Quantitative Data Analysis: A Comprehensive Guide
By: Ofem Eteng | Published: May 18, 2022
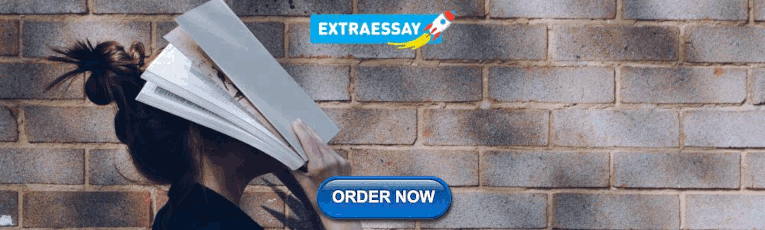
Related Articles
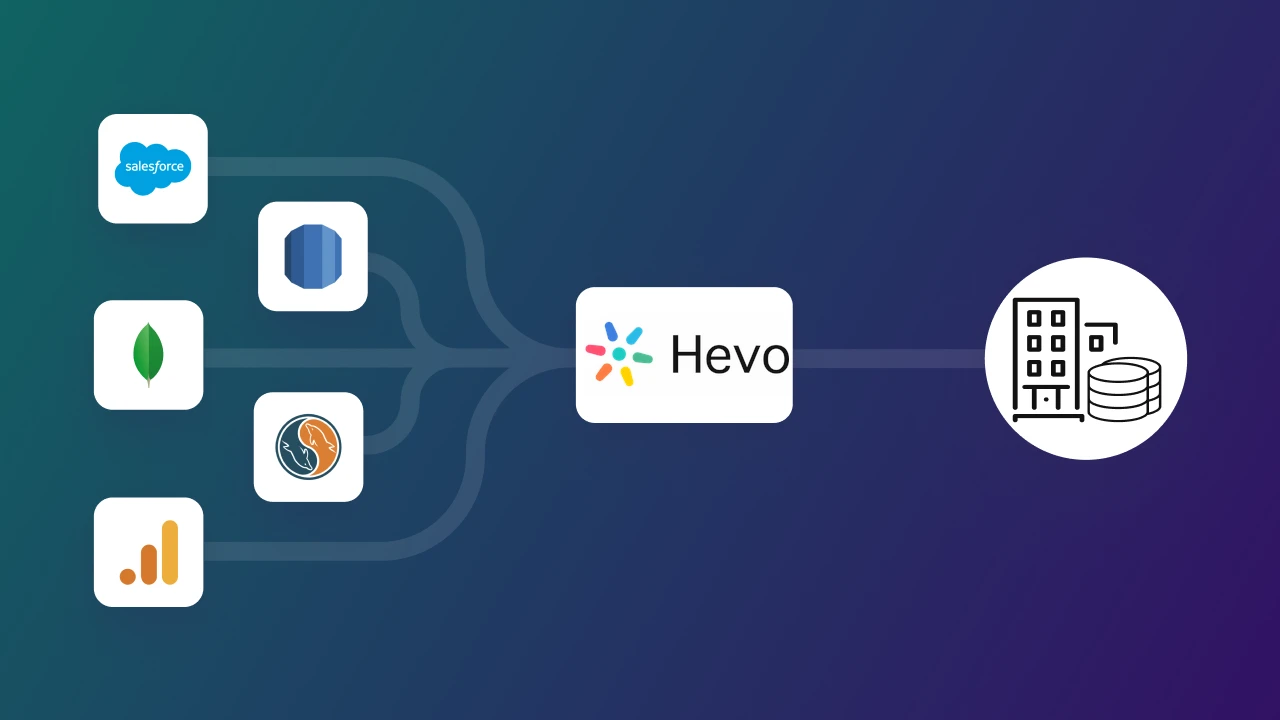
A healthcare giant successfully introduces the most effective drug dosage through rigorous statistical modeling, saving countless lives. A marketing team predicts consumer trends with uncanny accuracy, tailoring campaigns for maximum impact.
Table of Contents
These trends and dosages are not just any numbers but are a result of meticulous quantitative data analysis. Quantitative data analysis offers a robust framework for understanding complex phenomena, evaluating hypotheses, and predicting future outcomes.
