of a research approach depends on a number of factors, including the purpose of the research, the type of research questions to be answered, and the availability of resources. The purpose of this article is to describe survey research as one approach to the conduct of research so that the reader can critically evaluate the appropriateness of the conclusions from studies employing survey research.
SURVEY RESEARCH
Survey research is defined as "the collection of information from a sample of individuals through their responses to questions" ( Check & Schutt, 2012, p. 160 ). This type of research allows for a variety of methods to recruit participants, collect data, and utilize various methods of instrumentation. Survey research can use quantitative research strategies (e.g., using questionnaires with numerically rated items), qualitative research strategies (e.g., using open-ended questions), or both strategies (i.e., mixed methods). As it is often used to describe and explore human behavior, surveys are therefore frequently used in social and psychological research ( Singleton & Straits, 2009 ).
Information has been obtained from individuals and groups through the use of survey research for decades. It can range from asking a few targeted questions of individuals on a street corner to obtain information related to behaviors and preferences, to a more rigorous study using multiple valid and reliable instruments. Common examples of less rigorous surveys include marketing or political surveys of consumer patterns and public opinion polls.
Survey research has historically included large population-based data collection. The primary purpose of this type of survey research was to obtain information describing characteristics of a large sample of individuals of interest relatively quickly. Large census surveys obtaining information reflecting demographic and personal characteristics and consumer feedback surveys are prime examples. These surveys were often provided through the mail and were intended to describe demographic characteristics of individuals or obtain opinions on which to base programs or products for a population or group.
More recently, survey research has developed into a rigorous approach to research, with scientifically tested strategies detailing who to include (representative sample), what and how to distribute (survey method), and when to initiate the survey and follow up with nonresponders (reducing nonresponse error), in order to ensure a high-quality research process and outcome. Currently, the term "survey" can reflect a range of research aims, sampling and recruitment strategies, data collection instruments, and methods of survey administration.
Given this range of options in the conduct of survey research, it is imperative for the consumer/reader of survey research to understand the potential for bias in survey research as well as the tested techniques for reducing bias, in order to draw appropriate conclusions about the information reported in this manner. Common types of error in research, along with the sources of error and strategies for reducing error as described throughout this article, are summarized in the Table .

Sources of Error in Survey Research and Strategies to Reduce Error
The goal of sampling strategies in survey research is to obtain a sufficient sample that is representative of the population of interest. It is often not feasible to collect data from an entire population of interest (e.g., all individuals with lung cancer); therefore, a subset of the population or sample is used to estimate the population responses (e.g., individuals with lung cancer currently receiving treatment). A large random sample increases the likelihood that the responses from the sample will accurately reflect the entire population. In order to accurately draw conclusions about the population, the sample must include individuals with characteristics similar to the population.
It is therefore necessary to correctly identify the population of interest (e.g., individuals with lung cancer currently receiving treatment vs. all individuals with lung cancer). The sample will ideally include individuals who reflect the intended population in terms of all characteristics of the population (e.g., sex, socioeconomic characteristics, symptom experience) and contain a similar distribution of individuals with those characteristics. As discussed by Mady Stovall beginning on page 162, Fujimori et al. ( 2014 ), for example, were interested in the population of oncologists. The authors obtained a sample of oncologists from two hospitals in Japan. These participants may or may not have similar characteristics to all oncologists in Japan.
Participant recruitment strategies can affect the adequacy and representativeness of the sample obtained. Using diverse recruitment strategies can help improve the size of the sample and help ensure adequate coverage of the intended population. For example, if a survey researcher intends to obtain a sample of individuals with breast cancer representative of all individuals with breast cancer in the United States, the researcher would want to use recruitment strategies that would recruit both women and men, individuals from rural and urban settings, individuals receiving and not receiving active treatment, and so on. Because of the difficulty in obtaining samples representative of a large population, researchers may focus the population of interest to a subset of individuals (e.g., women with stage III or IV breast cancer). Large census surveys require extremely large samples to adequately represent the characteristics of the population because they are intended to represent the entire population.
DATA COLLECTION METHODS
Survey research may use a variety of data collection methods with the most common being questionnaires and interviews. Questionnaires may be self-administered or administered by a professional, may be administered individually or in a group, and typically include a series of items reflecting the research aims. Questionnaires may include demographic questions in addition to valid and reliable research instruments ( Costanzo, Stawski, Ryff, Coe, & Almeida, 2012 ; DuBenske et al., 2014 ; Ponto, Ellington, Mellon, & Beck, 2010 ). It is helpful to the reader when authors describe the contents of the survey questionnaire so that the reader can interpret and evaluate the potential for errors of validity (e.g., items or instruments that do not measure what they are intended to measure) and reliability (e.g., items or instruments that do not measure a construct consistently). Helpful examples of articles that describe the survey instruments exist in the literature ( Buerhaus et al., 2012 ).
Questionnaires may be in paper form and mailed to participants, delivered in an electronic format via email or an Internet-based program such as SurveyMonkey, or a combination of both, giving the participant the option to choose which method is preferred ( Ponto et al., 2010 ). Using a combination of methods of survey administration can help to ensure better sample coverage (i.e., all individuals in the population having a chance of inclusion in the sample) therefore reducing coverage error ( Dillman, Smyth, & Christian, 2014 ; Singleton & Straits, 2009 ). For example, if a researcher were to only use an Internet-delivered questionnaire, individuals without access to a computer would be excluded from participation. Self-administered mailed, group, or Internet-based questionnaires are relatively low cost and practical for a large sample ( Check & Schutt, 2012 ).
Dillman et al. ( 2014 ) have described and tested a tailored design method for survey research. Improving the visual appeal and graphics of surveys by using a font size appropriate for the respondents, ordering items logically without creating unintended response bias, and arranging items clearly on each page can increase the response rate to electronic questionnaires. Attending to these and other issues in electronic questionnaires can help reduce measurement error (i.e., lack of validity or reliability) and help ensure a better response rate.
Conducting interviews is another approach to data collection used in survey research. Interviews may be conducted by phone, computer, or in person and have the benefit of visually identifying the nonverbal response(s) of the interviewee and subsequently being able to clarify the intended question. An interviewer can use probing comments to obtain more information about a question or topic and can request clarification of an unclear response ( Singleton & Straits, 2009 ). Interviews can be costly and time intensive, and therefore are relatively impractical for large samples.
Some authors advocate for using mixed methods for survey research when no one method is adequate to address the planned research aims, to reduce the potential for measurement and non-response error, and to better tailor the study methods to the intended sample ( Dillman et al., 2014 ; Singleton & Straits, 2009 ). For example, a mixed methods survey research approach may begin with distributing a questionnaire and following up with telephone interviews to clarify unclear survey responses ( Singleton & Straits, 2009 ). Mixed methods might also be used when visual or auditory deficits preclude an individual from completing a questionnaire or participating in an interview.
FUJIMORI ET AL.: SURVEY RESEARCH
Fujimori et al. ( 2014 ) described the use of survey research in a study of the effect of communication skills training for oncologists on oncologist and patient outcomes (e.g., oncologist’s performance and confidence and patient’s distress, satisfaction, and trust). A sample of 30 oncologists from two hospitals was obtained and though the authors provided a power analysis concluding an adequate number of oncologist participants to detect differences between baseline and follow-up scores, the conclusions of the study may not be generalizable to a broader population of oncologists. Oncologists were randomized to either an intervention group (i.e., communication skills training) or a control group (i.e., no training).
Fujimori et al. ( 2014 ) chose a quantitative approach to collect data from oncologist and patient participants regarding the study outcome variables. Self-report numeric ratings were used to measure oncologist confidence and patient distress, satisfaction, and trust. Oncologist confidence was measured using two instruments each using 10-point Likert rating scales. The Hospital Anxiety and Depression Scale (HADS) was used to measure patient distress and has demonstrated validity and reliability in a number of populations including individuals with cancer ( Bjelland, Dahl, Haug, & Neckelmann, 2002 ). Patient satisfaction and trust were measured using 0 to 10 numeric rating scales. Numeric observer ratings were used to measure oncologist performance of communication skills based on a videotaped interaction with a standardized patient. Participants completed the same questionnaires at baseline and follow-up.
The authors clearly describe what data were collected from all participants. Providing additional information about the manner in which questionnaires were distributed (i.e., electronic, mail), the setting in which data were collected (e.g., home, clinic), and the design of the survey instruments (e.g., visual appeal, format, content, arrangement of items) would assist the reader in drawing conclusions about the potential for measurement and nonresponse error. The authors describe conducting a follow-up phone call or mail inquiry for nonresponders, using the Dillman et al. ( 2014 ) tailored design for survey research follow-up may have reduced nonresponse error.
CONCLUSIONS
Survey research is a useful and legitimate approach to research that has clear benefits in helping to describe and explore variables and constructs of interest. Survey research, like all research, has the potential for a variety of sources of error, but several strategies exist to reduce the potential for error. Advanced practitioners aware of the potential sources of error and strategies to improve survey research can better determine how and whether the conclusions from a survey research study apply to practice.
The author has no potential conflicts of interest to disclose.
Survey Sampling and Research: Definition and Methods
Design of Experiments > Survey Sampling
- Survey Sampling
- Survey Research
What is Survey Sampling?
Survey sampling is selecting members from a target population to be in a sample for a sample survey. Usually the survey is some type of questionnaire (i.e. in-person, phone or internet survey).
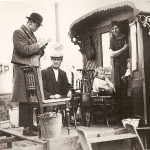
The three parts of survey sampling are:
- Sample selection.
- Data Collection : collecting the data through mail, phone, or in-person.
- Estimation: using estimators from the collected data to make inferences about the population as a whole.
Survey Sampling: Sample Selection
Sample selection for survey samples fall into two main types:
- Probability-based samples , which chooses members based on a known probability. This uses random selection methods like simple random sampling or systematic sampling . For a list of probability-based sampling methods, see this article: Probability Sampling .
- Non-probability samples , where the probability of choosing a member cannot be calculated. Instead, non-random selection methods use the researcher’s judgment, proximity of subjects, or other non-random factor. For a list of non-probability sampling methods, see this article: Non-Probability Sampling .
Usually, probability-based samples have preference over non-probability samples. Most larger organizations and institutions publish guidelines for the types of survey sampling permitted. For example, t his Whitehouse document titled “Lists of Standards for Statistical Surveys states that:
Agencies must develop a survey design…selecting samples using generally accepted statistical methods (e.g., probabilistic methods that can provide estimates of sampling error ). You should justify any use of nonprobability sampling methods (e.g., cut-off or model-based samples) and you should also measure estimation error.
The “estimation error” usually refers to being able to calculate a confidence interval and margin of error .
The Kish Grid is one way to select survey participants. It assigns equal probabilities to each person and so avoids selection bias .
What is Survey Research?
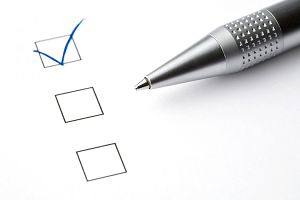
- Questionnaires : written questions (on paper or on a computer), which can include open ended questions, closed ended questions, multiple choice questions, or questions that require the respondent to rate something on a scale (i.e. 1 to 10).
- Interviews : oral questions (in person or via a phone or computer) — closed-ended or open-ended, multiple choice or on a scale.
- Surveys : brief interviews about a specific topic. Like questionnaires and interviews, these can also come in a variety of question formats.
Advantages of Survey Research:
- It is an effective tool for collecting data from a wide variety of people about a wide variety of topics.
- It’s versatile and can provide a deeper understanding of just about any issue.
- It can be both time and cost efficient. Many questions are asked without increasing cost or time factors. If well-designed, survey research can also be relatively low cost.
- It is sometimes the only available tool for obtaining data from a large population.
Disadvantages of Survey Research
- Response rates can be very low, especially for mail surveys.
- Misleading or difficult to understand questions can taint results. Language barriers can also cause issues with responses.
- Personal interviews can be expensive, especially if the sample is spread across a wide geographic area. Cost can also be a factor with the postage involved in sending out mass-mail surveys. Telephone surveys can also quickly become cost prohibitive when factoring in the cost of training staff and payroll.
Surveys have been in popular use for decades. The odds are, if you want to find out something about a population, someone has performed survey research for that topic before you. Building on someone else’s survey research design can help you avoid many of the pitfalls of survey research, like irrelevant questions or omitting questions that are crucial to understanding the topic.
Brick, J. The Future of Survey Sampling. Public Opinion Quarterly. Available online here . Schaeffer, R. (2011). Elementary Survey Sampling 7th Edition . Cengage Learning.
7.3 Conducting Surveys
Learning objectives.
- Explain the difference between probability and non-probability sampling, and describe the major types of probability sampling.
- Define sampling bias in general and non-response bias in particular. List some techniques that can be used to increase the response rate and reduce non-response bias.
- List the four major ways to conduct a survey along with some pros and cons of each.
In this section, we consider how to go about conducting a survey. We first consider the issue of sampling, followed by some different methods of actually collecting survey data.
Essentially all psychological research involves sampling—selecting a sample to study from the population of interest. Sampling falls into two broad categories. Probability sampling occurs when the researcher can specify the probability that each member of the population will be selected for the sample. Non-probability sampling occurs when the researcher cannot specify these probabilities. Most psychological research involves non-probability sampling. Convenience sampling—studying individuals who happen to be nearby and willing to participate—is a very common form of non-probability sampling used in psychological research.
Survey researchers, however, are much more likely to use some form of probability sampling. This tendency is because the goal of most survey research is to make accurate estimates about what is true in a particular population, and these estimates are most accurate when based on a probability sample. For example, it is important for survey researchers to base their estimates of election outcomes—which are often decided by only a few percentage points—on probability samples of likely registered voters.
Compared with non-probability sampling, probability sampling requires a very clear specification of the population, which of course depends on the research questions to be answered. The population might be all registered voters in Washington State , all American consumers who have purchased a car in the past year, women in the Seattle over 40 years old who have received a mammogram in the past decade, or all the alumni of a particular university. Once the population has been specified, probability sampling requires a sampling frame . This sampling frame is essentially a list of all the members of the population from which to select the respondents. Sampling frames can come from a variety of sources, including telephone directories, lists of registered voters, and hospital or insurance records. In some cases, a map can serve as a sampling frame, allowing for the selection of cities, streets, or households.
There are a variety of different probability sampling methods. Simple random sampling is done in such a way that each individual in the population has an equal probability of being selected for the sample. This type of sampling could involve putting the names of all individuals in the sampling frame into a hat, mixing them up, and then drawing out the number needed for the sample. Given that most sampling frames take the form of computer files, random sampling is more likely to involve computerized sorting or selection of respondents. A common approach in telephone surveys is random-digit dialing, in which a computer randomly generates phone numbers from among the possible phone numbers within a given geographic area.
A common alternative to simple random sampling is stratified random sampling , in which the population is divided into different subgroups or “strata” (usually based on demographic characteristics) and then a random sample is taken from each “stratum.” Proportionate stratified random sampling can be used to select a sample in which the proportion of respondents in each of various subgroups matches the proportion in the population. For example, because about 15.3 % of the Canadian population is Asian , stratified random sampling can be used to ensure that a survey of 1,000 Canadian adults includes about 153 Asian Canadian respondents. Disproportionate stratified random sampling can also be used to sample extra respondents from particularly small subgroups—allowing valid conclusions to be drawn about those subgroups. For example, because Black Canadians make up a fairly small percentage of the Canadian population (about 2.9 %), a simple random sample of 1,000 Canadian adults might include too few Black Canadians to draw any conclusions about them as distinct from any other subgroup. If this is important to the research question, however, then disproportionate stratified random sampling could be used to ensure that enough Black Canadian respondents are included in the sample to draw valid conclusions about Black Canadians as a whole.
Yet another type of probability sampling is cluster sampling , in which larger clusters of individuals are randomly sampled and then individuals within each cluster are randomly sampled. This is the only probability sampling method that does not require a sampling frame. For example, to select a sample of small-town residents in Washington , a researcher might randomly select several small towns and then randomly select several individuals within each town. Cluster sampling is especially useful for surveys that involve face-to-face interviewing because it minimizes the amount of traveling that the interviewers must do. For example, instead of traveling to 200 small towns to interview 200 residents, a research team could travel to 10 small towns and interview 20 residents of each. The National Comorbidity Survey was done using a form of cluster sampling.
How large does a survey sample need to be? In general, this estimate depends on two factors. One is the level of confidence in the result that the researcher wants. The larger the sample, the closer any statistic based on that sample will tend to be to the corresponding value in the population. The other factor is the budget of the study. Larger samples provide greater confidence, but they take more time, effort, and money to obtain. Taking these two factors into account, most survey research uses sample sizes that range from about 100 to about 1,000.
Sample Size and Population Size
Why is a sample of 1,000 considered to be adequate for most survey research—even when the population is much larger than that? Consider, for example, that a sample of only 1,000 registered voters is generally considered a good sample of the roughly 25 million registered voters in the Canadian population—even though it includes only about 0.00004% of the population! The answer is a bit surprising.
One part of the answer is that a statistic based on a larger sample will tend to be closer to the population value and that this can be characterized mathematically. Imagine, for example, that in a sample of registered voters, exactly 50% say they intend to vote for the incumbent. If there are 100 voters in this sample, then there is a 95% chance that the true percentage in the population is between 40 and 60. But if there are 1,000 voters in the sample, then there is a 95% chance that the true percentage in the population is between 47 and 53. Although this “95% confidence interval” continues to shrink as the sample size increases, it does so at a slower rate. For example, if there are 2,000 voters in the sample, then this reduction only reduces the 95% confidence interval to 48 to 52. In many situations, the small increase in confidence beyond a sample size of 1,000 is not considered to be worth the additional time, effort, and money.
Another part of the answer—and perhaps the more surprising part—is that confidence intervals depend only on the size of the sample and not on the size of the population. So a sample of 1,000 would produce a 95% confidence interval of 47 to 53 regardless of whether the population size was a hundred thousand, a million, or a hundred million.
Sampling Bias
Probability sampling was developed in large part to address the issue of sampling bias. Sampling bias occurs when a sample is selected in such a way that it is not representative of the entire population and therefore produces inaccurate results. This bias was the reason that the Literary Digest straw poll was so far off in its prediction of the 1936 presidential election. The mailing lists used came largely from telephone directories and lists of registered automobile owners, which over-represented wealthier people, who were more likely to vote for Landon. Gallup was successful because he knew about this bias and found ways to sample less wealthy people as well.
There is one form of sampling bias that even careful random sampling is subject to. It is almost never the case that everyone selected for the sample actually responds to the survey. Some may have died or moved away, and others may decline to participate because they are too busy, are not interested in the survey topic, or do not participate in surveys on principle. If these survey non-responders differ from survey responders in systematic ways, then this difference can produce non-response bias . For example, in a mail survey on alcohol consumption, researcher Vivienne Lahaut and colleagues found that only about half the sample responded after the initial contact and two follow-up reminders (Lahaut, Jansen, van de Mheen, & Garretsen, 2002) [1] . The danger here is that the half who responded might have different patterns of alcohol consumption than the half who did not, which could lead to inaccurate conclusions on the part of the researchers. So to test for non-response bias, the researchers later made unannounced visits to the homes of a subset of the non-responders—coming back up to five times if they did not find them at home. They found that the original non-responders included an especially high proportion of abstainers (nondrinkers), which meant that their estimates of alcohol consumption based only on the original responders were too high.
Although there are methods for statistically correcting for non-response bias, they are based on assumptions about the non-responders—for example, that they are more similar to late responders than to early responders—which may not be correct. For this reason, the best approach to minimizing non-response bias is to minimize the number of non-responders—that is, to maximize the response rate. There is a large research literature on the factors that affect survey response rates (Groves et al., 2004) [2] . In general, in-person interviews have the highest response rates, followed by telephone surveys, and then mail and Internet surveys. Among the other factors that increase response rates are sending potential respondents a short pre-notification message informing them that they will be asked to participate in a survey in the near future and sending simple follow-up reminders to non-responders after a few weeks. The perceived length and complexity of the survey can also make a difference, which is why it is important to keep survey questionnaires as short, simple, and on topic as possible. Finally, offering an incentive—especially cash—is a reliable way to increase response rates. However, ethically, there are limits to offering incentives that may be so large as to be considered coercive.
Conducting the Survey
The four main ways to conduct surveys are through in-person interviews, by telephone, through the mail, and over the internet. As with other aspects of survey design, the choice depends on both the researcher’s goals and the budget. In-person interviews have the highest response rates and provide the closest personal contact with respondents. Personal contact can be important, for example, when the interviewer must see and make judgments about respondents, as is the case with some mental health interviews. But in-person interviewing is by far the most costly approach. Telephone surveys have lower response rates and still provide some personal contact with respondents. They can also be costly but are generally less so than in-person interviews. Traditionally, telephone directories have provided fairly comprehensive sampling frames. However, this trend is less true today as more people choose to only have cell phones and do not install land lines that would be included in telephone directories. Mail surveys are less costly still but generally have even lower response rates—making them most susceptible to non-response bias.
Not surprisingly, internet surveys are becoming more common. They are increasingly easy to construct and use (see “Online Survey Creation”). Although initial contact can be made by mail with a link provided to the survey, this approach does not necessarily produce higher response rates than an ordinary mail survey. A better approach is to make initial contact by email with a link directly to the survey. This approach can work well when the population consists of the members of an organization who have known email addresses and regularly use them (e.g., a university community). For other populations, it can be difficult or impossible to find a comprehensive list of email addresses to serve as a sampling frame. Alternatively, a request to participate in the survey with a link to it can be posted on websites known to be visited by members of the population. But again it is very difficult to get anything approaching a random sample this way because the members of the population who visit the websites are likely to be different from the population as a whole. However, internet survey methods are in rapid development. Because of their low cost, and because more people are online than ever before, internet surveys are likely to become the dominant approach to survey data collection in the near future.
Finally, it is important to note that some of the concerns that people have about collecting data online (e.g., that internet-based findings differ from those obtained with other methods) have been found to be myths. Table 7.3 (adapted from Gosling, Vazire, Srivastava, & John, 2004) [3] addresses three such preconceptions about data collected in web-based studies:
Table 7.3 Some Preconceptions and Findings Pertaining to Web-based Studies
Internet samples are not demographically diverse | Internet samples are more diverse than traditional samples in many domains, although they are not completely representative of the population |
Internet samples are maladjusted, socially isolated, or depressed | Internet users do not differs from nonusers on markers of adjustment and depression |
Internet-based findings differ from those obtained with other methods | Evidence so far suggests that internet-based findings are consistent with findings based on traditional methods (e.g., on self-esteem, personality), but more data are needed. |
Online Survey Creation
There are now several online tools for creating online questionnaires. After a questionnaire is created, a link to it can then be emailed to potential respondents or embedded in a web page. The following websites are among those that offer free accounts. Although the free accounts limit the number of questionnaire items and the number of respondents, they can be useful for doing small-scale surveys and for practicing the principles of good questionnaire construction. A small note of caution: the data from US survey software are held on US servers, and are subject to be seized as granted through the Patriot Act. To avoid infringing on any rights, the following is a list of online survey sites that are hosted in Canada:
- Fluid Surveys— http://fluidsurveys.com/
- Simple Survey— http://www.simplesurvey.com/
- Lime Survey— https://www.limesurvey.org
There are also survey sites hosted in other countries outside of North America.
Another new tool for survey researchers is Mechanical Turk (MTurk) created by Amazon.com https://www.mturk.com Originally created for simple usability testing, MTurk has a database of over 500,000 workers from over 190 countries [4] . You can put simple tasks (for example, different question wording to test your survey items), set parameters as your sample frame dictates and deploy your experiment at a very low cost (for example, a few cents for less than 5 minutes). MTurk has been lauded as an inexpensive way to gather high-quality data (Buhrmester, Kwang, & Gosling, 2011) [5] .
Key Takeaways
- Survey research usually involves probability sampling, in which each member of the population has a known probability of being selected for the sample. Types of probability sampling include simple random sampling, stratified random sampling, and cluster sampling.
- Sampling bias occurs when a sample is selected in such a way that it is not representative of the population and therefore produces inaccurate results. The most pervasive form of sampling bias is non-response bias, which occurs when people who do not respond to the survey differ in important ways from people who do respond. The best way to minimize non-response bias is to maximize the response rate by prenotifying respondents, sending them reminders, constructing questionnaires that are short and easy to complete, and offering incentives.
- Surveys can be conducted in person, by telephone, through the mail, and on the internet. In-person interviewing has the highest response rates but is the most expensive. Mail and internet surveys are less expensive but have much lower response rates. Internet surveys are likely to become the dominant approach because of their low cost.
- students at a particular university
- adults living in the state of Washington
- households in Pullman, Washington
- people with low self-esteem
- Practice: Use one of the online survey creation tools to create a 10-item survey questionnaire on a topic of your choice.
- Lahaut, V. M. H. C. J., Jansen, H. A. M., van de Mheen, D., & Garretsen, H. F. L. (2002). Non-response bias in a sample survey on alcohol consumption. Alcohol and Alcoholism, 37 , 256–260. ↵
- Groves, R. M., Fowler, F. J., Couper, M. P., Lepkowski, J. M., Singer, E., & Tourangeau, R. (2004). Survey methodology . Hoboken, NJ: Wiley. ↵
- Gosling, S. D., Vazire, S., Srivastava, S., & John, O. P. (2004). Should we trust web-based studies? A comparative analysis of six preconceptions about internet questionnaires. American Psychologist, 59 (2), 93-104. ↵
- https://forums.aws.amazon.com/thread.jspa?threadID=58891 ↵
- Buhrmester, M., Kwang, T., & Gosling, S.D. (2011). Amazon’s Mechanical Turk: A new source of inexpensive, yet high quality, data? Perspectives on Psychological Science, 6 (1), 3-5. ↵

