Thank you for visiting nature.com. You are using a browser version with limited support for CSS. To obtain the best experience, we recommend you use a more up to date browser (or turn off compatibility mode in Internet Explorer). In the meantime, to ensure continued support, we are displaying the site without styles and JavaScript.
- View all journals
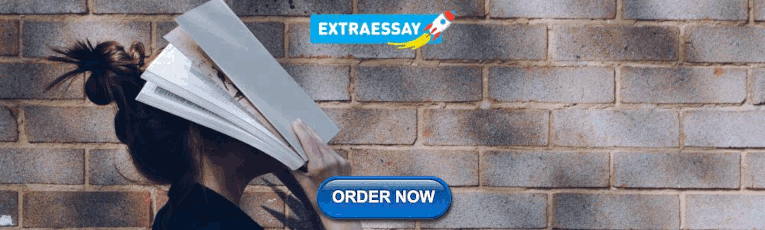
Business and commerce articles from across Nature Portfolio
Related subjects.
- Business and management
- Information systems and information technology
- Operational research
Latest Research and Reviews
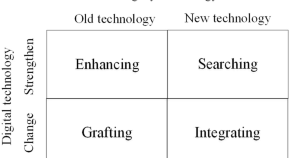
Combining digital and legacy technologies: firm digital transformation strategies—evidence from Chinese manufacturing companies
- Hongfang Cui
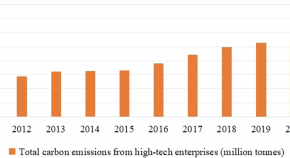
Investor attention and environmental performance of Chinese high-tech companies: the moderating effects of media attention and coverage sentiment
- Yanpeng Chen
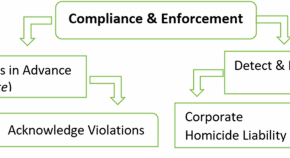
Intertwine preventive ( ex-ante ) and deterrent ( ex-post ) mechanisms for compliance with and enforcement of labor laws: a comprehensive approach for Bangladesh
- Robayet Ferdous Syed
CSR decoupling and stock price crash risk: Evidence from China
- Mengjiao Xu
- Xiangyu Chen
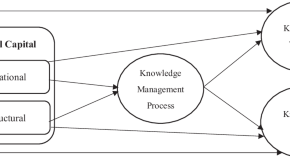
Breaking down barriers: exploring the impact of social capital on knowledge sharing and transfer in the workplace
- Michael Yao-Ping Peng
Regional cultural inclusiveness and firm performance in China
- Guangfan Sun
- Changwei Guo
News and Comment
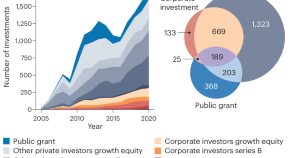
Rapid rise in corporate climate-tech investments complements support from public grants
Investment in climate and energy (climate-tech) startups is growing in the US and worldwide, with public grants backing high-risk sectors and publicly funded startups exiting at higher rates with corporate investment. Public policies to incentivize corporate investment in these startups can therefore be an important, yet sometimes underestimated, part of meeting net-zero goals.
- Kathleen M. Kennedy
- Morgan R. Edwards
- Kavita Surana
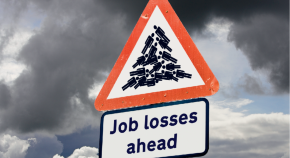
Job–environment feedbacks
- Angelos Alamanos
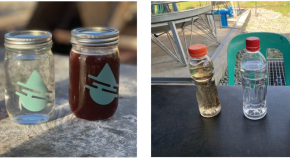
Five journeys from nanotechnology research to successful products in the water industry
Nanotechnology-based semipermeable membranes have been actively studied for their potential application in the production of clean water. Fundamental nanotechnology research has been turned into award-winning water industry products.
- Adrian M. Brozell
- Joris de Grooth
- Eric M. V. Hoek
Innovative dietary fat production
Fats and oils are crucial dietary supplements for human health. However, animal fats and palm oil dominate the food industry, with concerns over health and the environment that call for the development of fat alternatives. The fat industry offers a vast market potential, with several startups pioneering new technical solutions and attracting venture capital interest.
- Enrico Costanzo
- Giorgia Spigno
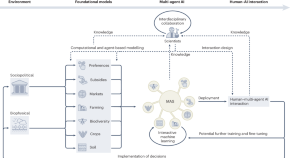
Hybrid intelligence for reconciling biodiversity and productivity in agriculture
Hybrid intelligence — arising from the sensible, targeted fusion of human minds and cutting-edge computational systems — holds great potential for enhancing the sustainability of agriculture. Leveraging the combined strengths of both collective human and artificial intelligence helps identify and stress-test pathways towards the reconciliation of biodiversity and productivity.
Can cities shape future tech regulation?
US cities are regulating private use of technology more actively than the federal government, but the likely effects of this phenomenon are unclear. City lawmaking could make up for national regulatory shortfalls, but only if cities can thread the needle of special interests and partisanship.
- Aileen Nielsen
Quick links
- Explore articles by subject
- Guide to authors
- Editorial policies

May 24, 2022 | 8 min read
The 7 Best Ecommerce Articles You Need to Read

Ecommerce is experiencing monumental growth. It went from being a $1.3 trillion industry in 2014 to a $3.5 trillion industry in 2019.
And experts predict that by 2023, it will reach more than $6.5 trillion.
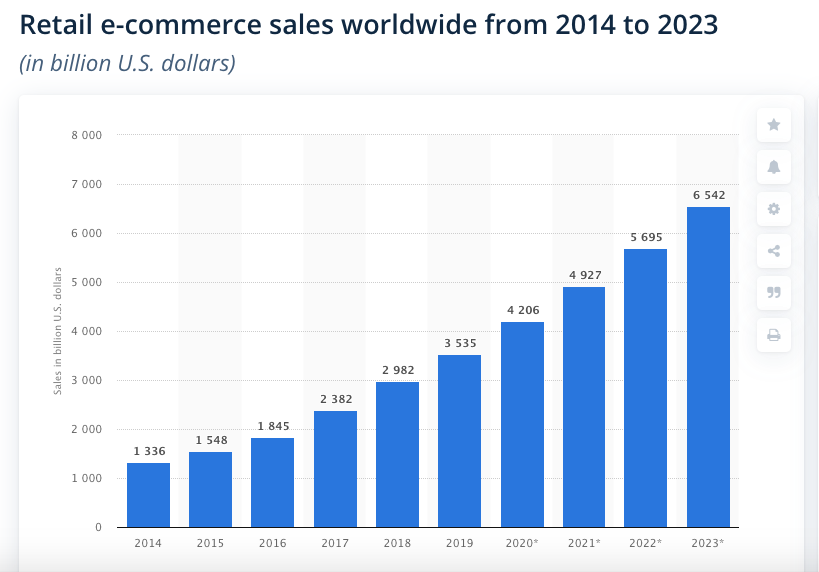
This means there’s a tremendous opportunity for ecommerce brands, and the sky is truly the limit.
But to be competitive and continually build your brand, you need to stay on top of trends, which aspects of ecommerce are changing, what customers are most receptive to with marketing, and so on.
For this post, I’ve compiled a list of the best ecommerce articles that cover these topics and more.
Here are seven of my favorites.
7 Best Ecommerce Articles You Need to Read
1. the ultimate guide to ecommerce (hubspot), 2. global ecommerce statistics and trends to launch your business beyond borders (shopify), 3. 7 of the best shopify stores to inspire new e-tailers (drip).
- 4. The Future of Ecommerce (Oberlo)
- 5. Selling on Online Marketplaces (BigCommerce)
- 6. The ‘Amazon Effect' (Entrepreneur)
- 7. How to Create an App for Your Store (A Better Lemonade Stand)
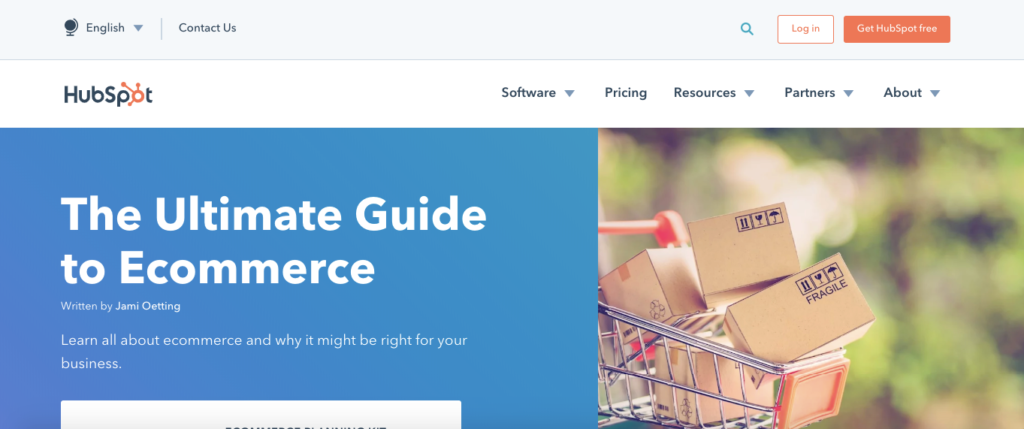
HubSpot is one of the top marketing and sales platforms on the market and used by countless ecommerce businesses.
They’re also known for their massive archive of epic content, including eBooks, guides, research reports, and of course, their multiple blogs, which cover marketing, sales, service, news, and trends.
This particular blog post is a must-read for new ecommerce business owners and is vital for learning the ropes.
That said, it’s still a great resource for more experienced ecommerce business owners as well, and can help you take your brand to the next level.
Like most other content from HubSpot, this article is comprehensive and highly detailed and covers six main areas.
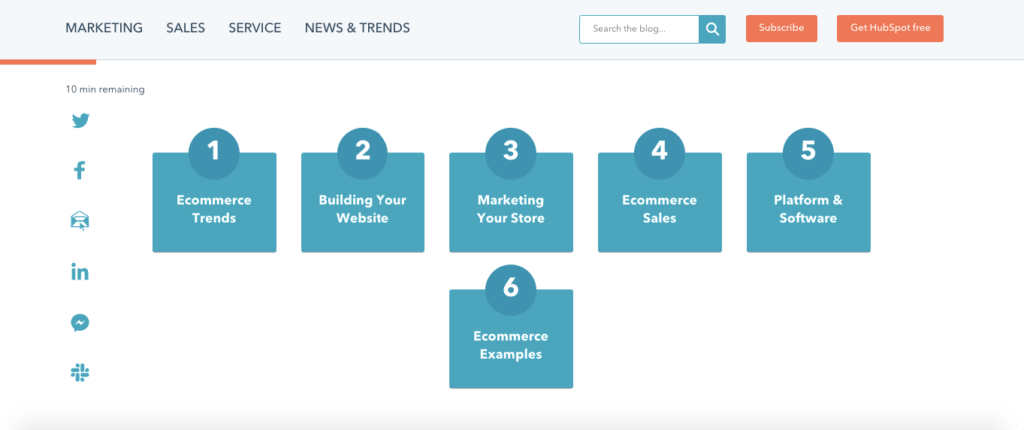
It’s jam-packed with a lot of great visuals and examples.
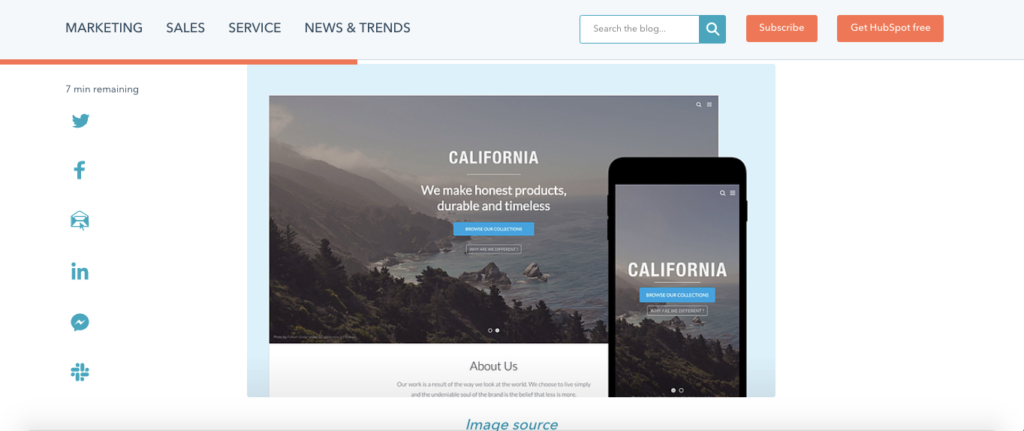
And the information is presented clearly and succinctly so that it flows logically, making it is easy to digest.
I especially like the section about how to increase ecommerce sales .
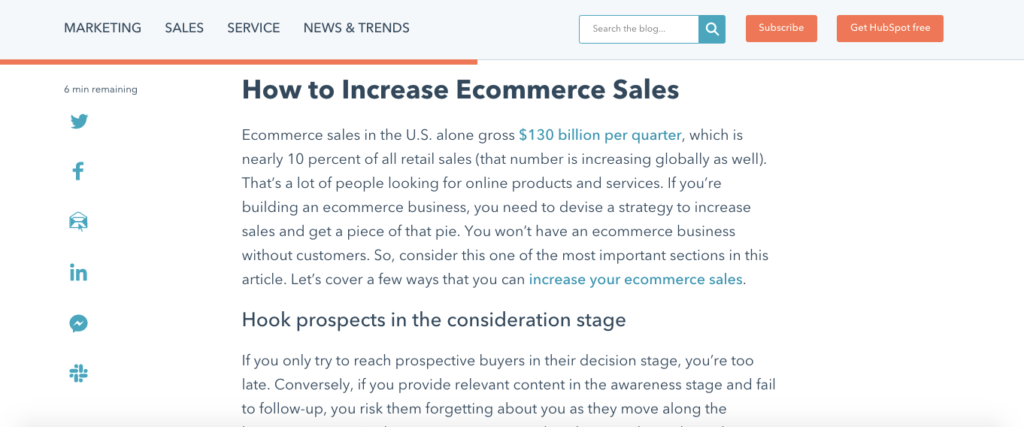
If you’re looking for a broad overview of ecommerce where you can quickly learn and ins-and-outs, I highly suggest checking out this article.
It’s a great starting point.
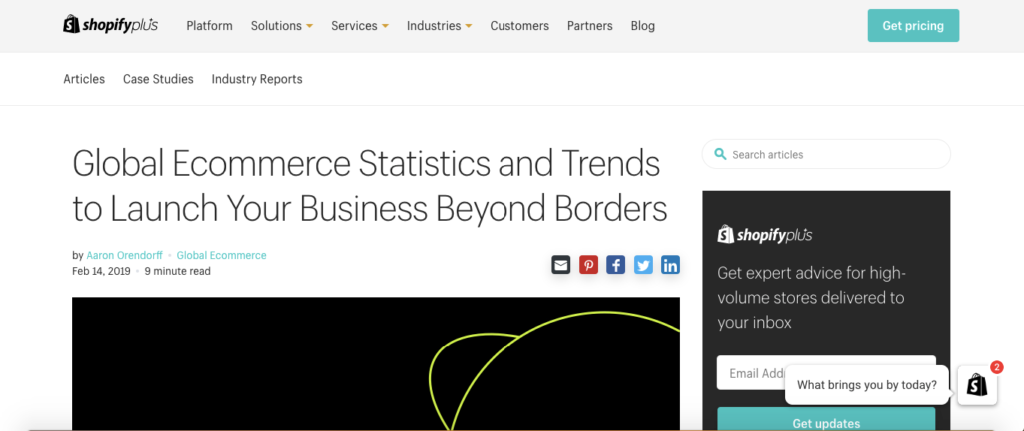
Shopify is one of my favorite ecommerce platforms.
It’s incredibly easy and intuitive to use and allows anyone to create a professional store that’s fully mobile responsive. And apparently, many other people love Shopify as well, considering that it powered over 800,000 digital stores in 2019.
I’m also a huge fan of their content.
They have some captivating case studies and industry reports that are super insightful, along with a fantastic blog that’s brimming with great insights.
One of my favorite articles from 2019 is this one about global ecommerce statistics and trends.
It covers 11 core topics.
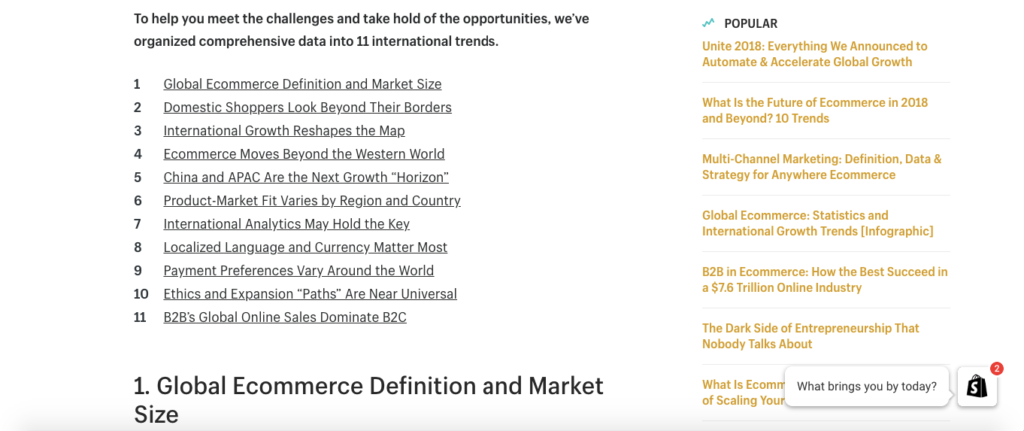
The entire article is compelling, but one of the more interesting points is that ecommerce is becoming highly globalized.
According to the article, “Online shoppers are increasingly looking outside their country’s borders for purchases. In fact, during one six-month evaluation, overseas purchases were in the majority on all but one continent: North America.”
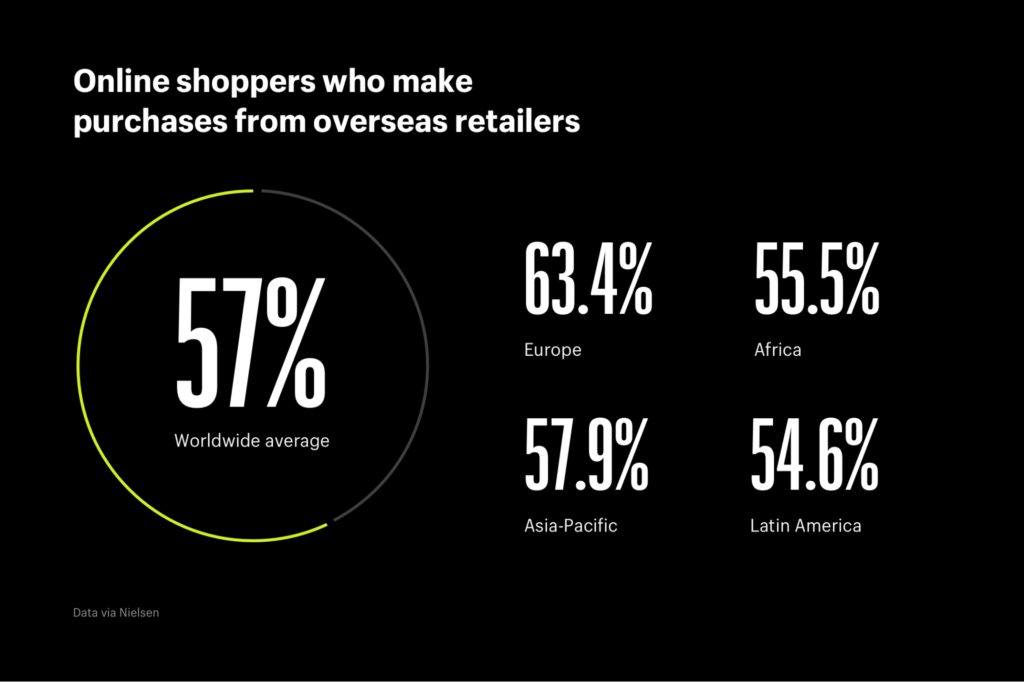
This shows the importance of thinking beyond your country’s borders and tapping into international markets.
I also like this graphic Shopify offers to visualize the 10 largest ecommerce markets in 2019.
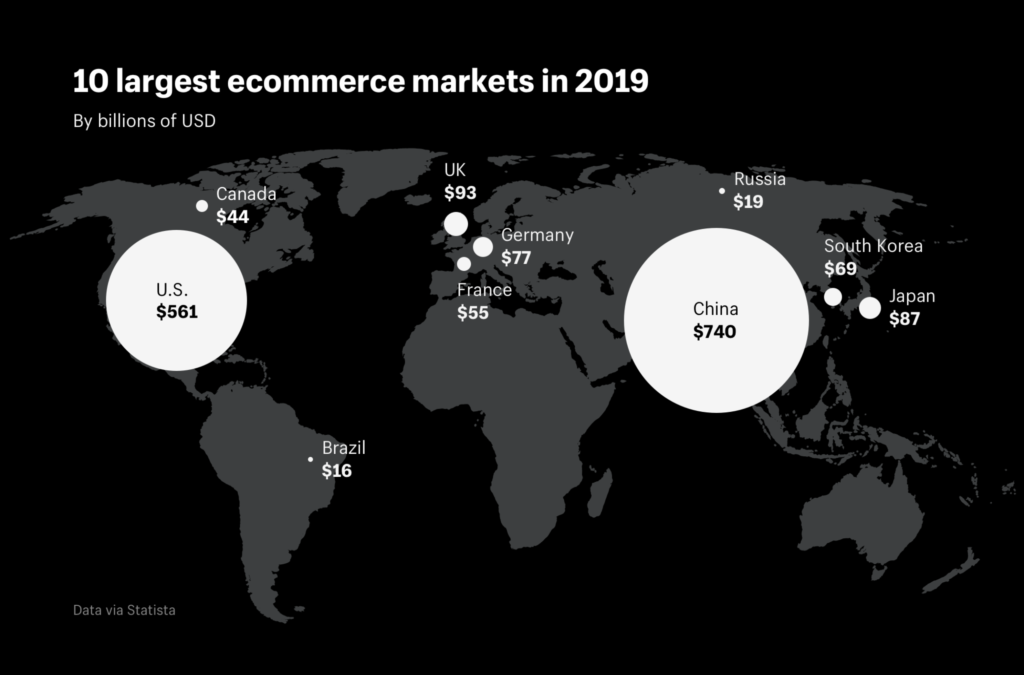
Besides that, they talk about the importance of using international analytics as well as a shopper’s native language and currency on your site. If you can do that, you’re going to have a huge competitive edge moving forward.
When it comes to the topic of selling internationally and elevating your ecommerce business to become a global brand , this is one of the top articles out there.
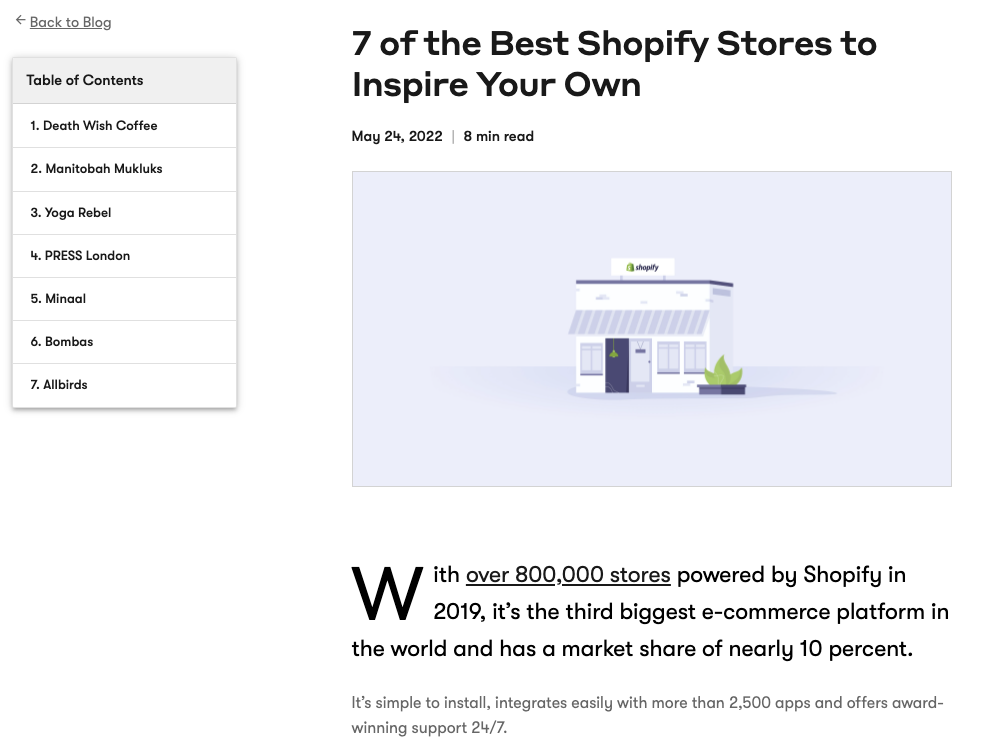
Speaking of Shopify, we created an article in 2019 that highlights seven of the best Shopify stores you can use for inspiration. I’m a big believer that one of the best ways to learn is to study examples of others that have already made it.
And this article does just that.
It outlines brands like Death Wish Coffee , Manitobah Mukluks , and Yoga Rebel, explaining what their unique selling propositions are and exactly what they do to create a seamless experience for digital shoppers.
For example, this article talks about how Manitobah Mukluks—a company that specializes in high quality, super warm winter boots—writes killer copy, effectively demonstrates what makes their boots unique and offers straightforward navigation.
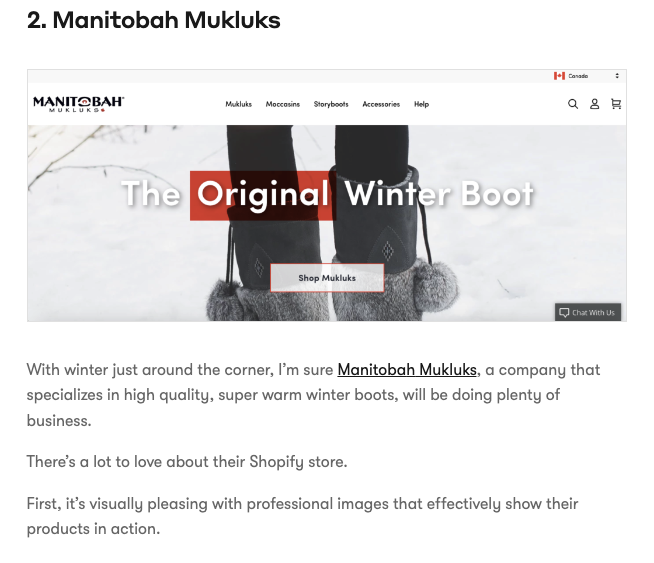
And even if you use another platform like Magento or WooCommerce, you can still get some helpful insights.
4. The Future of Ecommerce: How Ecommerce Will Change in 2019 and Beyond (Oberlo)
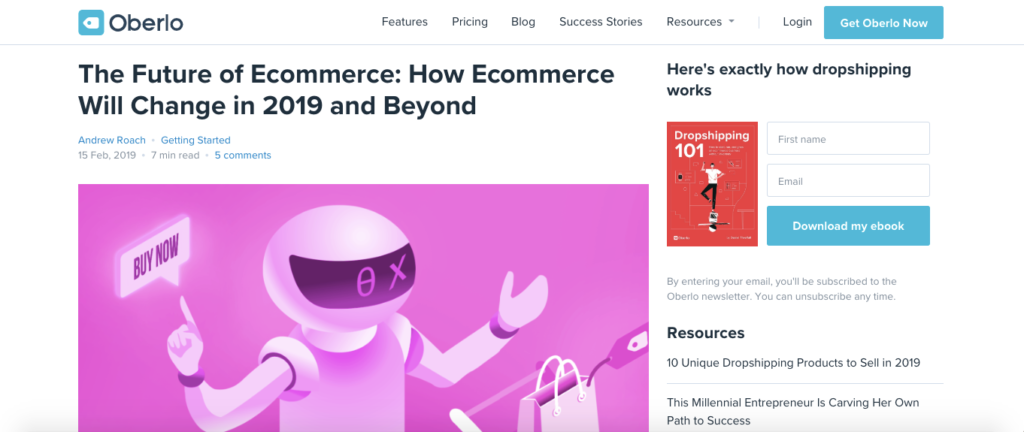
Oberlo is a dropshipping platform that “allows you to easily import products from suppliers directly into your Shopify store and ship directly to your customers—in only a few clicks.”
They’ve also got a great blog that runs the gamut in terms of ecommerce topics .
I think this article about the future of ecommerce is incredibly timely and relevant and gives you a good idea of where the industry is heading over the next few years.
It starts with some interesting stats, saying there was a 23.3 percent increase in ecommerce sales from 2017 to 2018, and the average growth rate of ecommerce sales was 25.6 percent from 2014 to 2017.
Then it moves onto talking about the importance of creating a robust digital shopping experience where customers can easily access your site across multiple devices.
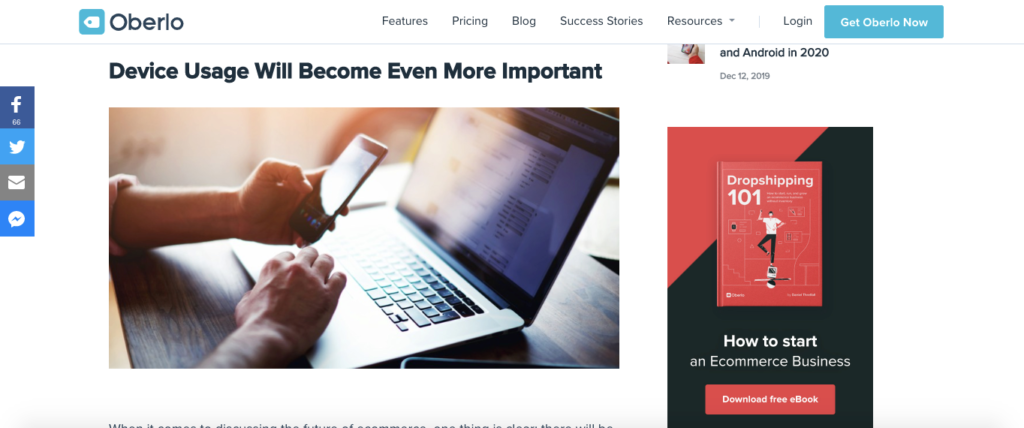
From there, it discusses emerging global markets like India, China, Brazil, Russia, and South Africa—a topic that overlaps with the article from Shopify that I mentioned earlier about globalization.
And there’s a ton of other interesting information like the growing role video is playing in ecommerce shopping, why more marketers are incorporating content into their strategy, the importance of personalization, and more.
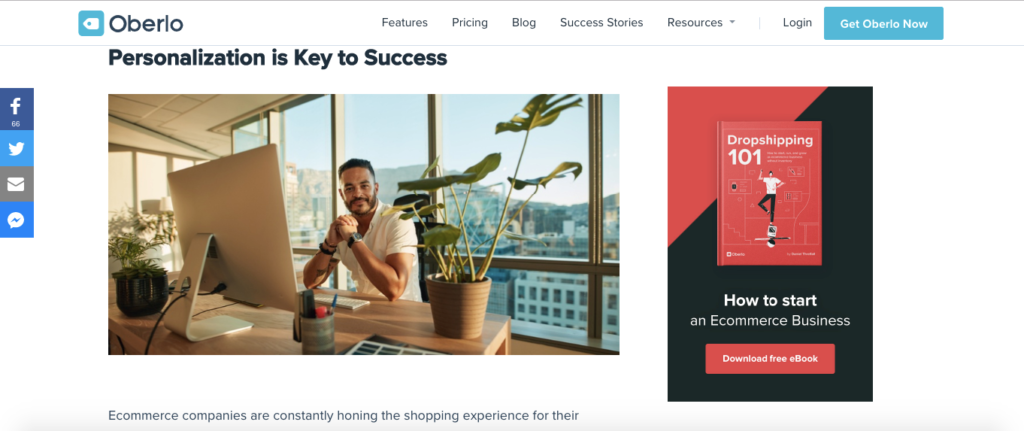
If you’re a future-oriented ecommerce owner (which you should be), this article is a great read and will get you up to speed on what’s in store.
5. Selling on Online Marketplaces: Best Platforms for Selling Your Products (BigCommerce)
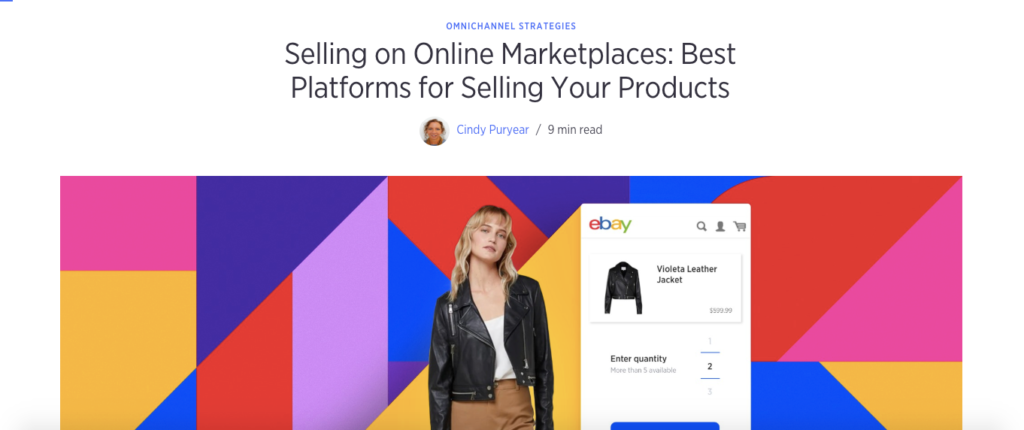
When you get right down to it, the ecommerce brands that are the most successful are usually the ones that get in front of the largest audiences. It’s all about bringing in traffic, and lots of it.
That’s what this article from BigCommerce—a top-rated ecommerce platform—addresses. More specifically, it talks about how getting your products listed on major online marketplaces like Amazon, eBay, and Facebook Marketplace can dramatically increase your sales.
It also mentions that there are numerous niche online marketplaces like Bonanza , Fruugo , Hollar and Newegg that allow ecommerce brands to target specific audiences.
For instance, Bonanza focuses on fashion and home decor, while Newegg focuses on computer parts and hardware.
According to BigCommerce, “There are now over 100 online marketplaces you could potentially leverage to reach customers doing their online shopping—both within the United States and overseas in areas like Japan and Europe, with trillions of dollars in revenue potential just waiting to be tapped.”
BigCommerce goes over the benefits of selling on online marketplaces, including fast launch times, access to established programs, and large customer bases.
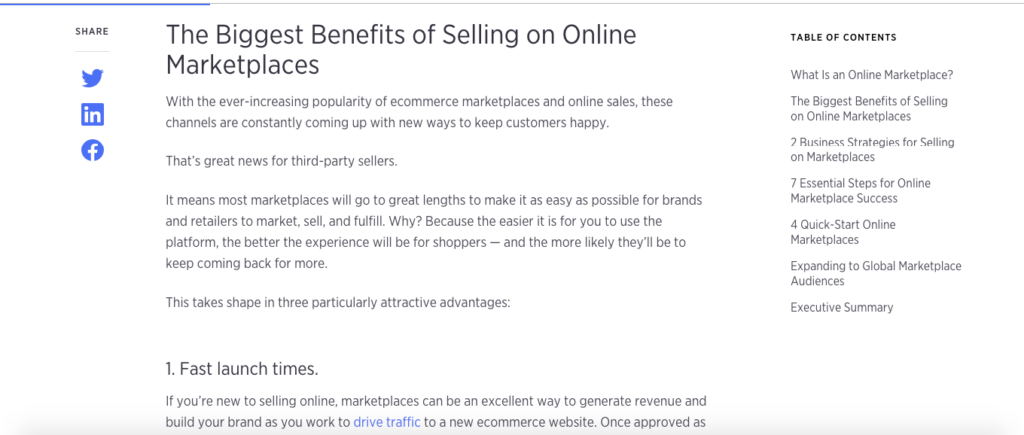
They also cover strategies for selling on these marketplaces and offer tips for increasing your chances of success.
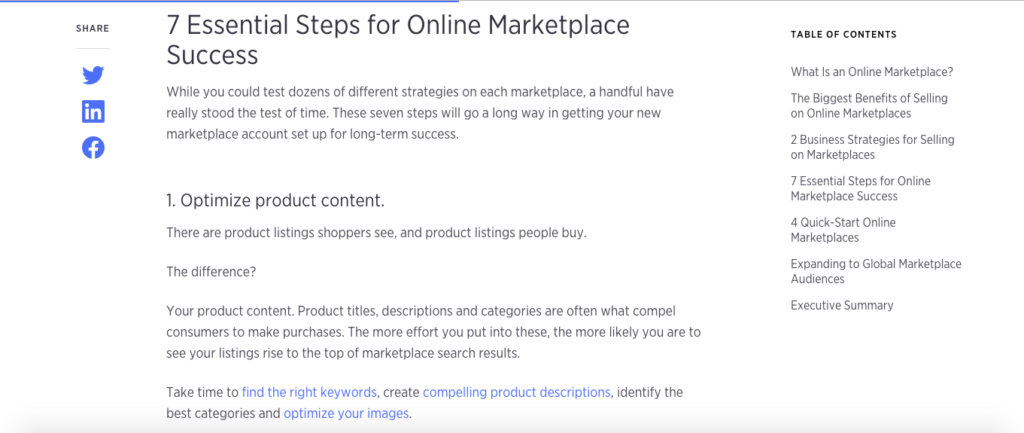
Although not every ecommerce business owner is interested in this type of partnership, this is an excellent article for those who are.
6. The ‘Amazon Effect’: How Ecommerce Will Change in 2019 and Beyond (Entrepreneur)
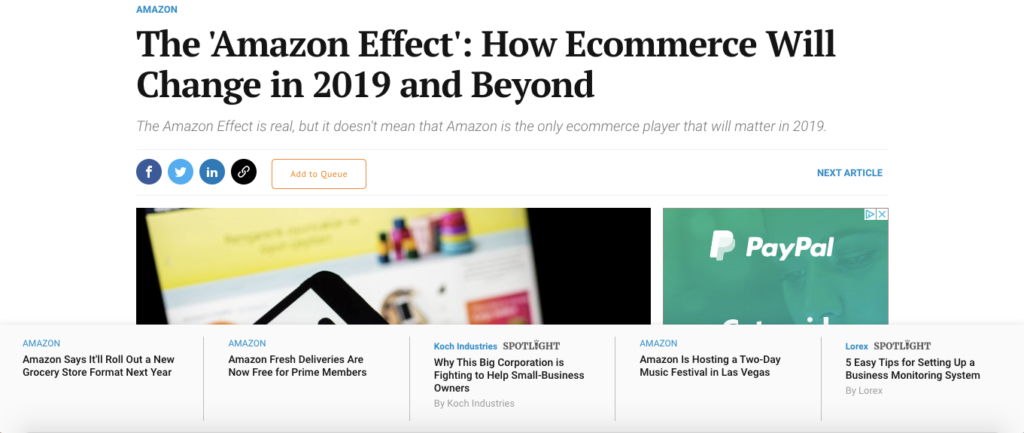
I probably don’t need to tell you how much of an influence Entrepreneur Magazine has on the business world. It’s been a leading publication for years and is one of the main resources ecommerce brands turn to for advice.
They cover about every topic imaginable and currently have nearly 42,000 articles involving ecommerce.
One that I found especially interesting in 2019 was this piece on the “Amazon Effect,” which refers to the difficulty modern brands have competing with Amazon.
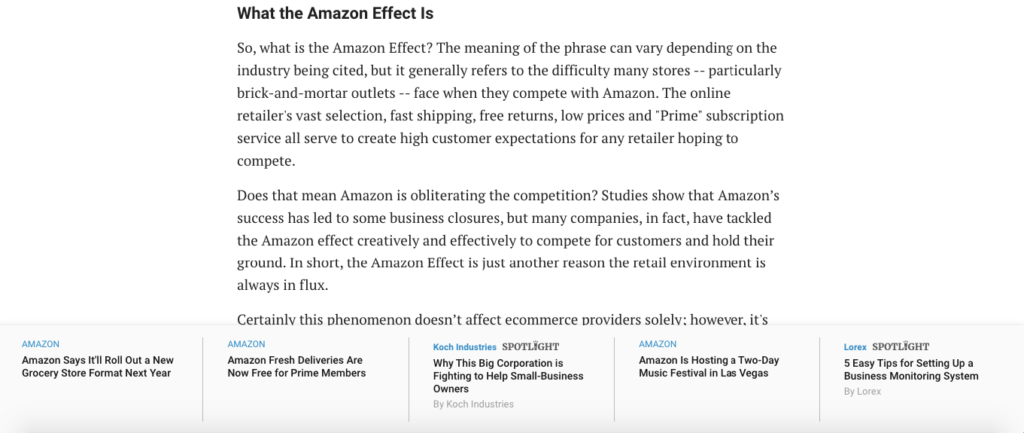
Given that Amazon accounted for roughly 50 percent of American ecommerce sales in 2018 and 5 percent of combined offline and online sales, I’d say the effect is real.
It’s a fairly short article, but it paints a realistic picture of what ecommerce brands must contend with in 2019 and beyond. More specifically, it says, “it’s these providers that are most on the front lines and need to stay agile lest their behemoth competitor, Amazon, swipe in and take their customers.”
This doesn’t mean that you need to panic or throw in the towel. But it does mean that you may need to adjust your ecommerce strategy to coexist with this digital juggernaut.
The author also touches on some things ecommerce store owners can do to keep up, including speeding up delivery times, taking a mobile-first approach, and using AI-driven techniques like product suggestions to personalize the shopping experience.
So if you have any concern with how Amazon’s popularity is impacting smaller ecommerce brands, I highly recommend reading this article.
7. How to Create an App for Your Store: Why & How to Use No-Code Ecommerce App Development (A Better Lemonade Stand)
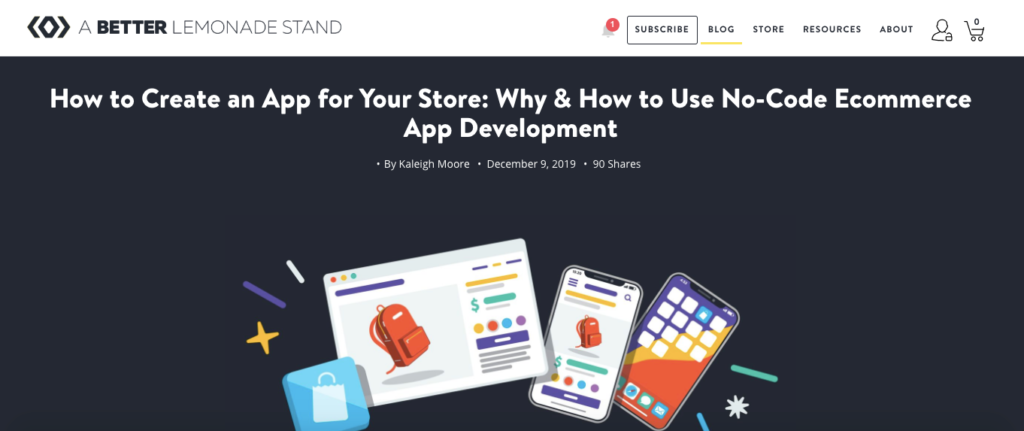
This particular article talks about a topic that’s becoming increasingly important to many ecommerce brands—building a store app.
And there are two main reasons why.
- “For retailers who invest in both mobile and web shopping apps, these apps account for 70 percent of sales.”
- “Shopping apps feature a conversion rate up to 3x higher than those on mobile alone.”
When developed correctly, having an app for your store can be a serious game-changer. Not only does it create a far more streamlined customer experience, it can send conversions through the roof.
If you look at the world’s top ecommerce companies, they pretty much all have their own apps.
Online fashion brand ASOS is a great example.
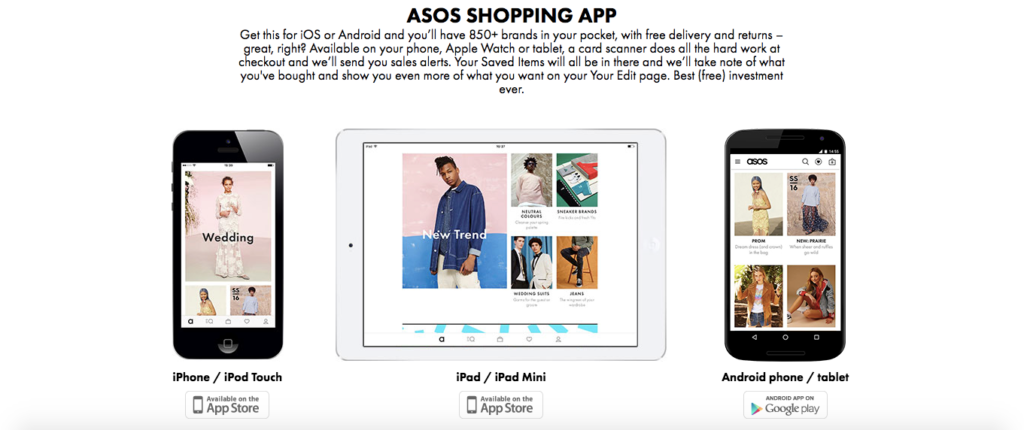
It allows shoppers to conveniently browse through their products, save items, and make purchases.
But here’s the thing.
I think many smaller ecommerce brands feel a little intimidated by the idea of building an app. After all, it sounds complicated and costly.
But we’re at an interesting point in time where you don’t necessarily need a team of brainy developers and a massive budget to create an app.
It’s becoming surprisingly attainable, even for microbrands. And that’s the crux of this article.
Now entrepreneurs can use what’s known as a “no-code” platform like GoodBarber to develop an app.
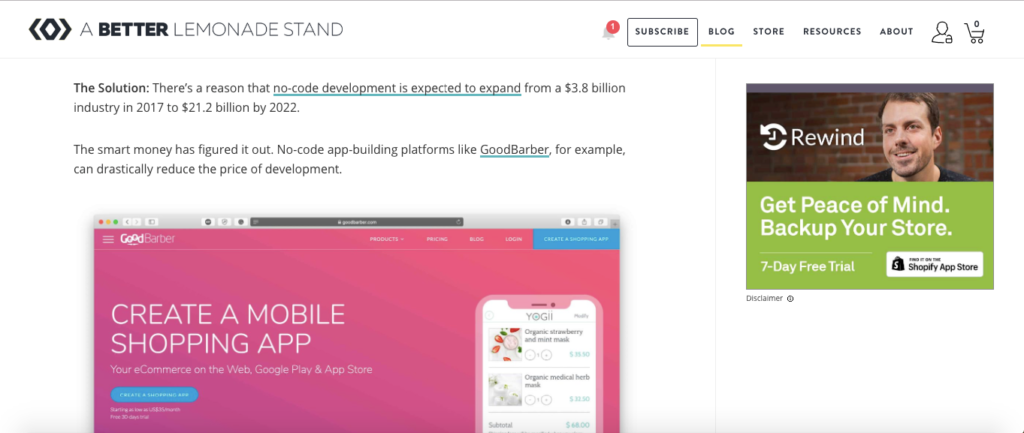
This is a tool that makes the app-building process incredibly simple without having to use any code.
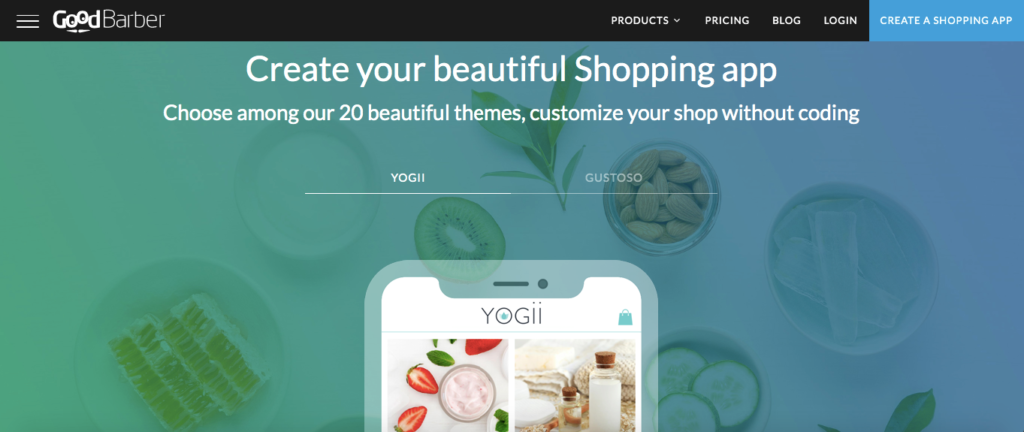
It’s also quite affordable and can be as low as $35 per month. When you consider that expert app firms charge up to $171,450 , this is a major breakthrough and helps level the playing field.
In this article, you’ll learn about the main pain points that have historically existed with app development and learn the basics of how to create your own ecommerce app.
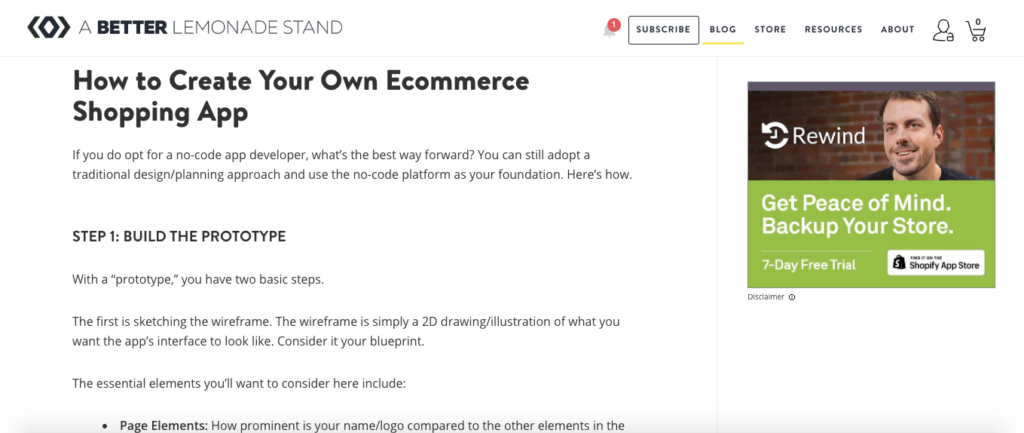
It’s really informative. So if this is something you’ve been interested in but just didn’t think was possible, this is the article for you.
Evolving technology, globalization, AI, and the Amazon Effect are just a few factors that are leading to big changes.
To stay ahead of the game, it’s crucial to stay up-to-date on current trends and learn how to upgrade your brand continuously.
Fortunately, there’s no lack of excellent blogs that can teach you everything you need to know. There was a lot of great content created this year about these topics, but these are some of the best ecommerce articles to bookmark.
Hopefully, you’ll find them as compelling as I did and can use the information to propel your brand into 2021 and beyond.