Share This Book
- Increase Font Size
- Technical Support
- Technical Papers
- Knowledge Base
- Question Library
Call our friendly, no-pressure support team.
Sampling Methods in Survey Research: Definition, Types, Methods, and More
Table of Contents
What Is Sampling? Why Is Sampling Important?
Sampling is the backbone for primary data collection and analysis in marketing research and the social sciences. It involves selecting a subset of individuals from a larger population to participate in a study, with the aim of making valid inferences about the entire population without needing to survey every individual within that population. Sampling makes research both practical (cost and time effective) and feasible.
The key to effective sampling lies in the concept of a representative sample —a subset that fairly mirrors the diverse characteristics of the population it is drawn from. Achieving this ensures the data collection and data analysis phases yield accurate and meaningful insights, minimizing the risk of biased (systematically wrong) or irrelevant findings.
Get Started with Your Survey Research Today!
Ready for your next research study? Get access to our free survey research tool. In just a few minutes, you can create powerful surveys with our easy-to-use interface.
Start Survey Research for Free or Request a Product Tour
“Dewey Beats Truman” USA Presidential Election 1948 Example
The classic example showing how poor sampling procedures can lead to erroneous conclusions about the population is had in the oft-cited “Dewey Beats Truman” false headline newspaper article on November 3, 1948, the day after Truman had actually beaten Dewey to win the presidency of the United States of America.
How did the newspaper get it wrong? Conventional wisdom, based on various polls with large sample sizes, predicted that Dewey would win. Facing the printing deadline and anxious to be able to report the winner of the election the next morning, they decided to print the expected results prior to the election results being finalized. “Dewey Defeats Truman” the headline trumpeted; but they had gotten it wrong.
(President Harry Truman gleefully showing the erroneous headline) Image Credit: Byron Rollins, The Washington Post
There were multiple reasons that the polls didn’t get it right. Prominent among those was that telephone polls in 1948 led to a biased sample of respondents who tended to favor Dewey, because telephones were something more commonly found in well-to-do households. Well-to-do households were more likely to vote for Dewey. The sample used to project that Dewey would beat Truman, though substantial, was nonetheless biased.
Key Sampling Definitions: Population vs. Sample
Understanding the distinction between a population and sample is crucial in sampling:
- Sampling : The process of selecting a sample from a population.
- Population : The entire group of individuals or entities relevant to a particular research study, from which a sample is drawn.
- Sample : A subset of the population selected for participation in the research study.
- Sample Frame : A list or database from which the sample is drawn, ideally encompassing the entire population.
These foundational concepts lay the groundwork for understanding the various sampling methods and their applications in research, guiding the choice of method based on the research objectives and constraints.
Types of Sampling Methods in Research
Sampling methods are broadly categorized into probability and non-probability sampling , each with distinct approaches and implications for research accuracy and validity.
Probability Sampling Method
Probability sampling , the first of two primary sampling methods, ensures every member of the population has a known and non-zero chance of being selected. This method fosters objectivity and minimizes sampling bias, enhancing the representativeness of the sample.
1. Simple Random Sampling (SRS) Method
Simple random sampling (SRS) epitomizes equal chance selection, where each population member has an identical probability of being chosen. Its advantages include simplicity and reduced bias, while disadvantages may involve logistical challenges in large populations. Theory-based classroom examples involve drawing balls of different colors out of a cylinder where the balls have been thoroughly mixed. A real-world application is selecting respondents for a customer satisfaction survey from a database of all customers.
2. Systematic Sampling Method
Systemic sampling selection is made at regular intervals from an ordered list, combining efficiency with a reduced risk of selection bias. However, its systematic nature can introduce bias if the list has an underlying pattern. An application example is selecting every 100th theme park visitor coming through the turnstiles to provide feedback.
3. Stratified Sampling Method
Stratified sampling involves dividing the population into subgroups based on identifiable characteristics and randomly sampling from each. It enhances representation but is more complex to implement. A practical use case is conducting a health survey across different age groups to ensure all are adequately represented.
Stratified sampling reduces the likelihood of obtaining an unlucky sample that is not representative. For example, if we sampled 30 respondents, it’s possible you’ll obtain only a very few male respondents. If we divided the sample frame into males and females and drew 15 females and 15 males, we could ensure good representation with respect to gender. In practice, stratified sampling typically involves consideration of more than one demographic (or otherwise known) characteristic of the sample elements.
4. Cluster Sampling Method
Cluster sampling selection occurs by dividing the population into clusters and randomly selecting entire clusters. This approach is cost-effective, especially for geographically dispersed populations, but can increase sampling error. It's sometimes used in field surveys where researchers select specific neighborhoods or schools to study.
Non-Probability Sampling Method
Non-probability sampling, the second primary sampling method, does not provide every individual with a known and non-zero chance of selection, often used when probability sampling is impractical or unnecessary.
1. Convenience Sampling Method
Convenience sampling selection is based on ease of access, favoring quick and easy data collection despite inherent bias risks. Examples related to survey research involve placing a link to take a survey on social media, or using Mechanical Turk (Amazon’s crowdsourcing marketplace) where people go to do a variety of tasks, such as completing surveys, to make money.
Although we would like to think otherwise, many sample sources we rely on for marketing and social survey research purposes, including online panel samples, are in reality convenience samples.
2. Quota Sampling Method
Quota sampling involves selecting individuals to fill a "quota" such as 500 smokers in a non-random manner, such as through convenience sampling approaches.
3. Judgement Sampling Method
In judgement sampling, researchers select participants based on specific criteria and judgment, useful for targeted studies but susceptible to subjective bias. For example, selecting experts in a field for in-depth interviews.
4. Snowball Sampling Method
For hard-to-reach populations, snowball sampling relies on referrals from initial subjects to recruit further participants. While it can be effective for niche studies, it can lead to bias. A case in point is researching a rare medical condition where patients might know and might be able to refer other patients. (Snowball sampling is rarely used in practice.)
Each sampling method has its context of application, influenced by the study's objectives, the population's nature, and logistical considerations. Choosing the appropriate method is important for research validity and reliability.
Get Started with Market Research Today!
Ready for your next market research study? Get access to our free survey research tool. In just a few minutes, you can create powerful surveys with our easy-to-use interface.
Start Market Research for Free or Request a Product Tour
Differences Between Probability and Non-Probability Sampling Methods
Each sampling method has its own set of advantages and limitations, influencing the accuracy, applicability, and generalizability of research findings. Here's a summary exploration of the differences:
|
|
|
| Random selection, every member has a known and non-zero chance of being selected. | Non-random selection, not all members have a known and non-zero chance of being selected. |
| Simple random sampling, systematic sampling, stratified sampling, cluster sampling. | Convenience sampling, quota sampling, judgement sampling, snowball sampling. |
| Generally results in a representative sample. | Usually does not produce a representative sample. |
| Appropriate for inferential statistics and studies requiring generalization. | Used in exploratory research, qualitative studies, or specific subgroup focuses. |
| Reduces sampling bias, allows calculation of sampling error, more reliable. | Easier and less expensive to implement, useful for inaccessible populations. |
| More time-consuming and costly, requires comprehensive population list. | May introduce bias, limits generalization of findings to the entire population. |
Choosing the Right Sampling Method in Research
Selecting the appropriate sampling method can significantly affect the validity of your research findings. This choice should be guided by a structured decision-making process, taking into account several key considerations:
- Research Goals : Begin by clarifying whether your study seeks to gain general insights applicable to the broader population or if it focuses on specific segments or behaviors. This determination will influence whether a probability or non-probability sampling method is more appropriate.
- Nature of the Population : Consider the diversity, geographic distribution, and accessibility of your target population. Probability sampling methods are preferable for a diverse and widespread population to ensure representativeness, while non-probability methods might suffice for more homogeneous or accessible groups.
- Constraints : Practical constraints such as time, budget, and resources available for your study can significantly affect your sampling strategy. Non-probability methods often require less time and resources, making them suitable for studies with tight constraints.
- Reach of Findings : Decide on the importance of the study’s findings being representative of the broader population. Studies aiming for broad applicability should lean towards probability methods to ensure a representative sample.
- Feedback : Engaging with peers or experts in your field can provide valuable insights and feedback on your chosen sampling method. Pilot tests or preliminary studies can also help validate your approach before full-scale implementation.
When interviewing human subjects for market or social survey research, we can do our best to try to follow sound sampling procedures, but non-response bias (when the people who don’t complete the survey systematically differ from those that do) can still be a significant problem, leading to incorrect inferences about the population.
Sampling Methods Theory vs. Practice
This article mainly focuses on the theory of sampling. The theory and science of sampling leads to well-established formulas that lead to probability-based inferences we can make about the population (such as a mean with its accompanying confidence interval). But, in the practice of marketing research and social sciences, we’re dealing with humans, rather than colored balls being drawn from buckets or samples of widgets being pulled off the line at a factory.
Humans self-select themselves out of the sample, by refusing to complete surveys or by having filters on their email servers that block our emailed invitations to complete a survey. The formulas we rely on typically assume simple random sampling (SRS), whereas we rarely ever achieve SRS when conducting survey research with human respondents. To the degree that our samples are biased, the measurements and confidence intervals we obtain in surveys are also systematically incorrect. No amount of sample size (short of interviewing every human in the population) can erase the negative effects of biased sampling procedures.
Avoiding Sampling Errors and Bias
The integrity of research findings hinges on the ability to control and minimize sampling errors and biases . Sampling error refers to the natural variation that occurs by chance because a sample, rather than the entire population, is surveyed. Non-sampling error, on the other hand, encompasses all other errors in the research process, from data collection to analysis. Bias, a systematic error, can significantly alter the results (e.g., too high, too low, not variable enough, or too variable), leading to inaccurate conclusions.
Sampling Error and Bias Mitigation Strategies
- Careful Design and Planning : Developing a robust sampling plan that considers the objectives and scope of the study can help in selecting the most suitable sampling method to minimize errors and bias.
- Randomization : Employing random selection methods where possible to ensure each population member has an equal chance of being included, thereby reducing selection bias.
- Stratification : Using stratified sampling to ensure that important subgroups within the population are adequately represented can minimize sampling bias related to specific characteristics.
By proactively addressing these areas, researchers can enhance the reliability and validity of their study outcomes, ensuring that conclusions drawn are reflective of the true population characteristics.
A Note on Online Panel Providers
Whereas phone and snail mail surveys were popular in the 1940s through the 1980s for sampling from the general population, online panel providers are the most commonly used approach today. Although panel providers may report that their samples are balanced to represent the demographic characteristics of the population as a whole, the samples are not unbiased. The kinds of people who participate in panels and self-select to take surveys may be systematically different on the characteristics that are the subject of your study than those who do not participate in your survey.
Need Sample for Your Research?
Let us connect you with your ideal audience! Reach out to us to request sample for your survey research.
Request Sample
Figuring Out Sample Size
Figuring out (determining) the appropriate sample size is crucial for ensuring that your study results are statistically significant and representative of the population. An inadequately small sample size may lead to unreliable results, while an unnecessarily large sample can waste resources and time.
Factors to consider when determining sample size include:
- Population Size : Larger populations may require a larger sample to accurately reflect the population's characteristics, although the relationship is not linear.
- Confidence Level : This reflects how sure you can be that the population’s true mean falls within a certain range. 95% confidence is a common threshold. A higher confidence level requires a larger sample size.
- Margin of Error : Also known as the confidence interval, it indicates the range within which the true value is likely to lie (given a specified degree of confidence, such as 95%). A smaller margin of error requires a larger sample size.
- Power: The ability to detect true differences or relationships in statistics/variables if they in actuality exist.
Combining the elements of confidence level and margin of error, it’s common to read that a poll found that 30% of respondents said they would vote for candidate A with a margin of error of +/- 3% at the 95% confidence level. (If it isn’t explicitly stated, it is generally assumed that the research organization has assumed a 95% confidence level when calculating the margin of error.)
Several sample size calculators and formulas are available to assist researchers in selecting the sample size for determining margins of error at given confidence levels. Consulting with statistical experts or utilizing specialized software can provide additional accuracy and confidence in these calculations, especially regarding power .
False Positives (Type 1 error) vs. False Negatives (Type 2 error)
Most common sample size calculators used for survey research focus on achieving reasonably good precision, meaning tight confidence intervals (such as the common +/-3% confidence interval for proportions at 95% confidence).
Most researchers applying tests for significance (of effects or parameters) want a likelihood of 5% or lower of signaling that a difference or impact (effect) is non-zero when in reality it is not. This is called a False Positive, or a Type 1 error, and 5% “alpha” threshold for Type 1 errors is typical.
Most researchers don’t think about the danger of False Negatives (Type 2 error) when calculating needed sample size. Setting the likelihood of Type 1 errors at 5% means that you are only 50% likely to find a significant effect that actually exists for hypothesis testing purposes. To increase the power that you will be able to detect significant effects if they exist requires additional sample size. Our consultants at Sawtooth Analytics can help you with decisions regarding precision and power related to selecting an appropriate sample size.
Understanding and correctly applying sampling methods are pivotal for the success of market and survey research. The choice of sampling method directly impacts the accuracy, reliability, and applicability of the research findings. By carefully considering the research objectives, population characteristics, practical constraints, and by diligently working to avoid errors and biases, researchers can select the most appropriate sampling strategy for their studies.
Furthermore, determining the right sample size is essential for achieving statistically significant results without overextending resources. Through thoughtful planning and execution, researchers can ensure their studies contribute valuable insights that lead to good policy, decision-making, and inferences about the population.
Sawtooth Software
3210 N Canyon Rd Ste 202
Provo UT 84604-6508
United States of America
Support: [email protected]
Consulting: [email protected]
Sales: [email protected]
Products & Services
Support & Resources
- Online panels
- Data-Collection Services
- Full-Service Research
- Global Omnibus
- Case Studies
- Quality Assurance
- Work with us
- Affiliation
- TGM Content Hub
- Bid Request
- Exploring Survey Sampling Methods
Exploring Survey Sampling Methods: Types, Techniques, and Tips For Academia
- Grasp essential sampling terminology to speak the language of academic research
- Weigh the pros, cons, and appropriate uses of diverse sampling methods
- Fortify research designs against sampling and non-sampling errors
- Balance scientific precision with practical limitations in real-world studies
- Apply sampling techniques correctly to qualitative and quantitative research
- Achieve representative, unbiased, and generalizable samples
Why Sampling Demands Academic Attention
- Generalizability — Conclusions extend beyond isolated samples to broader groups. Such transferability is the lifeblood of impactful academic research.
- Accuracy — Representative samples that avoid systematic biases unlock accurate insights on real-world phenomena.
- Feasibility — Sampling makes research achievable within practical constraints by studying manageable yet meaningful subsets of vast populations.
Sampling ABCs: Key Terminology & Concepts
- Population: The full collection of subjects, objects, or events of interest to the research question make up the study population. This is the total “target” group the project aims to describe or draw conclusions about.
- Sample: A subgroup selected from the population and studied to represent the overall population. This forms the actual set of participants, materials, or data included in the research.
- Sampling Frame: The source material listing all units of the target population (like a directory, catalog, or database). This often serves as the instrument for selecting samples.
- Parameter vs. Statistic: Parameters describe population attributes, while statistics describe sample attributes. The goal is to use sample statistics to estimate analogous population parameters.
- Random Sampling: The selection approach gives all population units equal chances of inclusion. This aims to create representative, unbiased subsets absent researcher manipulation.
- Non-Random Sampling: An umbrella term for sampling techniques where certain population elements carry an advantage (or disadvantage) in a selection over others. Risks sample biases.
Survey Sampling in Technicolor: Types of Sampling Methods
1. probability sampling, 2. non-probability sampling.
What is Random Sampling?
Simple random sampling (srs).

Systematic Sampling

Stratified Sampling

Cluster Sampling
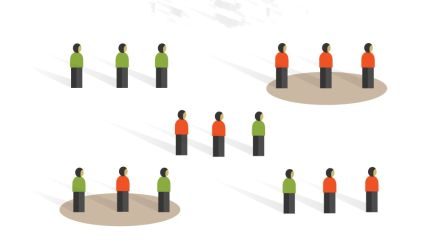
What is Non-Random Sampling?
Convenience sampling.

Purposive or Judgment Sampling
Quota sampling.
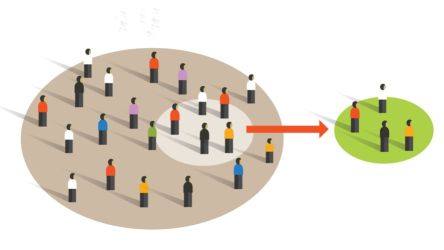
Sampling Scientificamente: Key Implementation Insights
1. sampling error vs. non-sampling error, 2. sample size - how many is enough for my survey, 3. stratified samples & pooling, 4. cluster sampling efficiency, 5. weighting samples, 6. deliberately non-random sampling, mixing & matching: sampling for quantitative vs. qualitative research, wrapping up: springboard to survey sampling success.
As the leading online data collection agency, TGM Research conducted multiple market research projects across the regions. To discover more about our research practices and methodologies reach out to us .
Transform your approach. Let's talk research!
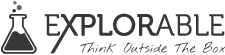
- Foundations
- Write Paper
Search form
- Experiments
- Anthropology
- Self-Esteem
- Social Anxiety
- Survey Guide >
Methods of Survey Sampling
It is important to have a group of people who will participate in the survey and be able to represent the whole target population. This group is called a “sample”. Determining the right kind and number of participants in a sample group, also known as sampling, is one of the basic steps in conducting surveys.
This article is a part of the guide:
- Response Scales
- Example - Questionnaire
- Advantages and Disadvantages
- Surveys and Questionnaires - Guide
- Types of Surveys
Browse Full Outline
- 1 Surveys and Questionnaires - Guide
- 2.1 Research and Surveys
- 2.2 Advantages and Disadvantages
- 2.3 Survey Design
- 2.4 Sampling
- 3.1 Defining Goals
- 4.1 Survey Layout
- 4.2 Types of Questions
- 4.3 Constructing Questions
- 4.4 Response Formats
- 4.5 Response Scales
- 5.1 Selecting Method
- 5.2 Personal Interview
- 5.3 Telephone
- 5.4.1 Preparing Online Surveys
- 5.4.2 Online Tools
- 5.5 Focus Group
- 5.6 Panel Study
- 6.1 Pilot Survey
- 6.2 Increasing Response Rates
- 7.1 Analysis and Data
- 7.2 Conclusion
- 7.3 Presenting the Results
- 8 Example - Questionnaire
- 9 Checklist
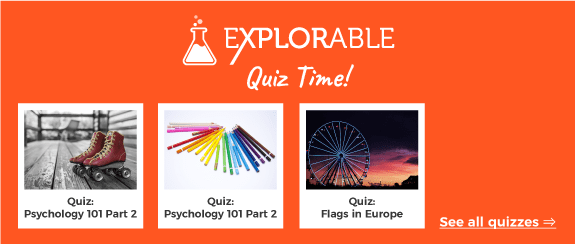
Selecting Target Population
Before you can be able to have a sample for your survey, you need to define your target population first. If your survey goal is to know the effectiveness of a product or service, then the target population should be the customers who have utilized it. It is critical to select the most appropriate target population in order to satisfy the purpose of executing the survey.
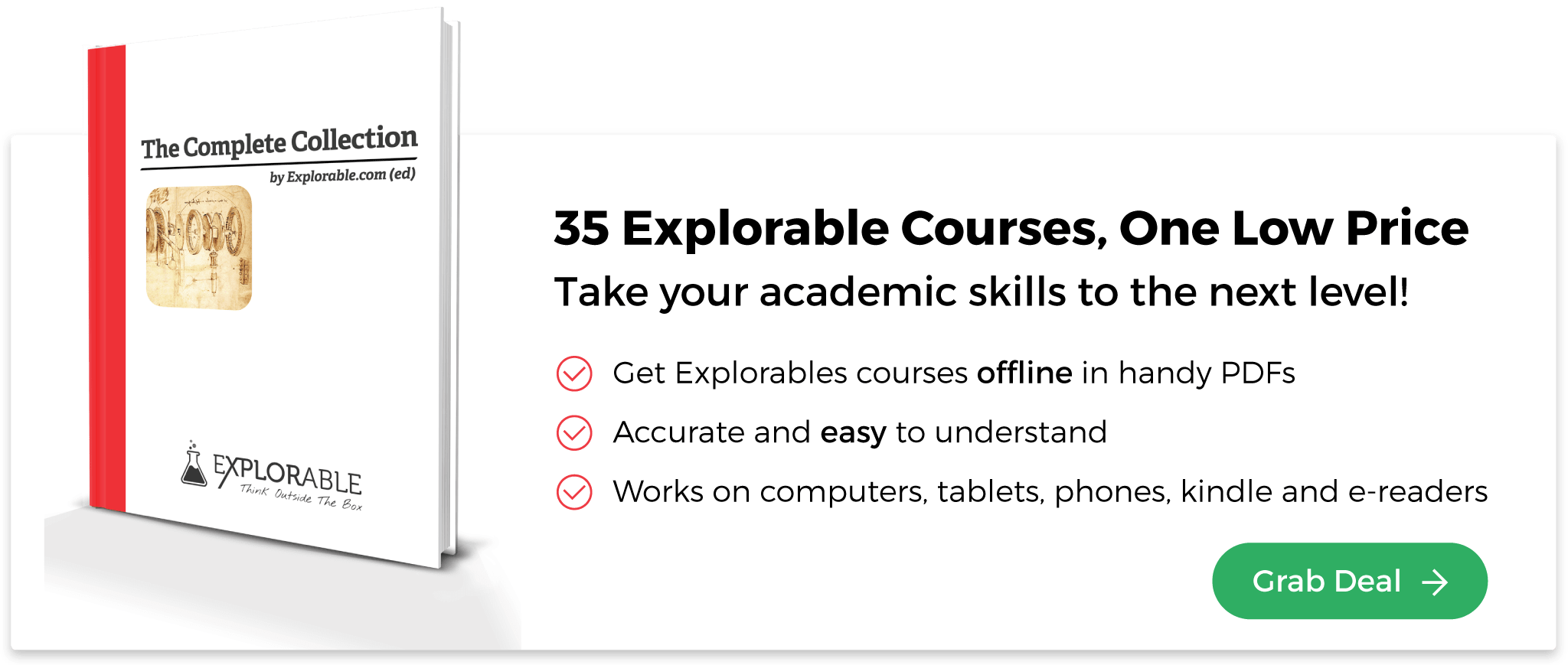
Basic Sampling Techniques
There are numerous ways of getting a sample, but here are the most commonly used sampling methods:
1. Random Sampling
The purest form of sampling under the probability approach, random sampling provides equal chances of being picked for each member of the target population.
2. Stratified Sampling
Stratified sampling involves the use of “stratum”, or a subset of the target population wherein the members possess one or more common attribute. Examples of stratum include mothers, fathers, students, teachers, females, males, etc. Sampling error is usually lower in stratified sampling than in random sampling.
3. Systematic Sampling
In systematic sampling , every Nth name is selected from the list of the members of the target population. For instance, the sample will include the participants listed in every 10th from the list. That means the 10th, 20th, 30th and so on will be selected to become the members of the sample group .
4. Convenience Sampling
This non-probability sampling method is used when there are only a few available members of the target population who can become the participants in the survey.
5. Quota Sampling
Another non-probability method, quota sampling also identifies strata like stratified sampling, but it also uses a convenience sampling approach as the researcher will be the one to choose the necessary number of participants per stratum.
6. Purposive Sampling
As the name suggests, purposive sampling means the researcher selects participants according to the criteria he has set. This is only used when you are confident enough about the representativeness of the participant regarding the whole target population.
Determining Sample Size
Knowing the target population, you have to decide the number of the participants in a sample, which is termed as the “sample size”. Aside from the estimated number of people in the target population, the sample size can be influenced by other factors such as budget, time available, and the target degree of precision. The sample size can be calculated using the formula:
n= | t² x p(1-p) |
n = required sample size t = confidence level at 95% (standard value of 1.96) p = estimated prevalence of the variable of interest (e.g. 20% or 0.2 of the population are smokers) m = margin of error at 5% (standard value of 0.05)
Strictly adhering to the sample size facilitates a higher precision in the results because having participants less than the sample size leads to low representativeness of the target population. On the other hand, going over the sample size may cause a diminished rate of enhancement in the precision of the survey outcomes.
- Psychology 101
- Flags and Countries
- Capitals and Countries
Sarah Mae Sincero (May 10, 2012). Methods of Survey Sampling . Retrieved Aug 10, 2024 from Explorable.com: https://explorable.com/methods-of-survey-sampling
You Are Allowed To Copy The Text
The text in this article is licensed under the Creative Commons-License Attribution 4.0 International (CC BY 4.0) .
This means you're free to copy, share and adapt any parts (or all) of the text in the article, as long as you give appropriate credit and provide a link/reference to this page.
That is it. You don't need our permission to copy the article; just include a link/reference back to this page. You can use it freely (with some kind of link), and we're also okay with people reprinting in publications like books, blogs, newsletters, course-material, papers, wikipedia and presentations (with clear attribution).
Want to stay up to date? Follow us!
Save this course for later.
Don't have time for it all now? No problem, save it as a course and come back to it later.
Footer bottom
- Privacy Policy
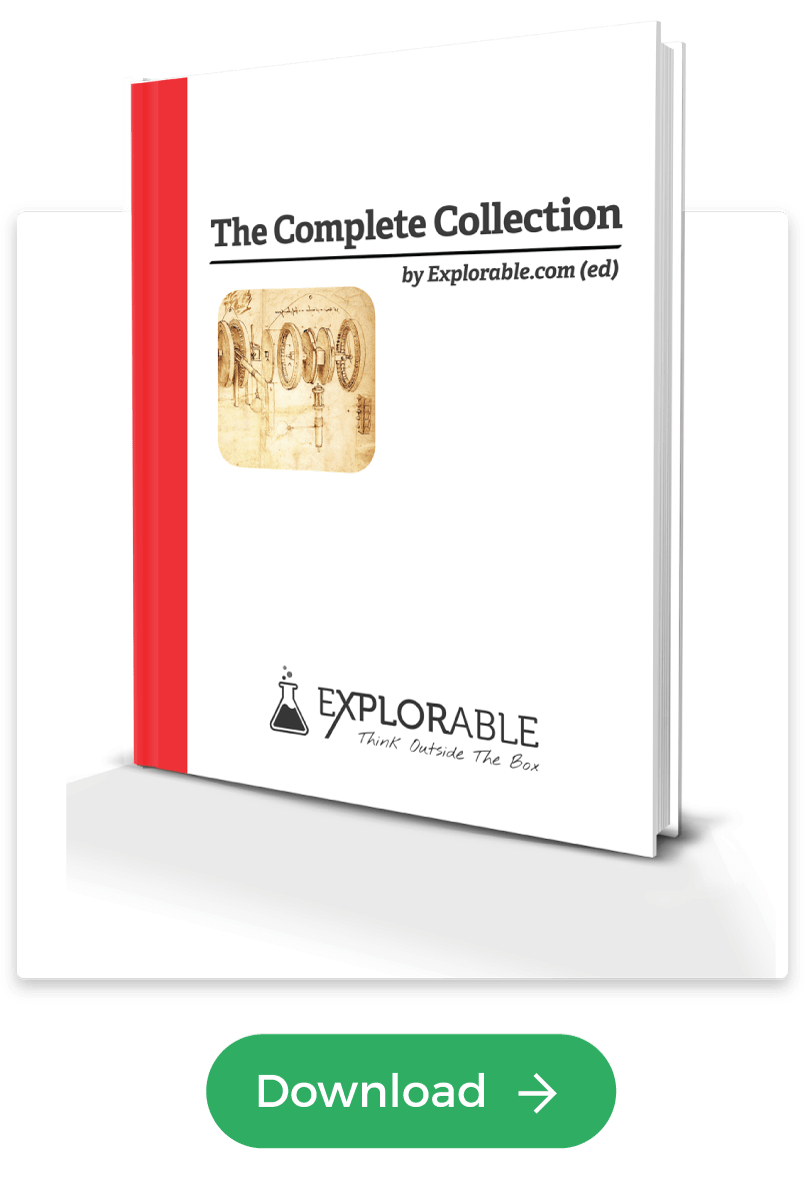
- Subscribe to our RSS Feed
- Like us on Facebook
- Follow us on Twitter
8 Types of Survey Sampling + Pros & Cons, Errors & Bias, and More
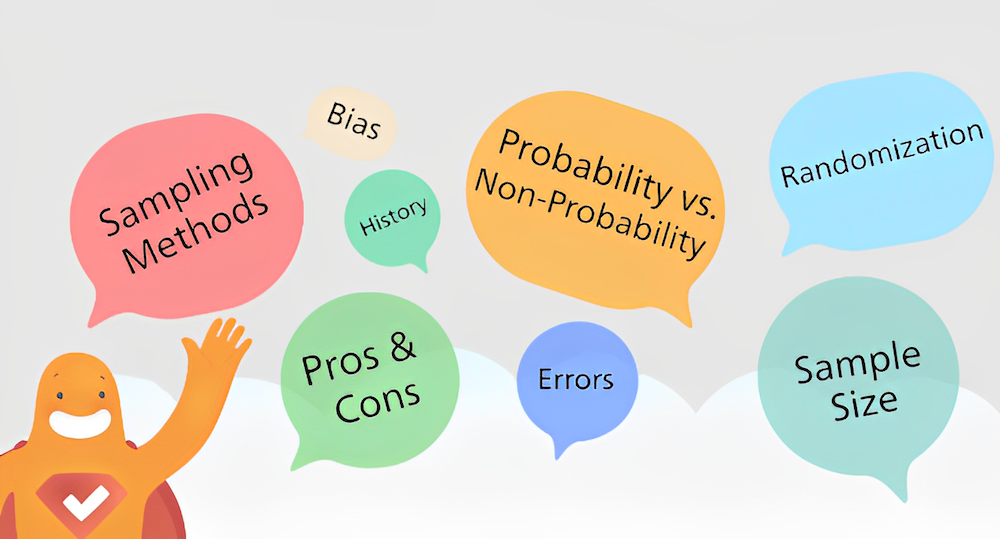
Today, there are about 125 million households in the United States. To help communities and the government understand the direction the country is moving in (and much more), the Constitution requires a census. This census is designed to count every resident of the country every 10 years. So how does the U.S. Census Bureau go about surveying 125 million homes? It doesn’t. The office uses sampling to get the job done. In this blog, we’ll take a look at eight different types of sampling methods and much more.
Create Your FREE Survey, Poll, or Questionnaire now!
What is Survey Sampling?
Sampling is a method of surveying a small portion of the population that represents a larger whole. To go back to our initial example, the U.S. Census Bureau surveys a sample population of 3.5 million households to arrive at conclusions about the population, such as employment, income, poverty, health, insurance coverage, educational attainment, and crime victimization.
While still an extremely large sample, it is significantly less than the 125 million households that makeup the country (a surveying feat that would be near impossible). Thankfully, most surveys aren’t quite such an undertaking and sampling makes them fairly easy to conduct.
History of Survey Sampling
So, who do we have to thank for the idea of sampling? Valery Ivanovich Glivenko and Francesco Paolo Cantelli. In the early 20th century, these two mathematicians discovered that observations randomly drawn from a population gradually take on the shape of the greater population as sample size is increased. When a sufficient sample size is achieved, the sample will have characteristics that mirror those of the population, give or take a few points (called a margin of error).
Determining Survey Sample Size
There are a number of considerations at play when determining sample size, or the number of people chosen for a survey from the total population of that demographic. To begin, most statisticians agree that the minimum sample size to get any kind of meaningful result is 100. If your population is less than 100 then you really need to survey all of them.
So, let’s say you want to know what high school students think about virtual learning. Now, it’d be nearly impossible to survey every high school kid in the country, so you choose a sample to represent them. This could be a couple of thousand kids, for example, versus the millions of them out there. On the other hand, pretend you’re a small business owner with 40 employees. In that case, you’ll want to survey them all to arrive at meaningful conclusions.
Learn more about determining sample size and find a free margin of error calculator in our blog, How to Determine a Sample Size for Your Survey .
Avoiding Survey Sampling Errors
Sampling isn’t always perfect, of course. Sampling errors can be introduced that lead to unacceptable margins of error. There are seven main types of sampling errors:
- Sample Frame Errors. This occurs when the population subset is targeted incorrectly, for example only selecting people from a phonebook, which excludes anyone who is unlisted or doesn’t have a landline.
- Selection Errors. This happens when the sample is composed of volunteers who feel strongly about the topic. However, by leaving out those who don’t feel as strongly, the results will likely be biased.
- Non-response Errors. When not enough people respond to a survey, the sample size becomes smaller and thus is less likely to be representative of the larger population.
- Population-specific Errors. This is common when a researcher is unsure of his target audience. For example, consider a survey about health issues among the elderly. Who should be targeted – the elderly, their caregivers, or their physicians?
- Researcher Bias. This occurs when samples are chosen deliberately by a researcher rather than randomly. Deliberate sampling could result in a researcher introducing their personal bias intentionally or unintentionally. This is most common in in-person surveys, when a researcher could choose participants based on gender, age, race, ethnicity, and so on.
For more, check out our blog 7 Types of Sampling Errors and How to Avoid Them .
What is Sampling Bias?
Sampling bias occurs when researchers fail to choose participants properly or at random. When randomization isn’t used, a survey’s validity can be seriously impacted because it doesn’t accurately reflect the greater population.
A perfect example of sampling bias is demonstrated by an event back in 1948. At the time, Harry Truman and Thomas Dewey were battling it out for the United States Presidency. To predict the winner, a nationwide telephone survey was conducted, and the results heavily favor Dewey. The Chicago Daily Tribune, confident in the survey and wanting to be the first to break the news, prints their newspaper with the headline “Dewey Defeats Truman.” Of course, Dewey didn’t win, and the paper had egg on its face (the snafu resulted in this famous photo of ultimate winner Truman holding up the paper with the incorrect headline while smiling triumphantly).
What went wrong? In 1948, only wealthy families owned telephones, and they tended to favor Dewey. Those who couldn’t afford a phone mostly backed Truman, but they were completely left out of the survey due to the phone sampling bias. So, be sure to be aware of survey biases when sampling, and read more about it in our blog Different Types of Survey Bias and How to Avoid Them .
Main Types of Survey Sampling
There are two main types of sampling methods: Probability sampling and non-probability sampling. Beneath each of these buckets falls four additional types of survey sampling. Let’s dive in.
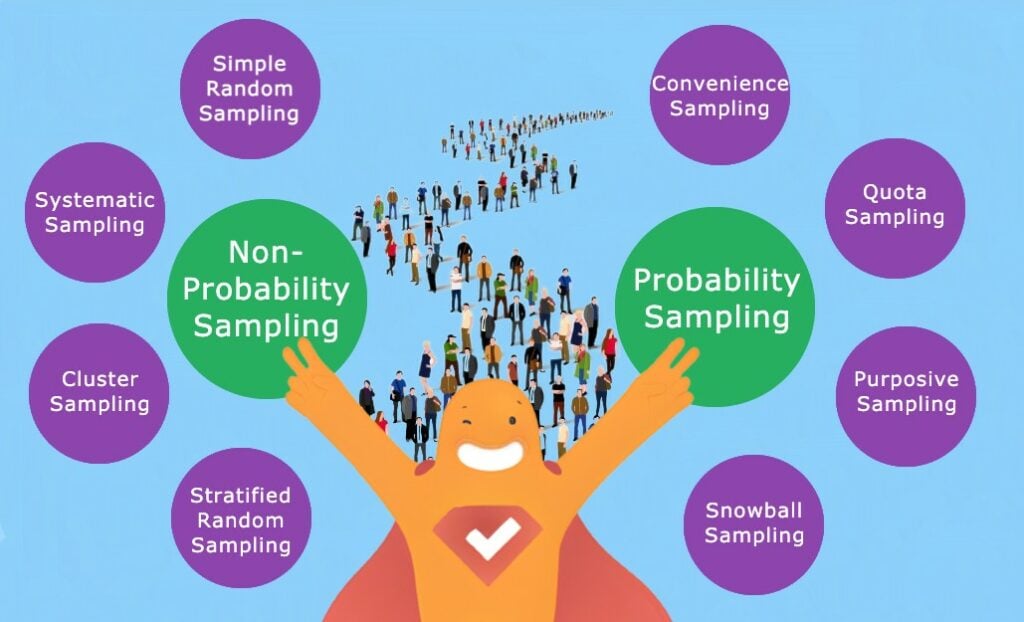
Probability Sampling
With probability sampling, participants are selected completely at random with the goal of ensuring that everyone in the population has an opportunity to be surveyed. Thanks to randomization techniques (such as online random number generators , Excel’s RAND function , or simply drawing names from a hat) this method generally offers results that are representative of the target population. Plus, by choosing participants at random, a researcher’s personal views and opinions cannot influence the sample. There are a feww drawbacks:
- Ensuring randomization can mean more research must be done prior to crafting the survey
- Data analysis following completion of the survey can be more complicated
- If response rates are low, randomization minus those previously surveyed must be performed again.
Read more in our blog, What is Probability Sampling? Pros, Cons, and Examples .
Non-Probability Sampling
With non-probability sampling, randomization techniques are not employed, meaning that not everyone will have a fair chance of being surveyed. However, this makes it a fast and inexpensive way to collect data as little research is required prior to surveying. This makes it a great starting point in which to form quick hypotheses and then determine if further probability sampling would be beneficial. Another plus? If response rates are low, the researcher can simply continue sending surveys until they meet their goal without having to worry about randomization. Of course, there are drawbacks here as well:
- Because participants tend to be surveyed based on their ease of access rather than randomly, there’s a high chance they may not be representative of the greater population.
- Samples can sometimes be filled with people who want to be part of research because they want the incentive or hold strong views that they want to share, skewing results.
- There is a high potential for sampling bias because sample selection is deliberate. This means a researcher’s personal views and opinions could easily influence the sample, or he or she may be selective about who they choose to participate.
Read more in our blog, What is Non-Probability Sampling: Pros, Cons, and Examples .
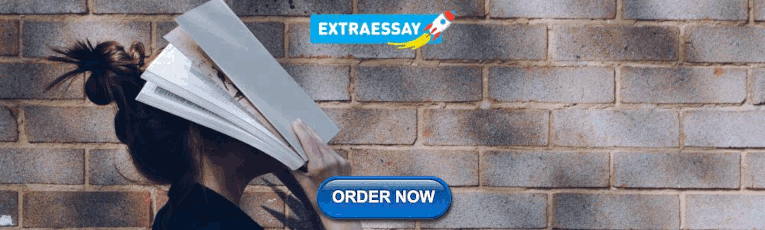
Example of Probability and Non-Probability Sampling
To better understand these sampling methods, let’s consider a few examples of sampling. Let’s say you’re the owner of Bob’s Bait & Tackle. It’s a relatively small business, though you do have a database of 5,000 customers. You want to know how customers feel about your store, but surveying all 5,000 of them would be too time consuming. So, you survey 10% of them, or 500.
With probability sampling, all customers must have an equal chance of participating,. So, you use a number generator (1 – 5,000) and choose 500 customers at random who correspond with the numbers and survey them. In this scenario, all 5,000 customers had the opportunity to be part of the survey so there is less opportunity for survey bias or error.
With non-probability sampling, you’re not going to worry about randomization; you just want to quickly and easily survey 500 people total. So, you simply survey to the first 500 customers in your database. 4,500 people were effectively excluded, which increases the chances of survey bias or sampling errors but allows you to complete the survey quickly and easily.
Probability Sampling Methods
There are four probability sampling techniques. Here’s a quick look at each.
1. Simple Random Sampling
This is the easiest way of randomly sampling from a population. Simply give numbers to everyone in the sample and then randomly choose those numbers (pull them from a hat if it’s a small sample, or use an automated process if it’s large). Read more: Simple random sampling .
2. Systematic Sampling
A bit more complex, this method draws a random sample from the target population by selecting units at regular intervals starting from a random point. Researchers call this “choosing every ‘nth’” (e.g. 5th, 10th, 12th, etc) individual. Read more: Systematic Sampling .
3. Cluster Sampling
This sampling technique divides the target population into groups or “clusters.” Next, the researcher choose a subsection of each group at random. This method is more beneficial when working with a large and geographically spread out population that share similarities (e.g. number of children, occupation, or college major). Read more: Single, Double, and Multistage Cluster Sampling .
4. Stratified Random Sampling
This method involves dividing a large population into smaller groups that typically don’t overlap but represent the entire population. Often, this means classifying groups by demographic factors such as gender, age, race, ethnicity, etc. To determine samples, the researcher splits subjects into mutually exclusive demographic groups and then uses simple random sampling to select members.
Non-Probability Sampling Methods
There are four non-probability sampling techniques. Here’s a quick look at each.
1. Convenience Sampling
This sampling method is quick and easy, involving surveying those within easy reach. In this type of sampling, the researcher doesn’t care whether the sample is representative of the entire population; everyone is eligible. For example, a college student wants to learn about alcohol consumption among undergraduates so she surveys people in her dorm because they’re conveniently located. Read more: Convenience sampling .
2. Quota Sampling
Similar to convenience sampling, however the researcher has a specific number of people they intend to survey (e.g. 50 men and 50 women). This provides a more diverse number of respondents from different subpopulations. Read more: Quota sampling .
3. Purposive Sampling
With this technique, the researcher uses their understanding of the survey’s purpose and their knowledge of the population to decide who to include in the sample. They may choose one of the following purposive sampling methods:
- Heterogeneity sampling: collecting the widest range of opinions from different people
- Homogeneous sampling: collecting opinions from like-minded participants
- Deviant sampling: collecting opinions from people with a specific or unusual trait
- Expert sampling: collecting opinions from experts on the topic
4. Snowball Sampling
Researchers use snowball sampling when targeting specific, hard-to-reach groups. This people may be hesitant to participate, for example illegal immigrants, drug users, or those with health conditions. In these instances, the researcher uses people who fit the necessary criteria to recruit others in their social circle who also fit. In this way, the sample size “snowballs,” becoming larger as more recruits participate resulting in a more accurate survey.
Online surveys are a great way to conduct both probability and non-probability sampling. Online surveys allow you to cast a wide net, reaching across demographics and geographical boundaries. With SurveyLegend, our online surveys are easy to create and easy on the eyes – that’s because you can add pictures to surveys, boosting engagement, triggering respondent emotion and memory, and crossing language barriers. Below is an example of one of our photo surveys that plays off the example earlier of a college student surveying classmates about alcohol.
The survey below is LIVE, so give it a try !
Frequently Asked Questions (FAQs)
A quick, easy, and inexpensive way to survey a subset of a larger population, non-probability sampling is a subjective (or non-random) method of sampling.
A sampling technique that aims to ensure everyone in a population has an equal chance of being surveyed, non-probability sampling employs random sampling methods.
Probability sampling uses randomization to ensure samples representative of the greater population, while non-probability forgoes randomization for simple, convenient sampling that is generally less accurate.
There are four main methods of probability sampling: simple random sampling, stratified random sampling, cluster sampling, and systematic sampling.
There are four main methods of non-probability sampling: convenience sampling, quota sampling, purposive sampling, and snowball sampling.
The most common methods of randomization include lottery methods (drawing names from a hat), online number generator tools, and the RAND function in Microsoft Excel.
Jasko Mahmutovic
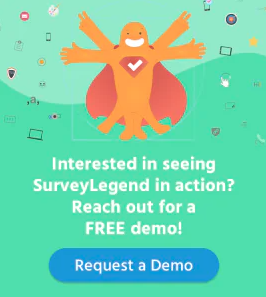
Related Articles You Might Like
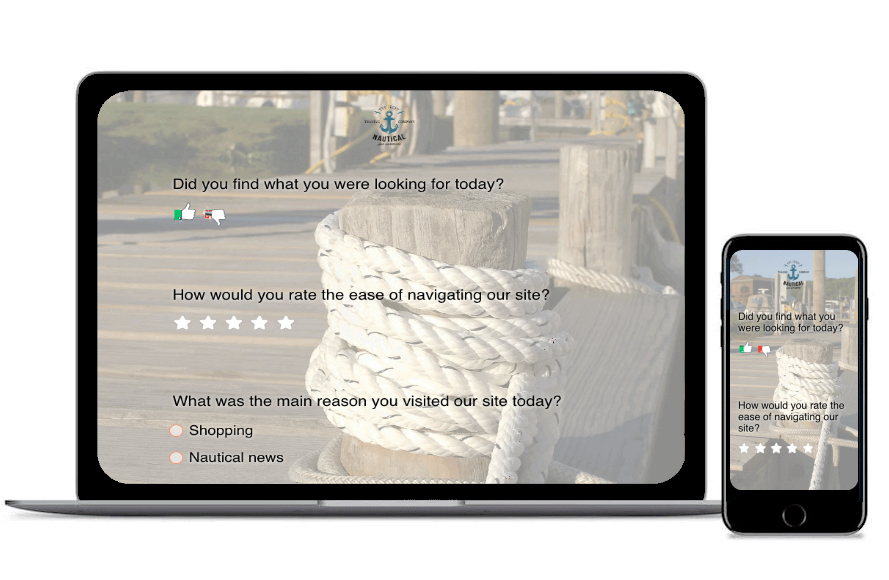
How To Use An Exit Intent Survey + Pros & Cons
“Hey, where you going?” In a nutshell, that’s the gist of an exit intent survey. These online surveys are designed to capture feedback from website visitors who are about...
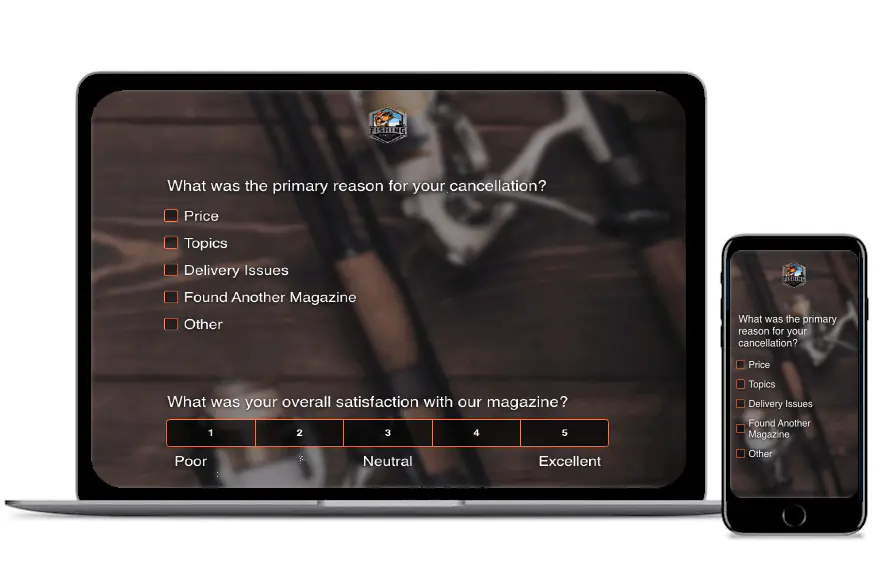
7 Reasons To Use A Cancellation Survey & Questions To Ask
No company likes to see a customer leave. For one, it’s been drilled into all of us that it costs a lot more to acquire a new customer than...
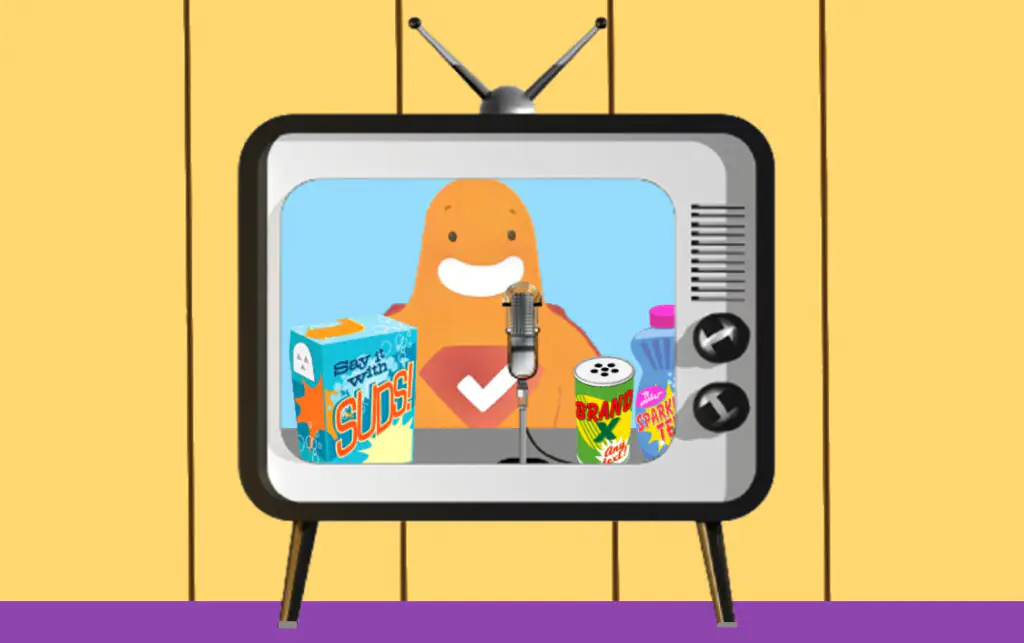
How To Create An Advertising Survey To Measure Effectiveness
Ads are inescapable today. They’re on your television, on the radio, on the road, and popping up on your computer or phone. But how effective are they? Is the...
Privacy Overview
Numbers, Facts and Trends Shaping Your World
Read our research on:
Full Topic List
Regions & Countries
- Publications
- Our Methods
- Short Reads
- Tools & Resources
Read Our Research On:
U.S. Survey Methodology
A typical Pew Research Center national survey – regardless of mode – is designed and implemented with a total survey error approach in mind, aimed at minimizing coverage error (deviations of frame population from target population), sampling error (deviation of sample from sampling frame), nonresponse error (respondents’ deviation from sample), measurement error (deviations of responses from underlying measurements/attributes), and processing and adjustment error (error introduced post data collection but prior to data analysis).
Since 2014, Pew Research Center has conducted surveys online in the United States using our American Trends Panel (ATP), a randomly selected, probability-based sample of U.S. adults ages 18 and older. The panel was initially built to supplement the prevalent mode of data collection at the Center during that time: random-digit-dial (RDD) telephone surveys. However, at this point, the Center has switched almost completely to conducting its U.S. surveys online using the ATP.
Panel members are recruited offline, and survey questionnaires are taken via self-administered online surveys. Those who don’t have internet access can take our surveys on internet-enabled tablets we provide to them. Panelists typically take one to three surveys each month.
Most of our surveys are representative of the entire noninstitutionalized adult population of the United States. We do not exclude anyone from our analyses based on demographic characteristics. Some surveys are conducted among subgroups in the panel, such as Black Americans or young people, and may include a supplement sample (called “ oversamples ”) from another panel such as Ipsos’ KnowledgePanel. Pew Research Center also conducts international surveys that involve sampling and interviewing people in multiple countries.
On occasion, Center researchers conduct surveys with special populations, such as topic experts (e.g., technologists ) or members of a certain profession (e.g., journalists ). The principles are the same whether the sample is of the general population or some other group. Decisions must be made about the size of the sample and the level of precision desired so that the survey can provide accurate estimates for the population of interest and any subgroups within the population that will be analyzed. Some special challenges arise when sampling these populations. In particular, it may be difficult to find a sampling frame or list for the population of interest, and this may influence how the population is defined. In addition, information may be available for only some methods of contacting potential respondents (e.g., email addresses but not phone numbers) and may vary for people within the sample. If most members in the population of interest have internet access, and email addresses are available for contacting them, the web often provides a convenient and inexpensive way to survey experts or other industry-based populations.
Creating good measures requires asking unambiguous and unbiased questions . There are several steps involved in developing a survey questionnaire . The first is identifying what topics will be covered in the survey. For Pew Research Center surveys, this involves thinking about what is happening in our nation and the world and what will be relevant to the public, policymakers and the media. We also track opinion on a variety of issues over time, so we often ensure that we update these trends on a regular basis so we can understand whether people’s opinions are changing.
Each Pew Research Center survey report includes a “topline questionnaire” with all of the questions from that survey with the exact question wording and response options as they were read to respondents. This topline provides the results from the current survey for each question, as well as results from previous surveys in which the same questions were asked. For most studies, it is our policy to release ATP datasets within twelve months of data collection. Please visit our datasets page for further information.
U.S. Surveys
Other research methods.
901 E St. NW, Suite 300 Washington, DC 20004 USA (+1) 202-419-4300 | Main (+1) 202-857-8562 | Fax (+1) 202-419-4372 | Media Inquiries
Research Topics
- Email Newsletters
ABOUT PEW RESEARCH CENTER Pew Research Center is a nonpartisan fact tank that informs the public about the issues, attitudes and trends shaping the world. It conducts public opinion polling, demographic research, media content analysis and other empirical social science research. Pew Research Center does not take policy positions. It is a subsidiary of The Pew Charitable Trusts .
© 2024 Pew Research Center
- Privacy Policy

Home » Research Methodology – Types, Examples and writing Guide
Research Methodology – Types, Examples and writing Guide
Table of Contents

Research Methodology
Definition:
Research Methodology refers to the systematic and scientific approach used to conduct research, investigate problems, and gather data and information for a specific purpose. It involves the techniques and procedures used to identify, collect , analyze , and interpret data to answer research questions or solve research problems . Moreover, They are philosophical and theoretical frameworks that guide the research process.
Structure of Research Methodology
Research methodology formats can vary depending on the specific requirements of the research project, but the following is a basic example of a structure for a research methodology section:
I. Introduction
- Provide an overview of the research problem and the need for a research methodology section
- Outline the main research questions and objectives
II. Research Design
- Explain the research design chosen and why it is appropriate for the research question(s) and objectives
- Discuss any alternative research designs considered and why they were not chosen
- Describe the research setting and participants (if applicable)
III. Data Collection Methods
- Describe the methods used to collect data (e.g., surveys, interviews, observations)
- Explain how the data collection methods were chosen and why they are appropriate for the research question(s) and objectives
- Detail any procedures or instruments used for data collection
IV. Data Analysis Methods
- Describe the methods used to analyze the data (e.g., statistical analysis, content analysis )
- Explain how the data analysis methods were chosen and why they are appropriate for the research question(s) and objectives
- Detail any procedures or software used for data analysis
V. Ethical Considerations
- Discuss any ethical issues that may arise from the research and how they were addressed
- Explain how informed consent was obtained (if applicable)
- Detail any measures taken to ensure confidentiality and anonymity
VI. Limitations
- Identify any potential limitations of the research methodology and how they may impact the results and conclusions
VII. Conclusion
- Summarize the key aspects of the research methodology section
- Explain how the research methodology addresses the research question(s) and objectives
Research Methodology Types
Types of Research Methodology are as follows:
Quantitative Research Methodology
This is a research methodology that involves the collection and analysis of numerical data using statistical methods. This type of research is often used to study cause-and-effect relationships and to make predictions.
Qualitative Research Methodology
This is a research methodology that involves the collection and analysis of non-numerical data such as words, images, and observations. This type of research is often used to explore complex phenomena, to gain an in-depth understanding of a particular topic, and to generate hypotheses.
Mixed-Methods Research Methodology
This is a research methodology that combines elements of both quantitative and qualitative research. This approach can be particularly useful for studies that aim to explore complex phenomena and to provide a more comprehensive understanding of a particular topic.
Case Study Research Methodology
This is a research methodology that involves in-depth examination of a single case or a small number of cases. Case studies are often used in psychology, sociology, and anthropology to gain a detailed understanding of a particular individual or group.
Action Research Methodology
This is a research methodology that involves a collaborative process between researchers and practitioners to identify and solve real-world problems. Action research is often used in education, healthcare, and social work.
Experimental Research Methodology
This is a research methodology that involves the manipulation of one or more independent variables to observe their effects on a dependent variable. Experimental research is often used to study cause-and-effect relationships and to make predictions.
Survey Research Methodology
This is a research methodology that involves the collection of data from a sample of individuals using questionnaires or interviews. Survey research is often used to study attitudes, opinions, and behaviors.
Grounded Theory Research Methodology
This is a research methodology that involves the development of theories based on the data collected during the research process. Grounded theory is often used in sociology and anthropology to generate theories about social phenomena.
Research Methodology Example
An Example of Research Methodology could be the following:
Research Methodology for Investigating the Effectiveness of Cognitive Behavioral Therapy in Reducing Symptoms of Depression in Adults
Introduction:
The aim of this research is to investigate the effectiveness of cognitive-behavioral therapy (CBT) in reducing symptoms of depression in adults. To achieve this objective, a randomized controlled trial (RCT) will be conducted using a mixed-methods approach.
Research Design:
The study will follow a pre-test and post-test design with two groups: an experimental group receiving CBT and a control group receiving no intervention. The study will also include a qualitative component, in which semi-structured interviews will be conducted with a subset of participants to explore their experiences of receiving CBT.
Participants:
Participants will be recruited from community mental health clinics in the local area. The sample will consist of 100 adults aged 18-65 years old who meet the diagnostic criteria for major depressive disorder. Participants will be randomly assigned to either the experimental group or the control group.
Intervention :
The experimental group will receive 12 weekly sessions of CBT, each lasting 60 minutes. The intervention will be delivered by licensed mental health professionals who have been trained in CBT. The control group will receive no intervention during the study period.
Data Collection:
Quantitative data will be collected through the use of standardized measures such as the Beck Depression Inventory-II (BDI-II) and the Generalized Anxiety Disorder-7 (GAD-7). Data will be collected at baseline, immediately after the intervention, and at a 3-month follow-up. Qualitative data will be collected through semi-structured interviews with a subset of participants from the experimental group. The interviews will be conducted at the end of the intervention period, and will explore participants’ experiences of receiving CBT.
Data Analysis:
Quantitative data will be analyzed using descriptive statistics, t-tests, and mixed-model analyses of variance (ANOVA) to assess the effectiveness of the intervention. Qualitative data will be analyzed using thematic analysis to identify common themes and patterns in participants’ experiences of receiving CBT.
Ethical Considerations:
This study will comply with ethical guidelines for research involving human subjects. Participants will provide informed consent before participating in the study, and their privacy and confidentiality will be protected throughout the study. Any adverse events or reactions will be reported and managed appropriately.
Data Management:
All data collected will be kept confidential and stored securely using password-protected databases. Identifying information will be removed from qualitative data transcripts to ensure participants’ anonymity.
Limitations:
One potential limitation of this study is that it only focuses on one type of psychotherapy, CBT, and may not generalize to other types of therapy or interventions. Another limitation is that the study will only include participants from community mental health clinics, which may not be representative of the general population.
Conclusion:
This research aims to investigate the effectiveness of CBT in reducing symptoms of depression in adults. By using a randomized controlled trial and a mixed-methods approach, the study will provide valuable insights into the mechanisms underlying the relationship between CBT and depression. The results of this study will have important implications for the development of effective treatments for depression in clinical settings.
How to Write Research Methodology
Writing a research methodology involves explaining the methods and techniques you used to conduct research, collect data, and analyze results. It’s an essential section of any research paper or thesis, as it helps readers understand the validity and reliability of your findings. Here are the steps to write a research methodology:
- Start by explaining your research question: Begin the methodology section by restating your research question and explaining why it’s important. This helps readers understand the purpose of your research and the rationale behind your methods.
- Describe your research design: Explain the overall approach you used to conduct research. This could be a qualitative or quantitative research design, experimental or non-experimental, case study or survey, etc. Discuss the advantages and limitations of the chosen design.
- Discuss your sample: Describe the participants or subjects you included in your study. Include details such as their demographics, sampling method, sample size, and any exclusion criteria used.
- Describe your data collection methods : Explain how you collected data from your participants. This could include surveys, interviews, observations, questionnaires, or experiments. Include details on how you obtained informed consent, how you administered the tools, and how you minimized the risk of bias.
- Explain your data analysis techniques: Describe the methods you used to analyze the data you collected. This could include statistical analysis, content analysis, thematic analysis, or discourse analysis. Explain how you dealt with missing data, outliers, and any other issues that arose during the analysis.
- Discuss the validity and reliability of your research : Explain how you ensured the validity and reliability of your study. This could include measures such as triangulation, member checking, peer review, or inter-coder reliability.
- Acknowledge any limitations of your research: Discuss any limitations of your study, including any potential threats to validity or generalizability. This helps readers understand the scope of your findings and how they might apply to other contexts.
- Provide a summary: End the methodology section by summarizing the methods and techniques you used to conduct your research. This provides a clear overview of your research methodology and helps readers understand the process you followed to arrive at your findings.
When to Write Research Methodology
Research methodology is typically written after the research proposal has been approved and before the actual research is conducted. It should be written prior to data collection and analysis, as it provides a clear roadmap for the research project.
The research methodology is an important section of any research paper or thesis, as it describes the methods and procedures that will be used to conduct the research. It should include details about the research design, data collection methods, data analysis techniques, and any ethical considerations.
The methodology should be written in a clear and concise manner, and it should be based on established research practices and standards. It is important to provide enough detail so that the reader can understand how the research was conducted and evaluate the validity of the results.
Applications of Research Methodology
Here are some of the applications of research methodology:
- To identify the research problem: Research methodology is used to identify the research problem, which is the first step in conducting any research.
- To design the research: Research methodology helps in designing the research by selecting the appropriate research method, research design, and sampling technique.
- To collect data: Research methodology provides a systematic approach to collect data from primary and secondary sources.
- To analyze data: Research methodology helps in analyzing the collected data using various statistical and non-statistical techniques.
- To test hypotheses: Research methodology provides a framework for testing hypotheses and drawing conclusions based on the analysis of data.
- To generalize findings: Research methodology helps in generalizing the findings of the research to the target population.
- To develop theories : Research methodology is used to develop new theories and modify existing theories based on the findings of the research.
- To evaluate programs and policies : Research methodology is used to evaluate the effectiveness of programs and policies by collecting data and analyzing it.
- To improve decision-making: Research methodology helps in making informed decisions by providing reliable and valid data.
Purpose of Research Methodology
Research methodology serves several important purposes, including:
- To guide the research process: Research methodology provides a systematic framework for conducting research. It helps researchers to plan their research, define their research questions, and select appropriate methods and techniques for collecting and analyzing data.
- To ensure research quality: Research methodology helps researchers to ensure that their research is rigorous, reliable, and valid. It provides guidelines for minimizing bias and error in data collection and analysis, and for ensuring that research findings are accurate and trustworthy.
- To replicate research: Research methodology provides a clear and detailed account of the research process, making it possible for other researchers to replicate the study and verify its findings.
- To advance knowledge: Research methodology enables researchers to generate new knowledge and to contribute to the body of knowledge in their field. It provides a means for testing hypotheses, exploring new ideas, and discovering new insights.
- To inform decision-making: Research methodology provides evidence-based information that can inform policy and decision-making in a variety of fields, including medicine, public health, education, and business.
Advantages of Research Methodology
Research methodology has several advantages that make it a valuable tool for conducting research in various fields. Here are some of the key advantages of research methodology:
- Systematic and structured approach : Research methodology provides a systematic and structured approach to conducting research, which ensures that the research is conducted in a rigorous and comprehensive manner.
- Objectivity : Research methodology aims to ensure objectivity in the research process, which means that the research findings are based on evidence and not influenced by personal bias or subjective opinions.
- Replicability : Research methodology ensures that research can be replicated by other researchers, which is essential for validating research findings and ensuring their accuracy.
- Reliability : Research methodology aims to ensure that the research findings are reliable, which means that they are consistent and can be depended upon.
- Validity : Research methodology ensures that the research findings are valid, which means that they accurately reflect the research question or hypothesis being tested.
- Efficiency : Research methodology provides a structured and efficient way of conducting research, which helps to save time and resources.
- Flexibility : Research methodology allows researchers to choose the most appropriate research methods and techniques based on the research question, data availability, and other relevant factors.
- Scope for innovation: Research methodology provides scope for innovation and creativity in designing research studies and developing new research techniques.
Research Methodology Vs Research Methods
Research Methodology | Research Methods |
---|---|
Research methodology refers to the philosophical and theoretical frameworks that guide the research process. | refer to the techniques and procedures used to collect and analyze data. |
It is concerned with the underlying principles and assumptions of research. | It is concerned with the practical aspects of research. |
It provides a rationale for why certain research methods are used. | It determines the specific steps that will be taken to conduct research. |
It is broader in scope and involves understanding the overall approach to research. | It is narrower in scope and focuses on specific techniques and tools used in research. |
It is concerned with identifying research questions, defining the research problem, and formulating hypotheses. | It is concerned with collecting data, analyzing data, and interpreting results. |
It is concerned with the validity and reliability of research. | It is concerned with the accuracy and precision of data. |
It is concerned with the ethical considerations of research. | It is concerned with the practical considerations of research. |
About the author
Muhammad Hassan
Researcher, Academic Writer, Web developer
You may also like

Implications in Research – Types, Examples and...