Emil Kristensen
Emil is the CMO of Drip. When he’s not busy writing awesome content and building the Drip brand, he spends his time reading blog posts and listening to podcasts.
Come for the automated marketing. Stay for the endless revenue growth.
Start a 14-day free trial, no credit card required.
Related posts
Learn more about drip.
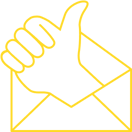

21+ Best And Interesting Research Topics For Commerce Students In 2023
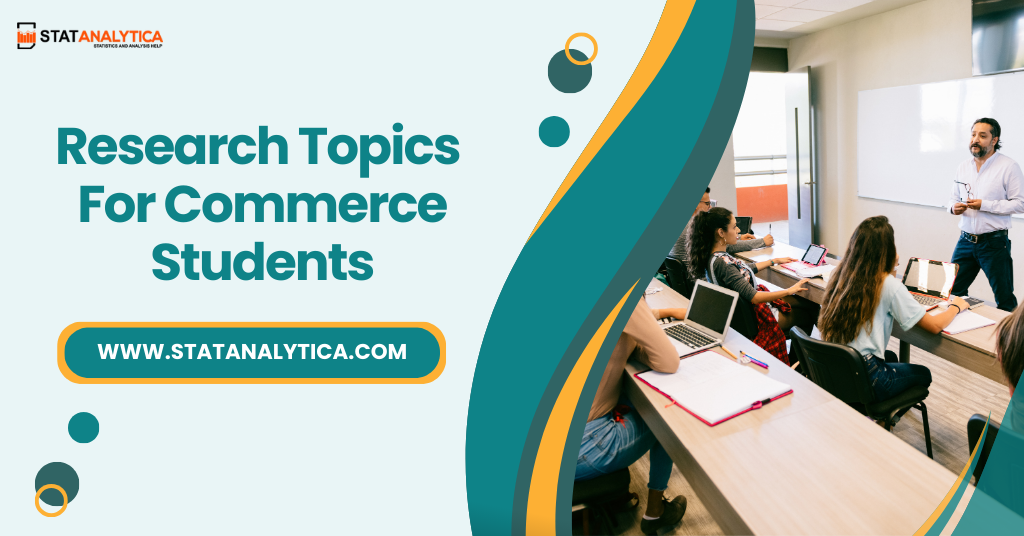
Research is the systematic quest for knowledge, a crucial component in the academic journey. It holds immense significance for commerce students, as it provides insights into the complex world of business, economics, and finance. Understanding the importance of research in commerce studies can unlock countless opportunities for growth and innovation.
Choosing a research topic in commerce is a vital step. It’s the compass that guides your academic expedition. In this blog, research topics for commerce students, we delve into the intricacies of this process, offering 21+ captivating ideas for 2023.
Our topics span various subfields, from Accounting and Finance to Marketing and Sales Management. We will also share valuable tips for successfully completing your commerce research and tackling the challenges commerce students face during their research journey.
Stay tuned with us to explore the exciting research topics for commerce students.
What Is Research?
Table of Contents
Research is like being a detective, but instead of solving crimes, you’re solving puzzles about the world. It’s a careful and organized way to find out more about something. Imagine you have a big question, like “How do plants grow?” or “What makes people happy?” Research helps you gather information, like reading books, asking experts, or doing experiments to find answers to your questions. It’s like putting together pieces of a jigsaw puzzle until you see the full picture, and it’s how we learn new things about the world around us.
Importance Of Research In Commerce Studies
Here are some importance of research in commerce studies:
1. Informed Decision-Making
Research in commerce studies provides the information needed to make smart choices, ensuring decisions are based on facts rather than guesswork, which is essential for success.
2. Keeping Up with Trends
Commerce research keeps you updated on what’s new and popular, helping you adapt your products or services to stay appealing and competitive in the market.
3. Solving Problems and Being Creative
Research encourages creative problem-solving, offering fresh ideas and solutions to navigate challenges and improve how you do business.
4. Understanding Your Customers
Your customers’ likes and needs can help you make your products and services better, which will make them happy and keep them coming back.
5. Planning for the Future
Commerce research serves as a crystal ball, helping you prepare for future challenges and opportunities, ensuring you’re ready for what lies ahead.
How To Choose A Research Topic In Commerce?
Choosing a research topic in commerce is a crucial step in the research process. Your topic should be interesting to you and relevant and significant in the field of commerce. Here are seven key considerations to help you select the right research topic:
- Identify Your Interests: Start by exploring your own interests and passion within the field of commerce. Choosing a topic that genuinely excites you will make the research process more enjoyable and productive.
- Review Current Trends: Stay updated on the latest trends and developments in commerce. A relevant and timely topic is more likely to capture the attention of your audience and contribute to the existing knowledge.
- Narrow Down Your Focus: Commerce is a broad field; therefore, it’s essential to narrow down your research focus. Consider specific aspects of commerce, such as e-commerce, international trade, or marketing strategies.
- Research Feasibility: Assess the availability of resources, data, and information for your chosen topic. Ensure you can access the necessary research materials to support your investigation.
- Consider the Impact: Think about the potential impact of your research. Will it address a particular problem, provide practical solutions, or offer valuable insights to the commerce community?
- Consult with Advisors : Talk to your academic teachers or mentors for help. Based on their knowledge, they can give you useful advice and help you narrow down your study topic.
- Pilot Research: Before finalizing your topic, consider conducting preliminary research or a pilot study to gauge the depth and scope of the chosen subject. This can help you refine your focus further.
Tips For Successfully Completing The Commerce Research
Here are some tips for completing the commerce research:
Tip 1: Define Your Research Question Clearly
To complete your commerce research successfully, start by defining your research question clearly. This question will guide your entire study and help you stay focused on what you want to investigate. Ensure it’s specific and well-defined to avoid getting lost in irrelevant information.
Tip 2: Plan Your Research Timeline
Create a research timeline to manage your time effectively. Break down your project into smaller tasks with deadlines. This will help you stay on top of things and avoid rushing at the last minute, giving you more time to do thorough research and analysis.
Tip 3: Gather Reliable Sources
For a successful commerce research project, gather information from reliable sources. Use academic journals, books, reputable websites, and official reports. Citing credible sources will strengthen your research and demonstrate your knowledge.
Tip 4: Analyze and Interpret Data
After gathering information, don’t just show it; look it over and figure out what it means. Explain what the results mean and how they connect to your study question. If they help you get your point across, use charts, graphs, or lists.
Tip 5: Revise and Proofread Your Work
Before submitting your research, take the time to revise and proofread. Check for spelling, grammar, and formatting errors. Ensure your paper flows logically and presents your research in a well-structured manner. A polished final product will leave a positive impression on your readers.
In this section, we will discuss 21+ best and interesting research topics for commerce students in 2023:
I. Accounting and Finance
Accounting and Finance involve the management of money and resources within an organization. Here are three project topics in this category:
1. Financial Statement Analysis
Financial statement analysis projects focus on evaluating a company’s financial health by examining its balance sheet, income statement, and cash flow statement. It helps in making informed investment decisions or assessing a company’s creditworthiness.
- Ratio analysis
- Trend analysis
- Comparative analysis
- Common-size analysis
- DuPont analysis
2. Budgeting and Forecasting
Budgeting and forecasting projects aim to create accurate financial plans for the future. This is crucial for organizations to allocate resources effectively and achieve financial goals.
- Zero-based budgeting
- Rolling budgets
- Forecasting methods (e.g., time series analysis)
- Budget variance analysis
- Flexible budgeting
3. Risk Management and Insurance
These projects explore strategies to identify, assess, and mitigate financial risks within a business. It also involves examining the role of insurance in managing various types of risk.
- Risk assessment
- Hedging strategies
- Catastrophic risk management
- Types of insurance (e.g., property, liability, health)
- Claims management
II. Business Management
Business management involves overseeing and coordinating various aspects of an organization to achieve its goals. Here are three project topics in this category:
5. Strategic Management
Strategic management projects delve into creating and implementing long-term plans to achieve a company’s vision and objectives. It includes assessing the competitive environment, formulating strategies, and ensuring their execution.
- SWOT analysis
- Porter’s Five Forces
- Strategy formulation models (e.g., Ansoff Matrix)
- Strategy execution and control
- Corporate social responsibility in strategy
6. Leadership and Team Management
Projects in this area explore leadership styles, team dynamics, and how effective leadership can enhance team performance and organizational success.
- Transformational leadership
- Conflict resolution
- Motivation and empowerment
- Building high-performance teams
- Leadership ethics
7. Change Management
Change management projects focus on planning and implementing organizational changes. It involves understanding the psychology of change, communication strategies, and overcoming resistance.
- Kotter’s 8-Step Change Model
- Change communication plans
- Employee resistance and engagement
- Change metrics and evaluation
- Change leadership roles
III. Economics
Economics examines how societies allocate resources and make choices. Here are three project topics in this category:
8. Microeconomics of Consumer Behavior
Projects in this area explore how individuals make choices regarding consumption, budgeting, and the impact of factors like prices and income on these choices.
- Utility theory
- Consumer preferences
- Budget constraints
- Price elasticity of demand
- Consumer surplus
9. Macroeconomic Policy Analysis
For these projects, you have to look at how fiscal and monetary policies, among other things, affect the economies of different countries. It looks at how they affect progress and economic stability.
- Monetary policy tools (e.g., open market operations)
- Fiscal policy tools (e.g., taxation)
- Inflation targeting
- Exchange rate policies
- Economic growth models
10. Environmental Economics
Environmental economics projects explore the economic aspects of environmental issues, including resource allocation, externalities, and the design of policies to address environmental challenges.
- The Tragedy of the Commons
- Carbon pricing mechanisms
- Cost-benefit analysis of environmental projects
- Emission trading systems
- Sustainable Development Economics
IV. E-Commerce and Information Technology
E-Commerce and Information Technology focus on online business operations and the use of technology in modern commerce. Here are three project topics in this category:
11. E-Commerce Website Development
Projects in this area involve designing, developing, and optimizing e-commerce websites, considering user experience, security, and effective product listings.
- User interface design
- Shopping cart functionality
- Payment gateway integration
- Mobile-responsive design
- Search engine optimization (SEO)
12. Cybersecurity in E-Commerce
These projects address the critical need for cybersecurity in the e-commerce sector, including risk assessments, encryption, and fraud prevention strategies.
- Data encryption protocols
- Payment card security standards (PCI DSS)
- Phishing and identity theft prevention
- Penetration testing
- Regulatory compliance (e.g., GDPR)
13. Big Data and Analytics in E-Commerce
Big data projects explore the collection and analysis of large datasets to gain insights into consumer behavior, optimize supply chains, and improve decision-making.
- Data mining
- Customer segmentation
- Predictive analytics
- Recommendation engines
- Market basket analysis
V. Entrepreneurship and Small Business Management
Entrepreneurship and small business management focus on the creation and operation of small businesses. Here are three project topics in this category:
14. Business Plan Development
Projects in this area involve creating comprehensive business plans that cover market analysis, financial projections, and strategies for success.
- Market research and validation
- Financial forecasts
- Marketing strategies
- Operational plans
- Exit strategies
15. Startup Financing and Funding
These projects explore various sources of capital for startups, such as venture capital, angel investors, loans, and crowdfunding.
- Pitching to investors
- Equity financing
- Debt financing
- Crowdfunding platforms
- Bootstrapping strategies
15. Small Business Marketing Strategies
Marketing projects focus on cost-effective strategies for small businesses, including digital marketing, social media, content marketing, and customer relationship management.
- Social media advertising
- Email marketing
- Content marketing
- Customer retention strategies
|
VI. Global Business and International Trade
Global business and international trade explore the dynamics of commerce on a global scale. Here are three project topics in this category:
16. Market Entry Strategies for Foreign Markets
Projects in this area examine the different methods and considerations for entering and expanding into international markets, including exporting, franchising, joint ventures, and mergers and acquisitions.
- Market research and selection
- Entry mode analysis
- Legal and cultural considerations
- Global branding
17. Global Supply Chain Management
These projects delve into the complexities of managing global supply chains, optimizing logistics, and mitigating risks associated with international sourcing and distribution.
- Supply chain visibility
- Inventory management
- Logistics and transportation
- Vendor relationship management
- Risk mitigation strategies
18. International Trade Policy and Agreements
This area explores the role of government policies and international agreements in shaping trade relations between countries and regions.
- Trade barriers (e.g., tariffs, quotas)
- Regional trade agreements (e.g., NAFTA, EU)
- Dispute resolution mechanisms (e.g., WTO)
- Trade liberalization
- Trade compliance and documentation
- Export-import regulations
- Trade finance and letters of credit
VI. Law and Commerce
Law and commerce explore the legal framework businesses operate within. Here are three project topics in this category:
19. Business Contracts and Negotiation
Projects in this area examine the principles of contract law, negotiation strategies, and the drafting of effective business contracts to protect the interests of all parties involved.
- Elements of a contract
- Contract enforcement
- Contract negotiation tactics
- Boilerplate clauses
- Remedies for contract breaches
20. Intellectual Property Rights
These projects focus on understanding and protecting intellectual property rights, including trademarks, patents, copyrights, and trade secrets, to safeguard a company’s innovations and creative works.
- Trademark registration
- Patent application process
- Copyright infringement
- Trade secret protection
- IP licensing and litigation
21. Business Ethics and Corporate Social Responsibility (CSR)
Examining the ethical dimensions of commerce, these projects address corporate social responsibility, ethical decision-making, and the legal aspects of ethical business practices.
- Ethical theories (e.g., utilitarianism, deontology)
- CSR strategies
- Whistleblowing and ethical reporting
- Environmental regulations
- Ethical codes of conduct
VI. Marketing and Sales Management
Marketing and sales management focus on promoting products and services and driving revenue growth. Here are three project topics in this category:
22. Digital Marketing and Social Media Strategy
Projects in this area explore the use of digital channels and social media platforms for marketing, including content creation, advertising, and audience engagement.
- Social media marketing
- Content creation and curation
- Search engine marketing (SEM)
- Influencer marketing
- Analytics and ROI measurement
23. Consumer Behavior Analysis
These projects delve into understanding the psychology and behavior of consumers to develop effective marketing strategies and product positioning.
- Market segmentation
- Consumer decision-making process
- Brand loyalty and perception
- Pricing strategies
- Neuromarketing techniques
24. Sales Force Management and Sales Techniques
Examining the strategies and tactics for building and managing successful sales teams, these projects explore techniques for increasing sales revenue.
- Sales coaching training and development
- Sales performance metrics
- Customer relationship management (CRM)
- Sales prospecting and lead generation
- Sales closing techniques
Challenges Face By Commerce Students In Research And Overcome Techniques
Here are some challenges that are faced by commerce students in research:
1. Lack of Research Skills
Many commerce students face challenges in conducting research due to a lack of research skills, such as finding relevant sources and data, and structuring their work effectively.
Overcome Technique
- Take research skills workshops.
- Seek guidance from professors or librarians.
- Practice by starting with smaller research projects.
2. Time Management
Balancing research with coursework and other responsibilities can be difficult for commerce students, leading to time management challenges.
- Create a study schedule and stick to it.
- Break research tasks into smaller, manageable chunks.
- Use time management tools and apps.
3. Data Collection and Analysis
Many commerce students struggle with gathering and analyzing data, as it can be overwhelming and complex.
- Learn statistical tools and software.
- Collaborate with experts in data analysis.
- Seek mentorship for guidance on data handling.
4. Writing and Presentation
Communicating research findings effectively through writing and presentations can be a challenge for commerce students.
- Take writing and presentation courses.
- Practice concise and clear communication.
- Use templates and guidelines for structuring papers and presentations.
5. Lack of Motivation
Staying motivated throughout the research process can be a hurdle, particularly when facing setbacks or obstacles.
- Set clear goals and rewards.
- Find a study group or research partner for mutual motivation.
- Remember the long-term benefits of your research for motivation.
Research topics for commerce students serves as a valuable compass for budding commerce scholars. We’ve unveiled the significance of research, emphasizing its role in enhancing understanding and driving innovation in this field. Selecting a research topic is a pivotal step, and we’ve provided a diverse array of 21+ engaging research topics for commerce students, ensuring ample choices for 2023.
Furthermore, we’ve shared practical tips for successful research completion and addressed common challenges faced by commerce students, offering effective techniques for overcoming them. With this guide, you’re well-equipped to embark on your research journey, unlocking the door to discovery and academic achievement in the realm of commerce.
Related Posts
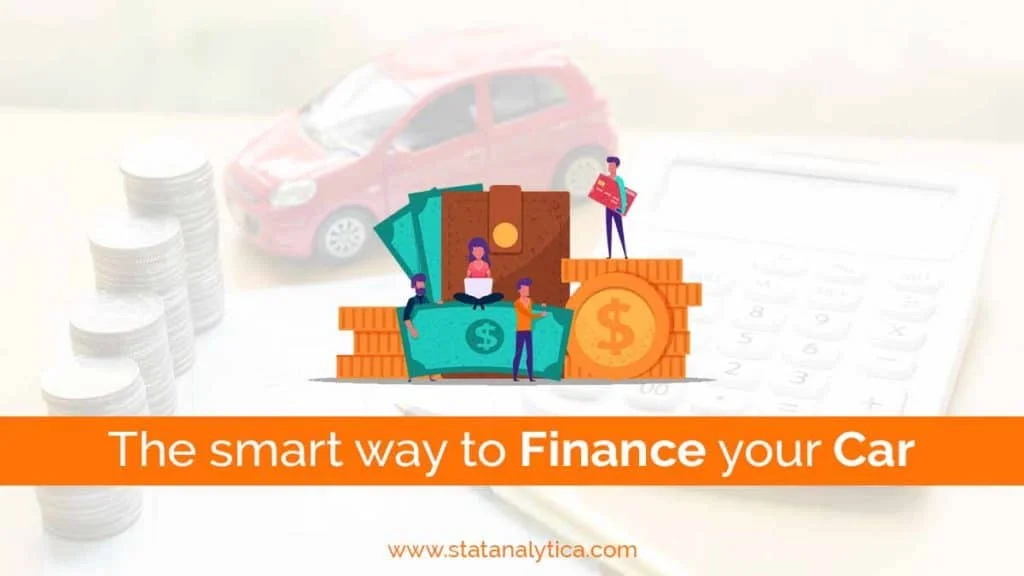
Step by Step Guide on The Best Way to Finance Car
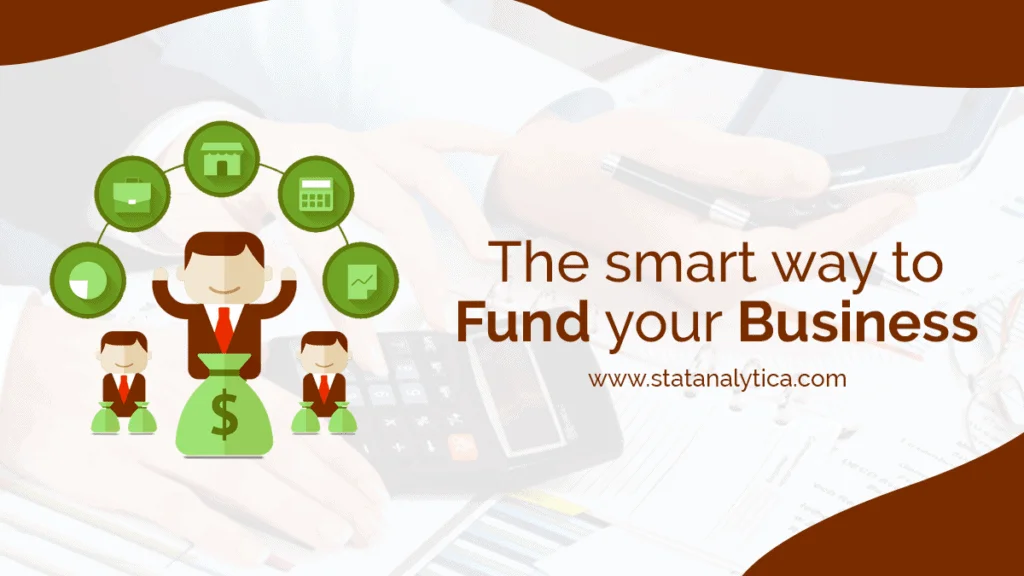
The Best Way on How to Get Fund For Business to Grow it Efficiently
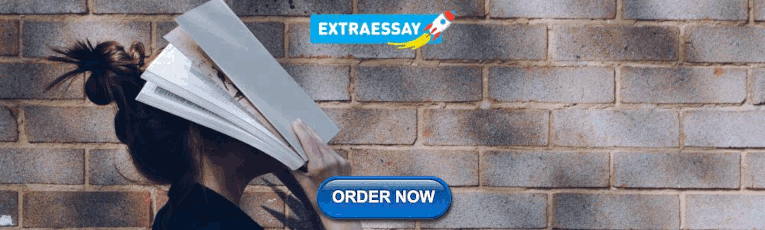
Artificial intelligence in E-Commerce: a bibliometric study and literature review
- Research Paper
- Published: 18 March 2022
- Volume 32 , pages 297–338, ( 2022 )
Cite this article
- Ransome Epie Bawack 1 ,
- Samuel Fosso Wamba 2 ,
- Kevin Daniel André Carillo 2 &
- Shahriar Akter 3
62k Accesses
69 Citations
9 Altmetric
Explore all metrics
This paper synthesises research on artificial intelligence (AI) in e-commerce and proposes guidelines on how information systems (IS) research could contribute to this research stream. To this end, the innovative approach of combining bibliometric analysis with an extensive literature review was used. Bibliometric data from 4335 documents were analysed, and 229 articles published in leading IS journals were reviewed. The bibliometric analysis revealed that research on AI in e-commerce focuses primarily on recommender systems. Sentiment analysis, trust, personalisation, and optimisation were identified as the core research themes. It also places China-based institutions as leaders in this researcher area. Also, most research papers on AI in e-commerce were published in computer science, AI, business, and management outlets. The literature review reveals the main research topics, styles and themes that have been of interest to IS scholars. Proposals for future research are made based on these findings. This paper presents the first study that attempts to synthesise research on AI in e-commerce. For researchers, it contributes ideas to the way forward in this research area. To practitioners, it provides an organised source of information on how AI can support their e-commerce endeavours.
Similar content being viewed by others
Artificial Intelligence in E-commerce: A Literature Review
Research on the Use, Characteristics, and Impact of e-Commerce Product Recommendation Agents: A Review and Update for 2007–2012
E-commerce and Business Analytics: A Literature Review
Avoid common mistakes on your manuscript.
Introduction
Electronic commerce (e-commerce) can be defined as activities or services related to buying and selling products or services over the internet (Holsapple & Singh, 2000 ; Kalakota & Whinston, 1997 ). Firms increasingly indulge in e-commerce because of customers' rising demand for online services and its ability to create a competitive advantage (Gielens & Steenkamp, 2019 ; Hamad et al., 2018 ; Tan et al., 2019 ). However, firms struggle with this e-business practice due to its integration with rapidly evolving, easily adopted, and highly affordable information technology (IT). This forces firms to constantly adapt their business models to changing customer needs (Gielens & Steenkamp, 2019 ; Klaus & Changchit, 2019 ; Tan et al., 2007 ). Artificial intelligence (AI) is the latest of such technologies. It is transforming e-commerce through its ability to “correctly interpret external data, to learn from such data, and to use those learnings to achieve specific goals and tasks through flexible adaptation” (Kaplan & Haenlein, 2019 . p. 15). Depending on the context, AI could be a system, a tool, a technique, or an algorithm (Akter et al., 2021 ; Bawack et al., 2021 ; Benbya et al., 2021 ). It creates opportunities for firms to gain a competitive advantage by using big data to uniquely meet their customers' needs through personalised services (Deng et al., 2019 ; Kumar, Rajan, et al., 2019 ; Kumar, Venugopal, et al., 2019 ).
AI in e-commerce can be defined as using AI techniques, systems, tools, or algorithms to support activities related to buying and selling products or services over the internet. Research on AI in e-commerce has been going on for the past three decades. About 4000 academic research articles have been published on the topic across multiple disciplines, both at the consumer (de Bellis & Venkataramani Johar, 2020 ; Sohn & Kwon, 2020 ) and organisational levels (Campbell et al., 2020 ; Kietzmann et al., 2018 ; Vanneschi et al., 2018 ). However, knowledge on the topic has not been synthesised despite its rapid growth and dispersion. This lack of synthesis makes it difficult for researchers to determine how much the extant literature covers concepts of interest or addresses relevant research gaps. Synthesising research on AI in e-commerce is an essential condition for advancing knowledge by providing the background needed to describe, understand, or explain phenomena, to develop/test new theories, and to develop teaching orientations in this research area (Cram et al., 2020 ; Paré et al., 2015 ). Thus, this study aims to synthesise research on AI in e-commerce and propose directions for future research in the IS discipline. The innovative approach of combining bibliometric analysis with an extensive literature review is used to answer two specific research questions: (i) what is the current state of research on AI in e-commerce? (ii) what research should be done next on AI in e-commence in general, and within information systems (IS) research in particular?
This study's findings show that AI in e-commerce primarily focuses on recommender systems and the main research themes are sentiment analysis, optimisation, trust, and personalisation. This study makes timely contributions to ongoing debates on the connections between business strategy and the use of AI technologies (Borges et al., 2020 ; Dwivedi et al., 2019 , 2020 ). It also contributes to research on how firms can address challenges regarding the use of AI-related benefits and opportunities for new product or service developments and productivity improvements (Makridakis, 2017 ). Furthermore, no study currently synthesises AI in e-commerce research despite its rapid evolution in the last decade triggered by big data, advanced machine learning (ML) algorithms, and cloud computing. Using well-established e-commerce classification frameworks (Ngai & Wat, 2002 ; Wareham et al., 2005 ), this study classifies information systems (IS) literature on AI in e-commerce. These classifications make it easier for researchers and managers to identify relevant literature based on the topic area, research style, and research theme. A future research agenda is proposed based on the gaps revealed during the classification to guide researchers on making meaningful contributions to AI knowledge in e-commerce.
Research method
Bibliometric analysis.
Bibliometric analysis has been increasingly used in academic research in general and in IS research to evaluate the quality, impact, and influence of authors, journals, and institutions in a specific research area (Hassan & Loebbecke, 2017 ; Lowry et al., 2004 , 2013 ). It has also been used extensively to understand AI research on specific fields or topics (Hinojo-Lucena et al., 2019 ; Tran et al., 2019 ; Zhao, Dai, et al., 2020 ; Zhao, Lou, et al., 2020 ). In this study, a bibliometric analysis was conducted to understand research on AI in e-commerce using the approach Aria and Cuccurullo ( 2017 ) proposed. This methodology involves three main phases: data collection, data analysis, and data visualisation & reporting. The data collection phase involves querying, selecting, and exporting data from selected databases. This study's data sample was obtained by querying the Web of Science (WoS) core databases for publications from 1975 to 2020. This database was chosen over others like Google Scholar or Scopus because WoS provides better quality bibliometric information due to its lower rate of duplicate records (Aria et al., 2020 ) and greater coverage of high-impact journals (Aghaei Chadegani et al., 2013 ). The following search string was used to query the title, keywords, and abstracts of all documents in the WoS collection:
(‘‘Electronic Commerce’’ OR ‘‘Electronic business’’ OR ‘‘Internet Commerce’’ OR “e-business” OR “ebusiness” OR "e-commerce” OR “ecommerce” OR “online shopping” OR “online purchase” OR “internet shopping” OR “e-purchase” OR “online store” OR “electronic shopping”). AND (“Artificial intelligence” OR “Artificial neural network” OR “case-based reasoning” OR “cognitive computing” OR “cognitive science” OR “computer vision” OR “data mining” OR “data science” OR “deep learning” OR “expert system” OR “fuzzy linguistic modelling” OR “fuzzy logic” OR “genetic algorithm” OR “image recognition” OR “k-means” OR “knowledge-based system” OR “logic programming” OR “machine learning” OR “machine vision” OR “natural language processing” OR “neural network” OR “pattern recognition” OR “recommendation system” OR “recommender system” OR “semantic network” OR “speech recognition” OR “support vector machine” OR “SVM” OR “text mining”).
This search string led to 4414 documents that made up the initial dataset of this study. For quality reasons, only document types tagged as articles, reviews, and proceeding papers were selected for this study because they are most likely to have undergone a rigorous peer-review process before publication (Milian et al., 2019 ). Thus, editorial material, letters, news items, meeting abstracts, and retracted publications were removed from the dataset, leaving 4335 documents that made up the final dataset used for bibliometric analysis. Figure 1 summarises the data collection phase.
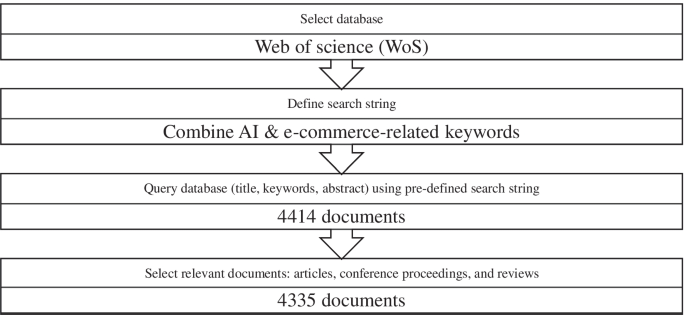
Summary of the data collection phase
Table 1 summarises the main information about the dataset regarding the timespan, document sources, document types, document contents, authors, and author collaborations. The dataset consists of documents from 2599 sources, published by 8663 authors and 84,474 references.
Bibliometrix Footnote 1 is the R package used to conduct bibliometric analysis (Aria & Cuccurullo, 2017 ). This package has been extensively used to conduct bibliometric studies published in top-tier journals. It incorporates the most renowned bibliometric tools for citation analysis (Esfahani et al., 2019 ; Fosso Wamba, 2020 ; Pourkhani et al., 2019 ). It was specifically used to analyse the sources, documents, conceptual, and intellectual structure of AI in e-commerce research. Publication sources and their source impacts were analysed based on their h-index quality factors (Hirsch, 2010 ). The most significant, impactful, prestigious, influential, and quality publication sources, affiliations, and countries regarding research on AI in e-commerce were identified. This contributed to the identification of the most relevant disciplines in this area of research. Documents were analysed using total citations to identify the most cited documents in the dataset. Through content analysis, the most relevant topics/concepts, AI technologies/techniques, research methods, and application domains were identified.
Furthermore, citation analysis and reference publication year spectroscopy (RPYS) were used to identify research contributions that form the foundations of research on AI in e-commerce (Marx et al., 2014 ; Rhaiem & Bornmann, 2018 ). These techniques were also used to identify the most significant changes in the research area. Co-word network analysis on author-provided keywords using the Louvain clustering algorithm was used to understand the research area's conceptual structure. This algorithm is a greedy optimisation method used to identify communities in large networks by comparing the density of links inside communities with links between communities (Blondel et al., 2008 ). This study used it to identify key research themes by analysing author-provided keywords. Co-citation network analysis using the Louvain clustering algorithm was also used to analyse publication sources through which journals communities were identified. It further contributed to identifying the most relevant disciplines in this research area by revealing journal clusters.
The bibliometric analysis results were reported from functionalist, normative, and interpretive perspectives (Hassan & Loebbecke, 2017 ). The functionalist perspective presents the results of the key concepts and topics investigated in this research area. The normative perspective focuses on the foundations and norms of the research area. The interpretive perspective emphasises the main themes that drive AI in e-commerce research.
- Literature review
An extensive review and classification of IS literature on AI in e-commerce complemented the bibliometric analysis. It provides more details on how research in this area is conducted in the IS discipline. The review was delimited to the most impactful and influential management information systems (MIS) journals identified during the bibliometric analysis and completed by other well-established MIS journals known for their contributions to e-commerce research (Ngai & Wat, 2002 ; Wareham et al., 2005 ). Thus, 20 journals were selected for this review: Decision sciences, Decision support systems, Electronic commerce research and applications, Electronic markets, E-service journal, European journal of information systems, Information and management, Information sciences, Information systems research, International journal of electronic commerce, International journal of information management, Journal of information systems, Journal of information technology, Journal of management information systems, Journal of organisational computing and electronic commerce, Journal of strategic information systems, Journal of the association for information systems, Knowledge-based systems, Management science, MIS Quarterly .
The literature review was conducted in three stages (Templier & Paré, 2015 ; Webster & Watson, 2002 ): (i) identify and analyse all relevant articles from the targeted journals found in the bibliometric dataset (ii) use the keyword string to search for other relevant articles found on the official publication platforms of the targeted journals, and (iii) identify relevant articles from the references of the articles identified in stages one and two found within the target journals. All articles with content that did not focus on AI in e-commerce were eliminated. This process led to a final dataset of 229 research articles on AI in e-commerce. The articles were classified into three main categories: by topic area (Ngai & Wat, 2002 ), by research style (Wareham et al., 2005 ), and by research themes (from bibliometric analysis).
Classification by topic area involved classifying relevant literature into four broad categories: (i) applications, (ii) technological issues, (iii) support and implementation, and (iv) others. Applications refer to the specific domain in which the research was conducted (marketing, advertising, retailing…). Technological issues contain e-commerce research by AI technologies, systems, algorithms, or methodologies that support or enhance e-commerce applications. Support and Implementation include articles that discuss how AI supports public policy and corporate strategy. Others contain all other studies that do not fall into any of the above categories. It includes articles on foundational concepts, adoption, and usage. Classification by research style involved organizing the relevant literature by type of AI studied, the research approach, and the research method used in the studies. The research themes identified in the bibliometric analysis stage were used to classify the relevant IS literature by research theme.
Results of the bibliometric analysis
Scientific publications on AI in e-commerce began in 1991 with an annual publication growth rate of 10.45%. Figure 2 presents the number of publications per year. Observe the steady increase in the number of publications since 2013.
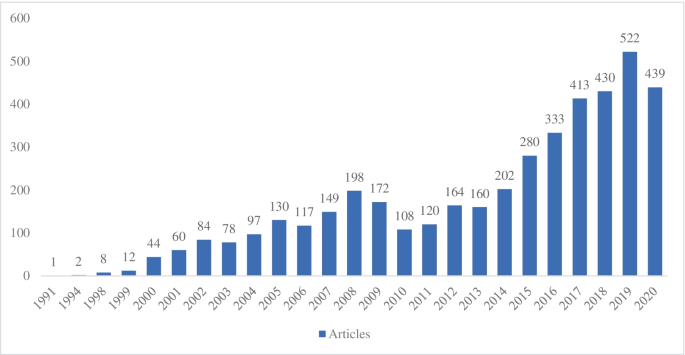
Number of publications on AI in e-commerce per year
Institutions in Asia, especially China, are leading this research area. The leading institution is Beijing University of Posts and Telecommunications, with 88 articles, followed by Hong Kong Polytechnic University with 84 articles. Table 2 presents the top 20 institutions publishing on AI in e-commerce.
As expected, China-based affiliations appear most frequently in publications (4261 times). They have over 2.5 times as many appearances as US-based affiliations (1481 times). Interestingly, publications with US-based affiliations attract more citations than those in China. Table 3 presents the number of times authors from a given country feature in publications and the corresponding total number of citations.
Functional perspective
Analysing the most globally cited documents Footnote 2 in the dataset (those with 100 citations) reveals that recommender systems are the main topic of interest in this research area (Appendix Table 10 ). Recommender systems are software agents that make recommendations for consumers by implicitly or explicitly evoking their interests or preferences (Bo et al., 2007 ). The topic has been investigated in many flavours, including hybrid recommender systems (Burke, 2002 ), personalised recommender systems (Cho et al., 2002 ), collaborative recommender systems (Lin et al., 2002 ) and social recommender systems (Li et al., 2013 ). The central concept of interest is personalisation, specifically leveraging recommender systems to offer more personalised product/service recommendations to customers using e-commerce platforms. Thus, designing recommender systems that surpass existing ones is the leading orientation of AI in e-commerce research. Researchers have mostly adopted experimental rather than theory-driven research designs to meet this overarching research objective. Research efforts focus more on improving the performance of recommendations using advanced AI algorithms than on understanding and modelling the interests and preferences of individual consumers. Nevertheless, the advanced AI algorithms developed are trained primarily using customer product reviews.
Interpretive perspective
Four themes characterise research on AI in e-commerce: sentiment analysis, trust & personalisation, optimisation, AI concepts, and related technologies. The keyword clusters that led to the identification of these themes are presented in Table 4 . The sentiment analysis theme represents the stream of research focused on interpreting and classifying emotions and opinions within text data in e-commerce using AI techniques like ML and natural language processing (NLP). The trust and personalisation theme represents research that focuses on establishing trust and making personalised recommendations for consumers in e-commerce using AI techniques like collaborative filtering, case-based reasoning, and clustering algorithms. The optimisation theme represents research that focuses on using AI algorithms like genetic algorithms to solve optimisation problems in e-commerce. Finally, the AI concepts and related technologies theme represent research that focuses on using different techniques and concepts used in the research area.
Normative perspective
Research on AI in e-commerce is published in two main journal subject areas: computer science & AI and business & management. This result confirms the multidisciplinary nature of this research area, which has both business and technical orientations. Table 5 presents the most active publication outlets in each subject area. The outlets listed in the table could help researchers from different disciplines to select the proper outlet for their research results. It could also help researchers identify the outlets wherein they are most likely to find relevant information for their research on AI in e-commerce.
However, some disciplines set the foundations and standards of research on AI in e-commerce through the impact of their contributions to its body of knowledge. Analysing document references shows that the most cited contributions come from journals in the IS, computer science, AI, management science, and operations research disciplines (Table 6 ). It shows the importance of these disciplines to AI's foundations and standards in e-commerce research and their major publication outlets.
The IS discipline is a significant contributor to AI in e-commerce research, given that 24 out of the 40 top publications in the area can be assimilated to IS sources. Table 7 also shows that 7 out of the top 10 most impactful publication sources are assimilated to the IS discipline. The leading paper from the IS field reviews approaches to automatic schema matching (Rahm & Bernstein, 2001 ) and it is the second most globally cited paper in the research area. Meanwhile, the leading paper from the MIS subfield reviews recommender system application developments (Lu et al., 2015 ).
Collaborative filtering, recommender systems, social information filtering, latent Dirichlet allocation, and matrix factoring techniques are the foundational topics in research on AI in e-commerce (Table 8 ). They were identified by analysing the most cited references in the dataset. These references were mostly literature reviews and documents that discussed the basic ideas and concepts behind specific technologies or techniques used in recommender systems.
Furthermore, the specific documents that set the foundations of research on AI in e-commerce and present the most significant historical contributions and turning points in the field were identified using RPYS (Appendix Table 11 ). 2001, 2005, 2007, 2011, and 2015 are the years with the highest number of documents referenced by the documents in the sample. The most cited studies published in 2001 focused on recommendation algorithms, especially item-based collaborative filtering, random forest, gradient boosting machine, and data mining. The main concept of interest was how to personalise product recommendations. In 2005, the most referenced documents focused on enhancing recommendation systems using hybrid collaborative filtering, advanced machine learning tools and techniques, and topic diversification. That year also contributed a solid foundation for research on trust in recommender systems. In 2007, significant contributions continued on enhanced collaborative filtering techniques for recommender systems. Meanwhile, Bo & Benbasat ( 2007 ) set the basis for research on recommender systems' characteristics, use, and impact, shifting from traditional studies focused on underlying algorithms towards a more consumer-centric approach. In 2011, major contributions were made to enhance recommender systems, like developing a new library for support vector machines (Chang & Lin, 2011 ) and the Scikit-learn package for machine learning in Python (Pedregosa et al., 2011 ). In 2015, the most critical contributions primarily focused on deep learning algorithms, especially with an essential contribution to using them in recommender systems (Wang et al., 2015 ).
Results of the literature review study
Classification by topic area.
Most articles on AI in e-commerce focus on technological issues (107 articles, 47%), followed by applications (87 articles, 38%), support and implementation (20 articles, 9%), then others (15 articles, 6%). Specifically, most articles focus on AI algorithms, models, and methodologies that support or improve e-commerce applications (76 articles, 33.2%) or emphasise the applications of AI in marketing, advertising, and sales-related issues (38 articles, 16.6%). Figure 3 presents the distribution of articles, while Appendix Table 12 presents the articles in each topic area.
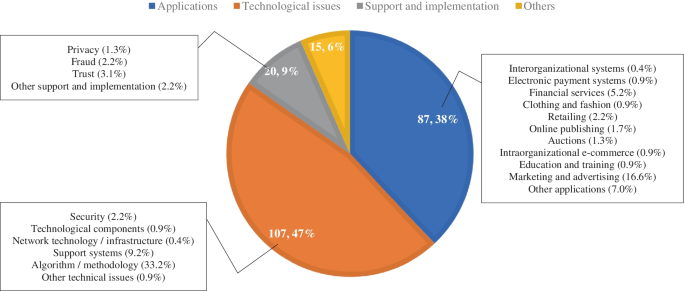