Research Project – Definition, Writing Guide and...

Ethical Considerations – Types, Examples and...

Context of the Study – Writing Guide and Examples

Critical Analysis – Types, Examples and Writing...

Research Methods – Types, Examples and Guide
- Search Search Please fill out this field.
What Is a Simple Random Sample?
- How It Works
- Conducting a Simple Random Sample
Random Sampling Techniques
- Simple Random vs. Other Methods
- Pros and Cons
- Simple Random Sample FAQs
The Bottom Line
- Corporate Finance
- Financial Analysis
Simple Random Sampling: 6 Basic Steps With Examples
Adam Hayes, Ph.D., CFA, is a financial writer with 15+ years Wall Street experience as a derivatives trader. Besides his extensive derivative trading expertise, Adam is an expert in economics and behavioral finance. Adam received his master's in economics from The New School for Social Research and his Ph.D. from the University of Wisconsin-Madison in sociology. He is a CFA charterholder as well as holding FINRA Series 7, 55 & 63 licenses. He currently researches and teaches economic sociology and the social studies of finance at the Hebrew University in Jerusalem.
:max_bytes(150000):strip_icc():format(webp)/adam_hayes-5bfc262a46e0fb005118b414.jpg)
A simple random sample is a subset of a statistical population in which each member of the subset has an equal probability of being chosen. A simple random sample is meant to be an unbiased representation of a group.
Key Takeaways
- A simple random sample takes a small, random portion of the entire population to represent the entire data set, where each member has an equal probability of being chosen.
- Researchers can create a simple random sample using methods such as lotteries or random draws.
- A sampling error can occur with a simple random sample if the sample does not end up accurately reflecting the population it is supposed to represent.
- Simple random samples are determined by assigning sequential values to each item within a population, then randomly selecting those values.
- Systematic sampling, stratified sampling, and cluster sampling are other types of sampling approaches that may be used instead of simple random sampling.
Investopedia / Madelyn Goodnight
Understanding a Simple Random Sample
Researchers can create a simple random sample using a couple of methods. With a lottery method, each member of the population is assigned a number, and numbers are then selected at random.
An example of a simple random sample would be to choose the names of 25 employees out of a hat from a company of 250 employees. In this case the population is all 250 employees, and the sample is random because each employee has an equal chance of being chosen. Random sampling is used in science to conduct randomized control tests or for blinded experiments.
The example in which the names of 25 employees out of 250 are chosen out of a hat is an example of the lottery method at work. Each of the 250 employees would be assigned a number between one and 250, after which 25 of those numbers would be chosen at random.
Because individuals who make up the subset of the larger group are chosen at random, each individual in the large population set has the same probability of being selected. In most cases this creates a balanced subset that carries the greatest potential for representing the larger group as a whole.
A manual lottery method can be quite onerous for larger populations. Selecting a random sample from a large population usually requires a computer-generated process. The same methodology as the lottery method is used, only the number assignments and subsequent selections are performed by computers, not humans.
Room for Error
With a simple random sample, there has to be room for error represented by a plus and minus variance ( sampling error ). For example, if a survey is taken to determine how many students are left-handed in a high school of 1,000 students, random sampling can determine that eight out of the 100 sampled are left-handed. The conclusion would then be that 8% of the student population of the high school are left-handed, when in fact the global average would be closer to 10%.
The same is true regardless of the subject matter. A survey on the percentage of the student population that has green eyes or a physical disability would result in a mathematical probability based on a simple random survey, but always with a plus or minus variance. The only way to have 100% accuracy rate would be to survey all 1,000 students which, while possible, would be impractical.
Although simple random sampling is intended to be an unbiased approach to surveying, sample selection bias can occur. When a sample set of the larger population is not inclusive enough, representation of the full population is skewed and requires additional sampling techniques.
How to Conduct a Simple Random Sample
The simple random sampling process entails six steps, each performed in sequential order.
Step 1: Define the Population
The starting point of statistical analysis is to determine the population base. This is the group about which you wish to learn more, confirm a hypothesis , or determine a statistical outcome. This step is simply to identify what that population base is and ensure that the group will adequately cover the outcome you are trying to ascertain.
Example: You want to learn how the stocks of the largest companies in the United States have performed over the past 20 years. Your population would be the largest companies in the United States as determined by the S&P 500.
Step 2: Choose the Sample Size
Before picking the units within a population, we need to determine how many to select. This sample size may be constrained by the amount of time, capital rationing , or other resources available to analyze the sample. However, be mindful to pick a sample size large enough to be genuinely representative of the population. In the example above, there are constraints in analyzing the performance for every stock in the S&P 500, so we only want to analyze a subset of this population.
Example: Your sample size will be 20 companies from the S&P 500.
Step 3: Determine Population Units
In our example the items within the population are easy to determine, as they've already been identified for us (i.e., the companies listed within the S&P 500). However, imagine analyzing the students currently enrolled at a university or food products being sold at a grocery store. This step entails crafting the entire list of all items within your population.
Example: Using exchange information, you copy the companies comprising the S&P 500 into an Excel spreadsheet.
Step 4: Assign Numerical Values
The simple random sample process calls for every unit within the population to receive an unrelated numerical value. This is often assigned based on how the data may be filtered. For example, you could assign the numbers one to 500 to the companies based on market cap , alphabetical order, or company formation date. How the values are assigned isn’t relevant; all that matters is that each value is sequential and has an equal chance of being selected.
Example: You assign the numbers one through 500 to the companies in the S&P 500 based on alphabetical order of the current CEO's surname, with the first company receiving the value one and the last company receiving the value 500.
Step 5: Select Random Values
In step 2 we chose 20 as the number of items we wanted to analyze within our population. We now randomly select 20 number values out of the 500. There are multiple ways to do this, as discussed later in this article.
Example: Using a random number table (see below), you select the numbers 2, 7, 17, 67, 68, 75, 77, 87, 92, 101, 145, 201, 222, 232, 311, 333, 376, 401, 478, and 489.
Step 6: Identify the Sample
Each of the random variables selected in the prior step corresponds to an item within our population. The group sample is selected by identifying which random values were chosen and which population items those values match.
Example: Your sample consists of the companies that correspond to the values chosen in step 5.
There is no single method for determining the random values to be selected in step 5. The analyst can’t choose completely random numbers on their own, as there may be factors influencing their decision. For example, the analyst’s wedding anniversary may be the 24th, so they may consciously (or subconsciously) pick the random value 24. Instead, the analyst may choose one of the following methods:
- Random lottery : Each population number receives an equivalent item, say a ping pong ball or slip of paper, on which it is written, and those items are stored in a box. Random numbers are then selected by pulling items from the container without looking at them.
- Physical methods : Simple, early methods of random selection may use dice, flipping coins, or spinning wheels. Each outcome is assigned a value or outcome relating to the population.
- Random number table : Many statistics and research books contain sample tables with randomized numbers.
- Online random number generator : Many online tools exist where an analyst inputs first the population size and then the sample size to be selected.
- Random numbers from Excel : Numbers can be selected in Excel using the =RANDBETWEEN formula. A cell containing =RANDBETWEEN(1,5) will select a single random number between one and 5.
When pulling together a sample, consider getting assistance from a colleague or an independent person. They may be able to identify biases or discrepancies of which you may not be aware.
Simple Random vs. Other Sampling Methods
Simple random vs. stratified random sample.
A simple random sample is used to represent the entire data population. A stratified random sample divides the population into smaller groups, known as “strata,” based on shared characteristics.
Unlike simple random samples, stratified random samples are used with populations that can be easily broken into different subgroups or subsets. These groups are based on certain criteria, then elements from each are randomly chosen in proportion to the group’s size versus the population. In our example above, S&P 500 companies could have subsets defined by type of industry or geographical region of the company’s headquarters.
This method of sampling means there will be selections from each different group—the size of which is based on its proportion to the entire population. Researchers must ensure that the strata do not overlap. Every point in the population must only belong to one stratum, because they should be mutually exclusive . Overlapping strata would increase the likelihood that some data are included, thus skewing the sample.
Simple Random vs. Systematic Sampling
Systematic sampling entails selecting a single random variable that determines the interval of how the population items are selected. For example, if the number 37 was chosen, the 37th company on the list sorted by last name of the CEO would be selected by the sample. Then, the 74th (i.e., the next 37th) and the 111st (i.e. the next 37th after that) would be added as well.
Simple random sampling does not have a starting point; therefore, there is the risk that the population items selected at random may cluster. In our example there may be an abundance of CEOs with a last name that starts with the letter 'F.' Systematic sampling strives to even further reduce bias by ensuring that these clusters do not happen.
Simple Random vs. Cluster Sampling
Cluster sampling (also known as “multistage random sampling”) can occur as a one-stage or two-stage cluster. In the former, items within a population are put into comparable groupings (using our example, companies are grouped by year formed), then sampling occurs within these clusters.
Two-stage cluster sampling occurs when clusters are formed through random selection. The population is not clustered with other similar items. Sample items are then randomly selected within each cluster.
Simple random sampling does not cluster any population sets. Clustering (especially two-stage clustering) can enhance the randomness of sample items. In addition, cluster sampling may provide a deeper analysis on a specific snapshot of a population, which may or may not enhance the analysis.
Advantages and Disadvantages of Simple Random Samples
While simple random samples are easy to use, they do come with key disadvantages that can render the data useless.
Advantages of a Simple Random Sample
Ease of use represents the biggest advantage of simple random sampling. Unlike more complicated sampling methods, such as stratified random sampling and probability sampling, there is no need to divide the population into subpopulations or take any other additional steps before selecting members of the population at random.
A simple random sample is meant to be an unbiased representation of a group. It is considered a fair way to select a sample from a larger population, as every member of the population has an equal chance of getting selected. Therefore, it has less chance of sampling bias.
Disadvantages of a Simple Random Sample
A sampling error can occur with a simple random sample if the sample does not end up accurately reflecting the population it is supposed to represent. For example, in a simple random sample of 25 employees, it would be possible to draw 25 men even if the population consisted of 125 women, 125 men, and 125 nonbinary people.
For this reason simple random sampling is more commonly used when the researcher knows little about the population. If the researcher knows more, it is better to use a different sampling technique, such as stratified random sampling, which helps to account for the differences within the population, such as age, race, or gender.
Other disadvantages include the fact that for sampling from large populations, the process can be time-consuming and costly compared with other methods. Researchers may find that a project not worth the endeavor of its cost-benefit analysis does not generate positive results.
As every unit has to be assigned an identifying or sequential number prior to the selection process, this task may be difficult based on the method of data collection or size of the data set.
Simple Random Sampling
Each item within a population has an equal chance of being selected.
There is less of a chance of sampling bias, as every item is randomly selected.
It is easy and convenient for data sets already listed or digitally stored.
Incomplete population demographics may exclude certain groups from being sampled.
Random selection means the sample may not be truly representative of the population.
Depending on the data set size and format, random sampling may be a time-intensive process.
Why Is a Simple Random Sample Simple?
No easier method exists to extract a research sample from a larger population than simple random sampling. Selecting enough subjects completely at random from the larger population also yields a sample that can be representative of the group being studied.
What Are Some Drawbacks of a Simple Random Sample?
Among the disadvantages of this technique are difficulty gaining access to respondents that can be drawn from the larger population, greater time, greater costs, and the fact that bias can still occur under certain circumstances.
What Is a Stratified Random Sample?
A stratified random sample first divides the population into smaller groups, or strata, based on shared characteristics. Therefore, a stratified sampling strategy will ensure that members from each subgroup are included in the data analysis. Stratified sampling is used to highlight differences among groups in a population, as opposed to simple random sampling, which treats all members of a population as equal, with an equal likelihood of being sampled.
How Are Random Samples Used?
Using simple random sampling allows researchers to make generalizations about a specific population and leave out any bias. Using statistical techniques, inferences and predictions can be made about the population without having to survey or collect data from every individual in that population.
Simple random sampling is the most basic form of analyzing a population, allowing every item within it to have the same probability of being selected. There are also more complicated sampling methods that attempt to correct for possible shortcomings in the simple method. However, they don’t match the ease of simple random sampling for smaller populations.
Yale University. " Sampling. "
Business Research Methodology. " Simple Random Sampling ."
U.S. Department of Commerce: National Institute of Standards and Technology. " Appendix B. Random Number Tables. "
Calculator.net. " Random Number Generator. "
Microsoft. " RANDBETWEEN Function ."
Penn State University Eberly College of Science. " 8.1 - Systematic Sampling ."
:max_bytes(150000):strip_icc():format(webp)/Sample-Final-blue-6d294ab8024a4cdca8050cc58ab20c42.jpg)
- Terms of Service
- Editorial Policy
- Privacy Policy
DepEd Survey Research Guide: Designing Effective Assessments for Action Research
The Department of Education (DepEd) in the Philippines emphasizes evidence-based decision-making to enhance the quality of basic education. As part of this commitment, DepEd encourages its personnel to conduct action research to address classroom problems and educational issues. Survey research serves as a crucial tool for assessing situations and gathering data to inform action research projects. This article provides a thorough guide for DepEd personnel on designing and implementing survey research as part of the initial assessment phase of action research.
Table of Contents
The Role of Survey Research in Action Research
Survey research plays a vital role in the action research process, particularly during the “assessing the situation” stage (Stage 1 of the Action Research cycle). This method allows researchers to systematically collect information from a sample of individuals through standardized questionnaires or interviews. For DepEd personnel, survey research can serve several critical functions:
- Problem Identification: Surveys can help pinpoint specific issues or challenges within the classroom, school, or educational system.
- Baseline Data Collection: Gathering initial data on student performance, attitudes, or behaviors provides a starting point for measuring the impact of interventions.
- Needs Assessment: Surveys can reveal the requirements and preferences of students, teachers, or the broader school community.
- Exploration of Potential Solutions: By asking targeted questions, surveys can help identify possible interventions or approaches to address educational challenges.
The efficiency of survey research in gathering both quantitative and qualitative data from a relatively large number of participants makes it an invaluable tool for DepEd personnel engaged in action research.
Alignment with the Basic Education Research Agenda
When designing survey research, DepEd personnel should consider aligning their studies with the Basic Education Research Agenda (DepEd Order No. 39, s. 2016). This agenda outlines four main thematic areas:
- Teaching and Learning
- Child Protection
- Human Resource Development
Survey research can be tailored to address specific questions within these themes. For example:
- Teaching and Learning: Surveys on instructional strategies, curriculum implementation, or assessment practices
- Child Protection: Surveys on school safety, bullying prevention, or student well-being
- Human Resource Development: Surveys on teacher training needs, professional development effectiveness, or staff satisfaction
- Governance: Surveys on school leadership, community engagement, or policy implementation
By aligning survey research with these themes, DepEd personnel can ensure their studies contribute to the broader goals of the education system and may be eligible for funding through the Basic Education Research Fund (BERF).
Types of Survey Research
DepEd personnel should be familiar with two main types of survey research:
1. Cross-sectional Surveys
Cross-sectional surveys collect data from a sample at a single point in time. This approach is particularly useful for:
- Comparing different groups within a population (e.g., students across grade levels or schools)
- Measuring current attitudes, practices, or performance levels
- Establishing relationships between variables (e.g., study habits and academic performance)
- Providing a snapshot of the current educational landscape
Example: A cross-sectional survey might assess teachers’ technology use across different subject areas or grade levels within a school district.
2. Longitudinal Surveys
Longitudinal surveys gather data from the same sample over an extended period. This method is valuable for:
- Tracking changes in attitudes, behaviors, or performance over time
- Identifying trends or patterns in educational outcomes
- Evaluating the long-term impact of interventions or programs
- Studying developmental changes in students
Example: A longitudinal survey could track student attitudes towards reading throughout their elementary school years.
While cross-sectional surveys are often more practical for short-term action research projects due to time and resource constraints, longitudinal surveys can provide valuable insights for longer-term studies or school-wide initiatives.
Detailed Steps in Designing Survey Research
1. define the research objectives.
Begin by clearly stating the purpose of your survey and the specific questions you want to answer. This step is crucial as it guides all subsequent decisions in the research process. Consider the following when defining your objectives:
- Specificity: Ensure your objectives are clear and focused.
- Measurability: Frame objectives in a way that allows for quantifiable results.
- Relevance: Align objectives with the broader goals of your action research and the Basic Education Research Agenda.
Examples of well-defined research objectives:
- To determine the percentage of students who have access to digital devices for learning at home
- To identify the most common challenges teachers face when implementing project-based learning
- To measure the correlation between parental involvement and student academic performance
2. Identify the Target Population and Sampling Method
Determine who you need to survey to achieve your research objectives. This step involves several key considerations:
a) Define the population:
- Who are you interested in studying? (e.g., all students in a school, teachers of a specific subject, parents of elementary students)
- What are the characteristics of this population?
b) Decide on sampling:
- Will you survey the entire population or select a sample?
- If selecting a sample, what sampling method will you use?
c) Choose a sampling method:
- Random sampling: Every member of the population has an equal chance of being selected.
- Stratified sampling: The population is divided into subgroups (strata) based on certain characteristics, and samples are taken from each stratum.
- Cluster sampling: The population is divided into clusters (e.g., schools), and a random sample of clusters is selected.
- Convenience sampling: Participants are selected based on their accessibility and willingness to participate.
d) Determine sample size:
- Consider factors such as population size, desired confidence level, and margin of error.
- Use sample size calculators or consult with statisticians to ensure your sample is large enough for reliable results.
3. Choose the Survey Method
Select the most appropriate method for administering your survey, considering factors such as cost, time, accessibility, and the nature of the information you need to collect. Common survey methods include:
a) Paper-and-pencil questionnaires:
- Advantages: Familiar format, no technology required, can be administered in group settings
- Disadvantages: Time-consuming to distribute and collect, potential for data entry errors
b) Online surveys:
- Advantages: Cost-effective, easy to distribute, automatic data collection
- Disadvantages: Requires internet access, potential for low response rates
c) Face-to-face interviews:
- Advantages: High response rates, ability to clarify questions, opportunity for in-depth responses
- Disadvantages: Time-consuming, potential for interviewer bias
d) Telephone interviews:
- Advantages: Faster than face-to-face interviews, can reach geographically dispersed participants
- Disadvantages: Limited to those with phone access, potential for response bias
Consider using a mixed-method approach, combining two or more survey methods to overcome the limitations of any single method and improve the validity of your results.
4. Develop the Survey Instrument
Create your questionnaire or interview guide, paying careful attention to the following elements:
a) Types of questions:
- Closed-ended questions: Provide predetermined response options (e.g., multiple choice, Likert scales)
- Open-ended questions: Allow respondents to provide their own answers
- Consider using a mix of both types to gather both quantitative and qualitative data
b) Question wording:
- Use clear, concise language
- Avoid leading or biased questions
- Ensure questions are relevant to your research objectives
c) Question order:
- Start with easy, non-threatening questions to build rapport
- Group related questions together
- Place sensitive questions towards the end of the survey
d) Response options:
- For closed-ended questions, provide mutually exclusive and exhaustive options
- Consider using Likert scales for attitude or opinion questions (e.g., strongly disagree to strongly agree)
- Include “Not applicable” or “Don’t know” options when appropriate
e) Survey length and formatting:
- Keep the survey as short as possible while still meeting your research objectives
- Use a clear, easy-to-read layout
- Provide clear instructions for completing the survey
5. Pilot Test the Survey
Before full implementation, test your survey instrument with a small group of respondents similar to your target population. This crucial step helps identify:
- Unclear or ambiguous questions
- Issues with survey length or format
- Potential problems with data analysis
- Unforeseen challenges in survey administration
During the pilot test:
- Administer the survey to a small group (typically 5-10 individuals)
- Ask participants to provide feedback on their experience
- Analyze the pilot data to ensure questions are yielding useful information
- Revise the survey based on feedback and analysis results
6. Administer the Survey
Implement your survey according to your chosen method. Consider the following factors:
a) Timing of survey administration:
- Choose a time when participants are likely to be available and receptive
- Avoid periods of high stress (e.g., exam weeks) or holidays
b) Instructions for respondents:
- Provide clear, written instructions at the beginning of the survey
- Include information about the purpose of the survey and how results will be used
- Specify the estimated time required to complete the survey
c) Follow-up procedures:
- Send reminders to non-respondents to improve response rates
- Consider offering incentives for participation (if appropriate and approved)
d) Data collection period:
- Set a specific timeframe for data collection
- Monitor response rates and adjust strategies if necessary
7. Analyze the Data
Process and analyze the collected data using appropriate statistical methods or qualitative analysis techniques:
a) Quantitative data analysis:
- Use descriptive statistics (e.g., means, frequencies, percentages) to summarize data
- Apply inferential statistics (e.g., t-tests, ANOVA, regression) to test hypotheses and examine relationships between variables
- Consider using statistical software like SPSS, R, or Excel for complex analyses
b) Qualitative data analysis:
- Code open-ended responses to identify themes and patterns
- Use content analysis techniques to categorize and interpret qualitative data
- Consider using qualitative data analysis software like NVivo or Atlas.ti for large datasets
c) Data cleaning:
- Check for and handle missing data
- Identify and address outliers or inconsistent responses
8. Interpret and Report Results
Draw conclusions based on your analysis and prepare a comprehensive report summarizing your findings, implications, and recommendations for action:
a) Structure your report:
- Executive summary
- Introduction and background
- Methodology
- Conclusions and recommendations
- Appendices (e.g., survey instrument, detailed statistical tables)
b) Present findings clearly:
- Use tables, charts, and graphs to visualize data
- Provide both statistical results and practical interpretations
- Address each research objective
c) Discuss implications:
- Relate findings to existing literature and theories
- Explain how results can inform educational practice or policy
d) Offer recommendations:
- Suggest specific actions based on your findings
- Propose areas for further research or investigation
Ethical Considerations in Survey Research
DepEd personnel must adhere to strict ethical guidelines when conducting survey research to protect participants and ensure the integrity of the research process:
- Informed Consent: Obtain voluntary, informed consent from all participants (and parents/guardians for minors) before conducting the survey. Provide clear information about:
- The purpose of the study
- What participation involves
- How data will be used and protected
- Any potential risks or benefits
- Confidentiality and Anonymity: Ensure the privacy of respondents by:
- Using anonymous surveys when possible
- Securely storing and handling data
- Reporting results in aggregate form to prevent identification of individuals
- Avoiding Harm: Design surveys to minimize any potential distress or discomfort to participants:
- Avoid sensitive or intrusive questions unless absolutely necessary
- Provide support resources if discussing potentially upsetting topics
- Voluntary Participation: Make it clear that participation is voluntary and that participants can:
- Skip any questions they are uncomfortable answering
- Withdraw from the study at any time without penalty
- Data Protection: Implement measures to protect collected data:
- Use secure, password-protected storage systems
- Limit access to raw data to authorized personnel only
- Have a plan for data destruction after the research is complete
- Transparency: Be open about the research process and findings:
- Share results with participants and stakeholders
- Be honest about any limitations or potential biases in the study
- Cultural Sensitivity: Ensure that survey content and administration methods are appropriate for the cultural context of the participants:
- Consider language and literacy levels
- Be aware of cultural norms and sensitivities
- Institutional Approval: Obtain necessary approvals from DepEd and any relevant ethics committees before conducting the research.
Special Considerations for Indigenous Peoples Education
When conducting survey research involving Indigenous Cultural Communities (ICCs), DepEd personnel must adhere to additional ethical considerations and guidelines:
- Free, Prior, and Informed Consent: Obtain consent from the community through its recognized representatives or elders involved in Indigenous Peoples Education (IPEd) implementation.
- Cultural Respect: Ensure that survey questions and methods respect the community’s cultural practices, beliefs, and traditions.
- Language: Provide surveys in the native language of the community when possible, or use qualified translators.
- Benefit Sharing: Clearly communicate how the research will benefit the community and involve them in the research process where appropriate.
- Intellectual Property Rights: Recognize the community’s rights to their traditional knowledge and cultural expressions.
- Community Validation: Allow the community to review and validate research findings before publication or dissemination.
- Capacity Building: Where possible, involve community members in the research process to build local research capacity.
Challenges and Limitations of Survey Research
While survey research is a powerful tool for assessing situations, DepEd personnel should be aware of its potential limitations and challenges:
- Response Bias: Participants may provide socially desirable answers rather than their true opinions. To mitigate this:
- Use anonymous surveys when possible
- Frame questions in a neutral manner
- Include social desirability scales in your survey
- Low Response Rates: This can lead to non-representative samples and biased results. To improve response rates:
- Send reminders to non-respondents
- Keep surveys concise and engaging
- Offer incentives for participation (if appropriate)
- Use mixed-mode surveys (e.g., offering both online and paper options)
- Self-reported Data: Responses may not always accurately reflect actual behaviors or attitudes. To address this:
- Use validated scales when possible
- Triangulate data with other sources (e.g., observations, records)
- Include questions that check for consistency in responses
- Limited Depth: Surveys may not capture the full complexity of educational issues. Consider:
- Including open-ended questions for more detailed responses
- Following up surveys with focus groups or interviews for in-depth exploration
- Using mixed methods research designs
- Misinterpretation of Questions: Respondents may understand questions differently than intended. To minimize this:
- Use clear, simple language
- Provide definitions for key terms
- Pilot test the survey and revise based on feedback
- Sampling Errors: The sample may not accurately represent the population. To reduce sampling errors:
- Use appropriate sampling techniques
- Ensure adequate sample size
- Weight data if necessary to adjust for under- or over-represented groups
- Technological Issues: For online surveys, technical problems can affect response rates and data quality. To address this:
- Test the survey on multiple devices and browsers
- Provide technical support for respondents
- Have a backup method for survey administration
- Longitudinal Attrition: For longitudinal surveys, participants may drop out over time, leading to biased results. To minimize attrition:
- Maintain regular contact with participants
- Provide incentives for continued participation
- Plan for attrition in your initial sample size calculations
Integrating Survey Research with Learning Action Cells (LACs)
Learning Action Cells (LACs) provide an excellent platform for collaborative learning and problem-solving among DepEd personnel. Survey research can be effectively integrated into LAC sessions in several ways:
- Collaborative Survey Design: Use LAC sessions to collaboratively design surveys, ensuring that multiple perspectives are considered.
- Peer Review: Present draft surveys during LAC meetings for peer review and feedback.
- Data Analysis Workshops: Conduct LAC sessions focused on analyzing and interpreting survey data, promoting collective understanding and insights.
- Action Planning: Use survey results as a basis for action planning discussions in LAC meetings.
- Sharing Best Practices: Dedicate LAC sessions to sharing experiences and best practices in survey research design and implementation.
- Capacity Building: Organize LAC sessions focused on developing survey research skills among team members.
Technology Integration in Survey Research
Given DepEd’s focus on Information and Communications Technology (ICT) in education, integrating technology into survey research can enhance efficiency and effectiveness:
- Online Survey Platforms: Utilize user-friendly platforms like Google Forms, SurveyMonkey, or Qualtrics for creating and distributing online surveys.
- Mobile Data Collection: Use mobile apps for data collection, especially useful for field-based surveys.
- Data Analysis Software: Employ statistical software like SPSS, R, or even Excel for data analysis and visualization.
- Cloud Storage: Use secure cloud storage solutions for collaborative data management and analysis.
- Virtual Meeting Platforms: Conduct virtual focus groups or interviews using platforms like Zoom or Google Meet when face-to-face meetings are not feasible.
- Automated Reporting Tools: Utilize tools that can generate automated reports from survey data, saving time in the analysis phase.
Local Contextualization of Survey Research
Adapting survey research to the specific contexts of different regions and schools in the Philippines is crucial for generating relevant and actionable insights:
- Language Adaptation: Translate surveys into local languages or dialects as needed, ensuring that questions are culturally appropriate and easily understood.
- Cultural Sensitivity: Consider local customs, beliefs, and practices when designing survey questions and administration methods.
- Regional Issues: Include questions that address specific educational challenges or priorities in the local area.
- Community Involvement: Engage local community leaders or stakeholders in the survey design process to ensure relevance and buy-in.
- Seasonal Considerations: Plan survey administration around local events, agricultural seasons, or other factors that might affect participation.
- Resource Availability: T ailor survey methods to the available resources and infrastructure in each location (e.g., using paper surveys in areas with limited internet access).
Capacity Building for DepEd Personnel in Survey Research
To enhance the quality of survey research conducted by DepEd personnel, consider the following capacity-building strategies:
- Training Workshops: Organize regular workshops on survey design, data analysis, and research ethics.
- Mentorship Programs: Pair experienced researchers with novice survey designers for ongoing support and guidance.
- Online Learning Resources: Develop or curate online modules and resources on survey research methodologies.
- Collaborative Research Projects: Encourage cross-school or cross-division research projects to promote peer learning.
- Conference Participation: Support attendance at research conferences to expose personnel to current trends and best practices in survey research.
- Partnerships with Higher Education Institutions: Collaborate with universities for advanced training and support in survey research methodologies.
Policy Implications of Survey Research
Survey research can significantly inform DepEd policy at various levels:
- School-level Policies: Use survey results to inform school improvement plans, resource allocation, and instructional strategies.
- Division-level Policies: Aggregate survey data across schools to identify division-wide trends and inform division-level initiatives.
- Regional Policies: Use survey research to understand regional disparities and tailor policies to address specific regional needs.
- National Policies: Incorporate survey findings into national policy discussions, curriculum development, and teacher training programs.
- Evidence-based Decision Making: Encourage the use of survey data in all levels of educational decision-making to promote evidence-based practices.
- Policy Evaluation: Use surveys as a tool for evaluating the implementation and effectiveness of existing policies.
Survey research is an invaluable method for DepEd personnel to assess situations and gather data to inform action research projects. By following a systematic approach to survey design and implementation, educators can collect reliable and valid information to guide their decision-making and improve educational practices. The process requires careful planning, from defining clear research objectives to analyzing and reporting results.
While surveys offer many advantages, including the ability to collect data from large samples efficiently, it is essential to consider their limitations and potential challenges. By addressing these issues through careful design, implementation, and analysis, DepEd personnel can maximize the benefits of survey research.
As with any research method, it is crucial to consider ethical implications when using surveys in educational settings. Protecting participants’ rights, ensuring confidentiality, and maintaining research integrity should be paramount throughout the research process.
By applying the principles and strategies outlined in this guide, DepEd personnel can conduct high-quality survey research that provides valuable insights to support evidence-based improvements in Philippine basic education. This approach not only enhances the effectiveness of action research projects but also contributes to the broader goal of continuous improvement in educational practices and outcomes.
The integration of survey research with Learning Action Cells, technology, and local contextualization further enhances its potential impact. As DepEd continues to build capacity in research among its personnel and align studies with the Basic Education Research Agenda, survey research will play an increasingly important role in shaping the future of education in the Philippines.
Copyright Notice :
This article, “DepEd Survey Research Guide: Designing Effective Assessments for Action Research,” was authored by Mark Anthony Llego and published on August 9, 2024.
Mark Anthony Llego
Mark Anthony Llego, a visionary from the Philippines, founded TeacherPH in October 2014 with a mission to transform the educational landscape. His platform has empowered thousands of Filipino teachers, providing them with crucial resources and a space for meaningful idea exchange, ultimately enhancing their instructional and supervisory capabilities. TeacherPH's influence extends far beyond its origins. Mark's insightful articles on education have garnered international attention, featuring on respected U.S. educational websites. Moreover, his work has become a valuable reference for researchers, contributing to the academic discourse on education.
Leave a Comment Cancel reply
Can't find what you're looking for.
We are here to help - please use the search box below.
- Download PDF
- CME & MOC
- Share X Facebook Email LinkedIn
- Permissions
Modernizing the Data Infrastructure for Clinical Research to Meet Evolving Demands for Evidence
- 1 Verily Life Sciences, South San Francisco, California
- 2 Center for Biostatistics & Qualitative Methodology, University of Pittsburgh School of Medicine, Pittsburgh, Pennsylvania
- 3 Bakar Computational Health Sciences Institute, University of California, San Francisco
- 4 Center for Data-Driven Insights and Innovation, University of California Health, Oakland
- 5 Faculty of Health Sciences, McMaster University, Hamilton, Ontario, Canada
- 6 Departments of Surgery and Radiology and Institute for Health Policy Studies, University of California, San Francisco
- 7 Anesthesiology and Critical Care, University of Pennsylvania Perelman School of Medicine, Philadelphia
- 8 Biogen, Boston, Massachusetts
- 9 Johns Hopkins Bloomberg School of Public Health, Baltimore, Maryland
- 10 Yale University School of Medicine, New Haven, Connecticut
- 11 National Institute for Health and Care Research (NIHR) Health and Social Care Delivery Research Programme, London, United Kingdom
- 12 Intensive Care National Audit & Research Centre (ICNARC), London, United Kingdom
- 13 Highlander Health, Dallas, Texas
Importance The ways in which we access, acquire, and use data in clinical trials have evolved very little over time, resulting in a fragmented and inefficient system that limits the amount and quality of evidence that can be generated.
Observations Clinical trial design has advanced steadily over several decades. Yet the infrastructure for clinical trial data collection remains expensive and labor intensive and limits the amount of evidence that can be collected to inform whether and how interventions work for different patient populations. Meanwhile, there is increasing demand for evidence from randomized clinical trials to inform regulatory decisions, payment decisions, and clinical care. Although substantial public and industry investment in advancing electronic health record interoperability, data standardization, and the technology systems used for data capture have resulted in significant progress on various aspects of data generation, there is now a need to combine the results of these efforts and apply them more directly to the clinical trial data infrastructure.
Conclusions and Relevance We describe a vision for a modernized infrastructure that is centered around 2 related concepts. First, allowing the collection and rigorous evaluation of multiple data sources and types and, second, enabling the possibility to reuse health data for multiple purposes. We address the need for multidisciplinary collaboration and suggest ways to measure progress toward this goal.
Read More About
Franklin JB , Marra C , Abebe KZ, et al. Modernizing the Data Infrastructure for Clinical Research to Meet Evolving Demands for Evidence. JAMA. Published online August 05, 2024. doi:10.1001/jama.2024.0268
Manage citations:
© 2024
Artificial Intelligence Resource Center
Cardiology in JAMA : Read the Latest
Browse and subscribe to JAMA Network podcasts!
Others Also Liked
Select your interests.
Customize your JAMA Network experience by selecting one or more topics from the list below.
- Academic Medicine
- Acid Base, Electrolytes, Fluids
- Allergy and Clinical Immunology
- American Indian or Alaska Natives
- Anesthesiology
- Anticoagulation
- Art and Images in Psychiatry
- Artificial Intelligence
- Assisted Reproduction
- Bleeding and Transfusion
- Caring for the Critically Ill Patient
- Challenges in Clinical Electrocardiography
- Climate and Health
- Climate Change
- Clinical Challenge
- Clinical Decision Support
- Clinical Implications of Basic Neuroscience
- Clinical Pharmacy and Pharmacology
- Complementary and Alternative Medicine
- Consensus Statements
- Coronavirus (COVID-19)
- Critical Care Medicine
- Cultural Competency
- Dental Medicine
- Dermatology
- Diabetes and Endocrinology
- Diagnostic Test Interpretation
- Drug Development
- Electronic Health Records
- Emergency Medicine
- End of Life, Hospice, Palliative Care
- Environmental Health
- Equity, Diversity, and Inclusion
- Facial Plastic Surgery
- Gastroenterology and Hepatology
- Genetics and Genomics
- Genomics and Precision Health