Classification of MIS literature on AI in e-commerce by topic area
Classification by research style
Most authors discuss AI algorithms, models, computational approaches, or methodologies (168 articles, 73%). Specifically, current research focuses on how AI algorithms like ML, deep learning (DL), NLP, and related techniques could be used to model and understand phenomena in the e-commerce environment. It also focuses on studies that involve designing intelligent agent algorithms that support learning processes in e-commerce systems. Many studies also focus on AI as systems (31 articles, 14%), especially on recommender systems and expert systems that leverage AI algorithms in the back end. The “others” category harboured all articles that did not clearly refer to AI as either an algorithm or as a system (30 articles, 13%) (see Fig. 4 and Appendix Table 13 ).
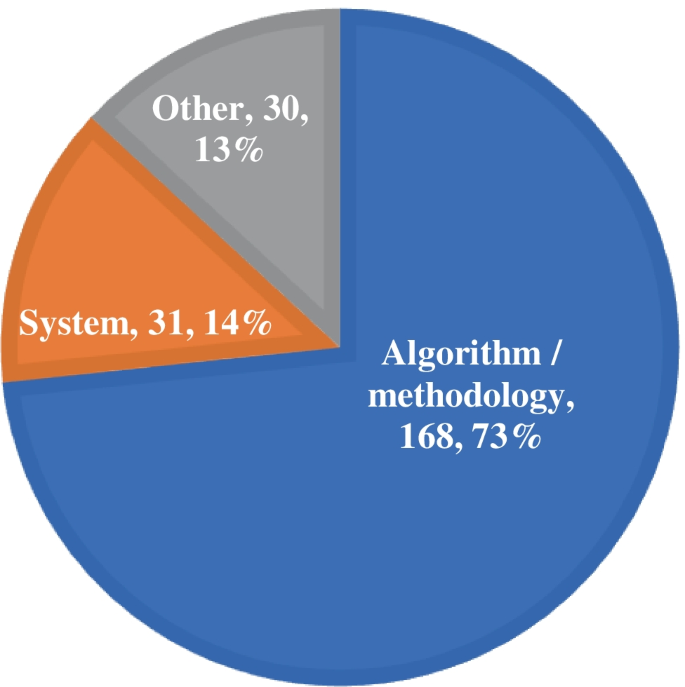
Classification of MIS literature on AI in e-commerce by type of AI
Furthermore, most publications use the design science research approach (198 articles, 86%). Researchers prefer this approach because it allows them to develop their algorithms and models or improve existing ones, thereby creating a new IS artefact (see Fig. 5 and Appendix Table 14 ).
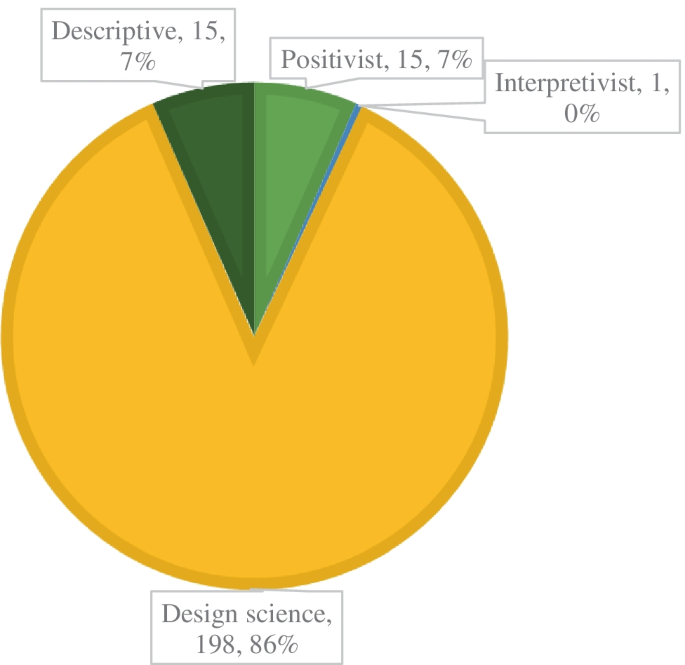
Classification of MIS literature on AI in e-commerce by research approach
Also, authors adopt experimental methods in their papers (157 articles, 69%), especially those who adopted a design science research approach. They mostly use experiments to test their algorithms or prove their concepts (see Fig. 6 and Appendix Table 15 ).
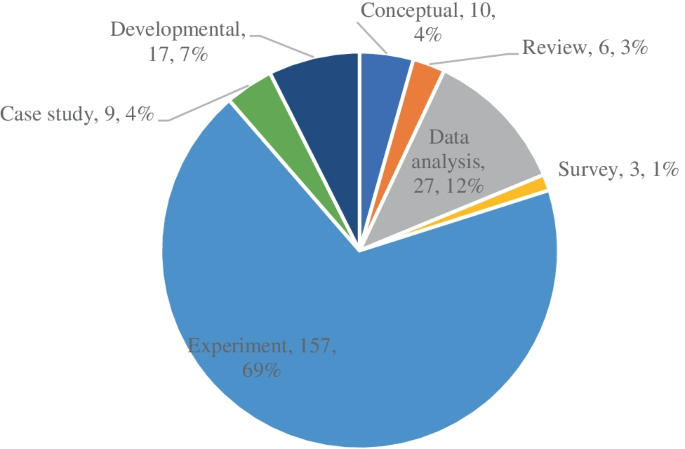
Classification of MIS literature on AI in e-commerce by research method
Classification by research theme
Based on the main research themes on AI in e-commerce identified during the bibliometric analysis, most authors published on optimisation (63 articles, 27%). They mostly focused on optimising recommender accuracy (25 articles), prediction accuracy (29 articles), and other optimisation aspects (9 articles) like storage optimisation. This trend was followed by publications on trust & personalisation (31 articles, 14%), wherein more articles were published on personalisation (17 articles) than on trust (14 articles). Twenty-nine articles focused on sentiment analysis (13%). The rest of the papers focus on AI design, tools and techniques (46 articles), decision support (30 articles), customer behaviour (13 articles), AI concepts (9 articles), and intelligent agents (8 articles) (see Fig. 7 and Appendix Table 16 ).
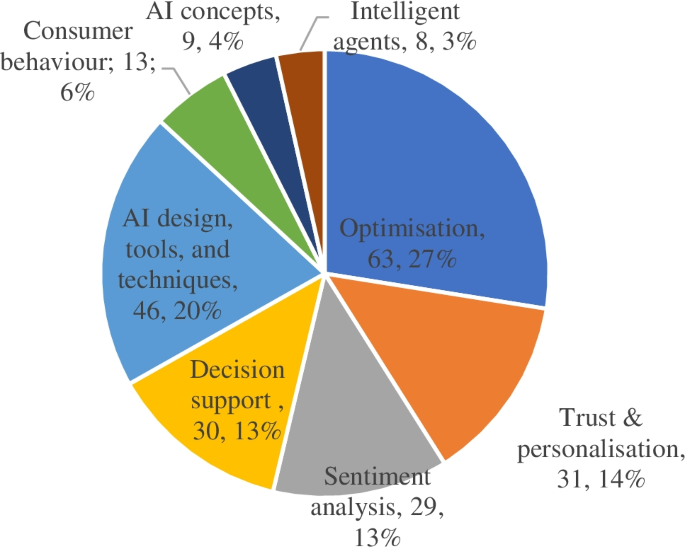
Classification of MIS literature on AI in e-commerce by current research themes
This study's overall objective was to synthesise research on AI in e-commerce and propose avenues for future research. Thus, it sought to answer two research questions: (i) what is the current state of research on AI in e-commerce? (ii) what research should be done next on AI in e-commerce in general and within IS research in particular? This section summarises the findings of the bibliometric analysis and literature review. It highlights some key insights from the results, starting with the leading role of China and the USA in this research area. This highlight is followed by discussions on the focus of current research on recommender systems, the extensive use of design science and experiments in this research area, and a limited focus on modelling consumer behaviour. This section also discusses the little research found on some research themes and the limited number of publications from some research areas. Implications for research and practice are discussed at the end of this section.
Need for more research from other countries
Research on AI in e-commerce has been rising steadily since 2013. Overall, these results indicate a growing interest in the applications of AI in e-commerce. China-based institutions lead this research area, although US-based affiliations attract more citations. Tables 2 and 3 indicate that China is in the leading position regarding research on AI in e-commerce. Observe that Amazon Inc. (USA), JD.com (China), Alibaba Group Holding Ltd. (China), Suning.com (China), Meituan (China), Wayfair (USA), eBay (USA), and Groupon (USA) are referenced among the largest e-commerce companies in the world (in terms of market capitalisation, revenue, and the number of employees). Footnote 3 These companies are primarily from China and the USA. These findings correlate with Table 3 , which could indicate that China and the USA are investing more in the research and development of AI applications in e-commerce (especially China, based on Table 2 ) because of the positions they occupy in the industry. This logic would imply that companies seeking to penetrate the e-commerce industry and remain competitive should also consider investing more in the research and development of AI applications in the area. The list of universities provided could become partner universities for countries with institutions that have less experience in the research area. Especially with the COVID-19 pandemic, e-commerce has become a global practice. Thus, other countries need to contribute more research on the realities of e-commerce in their respective contexts to develop more globally acceptable AI solutions in e-commerce practices. It is essential because different countries approach e-commerce differently. For example, although Amazon’s marketplace is well-developed in continents like Europe, Asia, and North America, it has difficulty penetrating Africa because the context is very different (culturally and infrastructurally). While mobile wallet payment systems are fully developed on the African continent, Amazon’s marketplace does not accommodate this payment method. Therefore, it would be impossible for many Africans to use Amazon’s Alexa to purchase products online. What does this mean for research on digital inclusion? Are there any other cross-cultural differences between countries that affect the adoption and use of AI in e-commerce? Are there any legal boundaries that affect the implementation and internationalisation of AI in e-commerce? Such questions highlight the need for more country-specific research on AI in e-commerce to ensure more inclusion.
Focus on recommender systems
AI in e-commerce research is essentially focused on recommender systems in the past years. The results indicate that in the last 20 years, AI in e-commerce research has primarily focused on using AI algorithms to enhance recommender systems. This trend is understandable because recommender systems have become an integral part of almost every e-commerce platform nowadays (Dokyun Lee & Hosanagar, 2021 ; Stöckli & Khobzi, 2021 ). As years go by, observe how novel AI algorithms have been proposed, the most recent being deep learning (Chaudhuri et al., 2021 ; Liu et al., 2020 ; Xiong et al., 2021 ; Zhang et al., 2021 ). Thus, researchers are increasingly interested in how advanced AI algorithms can enable recommender systems in e-commerce platforms to correctly interpret external data, learn from such data, and use those learnings to improve the quality of user recommendations through flexible adaptation. With the advent of AI-powered chatbots and voice assistants, firms increasingly include these technologies in their e-commerce platforms (Ngai et al., 2021 ). Thus, researchers are increasingly interested in conversational recommender systems (De Carolis et al., 2017 ; Jannach et al., 2021 ; Viswanathan et al., 2020 ). These systems can play the role of recommender systems and interact with the user through natural language (Iovine et al., 2020 ). Thus, conversational recommender systems is an up-and-coming research area for AI-powered recommender systems, especially given the ubiquitous presence of voice assistants in society today. Therefore, researchers may want to investigate how conversational recommender systems can be designed effectively and the factors that influence their adoption.
Limited research themes
The main research themes in AI in e-commerce are sentiment analysis, trust, personalisation, and optimisation. Researchers have focused on these themes to provide more personalised recommendations to recommendation system users. Personalising recommendations based on users’ sentiment and trust circle has been significantly researched. Extensive research has also been conducted on how to optimise the algorithmic performance of recommender systems. ML, DL, NLP are the leading AI algorithms and techniques currently researched in this area. The foundational topics for applying these algorithms include collaborative filtering, latent Dirichlet allocation, matrix factoring techniques, and social information filtering.
Current research shows how using AI for personalisation would enable firms to deliver high-quality customer experiences through precise personalisation based on real-time information (Huang & Rust, 2018 , 2020 ). It is highly effective in data-rich environments and can help firms to significantly improve customer satisfaction, acquisition, and retention rates, thereby ideal for service personalisation (Huang & Rust, 2018 ). AI could enable firms to personalise products based on preferences, personalise prices based on willingness to pay, personalise frontline interactions, and personalise promotional content in real-time (Huang & Rust, 2021 ).
Research also shows how AI could help firms optimise product prices by channel and customer (Huang & Rust, 2021 ; Huang & Rust, 2020 ) and develop accurate and personalised recommendations for customers. It is beneficial when the firm lacks initial data on customers that it can use to make recommendations (cold start problem) (Guan et al., 2019 ; Wang, Feng, et al., 2018 ; Wang, Jhou, et al., 2018 ; Wang, Li, et al., 2018 ; Wang, Lu, et al., 2018 ). It also gives firms the ability to automatically estimate optimal prices for their products/services and define dynamic pricing strategies that increase profits and revenue (Bauer & Jannach, 2018 ; Greenstein-Messica & Rokach, 2018 ). It also gives firms the ability to predict consumer behaviours like customer churn (Bose & Chen, 2009 ), preferences based on their personalities (Buettner, 2017 ), engagement (Ayvaz et al., 2021 ; Sung et al., 2021 ; Yim et al., 2021 ), and customer payment default (Vanneschi et al., 2018 ). AI also gives firms the ability to predict product, service, or feature demand and sales (Cardoso & Gomide, 2007 ; Castillo et al., 2017 ; Ryoba et al., 2021 ), thereby giving firms the ability to anticipate and dynamically adjust their advertising and sales strategies (Chen et al., 2014 ; Greenstein-Messica & Rokach, 2020 ). Even further, it gives firms the ability to predict the success or failure of these strategies (Chen & Chung, 2015 ).
Researchers have shown that using AI to build trust-based recommender systems can help e-commerce firms increase user acceptance of the recommendations made by e-commerce platforms (Bedi & Vashisth, 2014 ). This trust is created by accurately measuring the level of trust customers have in the recommendations made by the firm’s e-commerce platforms (Fang et al., 2018 ) or by making recommendations based on the recommendations of people the customers’ trust in their social sphere (Guo et al., 2014 ; Zhang et al., 2017 ).
Sentiment analytics using AI could give e-commerce firms the ability to provide accurate and personalised recommendations to customers by assessing their opinions expressed online such as through customer reviews (Al-Natour & Turetken, 2020 ; Qiu et al., 2018 ). It has also proven effective in helping brands better understand their customers over time and predict their behaviours (Das & Chen, 2007 ; Ghiassi et al., 2016 ; Pengnate & Riggins, 2020 ). For example, it helps firms better understand customer requirements for product improvements (Ou et al., 2018 ; Qi et al., 2016 ) and predict product sales based on customer sentiments (Li, Wang, et al., 2019 ; Li, Wu, et al., 2019 ; Li, Zhang, et al., 2019 ). Thus, firms can accurately guide their customers towards discovering desirable products (Liang & Wang, 2019 ) and predict the prices they would be willing to pay for products based on their sentiments (Tseng et al., 2018 ). Thus, firms that use AI-powered sentiment analytics would have the ability to constantly adapt their product development, sales, and pricing strategies while improving the quality of their e-commerce services and personalised recommendations for their customers.
While the current research themes are exciting and remain relevant in today’s context, it highlights the need for researchers to explore other research themes. For example, privacy, explainable, and ethical AI are trendy research themes in AI research nowadays. These themes are relevant to research on AI in e-commerce as well. Thus, developing these research themes would make significant contributions to research on AI in e-commerce. In the IS discipline, marketing & advertising is where AI applications in e-commerce have been researched the most. This finding complements Davenport et al. ( 2020 )’s argument, suggesting that marketing functions have the most to gain from AI. Most publications focus on technological issues like algorithms, support systems, and security. Very few studies investigated privacy, and none was found on topics like ethical, explainable, or sustainable AI. Therefore, future research should pay more attention to other relevant application domains like education & training, auctions, electronic payment systems, inter-organisational e-commerce, travel, hospitality, and leisure (Blöcher & Alt, 2021 ; Manthiou et al., 2021 ; Neuhofer et al., 2021 ). To this end, questions that may interest researchers include, what are the privacy challenges caused by using AI in e-commerce? How can AI improve e-commerce services in education and training? How can AI improve e-commerce services in healthcare? How can AI bring about sustainable e-commerce practices?
Furthermore, research on AI in e-commerce is published in two main journal categories: computer science & AI and business & management. Most citations come from the information systems, computer science, artificial intelligence, management science, and operations research disciplines. Thus, researchers interested in research on AI in e-commerce are most likely to find relevant information in such journals (see Tables 5 and 6 ). Researchers seeking to publish their research on AI in e-commerce can also target such journals. However, researchers are encouraged to publish their work in other equally important journal categories. For example, law and government-oriented journals would greatly benefit from research on AI in e-commerce. International laws and government policies could affect how AI is used in e-commerce. For example, due to the General Data Protection Regulation (GDPR), how firms use AI algorithms and applications to analyse user data in Europe may differ from how they would in the US. Such factors may have profound performance implications given that AI systems are as good as the volume and quality of data they can access for analysis. Thus, future research in categories other than those currently researched would benefit the research community.
More experiment than theory-driven research
Most of the research done on AI in e-commerce have adopted experimental approaches. Very few adopted theory-driven designs. This trend is also observed in IS research, where 69% of the studies used experimental research methods and 86% adopted a design science research approach instead of the positivist research approach often adopted in general e-commerce research (Wareham et al., 2005 ). However, this study's findings complement a recent review that shows that laboratory experiments and secondary data analysis were becoming increasingly popular in e-commerce research. Given that recommender systems support customer decision-making, this study also complements recent studies that show the rising use of design science research methods in decision support systems research (Arnott & Pervan, 2014 ) and in IS research in general (Jeyaraj & Zadeh, 2020 ). This finding could be explained by the fact that researchers primarily focused on enhancing the performance of AI algorithms used in recommender systems. Therefore, to test the performance of their algorithms in the real world, the researchers have to build a prototype and test it in real-life contexts. Using performance accuracy scores, the researchers would then tell the extent to which their proposed algorithm is performant. However, ML has been highlighted as a powerful tool that can help advance theory in behavioural IS research (Abdel-Karim et al., 2021 ). Therefore, key research questions on AI in e-commerce could be approached using ML as a tool for theory testing in behavioural studies. Researchers could consider going beyond using AI algorithms for optimising recommender systems to understand its users' behaviour. In Fig. 4 , observe that 73% of IS researcher papers reviewed approached AI as an algorithm or methodology to solve problems in e-commerce. Only 14% approached AI as a system. Researchers can adopt both approaches in the same study in the sense that they can leverage ML algorithms to understand human interactions with AI systems, not just for optimisation. This approach could provide users with insights by answering questions regarding the adoption and use of AI systems.
Furthermore, only 6% of the studies focus on consumer behaviour. Thus, most researchers on AI in e-commerce this far have focused more on algorithm performance than on modelling the behaviour of consumers who use AI systems. It is also clear that behavioural aspects of using recommender systems are often overlooked (Adomavicius et al., 2013 ). There is relatively limited research on the adoption, use, characteristics, and impact of AI algorithms or systems on its users. This issue was raised as a fundamental problem in this research area (Bo et al., 2007 ) and seems to remain the case today. However, understanding consumer behaviour could help improve the accuracy of AI algorithms. Thus, behavioural science researchers need to conduct more research on modelling consumer behaviours regarding consumers' acceptance, adoption, use, and post-adoption behaviours targeted by AI applications in e-commerce. As AI algorithms, systems, and use cases multiply in e-commerce, studies explaining their unique characteristics, adoption, use, and impact at different levels (individual, organisational, and societal) should also increase. It implies adopting a more theory-driven approach to research on AI in e-commerce. Therefore, behavioural science researchers should be looking into questions on the behavioural factors that affect the adoption of AI in e-commerce.
Implications for research
This study contributes to research by innovatively synthesising the literature on AI in e-commerce. Despite the recent evolution of AI and the steady rise of research on how it could affect e-commerce environments, no review has been conducted to understand this research area's state and evolution. Yet, a recent study shows that e-commerce and AI are currently key research topics and themes in the IS discipline (Jeyaraj & Zadeh, 2020 ). This paper has attempted to fill this research gap by providing researchers with a global view of AI research in e-commerce. It offers a multidimensional view of the knowledge structure and citation behaviour in this research area by presenting the study's findings from functional, normative, and interpretive perspectives. Specifically, it reveals the most relevant topics, concepts, and themes on AI in e-commerce from a multidisciplinary perspective.
This contribution could help researchers evaluate the value and contributions of their research topics in the research area with respect to other disciplines and choose the best publication outlets for their research projects. This study also reveals the importance of AI in designing recommender systems and shows the foundational literature on which this research area is built. Thus, researchers could use this study to design the content of AI or e-commerce courses in universities and higher education institutions. Its content provides future researchers and practitioners with the foundational knowledge required to build quality recommender systems. Researchers could also use this study to inform their fields on the relevance of their research topics and the specific gaps to fill therein. For example, this study reveals the extent to which the IS discipline has appropriated research on AI in e-commerce. It also shows contributions of the IS discipline to the current research themes, making it easier for IS researchers to identify research gaps as well as gaps between IS theory and practice.
Implications for practice
This study shows that AI in e-commerce primarily focuses on recommender systems. It highlights sentiment analysis, optimisation, trust, and personalisation as the core themes in the research area. Thus, managers could tap into these resources to improve the quality of their recommender systems. Specifically, it could help them understand how to develop optimised, personalised, trust-based and sentiment-based analytics supported by uniquely designed AI algorithms. This knowledge would make imitating or replicating the quality of recommendations rendered through e-commerce platforms practically impossible for competitors. Firms willing to use AI in e-commerce would need unique access and ownership of customer data, AI algorithms, and expertise in analytics (De Smedt et al., 2021 ; Kandula et al., 2021 ; Shi et al., 2020 ). The competition cannot imitate these resources because they are unique to the firm, especially if patented (Pantano & Pizzi, 2020 ). Also, this research paper classifies IS literature on AI in e-commerce by topic area, research style, and research theme. Thus, IS practitioners interested in implementing AI in e-commerce platforms would easily find the research papers that best meet their needs. It saves them the time to search for articles themselves, which may not always be relevant and reliable.
Limitations
This study has some limitations. It was challenging to select a category for each article in the sample dataset. Most of those articles could be rightfully placed in several categories of the classification frameworks. However, assigning articles to a single category in each framework simplifies the research area's conceptualisation and understanding (Wareham et al., 2005 ). Thus, categories were assigned to each article based on the most apparent orientation from the papers' titles, keywords, and abstracts. Another challenge was whether or not to include a research paper in the review. For example, although some studies on recommender systems featured in the keyword search results, the authors did not specify if the system's underlying algorithms were AI algorithms. Consequently, such articles were not classified to ensure that those included in this review certainly had an AI orientation. Despite our efforts, we humbly acknowledge that this study may have missed some publications, and others may have been published since this paper started the review process. Thus, in no way does this study claim to be exhaustive but rather extensive. Nonetheless, the findings from our rigorous literature review process strongly match the bibliometric analysis findings and those from similar studies we referenced. Therefore, we believe our contributions to IS research on AI in e-commerce remain relevant.
Future research
In addition to recommendations for future research discussed in the previous sections, the findings of this study are critically analysed through the lens of recent AI research published in leading IS journals. The aim is to identify other potential gaps for future research on AI in e-commerce that could interest the IS community.
One of the fundamental issues with AI research in IS today is understanding the AI concept (Ågerfalk, 2020 ). Our findings show that researchers have mostly considered algorithms and techniques like ML, DL, and NLP AI in their e-commerce research. Are these algorithms and techniques AI? Does the fact that an algorithm helps to analyse data and make predictions about e-commerce activities mean that the algorithm is AI? It is crucial for researchers to clearly explain what they mean by AI and differentiate between different types of AI used in their studies to avoid ambiguity. This explanation would help prevent confusion between AI and business intelligence & analytics in e-commerce. It would also help distinguish between AI as a social actor and AI as a technology with the computational capability to perform cognitive functions.
A second fundamental issue with AI research in IS is context (Ågerfalk, 2020 ). Using the same data, an AI system would/should be able to interpret the message communicated or sought by the user based on context. Context gives meaning to the data, making the AI system’s output relevant in the real world. Research on AI in e-commerce did not show much importance to context. Many authors used existing datasets to test their algorithms without connecting them to a social context. Thus, it is difficult to assess whether the performance of the proposed algorithms is relevant in every social context. Future research should consider using AI algorithms to analyse behavioural data alongside ‘hard’ data (facts) to identify patterns and draw conclusions in specific contexts. It implies answering the crucial question, what type of AI best suits which e-commerce context? Thus, researchers would need to collaborate with practitioners to better understand and delineate contexts (Ågerfalk, 2020 ) of investigation rather than make general claims on fraud detection or product prices, for example.
The IS community is also interested in understanding ethical choices and challenges organisations face when adopting AI systems and algorithms. What ethical decisions do e-commerce firms need to make when implementing AI solutions? What are the ethical challenges e-commerce firms face when implementing AI solutions? Following a sociotechnical approach, firms seeking to implement AI systems need to make ethical choices. These include transparent vs black-boxed algorithms, slow & careful vs expedited & timely designs, passive vs active implementation approach, obscure vs open system implementation, compliance vs risk-taking, and contextualised vs standardised use of AI systems (Marabelli et al., 2021 ). Thus, future research on AI in e-commerce should investigate how e-commerce firms address these ethical choices when implementing their AI solutions and the challenges they face in the process.
AI and the future of work is another primary source of controversy in the IS community (Huysman, 2020 ; Willcocks, 2020a , b ). Several researchers are investigating how AI is transforming the work configurations of organisations. Workplace technology platforms are increasingly observed to integrate office applications, social media features and AI-driven self-learning capabilities (Baptista et al., 2020 ; Grønsund & Aanestad, 2020 ; Lyytinen et al., 2020 ). Is this emergent digital/human work configuration also happening in e-commerce firms? How is this changing the future of work in the e-commerce industry?
IS researchers have increasingly called for research on how AI transforms decision making. For example, they are interested in understanding how AI could help augment mental processing, change managerial mindsets and actions, and affect the rationality of economic agents (Brynjolfsson et al., 2021 ). A recent study also makes several research propositions for IS researchers regarding conceptual and theoretical development, AI-human interaction, and AI implementation in the context of decision making (Duan et al., 2019 ). This study shows that decision-making is not a fundamental research theme as it accounts for only 13% of the research papers reviewed. Thus, future research on AI in e-commerce should contribute to developing this AI research theme in the e-commerce context. It involves proposing answers to questions like how AI affects managerial mindsets and actions in e-commerce? How is AI affecting the rationality of consumers who use e-commerce platforms?
This study shows that relatively few research papers on AI in e-commerce are theory-driven. Most adopted experimental research methods and design science research approaches wherein they use AI algorithms to explain phenomena. The IS community is increasingly interested in developing theories using AI algorithms (Abdel-Karim et al., 2021 ). Contrary to traditional theory development approaches, such theories developed based on AI algorithms like ML are called to be focused, context-specific, and as transparent as possible (Chiarini Tremblay et al., 2021 ). Thus, rather than altogether abandoning the algorithm-oriented approach used for AI in e-commerce research, researchers who master it should develop skills to use it as a basis for theorising.
Last but not least, more research is needed on the role of AI-powered voice-based AI in e-commerce. It is becoming common for consumers to use intelligent personal assistants like Google’s Google Assistant, Amazon’s Alexa, and Apple’s Siri for shopping activities since many retail organisations are making them an integral part of their e-commerce platforms (de Barcelos Silva et al., 2020 ). Given the rising adoption of smart speakers by consumers, research on voice commerce should become a priority for researchers on AI in e-commerce. Yet, this study shows that researchers are still mostly focused on web-based, social networking (social commerce), and mobile (m-commerce) platforms. Therefore, research on the factors that affect the adoption and use of voice assistants in e-commerce and the impact on consumers and e-commerce firms would make valuable contributions to e-commerce research. Table 9 summarises the main research directions recommended in this paper.
Conclusions
AI has emerged as a technology that can differentiate between two competing firms in e-commerce environments. This study presents the state of research of AI in e-commerce based on bibliometric analysis and a literature review of IS research. The bibliometric analysis highlights China and the USA as leaders in this research area. Recommender systems are the most investigated technology. The main research themes in this area of research are optimisation, trust & personalisation, sentiment analysis, and AI concepts & related technologies. Most research papers on AI in e-commerce are published in computer science, AI, business, and management outlets. Researchers in the IS discipline has focused on AI applications and technology-related issues like algorithm performance. Their focus has been more on AI algorithms and methodologies than AI systems. Also, most studies have adopted a design science research approach and experiment-style research methods. In addition to the core research themes of the area, IS researchers have also focused their research on AI design, tools and techniques, decision support, consumer behaviour, AI concepts, and intelligent agents. The paper discusses opportunities for future research revealed directly by analysing the results of this study. It also discusses future research directions based on current debates on AI research in the IS community. Thus, we hope that this paper will help inform future research on AI in e-commerce.
Download the bibliometrix R package and read more here: https://www.bibliometrix.org/index.html
Global citation refers to the total number of times the document has been cited in other documents in general and local citations refer to the total number of times a document has been cited by other documents in our dataset.
https://axiomq.com/blog/8-largest-e-commerce-companies-in-the-world/
Abbasi, A., Zhang, Z., Zimbra, D., Chen, H., & Nunamaker, J. F. (2010). Detecting fake websites: The contribution of statistical learning theory. MIS Quarterly, 34 (3), 435–461. https://doi.org/10.2307/25750686
Abdel-Karim, B. M., Pfeuffer, N., & Hinz, O. (2021). Machine learning in information systems - a bibliographic review and open research issues. Electronic Markets, 31 (3), 643–670. https://doi.org/10.1007/s12525-021-00459-2
Article Google Scholar
Adomavicius, G., Bockstedt, J. C., Curley, S. P., & Zhang, J. (2013). Do Recommender Systems Manipulate Consumer Preferences? A Study of Anchoring Effects. Information Systems Research, 24 (4), 956–975. https://doi.org/10.1057/isre.2013.0497
Adomavicius, G., Sankaranarayanan, R., Sen, S., & Tuzhilin, A. (2005). Incorporating contextual information in recommender systems using a multidimensional approach. ACM Transactions on Information Systems, 23 (1), 103–145. https://doi.org/10.1145/1055709.1055714
Adomavicius, G., & Tuzhilin, A. (2005). Toward the next generation of recommender systems: A survey of the state-of-the-art and possible extensions. IEEE Transactions on Knowledge and Data Engineering, 17 (6), 734–749. https://doi.org/10.1109/TKDE.2005.99
Ågerfalk, P. J. (2020). Artificial intelligence as digital agency. European Journal of Information Systems, 29 (1), 1–8. https://doi.org/10.1080/0960085X.2020.1721947
Aghaei Chadegani, A., Salehi, H., Md Yunus, M. M., Farhadi, H., Fooladi, M., Farhadi, M., & Ale Ebrahim, N. (2013). A comparison between two main academic literature collections: Web of science and scopus databases. Asian Social Science, 9 (5), 18–26. https://doi.org/10.5539/ass.v9n5p18
Agrawal, R., Imieliński, T., & Swami, A. (1993). Mining Association Rules Between Sets of Items in Large Databases. ACM SIGMOD Record, 22 (2), 207–216. https://doi.org/10.1145/170036.170072
Aguwa, C., Olya, M. H., & Monplaisir, L. (2017). Modeling of fuzzy-based voice of customer for business decision analytics. Knowledge-Based Systems, 125 , 136–145. https://doi.org/10.1016/j.knosys.2017.03.019
Aher, S. B., & Lobo, L. M. R. J. (2013). Combination of machine learning algorithms for recommendation of courses in E-Learning System based on historical data. Knowledge-Based Systems, 51 , 1–14. https://doi.org/10.1016/j.knosys.2013.04.015
Akter, S., & Wamba, S. F. (2016). Big data analytics in E-commerce: A systematic review and agenda for future research. Electronic Markets, 26 (2), 173–194. https://doi.org/10.1007/s12525-016-0219-0
Akter, S., Wamba, S. F., Mariani, M., & Hani, U. (2021). How to Build an AI Climate-Driven Service Analytics Capability for Innovation and Performance in Industrial Markets? Industrial Marketing Management, 97 , 258–273. https://doi.org/10.1016/j.indmarman.2021.07.014
Al-Natour, S., Benbasat, I., & Cenfetelli, R. (2011). The adoption of online shopping assistants: Perceived similarity as an antecedent to evaluative beliefs. Journal of the Association for Information Systems, 12 (5), 347–374. https://doi.org/10.17705/1jais.00267
Al-Natour, S., Benbasat, I., & Cenfetelli, R. T. (2006). The role of design characteristics in shaping perceptions of similarity: The case of online shopping assistants. Journal of the Association for Information Systems, 7 (12), 821–861.
Al-Natour, S., & Turetken, O. (2020). A comparative assessment of sentiment analysis and star ratings for consumer reviews. International Journal of Information Management, 54 , 102132. https://doi.org/10.1016/j.ijinfomgt.2020.102132
Al-Shamri, M. Y. H. (2016). User profiling approaches for demographic recommender systems. Knowledge-Based Systems, 100 , 175–187. https://doi.org/10.1016/j.knosys.2016.03.006
Alt, R., Ehmke, J. F., Haux, R., Henke, T., Mattfeld, D. C., Oberweis, A., Paech, B., & Winter, A. (2019). Towards customer-induced service orchestration - requirements for the next step of customer orientation. Electronic Markets, 29 (1), 79–91. https://doi.org/10.1007/s12525-019-00340-3
Aria, M., & Cuccurullo, C. (2017). bibliometrix: An R-tool for comprehensive science mapping analysis. Journal of Informetrics, 11 (4), 959–975. https://doi.org/10.1016/j.joi.2017.08.007
Aria, M., Misuraca, M., & Spano, M. (2020). Mapping the Evolution of Social Research and Data Science on 30 Years of Social Indicators Research. Social Indicators Research, 149 (3), 803–831. https://doi.org/10.1007/s11205-020-02281-3
Ariyaluran Habeeb, R. A., Nasaruddin, F., Gani, A., Targio Hashem, I. A., Ahmed, E., & Imran, M. (2019). Real-time big data processing for anomaly detection: A Survey. International Journal of Information Management, 45 , 289–307. https://doi.org/10.1016/j.ijinfomgt.2018.08.006
Arnott, D., & Pervan, G. (2014). A critical analysis of decision support systems research revisited: The rise of design science. Journal of Information Technology, 29 (4), 269–293. https://doi.org/10.1057/jit.2014.16
Ayvaz, D., Aydoğan, R., Akçura, M. T., & Şensoy, M. (2021). Campaign participation prediction with deep learning. Electronic Commerce Research and Applications, 48 , 101058. https://doi.org/10.1016/j.elerap.2021.101058
Azadjalal, M. M., Moradi, P., Abdollahpouri, A., & Jalili, M. (2017). A trust-aware recommendation method based on Pareto dominance and confidence concepts. Knowledge-Based Systems, 116 , 130–143. https://doi.org/10.1016/j.knosys.2016.10.025
Bag, S., Kumar, S. K., & Tiwari, M. K. (2019). An efficient recommendation generation using relevant Jaccard similarity. Information Sciences, 483 , 53–64. https://doi.org/10.1016/j.ins.2019.01.023
Bai, X., Marsden, J. R., Ross, W. T., & Wang, G. (2020). A note on the impact of daily deals on local retailers’ online reputation: Mediation effects of the consumer experience. Information Systems Research, 31 (4), 1132–1143. https://doi.org/10.1287/isre.2020.0935
Balabanović, M., & Shoham, Y. (1997). Content-Based, Collaborative Recommendation. Communications of the ACM, 40 (3), 66–72. https://doi.org/10.1145/245108.245124
Bandyopadhyay, S., Rees, J., & Barron, J. M. (2008). Reverse auctions with multiple reinforcement learning agents. Decision Sciences, 39 (1), 33–63. https://doi.org/10.1111/j.1540-5915.2008.00181.x
Baptista, J., Stein, M.-K., Klein, S., Watson-Manheim, M. B., & Lee, J. (2020). Digital work and organisational transformation: Emergent Digital/Human work configurations in modern organisations. The Journal of Strategic Information Systems, 29 (2), 101618. https://doi.org/10.1016/j.jsis.2020.101618
Barzegar Nozari, R., & Koohi, H. (2020). A novel group recommender system based on members’ influence and leader impact. Knowledge-Based Systems, 205 , 106296. https://doi.org/10.1016/j.knosys.2020.106296
Bassano, C., Gaeta, M., Piciocchi, P., & Spohrer, J. C. (2017). Learning the Models of Customer Behavior: From Television Advertising to Online Marketing. International Journal of Electronic Commerce, 21 (4), 572–604. https://doi.org/10.1080/10864415.2016.1355654
Bauer, J., & Jannach, D. (2018). Optimal pricing in e-commerce based on sparse and noisy data. Decision Support Systems, 106 , 53–63. https://doi.org/10.1016/j.dss.2017.12.002
Bawack, R. E., Wamba, S. F., & Carillo, K. (2021). A framework for understanding artificial intelligence research: insights from practice. Journal of Enterprise Information Management, 34 (2), 645–678. https://doi.org/10.1108/JEIM-07-2020-0284
Bedi, P., & Vashisth, P. (2014). Empowering recommender systems using trust and argumentation. Information Sciences, 279 , 569–586. https://doi.org/10.1016/j.ins.2014.04.012
Beladev, M., Rokach, L., & Shapira, B. (2016). Recommender systems for product bundling. Knowledge-Based Systems, 111 , 193–206. https://doi.org/10.1016/j.knosys.2016.08.013
Benbya, H., Pachidi, S., & Jarvenpaa, S. L. (2021). Special issue editorial: Artificial intelligence in organizations: Implications for information systems research. Journal of the Association for Information Systems, 22 (2), 281–303. https://doi.org/10.17705/1jais.00662
Blei, D. M., Ng, A. Y., & Jordan, M. T. (2002). Latent dirichlet allocation. Advances in Neural Information Processing Systems, 3 (Jan), 993–1022.
Google Scholar
Blöcher, K., & Alt, R. (2021). AI and robotics in the European restaurant sector: Assessing potentials for process innovation in a high-contact service industry. Electronic Markets, 31 (3), 529–551. https://doi.org/10.1007/s12525-020-00443-2
Blondel, V. D., Guillaume, J. L., Lambiotte, R., & Lefebvre, E. (2008). Fast unfolding of communities in large networks. Journal of Statistical Mechanics: Theory and Experiment, 2008 (10), P10008. https://doi.org/10.1088/1742-5468/2008/10/P10008
Bo, X., Benbasat, I., Xiao, B., & Benbasat, I. (2007). E-commerce product recommendation agents: Use, characteristics, and impact. MIS Quarterly, 31 (1), 137–209. https://doi.org/10.2307/25148784
Bobadilla, J., Ortega, F., Hernando, A., & Gutiérrez, A. (2013). Recommender systems survey. Knowledge-Based Systems, 46 , 109–132. https://doi.org/10.1016/j.knosys.2013.03.012
Bobadilla, J., Ortega, F., Hernando, A., & Bernal, J. (2012). A collaborative filtering approach to mitigate the new user cold start problem. Knowledge-Based Systems, 26 , 225–238. https://doi.org/10.1016/j.knosys.2011.07.021
Bolton, R. J., & Hand, D. J. (2002). Statistical fraud detection: A review. Statistical Science, 17 (3), 235–255. https://doi.org/10.1214/ss/1042727940
Bondielli, A., & Marcelloni, F. (2019). A survey on fake news and rumour detection techniques. Information Sciences, 497 , 38–55. https://doi.org/10.1016/j.ins.2019.05.035
Borges, A. F. S., Laurindo, F. J. B., Spínola, M. M., Gonçalves, R. F., & Mattos, C. A. (2020). The strategic use of artificial intelligence in the digital era: Systematic literature review and future research directions. International Journal of Information Management , 102225. https://doi.org/10.1016/j.ijinfomgt.2020.102225
Bose, I., & Chen, X. (2009). Hybrid models using unsupervised clustering for prediction of customer churn. Journal of Organizational Computing and Electronic Commerce, 19 (2), 133–151. https://doi.org/10.1080/10919390902821291
Brazier, F. M. T., Cornelissen, F., Gustavsson, R., Jonker, C. M., Lindeberg, O., Polak, B., & Treur, J. (2002). A multi-agent system performing one-to-many negotiation for load balancing of electricity use. Electronic Commerce Research and Applications, 1 (2), 208–224. https://doi.org/10.1016/S1567-4223(02)00013-3
Breiman, L. (2001). Random forests. Machine Learning, 45 (1), 5–32. https://doi.org/10.1023/A:1010933404324
Brusilovski, P., Kobsa, A., & Nejdl, W. (2007). The Adaptive Web Methods and Strategies of Web Personalization. In Lecture Notes in Computer Science (including subseries Lecture Notes in Artificial Intelligence and Lecture Notes in Bioinformatics): Vol 4321 LNCS . Springer Science & Business Media.
Brynjolfsson, E., Wang, C., & Zhang, X. (2021). The economics of IT and digitization: Eight questions for research. MIS Quarterly, 45 (1), 473–477.
Buettner, R. (2017). Predicting user behavior in electronic markets based on personality-mining in large online social networks: A personality-based product recommender framework. Electronic Markets, 27 (3), 247–265. https://doi.org/10.1007/s12525-016-0228-z
Bukhari, A. C., & Kim, Y.-G. (2012). Integration of a secure type-2 fuzzy ontology with a multi-agent platform: A proposal to automate the personalized flight ticket booking domain. Information Sciences, 198 , 24–47. https://doi.org/10.1016/j.ins.2012.02.036
Burke, R. (2002). Hybrid Recommender Systems: Survey and Experiments. User Modeling and User-Adapted Interaction, 12 (4), 331–370. https://doi.org/10.1023/A:1021240730564
Büyüközkan, G., Feyzioǧlu, O., & Nebol, E. (2008). Selection of the strategic alliance partner in logistics value chain. International Journal of Production Economics, 113 (1), 148–158. https://doi.org/10.1016/j.ijpe.2007.01.016
Cacheda, F., Carneiro, V., Fernández, D., & Formoso, V. (2011). Comparison of collaborative filtering algorithms: Limitations of current techniques and proposals for scalable, high-performance recommender systems. ACM Transactions on the Web , 5 (1). https://doi.org/10.1145/1921591.1921593
Cai, H., & Zhang, F. (2019). Detecting shilling attacks in recommender systems based on analysis of user rating behavior. Knowledge-Based Systems, 177 , 22–43. https://doi.org/10.1016/j.knosys.2019.04.001
Campbell, C., Sands, S., Ferraro, C., Tsao (Jody), H.-Y., & Mavrommatis, A. (2020). From data to action: How marketers can leverage AI. Business Horizons, 63 (2), 227–243. https://doi.org/10.1016/j.bushor.2019.12.002
Cao, Q., & Schniederjans, M. J. (2006). Agent-mediated architecture for reputation-based electronic tourism systems: A neural network approach. Information and Management, 43 (5), 598–606. https://doi.org/10.1016/j.im.2006.03.001
Cao, Y., & Li, Y. (2007). An intelligent fuzzy-based recommendation system for consumer electronic products. Expert Systems with Applications, 33 (1), 230–240. https://doi.org/10.1016/j.eswa.2006.04.012
Carbó, J., Molina, J. M., & Dávila, J. (2007). Avoiding malicious agents in E-commerce using fuzzy recommendations. Journal of Organizational Computing and Electronic Commerce, 17 (2), 101–117. https://doi.org/10.1080/10919390701293972
Cardoso, G., & Gomide, F. (2007). Newspaper demand prediction and replacement model based on fuzzy clustering and rules. Information Sciences, 177 (21), 4799–4809. https://doi.org/10.1016/j.ins.2007.05.009
Castillo, P. A., Mora, A. M., Faris, H., Merelo, J. J., García-Sánchez, P., Fernández-Ares, A. J., De las Cuevas, P., & García-Arenas, M. I. (2017). Applying computational intelligence methods for predicting the sales of newly published books in a real editorial business management environment. Knowledge-Based Systems, 115 , 133–151. https://doi.org/10.1016/j.knosys.2016.10.019
Chang, C. C., & Lin, C. J. (2011). LIBSVM: A Library for support vector machines. ACM Transactions on Intelligent Systems and Technology , 2 (3). https://doi.org/10.1145/1961189.1961199
Chang, J.-S., & Chang, W.-H. (2014). Analysis of fraudulent behavior strategies in online auctions for detecting latent fraudsters. Electronic Commerce Research and Applications, 13 (2), 79–97. https://doi.org/10.1016/j.elerap.2013.10.004
Chang, R. M., Kauffman, R. J., & Kwon, Y. (2014). Understanding the paradigm shift to computational social science in the presence of big data. Decision Support Systems, 63 , 67–80. https://doi.org/10.1016/j.dss.2013.08.008
Chang, S. E., & Jang, Y. T. (2009). Assessing customer satisfaction in a V-commerce environment. Journal of Organizational Computing and Electronic Commerce, 19 (1), 30–49. https://doi.org/10.1080/10919390802605083
Chang, W.-H., & Chang, J.-S. (2012). An effective early fraud detection method for online auctions. Electronic Commerce Research and Applications, 11 (4), 346–360. https://doi.org/10.1016/j.elerap.2012.02.005
Chang, W.-L. (2011). iValue: A knowledge-based system for estimating customer prospect value. Knowledge-Based Systems, 24 (8), 1181–1186. https://doi.org/10.1016/j.knosys.2011.05.004
Chaudhuri, N., Gupta, G., Vamsi, V., & Bose, I. (2021). On the platform but will they buy? Predicting customers’ purchase behavior using deep learning. Decision Support Systems, 149 , 113622. https://doi.org/10.1016/j.dss.2021.113622
Chen, C. C., & Chung, M.-C. (2015). Predicting the success of group buying auctions via classification. Knowledge-Based Systems, 89 , 627–640. https://doi.org/10.1016/j.knosys.2015.09.009
Chen, K., Luo, P., & Wang, H. (2017). An influence framework on product word-of-mouth (WoM) measurement. Information and Management, 54 (2), 228–240. https://doi.org/10.1016/j.im.2016.06.010
Chen, L., Chen, G., & Wang, F. (2015). Recommender systems based on user reviews: The state of the art. User Modeling and User-Adapted Interaction, 25 (2), 99–154. https://doi.org/10.1007/s11257-015-9155-5
Chen, L., & Wang, F. (2013). Preference-based clustering reviews for augmenting e-commerce recommendation. Knowledge-Based Systems, 50 , 44–59. https://doi.org/10.1016/j.knosys.2013.05.006
Chen, M.-Y. (2013). A hybrid ANFIS model for business failure prediction utilizing particle swarm optimization and subtractive clustering. Information Sciences, 220 , 180–195. https://doi.org/10.1016/j.ins.2011.09.013
Chen, M. Y., Kiciman, E., Fratkin, E., Fox, A., & Brewer, E. (2002). Pinpoint: Problem determination in large, dynamic internet services. Proceedings of the 2002 International Conference on Dependable Systems and Networks , 595–604. https://doi.org/10.1109/DSN.2002.1029005
Chen, R., Wang, Q., & Xu, W. (2019). Mining user requirements to facilitate mobile app quality upgrades with big data. Electronic Commerce Research and Applications, 38 , 100889. https://doi.org/10.1016/j.elerap.2019.100889
Chen, R., Zheng, Y., Xu, W., Liu, M., & Wang, J. (2018). Secondhand seller reputation in online markets: A text analytics framework. Decision Support Systems, 108 , 96–106. https://doi.org/10.1016/j.dss.2018.02.008
Chen, Y.-S. (2012). Classifying credit ratings for Asian banks using integrating feature selection and the CPDA-based rough sets approach. Knowledge-Based Systems, 26 , 259–270. https://doi.org/10.1016/j.knosys.2011.08.021
Chen, Y. L., Cheng, L. C., & Hsu, W. Y. (2013). A new approach to the group ranking problem: Finding consensus ordered segments from users’ preference data. Decision Sciences, 44 (6), 1091–1119. https://doi.org/10.1111/deci.12048
Chen, Y. L., Tang, K., Wu, C. C., & Jheng, R. Y. (2014). Predicting the influence of users’ posted information for eWOM advertising in social networks. Electronic Commerce Research and Applications, 13 (6), 431–439. https://doi.org/10.1016/j.elerap.2014.10.001
Cheung, K. W., Kwok, J. T., Law, M. H., & Tsui, K. C. (2003). Mining customer product ratings for personalized marketing. Decision Support Systems, 35 (2), 231–243. https://doi.org/10.1016/S0167-9236(02)00108-2
Chiarini Tremblay, M., Kohli, R., & Forsgren, N. (2021). Theories in Flux: Reimagining Theory Building in the Age of Machine Learning. MIS Quarterly, 45 (1), 455–459.
Cho, Y. H., Kim, J. K., & Kim, S. H. (2002). A personalized recommender system based on web usage mining and decision tree induction. Expert Systems with Applications, 23 (3), 329–342. https://doi.org/10.1016/S0957-4174(02)00052-0
Chong, A.Y.-L. (2013a). A two-staged SEM-neural network approach for understanding and predicting the determinants of m-commerce adoption. Expert Systems with Applications, 40 (4), 1240–1247. https://doi.org/10.1016/j.eswa.2012.08.067
Chong, A. Y. L. (2013b). Predicting m-commerce adoption determinants: A neural network approach. Expert Systems with Applications, 40 (2), 523–530. https://doi.org/10.1016/j.eswa.2012.07.068
Chou, T. H., & Seng, J. L. (2009). An intelligent multi-agent e-services method-An international telecommunication example. Information and Management, 46 (6), 342–350. https://doi.org/10.1016/j.im.2009.05.006
Chow, H. K. H., Choy, K. L., & Lee, W. B. (2007). A dynamic logistics process knowledge-based system - An RFID multi-agent approach. Knowledge-Based Systems, 20 (4), 357–372. https://doi.org/10.1016/j.knosys.2006.08.004
Chu, B.-H., Tsai, M.-S., & Ho, C.-S. (2007). Toward a hybrid data mining model for customer retention. Knowledge-Based Systems, 20 (8), 703–718. https://doi.org/10.1016/j.knosys.2006.10.003
Chung, W. (2014). BizPro: Extracting and categorizing business intelligence factors from textual news articles. International Journal of Information Management, 34 (2), 272–284. https://doi.org/10.1016/j.ijinfomgt.2014.01.001
Cram, W. A., Templier, M., & Paré, G. (2020). (Re)considering the concept of literature review reproducibility. Journal of the Association for Information Systems, 21 (5), 1103–1114. https://doi.org/10.17705/1jais.00630
Cui, G., Wong, M. L., & Lui, H. K. (2006). Machine learning for direct marketing response models: Bayesian networks with evolutionary programming. Management Science, 52 (4), 597–612. https://doi.org/10.1287/mnsc.1060.0514
Da’u, A., Salim, N., Rabiu, I., & Osman, A. (2020). Recommendation system exploiting aspect-based opinion mining with deep learning method. Information Sciences, 512 , 1279–1292. https://doi.org/10.1016/j.ins.2019.10.038
Das, S. R., & Chen, M. Y. (2007). Yahoo! for amazon: Sentiment extraction from small talk on the Web. Management Science, 53 (9), 1375–1388. https://doi.org/10.1287/mnsc.1070.0704
Dastani, M., Jacobs, N., Jonker, C. M., & Treur, J. (2005). Modelling user preferences and mediating agents in electronic commerce. Knowledge-Based Systems, 18 (7), 335–352. https://doi.org/10.1016/j.knosys.2005.05.001
Datta, S., Bhaduri, K., Giannella, C., Wolff, R., & Kargupta, H. (2006). Distributed Data Mining in Peer-to-Peer Networks. IEEE Internet Computing, 10 (4), 18–26. https://doi.org/10.1109/MIC.2006.74
Davenport, T., Guha, A., Grewal, D., & Bressgott, T. (2020). How artificial intelligence will change the future of marketing. Journal of the Academy of Marketing Science, 48 (1), 24–42. https://doi.org/10.1007/s11747-019-00696-0
de Barcelos Silva, A., Gomes, M. M., da Costa, C. A., da Rosa Righi, R., Barbosa, J. L. V., Pessin, G., De Doncker, G., & Federizzi, G. (2020). Intelligent personal assistants: A systematic literature review. Expert Systems with Applications, 147 , 113193. https://doi.org/10.1016/j.eswa.2020.113193
de Bellis, E., & Venkataramani Johar, G. (2020). Autonomous Shopping Systems: Identifying and Overcoming Barriers to Consumer Adoption. Journal of Retailing, 96 (1), 74–87. https://doi.org/10.1016/j.jretai.2019.12.004
De Carolis, B., de Gemmis, M., Lops, P., & Palestra, G. (2017). Recognizing users feedback from non-verbal communicative acts in conversational recommender systems. Pattern Recognition Letters, 99 , 87–95.
De Smedt, J., Lacka, E., Nita, S., Kohls, H. H., & Paton, R. (2021). Session stitching using sequence fingerprinting for web page visits. Decision Support Systems, 150 , 113579. https://doi.org/10.1016/j.dss.2021.113579
Decker, R., & Trusov, M. (2010). Estimating aggregate consumer preferences from online product reviews. International Journal of Research in Marketing, 27 (4), 293–307. https://doi.org/10.1016/j.ijresmar.2010.09.001
Deng, S., Tan, C. W., Wang, W., & Pan, Y. (2019). Smart Generation System of Personalized Advertising Copy and Its Application to Advertising Practice and Research. Journal of Advertising, 48 (4), 356–365. https://doi.org/10.1080/00913367.2019.1652121
Dong, M., Zeng, X., Koehl, L., & Zhang, J. (2020). An interactive knowledge-based recommender system for fashion product design in the big data environment. Information Sciences, 540 , 469–488. https://doi.org/10.1016/j.ins.2020.05.094
Duan, Y., Edwards, J. S., & Dwivedi, Y. K. (2019). Artificial intelligence for decision making in the era of Big Data – evolution, challenges and research agenda. International Journal of Information Management, 48 , 63–71. https://doi.org/10.1016/j.ijinfomgt.2019.01.021
Dwivedi, Y. K., Hughes, L., Ismagilova, E., Aarts, G., Coombs, C., Crick, T., Duan, Y., Dwivedi, R., Edwards, J., Eirug, A., Galanos, V., Ilavarasan, P. V., Janssen, M., Jones, P., Kar, A. K., Kizgin, H., Kronemann, B., Lal, B., Lucini, Medaglia, R., Le Meunier-FitzHugh, K., Le Meunier-FitzHugh, L. C., Misra, S., Mogaji, E., Sharma, S. K., Bahadur Singh, J., Raghavan, V., Raman, R., P. Rana, N., Samothrakis, S., Spencer, J., Tamilmani, K., Tubadji, A. Walton, P., & Williams, M. D. (2019). Artificial Intelligence (AI): Multidisciplinary perspectives on emerging challenges, opportunities, and agenda for research, practice and policy. International Journal of Information Management , 101994. https://doi.org/10.1016/j.ijinfomgt.2019.08.002
Dwivedi, Y. K., Ismagilova, E., Hughes, D. L., Carlson, J., Filieri, R., Jacobson, J., Jain, V., Karjaluoto, H., Kefi, H., Krishen, A. S., Kumar, V., Rahman, M. M., Raman, R., Rauschnabel, P. A., Rowley, J., Salo, J., Tran, G. A., & Wang, Y. (2020). Setting the future of digital and social media marketing research: Perspectives and research propositions. International Journal of Information Management , 102168. https://doi.org/10.1016/j.ijinfomgt.2020.102168
Esfahani, H. J., Tavasoli, K., & Jabbarzadeh, A. (2019). Big data and social media: A scientometrics analysis. International Journal of Data and Network Science, 3 (3), 145–164. https://doi.org/10.5267/j.ijdns.2019.2.007
Esmeli, R., Bader-El-Den, M., & Abdullahi, H. (2021). Towards early purchase intention prediction in online session based retailing systems. Electronic Markets, 31 (3). https://doi.org/10.1007/s12525-020-00448-x
Fang, H., Zhang, J., & Şensoy, M. (2018). A generalized stereotype learning approach and its instantiation in trust modeling. Electronic Commerce Research and Applications, 30 , 149–158. https://doi.org/10.1016/j.elerap.2018.06.004
Fang, X., Hu, P. J. H., Li, Z. L., & Tsai, W. (2013). Predicting adoption probabilities in social networks. Information Systems Research, 24 (1), 128–145. https://doi.org/10.1287/isre.1120.0461
Fasli, M., & Kovalchuk, Y. (2011). Learning approaches for developing successful seller strategies in dynamic supply chain management. Information Sciences, 181 (16), 3411–3426. https://doi.org/10.1016/j.ins.2011.04.014
Feng, S., Zhang, H., Wang, L., Liu, L., & Xu, Y. (2019). Detecting the latent associations hidden in multi-source information for better group recommendation. Knowledge-Based Systems, 171 , 56–68. https://doi.org/10.1016/j.knosys.2019.02.002
Ferrara, E., De Meo, P., Fiumara, G., & Baumgartner, R. (2014). Web data extraction, applications and techniques: A survey. Knowledge-Based Systems, 70 , 301–323. https://doi.org/10.1016/j.knosys.2014.07.007
Fiore, U., De Santis, A., Perla, F., Zanetti, P., & Palmieri, F. (2019). Using generative adversarial networks for improving classification effectiveness in credit card fraud detection. Information Sciences, 479 , 448–455. https://doi.org/10.1016/j.ins.2017.12.030
Fosso Wamba, S. (2020). Humanitarian supply chain: a bibliometric analysis and future research directions. Annals of Operations Research , 1–27. https://doi.org/10.1007/s10479-020-03594-9
Friedman, J. H. (2001). Greedy function approximation: A gradient boosting machine. Annals of Statistics, 29 (5), 1189–1232. https://doi.org/10.1214/aos/1013203451
Galitsky, B. (2006). Reasoning about attitudes of complaining customers. Knowledge-Based Systems, 19 (7), 592–615. https://doi.org/10.1016/j.knosys.2006.03.006
Geng, Q., Deng, S., Jia, D., & Jin, J. (2020). Cross-domain ontology construction and alignment from online customer product reviews. Information Sciences, 531 , 47–67. https://doi.org/10.1016/j.ins.2020.03.058
Ghavipour, M., & Meybodi, M. R. (2016). An adaptive fuzzy recommender system based on learning automata. Electronic Commerce Research and Applications, 20 , 105–115. https://doi.org/10.1016/j.elerap.2016.10.002
Ghiassi, M., Zimbra, D., & Lee, S. (2016). Targeted Twitter Sentiment Analysis for Brands Using Supervised Feature Engineering and the Dynamic Architecture for Artificial Neural Networks. Journal of Management Information Systems, 33 (4), 1034–1058. https://doi.org/10.1080/07421222.2016.1267526
Ghose, A., & Ipeirotis, P. G. (2011). Estimating the helpfulness and economic impact of product reviews: Mining text and reviewer characteristics. IEEE Transactions on Knowledge and Data Engineering, 23 (10), 1498–1512. https://doi.org/10.1109/TKDE.2010.188
Gielens, K., & Steenkamp, J.-B.E.M. (2019). Branding in the era of digital (dis)intermediation. International Journal of Research in Marketing, 36 (3), 367–384. https://doi.org/10.1016/j.ijresmar.2019.01.005
Gokmen, T., & Vlasov, Y. (2016). Acceleration of Deep Neural Network Training with Resistive Cross-Point Devices: Design Considerations. Frontiers in Neuroscience, 10 , 333. https://doi.org/10.3389/fnins.2016.00333
Goldberg, D., Nichols, D., Oki, B. M., & Terry, D. (1992). Using collaborative filtering to Weave an Information tapestry. Communications of the ACM, 35 (12), 61–70. https://doi.org/10.1145/138859.138867
Goldberg, K., Roeder, T., Gupta, D., & Perkins, C. (2001). Eigentaste: A Constant Time Collaborative Filtering Algorithm. Information Retrieval, 4 (2), 133–151. https://doi.org/10.1023/A:1011419012209
Gong, J., Abhishek, V., & Li, B. (2018). Examining the impact of keyword ambiguity on search advertising performance: A topic model approach. MIS Quarterly, 42 (3), 805–829. https://doi.org/10.25300/MISQ/2018/14042
Greenstein-Messica, A., & Rokach, L. (2018). Personal price aware multi-seller recommender system: Evidence from eBay. Knowledge-Based Systems, 150 , 14–26. https://doi.org/10.1016/j.knosys.2018.02.026
Greenstein-Messica, A., & Rokach, L. (2020). Machine learning and operation research based method for promotion optimization of products with no price elasticity history. Electronic Commerce Research and Applications, 40 , 100914. https://doi.org/10.1016/j.elerap.2019.100914
Griggs, K., & Wild, R. (2003). Intelligent support for sophisticated e-commerce services: An agent-based auction framework modeled after the New York stock exchange specialist system. E-Service Journal, 2 (2), 87–104. https://doi.org/10.2979/esj.2003.2.2.87
Grønsund, T., & Aanestad, M. (2020). Augmenting the algorithm: Emerging human-in-the-loop work configurations. Journal of Strategic Information Systems , 29 (2). https://doi.org/10.1016/j.jsis.2020.101614
Gu, X., Wu, S., Peng, P., Shou, L., Chen, K., & Chen, G. (2017). CSIR4G: An effective and efficient cross-scenario image retrieval model for glasses. Information Sciences, 417 , 310–327. https://doi.org/10.1016/j.ins.2017.07.027
Guan, J., Shi, D., Zurada, J. M., & Levitan, A. S. (2014). Analyzing Massive Data Sets: An Adaptive Fuzzy Neural Approach for Prediction, with a Real Estate Illustration. Journal of Organizational Computing and Electronic Commerce, 24 (1), 94–112. https://doi.org/10.1080/10919392.2014.866505
Guan, S.-U., Chan, T. K., & Zhu, F. (2005). Evolutionary intelligent agents for e-commerce: Generic preference detection with feature analysis. Electronic Commerce Research and Applications, 4 (4), 377–394. https://doi.org/10.1016/j.elerap.2005.07.002
Guan, Y., Wei, Q., & Chen, G. (2019). Deep learning based personalized recommendation with multi-view information integration. Decision Support Systems, 118 , 58–69. https://doi.org/10.1016/j.dss.2019.01.003
Gunnec, D., & Raghavan, S. (2017). Integrating Social Network Effects in the Share-Of-Choice Problem. Decision Sciences, 48 (6), 1098–1131. https://doi.org/10.1111/deci.12246
Guo, G., Qiu, H., Tan, Z., Liu, Y., Ma, J., & Wang, X. (2017a). Resolving data sparsity by multi-type auxiliary implicit feedback for recommender systems. Knowledge-Based Systems, 138 , 202–207. https://doi.org/10.1016/j.knosys.2017.10.005
Guo, G., Zhang, J., & Thalmann, D. (2014). Merging trust in collaborative filtering to alleviate data sparsity and cold start. Knowledge-Based Systems, 57 , 57–68. https://doi.org/10.1016/j.knosys.2013.12.007
Guo, G., Zhang, J., Zhu, F., & Wang, X. (2017b). Factored similarity models with social trust for top-N item recommendation. Knowledge-Based Systems, 122 , 17–25. https://doi.org/10.1016/j.knosys.2017.01.027
Guo, H., Pathak, P., & Cheng, H. K. (2015). Estimating Social Influences from Social Networking Sites-Articulated Friendships versus Communication Interactions. Decision Sciences, 46 (1), 135–163. https://doi.org/10.1111/deci.12118
Guo, X., Wei, Q., Chen, G., Zhang, J., & Qiao, D. (2017). Extracting representative information on intra-organizational blogging platforms. MIS Quarterly, 41 (4), 1105–1127. https://doi.org/10.25300/MISQ/2017/41.4.05
Gupta, S., & Kant, V. (2020). Credibility score based multi-criteria recommender system. Knowledge-Based Systems, 196 , 105756. https://doi.org/10.1016/j.knosys.2020.105756
Guttman, R. H., Moukas, A. G., & Maes, P. (1998). Agent-mediated electronic commerce: A survey. Knowledge Engineering Review, 13 (2), 147–159. https://doi.org/10.1017/S0269888998002082
Ha, S. H., & Lee, J. H. (2009). Dynamic dissemination of personalized content on the web. Journal of Organizational Computing and Electronic Commerce, 19 (2), 96–111. https://doi.org/10.1080/10919390902821218
Hamad, H., Elbeltagi, I., & El-Gohary, H. (2018). An empirical investigation of business-to-business e-commerce adoption and its impact on SMEs competitive advantage: The case of Egyptian manufacturing SMEs. Strategic Change, 27 (3), 209–229. https://doi.org/10.1002/jsc.2196
Han, J., Zheng, L., Huang, H., Xu, Y., Yu, P. S., & Zuo, W. (2019). Deep Latent Factor Model with Hierarchical Similarity Measure for recommender systems. Information Sciences, 503 , 521–532. https://doi.org/10.1016/j.ins.2019.07.024
Han, J., Kamber, M., & Pei, J. (2001). Data mining: Concepts and technologies. Data Mining Concepts Models Methods & Algorithms, 5 (4), 1–18.
Hanani, U., Shapira, B., & Shoval, P. (2001). Information filtering: Overview of issues, research and systems. User Modeling and User-Adapted Interaction, 11 (3), 203–259. https://doi.org/10.1023/A:1011196000674
Hansen, J. H. L., & Hasan, T. (2015). Speaker recognition by machines and humans: A tutorial review. IEEE Signal Processing Magazine, 32 (6), 74–99. https://doi.org/10.1109/MSP.2015.2462851
Hassan, N. R., & Loebbecke, C. (2017). Engaging scientometrics in information systems. Journal of Information Technology, 32 (1), 85–109.
He, J., Fang, X., Liu, H., & Li, X. (2019). Mobile app recommendation: An involvement-enhanced approach. MIS Quarterly, 43 (3), 827–850. https://doi.org/10.25300/MISQ/2019/15049
He, W., Zhang, Z., & Akula, V. (2018). Comparing consumer-produced product reviews across multiple websites with sentiment classification. Journal of Organizational Computing and Electronic Commerce, 28 (2), 142–156. https://doi.org/10.1080/10919392.2018.1444350
Herce-Zelaya, J., Porcel, C., Bernabé-Moreno, J., Tejeda-Lorente, A., & Herrera-Viedma, E. (2020). New technique to alleviate the cold start problem in recommender systems using information from social media and random decision forests. Information Sciences, 536 , 156–170. https://doi.org/10.1016/j.ins.2020.05.071
Herlocker, J. L., Konstan, J. A., Terveen, L. G., & Riedl, J. T. (2004). Evaluating collaborative filtering recommender systems. ACM Transactions on Information Systems, 22 (1), 5–53. https://doi.org/10.1145/963770.963772
Hernando, A., Bobadilla, J., Ortega, F., & Gutiérrez, A. (2017). A probabilistic model for recommending to new cold-start non-registered users. Information Sciences, 376 , 216–232. https://doi.org/10.1016/j.ins.2016.10.009
Hill, S., & Ready-Campbell, N. (2011). Expert Stock Picker: The Wisdom of (Experts in) Crowds. International Journal of Electronic Commerce, 15 (3), 73–102. https://doi.org/10.1093/JEC1086-4415150304
Himabindu, T. V. R., Padmanabhan, V., & Pujari, A. K. (2018). Conformal matrix factorization based recommender system. Information Sciences, 467 , 685–707. https://doi.org/10.1016/j.ins.2018.04.004
Hinojo-Lucena, F. J., Aznar-Díaz, I., Cáceres-Reche, M. P., & Romero-Rodríguez, J. M. (2019). Artificial intelligence in higher education: A bibliometric study on its impact in the scientific literature. Education Sciences, 9 (1), 51. https://doi.org/10.3390/educsci9010051
Hirsch, J. E. (2010). An index to quantify an individual’s scientific research output that takes into account the effect of multiple coauthorship. Scientometrics, 85 (3), 741–754. https://doi.org/10.1007/s11192-010-0193-9
Hirt, R., Kühl, N., & Satzger, G. (2019). Cognitive computing for customer profiling: Meta classification for gender prediction. Electronic Markets, 29 (1), 93–106. https://doi.org/10.1007/s12525-019-00336-z
Hogenboom, A., Ketter, W., van Dalen, J., Kaymak, U., Collins, J., & Gupta, A. (2015). Adaptive Tactical Pricing in Multi-Agent Supply Chain Markets Using Economic Regimes. Decision Sciences, 46 (4), 791–818. https://doi.org/10.1111/deci.12146
Holsapple, C. W., & Singh, M. (2000). Electronic commerce: From a definitional taxonomy toward a knowledge-management view. Journal of Organizational Computing and Electronic Commerce, 10 (3), 149–170. https://doi.org/10.1207/S15327744JOCE1003_01
Hong, W., Thong, J. Y. L., & Tam, K. Y. (2004). The effects of information format and shopping task on consumers’ online shopping behavior: A cognitive fit perspective. Journal of Management Information Systems, 21 (3), 149–184. https://doi.org/10.1080/07421222.2004.11045812
Hopkins, J., Kafali, Ö., Alrayes, B., & Stathis, K. (2019). Pirasa: Strategic protocol selection for e-commerce agents. Electronic Markets, 29 (2), 239–252. https://doi.org/10.1007/s12525-018-0307-4
Hu, Y.-C. (2014). Recommendation using neighborhood methods with preference-relation-based similarity. Information Sciences, 284 , 18–30. https://doi.org/10.1016/j.ins.2014.06.043
Huang, M.-H., & Rust, R. T. (2021). A strategic framework for artificial intelligence in marketing. Journal of the Academy of Marketing Science, 49 (1), 30–50. https://doi.org/10.3239/s11747-020-00749-9
Huang, M. H., & Rust, R. T. (2018). Artificial Intelligence in Service. Journal of Service Research, 21 (2), 155–172. https://doi.org/10.1177/1094670517752459
Huang, M. H., & Rust, R. T. (2020). Engaged to a robot? The role of AI in service. Journal of Service Research , 24(1), 30–41. https://doi.org/10.1177/1094670520902266
Huang, Z., Zeng, D., & Chen, H. (2007). A comparison of collaborative-filtering algorithms for ecommerce. IEEE Intelligent Systems, 22 (5), 68–78. https://doi.org/10.1109/MIS.2007.4338497
Huysman, M. (2020). Information systems research on artificial intelligence and work: A commentary on “Robo-Apocalypse cancelled? Reframing the automation and future of work debate.” Journal of Information Technology, 35 (4), 307–309. https://doi.org/10.1177/0268396220926511
Iovine, A., Narducci, F., & Semeraro, G. (2020). Conversational Recommender Systems and natural language: A study through the ConveRSE framework. Decision Support Systems, 131 , 113250. https://doi.org/10.1016/j.dss.2020.113250
Isinkaye, F. O., Folajimi, Y. O., & Ojokoh, B. A. (2015). Recommendation systems: Principles, methods and evaluation. Egyptian Informatics Journal, 16 (3), 261–273. https://doi.org/10.1016/j.eij.2015.06.005
Ito, T., Hattori, H., & Shintani, T. (2002). A cooperative exchanging mechanism among seller agents for group-based sales. Electronic Commerce Research and Applications, 1 (2), 138–149. https://doi.org/10.1016/S1567-4223(02)00010-8
Iwański, J., Suchacka, G., & Chodak, G. (2018). Application of the Information Bottleneck method to discover user profiles in a Web store. Journal of Organizational Computing and Electronic Commerce, 28 (2), 98–121. https://doi.org/10.1080/10919392.2018.1444340
Jannach, D., Manzoor, A., Cai, W., & Chen, L. (2021). A Survey on Conversational Recommender Systems. ACM Computing Surveys (CSUR), 54 (5), 1–36.
Jeong, W. S., Han, S. G., & Jo, G. S. (2003). Intelligent Cyber Logistics Using Reverse Auction in Electronic Commerce. Journal of Organizational Computing and Electronic Commerce, 13 (3–4), 191–209. https://doi.org/10.1207/s15327744joce133&4_03
Jeyaraj, A., & Zadeh, A. H. (2020). Evolution of information systems research: Insights from topic modeling. Information & Management, 57 (4), 103207. https://doi.org/10.1016/j.im.2019.103207
Ji, K., & Shen, H. (2015). Addressing cold-start: Scalable recommendation with tags and keywords. Knowledge-Based Systems, 83 , 42–50. https://doi.org/10.1016/j.knosys.2015.03.008
Ji, S., & juan, Zhang, Q., Li, J., Chiu, D. K. W., Xu, S., Yi, L., & Gong, M. (2020). A burst-based unsupervised method for detecting review spammer groups. Information Sciences, 536 , 454–469. https://doi.org/10.1016/j.ins.2020.05.084
Jiang, G., Ma, F., Shang, J., & Chau, P. Y. K. (2014). Evolution of knowledge sharing behavior in social commerce: An agent-based computational approach. Information Sciences, 278 , 250–266. https://doi.org/10.1016/j.ins.2014.03.051
Jiang, Z., Mookerjee, V. S., & Sarkar, S. (2005). Lying on the web: Implications for expert systems redesign. Information Systems Research, 16 (2), 131–148. https://doi.org/10.1287/isre.1050.0046
Jøsang, A., Ismail, R., & Boyd, C. (2007). A survey of trust and reputation systems for online service provision. Decision Support Systems, 43 (2), 618–644. https://doi.org/10.1016/j.dss.2005.05.019
Julià, C., Sappa, A. D., Lumbreras, F., Serrat, J., & López, A. (2009). Predicting Missing Ratings in Recommender Systems: Adapted Factorization Approach. International Journal of Electronic Commerce, 14 (2), 89–108. https://doi.org/10.1093/JEC1086-4415140203
Kagan, S., & Bekkerman, R. (2018). Predicting Purchase Behavior of Website Audiences. International Journal of Electronic Commerce, 22 (4), 510–539. https://doi.org/10.0456/10864415.2018.1485084
Kaiser, C., Schlick, S., & Bodendorf, F. (2011). Warning system for online market research - Identifying critical situations in online opinion formation. Knowledge-Based Systems, 24 (6), 824–836. https://doi.org/10.1016/j.knosys.2011.03.004
Kalakota, R., & Whinston, A. B. (1997). Electronic commerce: a manager’s guide . Addison-Wesley Professional.
Kandula, S., Krishnamoorthy, S., & Roy, D. (2021). A prescriptive analytics framework for efficient E-commerce order delivery. Decision Support Systems, 147 , 113584. https://doi.org/10.1016/j.dss.2021.113584
Kaplan, A., & Haenlein, M. (2019). Siri, Siri, in my hand: Who’s the fairest in the land? On the interpretations, illustrations, and implications of artificial intelligence. Business Horizons, 62 (1), 15–25. https://doi.org/10.1016/j.bushor.2018.08.004
Kauffman, R. J., Kim, K., Lee, S.-Y.T., Hoang, A.-P., & Ren, J. (2017). Combining machine-based and econometrics methods for policy analytics insights. Electronic Commerce Research and Applications, 25 , 115–140. https://doi.org/10.1016/j.elerap.2017.04.004
Kazienko, P., & Adamski, M. (2007). AdROSA-Adaptive personalization of web advertising. Information Sciences, 177 (11), 2269–2295. https://doi.org/10.1016/j.ins.2007.01.002
Keegan, S., O’Hare, G. M. P., & O’Grady, M. J. (2008). Easishop: Ambient intelligence assists everyday shopping. Information Sciences, 178 (3), 588–611. https://doi.org/10.1016/j.ins.2007.08.027
Ketter, W., Collins, J., Gini, M., Gupta, A., & Schrater, P. (2012). Real-Time tactical and strategic sales management for intelligent agents guided by economic regimes. Information Systems Research, 23 (4), 1263–1283. https://doi.org/10.1287/isre.1110.0415
Khare, V. R., & Chougule, R. (2012). Decision support for improved service effectiveness using domain aware text mining. Knowledge-Based Systems, 33 , 29–40. https://doi.org/10.1016/j.knosys.2012.03.005
Khopkar, S. S., & Nikolaev, A. G. (2017). Predicting long-term product ratings based on few early ratings and user base analysis. Electronic Commerce Research and Applications, 21 , 38–49. https://doi.org/10.1016/j.elerap.2016.12.002
Kiekintveld, C., Miller, J., Jordan, P. R., Callender, L. F., & Wellman, M. P. (2009). Forecasting market prices in a supply chain game. Electronic Commerce Research and Applications, 8 (2), 63–77. https://doi.org/10.1016/j.elerap.2008.11.005
Kietzmann, J., Paschen, J., & Treen, E. (2018). Artificial intelligence in advertising: How marketers can leverage artificial intelligence along the consumer journey. Journal of Advertising Research, 58 (3), 263–267. https://doi.org/10.2501/JAR-2018-035
Kim, D. J., Song, Y. I., Braynov, S. B., & Rao, H. R. (2005a). A multidimensional trust formation model in B-to-C e-commerce: A conceptual framework and content analyses of academia/practitioner perspectives. Decision Support Systems, 40 (2), 143–165. https://doi.org/10.1016/j.dss.2004.01.006
Kim, D., Park, C., Oh, J., & Yu, H. (2017). Deep hybrid recommender systems via exploiting document context and statistics of items. Information Sciences, 417 , 72–87. https://doi.org/10.1016/j.ins.2017.06.026
Kim, J. W., Lee, B. H., Shaw, M. J., Chang, H. L., & Nelson, M. (2001). Application of decision-tree induction techniques to personalized advertisements on internet storefronts. International Journal of Electronic Commerce, 5 (3), 45–62. https://doi.org/10.1080/10864415.2001.11044215
Kim, K., & Ahn, H. (2008). A recommender system using GA K-means clustering in an online shopping market. Expert Systems with Applications, 34 (2), 1200–1209. https://doi.org/10.1016/j.eswa.2006.12.025
Kim, W., Kerschberg, L., & Scime, A. (2002). Learning for automatic personalization in a semantic taxonomy-based meta-search agent. Electronic Commerce Research and Applications, 1 (2), 150–173. https://doi.org/10.1016/S1567-4223(02)00011-X
Kim, Y. S., Yum, B. J., Song, J., & Kim, S. M. (2005b). Development of a recommender system based on navigational and behavioral patterns of customers in e-commerce sites. Expert Systems with Applications, 28 (2), 381–393. https://doi.org/10.1016/j.eswa.2004.10.017
Klaus, T., & Changchit, C. (2019). Toward an Understanding of Consumer Attitudes on Online Review Usage. Journal of Computer Information Systems, 59 (3), 277–286. https://doi.org/10.1080/08874417.2017.1348916
Knorr, E. M., Ng, R. T., & Tucakov, V. (2000). Distance-based outliers: Algorithms and applications. The VLDB Journal, 8 (3), 237–253. https://doi.org/10.1007/s007780050006
Kohavi, R., Longbotham, R., Sommerfield, D., & Henne, R. M. (2009). Controlled experiments on the web: Survey and practical guide. Data Mining and Knowledge Discovery, 18 (1), 140–181. https://doi.org/10.1007/s10618-008-0114-1
Konstan, J. A., Miller, B. N., Maltz, D., Herlocker, J. L., Gordon, L. R., & Riedl, J. (1997). Applying Collaborative Filtering to Usenet News. Communications of the ACM, 40 (3), 77–87. https://doi.org/10.1145/245108.245126
Koren, Y., Bell, R., & Volinsky, C. (2009). Matrix Factorization Techniques for Recommender Systems. Computer, 42 (8), 30–37. https://doi.org/10.1109/MC.2009.263
Kühl, N., Mühlthaler, M., & Goutier, M. (2020). Supporting customer-oriented marketing with artificial intelligence: Automatically quantifying customer needs from social media. Electronic Markets, 30 (2), 351–367. https://doi.org/10.1007/s12525-019-00351-0
Kumar, N., Venugopal, D., Qiu, L., & Kumar, S. (2018). Detecting Review Manipulation on Online Platforms with Hierarchical Supervised Learning. Journal of Management Information Systems, 35 (1), 350–380. https://doi.org/10.1080/07421222.2018.1440758
Kumar, N., Venugopal, D., Qiu, L., & Kumar, S. (2019a). Detecting Anomalous Online Reviewers: An Unsupervised Approach Using Mixture Models. Journal of Management Information Systems, 36 (4), 1313–1346. https://doi.org/10.1080/07421222.2019.1661089
Kumar, V., Rajan, B., Venkatesan, R., & Lecinski, J. (2019b). Understanding the role of artificial intelligence in personalized engagement marketing. California Management Review, 61 (4), 135–155. https://doi.org/10.1177/0008125619859317
Kuo, R. J., Chang, K., & Chien, S. Y. (2004). Integration of Self-Organizing Feature Maps and Genetic-Algorithm-Based Clustering Method for Market Segmentation. Journal of Organizational Computing and Electronic Commerce, 14 (1), 43–60. https://doi.org/10.1207/s15327744joce1401_3
Kuo, R. J., Liao, J. L., & Tu, C. (2005). Integration of ART2 neural network and genetic K-means algorithm for analyzing Web browsing paths in electronic commerce. Decision Support Systems, 40 (2), 355–374. https://doi.org/10.1016/j.dss.2004.04.010
Kwon, O., Yoo, K., & Suh, E. (2006). ubiES: Applying ubiquitous computing technologies to an expert system for context-aware proactive services. Electronic Commerce Research and Applications, 5 (3), 209–219. https://doi.org/10.1016/j.elerap.2005.10.011
Laorden, C., Santos, I., Sanz, B., Alvarez, G., & Bringas, P. G. (2012). Word sense disambiguation for spam filtering. Electronic Commerce Research and Applications, 11 (3), 290–298. https://doi.org/10.1016/j.elerap.2011.11.004
Lau, R. Y. K. (2007). Towards a web services and intelligent agents-based negotiation system for B2B eCommerce. Electronic Commerce Research and Applications, 6 (3), 260–273. https://doi.org/10.1016/j.elerap.2006.06.007
Law, R., Leung, R., & Buhalis, D. (2009). Information technology applications in hospitality and tourism: A review of publications from 2005 to 2007. Journal of Travel and Tourism Marketing, 26 (5–6), 599–623. https://doi.org/10.1080/10548400903163160
Lawrence, R. D., Almasi, G. S., Kotlyar, V., Viveros, M. S., & Duri, S. S. (2001). Personalization of supermarket product recommendations. In Data Mining and Knowledge Discovery (Vol. 5, Issues 1–2, pp. 11–32). Springer. https://doi.org/10.1023/A:1009835726774
LeCun, Y., Bengio, Y., & Hinton, G. (2015). Deep learning. Nature, 521 (7553), 436–444.
Lee, D., & Hosanagar, K. (2021). How do product attributes and reviews moderate the impact of recommender systems through purchase stages? Management Science, 67 (1), 524–546. https://doi.org/10.1287/mnsc.2019.3546
Lee, D., Gopal, A., & Park, S. H. (2020). Different but equal? a field experiment on the impact of recommendation systems on mobile and personal computer channels in retail. Information Systems Research, 31 (3), 892–912. https://doi.org/10.1287/ISRE.2020.0922
Lee, H.-C., Rim, H.-C., & Lee, D.-G. (2019). Learning to rank products based on online product reviews using a hierarchical deep neural network. Electronic Commerce Research and Applications, 36 , 100874. https://doi.org/10.1016/j.elerap.2019.100874
Lee, J., Podlaseck, M., Schonberg, E., & Hoch, R. (2001). Visualization and analysis of clickstream data of online stores for understanding web merchandising. Data Mining and Knowledge Discovery, 5 (1–2), 59–84. https://doi.org/10.1023/A:1009843912662
Lee, S. K., Cho, Y. H., & Kim, S. H. (2010). Collaborative filtering with ordinal scale-based implicit ratings for mobile music recommendations. Information Sciences, 180 (11), 2142–2155. https://doi.org/10.1016/j.ins.2010.02.004
Lee, S., & Kim, W. (2017). Sentiment labeling for extending initial labeled data to improve semi-supervised sentiment classification. Electronic Commerce Research and Applications, 26 , 35–49. https://doi.org/10.1016/j.elerap.2017.09.006
Lee, Y. H., Hu, P. J. H., Cheng, T. H., & Hsieh, Y. F. (2012). A cost-sensitive technique for positive-example learning supporting content-based product recommendations in B-to-C e-commerce. Decision Support Systems, 53 (1), 245–256. https://doi.org/10.1016/j.dss.2012.01.018
Leloup, B. (2003). Pricing with local interactions on agent-based electronic marketplaces. Electronic Commerce Research and Applications, 2 (2), 187–198. https://doi.org/10.1016/S1567-4223(03)00023-1
Lessmann, S., Haupt, J., Coussement, K., & De Bock, K. W. (2019). Targeting customers for profit: An ensemble learning framework to support marketing decision-making. Information Sciences . https://doi.org/10.1016/j.ins.2019.05.027
Li, H., Su, S. Y. W., & Lam, H. (2006). On automated e-business negotiations: Goal, policy, strategy, and plans of decision and action. Journal of Organizational Computing and Electronic Commerce, 16 (1), 1–29. https://doi.org/10.1080/10919390609540288
Li, J., Chen, C., Chen, H., & Tong, C. (2017). Towards Context-aware Social Recommendation via Individual Trust. Knowledge-Based Systems, 127 , 58–66. https://doi.org/10.1016/j.knosys.2017.02.032
Li, S., Zhang, Y., Yu, Z., Zhang, F., & Lu, H. (2019a). Predicting the influence of viral message for VM campaign on Weibo. Electronic Commerce Research and Applications, 36 , 100875. https://doi.org/10.1016/j.elerap.2019.100875
Li, X., Wu, C., & Mai, F. (2019b). The effect of online reviews on product sales: A joint sentiment-topic analysis. Information & Management, 56 (2), 172–184. https://doi.org/10.1016/j.im.2018.04.007
Li, Y.-M., Chou, C.-L., & Lin, L.-F. (2014). A social recommender mechanism for location-based group commerce. Information Sciences, 274 , 125–142. https://doi.org/10.1016/j.ins.2014.02.079
Li, Y. M., Wu, C. T., & Lai, C. Y. (2013). A social recommender mechanism for e-commerce: Combining similarity, trust, and relationship. Decision Support Systems, 55 (3), 740–752. https://doi.org/10.1016/j.dss.2013.02.009
Li, Y., Wang, S., Pan, Q., Peng, H., Yang, T., & Cambria, E. (2019c). Learning binary codes with neural collaborative filtering for efficient recommendation systems. Knowledge-Based Systems, 172 , 64–75. https://doi.org/10.1016/j.knosys.2019.02.012
Li, Yu., Lu, L., & Xuefeng, L. (2005). A hybrid collaborative filtering method for multiple-interests and multiple-content recommendation in E-Commerce. Expert Systems with Applications, 28 (1), 67–77. https://doi.org/10.1016/j.eswa.2004.08.013