- Global Health
- Guide to Statistics and Methods
- Hair Disorders
- Health Care Delivery Models
- Health Care Economics, Insurance, Payment
- Health Care Quality
- Health Care Reform
- Health Care Safety
- Health Care Workforce
- Health Disparities
- Health Inequities
- Health Policy
- Health Systems Science
- History of Medicine
- Hypertension
- Images in Neurology
- Implementation Science
- Infectious Diseases
- Innovations in Health Care Delivery
- JAMA Infographic
- Law and Medicine
- Leading Change
- Less is More
- LGBTQIA Medicine
- Lifestyle Behaviors
- Medical Coding
- Medical Devices and Equipment
- Medical Education
- Medical Education and Training
- Medical Journals and Publishing
- Mobile Health and Telemedicine
- Narrative Medicine
- Neuroscience and Psychiatry
- Notable Notes
- Nutrition, Obesity, Exercise
- Obstetrics and Gynecology
- Occupational Health
- Ophthalmology
- Orthopedics
- Otolaryngology
- Pain Medicine
- Palliative Care
- Pathology and Laboratory Medicine
- Patient Care
- Patient Information
- Performance Improvement
- Performance Measures
- Perioperative Care and Consultation
- Pharmacoeconomics
- Pharmacoepidemiology
- Pharmacogenetics
- Pharmacy and Clinical Pharmacology
- Physical Medicine and Rehabilitation
- Physical Therapy
- Physician Leadership
- Population Health
- Primary Care
- Professional Well-being
- Professionalism
- Psychiatry and Behavioral Health
- Public Health
- Pulmonary Medicine
- Regulatory Agencies
- Reproductive Health
- Research, Methods, Statistics
- Resuscitation
- Rheumatology
- Risk Management
- Scientific Discovery and the Future of Medicine
- Shared Decision Making and Communication
- Sleep Medicine
- Sports Medicine
- Stem Cell Transplantation
- Substance Use and Addiction Medicine
- Surgical Innovation
- Surgical Pearls
- Teachable Moment
- Technology and Finance
- The Art of JAMA
- The Arts and Medicine
- The Rational Clinical Examination
- Tobacco and e-Cigarettes
- Translational Medicine
- Trauma and Injury
- Treatment Adherence
- Ultrasonography
- Users' Guide to the Medical Literature
- Vaccination
- Venous Thromboembolism
- Veterans Health
- Women's Health
- Workflow and Process
- Wound Care, Infection, Healing
- Register for email alerts with links to free full-text articles
- Access PDFs of free articles
- Manage your interests
- Save searches and receive search alerts
New Modification of Ranked Set Sampling for Estimating Population Mean: Neutrosophic Median Ranked Set Sampling with an Application to Demographic Data
- Research Article
- Open access
- Published: 08 August 2024
- Volume 17 , article number 210 , ( 2024 )
Cite this article
You have full access to this open access article
- Anamika Kumari ORCID: orcid.org/0000-0001-7411-7920 1 ,
- Rajesh Singh 1 na1 &
- Florentin Smarandache 2 na1
21 Accesses
Explore all metrics
The study addressed the limitations of classical statistical methods when dealing with ambiguous data, emphasizing the importance of adopting neutrosophic statistics as a more effective alternative. Classical methods falter in managing uncertainty inherent in such data, necessitating a shift towards methodologies like neutrosophic statistics. To address this gap, the research introduced a novel sampling approach called “neutrosophic median ranked set sampling” and incorporated neutrosophic estimators tailored for estimating the population mean in the presence of ambiguity. This modification aims to address the inherent challenges associated with estimating the population mean when dealing with neutrosophic data. The methods employed involved modifying traditional ranked set sampling techniques to accommodate neutrosophic data characteristics. Additionally, neutrosophic estimators were developed to leverage auxiliary information within the framework of median-ranked set sampling, enhancing the accuracy of population mean estimation under uncertain conditions. The methods employed involved modifying traditional ranked set sampling techniques to accommodate neutrosophic data characteristics. Bias and mean squared error equations for the suggested estimators were provided, offering insights into their theoretical underpinnings. To illustrate the effectiveness and practical applications of the proposed methodology and estimators, a numerical demonstration and simulation study have been conducted using the R programming language. The key results highlighted the superior performance of the proposed estimators compared to existing alternatives, as demonstrated through comprehensive evaluations based on mean squared error and percentage relative efficiency criteria. The conclusions drawn underscored the effectiveness of the neutrosophic median ranked set sampling approach and suggested estimators in estimating the population mean under conditions of uncertainty, particularly when utilizing neutrosophic auxiliary information and validated real-life applicability. The methodology and estimators presented in the study were shown to yield interval-based results, providing a more realistic representation of uncertainty associated with population parameters. This interval estimation, coupled with minimum mean squared error considerations, enhanced the efficacy of the estimators in determining population mean values. The novelty of the work lies in its introduction of a tailored sampling approach and estimators designed specifically for neutrosophic data, filling a significant gap in the literature. By extending classical statistics to accommodate ambiguity, the study offers a substantial advancement in statistical methodology, particularly in domains where precise data is scarce and uncertainty is prevalent. Furthermore, the empirical validation through numerical demonstrations and simulation studies using the R programming language adds robustness to the proposed methodology and contributes to its practical applicability.
Similar content being viewed by others
Mean Estimation Based on FWA Using Ranked Set Sampling with Single and Multiple Rankers
Combining the Information of Multiple Ranker in Ranked Set Sampling with Fuzzy Set Approach
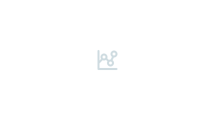
Ratio estimation using multistage median ranked set sampling approach
Avoid common mistakes on your manuscript.
1 Introduction
Sampling is a crucial practice for a variety of reasons, such as cost and time constraints. In sampling theory, the goal of estimation procedures is to enhance the effectiveness of estimators for population parameters while minimizing sampling errors. To achieve this, auxiliary information is utilized to improve estimator efficiency, and this information can be incorporated at various stages of the process. When highly correlated auxiliary information is not readily available, it can be gathered from previous surveys. Estimation techniques like ratio, product, and regression are commonly employed in this context.
For instance, [ 25 ] introduced a modified ratio estimator that incorporates the coefficient of variation of auxiliary information. References [ 3 , 9 , 14 , 16 , 19 , 21 ] have also proposed population parameter estimation methods using auxiliary information. However, our focus here is on ranked set sampling.
Efforts in sampling continually strive for improvements in estimator efficiency, cost-effectiveness, simplicity, and time savings. Ranked set sampling (RSS) offers a superior alternative to simple random sampling (SRS) in various fields, including medicine, agriculture, earth sciences, statistics, and mathematics, especially when measurements are cumbersome, time-consuming, or expensive. The RSS technique was initially described for population mean estimation by [ 13 ], and the mathematical theory behind RSS was provided by [ 35 ]. Dell and Cultter [ 7 ] demonstrated that under both perfect and imperfect ranking scenarios, the mean estimate in RSS remains unbiased.
Numerous researchers, such as [ 2 , 5 , 6 , 8 , 10 , 12 , 17 , 20 , 33 ] have contributed to the field of RSS. For recent work, one can prefer [ 4 , 23 , 36 , 37 ].
Only when the data have a symmetric distribution, RSS provide an efficient estimate for the estimation of population parameters; otherwise, the results will be less favorable. To acquire the most accurate parameter estimates, various RSS sampling methods are available. These include double RSS, median ranked set sampling (MRSS), quantile RSS, extreme RSS, a combination of median and extreme RSS, except extreme RSS, and more. For asymmetric one can go for MRSS. In this context, our focus will be on MRSS. [ 15 ] originally introduced MRSS, and subsequently, [ 18 ] presented a ratio estimator under MRSS. Al-Omari [ 1 ] provided ratio estimation under both SRS and MRSS, while [ 11 ] contributed a difference-cum-ratio and exponential-type estimator under MRSS.
Classical RSS deals solely with precise data, assuming no uncertainty exists. However, in practice, data can be uncertain and imprecise, containing sets or intervals. To address such situations, fuzzy logic is a valuable tool that handles data with imprecision. When analyzing fuzzy data, fuzzy statistics are employed, with ambiguous, uncertain, unclear, or imprecise characteristics. Yet, they do not account for the degree of indeterminacy inherent in the data. Neutrosophic statistics, an extension of fuzzy logic, offer a way to measure both indeterminacy and determinate aspects of uncertain or imprecise data. When dealing with data that contains indeterminacy, neutrosophic statistics are employed. Neutrosophic statistics expand upon classical statistics and encompass fuzzy and intuitionistic statistics. Neutrosophy is applicable when observations in a population or sample lack precision, are indeterminate, or are vague. Some examples of neutrosophic data include district-wise water level measurements with intervals, variations in machinery part sizes due to measurement errors, and day-wise temperature measurements resulting in interval-type data.
The concept of neutrosophy was initially introduced by [ 26 , 27 , 28 , 29 , 30 , 31 , 32 ], and extensive literature on neutrosophic sets, logic, and statistics can be found in his works. In the realm of sampling theory, [ 34 ] recently addressed the estimation of population parameters under a neutrosophic environment, introducing neutrosophic ratio-type estimators for population means under SRS. One can also prefer [ 22 , 24 ] for neutrosophic estimators. However, there has been little focus on neutrosophic RSS (NRSS) for estimating population parameters. References [ 36 , 37 ] proposed NRSS and a generalized estimator for the computation of the population mean.
Efficiency improvements in sampling framework and estimators are a constant objective in sampling. In this context, we propose an enhanced novel sampling framework neutrosophic median ranked set sampling (NMRSS) and improved NMRSS estimators for population mean estimation, with a particular emphasis on minimizing MSE and enhancing precision.
There is not much emphasis on the problem of estimation when dealing with indeterminate, vague, imprecise, set-type, or interval-type data that is characteristic of neutrosophic data, which is more prevalent in real-world scenarios than crisp data. It is a well-proven theory through a literature review that RSS is an improvement over SRS and MRSS is most efficient when data do not follow symmetric distribution. There is no work in the estimation of parameters using the MRSS technique when there is the presence of errors, unclear, inaccurate, indeterminate, vague, imprecise, set-type, or interval-type data. Hence, our research is novel and significant in the field of sampling where we came with neutrosophic data and SRS and RSS are not efficient sampling techniques to work with. Our work may be more desirable than other work in several real situations like agriculture sciences, mathematical sciences, biological sciences, poultry, business, economics, commerce, social sciences, etc.
The efficacy of the innovative sampling framework, neutrosophic median ranked set Sampling (NMRSS), alongside enhanced NMRSS estimators for population mean estimation, was validated through empirical analysis using both real and simulated population data. Results unequivocally demonstrated the superiority of the recommended estimators over existing ones, showcasing lower MSEs and higher PREs, with \(t_{p2}\) emerging as the optimal estimator. As sample sizes and correlation coefficients increased, the MSE decreased and PRE increased for the recommended estimator, indicating its robustness and efficiency. NMRSS estimators surpassed their counterparts NSRS, with RSS proving to be a superior replacement for SRS. Similarly, under NRSS, MRSS outperformed RSS, particularly when data exhibited non-symmetric distributions. Comparative analysis revealed that MSEs and PREs of suggested estimators obtained through classical MRSS fell between those obtained using NMRSS, suggesting the latter’s superiority. The study underscored the inadequacy of classical RSS in handling vague or indeterminate data, emphasizing NMRSS as a superior method for estimating uncertain or interval data.
It can be computationally burdensome to analyze neutrosophic data, particularly when processing large datasets with advanced models or algorithms. It can be difficult to interpret analysis based on neutrosophic data, particularly when dealing with contradicting or ambiguous results. Compared to other approaches or even just SRS, RSS implementation can be more complicated. Before sampling, the population must be carefully ranked and sorted, which can take time and require specialized knowledge.
Our study is designed as follows: Sect. 1 presents an introduction, and Sect. 2 outlines motivation, needs, and research gaps. Section 3 outlines the modified and novel sampling method, neutrosophic median ranked set sampling method. Section 4 presents existing NMRSS estimators. Section 5 presents proposed NMRSS estimators, while Sect. 6 presents an application with the help of empirical study using demographic data, and Sect. 7 offers a simulation study using artificial data for different sets of values. Section 8 is dedicated to a discussion, and Sect. 9 covers concluding remarks and future research directions.
2 Motivation, Need and Research Gap
This article’s main objective is to introduce a novel approach known as the “neutrosophic median ranked set sampling” for dealing with interval-type or neutrosophic data. Our study focuses on sampling theory, marking the first instance of proposing an MRSS technique tailored to neutrosophic data, along with the development of NMRSS estimators for population mean estimation. This marks a significant step in expanding the field of sampling theory by comparing these estimators with existing neutrosophic methods such as ratio, product, and regression estimators. MRSS is considered a superior alternative to SRS and RSS when data does not follow symmetric distribution, making it an attractive avenue for further exploration in the context of NMRSS.
Several factors drive our exploration of NMRSS and its associated estimators for population parameter estimation. A primary motivation is to introduce MRSS and MRSS estimators in a neutrosophic setting. Previous research in survey sampling has predominantly focused on clear, well-defined data, while classical sampling methods yield precise results, albeit with potential risks of inaccuracies, overestimations, or underestimations. However, classical methods fall short when handling set-type or undetermined data, characteristic of neutrosophic data, which is more prevalent in real-world scenarios than crisp data. Thus, there is a growing need for additional neutrosophic statistical techniques. Traditional statistical approaches are ill-suited to compute accurate estimates of unknown parameters when dealing with indeterminate, vague, imprecise, or interval-type data. Neutrosophic statistics serve as a suitable replacement for classical statistics in such scenarios.
The necessity to close the gap between classical and neutrosophic statistics, and inspired by [ 36 , 37 ] work, our work introduces a new sampling technique NMRSS, and enhanced NMRSS estimators for population mean estimation. Despite thorough research in the field, we found no prior studies in survey sampling that addressed the estimation of population means in the presence of auxiliary variables using neutrosophic data under MRSS. This research represents a significant step toward filling this gap and contributes to the evolving domain of neutrosophic statistics.
It has been well established by multiple authors that RSS is a more suitable option than SRS when dealing with cumbersome, expensive, or time-consuming measurements and MRSS is a better option than RSS when data does not follow the symmetric distribution. The challenges associated with measurements in a neutrosophic context exacerbate these problems. Therefore, our research introduces an NMRSS method to enhance the accuracy of the population mean estimators in this unique context.
3 Sampling Methodology
Numerous methods can be used to display the neutrosophic observations, and the neutrosophic numbers may include an unknown interval [ a , b ]. We are describing neutrosophic values as \(Z_{rssN} \equiv Z_{rssL} + Z_{rssU} I_{rssN}\) with \(I_{rssN} \in [I_{rssL}, I_{rssU} ] \) , N is here to represent the neutrosophic number. Hence, our neutrosophic observations will lie in an interval \(Z_{rssN} \in [a, b]\) , where ‘a’ and ‘b’ denote the neutrosophic data’s lower and upper values.
In MRSS, a small subset of randomly chosen population units are measured after they have been ranked solely based on observation or experience. In the context of MRSS, m independent sets of random samples, each consisting of m units, are drawn from the overall population. Every unit within a set has an equal probability of selection. The members of each random set are then arranged in order based on the characteristics of the auxiliary variable. Then, if the sample size m is even, identify \((\frac{m}{2})\) th smallest ranked unit from the first \((\frac{m}{2})\) sets, and select \((\frac{m+2}{2})\) th smallest ranked unit from the other \((\frac{m}{2})\) sets. In case when the sample size m is odd, identify \((\frac{m+1}{2})\) th smallest ranked unit from all sets for actual quantification. Throughout the process, rm (= n ) units have been acquired as this cycle is replicated r times.
The method of NMRSS consists of selecting \(m_{N} \in [m_{L}, m_{U} ]\) bivariate random samples of size \(m _{N} \in [m_{L}, m_{U}]\) from a population of size N, and then ranking inside each sample concerning for auxiliary variable \(X_{N} \in [X_{L}, X_{U} ]\) associated with \(Y_{N} \in [Y_{L}, Y_{U} ]\) . The book by [ 32 ] entitled “Introduction to Neutrosophic Statistics” will be the basis for the ranking of the neutrosophic number. To show the process of ranking, we are utilizing here two sets as \(X_{1N} \in [X_{1L}, X_{1U} ]\) and \(X_{2N} \in [X_{2L}, X_{2U} ]\) , also their mid-points are as \(X_{1\mathrm{{mid}}N} = [X_{1L} + X_{1U} ]/2\) and \(X_{2\mathrm{{mid}}N} = [X_{2\,L} + X_{2U} ]/2\) . The ordering of neutrosophic numbers \(m_{N} \in [m_{L}, m_{U} ]\) can be done as \(X_{1N} \in [X_{1L}, X_{1U} ]\) will be less than \(X_{2N} \in [X_{2L}, X_{2U} ]\) if \(X_{1\mathrm{{mid}}N} \le X_{2\mathrm{{mid}}N}\) , also if both are same that is \(X_{1\mathrm{{mid}}N} = X_{2\mathrm{{mid}}N}\) then we will compare or see by \(X_{1L} \le X_{2L}\) . Further, if again \(X_{1\,L} = X_{2\,L}\) then this implies \(X_{1U} = X_{2U}\) and hence \(X_{1N} \in [X_{1\,L}, X_{1U} ] = X_{2N} \in [X_{2\,L}, X_{2U} ]\) , so the neutrosophic number ranking will be carried out in this manner.
In the whole NMRSS structure if \(m_{N} \in [m_{L}, m_{U} ]\) is even, we count \((\frac{m_N}{2}){th}\) smallest rank unit of the first \((\frac{m_N}{2})\) data of set size \(m_{N} \in [m_{L}, m_{U} ]\) , for the first \((\frac{m_N}{2})\) measurement units in the entire NMRSS structure, and then we scrap the remaining units. In a similar manner, we count the \((\frac{m_N+2}{2}){th}\) smallest observation from the remaining \((\frac{m_N}{2})\) data set for the remaining \((\frac{m_N}{2})\) measurement units and discard the remaining observations. If \(m_{N} \in [m_{L}, m_{U} ]\) is odd, we select \((\frac{m_N+1}{2}){th}\) smallest rank unit from all \(m_{N} \in [m_{L}, m_{U} ]\) sets of size \(m_{N} \in [m_{L}, m_{U} ]\) . This process counts the total \(m_{N} \in [m_{L}, m_{U} ]\) neutrosophic bivariate units. After r cycles of these steps, the total \(n_{N}=m_{N}r \in [n_{L}, n_{U} ]\) bivariate NMRSS units are obtained.
Here, we have set sample NMRSSo for an odd set size and NMRSSe for an even set size. For j th cycle MRSSo is denoted by \((X_{1(\frac{m_N+1}{2})j},Y_{1[\frac{m_N+1}{2}]j}), (X_{2(\frac{m_N+1}{2})j},Y_{2[\frac{m_N+1}{2}]j}),..., (X_{m_N(\frac{m_N+1}{2})j},Y_{m_N[\frac{m_N+1}{2}]j}); (j=1,2,..., r).\) Let the sample means of \(X_N\) and \(Y_N\) are, respectively, as
and their corresponding variances are given as
Similarly, for j th cycle NMRSSe is denoted by \((X_{1(\frac{m}{2})j},Y_{1[\frac{m}{2}]j}), (X_{2(\frac{m}{2})j},Y_{2[\frac{m}{2}]j}),..., (X_{\frac{m}{2}(\frac{m}{2})j},Y_{\frac{m}{2}[\frac{m}{2}]j}),(X_{\frac{m}{2}+1(\frac{m+2}{2})j},Y_{\frac{m}{2}+1[\frac{m+2}{2}]j}),\) \((X_{\frac{m}{2}+2(\frac{m+2}{2})j},Y_{\frac{m}{2}+2[\frac{m+2}{2}]j}),..., (X_{m(\frac{m+2}{2})j},Y_{m[\frac{m+2}{2}]j})\) . Again, let the sample means of \(X_N\) and \(Y_N\) are, respectively, as
Consider a neutrosophic random sample of size \(n_{N} \in [n_{L}, n_{U} ]\) using NMRSS, which is acquired from a finite population of N units \((U_{1}, U_{2},..., U_{N} )\) . The neutrosophic study and auxiliary variable are \(Y_{N} \in [Y_{L}, Y_{U}]\) and \(X_{N} \in [X_{L}, X_{U}]\) . Let \({\overline{y}}_{[n]N} \in [{\overline{y}}_{[n]L}, {\overline{y}}_{[n]U}] \) and \({\overline{x}}_{(n)N} \in [{\overline{x}}_{(n)L}, {\overline{x}}_{(n)U}] \) be the sample means of the neutrosophic study and auxiliary variables respectively, and also, let \({\overline{Y}}_{N} \in [{\overline{Y}}_{L}, {\overline{Y}}_{U}] \) and \({\overline{X}}_{N} \in [{\overline{X}}_{L}, {\overline{X}}_{U}] \) be the population means of the neutrosophic study and auxiliary variables, respectively. The correlation coefficient between both neutrosophic study and auxiliary variables is \( \rho _{yxN} \in [\rho _{yxL}, \rho _{yxU}] \) .
Let the neutrosophic mean error terms are \(\epsilon _{0N} \in [\epsilon _{0\,L}, \epsilon _{0U} ] \) and \(\epsilon _{1N} \in [\epsilon _{1\,L}, \epsilon _{1U} ] \) . To obtain the bias and MSE of the estimators, we write
\({\overline{y}}_{[n]N}={\overline{Y}}_{N} (1+ \epsilon _{0N} )\) , \({\overline{x}}_{(n)N}={\overline{X}}_{N} (1+ \epsilon _{1N} )\)
\(E(\epsilon _{0N}^2)=\frac{\mathrm{{Var}}({\overline{y}}_{[n]N})}{{\overline{Y}}_{N}^2}=V_{yrN}\)
\(E(\epsilon _{1N}^2)=\frac{\mathrm{{Var}}({\overline{x}}_{(n)N})}{{\overline{X}}_{N}^2}=V_{xrN}\)
\(E(\epsilon _{0N},\epsilon _{0N})=\frac{\mathrm{{Cov}}({\overline{y}}_{[n]N},{\overline{x}}_{(n)N})}{{\overline{Y}}_{N}{\overline{X}}_{N}}=V_{yxrN}\)
\(e_{0N}^2 \in [e_{0L}^2, e_{0U}^2]\) ; \(e_{1N}^2 \in [e_{1\,L}^2, e_{1U}^2]\) ; \(e_{0N}e_{1N} \in [e_{0\,L}e_{1\,L}, e_{0U}e_{0U}]\) ;
\(V_{yrN} \in [V_{yrL}, V_{yrU}]\) ; \(V_{xrN} \in [V_{xrL}, V_{xrU}]\) ; \(V_{yxrN} \in [V_{yxrL}, V_{yxrU}]\) .
4 Existing Estimators
The usual unbiased estimator for the population mean \({\overline{Y}}\) using NMRSS technique is given by
The variance of the estimator \({\overline{y}}_{[n]N}\) is given by
The ratio estimator under NMRSS for the population mean \({\overline{Y}}\)
The MSE of the estimator \({\overline{y}}_{rN}\) is given by
The product estimator under NMRSS for the population mean \({\overline{Y}}\)
The MSE of the estimator \({\overline{y}}_{pN}\) is given by
The regression estimator under NMRSS for the population mean \({\overline{Y}}\)
The MSE of the estimator \({\overline{y}}_{\mathrm{{reg}}N}\) is given by
The exponential ratio-type estimator under NMRSS for the population mean \({\overline{Y}}\) is represented as
The MSE of the estimator \({\overline{y}}_{\mathrm{{exp}}rN}\) is given by
The exponential product type estimator under NMRSS for the population mean \({\overline{Y}}\) is represented as
The MSE of the estimator \({\overline{y}}_{\mathrm{{exp}}pN}\) is given by
5 Proposed Estimators
No single estimator is universally effective in all situations. Hence, it is preferable to employ estimators that yield minimal MSE and high precision. The objective of this section is to formulate estimators that demonstrate effective performance across a broader range of circumstances. In pursuit of this goal, we have embraced the [ 14 ] estimator within the framework of NMRSS and introduced two novel estimators for the mean of a finite population under NMRSS, utilizing auxiliary variables.
where the constants \(g_{1N}\) and \(g_{2N}\) ensure that the MSE of the estimators is minimized.
When we express the estimator \(P_{1N}\) from Eq. ( 21 ) into terms of \(\epsilon \) ’s, we obtain
Taking expectations by focusing on first-order approximation, we obtain MSE,
To find out the minimum MSE for \(P_{1N}\) , we partially differentiate equation ( 23 ) w.r.t. \(g_{1N}\) & \(g_{2N} \) and equating to zero, we obtain
By putting the optimum value of \(g_{1N}\) & \(g_{2N}\) in the Eq. ( 23 ), we can determine the minimum MSE of \(P_{1N}\) as
When we express the estimator \(P_{2N}\) from Eq. ( 27 ) into terms of \(\epsilon \) ’s, we obtain
Case 1: If sum of weights is fixed ( \(g_{3N}+g_{4N}=1\) )
The MSE of the estimator \(P_{2N}\) is shown as
To find out the minimum value of MSE for \(P_{2N}\) , we partially differentiate equation ( 31 ) w.r.t. \(g_{4N}\) , and equating to zero we get
By putting the optimum value of \(g_{4N}\) in Eq. ( 31 ), we can determine the minimum MSE of \(P_{2N}\) as
Case 2: If the sum of weights is adjustable ( \(g_{3N}\) + \(g_{4N}\) \(\ne \) 1)
Squaring on both sides we get
To find out the minimum MSE for \(P_{2N}\) , we partially differentiate equation ( 36 ) w.r.t. \( g_{3N}\) & \(g_{4N}\) and equating to zero we obtain
By putting the optimum value of \(g_{3N}\) & \(g_{4N}\) in the Eq. ( 36 ), we can determine the minimum MSE of \(P_{2N}\) as
When we express the estimator \(P_{3N}\) from Eq. ( 40 ) into terms of \(\epsilon 's\) , we obtain
Case 1: If sum of weights is fixed ( \(g_{5N} + g_{6N} = 1\) )
The MSE of the estimator \(P_{3N}\) is shown as
To find out the minimum value of MSE for \({\ P}_{3N}\) , we partially differentiate equation ( 44 ) w.r.t. \(g_{6N}\) and equating to zero, we get
By putting the optimum value of \(g_{6N}\) in the Eq. ( 44 ), we can determine the minimum MSE of \(P_{3N}\) as
Case 2: If the sum of weights is adjustable ( \(g_{5N}\) + \(g_{6N}\) \(\ne \) 1)
To find out the minimum MSE for \(P_{3N}\) , we partially differentiate equation ( 49 ) w.r.t. \(g_{5N}\) & \(g_{6N} \) and equating to zero, we get
By putting the optimum value of \(g_{5N}\) & \(g_{6N}\) in the Eq. ( 49 ), we can determine the minimum MSE of \(P_{3N}\) as
where \(P_{iN} \in [P_{iL},P_{iU}]; i=1,2,3 \) , \(A_{iN} \in [A_{iL},A_{iU}]; i=1,2,3 \) , \(B_{iN} \in [B_{iL},B_{iU}]; i=1,2,3 \)
\(C_{iN} \in [C_{iL},C_{iU}]; i=1,2,3 \) , \(D_{iN} \in [D_{iL},D_{iU}]; i=1,2,3 \) , \(E_{iN} \in [E_{iL},E_{iU}]; i=1,2,3 \) .
6 Numerical Illustrations
In this section, we compare the performance of the suggested estimators to the other existing estimators considered in this paper. We have taken real-life natural growth rate data from the sample registration system (2020). The data mentioned in the sample registration system (2020) have four neutrosophic variables for every state, but in our research, we use birth rate vs natural growth rate. Here, birth rate is the neutrosophic auxiliary variable \(X_{N} \in [X_{L}, X_{U} ]\) and natural growth rate is a neutrosophic study variable \(Y_{N} \in [Y_{L}, Y_{U} ]\) .
To obtain the relative performance of proposed estimators under NMRSS, we have taken two cases of odd and even set sizes. Further, in odd case we have drawn total \(n_{N}= m_{N}r= 12\) samples with set size \(m_{N} = [3, 3]\) and replication \(r=4\) and for even case we have drawn total \(n_{N}= m_{N}r= 16\) samples with set size \(m_{N} = [4, 4]\) with replication \(r=4\) from the given population of size 36 by utilizing the method of NMRSS. The NMRSS sample for the study and auxiliary variables are drawn simultaneously using the NMRSS technique outlined in Sect. 3 . The following is a definition of the percent relative efficiency (PRE) formula:
7 Simulation Studies
We verify the effectiveness of the suggested estimator through simulation studies with other existing estimators like the conventional, ratio, regression estimator, etc. This is done via the following steps
1. It is well known that a neutrosophic normal distribution (NND) will be followed by neutrosophic random variables (NRV), i.e. \((X_{N}, Y_{N}) \sim NN[(\mu _{xN}, \sigma ^2_{xN}),(\mu _{yN}, \sigma ^2_{yN})]\) , \(X_{N} \in [X_{L}, X_{U}]\) , \(Y_{N} \in [Y_{L}, Y_{U}]\) , \(\mu _{xN} \in [(\mu _{xL}, \mu _{xU})]\) , \(\mu _{yN} \in [(\mu _{yL}, \mu _{yU})]\) , \(\sigma ^2_{xN} \in [\sigma ^2_{xL}, \sigma ^2_{xU}]\) , \(\sigma ^2_{yN} \in [\sigma ^2_{yL}, \sigma ^2_{yU}]\) .
4-variate random observations with a size of N = 1000 have been produced from a 4-variate normal distribution with mean ( \({\mu }_{xL}{,\mu }_{yL}, \mu _{xU}, \mu _{yU})=\left( 50,50,60,60\right) \) and covariance matrix \(\left[ \begin{array}{cccc} {\sigma }^2_{xL} &{} {\rho }_{xyL}{\sigma }_{xL}{\sigma }_{yL} &{} 0 &{} 0 \\ {\rho }_{xyL}{\sigma }_{xL}{\sigma }_{yL} &{} {\sigma }^2_{yL} &{} 0 &{} 0 \\ 0 &{} 0 &{} {\sigma }^2_{xU} &{} {\rho }_{xyU}{\sigma }_{xU}{\sigma }_{yU} \\ 0 &{} 0 &{} {\rho }_{xyU}{\sigma }_{xU}{\sigma }_{yU} &{} {\sigma }^2_{yU} \end{array} \right] \) , where we have \({\sigma }^2_{xL}=100\,{\sigma }^2_{yL}=100\) , \({\sigma }^2_{xU}=121\,{\sigma }^2_{yU}=121\) .
2. For this N = 1000 simulated population, the parameters were computed.
3. A sample of size n with \(m_{N}=3\) for odd case and \(m_{N}=4\) for even case with \(r=4\) has been selected from this simulated population.
4. To find the MSE of each estimator under study, use the sample data.
5. To get MSEs, the entire step 3–4 process was repeated 10,000 times. The MSE of each estimator of the population mean is the average of the 10,000 values that were obtained.
6. The formula has been used to find each estimator’s PRE with regard to \({\overline{y}}_{[n]N}.\)
8 Discussion
The study established mathematical expressions for the very first NMRSS estimators, approximating up to the first order. Subsequently, to examine the properties of the proposed NMRSS estimators, numerical illustrations and simulation studies were conducted. The former used real-world natural growth rate data, while the latter involved an artificial neutrosophic dataset with varying correlation coefficients and sample sizes. The results were encapsulated in Tables 2 , 3 , 4 , and 5 , showcasing MSEs and PREs for both existing and proposed NMRSS estimators for odd and even cases. The PREs of the NMRSS estimators were calculated over estimators under NSRS and NRSS, and these outcomes are shown in Tables 6 and 7 .
In Tables 2 and 3 , the MSEs of the existing and suggested estimators are given along with PRE for odd and even cases respectively. The superiority of the suggested NMRSS estimators over the existing ones is displayed in Tables 2 and 3 in the bolded text. We also see that the MSE and PRE of the recommended estimator are lesser and higher than those of the other existing estimators. The tables make this clear that recommended estimators outperformed existing ones, offering lower MSEs and higher PREs, and \(t_{p2}\) being the best estimator.
Similarly, in Tables 4 and 5 , the MSEs of the recommended and existing estimators are given along with PRE through a simulation study based on artificial neutrosophic data for different values of the correlation coefficient and different sample sizes. Like Tables 2 and 3 , the superiority of the suggested NMRSS estimators over the existing ones is displayed in Tables 4 and 5 . We also see that the MSEs and PREs of the recommended estimators are lesser and higher, respectively than those of other existing estimators. Hence, Tables 4 and 5 mirrored these findings, with the proposed estimators continuing to outshine existing ones, demonstrating lower MSEs and higher PREs in the simulation study too.
Tables 4 and 5 show that with the increase in sample sizes and correlation coefficients, the MSE decreases, and the PRE increases for the recommended estimator. Therefore, under NMRSS, the suggested estimators exhibit sensitivity similar to that of classical ranked set sampling.
Table 6 features PRE values of the proposed NMRSS estimators compared to NSRS counterparts. We observe from Table 6 that all PRE values exceeded 100 indicating that all the NMRSS estimators are superior to corresponding estimators under NSRS, as RSS is the best replacement for SRS.
Table 7 features PRE values of the proposed NMRSS estimators over NRSS counterparts. We see from Table 7 that all PRE values exceeded 100, indicating that all the NMRSS estimators are superior to corresponding estimators under NRSS, as MRSS is the best replacement for RSS when data do not follow the symmetric distribution.
The comparison between classical MRSS and NMRSS using MSEs and PREs is provided in Tables 8 and 9 . Tables 8 and 9 demonstrate that the MSEs of the suggested estimators obtained through classical MRSS are between the lower and higher value of MSE obtained using NMRSS, and the same goes for the PREs, indicating that the latter method is more effective than the former.
The study highlights that classical ranked set sampling is ill-suited for dealing with vague or indeterminate data. NMRSS proves superior for estimating uncertain or interval data. The tables present dependable results for neutrosophic data compared to classical results.
9 Conclusion
This study aims to tackle the challenges posed by ambiguous or indeterminate data within the realm of classical statistics. Through the utilization of MRSS, an enhancement over SRS and RSS, the study addresses the complexities associated with expensive and asymmetric data. Given the absence of prior research addressing this specific issue, our work represents a novel and significant advancement in survey sampling methodologies, particularly in dealing with imprecise data featuring asymmetric distributions.
In this research paper, we have proposed a new modification of RSS: the very first neutrosophic median ranked set method in sampling theory. We’ve put forth some enhanced NMRSS estimators designed for estimating population means while making use of auxiliary information. To assess their accuracy, we calculated both bias and MSE for these suggested estimators, focusing on first-order approximations. We compared our recommended estimators against existing ones using a natural population data on natural growth rates and a simulated population.
Through a combination of numerical illustrations and simulation studies, we’ve found compelling evidence that our proposed estimators outperform existing ones within the framework of NMRSS. Among these estimators, \(t_{p2} \) emerged as the top performer. It’s important to note that the sensitivity analysis of our recommended estimators under NMRSS mirrors that of classical MRSS. The MSE and PRE of the recommended estimator decrease and increase, respectively, with the increase in values of sample sizes and correlation coefficients.
Moreover, a comparison between the recommended estimators under NMRSS and the estimators under NSRS and NRSS revealed that NMRSS is a more effective alternative to NSRS and NRSS, much like classical MRSS to classical SRS and classical RSS. The MSEs of the proposed estimators derived from classical MRSS fall within the range between the lower and higher MSE values obtained through NMRSS. Similarly, the PREs exhibit a similar pattern. These findings suggest that the suggested method surpasses the effectiveness of classical approaches. Our study underscores the efficiency and reliability of NMRSS for handling neutrosophic data, with our proposed NMRSS delivering superior mean estimations compared to existing methods.
Based on the numerical illustrations and simulation studies we’ve conducted, it’s reasonable to recommend the use of our proposed estimators over the alternatives presented in this paper in various real-world scenarios, spanning fields like agriculture, mathematics, biology, poultry farming, economics, commerce, and the social sciences.s
Furthermore, given the limited availability of neutrosophic MRSS estimators, there’s ample room for further exploration. Building upon this study, we can consider defining variations of NRSS, such as unbalanced NRSS, quantile NRSS, extreme NRSS, double NRSS, and percentile NRSS, akin to what exists in classical RSS. Additionally, we can explore the replacement of our proposed estimators with alternative methods or estimators. Expanding beyond sampling theory, further research avenues in statistics, encompassing fields like control charts, inference, reliability analysis, non-parametric estimation, hypothesis testing, and some other fields of science, present promising opportunities for exploration.
Data Availability
All data are provided in this study.
Al-Omari, A.I.: Ratio estimation of the population mean using auxiliary information in simple random sampling and median ranked set sampling. Stat. Probab. Lett. 82 , 1883–1890 (2012)
Article MathSciNet Google Scholar
Al-Omari, A.I., Bouza, C.N.: Ratio estimators of the population mean with missing values using ranked set sampling. Environmetrics 26 (2), 67–76 (2015)
Bahl, S., Tuteja, R.K.: Ratio and product type exponential estimators. J. Inf. Optim. Sci. 12 (1), 159–164 (1991)
MathSciNet Google Scholar
Bhushan, S., Kumar, A., Lone, S.A.: On some novel classes of estimators using RSS. Alex. Eng. J. 61 , 5465–5474 (2022)
Article Google Scholar
Bouza, C.N.: Ranked set sub-sampling of the non-response strata for estimating the difference of means. Biom. J. J. Math. Methods Biosci. 44 (7), 903–915 (2002)
Bouza, C.N.: Ranked set sampling for the product estimator. Rev. Investig. Oper. 29 (3), 201–206 (2008)
Dell, T., Clutter, J.: Ranked set sampling theory with order statistics background. Biometrics 28 , 545–555 (1972)
Ganesh, S., Ganeslingam, S.: Ranked set sampling vs simple random sampling in the estimation of the mean and the ratio. J. Stat. Manag. Syst. 9 (2), 459–472 (2006)
Kadilar, C., Cingi, H.: Ratio estimators in simple random sampling. Appl. Math. Comput. 151 , 893–902 (2004)
Kadilar, C., Unyazici, Y., Cingi, H.: Ratio estimator for the population mean using ranked set sampling. Stat. Pap. 50 , 301–309 (2009)
Koyuncu, N.: New difference-cum-ratio and exponential type estimators in median ranked set sampling. Hacet. J. Math. Stat. 45 (1), 207–225 (2016)
Mandowara, V.L., Mehta, N.: Efficient generalized ratio-product type estimators for finite population mean with ranked set sampling. Aust. J. Stat. 42 (3), 137–148 (2013)
McIntyre, G.A.: A method for unbiased selective sampling using ranked sets. Crop. Pasture Sci. 3 , 385–390 (1952)
Mishra, P., Adichwal, N.K., Singh, R.: A new log-product-type estimator using auxiliary information. J. Sci. Res. 61 , 179–183 (2017)
Google Scholar
Muttlak, H.A.: Median ranked set sampling. J. Appl. Stat. Sci. 6 , 245–255 (1997)
Pandey, B.N., Dubey, V.: Modified product estimator using coefficient of variation of the auxiliary variate. Assam Stat. Rev. 2 (2), 64–66 (1988)
Samawi, H.M., Muttlak, H.A.: Estimation of ratio using rank set sampling. Biom. J. 38 (6), 753–764 (1996)
Samawi, H.M., Muttlak, H.A.: On ratio estimation using median ranked set sampling. J. Appl. Stat. Sci. 10 (2), 89–98 (2002)
Singh, H.P., Tailor, R., Tailor, R., Kakran, M.S.: An improved estimator of population mean using power transformation. J. Indian Soc. Agric. Stat. 58 (2), 223–230 (2004)
Singh, H.P., Tailor, R., Singh, S.: General procedure for estimating the population means using ranked set sampling. J. Stat. Comput. Simul. 84 (5), 931–945 (2014)
Singh, P., Bouza, C., Singh, R.: Generalized exponential estimator for estimating the population mean using an auxiliary variable. J. Sci. Res. 63 , 273–280 (2019)
Singh, R., Mishra, R.: Neutrosophic transformed ratio estimators for estimating finite population mean in sample surveys. Adv. Sampl. Theory Appl. 1 , 39–47 (2021)
Singh, R., Kumari, A.: Improved estimators of population mean using auxiliary variables in ranked set sampling. Rev. Investig. Oper. 44 (2), 271–280 (2023)
Singh, R., Smarandache, F., Mishra, R.: Generalized robust-type neutrosophic ratio estimators of pharmaceutical daily stock prices. Cognitive intelligence with neutrosophic statistics in bioinformatics 417–429 (2023)
Sisodia, B., Dwivedi, V.K.: A modified ratio estimator using co-efficient of variation of auxiliary variable. J. Indian Soc. Agric. Stat. 33 (1), 13–18 (1981)
Smarandache, F.: Neutrosophy: neutrosophic probability, set, and logic: analytic synthesis & synthetic analysis. ProQuest Inf. Learn. 105 , 118–123 (1998)
Smarandache, F.: A unifying field in logics: neutrosophic logic. Philosophy. American Research Press, 1–141 (1999)
Smarandache, F.: A unifying field in logics: neutrosophic logic, neutrosophic set, neutrosophic probability, and statistics (2001) arXiv:math/0101228
Smarandache, F.: Neutrosophic set a generalization of the intuitionistic fuzzy set. Int. J. Pure Appl. Math. 24 (3), 287 (2005)
Smarandache, F.: Neutrosophic logic—a generalization of the intuitionistic fuzzy logic. Multispace Multi Struct. Neutrosophic Transdiscipl. 4 , 396 (2010)
Smarandache, F.: Introduction to neutrosophic measure, neutrosophic integral, and neutrosophic probability. In: Infinite study (2013)
Smarandache, F.: Introduction to neutrosophic statistics. In: Infinite study (2014)
Stokes, L.: Ranked set sampling with concomitant variables. Commun. Stat. Theory Methods 6 , 1207–1211 (1977)
Tahir, Z., Khan, H., Aslam, M., Shabbir, J., Mahmood, Y., Smarandache, F.: Neutrosophic ratio-type estimators for estimating the population mean. Complex Intell. Syst. 7 , 2991–3001 (2021)
Takahasi, K., Wakimoto, K.: On unbiased estimates of the population mean based on the sample stratified by means of ordering. Ann. Inst. Stat. Math. 20 , 1–31 (1968)
Vishwakarma, G., Singh, A.: Computing the effect of measurement errors on ranked set sampling estimators of the population mean. Concurr. Comput. Pract. Exp. 34 (27), e7333 (2022)
Vishwakarma, G.K., Singh, A.: Generalized estimator for computation of population mean under neutrosophic ranked set technique: an application to solar energy data. Comput. Appl. Math. 41 (4), 144 (2022)
Download references
No funding support.
Author information
Rajesh Singh and Florentin Smarandache contributed equally to this work.
Authors and Affiliations
Department of Statistics, Banaras Hindu University, Varanasi, Uttar Pradesh, 221005, India
Anamika Kumari & Rajesh Singh
Department of Mathematics, University of New Mexico, Gallup, USA
Florentin Smarandache
You can also search for this author in PubMed Google Scholar
Contributions
All authors contributed equally to this work.
Corresponding author
Correspondence to Anamika Kumari .
Ethics declarations
Conflict of interest.
The authors declare no conflict of interest.
Additional information
Publisher's note.
Springer Nature remains neutral with regard to jurisdictional claims in published maps and institutional affiliations.
Supplementary Information
Below is the link to the electronic supplementary material.
Supplementary file 1 (doc 42 KB)
Rights and permissions.
Open Access This article is licensed under a Creative Commons Attribution 4.0 International License, which permits use, sharing, adaptation, distribution and reproduction in any medium or format, as long as you give appropriate credit to the original author(s) and the source, provide a link to the Creative Commons licence, and indicate if changes were made. The images or other third party material in this article are included in the article’s Creative Commons licence, unless indicated otherwise in a credit line to the material. If material is not included in the article’s Creative Commons licence and your intended use is not permitted by statutory regulation or exceeds the permitted use, you will need to obtain permission directly from the copyright holder. To view a copy of this licence, visit http://creativecommons.org/licenses/by/4.0/ .
Reprints and permissions
About this article
Kumari, A., Singh, R. & Smarandache, F. New Modification of Ranked Set Sampling for Estimating Population Mean: Neutrosophic Median Ranked Set Sampling with an Application to Demographic Data. Int J Comput Intell Syst 17 , 210 (2024). https://doi.org/10.1007/s44196-024-00548-y
Download citation
Received : 28 December 2023
Accepted : 31 May 2024
Published : 08 August 2024
DOI : https://doi.org/10.1007/s44196-024-00548-y
Share this article
Anyone you share the following link with will be able to read this content:
Sorry, a shareable link is not currently available for this article.
Provided by the Springer Nature SharedIt content-sharing initiative
- Neutrosophic statistics
- Study variable
- Auxiliary variable
- Mean squared error
- Percentage relative efficiency
- Find a journal
- Publish with us
- Track your research
Click through the PLOS taxonomy to find articles in your field.
For more information about PLOS Subject Areas, click here .
Loading metrics
Open Access
Peer-reviewed
Research Article
Archaeology in space: The Sampling Quadrangle Assemblages Research Experiment (SQuARE) on the International Space Station. Report 1: Squares 03 and 05
Roles Conceptualization, Data curation, Formal analysis, Funding acquisition, Investigation, Methodology, Project administration, Resources, Supervision, Visualization, Writing – original draft, Writing – review & editing
* E-mail: [email protected]
Affiliations Department of Art, Chapman University, Orange, CA, United States of America, Space Engineering Research Center, University of Southern California, Marina del Rey, CA, United States of America