Liang, R., Wang, J., & qiang. (2019). A Linguistic Intuitionistic Cloud Decision Support Model with Sentiment Analysis for Product Selection in E-commerce. International Journal of Fuzzy Systems, 21 (3), 963–977. https://doi.org/10.1007/s40815-019-00606-0
Liebman, E., Saar-Tsechansky, M., & Stone, P. (2019). The right music at the right time: Adaptive personalized playlists based on sequence modeling. MIS Quarterly, 43 (3), 765–786. https://doi.org/10.25300/MISQ/2019/14750
Lin, Q.-Y., Chen, Y.-L., Chen, J.-S., & Chen, Y.-C. (2003). Mining inter-organizational retailing knowledge for an alliance formed by competitive firms. Information & Management, 40 (5), 431–442. https://doi.org/10.1016/S0378-7206(02)00062-9
Lin, W., Alvarez, S. A., & Ruiz, C. (2002). Efficient Adaptive-Support Association Rule Mining for Recommender Systems. Data Mining and Knowledge Discovery, 6 (1), 83–105. https://doi.org/10.1023/A:1013284820704
Lin, W. H., Wang, P., & Tsai, C. F. (2016). Face recognition using support vector model classifier for user authentication. Electronic Commerce Research and Applications, 18 , 71–82. https://doi.org/10.1016/j.elerap.2016.01.005
Linden, G., Smith, B., & York, J. (2003). Amazon.com recommendations: item-to-item collaborative filtering. IEEE Internet Computing, 7 (1), 76–80. https://doi.org/10.1109/MIC.2003.1167344
Liu, B., Hu, M., & Cheng, J. (2005). Opinion observer. Proceedings of the 14th International Conference on World Wide Web , 342. https://doi.org/10.1145/1060745.1060797
Liu, D.-R., Chen, K.-Y., Chou, Y.-C., & Lee, J.-H. (2018). Online recommendations based on dynamic adjustment of recommendation lists. Knowledge-Based Systems, 161 , 375–389. https://doi.org/10.1016/j.knosys.2018.07.038
Liu, H., Jiang, Z., Song, Y., Zhang, T., & Wu, Z. (2019). User preference modeling based on meta paths and diversity regularization in heterogeneous information networks. Knowledge-Based Systems, 181 , 104784. https://doi.org/10.1016/j.knosys.2019.05.027
Liu, K., Zeng, X., Bruniaux, P., Wang, J., Kamalha, E., & Tao, X. (2017). Fit evaluation of virtual garment try-on by learning from digital pressure data. Knowledge-Based Systems, 133 , 174–182. https://doi.org/10.1016/j.knosys.2017.07.007
Liu, N., & Shen, B. (2020). Aspect-based sentiment analysis with gated alternate neural network. Knowledge-Based Systems, 188 , 105010. https://doi.org/10.1016/j.knosys.2019.105010
Liu, R., Mai, F., Shan, Z., & Wu, Y. (2020). Predicting shareholder litigation on insider trading from financial text: An interpretable deep learning approach. Information & Management, 57 (8), 103387. https://doi.org/10.1016/j.im.2020.103387
Liu, X., Datta, A., & Rzadca, K. (2013). Trust beyond reputation: A computational trust model based on stereotypes. Electronic Commerce Research and Applications, 12 (1), 24–39. https://doi.org/10.1016/j.elerap.2012.07.001
Lowry, P. B., Moody, G. D., Gaskin, J., Galletta, D. F., Humpherys, S. L., Barlow, J. B., & Wilson, D. W. (2013). Evaluating journal quality and the association for information systems senior scholars’ journal basket via bibliometric measures: Do expert journal assessments add value? MIS Quarterly, 37 (4), 993–1012. https://doi.org/10.25300/MISQ/2013/37.4.01
Lowry, P., Romans, D., & Curtis, A. (2004). Global Journal Prestige and Supporting Disciplines: A Scientometric Study of Information Systems Journals. Journal of the Association for Information Systems, 5 (2), 29–77. https://doi.org/10.17705/1jais.00045
Lu, J., Wu, D., Mao, M., Wang, W., & Zhang, G. (2015). Recommender system application developments: A survey. Decision Support Systems, 74 , 12–32. https://doi.org/10.1016/j.dss.2015.03.008
Luo, X., Lu, X., & Li, J. (2019). When and How to Leverage E-commerce Cart Targeting: The relative and moderated effects of scarcity and price incentives with a two-stage field experiment and causal forest optimization. Information Systems Research, 30 (4), 1203–1227. https://doi.org/10.1287/isre.2019.0859
Lyytinen, K., Nickerson, J. V, & King, J. L. (2020). Metahuman systems = humans + machines that learn. Journal of Information Technology , 36 (4), 427–445. https://doi.org/10.1177/0268396220915917
Ma, X., Sha, J., Wang, D., Yu, Y., Yang, Q., & Niu, X. (2018). Study on a prediction of P2P network loan default based on the machine learning LightGBM and XGboost algorithms according to different high dimensional data cleaning. Electronic Commerce Research and Applications, 31 , 24–39. https://doi.org/10.1016/j.elerap.2018.08.002
Ma, Z., Pant, G., & Sheng, O. R. L. (2011). Mining competitor relationships from online news: A network-based approach. Electronic Commerce Research and Applications, 10 (4), 418–427. https://doi.org/10.1016/j.elerap.2010.11.006
Makridakis, S. (2017). The forthcoming Artificial Intelligence (AI) revolution: Its impact on society and firms. Futures, 90 , 46–60. https://doi.org/10.1016/j.futures.2017.03.006
Manahov, V., & Zhang, H. (2019). Forecasting Financial Markets Using High-Frequency Trading Data: Examination with Strongly Typed Genetic Programming. International Journal of Electronic Commerce, 23 (1), 12–32. https://doi.org/10.1080/10864415.2018.1512271
Manthiou, A., Klaus, P., Kuppelwieser, V. G., & Reeves, W. (2021). Man vs machine: Examining the three themes of service robotics in tourism and hospitality. Electronic Markets, 31 (3), 511–527. https://doi.org/10.1007/s12525-020-00434-3
Manvi, S. S., & Venkataram, P. (2005). An intelligent product-information presentation in E-commerce. Electronic Commerce Research and Applications, 4 (3), 220–239. https://doi.org/10.1016/j.elerap.2005.01.001
Mao, M., Lu, J., Han, J., & Zhang, G. (2019). Multiobjective e-commerce recommendations based on hypergraph ranking. Information Sciences, 471 , 269–287. https://doi.org/10.1016/j.ins.2018.07.029
Maqsood, H., Mehmood, I., Maqsood, M., Yasir, M., Afzal, S., Aadil, F., Selim, M. M., & Muhammad, K. (2020). A local and global event sentiment based efficient stock exchange forecasting using deep learning. International Journal of Information Management, 50 , 432–451. https://doi.org/10.1016/j.ijinfomgt.2019.07.011
Marabelli, M., Newell, S., & Handunge, V. (2021). The lifecycle of algorithmic decision-making systems: Organizational choices and ethical challenges. The Journal of Strategic Information Systems, 30 (3), 101683. https://doi.org/10.1016/j.jsis.2021.101683
Martens, D., & Provost, F. (2014). Explaining data-driven document classifications. MIS Quarterly, 38 (1), 73–99. https://doi.org/10.25300/MISQ/2014/38.1.04
Martinez-Cruz, C., Porcel, C., Bernabé-Moreno, J., & Herrera-Viedma, E. (2015). A model to represent users trust in recommender systems using ontologies and fuzzy linguistic modeling. Information Sciences, 311 , 102–118. https://doi.org/10.1016/j.ins.2015.03.013
Marx, W., Bornmann, L., Barth, A., & Leydesdorff, L. (2014). Detecting the historical roots of research fields by reference publication year spectroscopy (RPYS). Journal of the Association for Information Science and Technology, 65 (4), 751–764. https://doi.org/10.1002/asi.23089
McAuley, J., Targett, C., Shi, Q., & Van Den Hengel, A. (2015). Image-based recommendations on styles and substitutes. SIGIR 2015 - Proceedings of the 38th International ACM SIGIR Conference on Research and Development in Information Retrieval , 43–52. https://doi.org/10.1145/2766462.2767755
Milian, E. Z., de Spinola, M., & M., & Carvalho, M. M. d. (2019). Fintechs: A literature review and research agenda. Electronic Commerce Research and Applications, 34 , 100833. https://doi.org/10.1016/j.elerap.2019.100833
Miralles-Pechuán, L., Ponce, H., & Martínez-Villaseñor, L. (2018). A novel methodology for optimizing display advertising campaigns using genetic algorithms. Electronic Commerce Research and Applications, 27 , 39–51. https://doi.org/10.1016/j.elerap.2017.11.004
Mo, J., Sarkar, S., & Menon, S. (2018). Know when to run: Recommendations in crowdsourcing contests. MIS Quarterly, 42 (3), 919–943. https://doi.org/10.25300/MISQ/2018/14103
Mokryn, O., Bogina, V., & Kuflik, T. (2019). Will this session end with a purchase? Inferring current purchase intent of anonymous visitors. Electronic Commerce Research and Applications, 34 , 100836. https://doi.org/10.1016/j.elerap.2019.100836
Motiwalla, L. F., & Nunamaker, J. F. (1992). Mail-man: A knowledge-based mail assistant for managers. Journal of Organizational Computing, 2 (2), 131–154. https://doi.org/10.1080/10919399209540179
Moussawi, S., Koufaris, M., & Benbunan-Fich, R. (2020). How perceptions of intelligence and anthropomorphism affect adoption of personal intelligent agents. Electronic Markets . https://doi.org/10.1007/s12525-020-00411-w
Nassiri-Mofakham, F., Nematbakhsh, M. A., Baraani-Dastjerdi, A., & Ghasem-Aghaee, N. (2009). Electronic promotion to new customers using mkNN learning. Information Sciences, 179 (3), 248–266. https://doi.org/10.1016/j.ins.2008.09.019
Neuhofer, B., Magnus, B., & Celuch, K. (2021). The impact of artificial intelligence on event experiences: A scenario technique approach. Electronic Markets, 31 (3), 601–617. https://doi.org/10.1007/s12525-020-00433-4
Ngai, E. W. T., & Wat, F. K. T. (2002). A literature review and classification of electronic commerce research. Information and Management, 39 (5), 415–429. https://doi.org/10.1016/S0378-7206(01)00107-0
Ngai, E. W. T., Lee, M. C. M., Luo, M., Chan, P. S. L., & Liang, T. (2021). An intelligent knowledge-based chatbot for customer service. Electronic Commerce Research and Applications, 50 , 101098. https://doi.org/10.1016/j.elerap.2021.101098
Nikolay, A., Anindya, G., & Panagiotis, G. I. (2011). Deriving the pricing power of product features by mining consumer reviews. Management Science, 57 (8), 1485–1509. https://doi.org/10.1287/mnsc.1110.1370
Nilashi, M., & bin Ibrahim, O., Ithnin, N., & Sarmin, N. H. (2015). A multi-criteria collaborative filtering recommender system for the tourism domain using Expectation Maximization (EM) and PCA–ANFIS. Electronic Commerce Research and Applications, 14 (6), 542–562. https://doi.org/10.1016/j.elerap.2015.08.004
Nishimura, N., Sukegawa, N., Takano, Y., & Iwanaga, J. (2018). A latent-class model for estimating product-choice probabilities from clickstream data. Information Sciences, 429 , 406–420. https://doi.org/10.1016/j.ins.2017.11.014
Núñez-Valdez, E. R., Quintana, D., González Crespo, R., Isasi, P., & Herrera-Viedma, E. (2018). A recommender system based on implicit feedback for selective dissemination of ebooks. Information Sciences, 467 , 87–98. https://doi.org/10.1016/j.ins.2018.07.068
O’Donovan, J., & Smyth, B. (2005). Trust in recommender systems. International Conference on Intelligent User Interfaces, Proceedings IUI , 167–174. https://doi.org/10.1145/1040830.1040870
O’Neil, S., Zhao, X., Sun, D., & Wei, J. C. (2016). Newsvendor Problems with Demand Shocks and Unknown Demand Distributions. Decision Sciences, 47 (1), 125–156. https://doi.org/10.1111/deci.12187
Oliver, J. R. (1996). A Machine-Learning Approach to Automated Negotiation and Prospects for Electronic Commerce. Journal of Management Information Systems, 13 (3), 83–112. https://doi.org/10.1080/07421222.1996.11518135
Ortega, F., Hernando, A., Bobadilla, J., & Kang, J. H. (2016). Recommending items to group of users using Matrix Factorization based Collaborative Filtering. Information Sciences, 345 , 313–324. https://doi.org/10.1016/j.ins.2016.01.083
Ortega, F., Sánchez, J. L., Bobadilla, J., & Gutiérrez, A. (2013). Improving collaborative filtering-based recommender systems results using Pareto dominance. Information Sciences, 239 , 50–61. https://doi.org/10.1016/j.ins.2013.03.011
Ou, W., Huynh, V.-N., & Sriboonchitta, S. (2018). Training attractive attribute classifiers based on opinion features extracted from review data. Electronic Commerce Research and Applications, 32 , 13–22. https://doi.org/10.1016/j.elerap.2018.10.003
Padmanabhan, B., & Tuzhilin, A. (2003). On the use of optimization for data mining: Theoretical interactions and eCRM opportunities. Management Science, 49 (10), 1327–1343. https://doi.org/10.1287/mnsc.49.10.1327.17310
Pang, G., Wang, X., Hao, F., Xie, J., Wang, X., Lin, Y., & Qin, X. (2019). ACNN-FM: A novel recommender with attention-based convolutional neural network and factorization machines. Knowledge-Based Systems, 181 , 104786. https://doi.org/10.1016/j.knosys.2019.05.029
Pantano, E., & Pizzi, G. (2020). Forecasting artificial intelligence on online customer assistance: Evidence from chatbot patents analysis. Journal of Retailing and Consumer Services, 55 , 102096. https://doi.org/10.1016/j.jretconser.2020.102096
Paré, G., Trudel, M. C., Jaana, M., & Kitsiou, S. (2015). Synthesizing information systems knowledge: A typology of literature reviews. Information and Management, 52 (2), 183–199. https://doi.org/10.1016/j.im.2014.08.008
Park, C., Kim, D., Yang, M. C., Lee, J. T., & Yu, H. (2020a). Click-aware purchase prediction with push at the top. Information Sciences, 521 , 350–364. https://doi.org/10.1016/j.ins.2020.02.062
Park, C., Kim, D., & Yu, H. (2019). An encoder–decoder switch network for purchase prediction. Knowledge-Based Systems, 185 , 104932. https://doi.org/10.1016/j.knosys.2019.104932
Park, H., Song, M., & Shin, K.-S. (2020b). Deep learning models and datasets for aspect term sentiment classification: Implementing holistic recurrent attention on target-dependent memories. Knowledge-Based Systems, 187 , 104825. https://doi.org/10.1016/j.knosys.2019.06.033
Park, J. H., & Park, S. C. (2003). Agent-based merchandise management in business-to-business electronic commerce. Decision Support Systems, 35 (3), 311–333. https://doi.org/10.1016/S0167-9236(02)00111-2
Parvin, H., Moradi, P., Esmaeili, S., & Qader, N. N. (2019). A scalable and robust trust-based nonnegative matrix factorization recommender using the alternating direction method. Knowledge-Based Systems, 166 , 92–107. https://doi.org/10.1016/j.knosys.2018.12.016
Patcha, A., & Park, J.-M. (2007). An overview of anomaly detection techniques: Existing solutions and latest technological trends. Computer Networks, 51 (12), 3448–3470. https://doi.org/10.1016/j.comnet.2007.02.001
Patra, B. K., Launonen, R., Ollikainen, V., & Nandi, S. (2015). A new similarity measure using Bhattacharyya coefficient for collaborative filtering in sparse data. Knowledge-Based Systems, 82 , 163–177. https://doi.org/10.1016/j.knosys.2015.03.001
Pedregosa, F., Varoquaux, G., Gramfort, A., Michel, V., Thirion, B., Grisel, O., Blondel, M., Prettenhofer, P., Weiss, R., Dubourg, V., Vanderplas, J., Passos, A., Cournapeau, D., Brucher, M., Perrot, M., & Duchesnay, É. (2011). Scikit-learn: Machine learning in Python. Journal of Machine Learning Research, 12 , 2825–2830.
Pendharkar, P. C. (2006). Inductive Regression Tree and Genetic Programming Techniques for Learning User Web Search Preferences. Journal of Organizational Computing and Electronic Commerce, 16 (3–4), 223–245. https://doi.org/10.1080/10919392.2006.9681201
Pengnate (Fone), S., & Riggins, F. J. (2020). The role of emotion in P2P microfinance funding: A sentiment analysis approach. International Journal of Information Management, 54 , 102138. https://doi.org/10.1016/j.ijinfomgt.2020.102138
Pfeiffer, J., Pfeiffer, T., Meißner, M., & Weiß, E. (2020). Eye-tracking-based classification of information search behavior using machine learning: Evidence from experiments in physical shops and virtual reality shopping environments. Information Systems Research, 31 (3), 675–691. https://doi.org/10.1287/ISRE.2019.0907
Pontelli, E., & Son, T. C. (2003). Designing intelligent agents to support universal accessibility of E-commerce services. Electronic Commerce Research and Applications, 2 (2), 147–161. https://doi.org/10.1016/S1567-4223(03)00012-7
Pourgholamali, F., Kahani, M., & Bagheri, E. (2020). A neural graph embedding approach for selecting review sentences. Electronic Commerce Research and Applications, 40 , 100917. https://doi.org/10.1016/j.elerap.2019.100917
Pourkhani, A., Abdipour, K., Baher, B., & Moslehpour, M. (2019). The impact of social media in business growth and performance: A scientometrics analysis. International Journal of Data and Network Science, 3 (3), 223–244. https://doi.org/10.5267/j.ijdns.2019.2.003
Praet, S., & Martens, D. (2020). Efficient Parcel Delivery by Predicting Customers’ Locations*. Decision Sciences, 51 (5), 1202–1231. https://doi.org/10.1111/deci.12376
Pranata, I., & Susilo, W. (2016). Are the most popular users always trustworthy? The case of Yelp. Electronic Commerce Research and Applications, 20 , 30–41. https://doi.org/10.1016/j.elerap.2016.09.005
Preibusch, S., Peetz, T., Acar, G., & Berendt, B. (2016). Shopping for privacy: Purchase details leaked to PayPal. Electronic Commerce Research and Applications, 15 , 52–64. https://doi.org/10.1016/j.elerap.2015.11.004
Pröllochs, N., Feuerriegel, S., Lutz, B., & Neumann, D. (2020). Negation scope detection for sentiment analysis: A reinforcement learning framework for replicating human interpretations. Information Sciences, 536 , 205–221. https://doi.org/10.1016/j.ins.2020.05.022
Pu, P., & Chen, L. (2007). Trust-inspiring explanation interfaces for recommender systems. Knowledge-Based Systems, 20 (6), 542–556. https://doi.org/10.1016/j.knosys.2007.04.004
Pujahari, A., & Sisodia, D. S. (2019). Modeling Side Information in Preference Relation based Restricted Boltzmann Machine for recommender systems. Information Sciences, 490 , 126–145. https://doi.org/10.1016/j.ins.2019.03.064
Qi, J., Zhang, Z., Jeon, S., & Zhou, Y. (2016). Mining customer requirements from online reviews: A product improvement perspective. Information and Management, 53 (8), 951–963. https://doi.org/10.1016/j.im.2016.06.002
Qiu, J., Liu, C., Li, Y., & Lin, Z. (2018). Leveraging sentiment analysis at the aspects level to predict ratings of reviews. Information Sciences, 451–452 , 295–309. https://doi.org/10.1016/j.ins.2018.04.009
Rahm, E., & Bernstein, P. A. (2001). A survey of approaches to automatic schema matching. VLDB Journal, 10 (4), 334–350. https://doi.org/10.1007/s007780100057
Ranjbar Kermany, N., & Alizadeh, S. H. (2017). A hybrid multi-criteria recommender system using ontology and neuro-fuzzy techniques. Electronic Commerce Research and Applications, 21 , 50–64. https://doi.org/10.1016/j.elerap.2016.12.005
Rao, Y., Xie, H., Li, J., Jin, F., Wang, F. L., & Li, Q. (2016). Social emotion classification of short text via topic-level maximum entropy model. Information and Management, 53 (8), 978–986. https://doi.org/10.1016/j.im.2016.04.005
Ravi, K., & Ravi, V. (2015). A survey on opinion mining and sentiment analysis: Tasks, approaches and applications. Knowledge-Based Systems, 89 , 14–46. https://doi.org/10.1016/j.knosys.2015.06.015
Resnick, P., Iacovou, N., Suchak, M., Bergstrom, P., & Riedl, J. (1994). GroupLens: An open architecture for collaborative filtering of netnews. Proceedings of the 1994 ACM Conference on Computer Supported Cooperative Work, CSCW 1994 , 175–186. https://doi.org/10.1145/192844.192905
Resnick, P., & Varian, H. R. (1997). Recommender Systems. Communications of the ACM, 40 (3), 56–58. https://doi.org/10.1145/245108.245121
Rhaiem, M., & Bornmann, L. (2018). Reference Publication Year Spectroscopy (RPYS) with publications in the area of academic efficiency studies: What are the historical roots of this research topic? Applied Economics, 50 (13), 1442–1453. https://doi.org/10.1080/00036846.2017.1363865
Ricci, F., Rokach, L., & Shapira, B. (2011). Introduction to recommender systems handbook. In Recommender systems handbook (pp. 1–35). Springer.
Ryoba, M. J., Qu, S., & Zhou, Y. (2021). Feature subset selection for predicting the success of crowdfunding project campaigns. Electronic Markets, 31 (3), 671–684. https://doi.org/10.1007/s12525-020-00398-4
Sabater, J., & Sierra, C. (2005). Review on computational trust and reputation models. Artificial Intelligence Review, 24 (1), 33–60. https://doi.org/10.1007/s10462-004-0041-5
Salakhutdinov, R., Mnih, A., & Hinton, G. (2007). Restricted Boltzmann machines for collaborative filtering. ACM International Conference Proceeding Series, 227 , 791–798. https://doi.org/10.1145/1273496.1273596
Saleh, A. I., El Desouky, A. I., & Ali, S. H. (2015). Promoting the performance of vertical recommendation systems by applying new classification techniques. Knowledge-Based Systems, 75 , 192–223. https://doi.org/10.1016/j.knosys.2014.12.002
Sarwar, B., Karypis, G., Konstan, J., & Riedl, J. (2001). Item-based collaborative filtering recommendation algorithms. Proceedings of the 10th International Conference on World Wide Web, WWW 2001 , 285–295. https://doi.org/10.1145/371920.372071
Saumya, S., Singh, J. P., Baabdullah, A. M., Rana, N. P., & Dwivedi, Y. K. (2018). Ranking online consumer reviews. Electronic Commerce Research and Applications, 29 , 78–89. https://doi.org/10.1016/j.elerap.2018.03.008
Schafer, J. B., Konstan, J. A., & Riedl, J. (2001). E-Commerce Recommendation Applications. Data Mining and Knowledge Discovery, 5 (1), 115–153. https://doi.org/10.1023/A:1009804230409
Schmidhuber, J. (2015). Deep Learning in neural networks: An overview. Neural Networks, 61 , 85–117. https://doi.org/10.1016/j.neunet.2014.09.003
Shani, G., Heckerman, D., & Brafman, R. I. (2005). An MDP-based recommender system. Journal of Machine Learning Research, 6 (Sep), 1265–1295.
Shardanand, U., & Maes, P. (1995). Social information filtering. Proceedings of the SIGCHI Conference on Human Factors in Computing Systems , 210–217. https://doi.org/10.1145/223904.223931
Shi, Y., Wang, T., & Alwan, L. C. (2020). Analytics for Cross-Border E-Commerce: Inventory Risk Management of an Online Fashion Retailer. Decision Sciences, 51 (6), 1347–1376. https://doi.org/10.1111/deci.12429
Si, Y., Zhang, F., & Liu, W. (2017). CTF-ARA: An adaptive method for POI recommendation based on check-in and temporal features. Knowledge-Based Systems, 128 , 59–70. https://doi.org/10.1016/j.knosys.2017.04.013
Singh, A., & Tucker, C. S. (2017). A machine learning approach to product review disambiguation based on function, form and behavior classification. Decision Support Systems, 97 , 81–91. https://doi.org/10.1016/j.dss.2017.03.007
Sohn, K., & Kwon, O. (2020). Technology acceptance theories and factors influencing artificial Intelligence-based intelligent products. Telematics and Informatics, 47 , 101324. https://doi.org/10.1016/j.tele.2019.101324
Song, S., Hwang, K., Zhou, R., & Kwok, Y. K. (2005). Trusted P2P transactions with fuzzy reputation aggregation. IEEE Internet Computing, 9 (6), 24–34. https://doi.org/10.1109/MIC.2005.136
Stöckli, D. R., & Khobzi, H. (2021). Recommendation systems and convergence of online reviews: The type of product network matters! Decision Support Systems, 142 , 113475. https://doi.org/10.1016/j.dss.2020.113475
Stoeckli, E., Dremel, C., Uebernickel, F., & Brenner, W. (2020). How affordances of chatbots cross the chasm between social and traditional enterprise systems. Electronic Markets, 30 (2), 369–403. https://doi.org/10.1007/s12525-019-00359-6
Su, X., & Khoshgoftaar, T. M. (2009). A Survey of Collaborative Filtering Techniques. Advances in Artificial Intelligence, 2009 , 1–19. https://doi.org/10.1155/2009/421425
Suchacka, G., & Iwański, J. (2020). Identifying legitimate Web users and bots with different traffic profiles — an Information Bottleneck approach. Knowledge-Based Systems, 197 , 105875. https://doi.org/10.1016/j.knosys.2020.105875
Sul, H. K., Dennis, A. R., & Yuan, L. I. (2017). Trading on Twitter: Using Social Media Sentiment to Predict Stock Returns. Decision Sciences, 48 (3), 454–488. https://doi.org/10.1111/deci.12229
Sun, Y., Liu, X., Chen, G., Hao, Y., & Zhang (Justin), Z. (2020). How mood affects the stock market: Empirical evidence from microblogs. Information & Management, 57 (5), 103181. https://doi.org/10.1016/j.im.2019.103181
Sung (Christine), E., Bae, S., Han, D.-I.D., & Kwon, O. (2021). Consumer engagement via interactive artificial intelligence and mixed reality. International Journal of Information Management, 60 , 102382. https://doi.org/10.1016/j.ijinfomgt.2021.102382
Szegedy, C., Liu, W., Jia, Y., Sermanet, P., Reed, S., Anguelov, D., Erhan, D., Vanhoucke, V., & Rabinovich, A. (2015). Going deeper with convolutions. IEEE Conference on Computer Vision and Pattern Recognition (CVPR), 2015 , 1–9. https://doi.org/10.1109/CVPR.2015.7298594
Takeuchi, H., Subramaniam, L. V., Nasukawa, T., & Roy, S. (2009). Getting insights from the voices of customers: Conversation mining at a contact center. Information Sciences, 179 (11), 1584–1591. https://doi.org/10.1016/j.ins.2008.11.026
Tan, F. T. C., Pan, S. L., & Zuo, M. (2019). Realising platform operational agility through information technology–enabled capabilities: A resource-interdependence perspective. Information Systems Journal, 29 (3), 582–608. https://doi.org/10.0487/isj.12221
Tan, J., Tyler, K., & Manica, A. (2007). Business-to-business adoption of eCommerce in China. Information & Management, 44 (3), 332–351. https://doi.org/10.1016/j.im.2007.04.001
Tan, P.-N., & Kumar, V. (2002). Discovery of Web Robot Sessions Based on their Navigational Patterns. Data Mining and Knowledge Discovery, 6 (1), 9–35. https://doi.org/10.1023/A:1013228602957
Tan, Y.-H., & Thoen, W. (2000). INCAS: A legal expert system for contract terms in electronic commerce. Decision Support Systems, 29 (4), 389–411. https://doi.org/10.1016/S0167-9236(00)00085-3
Tang, P., Qiu, W., Huang, Z., Chen, S., Yan, M., Lian, H., & Li, Z. (2020). Anomaly detection in electronic invoice systems based on machine learning. Information Sciences, 535 , 172–186. https://doi.org/10.1016/j.ins.2020.03.089
Templier, M., & Paré, G. (2015). A framework for guiding and evaluating literature reviews. Communications of the Association for Information Systems, 37 (1), 112–137. https://doi.org/10.17705/1CAIS.03706
Thiebes, S., Lins, S., & Sunyaev, A. (2021). Trustworthy artificial intelligence. Electronic Markets, 31 (2). https://doi.org/10.1007/s12525-020-00441-4
Tian, F., Wu, F., Chao, K. M., Zheng, Q., Shah, N., Lan, T., & Yue, J. (2016). A topic sentence-based instance transfer method for imbalanced sentiment classification of Chinese product reviews. Electronic Commerce Research and Applications, 16 , 66–76. https://doi.org/10.1016/j.elerap.2015.10.003
Tran, B., Vu, G., Ha, G., Vuong, Q.-H., Ho, M.-T., Vuong, T.-T., La, V.-P., Ho, M.-T., Nghiem, K.-C., Nguyen, H., Latkin, C., Tam, W., Cheung, N.-M., Nguyen, H.-K., Ho, C., & Ho, R. (2019). Global Evolution of Research in Artificial Intelligence in Health and Medicine: A Bibliometric Study. Journal of Clinical Medicine, 8 (3), 360. https://doi.org/10.3390/jcm8030360
Tseng, K.-K., Lin, R.F.-Y., Zhou, H., Kurniajaya, K. J., & Li, Q. (2018). Price prediction of e-commerce products through Internet sentiment analysis. Electronic Commerce Research, 18 (1), 65–88. https://doi.org/10.1007/s10660-017-9272-9
Vanneschi, L., Horn, D. M., Castelli, M., & Popovič, A. (2018). An artificial intelligence system for predicting customer default in e-commerce. Expert Systems with Applications, 104 , 1–21. https://doi.org/10.1016/j.eswa.2018.03.025
Varshney, D., Kumar, S., & Gupta, V. (2017). Predicting information diffusion probabilities in social networks: A Bayesian networks based approach. Knowledge-Based Systems, 133 , 66–76. https://doi.org/10.1016/j.knosys.2017.07.003
Viejo, A., Sánchez, D., & Castellà-Roca, J. (2012). Preventing automatic user profiling in Web 2.0 applications. Knowledge-Based Systems, 36 , 191–205. https://doi.org/10.1016/j.knosys.2012.07.001
Villegas, N. M., Sánchez, C., Díaz-Cely, J., & Tamura, G. (2018). Characterizing context-aware recommender systems: A systematic literature review. Knowledge-Based Systems, 140 , 173–200. https://doi.org/10.1016/j.knosys.2017.11.003
Viswanathan, S., Guillot, F., & Grasso, A. M. (2020). What is natural?: Challenges and opportunities for conversational recommender systems. ACM International Conference Proceeding Series , 1–4. https://doi.org/10.1145/3405755.3406174
Vizine Pereira, A. L., & Hruschka, E. R. (2015). Simultaneous co-clustering and learning to address the cold start problem in recommender systems. Knowledge-Based Systems, 82 , 11–19. https://doi.org/10.1016/j.knosys.2015.02.016
Vozalis, M. G., & Margaritis, K. G. (2007). Using SVD and demographic data for the enhancement of generalized Collaborative Filtering. Information Sciences, 177 (15), 3017–3037. https://doi.org/10.1016/j.ins.2007.02.036
Wang, F.-H. (2008). On discovery of soft associations with “most” fuzzy quantifier for item promotion applications. Information Sciences, 178 (7), 1848–1876. https://doi.org/10.1016/j.ins.2007.11.018
Wang, G., Ma, J., Huang, L., & Xu, K. (2012). Two credit scoring models based on dual strategy ensemble trees. Knowledge-Based Systems, 26 , 61–68. https://doi.org/10.1016/j.knosys.2011.06.020
Wang, H.-C., Jhou, H.-T., & Tsai, Y.-S. (2018a). Adapting topic map and social influence to the personalized hybrid recommender system. Information Sciences . https://doi.org/10.1016/j.ins.2018.04.015
Wang, H. C., & Doong, H. S. (2010). Argument form and spokesperson type: The recommendation strategy of virtual salespersons. International Journal of Information Management, 30 (6), 493–501. https://doi.org/10.1016/j.ijinfomgt.2010.03.006
Wang, H., Wang, N., & Yeung, D. Y. (2015). Collaborative deep learning for recommender systems. Proceedings of the ACM SIGKDD International Conference on Knowledge Discovery and Data Mining , 2015 - Augus , 1235–1244. https://doi.org/10.1145/2783258.2783273
Wang, Q., Li, B., & Singh, P. V. (2018). Copycats vs. original mobile apps: A machine learning copycat-detection method and empirical analysis. Information Systems Research, 29 (2), 273–291. https://doi.org/10.1287/isre.2017.0735
Wang, W., Feng, Y., & Dai, W. (2018c). Topic analysis of online reviews for two competitive products using latent Dirichlet allocation. Electronic Commerce Research and Applications, 29 , 142–156. https://doi.org/10.1016/j.elerap.2018.04.003
Wang, Y., Lu, X., & Tan, Y. (2018d). Impact of product attributes on customer satisfaction: An analysis of online reviews for washing machines. Electronic Commerce Research and Applications, 29 , 1–11. https://doi.org/10.1016/j.elerap.2018.03.003
Wareham, J., Zheng, J. G., & Straub, D. (2005). Critical themes in electronic commerce research: A meta-analysis. Journal of Information Technology, 20 (1), 1–19. https://doi.org/10.1057/palgrave.jit.2000034
Watson, G. R., & Rasmussen, C. E. (2008). An integrated environment for the development of parallel applications. Proceedings of the 2nd International Workshop on Parallel Tools for High Performance Computing , 11 (2), 19–34. https://doi.org/10.1007/978-3-540-68564-7
Webster, J., & Watson, R. T. (2002). Analyzing the Past to Prepare for the Future: Writing a Literature Review. MIS Quarterly , 26 (2), xiii–xxiii. 10.1.1.104.6570
Wei, C. P., Hu, P. J., & Dong, Y. X. (2002). Managing document categories in e-commerce environments: An evolution-based approach. European Journal of Information Systems, 11 (3), 208–222. https://doi.org/10.1057/palgrave.ejis.3000429
Wei, J., He, J., Chen, K., Zhou, Y., & Tang, Z. (2017). Collaborative filtering and deep learning based recommendation system for cold start items. Expert Systems with Applications, 69 , 29–39. https://doi.org/10.1016/j.eswa.2016.09.040
Wenxuan Ding, A., Li, S., & Chatterjee, P. (2015). Learning User Real-Time Intent for Optimal Dynamic Web Page Transformation. Information Systems Research, 26 (2), 339–359. https://doi.org/10.1057/isre.2015.0568
Willcocks, L. (2020a). Robo-Apocalypse cancelled? Reframing the automation and future of work debate. Journal of Information Technology, 35 (4), 286–302. https://doi.org/10.1177/0268396220925830
Willcocks, L. (2020b). Robo-Apocalypse? Response and outlook on the post-COVID-19 future of work. Journal of Information Technology, 36 (2), 188–194. https://doi.org/10.1177/0268396220978660
Witten, I. H., Frank, E., Hall, M. A., & Pal, C. J. (2016). Data Mining: Practical Machine Learning Tools and Techniques. In Data Mining: Practical Machine Learning Tools and Techniques . Morgan Kaufmann. https://doi.org/10.1016/c2009-0-19715-5
Wu, B., Ye, Y., & Chen, Y. (2019). Visual appearance or functional complementarity: Which aspect affects your decision making? Information Sciences, 476 , 19–37. https://doi.org/10.1016/j.ins.2018.10.011
Wu, J., Huang, L., & Zhao, J. L. (2019). Operationalizing regulatory focus in the digital age: Evidence from an e-commerce context. MIS Quarterly, 43 (3), 745–764. https://doi.org/10.25300/MISQ/2019/14420
Wu, R. S., & Chou, P. H. (2011). Customer segmentation of multiple category data in e-commerce using a soft-clustering approach. Electronic Commerce Research and Applications, 10 (3), 331–341. https://doi.org/10.1016/j.elerap.2010.11.002
Xia, H., Wei, X., An, W., Zhang, Z. J., & Sun, Z. (2021). Design of electronic-commerce recommendation systems based on outlier mining. Electronic Markets, 31 (2). https://doi.org/10.1007/s12525-020-00435-2
Xie, F., Chen, Z., Shang, J., & Fox, G. C. (2014). Grey Forecast model for accurate recommendation in presence of data sparsity and correlation. Knowledge-Based Systems, 69 (1), 179–190. https://doi.org/10.1016/j.knosys.2014.04.011
Xiong, J., Yu, L., Zhang, D., & Leng, Y. (2021). DNCP: An attention-based deep learning approach enhanced with attractiveness and timeliness of News for online news click prediction. Information and Management, 58 (2), 103428. https://doi.org/10.1016/j.im.2021.103428
Xu, Y., Yang, Y., Han, J., Wang, E., Ming, J., & Xiong, H. (2019). Slanderous user detection with modified recurrent neural networks in recommender system. Information Sciences, 505 , 265–281. https://doi.org/10.1016/j.ins.2019.07.081
Xue, G. R., Lin, C., Yang, Q., Xi, W., Zeng, H. J., Yu, Y., & Chen, Z. (2005). Scalable collaborative filtering using cluster-based smoothing. SIGIR 2005 - Proceedings of the 28th Annual International ACM SIGIR Conference on Research and Development in Information Retrieval , 114–121. https://doi.org/10.1145/1076034.1076056
Yan, S. R., Zheng, X. L., Wang, Y., Song, W. W., & Zhang, W. Y. (2015). A graph-based comprehensive reputation model: Exploiting the social context of opinions to enhance trust in social commerce. Information Sciences, 318 , 51–72. https://doi.org/10.1016/j.ins.2014.09.036
Yan, Y., Huang, C., Wang, Q., & Hu, B. (2020). Data mining of customer choice behavior in internet of things within relationship network. International Journal of Information Management, 50 , 566–574. https://doi.org/10.1016/j.ijinfomgt.2018.11.013
Yang, Z., Cai, Z., & Guan, X. (2016a). Estimating user behavior toward detecting anomalous ratings in rating systems. Knowledge-Based Systems, 111 , 144–158. https://doi.org/10.1016/j.knosys.2016.08.011
Yang, Z., Xu, L., Cai, Z., & Xu, Z. (2016b). Re-scale AdaBoost for attack detection in collaborative filtering recommender systems. Knowledge-Based Systems, 100 , 74–88. https://doi.org/10.1016/j.knosys.2016.02.008
Ye, X., Dong, L., & Ma, D. (2018). Loan evaluation in P2P lending based on Random Forest optimized by genetic algorithm with profit score. Electronic Commerce Research and Applications, 32 , 23–36. https://doi.org/10.1016/j.elerap.2018.10.004
Yim, D., Malefyt, T., & Khuntia, J. (2021). Is a picture worth a thousand views? Measuring the effects of travel photos on user engagement using deep learning algorithms. Electronic Markets, 31 (3), 619–637. https://doi.org/10.1007/s12525-021-00472-5
Zaïane, O. R. (2002). Building a recommender agent for e-learning systems. Proceedings - International Conference on Computers in Education, ICCE, 2002 , 55–59. https://doi.org/10.1109/CIE.2002.1185862
Zhang, D., Yan, Z., Jiang, H., & Kim, T. (2014). A domain-feature enhanced classification model for the detection of Chinese phishing e-Business websites. Information and Management, 51 (7), 845–853. https://doi.org/10.1016/j.im.2014.08.003
Zhang, D., Xu, H., Su, Z., & Xu, Y. (2015). Chinese comments sentiment classification based on word2vec and SVMperf. Expert Systems with Applications, 42 (4), 1857–1863. https://doi.org/10.1016/j.eswa.2014.09.011
Zhang, Q., Yang, L. T., Chen, Z., & Li, P. (2018a). A survey on deep learning for big data. Information Fusion, 42 , 146–157. https://doi.org/10.1016/j.inffus.2017.10.006
Zhang, W., Wang, C., Zhang, Y., & Wang, J. (2020a). Credit risk evaluation model with textual features from loan descriptions for P2P lending. Electronic Commerce Research and Applications, 42 , 100989. https://doi.org/10.1016/j.elerap.2020.100989
Zhang, W., Du, Y., Yang, Y., & Yoshida, T. (2018b). DeRec: A data-driven approach to accurate recommendation with deep learning and weighted loss function. Electronic Commerce Research and Applications, 31 , 12–23. https://doi.org/10.1016/j.elerap.2018.08.001
Zhang, W., Du, Y., Yoshida, T., & Yang, Y. (2019a). DeepRec: A deep neural network approach to recommendation with item embedding and weighted loss function. Information Sciences, 470 , 121–140. https://doi.org/10.1016/j.ins.2018.08.039
Zhang, X., Liu, H., Chen, X., Zhong, J., & Wang, D. (2020b). A novel hybrid deep recommendation system to differentiate user’s preference and item’s attractiveness. Information Sciences, 519 , 306–316. https://doi.org/10.1016/j.ins.2020.01.044
Zhang, X., Han, Y., Xu, W., & Wang, Q. (2019b). HOBA: A novel feature engineering methodology for credit card fraud detection with a deep learning architecture. Information Sciences . https://doi.org/10.1016/j.ins.2019.05.023
Zhang, Y., Chen, H., Lu, J., & Zhang, G. (2017). Detecting and predicting the topic change of knowledge-based systems: A topic-based bibliometric analysis from 1991 to 2016. Knowledge-Based Systems, 133 , 255–268. https://doi.org/10.1016/j.knosys.2017.07.011
Zhang, Z., Wei, X., Zheng, X., & Zeng, D. D. (2021). Predicting product adoption intentions: An integrated behavioral model-inspired multiview learning approach. Information & Management, 58 (7), 103484. https://doi.org/10.1016/j.im.2021.103484
Zhao, G., Lou, P., Qian, X., & Hou, X. (2020a). Personalized location recommendation by fusing sentimental and spatial context. Knowledge-Based Systems, 196 , 105849. https://doi.org/10.1016/j.knosys.2020.105849
Zhao, L., Dai, T., Qiao, Z., Sun, P., Hao, J., & Yang, Y. (2020b). Application of artificial intelligence to wastewater treatment: A bibliometric analysis and systematic review of technology, economy, management, and wastewater reuse. Process Safety and Environmental Protection, 133 , 169–182. https://doi.org/10.1016/j.psep.2019.11.014
Zhao, Y., Yu, Y., Li, Y., Han, G., & Du, X. (2019). Machine learning based privacy-preserving fair data trading in big data market. Information Sciences, 478 , 449–460. https://doi.org/10.1016/j.ins.2018.11.028
Zheng, X., Zhu, S., & Lin, Z. (2013). Capturing the essence of word-of-mouth for social commerce: Assessing the quality of online e-commerce reviews by a semi-supervised approach. Decision Support Systems, 56 , 211–222. https://doi.org/10.1016/j.dss.2013.06.002
Zheng, Z., & Padmanabhan, B. (2006). Selectively acquiring customer information: A new data acquisition problem and an active learning-based solution. Management Science, 52 (5), 697–712. https://doi.org/10.1287/mnsc.1050.0488
Ziegler, C.-N., McNee, S. M., Konstan, J. A., & Lausen, G. (2005). Improving recommendation lists through topic diversification. Proceedings of the 14th International Conference on World Wide Web , 22. https://doi.org/10.1145/1060745.1060754
Zoghbi, S., Vulić, I., & Moens, M. F. (2016). Latent Dirichlet allocation for linking user-generated content and e-commerce data. Information Sciences, 367–368 , 573–599. https://doi.org/10.1016/j.ins.2016.05.047
Download references
Author information
Authors and affiliations.
ICN Business School, CEREFIGE - Université de Lorraine, 86 rue du Sergent Blandan, 54003, Nancy, France
Ransome Epie Bawack
TBS Business School, 6 Place Alfonse Jourdain, 31000, Toulouse, France
Samuel Fosso Wamba & Kevin Daniel André Carillo
School of Management and Marketing, University of Wollongong, Wollongong, NSW, 2522, Australia
Shahriar Akter
You can also search for this author in PubMed Google Scholar
Corresponding author
Correspondence to Ransome Epie Bawack .
Additional information
Responsible Editor: Jianwei Hou
Publisher's note
Springer Nature remains neutral with regard to jurisdictional claims in published maps and institutional affiliations.
Rights and permissions
Reprints and permissions
About this article
Bawack, R.E., Wamba, S.F., Carillo, K.D.A. et al. Artificial intelligence in E-Commerce: a bibliometric study and literature review. Electron Markets 32 , 297–338 (2022). https://doi.org/10.1007/s12525-022-00537-z
Download citation
Received : 11 September 2021
Accepted : 22 February 2022
Published : 18 March 2022
Issue Date : March 2022
DOI : https://doi.org/10.1007/s12525-022-00537-z
Share this article
Anyone you share the following link with will be able to read this content:
Sorry, a shareable link is not currently available for this article.
Provided by the Springer Nature SharedIt content-sharing initiative
- Artificial intelligence
- Bibliometrics
JEL classification
- Find a journal
- Publish with us
- Track your research
Academia.edu no longer supports Internet Explorer.
To browse Academia.edu and the wider internet faster and more securely, please take a few seconds to upgrade your browser .
- We're Hiring!
- Help Center
- Most Cited Papers
- Most Downloaded Papers
- Newest Papers
- Last »
- Networking Follow Following
- Consumer Behavior Follow Following
- Information Systems Follow Following
- E-learning Follow Following
- Information Technology Follow Following
- Marketing Follow Following
- Open Source E-Commerce Follow Following
- Business Process Networks Follow Following
- Social Media Marketing Follow Following
- Databases Follow Following
Enter the email address you signed up with and we'll email you a reset link.
- Academia.edu Journals
- We're Hiring!
- Help Center
- Find new research papers in:
- Health Sciences
- Earth Sciences
- Cognitive Science
- Mathematics
- Computer Science
- Academia ©2024

- SUGGESTED TOPICS
- The Magazine
- Newsletters
- Managing Yourself
- Managing Teams
- Work-life Balance
- The Big Idea
- Data & Visuals
- Reading Lists
- Case Selections
- HBR Learning
- Topic Feeds
- Account Settings
- Email Preferences
How E-Commerce Fits into Retail’s Post-Pandemic Future
- Kathy Gramling,
- Jeff Orschell,
- Joshua Chernoff

New data from Ernst & Young suggests it will be an important part of the consumer experience — but not everything.
The pandemic has changed consumer behavior in big and small ways — and retailers are responding in kind. Since the early days of the pandemic Ernst & Young has been tracking these shifting trends using the EY Future Consumer Index and EY embryonic platform, which show a significant and widespread industry shift toward e-commerce. In this article, the authors suggest that while e-commerce will continue to be an essential element of retail strategy, the future success of retailers will ultimately depend on creating a cohesive customer experience, both online and in stores.
If we have learned one thing from the past year, it’s that things can change in an instant — changes we thought we had years to prepare for, behaviors we assumed we’d stick to forever, expectations we have of ourselves and our organizations. This is true of the way we live, the way we work, and the way we shop and buy as consumers.

- KG Kathy Gramling is EY Americas Consumer Industry Markets Leader
- JO Jeff Orschell is EY Americas Consumer Retail Leader
- JC Joshua Chernoff is EY-Parthenon Americas Managing Director
Partner Center

An official website of the United States government
The .gov means it’s official. Federal government websites often end in .gov or .mil. Before sharing sensitive information, make sure you’re on a federal government site.
The site is secure. The https:// ensures that you are connecting to the official website and that any information you provide is encrypted and transmitted securely.
- Publications
- Account settings
Preview improvements coming to the PMC website in October 2024. Learn More or Try it out now .
- Advanced Search
- Journal List
- Springer Nature - PMC COVID-19 Collection

E-Commerce Policy and International Business
Douglas cumming.
1 Florida Atlantic University, Florida, United States
2 University of Birmingham, Birmingham, UK
Sofia Johan
3 University of Aberdeen, Aberdeen, UK
Zaheer Khan
4 Innolab, University of Vaasa, Vaasa, Finland
Martin Meyer
5 University of Vaasa, Vaasa, Finland
The rise of digitization and information and communication technologies (ICT) is playing a vital role in facilitating global trade and business activities and in overcoming cross-border transaction costs. In so doing, it offers firms significant benefits and opportunities to compete on a global scale, as witnessed during the ongoing COVID-19 pandemic. The growth and widespread diffusion of internet-enabled technologies and platforms have created numerous opportunities for firms to provide products and services across both developed and developing markets. Yet, limited research has been conducted in the international business domain to explore the rise of ecommerce and its implications for international business scholarship. In this focused issue, we present an examination of the role played by e-commerce in international business, paying particular attention to the policy aspect of e-commerce and issuing a call for a greater integration of e-commerce policy in international business research.
Introduction
Over the past two decades, e-commerce has significantly changed the landscape of innovative business models and activities across the globe. Fueled by emerging technologies such as artificial intelligence, the Internet of Things (IoTs), and big data, e-commerce activities are on the rise, creating significant opportunities for both small and large firms to operate and provide products and services on a global scale (cf. McKinsey, 2021 ). In 2019, the global cross-border e-commerce market was estimated to be worth around US$780 billion and is expected to reach around US$4,820 billion by 2026 (cf. AP News, 2020 ). Similarly, other estimates indicate that around 9.3 billion cross-border e-commerce orders were placed in 2020, compared to 3.2 billion in 2015 (e.g., McKinsey 2022 ). This shows the growing importance and rise of cross-border e-commerce activities, which offers important opportunities to both small and large firms to scale up their cross-border e-commerce business models.
Changing consumer behaviors and buying patterns, favorable regulatory environments, and a growing trend of digitalization are playing a significant role in the development of global online markets. Several countries have also enacted regulations aimed at supporting the development of online infrastructure; for instance, the EU digital single market (European Parliament, 2020 ) and China’s Belt and Road initiative have provided important opportunities for businesses to interact with their customers online (cf. Yang et al., 2017 ). This suggests that there is a huge potential for both small and medium-sized enterprises (SMEs) and large multinational ones (MNEs) to conduct their business activities through online channels (cf. Ojala et al., 2018 ; Tolstoy et al., 2021 ). Such growth has been further amplified by the rapid spread of COVID-19 pandemic, which has impacted and dramatically changed social and economic activities on a global scale. Many governments around the world took unprecedented strict measures to contain the spread of the virus, including the imposition of lockdowns, social distancing, and border closures (cf. Dörrenbächer et al., 2021 ). These adversely affected economic activities, severely damaging many brick and mortar businesses, several of which, including both large and small firms, even went bankrupt and failed (cf. Amankwah-Amoah et al., 2021a ). Besides social activities, those measures had a profound impact on international business activities, including global e-commerce (e.g., Mena et al., 2022 ; McKinsey, 2021 ).
The COVID-19 pandemic also accelerated the pace of digitalization and the adoption of emerging technologies in all spheres of life, changing our buying patterns and causing many brick and mortar stores to shift from offline to online business models (cf. Amankwah-Amoah et al., 2021b ). In essence, we are witnessing the rise of digital globalization, whereby digital capabilities and business models are taking on a more prominent role in the global economy (cf. Luo, 2022 ). In such a context, McKinsey ( 2021 ) noted that COVID-19 has amplified e-commerce related activities, which were instrumental in surviving the challenges of the pandemic and will remain pivotal in the new normal . However, the growth of e-commerce is not uniform across the globe, as the internet penetration rate is quite uneven, especially across many developing and late liberalizing economies; this hinders firms from participating in e-commerce activities (cf. Zhu & Thatcher, 2010 ). Much e-commerce activity is now taking place in a number of emerging markets – such as China and India – potentially providing important opportunities for firms to locate their value chain activities. Yet, the location whence the virus originated and spread may have adversely affected the locational advantages of countries, potentially forcing firms to shift to other locations in order to mitigate the prolonged impact of the crisis on their business activities. Such issues deserve greater attention from international business (IB) scholars interested in understanding the fine-slicing of MNE value chain activities and the impact of locational advantage on e-commerce growth.
Extant studies have examined e-commerce strategy; i.e., the barriers challenges related to the adoption of e-commerce (cf. Agarwal & Wu, 2015 for an overview). Within the IB field, one stream of research applies Dunning’s OLI paradigm to explain the rise of e-commerce firms (cf. Singh & Kundu, 2002 ). Recent IB scholarship has focused on examining the rise of platform-based firms and has highlighted the importance of both direct and indirect networks effects in explaining their internationalization and cross-border activities (e.g., Brouthers et al., 2016 ; Chen et al., 2019 ; Zeng et al., 2019 ). The rise of e-platforms has been noted for being crucial to facilitate exports from emerging markets in relation to lessening the role played by information intermediaries (e.g., Jean et al., 2021 ). Despite the contributions made by the extant literature, limited discussions have been conducted around e-commerce-related policy and on how it affects international business activities and the global value chain configurations of MNEs. The key aim of this focused issue was to solicit contributions at the intersection of the international business, public policy, and legal aspects of e-commerce. This introductory article as well as the articles that are part of this focused issue provide useful insights on e-commerce policy and international business. Below, we briefly discuss e-commerce policy and international business, and then some of the key enabling factors that are facilitating the growth of cross-border e-commerce. Next, we explore the constraining factors, and key theories that can be utilized to study cross-border e-commerce, as well as the articles which are part of this focused issue.
E-commerce Policy and International Business
The 18th century’s economic geography was affected by the cost of transporting raw materials to local production locations, where they could be processed to make end products. The primary challenge of home production was that the greatest amount of the capital remained idle for most of the time (cf. Leamer et al., 1999 ). In the 19th century, the impact of mechanization on manufacturing and the deeper division of labor brought about improvements in transportation systems – canals, roads, railways, and fast ships (Robinson & Wahl, 1990 ). Over the 20th century, further improvements in transportation and communication caused a greater geographical fragmentation of production (cf. Arndt & Kierzkowski, 2001 ). Businesses outsource many of their specialist activities to specialized firms, thus producing intermediate intellectual inputs. Also, the international distribution of value-added activities is encouraged, matching the relative competitive advantage of each geographic location. These business revolutions are observed mainly due to the acceleration of the information and communication technology (ICT) transformation that connects firms, suppliers, and consumers in seamless webs; as such, this new connectivity is playing a vital role in facilitating business activities across the globe (e.g., Luo 2022 ).
In particular, over the last decade, e-commerce has become an indispensable part of the global economy, as the advent of the internet has substantially transformed the world. During recent years, this has attracted significant attention from IB scholars (see Table 1 ). The digitalization of modern life has enabled consumers to virtually benefit from online transactions. As access to and the adoption of the Internet is growing globally, the number of digital buyers keeps rising yearly. According to Statista ( 2020 ), over two billion people purchase goods and services online and e-retail sales have surpassed US$4.2 trillion worldwide. This upsurge in online consumers was observed during the COVID19 pandemic, as online shopping became almost a necessity due to the restrictions and lockdowns imposed by governments across the globe (McKinsey, 2021 ). Also, the availability of e-commerce platforms, marketplaces, and digital solutions has enabled different types of firms to navigate and pursue opportunities in international business environments (Schu et al., 2016 ). The usage of the Internet enables firms to acquire and interpret external knowledge, thereby gaining cheaper access to a wide variety of foreign markets (cf. Tolstoy et al., 2021 ). For instance, Farfetch and Asos, as well as many other similar firms are utilizing e-commerce business models and rapidly expanding into foreign markets. Some of these firms (e.g., Zalando) have initially expanded into close proximate markets (cf. Swoboda & Sinning, 2022 ). This suggests that e-commerce firms also face significant institutional challenges stemming from the policy and contextual differences found across different markets.
Number of papers on e-commerce published in international business journals
Journal | Number of papers | % of total | 1999 | 2001 | 2002 | 2005 | 2006 | 2010 | 2011 | 2016 | 2018 | 2019 | 2020 | 2021 | 2022 |
---|---|---|---|---|---|---|---|---|---|---|---|---|---|---|---|
Journal of International Business Studies | 12 | 24.49 | 0 | 4 | 1 | 0 | 0 | 0 | 0 | 1 | 0 | 1 | 0 | 3 | 2 |
Journal of World Business | 7 | 14.29 | 1 | 0 | 0 | 0 | 1 | 0 | 0 | 0 | 0 | 1 | 0 | 2 | 2 |
Asia Pacific Journal of Management | 4 | 8.16 | 0 | 0 | 0 | 0 | 0 | 0 | 0 | 0 | 0 | 1 | 0 | 1 | 2 |
International Business Review | 7 | 14.29 | 0 | 0 | 0 | 0 | 1 | 1 | 1 | 0 | 1 | 0 | 0 | 0 | 3 |
Journal of International Management | 11 | 22.45 | 0 | 1 | 0 | 3 | 0 | 0 | 0 | 0 | 0 | 0 | 2 | 0 | 5 |
Management International Review | 4 | 8.16 | 0 | 0 | 0 | 0 | 1 | 0 | 0 | 1 | 0 | 1 | 0 | 0 | 1 |
Global Strategy Journal | 4 | 8.16 | 0 | 0 | 0 | 0 | 0 | 0 | 0 | 1 | 1 | 0 | 1 | 0 | 1 |
To understand how the field has progressed, we also conducted a keyword search on articles published in major IB journals between 1999 and May 2022. We selected this time period because, during the last two decades, interest in e-commerce adoption and growth has risen across the globe. Table 1 provides an overview of the number of articles published in leading IB journals.
To further understand how the international e-commerce policy field has progressed relative to the more general IB one, we carried out searches on Google Scholar by year from 1999 to May 2022. The results are shown in Fig. 1, which takes as its benchmark the year 2010 = 100. We noted that, in 2010, there were 1,280, 40,200, 13,700, and 22,900 hits for the search terms “e-commerce” “policy”, “international business”, and “international business” “policy”, respectively. The graph presents some noteworthy findings. First, it shows that IB policy and, more generally, IB are topic areas that have exhibited very similar patterns of interest over time, with a rapid growth over the 2010–2015 period but a decline in recent years. Second, it shows that, although international business e-commerce policy and, more generally, e-commerce policy have recorded similar levels of interest over time, the former has had a relatively stronger growth over the last few years, with the highest level of growth over the 1999–2022 Q2 period. These trends likely reflect the global nature of e-commerce and the relevance of its related policies and regulations across countries due to linkages and spillovers among them (Allen et al., 2021 ). This also reflects the growing importance of financial technology (fintech) in international e-commerce, including but not being limited to the role played by digital currencies, initial coin offerings, and crowdfunding (Coakley & Lazos, 2021 ; Corbet et al., 2022 ; Philippi et al., 2021 ; Rossi et al., 2021 ).