Roles Data curation, Formal analysis, Investigation, Methodology, Resources, Software, Supervision, Validation, Visualization, Writing – original draft, Writing – review & editing
Affiliation Department of History, Carleton University, Ottawa, ON, United States of America
Roles Conceptualization, Data curation, Methodology, Project administration, Supervision, Writing – review & editing
Affiliation College of Humanities, Arts and Social Sciences, Flinders University, Adelaide, Australia
Roles Software, Writing – original draft
Roles Investigation, Writing – original draft
Affiliation Archaeology Research Center, University of Southern California, Los Angeles, CA, United States of America
- Justin St. P. Walsh,
- Shawn Graham,
- Alice C. Gorman,
- Chantal Brousseau,
- Salma Abdullah
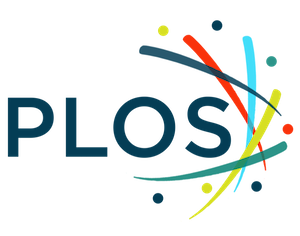
- Published: August 7, 2024
- https://doi.org/10.1371/journal.pone.0304229
- Reader Comments
Between January and March 2022, crew aboard the International Space Station (ISS) performed the first archaeological fieldwork in space, the Sampling Quadrangle Assemblages Research Experiment (SQuARE). The experiment aimed to: (1) develop a new understanding of how humans adapt to life in an environmental context for which we are not evolutionarily adapted, using evidence from the observation of material culture; (2) identify disjunctions between planned and actual usage of facilities on a space station; (3) develop and test techniques that enable archaeological research at a distance; and (4) demonstrate the relevance of social science methods and perspectives for improving life in space. In this article, we describe our methodology, which involves a creative re-imagining of a long-standing sampling practice for the characterization of a site, the shovel test pit. The ISS crew marked out six sample locations (“squares”) around the ISS and documented them through daily photography over a 60-day period. Here we present the results from two of the six squares: an equipment maintenance area, and an area near exercise equipment and the latrine. Using the photographs and an innovative webtool, we identified 5,438 instances of items, labeling them by type and function. We then performed chronological analyses to determine how the documented areas were actually used. Our results show differences between intended and actual use, with storage the most common function of the maintenance area, and personal hygiene activities most common in an undesignated area near locations for exercise and waste.
Citation: Walsh JSP, Graham S, Gorman AC, Brousseau C, Abdullah S (2024) Archaeology in space: The Sampling Quadrangle Assemblages Research Experiment (SQuARE) on the International Space Station. Report 1: Squares 03 and 05. PLoS ONE 19(8): e0304229. https://doi.org/10.1371/journal.pone.0304229
Editor: Peter F. Biehl, University of California Santa Cruz, UNITED STATES OF AMERICA
Received: March 9, 2024; Accepted: May 7, 2024; Published: August 7, 2024
Copyright: © 2024 Walsh et al. This is an open access article distributed under the terms of the Creative Commons Attribution License , which permits unrestricted use, distribution, and reproduction in any medium, provided the original author and source are credited.
Data Availability: All relevant data are within the paper and its Supporting Information files.
Funding: JW was the recipient of funding from Chapman University’s Office of Research and Sponsored Programs to support the activities of Axiom Space as implementation partner for the research presented in this article. There are no associated grant numbers for this financial support. Axiom Space served in the role of a contractor hired by Chapman University for the purpose of overseeing logistics relating to our research. In-kind support in the form of ISS crew time and access to the space station’s facilities, also awarded to JW from the ISS National Laboratory, resulted from an unsolicited proposal, and therefore there is no opportunity title or number associated with our work. No salary was received by any of the investigators as a result of the grant support. No additional external funding was received for this study.
Competing interests: The authors have declared that no competing interests exist.
Introduction
The International Space Station Archaeological Project (ISSAP) aims to fill a gap in social science investigation into the human experience of long-duration spaceflight [ 1 – 3 ]. As the largest, most intensively inhabited space station to date, with over 270 visitors from 23 countries during more than 23 years of continuous habitation, the International Space Station (ISS) is the ideal example of a new kind of spacefaring community—“a microsociety in a miniworld” [ 4 ]. While it is possible to interview crew members about their experiences, the value of an approach focused on material culture is that it allows identification of longer-term patterns of behaviors and associations that interlocutors are unable or even unwilling to articulate. In this respect, we are inspired by previous examples of contemporary archaeology such as the Tucson Garbage Project and the Undocumented Migration Project [ 5 – 7 ]. We also follow previous discussions of material culture in space contexts that highlight the social and cultural features of space technology [ 8 , 9 ].
Our primary goal is to identify how humans adapt to life in a new environment for which our species has not evolved, one characterized by isolation, confinement, and especially microgravity. Microgravity introduces opportunities, such as the ability to move and work in 360 degrees, and to carry out experiments impossible in full Earth gravity, but also limitations, as unrestrained objects float away. The most routine activities carried out on Earth become the focus of intense planning and technological intervention in microgravity. By extension, our project also seeks to develop archaeological techniques that permit the study of other habitats in remote, extreme, or dangerous environments [ 10 , 11 ]. Since it is too costly and difficult to visit our archaeological site in person, we have to creatively re-imagine traditional archaeological methods to answer key questions. To date, our team has studied crew-created visual displays [ 12 , 13 ], meanings and processes associated with items returned to Earth [ 14 ], distribution of different population groups around the various modules [ 15 ], and the development of machine learning (ML) computational techniques to extract data about people and places, all from historic photographs of life on the ISS [ 16 ].
From January to March 2022, we developed a new dataset through the first archaeological work conducted off-Earth. We documented material culture in six locations around the ISS habitat, using daily photography taken by the crew which we then annotated and studied as evidence for changes in archaeological assemblages of material culture over time. This was the first time such data had been captured in a way that allowed statistical analysis. Here, we present the data and results from Squares 03 and 05, the first two sample locations to be completed.
Materials and methods
Square concept and planning.
Gorman proposed the concept behind the investigation, deriving it from one of the most traditional terrestrial archaeological techniques, the shovel test pit. This method is used to understand the overall characteristics of a site quickly through sampling. A site is mapped with a grid of one-meter squares. Some of the squares are selected for initial excavation to understand the likely spatial and chronological distribution of features across the entire site. In effect, the technique is a way to sample a known percentage of the entire site systematically. In the ISS application of this method, we documented a notional stratigraphy through daily photography, rather than excavation.
Historic photography is a key dataset for the International Space Station Archaeological Project. Tens of thousands of images have been made available to us, either through publication [ 17 ], or through an arrangement with the ISS Research Integration Office, which supplied previously unpublished images from the first eight years of the station’s habitation. These photographs are informative about the relationships between people, places, and objects over time in the ISS. However, they were taken randomly (from an archaeological perspective) and released only according to NASA’s priorities and rules. Most significantly, they were not made with the purpose of answering archaeological questions. By contrast, the photographs taken during the present investigation were systematic, representative of a defined proportion of the habitat’s area, and targeted towards capturing archaeology’s primary evidence: material culture. We were interested in how objects move around individual spaces and the station, what these movements revealed about crew adherence to terrestrial planning, and the creative use of material culture to make the laboratory-like interior of the ISS more habitable.
Access to the field site was gained through approval of a proposal submitted to the Center for the Advancement of Science in Space (also known as the ISS National Laboratory [ISS NL]). Upon acceptance, Axiom Space was assigned as the Implementation Partner for carriage of the experiment according to standard procedure. No other permits were required for this work.
Experiment design
Since our work envisioned one-meter sample squares, and recognizing the use of acronyms as a persistent element of spacefaring culture, we named our payload the Sampling Quadrangle Assemblages Research Experiment (SQuARE). Permission from the ISS NL to conduct SQuARE was contingent on using equipment that was already on board the space station. SQuARE required only five items: a camera, a wide-angle lens, adhesive tape (for marking the boundaries of the sample locations), a ruler (for scale), and a color calibration card (for post-processing of the images). All of these were already present on the ISS.
Walsh performed tests on the walls of a terrestrial art gallery to assess the feasibility of creating perfect one-meter squares in microgravity. He worked on a vertical surface, using the Pythagorean theorem to determine where the corners should be located. The only additional items used for these tests were two metric measuring tapes and a pencil for marking the wall (these were also already on the ISS). While it was possible to make a square this way, it also became clear that at least two people were needed to manage holding the tape measures in position while marking the points for the corners. This was not possible in the ISS context.
Walsh and Gorman identified seven locations for the placement of squares. Five of these were in the US Orbital Segment (USOS, consisting of American, European, and Japanese modules) and two in the Russian Orbital Segment. Unfortunately, tense relations between the US and Russian governments meant we could only document areas in the USOS. The five locations were (with their SQuARE designations):
- 01—an experimental rack on the forward wall, starboard end, of the Japanese Experiment Module
- 02—an experimental rack on the forward wall, port end, of the European laboratory module Columbus
- 03—the starboard Maintenance Work Area (workstation) in the US Node 2 module
- 04—the wall area “above” (according to typical crew body orientation) the galley table in the US Node 1 module
- 05—the aft wall, center location, of the US Node 3 module
Our square selection encompassed different modules and activities, including work and leisure. We also asked the crew to select a sixth sample location based on their understanding of the experiment and what they thought would be interesting to document. They chose a workstation on the port wall of the US laboratory module, at the aft end, which they described in a debriefing following their return to Earth in June 2022 as “our central command post, like our shared office situation in the lab.” Results from the four squares not included here will appear in future publications.
Walsh worked with NASA staff to determine payload procedures, including precise locations for the placement of the tape that would mark the square boundaries. The squares could not obstruct other facilities or experiments, so (unlike in terrestrial excavations, where string is typically used to demarcate trench boundaries) only the corners of each square were marked, not the entire perimeter. We used Kapton tape due to its bright yellow-orange color, which aided visibility for the crew taking photographs and for us when cropping the images. In practice, due to space constraints, the procedures that could actually be performed by crew in the ISS context, and the need to avoid interfering with other ongoing experiments, none of the locations actually measured one square meter or had precise 90° corners like a trench on Earth.
On January 14, 2022, NASA astronaut Kayla Barron set up the sample locations, marking the beginning of archaeological work in space ( S1 Movie ). For 30 days, starting on January 21, a crew member took photos of the sample locations at approximately the same time each day; the process was repeated at a random time each day for a second 30-day period to eliminate biases. Photography ended on March 21, 2022. The crew were instructed not to move any items prior to taking the photographs. Walsh led image management, including color and barrel distortion correction, fixing the alignment of each image, and cropping them to the boundaries of the taped corners.
Data processing—Item tagging, statistics, visualizations
We refer to each day’s photo as a “context” by analogy with chronologically-linked assemblages of artifacts and installations at terrestrial archaeological sites ( S1 and S2 Datasets). As previously noted, each context represented a moment roughly 24 hours distant from the previous one, showing evidence of changes in that time. ISS mission planners attempted to schedule the activity at the same time in the first month, but there were inevitable changes due to contingencies. Remarkably, the average time between contexts in Phase 1 was an almost-perfect 24h 0m 13s. Most of the Phase 1 photos were taken between 1200 and 1300 GMT (the time zone in which life on the ISS is organized). In Phase 2, the times were much more variable, but the average time between contexts during this period was still 23h 31m 45s. The earliest Phase 2 photo was taken at 0815 GMT, and the latest at 2101. We did not identify any meaningful differences between results from the two phases.
Since the “test pits” were formed of images rather than soil matrices, we needed a tool to capture information about the identity, nature, and location of every object. An open-source image annotator platform [ 18 ] mostly suited our needs. Brousseau rebuilt the platform to work within the constraints of our access to the imagery (turning it into a desktop tool with secure access to our private server), to permit a greater range of metadata to be added to each item or be imported, to autosave, and to export the resulting annotations. The tool also had to respect privacy and security limitations required by NASA.
The platform Brousseau developed and iterated was rechristened “Rocket-Anno” ( S1 File ). For each context photograph, the user draws an outline around every object, creating a polygon; each polygon is assigned a unique ID and the user provides the relevant descriptive information, using a controlled vocabulary developed for ISS material culture by Walsh and Gorman. Walsh and Abdullah used Rocket-Anno to tag the items in each context for Squares 03 and 05. Once all the objects were outlined for every context’s photograph, the tool exported a JSON file with all of the metadata for both the images themselves and all of the annotations, including the coordinate points for every polygon ( S3 Dataset ). We then developed Python code using Jupyter “notebooks” (an interactive development environment) that ingests the JSON file and generates dataframes for various facets of the data. Graham created a “core” notebook that exports summary statistics, calculates Brainerd-Robinson coefficients of similarity, and visualizes the changing use of the square over time by indicating use-areas based on artifact types and subtypes ( S2 File ). Walsh and Abdullah also wrote detailed square notes with context-by-context discussions and interpretations of features and patterns.
We asked NASA for access to the ISS Crew Planner, a computer system that shows each astronaut’s tasks in five-minute increments, to aid with our interpretation of contexts, but were denied. As a proxy, we use another, less detailed source: the ISS Daily Summary Reports (DSRs), published on a semi-regular basis by NASA on its website [ 19 ]. Any activities mentioned in the DSRs often must be connected with a context by inference. Therefore, our conclusions are likely less precise than if we had seen the Crew Planner, but they also more clearly represent the result of simply observing and interpreting the material culture record.
The crew during our sample period formed ISS Expedition 66 (October 2021-March 2022). They were responsible for the movement of objects in the sample squares as they carried out their daily tasks. The group consisted of two Russians affiliated with Roscosmos (the Russian space agency, 26%), one German belonging to the European Space Agency (ESA, 14%), and four Americans employed by NASA (57%). There were six men (86%) and one woman (14%), approximately equivalent to the historic proportions in the ISS population (84% and 16%, respectively). The Russian crew had their sleeping quarters at the aft end of the station, in the Zvezda module. The ESA astronaut slept in the European Columbus laboratory module. The four NASA crew slept in the US Node 2 module (see below). These arrangements emphasize the national character of discrete spaces around the ISS, also evident in our previous study of population distributions [ 15 ]. Both of the sample areas in this study were located in US modules.
Square 03 was placed in the starboard Maintenance Work Area (MWA, Fig 1 ), one of a pair of workstations located opposite one another in the center of the Node 2 module, with four crew berths towards the aft and a series of five ports for the docking of visiting crew/cargo vehicles and two modules on the forward end ( Fig 2 ). Node 2 (sometimes called “Harmony”) is a connector that links the US, Japanese, and European lab modules. According to prevailing design standards when the workstation was developed, an MWA “shall serve as the primary location for servicing and repair of maximum sized replacement unit/system components” [ 20 ]. Historic images published by NASA showing its use suggested that its primary function was maintenance of equipment and also scientific work that did not require a specific facility such as a centrifuge or furnace.
- PPT PowerPoint slide
- PNG larger image
- TIFF original image
An open crew berth is visible at right. The yellow dotted line indicates the boundaries of the sample area. Credit: NASA/ISSAP.
https://doi.org/10.1371/journal.pone.0304229.g001
Credit: Tor Finseth, by permission, modified by Justin Walsh.
https://doi.org/10.1371/journal.pone.0304229.g002
Square 03 measured 90.3 cm (top) x 87.8 (left) x 89.4 (bottom) x 87.6 (right), for an area of approximately 0.79 m 2 . Its primary feature was a blue metal panel with 40 square loop-type Velcro patches arranged in four rows of ten. During daily photography, many items were attached to the Velcro patches (or held by a clip or in a resealable bag which had its own hook-type Velcro). Above and below the blue panel were additional Velcro patches placed directly on the white plastic wall surface. These patches were white, in different sizes and shapes and irregularly arranged, indicating that they had been placed on the wall in response to different needs. Some were dirty, indicating long use. The patches below the blue panel were rarely used during the sample period, but the patches above were used frequently to hold packages of wet wipes, as well as resealable bags with electrostatic dispersion kits and other items. Outside the sample area, the primary features were a crew berth to the right, and a blue metal table attached to the wall below. This table, the primary component of the MWA, “provides a rigid surface on which to perform maintenance tasks,” according to NASA [ 21 ]. It is modular and can be oriented in several configurations, from flat against the wall to horizontal ( i . e ., perpendicular to the wall). A laptop to the left of the square occasionally showed information about work happening in the area.
In the 60 context photos of Square 03, we recorded 3,608 instances of items, an average of 60.1 (median = 60.5) per context. The lowest count was 24 in context 2 (where most of the wall was hidden from view behind an opaque storage bag), and the highest was 75 in both contexts 20 and 21. For comparison between squares, we can also calculate the item densities per m 2 . The average count was 76.1/m 2 (minimum = 30, maximum = 95). The count per context ( Fig 3(A)) began much lower than average in the first three contexts because of a portable glovebag and a stowage bag that obscured much of the sample square. It rose to an above-average level which was sustained (with the exception of contexts 11 and 12, which involved the appearance of another portable glovebag) until about context 43, when the count dipped again and the area seemed to show less use. Contexts 42–59 showed below-average numbers, as much as 20% lower than previously.
(a) Count of artifacts in Square 03 over time. (b) Proportions of artifacts by function in Square 03. Credit: Rao Hamza Ali.
https://doi.org/10.1371/journal.pone.0304229.g003
74 types of items appeared at least once here, belonging to six categories: equipment (41%), office supplies (31%), electronic (17%), stowage (9%), media (1%), and food (<1%). To better understand the significance of various items in the archaeological record, we assigned them to functional categories ( Table 1 , Fig 3(B)) . 35% of artifacts were restraints, or items used for holding other things in place; 12% for tools; 9% for containers; 9% for writing items; 6% for audiovisual items; 6% for experimental items; 4% for lights; 4% for safety items; 4% for body maintenance; 4% for power items; 3% for computing items; 1% for labels; and less than 1% drinks. We could not identify a function for two percent of the items.
https://doi.org/10.1371/journal.pone.0304229.t001
One of the project goals is understanding cultural adaptations to the microgravity environment. We placed special attention on “gravity surrogates,” pieces of (often simple) technology that are used in space to replicate the terrestrial experience of things staying where they are placed. Gravity surrogates include restraints and containers. It is quite noticeable that gravity surrogates comprise close to half of all items (44%) in Square 03, while the tools category, which might have been expected to be most prominent in an area designated for maintenance, is less than one-third as large (12%). Adding other groups associated with work, such as “experiment” and “light,” only brings the total to 22%.
Square 05 (Figs 2 and 4 ) was placed in a central location on the aft wall of the multipurpose Node 3 (“Tranquility”) module. This module does not include any specific science facilities. Instead, there are two large pieces of exercise equipment, the TVIS (Treadmill with Vibration Isolation Stabilization System, on the forward wall at the starboard end), and the ARED (Advanced Resistive Exercise Device, on the overhead wall at the port end). Use of the machines forms a significant part of crew activities, as they are required to exercise for two hours each day to counteract loss of muscle mass and bone density, and enable readjustment to terrestrial gravity on their return. The Waste and Hygiene Compartment (WHC), which includes the USOS latrine, is also here, on the forward wall in the center of the module, opposite Square 05. Finally, three modules are docked at Node 3’s port end. Most notable is the Cupola, a kind of miniature module on the nadir side with a panoramic window looking at Earth. This is the most popular leisure space for the crew, who often describe the hours they spend there. The Permanent Multipurpose Module (PMM) is docked on the forward side, storing equipment, food, and trash. In previous expeditions, some crew described installing a curtain in the PMM to create a private space for changing clothes and performing body maintenance activities such as cleaning oneself [ 22 , 23 ], but it was unclear whether that continued to be its function during the expedition we observed. One crew member during our sample period posted a video on Instagram showing the PMM interior and their efforts to re-stow equipment in a bag [ 24 ]. The last space attached to Node 3 is an experimental inflatable module docked on the aft side, called the Bigelow Expandable Activity Module (BEAM), which is used for storage of equipment.
The yellow dotted line indicates the boundaries of the sample area. The ARED machine is at the far upper right, on the overhead wall. The TVIS treadmill is outside this image to the left, on the forward wall. The WHC is directly behind the photographer. Credit: NASA/ISSAP.
https://doi.org/10.1371/journal.pone.0304229.g004
Square 05 was on a mostly featureless wall, with a vertical handrail in the middle. Handrails are metal bars located throughout the ISS that are used by the crew to hold themselves in place or provide a point from which to propel oneself to another location. NASA’s most recent design standards acknowledge that “[t]hey also serve as convenient locations for temporary mounting, affixing, or restraint of loose equipment and as attachment points for equipment” [ 25 ]. The handrail in Square 05 was used as an impromptu object restraint when a resealable bag filled with other bags was squeezed between the handrail and the wall.
The Brine Processing Assembly (BPA), a white plastic box which separates water from other components of urine for treatment and re-introduction to the station’s drinkable water supply [ 26 ], was fixed to the wall outside the square boundaries at lower left. A bungee cord was attached to both sides of the box; the one on the right was connected at its other end to the handrail attachment bracket. Numerous items were attached to or wedged into this bungee cord during the survey, bringing “gravity” into being. A red plastic duct ran through the square from top center into the BPA. This duct led from the latrine via the overhead wall. About halfway through the survey period, in context 32, the duct was wrapped in Kapton tape. According to the DSR for that day, “the crew used duct tape [ sic ] to make a seal around the BPA exhaust to prevent odor permeation in the cabin” [ 27 ], revealing an aspect of the crew’s experience of this area that is captured only indirectly in the context photograph. Permanently attached to the wall were approximately 20 loop-type Velcro patches in many shapes and sizes, placed in a seemingly random pattern that likely indicates that they were put there at different times and for different reasons.
Other common items in Square 05 were a mirror, a laptop computer, and an experimental item belonging to the German space agency DLR called the Touch Array Assembly [ 28 ]. The laptop moved just three times, and only by a few centimeters each time, during the sample period. The Touch Array was a black frame enclosing three metal surfaces which were being tested for their bacterial resistance; members of the crew touched the surfaces at various moments during the sample period. Finally, and most prominent due to its size, frequency of appearance, and use (judged by its movement between context photos) was an unidentified crew member’s toiletry kit.
By contrast with Square 03, 05 was the most irregular sample location, roughly twice as wide as it was tall. Its dimensions were 111 cm (top) x 61.9 (left) x 111.4 (bottom) x 64.6 (right), for an area of approximately 0.7 m 2 , about 89% of Square 03. We identified 1,830 instances of items in the 60 contexts, an average of 30.5 (median = 32) per context. The minimum was 18 items in context 5, and the maximum was 39 in contexts 24, 51, and 52. The average item density was 43.6/m 2 (minimum = 26, maximum = 56), 57% of Square 03.
The number of items trended upward throughout the sample period ( Fig 5(A)) . The largest spike occurred in context 6 with the appearance of the toiletry kit, which stored (and revealed) a number of related items. The kit can also be linked to one of the largest dips in item count, seen from contexts 52 to 53, when it was closed (but remained in the square). Other major changes can often be attributed to the addition and removal of bungee cords, which had other items such as carabiners and brackets attached. For example, the dip seen in context 25 correlates with the removal of a bungee cord with four carabiners.
(a) Count of artifacts and average count in Square 05 over time. (b) Proportions of artifacts by function in Square 05. Credit: Rao Hamza Ali.
https://doi.org/10.1371/journal.pone.0304229.g005
41 different item types were found in Square 05, about 55% as many as in Square 03. These belonged to five different categories: equipment (63%), electronic (17%), stowage (10%), office supplies (5%), and food (2%). The distribution of function proportions was quite different in this sample location ( Table 2 and Fig 5(B)) . Even though restraints were still most prominent, making up 32% of all items, body maintenance was almost as high (30%), indicating how strongly this area was associated with the activity of cleaning and caring for oneself. Computing (8%, represented by the laptop, which seems not to have been used), power (8%, from various cables), container (7%, resealable bags and Cargo Transfer Bags), and hygiene (6%, primarily the BPA duct) were the next most common items. Experiment was the function of 4% of the items, mostly the Touch Array, which appeared in every context, followed by drink (2%) and life support (1%). Safety, audiovisual, food, and light each made up less than 1% of the functional categories.
https://doi.org/10.1371/journal.pone.0304229.t002
Tracking changes over time is critical to understanding the activity happening in each area. We now explore how the assemblages change by calculating the Brainerd-Robinson Coefficient of Similarity [ 29 , 30 ] as operationalized by Peeples [ 31 , 32 ]. This metric is used in archaeology for comparing all pairs of the contexts by the proportions of categorical artifact data, here functional type. Applying the coefficient to the SQuARE contexts enables identification of time periods for distinct activities using artifact function and frequency alone, independent of documentary or oral evidence.
Multiple phases of activities took place in the square. Moments of connected activity are visible as red clusters in contexts 0–2, 11–12, 28–32, and 41 ( Fig 6(A)) . Combining this visualization with close observation of the photos themselves, we argue that there are actually eight distinct chronological periods.