Google Scholar Hits Indexed to 100 in 2010
In some countries, the government plays an administrative role in regulating Internet activity content and form. For example, the European Union has taken strategy initiatives, such as the ‘ Digital Single Market’ , aimed at harmonizing the rules on digital content and online sales and at facilitating global ecommerce (European Union, 2022a ). Also, in 2017, in an attempt to end geo-blockage, a new ‘ cross-border portability ’ regulation was developed in order to promote cross-border e-commerce sales (European Union, 2022b ). However, some governments act opportunistically and reserve policy obligations at will. Particularly, the rise of ecommerce firms in developing countries worries policy makers concerning legal challenges related to competition, antitrust and data localization (Oxley & Yeung, 2001 ). Government officials feel that, given the absence of adequate infrastructure investment and human resource development, less developed countries may become further marginalized in the e-commerce world. As a result, e-commerce firms are discouraged by sunk costs in technology and legitimacy building (cf. Leamer & Storper, 2001 ). In this light, it can be argued that the regulatory environment differences found between developed and developing countries hamper the foreign market decisions of e-commerce firms.
Host country policies and regulations place pressure on e-commerce firms, as they require legal compellability to gain foreign market access and external legitimacy (Dong et al., 2017 ). In the presence of policy and regulatory distance, e-commerce firms experience challenges to the transfer of firm-specific advantages to the global stage (Ang et al., 2015 ). Expanding to such countries becomes even more difficult given the complexity involved in setting up reliable contracts with local logistics service providers and channel partners (Swoboda & Sinning, 2022 ). This also hinders firms from efficiently interacting with customers based in the more rural areas of developing markets; this makes it necessary for firms to create new types of business models suited to interact with those customers or buyers/sellers through digital platforms. Further, the Internet policies enacted in some countries hamper the learning of e-commerce firms and increase their need to integrate prior knowledge, forcing them to internationalize to similar markets, as was the case with Zalando (Johanson & Vahlne, 1977 ; Swoboda & Sinning, 2022 ). Hence, policy differences can cause e-commerce firms to be negatively affected by a liability of outsidership, thereby discouraging their internationalization process. However, one could also argue that geographical distances matters less for e-commerce firms, as they can rapidly combine the upstream and downstream resources of their network partners and, in turn, provide products and services through digital platforms. In such a context, platform firms are developing novel business solutions for their customers through direct and indirect network effects, and are rapidly expanding across the globe (cf. Zeng et al., 2019 ; Stallkamp & Schotter, 2021 ). Institutional reforms and supportive environment are therefore vital to extend the rule of law to the policy agenda of those host countries that seek effective participation in the digital economy. Oxley & Yeung ( 2001 ) indicate that supportive institutional environment play a vital role in e-commerce growth.
The Key Enabling Factors of Cross-border E-commerce
The exponential growth of cross-border e-commerce is facilitated by economic , sociocultural , emerging technology , and legal key enabling factors that promote IB activities. Our keyword search papers published in major IB journals also suggest the vital role played by some of these factors in cross-border e-commerce activities. Below, we discuss each of these key enabling factors and draw implications for IB scholarship.
Economic factors
The rise of middle class, affluent consumers in many emerging markets is creating enabling environments for firms to provide them with one-click shopping experiences (cf. Cavusgil, 2021 ; Kravets & Sandikci, 2014 ). Global income level and GDP growth is spurring online business activities and supporting digital markets. This is providing firms like Amazon, eBay, Alibaba, Farfetch, Facebook, and Google with opportunities to interact with their customers and offer goods and services through online platforms. Furthermore, rapid urbanization is also supporting the growth of cross-border e-commerce activities. Increasing demand for goods and services is creating environments conducive to traditional brick and mortar businesses converting their static business models to more dynamic and online-based ones in order to engage with their customers. During the COVID-19 pandemic, many businesses switched to online modes of delivery and engagement with their customers in order to mitigate any adverse impacts on their business activities. The impositions of lockdowns, social distancing, border closures – and the resulting panic buying – also acted as enablers and supported the global growth of e-commerce.
However, the growth of e-commerce has created potential risks for customers and sellers, as fake goods can also be advertised through online channels. This can create uncertainties because, in less developed markets, the online infrastructure is not well developed and, due to rising inflation, many customers may not be able to afford products. Also, in such markets, digital illiteracy can potentially hinder the growth of e-commerce. This offers IB scholars important opportunities to examine the impact of the rise of middle classes on global e-commerce activities and how changing demand patterns are affecting virtual intermediaries and the development of the necessary online infrastructure.
Sociocultural factors
Changes in demographics (e.g., average population age and education levels) are key enabling factors for the growth of cross-border e-commerce. Increasing numbers of young consumers are engaging with digital technologies and social media and developing virtual communities; this offers firms important opportunities to advertise and supply products and services through digital markets- see for instance luxury e-commerce platform firms like Farfetch ( https://aboutfarfetch.com/ ). This enables digital platforms to disrupt traditional business models, replacing any established incumbents and reshaping firm design and structure (e.g., Ojala et al., 2018 ). Against this backdrop, IB scholars could examine the role played by changing demographics and by the emergence of virtual social communities in supporting cross-border e-commerce and the associated policies related to antitrust, tariff, competition, privacy, data security, and data localization at both the national and regional levels, and how do such policies facilitate the entry of small firms from developing markets to become part of the global e-commerce value chain.
Emerging technology factors
Emerging technologies and digital connectivity are enabling firms to scale up their digital business models (e.g., Luo 2022 ; Shaheer & Li, 2020 ). The accelerated development of digitalization, artificial intelligence, and big data technologies – as well as the penetration of the Internet across the globe – is creating substantial opportunities for firms to offer their products and services through digital markets. For example, e-platforms are supporting many emerging market firms in engaging in export activities (e.g., Jean et al., 2021 ). Emerging technologies, which are facilitating IB activities and creating substantial opportunities for platform-based MNEs and SMEs (e.g., Ojala et al., 2018 ; Zeng et al., 2019 ), are also paving the way for the adoption of mobile payments in many emerging markets (e.g., Kumar et al., 2021 ). However, variations in Internet penetration rates across developed and developing markets hinder the growth of digital markets; IB scholars could thus examine the enabling role played by emerging technologies in supporting the growth of cross-border e-commerce as well as the potential risks associated with them in relation to global digital markets. In addition, given the significant sub-national institutional variations found across emerging markets, IB scholars could examine the value creation and capture of digital firms across different regions (cf. Onuklu et al., 2021 ; Röell et al., 2022 ).
Legal factors
The legal factors and changing regulatory regimes are also playing important role in the growth of cross-border ecommerce. For instance, the EU’s single digital market initiative and China’s Belt and Road initiative are vital regulatory mechanisms underpinning the growth of global digital markets (e.g., European Parliament 2020 ; Yang et al., 2017 ). However, such initiatives are not providing small retailers with a level playing field, as e-commerce giants are engaging in predatory trade practices. Oxley & Yeung ( 2001 ) examined the barriers to the adoption and growth of e-commerce and suggested that a conducive legal system plays a significant role in them. In such a context, it would be interesting for IB scholars to examine the roles played by legal issues and by the predatory practices of giant e-commerce firms in enabling or constraining the growth of digital markets. The constantly evolving legal prescriptions in the areas of digital currency markets, crowdfunding, and other fintech applications (Allen et al., 2021 ) could likewise provide IB scholars with significant new research opportunities.
The Factors Constraining Cross-Border E-Commerce
Despite the potential role played by the above enabling factors, some prominent management consulting firms, including the Boston Consulting group (BCG, 2014 ), have suggested that cross-border e-commerce will make the world flatter. The BCG ( 2014 ) noted six factors that are constraining cross-border e-commerce growth. Managers of both MNEs and SMEs could use this checklist and formulate strategies suited to respond to such factors.
The first factor noted by the BCG is represented by unreliable and lengthy transit times. Customers want their products to be delivered quickly; global e-commerce firms should thus pay attention to minimizing the impact of intermediaries. During the COVID-19 pandemic, the high pressure to which global supply chains were subjected due to their length caused many to question their viability (cf. Ali et al., 2022 ).
The second factor noted by the BCG is related to the complexity and ambiguity of product return processes. This suggests that firms need to work with a range of stakeholders – including intermediaries and government agencies – in order to make the return of products purchased online simpler and less ambiguous. In this context, e-commerce policies play an important role in aiding virtual platform buyers and sellers and supporting customs agencies in enforcing fair taxes and tariffs, and could potentially stop the entry of illicit and counterfeit products.
The BCG further identified customs bottlenecks as the third factor hindering the growth of global e-commerce. In this regard, the EU single digital market initiative is an important step toward removing bottlenecks and enhancing customer experience (cf. European Parliament, 2020 ).
The fourth constraining factor is related to limited transparency on delivery. Firms need to work with the governments of the countries of origin of products in order to make the tracking of items in transit more transparent by linking it with their actual delivery time.
The BCG further reported price opacity due to complicated global VAT and custom charges as the fifth constraining factor, and the limited ability to alter items’ international delivery times and locations as the sixth.
These constraining factors suggest that both MNEs and SMEs involved in global e-commerce need to work closely with a range of stakeholders – including logistics service providers, sellers, and governments – to set up a supportive infrastructure for digital markets. IB scholars are uniquely positioned to investigate how firms can use their specific advantages and non-market strategies to overcome these constraints.
In addition to those identified by the BCG, legal and policy uncertainty is a significant barrier to cross-border e-commerce. For example, there is uncertainty in global fintech regulation, including but not limited to the use of digital currencies (Allen et al., 2021 ). How this uncertainty evolves over time and its impact on international business provide potential avenues for future scholarly work.
The Key Theoretical Perspectives of the Study of Cross-Border E-Commerce Policy
Due to its global growth, cross-border e-commerce and the associated policy regimes at the local and global level provides an important avenue in which to develop new theoretical and empirical insights and extend the existing understanding about cross-border international business activities and the rise of digital firms. In this section, we discuss a selection of the key theories that are relevant to the examination of some of the questions pertaining to this focused issue. We start with the institutional theory as institutions shape firms strategic choices including their overseas investment decisions (cf. Peng, 2003 ).
Institutional theory
Institutional theory asserts that the strategic choices made by firms are influenced by the social contexts in which they operate (Meyer & Rowan, 1977 ; North, 1990 ; Peng, 2003 ). In explaining the behaviors of firms, institutional theorists emphasize social norms, processes, and expectations (DiMaggio & Powell, 1983 ). Institutional theory provides valuable theoretical insights into the cross-border activities of e-commerce firms. Specifically, institutional distances – in terms of regulations, norms, and cultures – act as important boundary conditions for international e-commerce firms, accordingly affecting their internationalization process decisions (Swoboda & Sinning, 2022 ). While digitalization is connecting the world and providing customers, resources, and information with global reach (Shi et al., 2020 ; Qi et al., 2020 ), the institutional environment – e.g., the policies pertaining to online businesses and customer attitudes toward online reputation – varies across countries (cf. Luo et al., 2020 ). Such institutional variation implies that e-commerce firms engaged in cross-border activities are often embedded in sets of challenges that differ from those involved in conducting business in a single country. Thus, institutional theory provides an important lens for the examination of how global e-commerce firms overcome the challenges associated with weak institutions and how they exploit their firm-specific advantages (FSAs) to mitigate the negative impact of such challenges on the adoption and growth of e-commerce in a given market. Future research could use institutional theory to shed light on the sustainability and non-market strategies of e-commerce firms across both developed and emerging markets, as data localization, antirust, data security and privacy issues are becoming extremely important in the context of cross-border e-commerce activities.
Internalization theory
Internalization theory suggests that firms internally organize bundles of activities to develop and exploit their FSAs, such as knowledge, brand reputation, and management skills (Buckley & Casson, 1976 , 2009 ; Hennart, 1982 ; Rugman, 1981 ). Internalization theory is centered on explaining the foreign direct investments of firms with a focus on their strategic decision-making and behavioral heterogeneity. Therefore, this theory has long provided one of the main theoretical rationales explaining the progress of internationalizing firms (Benito et al., 2019 ; Kano et al., 2021 ; Narula et al., 2019 ). As per new internalization theory , MNE foreign direct investment and performance in foreign markets is shaped by the combination and exploitation of both FSAs and country-specific advantages (CSAs) (cf. Narula & Verbeke, 2015 ; Rugman & Verbeke, 2003 ). Market imperfections mean that firms need to internalize their intangible assets. As Narula & Verbeke ( 2015 , p. 1615) indicate: “bundles of CSAs and FSAs as a starting point for the analysis, but with the combination of international business opportunities and the MNE’s resources reservoir, subjected to a dual Coasean and Penrosean assessment, leading ultimately to an appropriate level of entrepreneurial resource orchestration that will in turn affect both business opportunities and the firm’s resource reservoir in the next period.”
In regard to cross-border e-commerce, the proponents of internalization theory suggest that firms should accumulate, into their digital platforms, technological assets that can work in concert with human capital (cf. Banalieva & Dhanaraj, 2019 ). When technological FSAs – which include patents, copyrights, trademarks, and ICT-based innovations – are combined with human capital, they ensure the easy cross-border transferability of knowledge (Zeng et al., 2019 ). However, e-commerce firms should invest in protecting their FSAs from imitation in international markets, as superior performance can be achieved through their recombination and exploitation of FSAs across different markets. Recently, scholars have called for research aimed at understanding the challenges associated with the recombination of FSAs (cf. Grøgaard et al., 2022 ; Lee et al., 2021 ; Verbeke & Kano, 2016 ); in this regard, cross-border e-commerce activities can offer important opportunities to investigate how e-commerce firms exploit, bundle, and recombine their FSAs across their value chains and in different markets (Hennart, 2009 ; Narula & Verbeke, 2015 ). Also, advantages developed based on intangible assets (e.g., web-traffic and reputation) in home market can be potentially deployed by internet firms in foreign markets (cf. Kotha et al., 2001 ). Going forward, scholars could draw upon insights from the institutional and new internalization theory in examining the creation and capture of value through the utilization of intangible assets by e-commerce firms across different markets.
Network theory
Network theory relates to the mechanisms and processes that interact with network structures to yield outcomes for individuals and groups (e.g., Emerson 1976 ; Johanson & Mattsson, 1988 ). It is about the complementarities among network partners that influence the behaviors and actions of actors to create value. Much of the theoretical research wealth of networks is focused on describing their structures (e.g., global, small) and node positions (e.g., central) (cf. Borgatti & Halgin, 2011 ). Research utilizing network theory suggests that cross-border e-commerce benefits from networks of partners located in different countries (Couclelis, 2004 ; Doern & Fey, 2006 ). By being embedded in international electronic networks, e-commerce firms gain access to external resources while efficiently governing the behavior of their cross-border partners (cf. Agarwal & Wu, 2015 ). The benefits of international electronic networks represent a powerful asset for e-commerce firms operating in emerging markets (Benmamoun et al., 2018 ). The prevalence of networks across the Internet lowers not only the entry and exit costs for emerging market e-commerce firms but also coordination and search costs. Thus, e-commerce firms are motivated to form Internet-based networks in order to gain access to complementary assets (Agarwal & Wu, 2015 ; Shim & Shin, 2016 ). Network theory can provide novel insights into the resource acquisition strategies of e-commerce firms and the impact of direct and indirect network effects on e-commerce growth.
Resource dependence theory
The central tenant of resource dependence theory is that firms are open systems dependent on the contingencies occurring in the external environment (cf. Pfeffer & Salancik, 1978 ; Hillman et al., 2009 ). As stated by Pfeffer & Salancik ( 1978 , p. 1), “ to understand the behavior of an organization you must understand the context of that behavior – that is, the ecology of the organization .” Resource dependence theory deals with the influence of external factors on the organizational environment and posits that, regardless of contextual constraints, managers can reduce any external environment uncertainty and dependence. In this regard, power – i.e., control over critical resources – is vital (Ulrich & Barney, 1984 ). E-commerce firms engaged in cross-border operations can manage the costs of dependence and attain any constrained resources. Particularly, the Internet and other emerging technologies make any needed resources available by enabling the establishment of linkages with trading partners located in international markets (cf. Alsaad et al., 2019 ). Also, the use of technologies facilitates the procurement and support the transmission of information (Chatterjee & Ravichandran, 2013 ). Furthermore, e-commerce firms can limit the power of any international partners by switching to or relying on multiple one through the use of digital technologies, thereby supporting their own international expansion activities (Zhou et al., 2018 ). It would be interesting to examine the power imbalances created by digital platforms and their resource mobilization efforts across developed and developing markets. Firms can gain resources through direct and indirect network effects, and holding a central position in a given network facilitates their resource acquisition and innovation (Wu et al., 2021). Further, future studies could combine network and institutional theory with resource dependence theory and shed light on how the characteristics of emerging technologies and platforms influence the dynamics of power and interdependencies between firms and their ecosystem partners and local governments in the diffusion and growth of e-commerce across both developed and developing markets.
The dynamic capabilities perspective
The dynamic capabilities perspective proposes that markets are dynamic and that, rather than being heterogenous in their resource endowments, firms exhibit differences in the capabilities through which they acquire and deploy resources (Teece et al., 1997 ; Teece, 2014 ). By exploiting their own dynamic capabilities, firms can implement new strategies that reflect changing market conditions by combining and transforming their resources in new and unique ways (Eisenhardt & Martin, 2000 ). That is to say that dynamic capabilities are change-oriented ones through which resources can be redeployed and competencies can be renewed in order to sustain competitive advantages with shifting business landscapes (Teece et al., 1997 ; Teece, 2014 ). Research based on the dynamic capabilities perspective suggests that the development of an e-business dynamic capability is vital to engage in cross-border e-commerce activities and achieve sustained competitive advantages in international markets (Daniel & Wilson, 2003 ; Rindova & Kotha, 2001 ). The e-business dynamic capability enables e-commerce transformation and fosters firms’ ability to create cross-border customer value through the use of innovative technologies (Wu & Hisa, 2008 ). E-business dynamic capabilities can involve digital sensing, digital seizing, and digital transforming, which enable digital transformation and facilitate cross-border e-commerce (Ghosh et al., 2022 ; Warner & Wäger, 2019 ). But the ways in which firms develop and deploy their various capabilities and scale up their e-commerce business models require further research; therefore, dynamic capabilities and resource orchestration approaches (cf. Teece, 2017 ; Sirmon et al., 2007 ; Sirmon et al., 2011 ; Zeng et al., 2021 ) can be useful lenses through which to examine how e-commerce firms leverage different types of capabilities for superior performance across different markets. Institutional theory suggests that firms’ strategies are shaped by institutions and that firms need to establish their host market legitimacy; future studies could thus integrate the dynamic capabilities perspective and institutional theory to investigate which kind of capabilities are central to managing institutional uncertainties and, in turn, enable e-commerce firms to develop their host market legitimacy.
Research Questions Featured in the Call for the MIR Focused Issue on E-Commerce Policy and International Business
We solicited contributions centered around these questions and related topic areas:
- How does e-commerce affect global capital flows to emerging and developed markets?
- What are the ways that SMEs can use crowdfunding to internationalize?
- How are multinationals affected by e-commerce policy?
- How does e-commerce policy affect the IB and SME ecosystem?
- How does e-commerce affect trade flows in different institutional contexts?
- What is the role of WTO in shaping e-commerce policy, and how does regulatory harmonization affect e-commerce policies?
- Does e-commerce policy affect cross-border investment from venture capitalists and private equity funds?
- How do regulatory changes affect e-commerce policies and adoption of e-commerce across different countries?
- How does e-commerce policy affect multinational value chains?
- How does e-commerce policy affect the link between SMEs and multinationals?
An Overview of the Articles Included in this Focused Issue
The aim of this focused issue was to encourage scholars to explore the potential role of e-commerce policy and IB. We received an encouraging response to the call and we appreciate the time and dedication of the anonymous reviewers in providing insightful comments to the manuscripts included in this focused issue. We received submissions covering a wide variety of topics related to e-commerce policy and IB. The five articles included in this focused issue are summarized in Table 2 .
Summary of the Articles Included in this Focused Issue on E-commerce Policy and IB
Authors | Research question(s) | Theory/perspective | Context | Key Findings |
---|---|---|---|---|
Ahi, Sinkovics and Sinkovics (2022) | What are the implications of policy for e-commerce? | National institutions | Theoretical/Conceptual | An inclusive international e-commerce needs the participation of SMEs and less developed economies through reforms in national institutional environments and the development of the infrastructure. |
Lee, Yang and Ghauri (2022) | How does institutional pressure affect digital platform risk? | Institutional perspective | A quantitative survey of 260 Chinese international new ventures (INVs) with 394 responses | The uncertainties associated with domestic and foreign market e-commerce policy affect the risks of digital platforms for INVs. Further, the COVID-19 pandemic acted as a moderating mechanism affecting digital platform risk and the internationalization scope of INVs. |
Singh, Munjal, Kundu and Rangarajan (2022) | RQ1: What impact do the Indian government’s policies have on mitigating home country-level market barriers to SME internationalization through e-commerce platforms? RQ2: What impact do the Indian government’s policies have on mitigating industry-level market barriers to SME internationalization using e-commerce platforms? | Institutional perspective | A qualitative study of a sample of Indian SMEs | The e-commerce policies enacted by the Indian government support platform-based SME internationalization due to improved procedural efficiency and educational programs. However, burdensome compliance procedures. logistical hurdles, and unfair trading practices reduce the impact of the export efficiency of e-commerce policies. |
Troise, Battisti, Christofi, van Vulpen, and Tarba (2022) | RQ1: How can SMEs use equity and reward crowdfunding to internationalize and bypass traditional systems/channels? RQ2: What are the limitations related to the use of equity and reward crowdfunding by SMEs to internationalize? | The resource-based view (RBV) and knowledge-based view (KBV) | A qualitative study of a sample of Italian SMEs | The findings show that equity and reward crowdfunding play a vital role in helping SMEs to overcome their financial and knowledge resource limitations in regard to internationalization. However, the lack of e-commerce policies in relation to equity crowdfunding and the regulation of the pre-ordering mechanism limit the use of crowdfunding by SMEs. |
Cha, Kotabe and Wu (2022) | How can the divergence of global e-commerce policies affect the internationalization of sharing economy firms across different countries? | Hayek’s knowledge economy approach | Conceptual | The quality of digital platforms matters for the persistence of digital platform-based transactions. In this case, detailed and well-organized e-commerce policies are needed to separate the trade-offs between value creation from data ownership and control against national security threats. |
In the first article of this focused issue, Ahi et al. (2022), emphasize the issues related to policy and the development of international e-commerce. They argue that, in terms of data protection, legal protection, and business laws, governmental policy influences the adoption of e-commerce. The authors perform a thematic analysis of academic papers and reports published by government organizations. They argue that national infrastructure environments (i.e., consumer protection environment, data security and protection, and mitigation of e-business risks) and infrastructure (i.e., access to finance, digital literacy, and advice on legal issues like taxation and exporting) determine the development of an inclusive international e-commerce environment for firms – particularly SMEs – from less developed economies. The authors conclude the paper by outlining areas for future research, including national e-commerce readiness, e-commerce taxation policy, and international e-commerce policy for SMEs. Future studies could use these conceptual insights to empirically test the relevance of various policy-related initiatives for e-commerce in less developed countries and the ways in which national level e-commerce policy affects the internationalization of SMEs as well as the inward foreign direct investment by MNEs.
In the second article, Lee and colleagues (2022), argue the role played by domestic and foreign market e-commerce policy uncertainties as well as by the COVID-19 pandemic in affecting the digital platform risk and internationalization scope of INVs. Unlike most previous work conducted from the institutional perspective, Lee and colleagues compare the effect of domestic and foreign market e-commerce policy uncertainties for digital platform risk and determine the contingency role played by the COVID-19 pandemic. The authors conducted a rigorous quantitative study by using two-by-two waves of surveys with 260 Chinese INVs, resulting in 394 responses. The results of structural equation modeling suggest that foreign market e-commerce policy uncertainty, as compared to its domestic market counterpart, has a stronger positive impact on digital platform risks for INVs, which in turn negatively influences the internationalization scope of INVs. Additionally, the findings reveal that the COVID-19 pandemic acted as a moderator, so that it (1) weakened the positive relationship between domestic market e-commerce policy uncertainty and perceived digital platform risk; (2) strengthened the positive relationship between foreign market e-commerce policy uncertainty and digital platform risk for INVs; and (3) strengthened the negative relationship between digital platform risk and the internationalization scope of INVs. The contributions of this study lie in demonstrating the role played by e-commerce policy uncertainty, digital platform risk, the COVID-19 pandemic, and the internationalization scope of INVs from an institutional perspective.
The study of Singh et al. (2022) discusses the relevance of policy initiatives in regulating e-commerce platforms. They argue that the Indian government’s policies mitigate home market barriers (i.e., enhance export efficiency, mitigate governance concerns related to e-commerce, and overcome knowledge and skills barriers) and industry-related ones (firm size, restrictive industry regulations, price competition, technological limitations, domestic and foreign competition, unfair trading practices, and industry concentration). This is in line with the claims made by Lee and colleagues in their article on the role played by policy initiatives in support of the international e-commerce activities of SMEs from emerging markets. The empirical context of platform-based internationalizing SMEs, as compared to traditional SMEs from India, opens both theories to fresh examination, and reopens debates on the importance of platform-based SMEs for international development. Based on the analysis of 16 semi-structured interviews with SME managers, government officials, and industry expert in India, the findings show that India’s Draft National E-commerce policy has enhanced efficiency by simplifying any administrative hurdles and managing anticompetitive threats in the Indian platform-economy context. The Indian government has also made a concerted effort toward educating and training SMEs to adopt e-commerce for export. However, the bureaucracy, procedural complexity, and unfair trading practices create challenges for SME exploitation of e-commerce policy initiatives. The paper extends the literature on e-commerce by showing how government policy reforms shape the internationalization activities of platform-based SMEs. As studies have demonstrated that platform firms drive superior performance through direct and indirect network effects (cf. Zeng et al., 2019 ; Stallkamp & Schotter, 2021 ), it would be interesting for future studies to explore the effects of domestic and foreign networks on the internationalization of e-commerce firms from emerging/developing markets and their value creation and capture in different institutional settings. In future, there is also a need for studies aimed at examining the network effects as well as the national and global institutional factors and the digital intensity of particular industries, and how these factors influence the initial internationalization and post-entry performance of e-commerce firms. Such studies could integrate comparative institutions and examine their impact on digital globalization in facilitating or hindering the growth of e-commerce in a particular market (e.g., Oxley & Yeung 2001 ; Hempel & Kwong, 2001 ).
The fourth article, by Troise et al. (2022), focuses on the role played by equity and reward crowdfunding. The study took the RBV and KBV to argue that equity and reward crowdfunding – albeit to different extents – provide SMEs with additional valuable resources (e.g., new knowledge on markets, products, and strategy) for internationalization that go beyond the traditional effect of crowdfunding for fundraising. This reflects Cumming and Johan’s ( 2017 , 2019 ) and Ahsan and Musteen’s ( 2021 ) idea and evidence that international opportunities are identified and exploited through crowdfunding. Therefore, Troise and colleagues add to the scant body of research on the role played by crowdfunding in the international venturing of SMEs and address the neglected questions of how SMEs use equity and reward crowdfunding to internationalize and bypass traditional systems/channels, and of what limitations related to the use of equity and reward crowdfunding affect SME internationalization. The authors adopted a multiple case study design and collected qualitative data through 48 semi-structured interviews conducted with SMEs located in Italy. The focus on Italian SMEs primarily from the service sector (e.g., information and communication services) brings our focus back to the debate surrounding the knowledge-based economies (Harris, 2001 ) and the prominence of the service sector in the international business context (cf. Doh et al., 2021 ). The authors found that both equity and reward crowdfunding are vital for the internationalization of SMEs, given their role in providing financial resources, developing international networks, increasing foreign market awareness, and establishing international agreements/brands. They further noted that Italian SMEs face challenges in the use of crowdfunding linked to the absence of e-commerce policies, restricted domestic platforms, and limitations for foreign investors. To further extend this work, future studies could consider the concept of ‘culture’ and understand its relevance for equity vs. reward crowdfunding in relation to SME internationalization (e.g., Jancenelle et al., 2019 ; Nambisan et al., 2019 ).
In the final article in this issue, Cha, Kotabe, and Wu (2022) address a gap in our understanding of how the divergence of global e-commerce policies can affect the internationalization process of sharing economy firms over time. While previous studies were focused on the global strategies adopted by firms on the basis of existing network advantages (cf. Brouthers et al., 2016 ; Chen et al., 2019 ), their static approach, which involved a core-periphery network model, offered a limited understanding of the internationalization of sharing economy firms that form business ecosystems with exponential and rapid growth over time. Cha and colleagues adopted the Hayekian perspective ( 1945 , 1988 ) to effectively account for the internationalizing problems faced by sharing economy firms in the presence of diverging global e-commerce policies. The paper suggests that it is vital for sharing economy firms to govern business ecosystems by facilitating the self-organization of their participants through digital protocols and code-based algorithmic rules. This can make a sharing economy firm more likely to benefit from lower profit volatility and greater flexibility through economies of scale and continuous innovation by collective intelligence. The contributions made by this study are three-fold. First, it applies Hayek’s concepts related to the knowledge economy and focuses on the attributes of digital platform-based transactional opportunities for value propositions and value creation through ecosystem-specific advantages. Second, the authors concentrate on how the sharing economy can internationalize its business models based on digital algorithmic rules. Third, it is argued that, since 1998 within the WTO, the debate and discussion on e-commerce policies have not developed; the authors therefore suggest propositions suited to address these questions in future studies. Future scholarly work could build upon this study and integrate dynamic capabilities and knowledge based approaches to examine how sharing economy-based e-commerce platform firms combine heterogeneous ecosystem knowledge assets and create and capture value (cf. Teece et al., 1997 ; Teece, 2007; Grant 1996 ; Grant & Phene, 2022 ; Felin & Hesterly, 2007 ). It would also be interesting to examine the role played by national and global institutions and managerial actions in the scaling up of global business models of e-commerce firms. As institutional theory states that firms expanding into foreign markets face different institutional logics, it would be important to study how a global e-commerce firm “ recombines the knowledge acquired in its home market with the gradual accumulation of learning in the foreign market ” (Kogut & Zander, 1993 , p. 636), and develops new set of digital capabilities that are scalable across network of subsidiaries.
Finally, each of the articles examines important issues related to e-commerce policy and its ramifications for IB. Collectively, these articles provide unique insights into and advance our understanding of this important topic as many governments such as India are making e-commerce policy a key part of their foreign trade policy (e.g., Mint 2022 ). The focused issue also suggests that there is further scope for more integrative and comparative studies on e-commerce policy and its implications for IB. Future studies could make national and global policies on e-commerce a central part of their theorizing in order to provide a more fine-grained understanding of the role played by e-commerce policy on cross-border e-commerce activities.
Summary and Concluding Remarks
In this paper, we presented evidence that e-commerce policy in international business is growing at a substantially faster rate than the average research area within the field of international business. This research interest is driven by the natural intersection between e-commerce and international business and the relevance of policy and regulatory spillovers from one country to another. We provided a summary of the major factors that both enable and inhibit the expansion of e-commerce around the world, we illustrated the theoretical lenses through which these topics could be examined in future research, and we explained how the excellent papers in this special issue contribute to the literature. We expect the evolving international policy landscape surrounding e-commerce and new technologies aimed at financing e-commerce to continue to offer significant areas of scholarly inquiry in the future.
Publisher’s Note
Springer Nature remains neutral with regard to jurisdictional claims in published maps and institutional affiliations.
Contributor Information
Douglas Cumming, Email: ude.uaf@dgnimmuc .
Sofia Johan, Email: ude.uaf@nahojs .
Zaheer Khan, Email: [email protected] .
Martin Meyer, Email: [email protected] .
- Agarwal J, Wu T. Factors influencing growth potential of e-commerce in emerging economies: An institution‐based N‐OLI framework and research propositions. Thunderbird International Business Review. 2015; 57 (3):197–215. doi: 10.1002/tie.21694. [ CrossRef ] [ Google Scholar ]
- Ahsan M, Musteen M. International opportunity development on crowdfunding platforms: A spatial, temporal, and structural framework. International Business Review. 2021; 30 (6):101912. doi: 10.1016/j.ibusrev.2021.101912. [ CrossRef ] [ Google Scholar ]
- Allen F, Gu X, Jagtiani J. A survey of fintech research and policy discussion. Review of Corporate Finance. 2021; 1 (3–4):259–339. doi: 10.1561/114.00000007. [ CrossRef ] [ Google Scholar ]
- Alsaad A, Mohamad R, Ismail NA. The contingent role of dependency in predicting the intention to adopt B2B e-commerce. Information Technology for Development. 2019; 25 (4):686–714. doi: 10.1080/02681102.2018.1476830. [ CrossRef ] [ Google Scholar ]
- Ang SH, Benischke MH, Doh JP. The interactions of institutions on foreign market entry mode. Strategic Management Journal. 2015; 36 (10):1536–1553. doi: 10.1002/smj.2295. [ CrossRef ] [ Google Scholar ]
- Arndt, S. W., & Kierzkowski, H. (Eds.). (2001). Fragmentation: New production patterns in the world economy . OUP Oxford
- Ali I, Arslan A, Chowdhury M, Khan Z, Tarba SY. Reimagining global food value chains through effective resilience to COVID-19 shocks and similar future events: A dynamic capability perspective. Journal of Business Research. 2022; 141 :1–12. doi: 10.1016/j.jbusres.2021.12.006. [ PMC free article ] [ PubMed ] [ CrossRef ] [ Google Scholar ]
- Amankwah-Amoah, J., Khan, Z., & Wood, G. (2021a). COVID-19 and business failures: The paradoxes of experience, scale, and scope for theory and practice. European Management Journal , 39 (2) , 179–184
- Amankwah-Amoah, J., Khan, Z., Wood, G., & Knight, G. (2021b). COVID-19 and digitalization: The great acceleration. Journal of Business Research , 136 , 602–611 [ PMC free article ] [ PubMed ]
- AP NEWS (2020). Global Trends in Cross-Border B2C E-Commerce Market to Exceed USD 4,820 Billion by 2026. Available at: https://apnews.com/press-release/marketersmedia/lifestyle-technology-business-e-commerce-fashion-20c0e999da913c13e69f06b581a9b3 ab Accessed on 15 April, 2022
- Banalieva ER, Dhanaraj C. Internalization theory for the digital economy. Journal of International Business Studies. 2019; 50 (8):1372–1387. doi: 10.1057/s41267-019-00243-7. [ CrossRef ] [ Google Scholar ]
- BCG (2014). Cross-Border E-Commerce Makes the World Flatter. Available at: https://www.bcg.com/publications/2014/post-parcel-sales-channel-transformation-cross-border-e-commerce Accessed on 18 April, 2022
- Benito GR, Petersen B, Welch LS. The global value chain and internalization theory. Journal of International Business Studies. 2019; 50 (8):1414–1423. doi: 10.1057/s41267-019-00218-8. [ CrossRef ] [ Google Scholar ]
- Benmamoun M, Singh N, Lehnert K, Lee SB. Internationalization of e-commerce corporations (ECCs): Advanced vs emerging markets ECCs. Multinational Business Review. 2018; 27 (4):317–338. doi: 10.1108/MBR-02-2018-0010. [ CrossRef ] [ Google Scholar ]
- Borgatti SP, Halgin DS. On network theory. Organization science. 2011; 22 (5):1168–1181. doi: 10.1287/orsc.1100.0641. [ CrossRef ] [ Google Scholar ]
- Brouthers KD, Geisser KD, Rothlauf F. Explaining the internationalization of ibusiness firms. Journal of International Business Studies. 2016; 47 (5):513–534. doi: 10.1057/jibs.2015.20. [ CrossRef ] [ Google Scholar ]
- Buckley PJ, Casson MC. The internalisation theory of the multinational enterprise: A review of the progress of a research agenda after 30 years. Journal of International Business Studies. 2009; 40 (9):1563–1580. doi: 10.1057/jibs.2009.49. [ CrossRef ] [ Google Scholar ]
- Buckley PJ, Casson M. The Future of the Multinational Enterprise. Basingstoke: Macmillan; 1976. [ Google Scholar ]
- Cavusgil ST. Advancing knowledge on emerging markets: Past and future research in perspective. International Business Review. 2021; 30 (2):101796. doi: 10.1016/j.ibusrev.2021.101796. [ CrossRef ] [ Google Scholar ]
- Chatterjee D, Ravichandran T. Governance of interorganizational information systems: A resource dependence perspective. Information Systems Research. 2013; 24 (2):261–278. doi: 10.1287/isre.1120.0432. [ CrossRef ] [ Google Scholar ]
- Chen L, Shaheer N, Yi J, Li S. The international penetration of ibusiness firms: Network effects, liabilities of outsidership and country clout. Journal of International Business Studies. 2019; 50 (2):172–192. doi: 10.1057/s41267-018-0176-2. [ CrossRef ] [ Google Scholar ]
- Coakley J, Lazos A. New developments in equity crowdfunding: A review. Review of Corporate Finance. 2021; 1 (3–4):341–405. doi: 10.1561/114.00000008. [ CrossRef ] [ Google Scholar ]
- Corbet, S., Greene, M., & Larkin, C. (2022). “The Impact of Central Bank Digital Currency (CBDC) Development on Cryptocurrency: A Taxonomic Analysis” Review of Corporate Finance, 2 , forthcoming
- Couclelis H. Pizza over the Internet: e-commerce, the fragmentation of activity and the tyranny of the region. Entrepreneurship & Regional Development. 2004; 16 (1):41–54. doi: 10.1080/0898562042000205027. [ CrossRef ] [ Google Scholar ]
- Cumming, D., & Johan, S. (2017). Crowdfunding and entrepreneurial internationalization, The world scientific reference on entrepreneurship: Volume 2: Entrepreneurial finance—Managerial and policy implications , N. Dai and D. Siegel, Eds., pages 109–126
- Cumming, D. J., & Johan, S. A. (2019). Crowdfunding: Fundamental cases, facts, and insights . Elsevier Science Academic Press
- Daniel EM, Wilson HM. The role of dynamic capabilities in e-business transformation. European Journal of Information Systems. 2003; 12 (4):282–296. doi: 10.1057/palgrave.ejis.3000478. [ CrossRef ] [ Google Scholar ]
- DiMaggio PJ, Powell WW. The iron cage revisited: institutional isomorphism and collective rationality in organizational fields. American Sociological Review. 1983; 48 :147–160. doi: 10.2307/2095101. [ CrossRef ] [ Google Scholar ]
- Doern RR, Fey CF. E-commerce developments and strategies for value creation: The case of Russia. Journal of World Business. 2006; 41 (4):315–327. doi: 10.1016/j.jwb.2006.08.001. [ CrossRef ] [ Google Scholar ]
- Doh J, Budhwar P, Wood G. Long-term energy transitions and international business: Concepts, theory, methods, and a research agenda. Journal of International Business Studies. 2021; 52 (5):951–970. doi: 10.1057/s41267-021-00405-6. [ PMC free article ] [ PubMed ] [ CrossRef ] [ Google Scholar ]
- Dong MC, Fang Y, Straub DW. The impact of institutional distance on the joint performance of collaborating firms: The role of adaptive interorganizational systems. Information Systems Research. 2017; 28 (2):309–331. doi: 10.1287/isre.2016.0675. [ CrossRef ] [ Google Scholar ]
- Dörrenbächer C, Sinkovics RR, Becker-Ritterspach F, Boussebaa M, Curran L, de Jonge A, Khan Z. The Covid-19 pandemic: Towards a societally engaged IB perspective. critical perspectives on international business. 2021; 17 (2):149–164. doi: 10.1108/cpoib-02-2021-0021. [ CrossRef ] [ Google Scholar ]
- Eisenhardt KM, Martin JA. Dynamic capabilities: what are they? Strategic management journal. 2000; 21 (10-11):1105–1121. doi: 10.1002/1097-0266(200010/11)21:10/11<1105::AID-SMJ133>3.0.CO;2-E. [ CrossRef ] [ Google Scholar ]
- Emerson RM. Social exchange theory. Annual Review of Sociology. 1976; 2 :335–362. doi: 10.1146/annurev.so.02.080176.002003. [ CrossRef ] [ Google Scholar ]
- European Parliament . How to fully reap the benefits of the internal market for e-commerce? Luxembourg: European Parliament; 2020. [ Google Scholar ]
- European Union (2022a). What is the digital single market about? Retrieved May 20, 2022, https://ec.europa.eu/eurostat/cache/infographs/ict/bloc-4.html
- European Union (2022b). Regulation (EU) 2017/1128 of the European Parliament and of the Council of 14 June 2017 on cross-border portability of online content services in the internal marketText with EEA relevance. Retrieved May 20, 2022, https://eur-lex.europa.eu/legal-content/EN/ALL/?uri=CELEX%3A32017R1128
- Felin T, Hesterly WS. The knowledge-based view, nested heterogeneity, and new value creation: Philosophical considerations on the locus of knowledge. Academy of Management Review. 2007; 32 (1):195–218. doi: 10.5465/amr.2007.23464020. [ CrossRef ] [ Google Scholar ]
- Ghosh S, Hughes M, Hodgkinson I, Hughes P. Digital transformation of industrial businesses: A dynamic capability approach. Technovation. 2022; 113 :102414. doi: 10.1016/j.technovation.2021.102414. [ CrossRef ] [ Google Scholar ]
- Grant R, Phene A. The knowledge based view and global strategy: Past impact and future potential. Global Strategy Journal. 2022; 12 (1):3–30. doi: 10.1002/gsj.1399. [ CrossRef ] [ Google Scholar ]
- Grant RM. Toward a knowledge-based theory of the firm. Strategic Management Journal. 1996; 17 :109–122. doi: 10.1002/smj.4250171110. [ CrossRef ] [ Google Scholar ]
- Grøgaard, B., Colman, H. L., & Stensaker, I. G. (2022). Legitimizing, leveraging, and launching: Developing dynamic capabilities in the MNE. Journal of International Business Studies , 53 (4), 636–656
- Harris RG. The knowledge-based economy: intellectual origins and new economic perspectives. International journal of management reviews. 2001; 3 (1):21–40. doi: 10.1111/1468-2370.00052. [ CrossRef ] [ Google Scholar ]
- Hayek FA. The use of knowledge in society. American Economic Review. 1945; 35 (4):519–530. [ Google Scholar ]
- Hayek FA. The Fatal Conceit: The Errors of Socialism. Chicago: University of Chicago Press; 1988. [ Google Scholar ]
- Hempel PS, Kwong YK. B2B e-Commerce in emerging economies: i-metal. com’s non-ferrous metals exchange in China. The Journal of Strategic Information Systems. 2001; 10 (4):335–355. doi: 10.1016/S0963-8687(01)00058-0. [ CrossRef ] [ Google Scholar ]
- Hennart JF. Down with MNE-centric theories! Market entry and expansion as the bundling of MNE and local assets. Journal of International Business Studies. 2009; 40 (9):1432–1454. doi: 10.1057/jibs.2009.42. [ CrossRef ] [ Google Scholar ]
- Hennart JF. A Theory of Multinational Enterprise. Ann Arbor, MI: University of Michigan; 1982. [ Google Scholar ]
- Hillman AJ, Withers MC, Collins BJ. Resource dependence theory: A review. Journal of Management. 2009; 35 (6):1404–1427. doi: 10.1177/0149206309343469. [ CrossRef ] [ Google Scholar ]
- Jancenelle VE, Javalgi RRG, Cavusgil E. Cultural entrepreneurship and legitimate distinctiveness in international prosocial crowdfunding. International Business Review. 2019; 28 (4):802–810. doi: 10.1016/j.ibusrev.2019.04.002. [ CrossRef ] [ Google Scholar ]
- Jean RJ, Kim D, Zhou KZ, Cavusgil ST. E-platform use and exporting in the context of Alibaba: A signaling theory perspective. Journal of International Business Studies. 2021; 52 (8):1501–1528. doi: 10.1057/s41267-020-00396-w. [ CrossRef ] [ Google Scholar ]
- Johanson, J., & Mattsson, L. G. (1988). Internationalization in industrial systems – a network approach. In N. Hood, & J. E. Vahlne (Eds.), Strategies in global competition (pp. 287–314). Croom Helm
- Johanson J, Vahlne JE. The internationalization process of the firm—a model of knowledge development and increasing foreign market commitments. Journal of international business studies. 1977; 8 (1):23–32. doi: 10.1057/palgrave.jibs.8490676. [ CrossRef ] [ Google Scholar ]
- Kano L, Ciravegna L, Rattalino F. The family as a platform for FSA development: Enriching new internalization theory with insights from family firm research. Journal of International Business Studies. 2021; 52 (1):148–160. doi: 10.1057/s41267-020-00308-y. [ CrossRef ] [ Google Scholar ]
- Kogut B, Zander U. Knowledge of the firm and the evolutionary theory of the multinational corporation. Journal of International Business Studies. 1993; 24 (4):625–645. doi: 10.1057/palgrave.jibs.8490248. [ CrossRef ] [ Google Scholar ]
- Kotha S, Rindova VP, Rothaermel FT. Assets and actions: Firm-specific factors in the internationalization of US Internet firms. Journal of International Business Studies. 2001; 32 (4):769–791. doi: 10.1057/palgrave.jibs.8490994. [ CrossRef ] [ Google Scholar ]
- Kravets O, Sandikci O. Competently ordinary: New middle class consumers in the emerging markets. Journal of Marketing. 2014; 78 (4):125–140. doi: 10.1509/jm.12.0190. [ CrossRef ] [ Google Scholar ]
- Kumar, V., Nim, N., & Agarwal, A. (2021). Platform-based mobile payments adoption in emerging and developed countries: Role of country-level heterogeneity and network effects. Journal of International Business Studies , 78 52(8), 1529–1558
- Leamer EE, Maul H, Rodriguez S, Schott PK. Does natural resource abundance increase Latin American income inequality? Journal of development Economics. 1999; 59 (1):3–42. doi: 10.1016/S0304-3878(99)00004-8. [ CrossRef ] [ Google Scholar ]
- Leamer EE, Storper M. The economic geography of the internet age. Journal of International Business Studies. 2001; 32 (4):641–665. doi: 10.1057/palgrave.jibs.84909988. [ CrossRef ] [ Google Scholar ]
- Lee JM, Narula R, Hillemann J. Unraveling asset recombination through the lens of firm-specific advantages: A dynamic capabilities perspective. Journal of World Business. 2021; 56 (2):101193. doi: 10.1016/j.jwb.2021.101193. [ CrossRef ] [ Google Scholar ]
- Luo, Y. (2022). New connectivity in the fragmented world.Journal of International Business Studies:1–19 [ PMC free article ] [ PubMed ]
- Luo, L., Ma, X., & Wang, Z. (2020). The moderate-reputation trap: Evidence from a Chinese cross-border business-to-business e-commerce portal.Asia Pacific Journal of Management,1–38
- McKinsey (2022). Signed, sealed, and delivered: Unpacking the cross-border parcel market’s promise. Available at: https://www.mckinsey.com/industries/travel-logistics-and-infrastructure/our-insights/signed-sealed-and-delivered-unpacking-the-cross-border-parcel-markets-promise Accessed on 20 April 2022
- McKinsey (2021). The six must-haves to achieve breakthrough growth in e-commerce D2C. Available at: https://www.mckinsey.com/business-functions/marketing-and-sales/our-insights/the-six-must-haves-to-achieve-breakthrough-growth-in-e-commerce-d2c Accessed on 18 April 2022
- Mena C, Karatzas A, Hansen C. International trade resilience and the Covid-19 pandemic. Journal of Business Research. 2022; 138 :77–91. doi: 10.1016/j.jbusres.2021.08.064. [ PMC free article ] [ PubMed ] [ CrossRef ] [ Google Scholar ]
- Meyer JW, Rowan B. Institutionalized organizations: formal structure as myth and ceremony. American Journal of Sociology. 1977; 83 (2):340–363. doi: 10.1086/226550. [ CrossRef ] [ Google Scholar ]
- Mint (2022). Foreign trade policy may include chapter on e-commerce biz. Available at
- https:// www.livemint.com/politics/policy/foreign-trade-policy-may-include-chapter-on-e-commerce-biz-11662403428104.html . Accessed on 08 September 2022.
- Nambisan S, Zahra SA, Luo Y. Global platforms and ecosystems: Implications for international business theories. Journal of International Business Studies. 2019; 50 (9):1464–1486. doi: 10.1057/s41267-019-00262-4. [ CrossRef ] [ Google Scholar ]
- Narula R, Asmussen CG, Chi T, Kundu SK. Applying and advancing internalization theory: The multinational enterprise in the twenty-first century. Journal of International Business Studies. 2019; 50 (8):1231–1252. doi: 10.1057/s41267-019-00260-6. [ CrossRef ] [ Google Scholar ]
- Narula R, Verbeke A. Making internalization theory good for practice: The essence of Alan Rugman’s contributions to international business. Journal of World Business. 2015; 50 (4):612–622. doi: 10.1016/j.jwb.2015.08.007. [ CrossRef ] [ Google Scholar ]
- North D. Institutions, Institutional Change, and Economic Performance. New York: Norton; 1990. [ Google Scholar ]
- Ojala A, Evers N, Rialp A. Extending the international new venture phenomenon to digital platform providers: A longitudinal case study. Journal of World Business. 2018; 53 (5):725–739. doi: 10.1016/j.jwb.2018.05.001. [ CrossRef ] [ Google Scholar ]
- Onuklu, A., Hill, T. T., Darendeli, I. S., & Genc, O. F. (2021). Poison or antidote: How subnational informal institutions exacerbate and ameliorate institutional voids. Journal of International Management , 27 (1), p.100806
- Oxley JE, Yeung B. E-commerce readiness: Institutional environment and international competitiveness. Journal of International Business Studies. 2001; 32 (4):705–723. doi: 10.1057/palgrave.jibs.8490991. [ CrossRef ] [ Google Scholar ]
- Peng MW. Institutional transitions and strategic choices. Academy of Management Review. 2003; 28 (2):275–296. doi: 10.2307/30040713. [ CrossRef ] [ Google Scholar ]
- Pfeffer J, Salancik GR. The external control of organizations: A resource dependence perspective. New York: Harper & Row; 1978. [ Google Scholar ]
- Philippi S, Schuhmacher M, Bastian N. Attracting investors in initial coin offerings: The relevance of specific technological capabilities for fundraising success. Review of Corporate Finance. 2021; 1 (3–4):455–485. doi: 10.1561/114.00000010. [ CrossRef ] [ Google Scholar ]
- Qi X, Chan JH, Hu J, Li Y. Motivations for selecting cross-border e-commerce as a foreign market entry mode. Industrial Marketing Management. 2020; 89 :50–60. doi: 10.1016/j.indmarman.2020.01.009. [ CrossRef ] [ Google Scholar ]
- Rindova VP, Kotha S. Continuous “morphing”: Competing through dynamic capabilities, form, and function. Academy of management journal. 2001; 44 (6):1263–1280. doi: 10.2307/3069400. [ CrossRef ] [ Google Scholar ]
- Röell, C., Osabutey, E., Rodgers, P., Arndt, F., Khan, Z., & Tarba, S. (2022). Managing socio-political risk at the subnational level: Lessons from MNE subsidiaries in Indonesia. Journal of World Business , 57 (3), p.101312
- Rugman AM, Verbeke A. Extending the theory of the multinational enterprise: Internalization and strategic management perspectives. Journal of International Business Studies. 2003; 34 (2):125–137. doi: 10.1057/palgrave.jibs.8400012. [ CrossRef ] [ Google Scholar ]
- Rugman AM. Inside the Multinationals: The Economics of Internal Markets. New York: Columbia University Press; 1981. [ Google Scholar ]
- Robinson, R. V., & Wahl, A. M. (1990). Industrial Employment and Wages of Women, Men, and Children in a 19th Century City: Indianapolis,1850–1880.American Sociological Review, 912–928
- Rossi A, Vanacker TR, Vismara S. Equity crowdfunding: New evidence from US and UK markets. Review of Corporate Finance. 2021; 1 (3–4):259–339. [ Google Scholar ]
- Shaheer NA, Li S. The CAGE around cyberspace? How digital innovations internationalize in a virtual world. Journal of Business Venturing. 2020; 35 (1):105892. doi: 10.1016/j.jbusvent.2018.08.002. [ CrossRef ] [ Google Scholar ]
- Sirmon DG, Hitt MA, Ireland RD, Gilbert BA. Resource orchestration to create competitive advantage: Breadth, depth, and life cycle effects. Journal of Management. 2011; 37 (5):1390–1412. doi: 10.1177/0149206310385695. [ CrossRef ] [ Google Scholar ]
- Sirmon DG, Hitt MA, Ireland RD. Managing firm resources in dynamic environments to create value: Looking inside the black box. Academy of Management Review. 2007; 32 (1):273–292. doi: 10.5465/amr.2007.23466005. [ CrossRef ] [ Google Scholar ]
- Stallkamp M, Schotter AP. Platforms without borders? The international strategies of digital platform firms. Global Strategy Journal. 2021; 11 (1):58–80. doi: 10.1002/gsj.1336. [ CrossRef ] [ Google Scholar ]
- Schu M, Morschett D, Swoboda B. Internationalization speed of online retailers: A resource-based perspective on the influence factors. Management International Review. 2016; 56 (5):733–757. doi: 10.1007/s11575-016-0279-6. [ CrossRef ] [ Google Scholar ]
- Shi Y, Wang T, Alwan LC. Analytics for cross-border e‐commerce: inventory risk management of an online fashion retailer. Decision Sciences. 2020; 51 (6):1347–1376. doi: 10.1111/deci.12429. [ CrossRef ] [ Google Scholar ]
- Shim Y, Shin DH. Analyzing China’s fintech industry from the perspective of actor–network theory. Telecommunications Policy. 2016; 40 (2–3):168–181. doi: 10.1016/j.telpol.2015.11.005. [ CrossRef ] [ Google Scholar ]
- Singh N, Kundu S. Explaining the growth of e-commerce corporations: An extension and application of the eclectic paradigm. Journal of International Business Studies. 2002; 33 (4):679–697. doi: 10.1057/palgrave.jibs.8491039. [ CrossRef ] [ Google Scholar ]
- Statista (2020). E-commerce worldwide. Retrieved May 20, 2022, https://www.statista.com/topics/871/online-shopping/
- Swoboda, B., & Sinning, C. (2022). Effects of Internationalization Rhythm and Speed on E-Commerce Firms’ Growth and the Role of Institutional Distances.Management International Review,1–33
- Teece, D. J. (2017). Dynamic capabilities and (digital) platform lifecycles. Entrepreneurship, innovation, and platforms . Emerald Publishing Limited
- Teece DJ. A dynamic capabilities-based entrepreneurial theory of the multinational enterprise. Journal of International Business Studies. 2014; 45 (1):8–37. doi: 10.1057/jibs.2013.54. [ CrossRef ] [ Google Scholar ]
- Teece DJ, Pisano G, Shuen A. Dynamic capabilities and strategic management. Strategic Management Journal. 1997; 18 (7):509–533. doi: 10.1002/(SICI)1097-0266(199708)18:7<509::AID-SMJ882>3.0.CO;2-Z. [ CrossRef ] [ Google Scholar ]
- Tolstoy D, Nordman ER, Hånell SM, Özbek N. The development of international e-commerce in retail SMEs: An effectuation perspective. Journal of World Business. 2021; 56 (3):101165. doi: 10.1016/j.jwb.2020.101165. [ CrossRef ] [ Google Scholar ]
- Ulrich D, Barney JB. Perspectives in organizations: Resource dependence, efficiency, and population. Academy of Management Review. 1984; 9 :471–481. doi: 10.5465/amr.1984.4279680. [ CrossRef ] [ Google Scholar ]
- Verbeke A, Kano L. An internalization theory perspective on the global and regional strategies of multinational enterprises. Journal of World Business. 2016; 51 (1):83–92. doi: 10.1016/j.jwb.2015.08.014. [ CrossRef ] [ Google Scholar ]
- Warner KS, Wäger M. Building dynamic capabilities for digital transformation: An ongoing process of strategic renewal. Long range planning. 2019; 52 (3):326–349. doi: 10.1016/j.lrp.2018.12.001. [ CrossRef ] [ Google Scholar ]
- Wu JH, Hisa TL. Developing e-business dynamic capabilities: an analysis of e-commerce innovation from I-, M-, to U-commerce. Journal of Organizational Computing and Electronic Commerce. 2008; 18 (2):95–111. doi: 10.1080/10919390701807525. [ CrossRef ] [ Google Scholar ]
- Yang N, Fu J, Wang Y. Study of the impact of the cross-border e-commerce model based on the belt and road on China’s international trade system. Revista de la Facultad de Ingeniería. 2017; 32 (8):490–496. [ Google Scholar ]
- Zeng J, Tavalaei MM, Khan Z. Sharing economy platform firms and their resource orchestration approaches. Journal of Business Research. 2021; 136 :451–465. doi: 10.1016/j.jbusres.2021.07.054. [ CrossRef ] [ Google Scholar ]
- Zeng J, Khan Z, De Silva M. The emergence of multi-sided platform MNEs: Internalization theory and networks. International Business Review. 2019; 28 (6):101598. doi: 10.1016/j.ibusrev.2019.101598. [ CrossRef ] [ Google Scholar ]
- Zhou W, Chong AYL, Zhen C, Bao H. E-supply chain integration adoption: examination of buyer–supplier relationships. Journal of Computer Information Systems. 2018; 58 (1):58–65. doi: 10.1080/08874417.2016.1189304. [ CrossRef ] [ Google Scholar ]
- Zhu L, Thatcher SM. National information ecology: A new institutional economics perspective on global e-commerce adoption. Journal of Electronic Commerce Research. 2010; 11 (1):53–72. [ Google Scholar ]