- Contexts 0–2: Period 1 (S1 Fig in S3 File ) is a three-day period of work involving a portable glovebag (contexts 0–1) and a large blue stowage bag (context 2). It is difficult to describe trends in functional types because the glovebag and stowage bag obstruct the view of many objects. Items which appear at the top of the sample area, such as audiovisual and body maintenance items, are overemphasized in the data as a result. It appears that some kind of science is happening here, perhaps medical sample collection due to the presence of several small resealable bags visible in the glovebag. The work appears particularly intense in context 1, with the positioning of the video camera and light to point into the glovebag. These items indicate observation and oversight of crew activities by ground control. A white cargo transfer bag for storage and the stowage bag for holding packing materials in the context 2 photo likely relate to the packing of a Cargo Dragon vehicle that was docked to Node 2. The Dragon departed from the ISS for Earth, full of scientific samples, equipment, and crew personal items, a little more than three hours after the context 2 photo was taken [ 33 ].
- Contexts 3–10: Period 2 (S2 Fig in S3 File ) was a “stable” eight-day period in the sample, when little activity is apparent, few objects were moved or transferred in or out the square, and the primary function of the area seems to be storage rather than work. In context 6, a large Post-It notepad appeared in the center of the metal panel with a phone number written on it. This number belonged to another astronaut, presumably indicating that someone on the ISS had been told to call that colleague on the ground (for reasons of privacy, and in accordance with NASA rules for disseminating imagery, we have blurred the number in the relevant images). In context 8, the same notepad sheet had new writing appear on it, this time reading “COL A1 L1,” the location of an experimental rack in the European lab module.
- Contexts 11–12: Period 3 (S3 Fig in S3 File ) involves a second appearance of a portable glovebag (a different one from that used in contexts 0–1, according to its serial number), this time for a known activity, a concrete hardening experiment belonging to the European Space Agency [ 34 , 35 ]. This two-day phase indicates how the MWA space can be shared with non-US agencies when required. It also demonstrates the utility of this flexible area for work beyond biology/medicine, such as material science. Oversight of the crew’s activities by ground staff is evident from the positioning of the video camera and LED light pointing into the glovebag.
- Contexts 13–27: Period 4 (S4 Fig in S3 File ) is another stable fifteen-day period, similar to Period 2. Many items continued to be stored on the aluminum panel. The LED light’s presence is a trace of the activity in Period 3 that persists throughout this phase. Only in context 25 can a movement of the lamp potentially be connected to an activity relating to one of the stored items on the wall: at least one nitrile glove was removed from a resealable bag behind the lamp. In general, the primary identifiable activity during Period 4 is storage.
- Contexts 28–32: Period 5 (S5 Fig in S3 File ), by contrast, represents a short period of five days of relatively high and diverse activity. In context 28, a Microsoft Hololens augmented reality headset appeared. According to the DSR for the previous day, a training activity called Sidekick was carried out using the headset [ 36 ]. The following day, a Saturday, showed no change in the quantity or type of objects, but many were moved around and grouped by function—adhesive tape rolls were placed together, tools were moved from Velcro patches into pouches or straightened, and writing implements were placed in a vertical orientation when previously they were tilted. Context 29 represents a cleaning and re-organization of the sample area, which is a common activity for the crew on Saturdays [ 37 ]. Finally, in context 32, an optical coherence tomography scanner—a large piece of equipment for medical research involving crew members’ eyes—appeared [ 38 ]. This device was used previously during the sample period, but on the same day as the ESA concrete experiment, so that earlier work seems to have happened elsewhere [ 39 ].
- Contexts 33–40: Period 6 (S6 Fig in S3 File ) is the third stable period, in which almost no changes are visible over eight days. The only sign of activity is a digital timer which was started six hours before the context 39 image was made and continued to run at least through context 42.
- Context 41: Period 7 (S7 Fig in S3 File ) is a single context in which medical sample collection may have occurred. Resealable bags (some holding others) appeared in the center of the image and at lower right. One of the bags at lower right had a printed label reading “Reservoir Containers.” We were not able to discern which type of reservoir containers the label refers to, although the DSR for the day mentions “[Human Research Facility] Generic Saliva Collection,” without stating the location for this work [ 40 ]. Evidence from photos of other squares shows that labeled bags could be re-used for other purposes, so our interpretation of medical activity for this context is not conclusive.
- Contexts 42–60: Period 8 (S8 Fig in S3 File ) is the last and longest period of stability and low activity—eighteen days in which no specific activity other than the storage of items can be detected. The most notable change is the appearance for the first time of a foil water pouch in the central part of the blue panel.
Visualization of Brainerd-Robinson similarity, compared context-by-context by item function, for (a) Square 03 and (b) Square 05. The more alike a pair of contexts is, the higher the coefficient value, with a context compared against itself where a value of 200 equals perfect similarity. The resulting matrix of coefficients is visualized on a scale from blue to red where blue is lowest and red is highest similarity. The dark red diagonal line indicates complete similarity, where each context is compared to itself. Dark blue represents a complete difference. Credit: Shawn Graham.
https://doi.org/10.1371/journal.pone.0304229.g006
In the standards used at the time of installation, “stowage space” was the sixth design requirement listed for the MWA after accessibility; equipment size capability; scratch-resistant surfaces; capabilities for electrical, mechanical, vacuum, and fluid support during maintenance; and the accommodation of diagnostic equipment [ 20 ]. Only capabilities for fabrication were listed lower than stowage. Yet 50 of the 60 contexts (83%) fell within stable periods where little or no activity is identifiable in Square 03. According to the sample results, therefore, this area seems to exist not for “maintenance,” but primarily for the storage and arrangement of items. The most recent update of the design standards does not mention the MWA, but states, “Stowage location of tool kits should be optimized for accessibility to workstations and/or maintenance workbenches” [ 25 ]. Our observation confirms the importance of this suggestion.
The MWA was also a flexible location for certain science work, like the concrete study or crew health monitoring. Actual maintenance of equipment was hardly in evidence in the sample (possibly contexts 25, 39, and 44), and may not even have happened at all in this location. Some training did happen here, such as review of procedures for the Electromagnetic Levitator camera (instructions for changing settings on a high-speed camera appeared on the laptop screen; the day’s DSR shows that this camera is part of the Electromagnetic Levitator facility, located in the Columbus module [ 41 ]. The training required the use of the Hololens system (context 28 DSR, cited above).
Although many item types were represented in Square 03, it became clear during data capture how many things were basically static, unmoving and therefore unused, especially certain tools, writing implements, and body maintenance items. The MWA was seen as an appropriate place to store these items. It may be the case that their presence here also indicates that their function was seen as an appropriate one for this space, but the function(s) may not be carried out—or perhaps not in this location. Actualization of object function was only visible to us when the state of the item changed—it appeared, it moved, it changed orientation, it disappeared, or, in the case of artifacts that were grouped in collections rather than found as singletons, its shape changed or it became visibly smaller/lesser. We therefore have the opportunity to explore not only actuality of object use, but also potentiality of use or function, and the meaning of that quality for archaeological interpretation [ 42 , 43 ]. This possibility is particularly intriguing in light of the archaeological turn towards recognizing the agency of objects to impact human activity [ 44 , 45 ]. We will explore these implications in a future publication.
We performed the same chronological analysis for Square 05. Fig 6(B) represents the analysis for both item types and for item functions. We identified three major phases of activity, corresponding to contexts 0–5, 6–52, and 53–59 (S9-S11 Figs in S3 File ). The primary characteristics of these phases relate to an early period of unclear associations (0–5) marked by the presence of rolls of adhesive tape and a few body maintenance items (toothpaste and toothbrush, wet wipes); the appearance of a toiletry kit on the right side of the sample area, fully open with clear views of many of the items contained within (6–52); and finally, the closure of the toiletry kit so that its contents can no longer be seen (53–59). We interpret the phases as follows:
- Contexts 0–5: In Period 1 (six days, S9 Fig in S3 File ), while items such as a mirror, dental floss picks, wet wipes, and a toothbrush held in the end of a toothpaste tube were visible, the presence of various other kinds of items confounds easy interpretation. Two rolls of duct tape were stored on the handrail in the center of the sample area, and the Touch Array and laptop appeared in the center. Little movement can be identified, apart from a blue nitrile glove that appeared in context 1 and moved left across the area until it was wedged into the bungee cord for contexts 3 and 4. The tape rolls were removed prior to context 5. A collection of resealable bags was wedged behind the handrail in context 3, remaining there until context 9. Overall, this appears to be a period characterized by eclectic associations, showing an area without a clear designated function.
- Contexts 6–52: Period 2 (S10 Fig in S3 File ) is clearly the most significant one for this location due to its duration (47 days). It was dominated by the number of body maintenance items located in and around the toiletry kit, especially a white hand towel (on which a brown stain was visible from context 11, allowing us to confirm that the same towel was present until context 46). A second towel appeared alongside the toiletry kit in context 47, and the first one was fixed at the same time to the handrail, where it remained through the end of the sample period. A third towel appeared in context 52, attached to the handrail together with the first one by a bungee cord, continuing to the end of the sample period. Individual body maintenance items moved frequently from one context to the next, showing the importance of this type of activity for this part of Node 3. For reasons that are unclear, the mirror shifted orientation from vertical to diagonal in context 22, and then was put back in a vertical orientation in context 31 (a Monday, a day which is not traditionally associated with cleaning and organization). Collections of resealable bags appeared at various times, including a large one labeled “KYNAR BAG OF ZIPLOCKS” in green marker at the upper left part of the sample area beginning of context 12 (Kynar is a non-flammable plastic material that NASA prefers for resealable bags to the generic commercial off-the-shelf variety because it is non-flammable; however, its resistance to heat makes it less desirable for creating custom sizes, so bags made from traditional but flammable low-density polyethylene still dominate on the ISS [ 14 ]). The Kynar bag contained varying numbers of bags within it over time; occasionally, it appeared to be empty. The Touch Array changed orientation on seven of 47 days in period 2, or 15% of the time (12% of all days in the survey), showing activity associated with scientific research in this area. In context 49, a life-support item, the Airborne Particulate Monitor (APM) was installed [ 46 ]. This device, which measures “real-time particulate data” to assess hazards to crew health [ 47 ], persisted through the end of the sample period.
- Contexts 53–59: Period 3 (S11 Fig in S3 File ) appears as a seven-day phase marked by low activity. Visually, the most notable feature is the closure of the toiletry kit, which led to much lower counts of body maintenance items. Hardly any of the items on the wall moved at all during this period.
While body maintenance in the form of cleaning and caring for oneself could be an expected function for an area with exercise and excretion facilities, it is worth noting that the ISS provides, at most, minimal accommodation for this activity. A description of the WHC stated, “To provide privacy…an enclosure was added to the front of the rack. This enclosure, referred to as the Cabin, is approximately the size of a typical bathroom stall and provides room for system consumables and hygiene item stowage. Space is available to also support limited hygiene functions such as hand and body washing” [ 48 ]. A diagram of the WHC in the same publication shows the Cabin without a scale but suggests that it measures roughly 2 m (h) x .75 (w) x .75 (d), a volume of approximately 1.125 m 3 . NASA’s current design standards state that the body volume of a 95th percentile male astronaut is 0.99 m 3 [ 20 ], meaning that a person of that size would take up 88% of the space of the Cabin, leaving little room for performing cleaning functions—especially if the Cabin is used as apparently intended, to also hold “system consumables and hygiene item[s]” that would further diminish the usable volume. This situation explains why crews try to adapt other spaces, such as storage areas like the PMM, for these activities instead. According to the crew debriefing statement, only one of them used the WHC for body maintenance purposes; it is not clear whether the toiletry kit belonged to that individual. But the appearance of the toiletry kit in Square 05—outside of the WHC, in a public space where others frequently pass by—may have been a response to the limitations of the WHC Cabin. It suggests a need for designers to re-evaluate affordances for body maintenance practices and storage for related items.
Although Square 03 and 05 were different sizes and shapes, comparing the density of items by function shows evidence of their usage ( Table 3 ). The typical context in Square 03 had twice as many restraints and containers, but less than one-quarter as many body maintenance items as Square 05. 03 also had many tools, lights, audiovisual equipment, and writing implements, while there were none of any of these types in 05. 05 had life support and hygiene items which were missing from 03. It appears that flexibility and multifunctionality were key elements for 03, while in 05 there was emphasis on one primary function (albeit an improvised one, designated by the crew rather than architects or ground control), cleaning and caring for one’s body, with a secondary function of housing static equipment for crew hygiene and life support.
https://doi.org/10.1371/journal.pone.0304229.t003
As this is the first time such an analysis has been performed, it is not yet possible to say how typical or unusual these squares are regarding the types of activities taking place; but they provide a baseline for eventual comparison with the other four squares and future work on ISS or other space habitats.
Some general characteristics are revealed by archaeological analysis of a space station’s material culture. First, even in a small, enclosed site, occupied by only a few people over a relatively short sample period, we can observe divergent patterns for different locations and activity phases. Second, while distinct functions are apparent for these two squares, they are not the functions that we expected prior to this research. As a result, our work fulfills the promise of the archaeological approach to understanding life in a space station by revealing new, previously unrecognized phenomena relating to life and work on the ISS. There is now systematically recorded archaeological data for a space habitat.
Squares 03 and 05 served quite different purposes. The reasons for this fact are their respective affordances and their locations relative to activity areas designated for science and exercise. Their national associations, especially the manifestation of the control wielded by NASA over its modules, also played a role in the use of certain materials, the placement of facilities, and the organization of work. How each area was used was also the result of an interplay between the original plans developed by mission planners and habitat designers (or the lack of such plans), the utility of the equipment and architecture in each location, and the contingent needs of the crew as they lived in the station. This interplay became visible in the station’s material culture, as certain areas were associated with particular behaviors, over time and through tradition—over the long duration across many crews (Node 2, location of Square 03, docked with the ISS in 2007, and Node 3, location of Square 05, docked in 2010), and during the specific period of this survey, from January to March 2022. During the crew debriefing, one astronaut said, “We were a pretty organized crew who was also pretty much on the same page about how to do things…. As time went on…we organized the lab and kind of got on the same page about where we put things and how we’re going to do things.” This statement shows how functional associations can become linked to different areas of the ISS through usage and mutual agreement. At the same time, the station is not frozen in time. Different people have divergent ideas about how and where to do things. It seems from the appearance of just one Russian item—a packet of generic wipes ( salfetky sukhiye ) stored in the toiletry kit throughout the sample period—that the people who used these spaces and carried out their functions did not typically include the ISS’s Russian crew. Enabling greater flexibility to define how spaces can be used could have a significant impact on improving crew autonomy over their lives, such as how and where to work. It could also lead to opening of all spaces within a habitat to the entire crew, which seems likely to improve general well-being.
An apparent disjunction between planned and actual usage appeared in Square 03. It is intended for maintenance as well as other kinds of work. But much of the time, there was nobody working here—a fact that is not captured by historic photos of the area, precisely because nothing is happening. The space has instead become the equivalent of a pegboard mounted on a wall in a home garage or shed, convenient for storage for all kinds of items—not necessarily items being used there—because it has an enormous number of attachment points. Storage has become its primary function. Designers of future workstations in space should consider that they might need to optimize for functions other than work, because most of the time, there might not be any work happening there. They could optimize for quick storage, considering whether to impose a system of organization, or allow users to organize as they want.
We expected from previous (though unsystematic) observation of historic photos and other research, that resealable plastic bags (combined with Velcro patches on the bags and walls) would be the primary means for creating gravity surrogates to control items in microgravity. They only comprise 7% of all items in Square 03 (256 instances). There are more than twice as many clips (572—more than 9 per context) in the sample. There were 193 instances of adhesive tape rolls, and more than 100 cable ties, but these were latent (not holding anything), representing potentiality of restraint rather than actualization. The squares showed different approaches to managing “gravity.” While Square 03 had a pre-existing structured array of Velcro patches, Square 05 showed a more expedient strategy with Velcro added in response to particular activities. Different needs require different affordances; creating “gravity” is a more nuanced endeavor than it initially appears. More work remains to be done to optimize gravity surrogates for future space habitats, because this is evidently one of the most critical adaptations that crews have to make in microgravity (44% of all items in Square 03, 39% in 05).
Square 05 is an empty space, seemingly just one side of a passageway for people going to use the lifting machine or the latrine, to look out of the Cupola, or get something out of deep storage in one of the ISS’s closets. In our survey, this square was a storage place for toiletries, resealable bags, and a computer that never (or almost never) gets used. It was associated with computing and hygiene simply by virtue of its location, rather than due to any particular facilities it possessed. It has no affordances for storage. There are no cabinets or drawers, as would be appropriate for organizing and holding crew personal items. A crew member decided that this was an appropriate place to leave their toiletry kit for almost two months. Whether this choice was appreciated or resented by fellow crew members cannot be discerned based on our evidence, but it seems to have been tolerated, given its long duration. The location of the other four USOS crew members’ toiletry kits during the sample period is unknown. A question raised by our observations is: how might a function be more clearly defined by designers for this area, perhaps by providing lockers for individual crew members to store their toiletries and towels? This would have a benefit not only for reducing clutter, but also for reducing exposure of toiletry kits and the items stored in them to flying sweat from the exercise equipment or other waste particles from the latrine. A larger compartment providing privacy for body maintenance and a greater range of motion would also be desirable.
As the first systematic collection of archaeological data from a space site outside Earth, this analysis of two areas on the ISS as part of the SQuARE payload has shown that novel insights into material culture use can be obtained, such as the use of wall areas as storage or staging posts between activities, the accretion of objects associated with different functions, and the complexity of using material replacements for gravity. These results enable better space station design and raise new questions that will be addressed through analysis of the remaining four squares.
Supporting information
S1 movie. nasa astronaut kayla barron installs the first square for the sampling quadrangle assemblages research experiment in the japanese experiment module (also known as kibo) on the international space station, january 14, 2022..
She places Kapton tape to mark the square’s upper right corner. Credit: NASA.
https://doi.org/10.1371/journal.pone.0304229.s001
S1 Dataset.
https://doi.org/10.1371/journal.pone.0304229.s002
S2 Dataset.
https://doi.org/10.1371/journal.pone.0304229.s003
S3 Dataset. The image annotations are represented according to sample square in json formatted text files.
The data is available in the ‘SQuARE-notebooks’ repository on Github.com in the ‘data’ subfolder at https://github.com/issarchaeologicalproject/SQuARE-notebooks/tree/main ; archived version of the repository is at Zenodo, DOI: 10.5281/zenodo.10654812 .
https://doi.org/10.1371/journal.pone.0304229.s004
S1 File. The ‘Rocket-Anno’ image annotation software is available on Github at https://github.com/issarchaeologicalproject/MRE-RocketAnno .
The archived version of the repository is at Zenodo, DOI: 10.5281/zenodo.10648399 .
https://doi.org/10.1371/journal.pone.0304229.s005
S2 File. The computational notebooks that process the data json files to reshape the data suitable for basic statistics as well as the computation of the Brainerd-Robinson coefficients of similarity are in the.ipynb notebook format.
The code is available in the ‘SQuARE-notebooks’ repository on Github.com in the ‘notebooks’ subfolder at https://github.com/issarchaeologicalproject/SQuARE-notebooks/tree/main ; archived version of the repository is at Zenodo, DOI: 10.5281/zenodo.10654812 . The software can be run online in the Google Colab environment ( https://colab.research.google.com ) or any system running Jupyter Notebooks ( https://jupyter.org/ ).
https://doi.org/10.1371/journal.pone.0304229.s006
https://doi.org/10.1371/journal.pone.0304229.s007
Acknowledgments
We thank Chapman University’s Office of Research and Sponsored Programs, and especially Dr. Thomas Piechota and Dr. Janeen Hill, for funding the Implementation Partner costs associated with the SQuARE payload. Chapman’s Leatherby Libraries’ Supporting Open Access Research and Scholarship (SOARS) program funded the article processing fee for this publication. Ken Savin and Ken Shields at the ISS National Laboratory gave major support by agreeing to sponsor SQuARE and providing access to ISS NL’s allocation of crew time. David Zuniga and Kryn Ambs at Axiom Space were key collaborators in managing payload logistics. NASA staff and contractors were critical to the experiment’s success, especially Kristen Fortson, Jay Weber, Crissy Canerday, Sierra Wolbert, and Jade Conway. We also gratefully acknowledge the help and resources provided by Dr. Erik Linstead, director of the Machine Learning and Affiliated Technology Lab at Chapman University. Aidan St. P. Walsh corrected the color and lens barrel distortion in all of the SQuARE imagery. Rao Hamza Ali produced charts using accessible color combinations for Figs 3 and 5 . And finally, of course, we are extremely appreciative of the efforts of the five USOS members of the Expedition 66 crew on the ISS—Kayla Barron, Raja Chari, Thomas Marshburn, Matthias Maurer, and Mark Vande Hei—who were the first archaeologists in space.
- 1. Buchli V. Extraterrestrial methods: Towards an ethnography of the ISS. In: Carroll T, Walford A, Walton S, editors. Lineages and advancements in material culture studies: Perspectives from UCL anthropology. London: Routledge; 2021, pp. 17–32.
- 2. Gorman A, Walsh J. Archaeology in a vacuum: obstacles to and solutions for developing a real space archaeology. In: Barnard H, editor. Archaeology outside the box: investigations at the edge of the discipline. Los Angeles, Cotsen Institute of Archaeology Press; 2023. pp. 131–123.
- 3. Walsh J. Adapting to space: The International Space Station Archaeological Project. In: Salazar Sutil JF, Gorman A, editors. Routledge handbook of social studies of outer space. London, Routledge; 2023. pp. 400–412. https://doi.org/10.4324/9781003280507-37
- View Article
- Google Scholar
- 6. Rathje W, Murphy C. Rubbish! The archaeology of garbage Tucson: University of Arizona Press; 2001.
- 7. De León J. The land of open graves: living and dying on the migrant trail. Berkeley, University of California Press; 2015.
- 8. Garrison Darrin A, O’Leary B, editors. Handbook of space engineering, archaeology, and heritage. Boca Raton, CRC Press; 2009.
- 9. Capelotti PJ. The human archaeology of space: Lunar, planetary, and interstellar relics of exploration. Jefferson, NC, McFarland Press; 2010.
- 11. Gorman A. Space and time through material culture: An account of space archaeology. In: Salazar Sutil JF, Gorman A, editors. Routledge handbook of social studies of outer space. London, Routledge; 2023. pp. 44–56. https://doi.org/10.4324/9781003280507-5
- 17. NASA. NASA Johnson. 2008 Aug [cited May 12 2024]. In: Flickr [Internet]. San Francisco. Available from https://www.flickr.com/photos/nasa2explore/
- 19. NASA. ISS Daily Status Reports. 2012 Mar 1 [Cited May 12 2024]. Available from: https://blogs.nasa.gov/stationreport/
- 20. NASA. Man-systems integration. STD-3000 Vol. 1. Houston, NASA Johnson; 1995, pp. 9–15, 78
- 21. NASA. Maintenance Work Area | Glenn Research Center. 2020 Mar 6 [cited May 12 2024]. Available from: https://www1.grc.nasa.gov/space/iss-research/mwa/
- 22. Cristoforetti S. Diario di un’apprendista astronauta. Milan, Le Polene; 2018. pp. 379.
- 23. Kelly S. Endurance: A year in space, a lifetime of discovery. New York, Knopf; 2017. pp. 175, 285–86.
- 24. Barron K. Instagram post, 2022 Feb 12 [cited 2024 May 12]. Available from: https://www.instagram.com/tv/CZ4pW9HJ2Wg/?igsh=ZDE1MWVjZGVmZQ==
- 25. NASA. NASA space flight human-system standard. STD-3001 Volume 1: Human integration design handbook. Rev. 1 Houston, NASA Johnson; 2014. pp. 814, 829–833.
- 27. Keeter B. ISS daily summary report– 2/21/2022. 2022 Feb 21 [cited May 12 2024]. In: NASA ISS On-Orbit Status Report blog [Internet]. Houston. Available from: https://blogs.nasa.gov/stationreport/2022/02/page/6/
- 28. DLR. Fingerprint research to combat harmful bacteria. 2022 Jan 18 [cited May 12 2024]. Available from: https://www.dlr.de/en/latest/news/2022/01/20220118_fingerprint-research-to-combat-harmful-bacteria
- 31. Peeples MA. R script for calculating the Brainerd-Robinson coefficient of similarity and assessing sampling error. 2011 [cited May 12 2024]. Available from: http://www.mattpeeples.net/br.html .
- 33. Garcia M. Cargo Dragon Splashes Down Ending SpaceX CRS-24 Mission. 2022 Jan 24 [cited May 12 2024]. NASA Space Station blog [Internet]. Available from: https://blogs.nasa.gov/spacestation/2022/01/24/cargo-dragon-splashes-down-ending-spacex-crs-24-mission/
- 34. ESA. Concrete Hardening | Cosmic Kiss 360°. 2022 Mar 5 [cited May 12 2024]. Available from: https://www.esa.int/ESA_Multimedia/Videos/2022/05/Concrete_Hardening_Cosmic_Kiss_360
- 35. Keeter B. ISS daily summary report– 2/01/2022. 2022 Feb 1 [cited May 12 2024]. In: NASA ISS On-Orbit Status Report blog [Internet]. Houston. Available from: https://blogs.nasa.gov/stationreport/2022/02/page/19/
- 36. Keeter B. ISS daily summary report– 2/17/2022. 2022 Feb 17 [cited May 12 2024]. In: NASA ISS On-Orbit Status Report blog [Internet]. Houston. Available from: https://blogs.nasa.gov/stationreport/2022/02/page/8/
- 37. T. Pultarova, How Do You Clean a Space Station? Astronaut Thomas Pesquet Shares Orbital Spring Cleaning Tips, Space.com, May 6, 2021. Online at https://www.space.com/space-station-cleaning-tips-astronaut-thomas-pesquet
- 38. Keeter B. ISS daily summary report– 2/22/2022. 2022 Feb 22 [cited May 12 2024]. In: NASA ISS On-Orbit Status Report blog [Internet]. Houston. Available from: https://blogs.nasa.gov/stationreport/2022/02/page/5/
- 39. Keeter B. ISS daily summary report– 2/02/2022. 2022 Feb 2 [cited May 12 2024]. NASA ISS On-Orbit Status Report blog [Internet]. Houston. Online at https://blogs.nasa.gov/stationreport/2022/02/page/18/
- 40. Keeter B. ISS daily summary report– 3/03/2022. 2022 Mar 3 [cited May 12 2024]. In: NASA ISS On-Orbit Status Report blog [Internet]. Houston. Available from: https://blogs.nasa.gov/stationreport/2022/03/page/21/
- 41. Keeter B. ISS daily summary report– 2/08/2022. 2022 Feb 8 [cited May 12 2024]. NASA ISS On-Orbit Status Report blog [Internet]. Houston. Available from: https://blogs.nasa.gov/stationreport/2022/02/page/15/
- 42. Aristotle of Stageira. Metaphysics, Volume I: Books 1–9, Tredennick H, translator. Loeb Classical Library 271. Cambridge, MA, Harvard University Press; 1933. pp. 429–473.
- 44. Hodder I. Entangled: An archaeology of the relationships between humans and things. Hoboken. NJ, Wiley-Blackwell; 2012.
- 45. Malafouris L., How Things Shape the Mind: A Theory of Material Engagement (MIT Press, 2016).
- 46. Keeter B. ISS daily summary report– 3/11/2022. 2022 Mar 11 [cited May 12 2024]. NASA ISS On-Orbit Status Report blog [Internet]. Houston. Available from: https://blogs.nasa.gov/stationreport/2022/03/page/15/