Top 8 e-Commerce Research Topic Ideas in 2024
Home Blog Career Top 8 e-Commerce Research Topic Ideas in 2024

In today's digital era, eCommerce has become a booming industry, offering convenience and accessibility to consumers worldwide. Among the plethora of eCommerce websites and apps, only a few manage to achieve significant recognition and success. Ever wondered what sets these successful ventures apart from the rest? The key lies in conducting effective and insightful research.
Research plays a pivotal role in determining the trajectory of an eCommerce project towards success. When done right, it becomes the driving force behind the growth and profitability of a business. However, the process of choosing suitable eCommerce research topics is not as simple as it may seem. As you delve into the vastness of the field, you'll realize the importance of finding the right focus.
So, how can you discover the perfect research topic for your eCommerce venture? The answer is straightforward - keep reading until the end! We've compiled a comprehensive list of compelling topics that can steer your research in the right direction and pave the way for your eCommerce business's success.
How is eCommerce Growing Important Every Day?
The eCommerce industry has undergone significant transformations over the past few years, and its growth hasn't gone unnoticed. The increasing importance of eCommerce can be attributed to several factors, including:
Expanding Customer Base : The advancement of eCommerce has led to a massive increase in the number of customers participating in online shopping. Understanding and analyzing the evolving trends and preferences of these newer customers have become essential for businesses.
Convenience for Customers : One of the primary reasons for the industry's growth is the convenience it offers to customers. Online shopping portals enable people to purchase their favorite products from the comfort of their homes, contributing to the industry's popularity.
Targeted Marketing Growth: The effectiveness of targeted marketing strategies has played a crucial role in eCommerce's success. From personalized messages addressing customers by name to tailored product recommendations, such tactics have significantly enhanced customer satisfaction and contributed to the industry's expansion.
Top E-commerce Research Ideas
To ensure your eCommerce research paper captures attention and relevance, it's essential to focus on the most pertinent topics in the industry. We've compiled a list of potential topics that can significantly enhance the chances of your research paper's success:
1. Role of Artificial Intelligence in Shaping Consumer Demand In E-commerce
Objective of the Paper: The topic emphasizes the importance of using Artificial Intelligence in shaping consumer demand in the e-commerce industry. It aims to explore how AI is utilized to provide personalized product recommendations, virtual assistants, dynamic pricing, chatbots, etc.
The paper aims to provide insights into the effectiveness of AI in influencing consumer decision-making, examining underlying mechanisms and assessing its impact on satisfaction, loyalty, and shopping experiences.
Why Choose this Topic?
The objective is to contribute towards a deeper understanding of the role of advanced AI in e-commerce and provide valuable insights for businesses and policymakers. This information will help policymakers make informed decisions and optimize their marketing strategies.
2. An Innovative E-commerce Platform Incorporating Metaverse to Live Commerce
Objective of the Paper: The paper's primary purpose is to explore the integration of the metaverse concept into e-commerce platforms and investigate its impact. The research would aim to understand how Metaverse can be leveraged to enhance and transform the typical e-commerce model.
Such e-commerce thesis topics aim to develop and assess an innovative e-commerce platform that incorporates elements like virtual reality, augmented reality, and immersive experiences into live commerce interactions. The research can also identify the hiccups one might face regarding technical requirements and ethical considerations when marrying e-commerce with Metaverse.
Metaverse has recently gained a lot of attention due to its vast advantages. If Metaverse is successfully implemented with live commerce, one can unleash the platform's full potential and use it to their advantage. This paper helps provide insights into the application of the Metaverse in creating innovative yet immersive platforms.
3. Autonomous Transaction Model For E-commerce Using Blockchain Technology
Objective of the Paper: This innovative topic helps investigate and develop a novel transaction model. The primary purpose of including blockchain in an e commerce research paper is to explore the potential of Blockchain in enabling autonomous transactions within the entire e-commerce ecosystem.
Using the study, one can design and implement an autonomous transaction model that utilizes Blockchain's decentralized and immutable nature. This would facilitate secure, transparent as well as efficient transactions in e-commerce. Such e-commerce research paper topics aim to discover how Blockchain can effectively streamline various aspects of eCommerce management , including order processing, inventory management, supply chain logistics, payment systems, etc.
This paper would significantly contribute to assessing the effectiveness of the autonomous transaction model in improving operational efficiency, cost-cutting, and mitigating funds and counterfeiting risks. This helps create a transparent e-commerce transaction ecosystem that various businesses can apply.
4. Product Advertising Recommendation in E-commerce Based on Deep Learning and Distributed Expression
Objective of the Paper: This paper helps explore and develop a sophisticated recommendation system for product advertising by utilizing deep learning techniques and distributed expression methods. The primary aim is to discover how deep learning algorithms can be applied to analyze vast amounts of data, user behavior, product information, contextual factors, etc.
One can develop recommendation systems that leverage deep learning models like CNNs, RNNs, or transformer models to get meaningful product images, descriptions, and user preferences. The research also evaluates the proposed recommendation system's performance, scalability, and privacy implications.
This paper would help gain valuable insights for companies and industries to optimize their marketing strategies which will ultimately help in improving the overall user experience.
5. Evaluating User Interface and Experience of VR in the Electronic Commerce Environment: A Hybrid Approach
Objective of the Paper: The purpose is to analyze and assess virtual reality's UI and UX aspects of virtual reality, one of the hottest topics of current times, in the context of electronic commerce. This research will help provide insights into the effectiveness and usability of VR technology in enhancing the shopping experience for consumers and the selling experience for traders.
The aim is to employ not a single method but a hybrid approach combining qualitative and quantitative research. This involves conducting user studies, usability testing, surveys, and even interviews to get hands-on data and participant feedback.
Conducting thorough research on this topic will help provide valuable information for designing and optimizing VR interfaces in e-commerce settings. Gaining the knowledge would help companies better fathom the potential of VR in e-commerce and identify areas of improvement.
6. Smart E-commerce Logistics Construction Model-based on Big Data Analytics
Objective of the Paper: To propose a probable advanced logistic construction model for e-commerce that uses big data analytics to optimize and enhance the efficiency of the entire logistics process.
The primary goal is to explore and identify how big data analytics can garner, analyze and extract essential information from vast logistics-related data in the e-commerce industry. The sources include order information, inventory data, transportation records, traffic patterns, etc.
Ultimately, the research would be used to develop an intelligent logistics construction model that could use big data analytics, including data mining machine learning, predictive analytics, and even optimization algorithms.
Why Choose this Paper?
By gathering information from real-time data, the model aims to improve accuracy, cut costs, minimize delays, and provide a seamless experience to the users. The paper would provide companies with information that would strengthen their logistics operation and help them meet the increasing demands and expectations of the eCommerce market.
7. Consumer Marketing Strategy and E-commerce in the Last Decade
Objective of the Paper: The paper helps analyze the changes in consumer marketing strategies over the last ten years. The aim is to explore the evolving trends in terms of consumer behavior, preferences, and expectations and how they have influenced marketing strategies.
The study also helps understand how digital technologies such as social media, mobile devices, and significant data analytics shape consumer behavior. It also helps recognize the best practices and successful marketing strategies various e-commerce businesses use to attract, engage and retain customers.
If one analyzes any latest research paper on e commerce, one will find data that talks about the various challenges and opportunities that businesses have faced and adopted in the last decade. Likewise, this paper will help develop and implement effective consumer marketing strategies in an e-commerce environment. It would more likely be a comprehensive guide that talks about the evolution of consumer marketing strategies and their relationship with e-commerce in the last decade.
8. E-commerce Opportunities in the 4.0 Era of Innovative Entrepreneurship Management Development
Objective of the Paper: The purpose is to identify the various unique opportunities that arise in e-commerce as per the Fourth Industrial Revolution. The paper investigates and analyzes the multiple dimensions of eCommerce in the 4.0 era, which includes various parameters like AI, IoT, Blockchain, cloud computing, and data analytics.
The primary goal of this paper is to understand how these advanced technologies can be implemented to enhance the e-commerce platform and enable new business models.
The paper also aims to find specific growth areas, such as cross-border e-commerce, mobile commerce, and social or platform-based business models.
The paper helps in providing valuable insights for budding entrepreneurs and established businesses. It helps to understand the innovative relationship between emerging technologies and e-commerce, resulting in a conducive and sustainable environment.
How To Write An Effective E-commerce Research Paper?
Some basic steps must be followed to write an influential e-commerce research paper. They are:
- Choose the Right Topic: Selecting a specific domain is necessary before writing a paper. Ensure the e commerce-related research topics are relevant to the current scenario and resonate with the market's current demand.
- Conduct Thorough Research: A research paper is nothing if it is just filled with some facts everyone can find in the newspapers and magazines. As a research scholar, you need to conduct thorough research and write the paper in a way that discusses even the most critical topics excitingly.
- Outline the Paper: Before writing, create an outline that will act as a guide throughout the research.
- Analyze and Interpret your Findings: Collecting data and merely representing it would not be enough. You must analyze your research findings and translate them into an actionable conclusion.
- Revise and Edit: Even after completing the paper, you must revise the whole thing to find mistakes, if any, are present while filling in the information.
You must always remember an e-commerce paper is only effective when it has the perfect balance between in-depth analysis, clarity of expression, and an engaging writing style.
In conclusion, the realm of eCommerce is experiencing rapid and dynamic changes, making it crucial for researchers and businesses to stay updated with the latest trends and topics. In 2024 and beyond, the key to success lies in exploring intriguing research ideas that hold the potential to revolutionize customer engagement, satisfaction, and conversion rates.
The first four research topics open up numerous opportunities for delving into customer-centric approaches, allowing businesses to better understand their target audience and cater to their needs effectively. Furthermore, the fifth and sixth topics emphasize the importance of efficient logistics and supply chain management, ensuring seamless delivery solutions and practices to meet customer expectations.
The final two topics highlight the indispensable role of data analytics and AI in the eCommerce landscape. Leveraging advanced technologies in these areas can drive better decision-making, fraud detection, and overall business efficiency.
By embracing these research areas and selecting the right eCommerce research title, researchers can gain valuable insights that will help them thrive in the ever-evolving world of eCommerce. Embracing innovation and staying attuned to the shifting industry trends will be key in achieving success in this fast-paced and competitive market.
Elevate your career with the highest salary jobs in world. Achieve your dreams and secure your financial future. The journey to top earnings starts now!
Frequently Asked Questions (FAQs)
The best way to choose a topic in commerce is to research and find out the most searched topics of the current times. This homework will help you understand the market's current demands and choose your topic accordingly.
In the simplest terms, e-commerce market research is the process of collecting and studying data and information related to the e-commerce industry.
If we consider previous data, the B2C model is the most successful form of eCommerce as the B2C model targets individual consumers rather than businesses providing a more significant customer base.

Abhresh Sugandhi
Abhresh is specialized as a corporate trainer, He has a decade of experience in technical training blended with virtual webinars and instructor-led session created courses, tutorials, and articles for organizations. He is also the founder of Nikasio.com, which offers multiple services in technical training, project consulting, content development, etc.
Avail your free 1:1 mentorship session.
Something went wrong
Invest in Your Future: Upcoming High-Paying Career Courses to Consider
Name | Date | Fee | Know more |
---|

Indian Journal of Commerce and Management Studies
About the journal.
For the past ten years, We, Educational Research Multimedia and Publications, India exhibited true commitment and excellence in inculcating high-quality research. We are a proud publisher of research contributions from global authors in the areas of Arts, Commerce, Management, Education and Social Sciences. Being headed by a team of Eminent Scientists, Professors, Professionals, Researchers, Key Opinion Leaders, Industrialists, our board of management holds 25+ years of collective experience in publishing research and educational materials. Our publications are open-access, double-blind peer-reviewed, plagiarism-free, updated and application-oriented that suit the current trends.
Indian Journal of Commerce & Management Studies is owned and managed by Educational Research Multimedia and Publications, India. IJCMS is an open access peer review Tri-Annual research journal that publishes articles in the field of commerce & management. The journal serves as a bridge between worldwide scholars and commerce and management studies researchers. The journal publishes research articles and papers in all areas of commerce and management. The journal aims to provide the most complete and reliable source of information on recent developments in business studies. Each issue brings you critical perspectives and cogent analyses, serving as an outlet for the best theoretical and research work in the field. The purpose of the journal is to further the understanding of the theory and practice of business studies by publishing articles of interest to practitioners and scholars.
Call for Papers - Volume XV, Issue 3, September 2024
Please submit to [email protected] or Click here as per Submission Guidelines
Current Issue
Blockchain technology and its importance for the growth and prosperity of indian small and medium-sized enterprises, innovation management among the indian small and medium-sized enterprises focusing on artificial intelligence: opportunities and the way forward, sustainability in startups/small and medium-sized enterprises: the influential role of entrepreneurial leadership and innovative behavior.
Online ISSN : 2229-5674 Print ISSN : 2249-0310
Tri-Annual (January, May & September)
crossref-doi
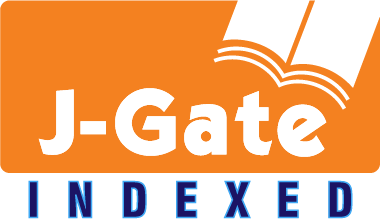
Information
- For Readers
- For Authors
- For Librarians
Make a Submission
Editorial Office:
Educational Research Multimedia & Publications, S.N. 21, Plot No 24, Mirza Ghalib Road Malegaon Nasik, Maharashtra India - 423203. +912554235588, +919764558895 [email protected] www.ijcms.in
Copyrights © 2010-2024 - ERM Publications, India
This work is licensed under https://creativecommons.org/licenses/by-sa/4.0/

The Effect of E-commerce Expansion on Local Retail
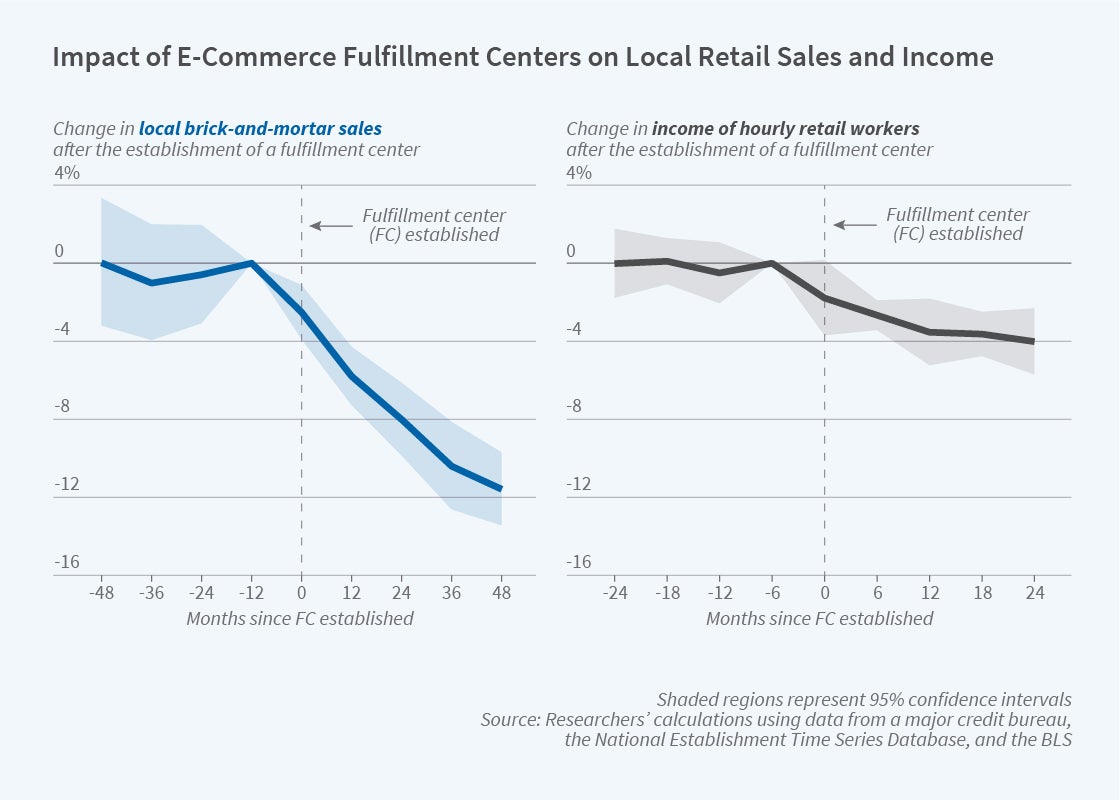
E -commerce has dramatically altered retail in the last two decades, with online sales growing from 0.63 percent of total retail sales in 1999 to 13.3 percent in 2021. In Creative Destruction? Impact of E-commerce on the Retail Sector (NBER Working Paper 30077), Sudheer Chava , Alexander Oettl , Manpreet Singh , and Linghang Zeng study how the rise of online selling has affected brick-and-mortar retail establishments and their employees.
The researchers measure the presence of e-commerce in an area using the staggered rollout of a major e-commerce retailer’s fulfillment centers across the United States. This firm had only three such centers in 2000, but more than 90 by the end of 2016. Establishing a center reduces shipment delivery times in surrounding areas, which may encourage local consumers to shift towards e-commerce and away from traditional brick-and-mortar retailers. The researchers study the trends in retail outcomes before and after a fulfillment center is established in proximate counties, and compare them with the trends in a control group of counties near where centers would be built at a later date.
Establishment of an e-commerce fulfillment center reduces retail employment growth in the host county by an average of almost 1,000 jobs per quarter.
The researchers first use a detailed employer-employee payroll dataset covering 2.6 million retail workers to explore how e-commerce growth affects traditional retail workers. The establishment of a fulfillment center is associated with a drop of 2.5 percent on average in the income of hourly retail workers in the same county, or about $825 annually. The income drop is similar for retail workers in counties within 100 miles of the center, but is negligible beyond that distance. Hourly workers’ income losses arise primarily from a reduction in hours worked.
A new fulfillment center is not associated with lower income among salaried retail workers, and the impacts of e-commerce on hourly workers’ income vary substantially with worker characteristics. Income falls the most among the youngest and oldest workers, with income falling by 3.6 percent among workers over age 64. Workers with longer tenure at retail firms see smaller losses in income, and income losses are larger among part-time workers. Income losses are largest among workers in general-merchandise and home-improvement stores, two retail subsectors where customers may place less value on their in-store experience.
The researchers next use National Establishment Time Series data to examine the impact of e-commerce fulfillment centers on traditional retail stores. Sales in the average brick-and-mortar retail store fall by about 4 percent after a local fulfillment center opens. Stores react to these drops by reducing hours per worker and by cutting employee headcount by 2.1 percent on average, or about 36 workers per 100 stores. The opening of a fulfillment center coincides with a 3 percentage point increase in the annual likelihood that brick-and-mortar stores close. The largest impacts are found among smaller and newer stores. Likewise, new stores’ rate of entry falls by 8.1 percentage points within 100 miles of a new center.
Finally, the researchers use data from the Quarterly Census of Employment and Wages to examine how e-commerce expansion affects retail employment and wage growth at the county level. Establishment of a fulfillment center is associated with reduced employment growth in retail of 2.9 percent within the same county, a loss of about 938 jobs per county per quarter, with smaller losses within a 100-mile radius. These retail losses are partially offset by increased local employment in transportation and warehousing, which gain about 256 jobs, and in restaurants, which gain about 143 jobs. Opening a new fulfillment center also coincides with increased wage growth in warehousing.
— Lucy E. Page
Researchers
Working groups.
NBER periodicals and newsletters may be reproduced freely with appropriate attribution.
More from NBER
In addition to working papers , the NBER disseminates affiliates’ latest findings through a range of free periodicals — the NBER Reporter , the NBER Digest , the Bulletin on Retirement and Disability , the Bulletin on Health , and the Bulletin on Entrepreneurship — as well as online conference reports , video lectures , and interviews .
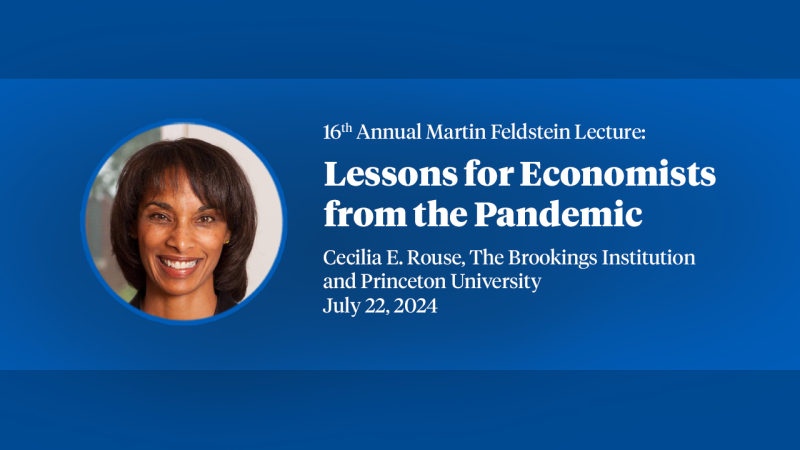
© 2023 National Bureau of Economic Research. Periodical content may be reproduced freely with appropriate attribution.
New Trends in E-Commerce Research: Linking Social Commerce and Sharing Commerce: A Systematic Literature Review
- November 2022
- Sustainability 14(23):16024
- 14(23):16024

- (Princess Nourah Bint Abdul Rahman University)

- Princess Nora bint Abdul Rahman University
- This person is not on ResearchGate, or hasn't claimed this research yet.

- Loughborough University
Abstract and Figures

Discover the world's research
- 25+ million members
- 160+ million publication pages
- 2.3+ billion citations

- Alaa M. S. Azazz

- Rein Suadamara
- Choerun Nisa Salsa Bila
- Yono Maulana

- Surjit Singha

- Maria Kocot

- Nikolaj Ambrusevič
- Xintian Wang
- Caiming Zhang
- Huang Xiang
- Ka Yin Chau

- Vishal Dagar
- J GLOB INF MANAG

- Larasati Ahluwalia
- Yen-Hao Hsieh
- Pejman Ebrahimi

- INT J INFORM MANAGE

- Tiago da Costa

- Warniancy Ariesty

- Lucian L. Visinescu

- Marcos Sivitanides
- Recruit researchers
- Join for free
- Login Email Tip: Most researchers use their institutional email address as their ResearchGate login Password Forgot password? Keep me logged in Log in or Continue with Google Welcome back! Please log in. Email · Hint Tip: Most researchers use their institutional email address as their ResearchGate login Password Forgot password? Keep me logged in Log in or Continue with Google No account? Sign up
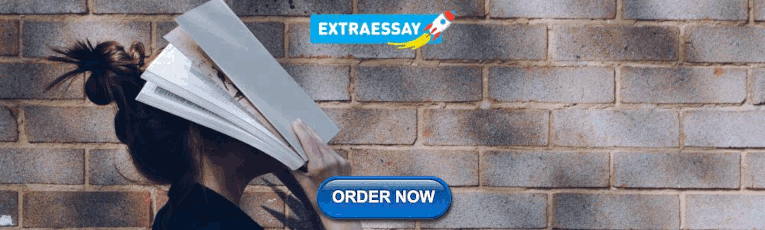
COMMENTS
Business and commerce articles from across Nature Portfolio. Business and management. Economics. Finance. Information systems and information technology. Operational research.
Explore 100 research topics in commerce field, from finance and marketing to emerging trends, offering insights into the business landscape.
This article reviews the literature on e-commerce logistics and proposes a framework for omni-channel decision making. Learn how to optimize your online business operations and customer satisfaction.
The Journal of Business Research aims to publish research that is rigorous, relevant, and potentially impactful. Recognizing the intricate relationships between the many areas of business activity, JBR examines a wide variety of business decision contexts, processes and activities, developing …. View full aims & scope.
Electronic Commerce Research is a peer-reviewed journal that disseminates the latest findings in all aspects of e-commerce. Explores core enabling technologies and their societal, economic, and individual implications. Publishes theoretical and empirical research contributing to a better understanding of electronic commerce.
Electronic Commerce Research is a peer-reviewed journal that disseminates the latest findings in all aspects of e-commerce. Explores core enabling ...
This article proposes a novel model to evaluate and improve E-commerce industries based on benefits, challenges, and pathways. Learn how to apply it in real cases.
PDF | Electronic commerce has become a prominent channel for which traditional and novel businesses reach out to consumers for selling their products.... | Find, read and cite all the research you ...
Despite the exponential growth of e-commerce in South Africa (Dennis & Piatti, 2015 ), research has not yet been conducted to identify and examine the online shopping attributes that affect customer satisfaction and loyalty, moderated by e-commerce experience, which is a unique contribution of this study.
In this article we provide you latest research topics for COMMERCE with full Phd thesis. By these research topics for COMMERCE you can get idea for your research work.
Looking for good e-commerce marketing articles to read? Here are 7 of the best e-commerce marketing articles we recommend.
Explore the concepts of research topics and 21+ best and interesting research topics for commerce students. Choose the best topic and start your research!
This paper synthesises research on artificial intelligence (AI) in e-commerce and proposes guidelines on how information systems (IS) research could contribute to this research stream. To this end, the innovative approach of combining bibliometric analysis with an extensive literature review was used. Bibliometric data from 4335 documents were analysed, and 229 articles published in leading IS ...
Design/Methodology/Approach: A systematic and rigorous methodology was employed to identify relevant scholarly articles and research studies related to consumer decision-making in e-commerce.
Analyzing the Spectacular Rise of E-Commerce and Online Delivery Services: The Road Ahead in India. This research paper has been undertaken to dissect the growth trajectory of e-commerce in India and understand its significance in conjunction with the SARS-CoV-2 outbreak across the World.
In this article, the authors suggest that while e-commerce will continue to be an essential element of retail strategy, the future success of retailers will ultimately depend on creating a ...
This research aims to investigate the multifaceted effects of the growing e-commerce sector on traditional retail businesses, considering economic, social, and policy dimensions.
However, since social commerce has grown rapidly in recent years, it is vital to incorporate new clues from current research in this field. This study provides a systematic review of social commerce and online consumer behavior.
In the first article of this focused issue, Ahi et al. (2022), emphasize the issues related to policy and the development of international e-commerce. They argue that, in terms of data protection, legal protection, and business laws, governmental policy influences the adoption of e-commerce.
Top E-commerce Research Ideas. To ensure your eCommerce research paper captures attention and relevance, it's essential to focus on the most pertinent topics in the industry. We've compiled a list of potential topics that can significantly enhance the chances of your research paper's success: 1. Role of Artificial Intelligence in Shaping ...
The journal publishes research articles and papers in all areas of commerce and management. The journal aims to provide the most complete and reliable source of information on recent developments in business studies.
The Effect of E-commerce Expansion on Local Retail. 08/01/2022. Summary of working paper 30077. Featured in print Digest. E -commerce has dramatically altered retail in the last two decades, with online sales growing from 0.63 percent of total retail sales in 1999 to 13.3 percent in 2021.
Focussing on this aspect, this study conducts a systematic review to identify research questions relevant to S-Commerce and sharing commerce, related challenges, and the benefits of linking S ...