An official website of the United States government
Here's how you know
Official websites use .gov A .gov website belongs to an official government organization in the United States.
Secure .gov websites use HTTPS A lock ( ) or https:// means you’ve safely connected to the .gov website. Share sensitive information only on official, secure websites.
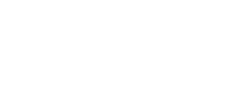
- Digg
Latest Earthquakes | Chat Share Social Media
San Diego River Shot Hole Borer Study (ver. 2.0, August 2024)
Data presented are results of vegetation sampling conducted in 2019-20 and 2023 in 24 survey plots to document the status of infestation by shot hole borers (Euwallacea sp.) on the riparian vegetation at the San Diego River. This dataset presents location, percent cover of different vegetation types, and number of seedlings for each survey plot. In addition, for each tree and sapling in each plot we present species, size, tree status (live, resprouting, recently dead from shot hole borer, or dead from another cause or unknown cause), infestation status (infested by shot hole borer, not infested by shot hole borer, or infestation not confirmed as shot hole borer), and crown condition. For every tree we also present location, tree tag number, and density of shot hole borer entrance holes.
Citation Information
Publication Year | 2023 |
---|---|
Title | San Diego River Shot Hole Borer Study (ver. 2.0, August 2024) |
DOI | |
Authors | Barbara E Kus, Shannon M Mendia |
Product Type | Data Release |
Record Source | |
USGS Organization | Western Ecological Research Center - Headquarters |
Related Content
Barbara kus, supervisory research ecologist.
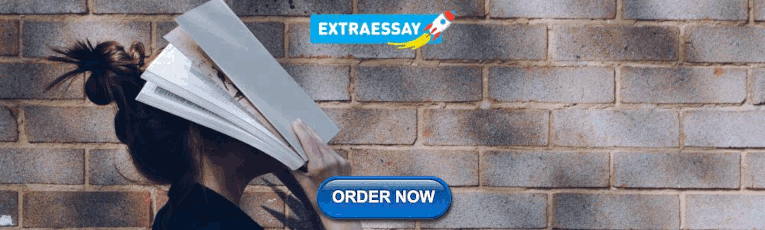
IMAGES
COMMENTS
In survey research, sampling is the process of using a subset of a population to represent the whole population. To help illustrate this further, let's look at data sampling methods with examples below. ... to understand the nuances and applications of each method to ensure that the chosen sampling strategy aligns with the research goals ...
You assign a number to every employee in the company database from 1 to 1000, and use a random number generator to select 100 numbers. 2. Systematic sampling. Systematic sampling is similar to simple random sampling, but it is usually slightly easier to conduct.
Survey research means collecting information about a group of people by asking them questions and analyzing the results. To conduct an effective survey, follow these six steps: Determine who will participate in the survey. Decide the type of survey (mail, online, or in-person) Design the survey questions and layout.
Survey sampling methods are essential techniques used in statistics and research to gather data from a subset of a larger population. The objective is to obtain accurate and reliable information about the entire population while minimizing costs and time. We'll now cover some common survey sampling methods.
You then choose the individual survey participants through the systematic sampling method. Clustered sampling. This method is used when you want to subdivide a sample into smaller groups or clusters that are geographically or organizationally related. Let's say you're doing quantitative research into candy sales.
survey research, and other areas of social science research. ... The main methodological issue that influences the generalizability of clinical research findings is the sampling method. In this ...
In survey research, sampling is the process of using a subset of a population to represent the whole population. Let's say you wanted to do some research on everyone in Europe. To ask every person would be almost impossible. ... Non-probability sampling methods don't offer the same bias-removal benefits as probability sampling, but there ...
Non-Probability Sampling Methods. Two of the main types of non-probability sampling methods are voluntary samples and convenience samples. Voluntary sample. A voluntary sample is made up of people who self-select into the survey. Often, these folks have a strong interest in the main topic of the survey.
Survey research is defined as "the collection of information from a sample of individuals through their responses to questions" ( Check & Schutt, 2012, p. 160 ). This type of research allows for a variety of methods to recruit participants, collect data, and utilize various methods of instrumentation. Survey research can use quantitative ...
Sample design is key to all surveys, fundamental to data collection, and to the analysis and interpretation of the data. Introduction to Survey Sampling, Second Edition provides an authoritative and accessible source on sample design strategies and procedures that is a required reading for anyone collecting or analyzing survey data.
The purpose of sampling is to reduce the cost and/or the amount of work that it would take to survey the entire target population. A survey that measures the entire target population is called a census. A sample refers to a group or section of a population from which information is to be obtained. Survey samples can be broadly divided into two ...
Survey sampling is selecting members from a target population to be in a sample for a sample survey. Usually the survey is some type of questionnaire (i.e. in-person, phone or internet survey). Census taking in The Netherlands, c. 1925. The science of survey sampling has greatly transformed the way we view society and issues facing society.
Choose the sampling method: Select an appropriate sampling method based on the research question, characteristics of the population, and available resources. ... Collect data: Once the sample has been selected, collect data from each member of the sample using appropriate research methods (e.g., surveys, interviews, observations).
Fundamentals of Survey Research Methodology April 2005 Priscilla A. Glasow (25988) Division: Department: W800 W804 . Washington C3 Center McLean, Virginia . ... The sampling plan is the methodology that will be used to select the sample from the population (p. 6). The sampling plan describes the approach that
Survey Research. Definition: Survey Research is a quantitative research method that involves collecting standardized data from a sample of individuals or groups through the use of structured questionnaires or interviews. The data collected is then analyzed statistically to identify patterns and relationships between variables, and to draw conclusions about the population being studied.
In this section, we consider how to go about conducting a survey. We first consider the issue of sampling, followed by some different methods of actually collecting survey data. Sampling. Essentially all psychological research involves sampling—selecting a sample to study from the population of interest. Sampling falls into two broad categories.
Call our friendly, no-pressure support team. +1 801 477 4700. Sampling Methods in Survey Research: Definition, Types, Methods, and More. Differences Between Probability and Non-Probability Sampling. and analysis in marketing research and the social sciences. It involves selecting a subset of individuals from a larger population to participate ...
Exploring Survey Sampling Methods: Types, Techniques, and Tips For Academia. Learn about the types, techniques, and tips for survey sampling methods to effectively gather data from your target population. Whether pursuing a PhD or compiling a graduate thesis, academic dissertations live and die by research methodology. At the heart of solid ...
1. Random Sampling. The purest form of sampling under the probability approach, random sampling provides equal chances of being picked for each member of the target population. 2. Stratified Sampling. Stratified sampling involves the use of "stratum", or a subset of the target population wherein the members possess one or more common attribute.
Online Survey, Research, Sampling 8 Types of Survey Sampling + Pros & Cons, Errors & Bias, and More. ... What is Survey Sampling? Sampling is a method of surveying a small portion of the population that represents a larger whole. To go back to our initial example, the U.S. Census Bureau surveys a sample population of 3.5 million households to ...
Since 2014, Pew Research Center has conducted surveys online in the United States using our American Trends Panel (ATP), a randomly selected, probability-based sample of U.S. adults ages 18 and older. The panel was initially built to supplement the prevalent mode of data collection at the Center during that time: random-digit-dial (RDD ...
At present, how to select a limited but representative sample dataset from the existing land information database to guide the new round of land survey and assessment sampling is a critical issue for land sampling strategy study. As a case study to determine and analyze the sample capacity and sample spatial location of land survey sampling for the study area, Panyu District in Guangzhou, the pape
Survey Research Methodology. This is a research methodology that involves the collection of data from a sample of individuals using questionnaires or interviews. Survey research is often used to study attitudes, opinions, and behaviors. ... Include details such as their demographics, sampling method, sample size, and any exclusion criteria used.
Simple Random Sample: A simple random sample is a subset of a statistical population in which each member of the subset has an equal probability of being chosen. An example of a simple random ...
Identify the Target Population and Sampling Method. Determine who you need to survey to achieve your research objectives. This step involves several key considerations: a) ... Survey research is an invaluable method for DepEd personnel to assess situations and gather data to inform action research projects. By following a systematic approach to ...
Importance The ways in which we access, acquire, and use data in clinical trials have evolved very little over time, resulting in a fragmented and inefficient system that limits the amount and quality of evidence that can be generated.. Observations Clinical trial design has advanced steadily over several decades. Yet the infrastructure for clinical trial data collection remains expensive and ...
Previous research in survey sampling has predominantly focused on clear, well-defined data, while classical sampling methods yield precise results, albeit with potential risks of inaccuracies, overestimations, or underestimations. However, classical methods fall short when handling set-type or undetermined data, characteristic of neutrosophic ...
Between January and March 2022, crew aboard the International Space Station (ISS) performed the first archaeological fieldwork in space, the Sampling Quadrangle Assemblages Research Experiment (SQuARE). The experiment aimed to: (1) develop a new understanding of how humans adapt to life in an environmental context for which we are not evolutionarily adapted, using evidence from the observation ...
To apply the method accurately, it is important to build appropriate CKMR models that do not violate assumptions linked to the species' and population's biology and sampling methods. In this study, we evaluated the implications of fitting overly simplistic CKMR models to populations with complex reproductive success dynamics or selective ...
Data presented are results of vegetation sampling conducted in 2019-20 and 2023 in 24 survey plots to document the status of infestation by shot hole borers (Euwallacea sp.) on the riparian vegetation at the San Diego River. This dataset presents location, percent cover of different vegetation types, and number of seedlings for each survey plot.