In this blog, we’ll walk through the concept of quantitative data analysis, the steps required, its advantages, and the methods and techniques that are used in this analysis. Read on!
What is Quantitative Data Analysis?
Quantitative data analysis is a systematic process of examining, interpreting, and drawing meaningful conclusions from numerical data. It involves the application of statistical methods, mathematical models, and computational techniques to understand patterns, relationships, and trends within datasets.
Quantitative data analysis methods typically work with algorithms, mathematical analysis tools, and software to gain insights from the data, answering questions such as how many, how often, and how much. Data for quantitative data analysis is usually collected from close-ended surveys, questionnaires, polls, etc. The data can also be obtained from sales figures, email click-through rates, number of website visitors, and percentage revenue increase.
Ditch the manual process of writing long commands to migrate your data and choose Hevo’s no-code platform to streamline your migration process to get analysis-ready data .
- Transform your data for analysis with features like drag and drop and custom Python scripts.
- 150+ connectors , including 60+ free sources.
- Eliminate the need for manual schema mapping with the auto-mapping feature.
Try Hevo and discover how companies like EdApp have chosen Hevo over tools like Stitch to “build faster and more granular in-app reporting for their customers.”
Quantitative Data Analysis vs Qualitative Data Analysis
When we talk about data, we directly think about the pattern, the relationship, and the connection between the datasets – analyzing the data in short. Therefore when it comes to data analysis, there are broadly two types – Quantitative Data Analysis and Qualitative Data Analysis.
Quantitative data analysis revolves around numerical data and statistics, which are suitable for functions that can be counted or measured. In contrast, qualitative data analysis includes description and subjective information – for things that can be observed but not measured.
Let us differentiate between Quantitative Data Analysis and Quantitative Data Analysis for a better understanding.
Numerical data – statistics, counts, metrics measurements | Text data – customer feedback, opinions, documents, notes, audio/video recordings | |
Close-ended surveys, polls and experiments. | Open-ended questions, descriptive interviews | |
What? How much? Why (to a certain extent)? | How? Why? What are individual experiences and motivations? | |
Statistical programming software like R, Python, SAS and Data visualization like Tableau, Power BI | NVivo, Atlas.ti for qualitative coding. Word processors and highlighters – Mindmaps and visual canvases | |
Best used for large sample sizes for quick answers. | Best used for small to middle sample sizes for descriptive insights |
Data Preparation Steps for Quantitative Data Analysis
Quantitative data has to be gathered and cleaned before proceeding to the stage of analyzing it. Below are the steps to prepare a data before quantitative research analysis:
- Step 1: Data Collection
Before beginning the analysis process, you need data. Data can be collected through rigorous quantitative research, which includes methods such as interviews, focus groups, surveys, and questionnaires.
- Step 2: Data Cleaning
Once the data is collected, begin the data cleaning process by scanning through the entire data for duplicates, errors, and omissions. Keep a close eye for outliers (data points that are significantly different from the majority of the dataset) because they can skew your analysis results if they are not removed.
This data-cleaning process ensures data accuracy, consistency and relevancy before analysis.
- Step 3: Data Analysis and Interpretation
Now that you have collected and cleaned your data, it is now time to carry out the quantitative analysis. There are two methods of quantitative data analysis, which we will discuss in the next section.
However, if you have data from multiple sources, collecting and cleaning it can be a cumbersome task. This is where Hevo Data steps in. With Hevo, extracting, transforming, and loading data from source to destination becomes a seamless task, eliminating the need for manual coding. This not only saves valuable time but also enhances the overall efficiency of data analysis and visualization, empowering users to derive insights quickly and with precision
Now that you are familiar with what quantitative data analysis is and how to prepare your data for analysis, the focus will shift to the purpose of this article, which is to describe the methods and techniques of quantitative data analysis.
Methods and Techniques of Quantitative Data Analysis
Quantitative data analysis employs two techniques to extract meaningful insights from datasets, broadly. The first method is descriptive statistics, which summarizes and portrays essential features of a dataset, such as mean, median, and standard deviation.
Inferential statistics, the second method, extrapolates insights and predictions from a sample dataset to make broader inferences about an entire population, such as hypothesis testing and regression analysis.
An in-depth explanation of both the methods is provided below:
- Descriptive Statistics
- Inferential Statistics
1) Descriptive Statistics
Descriptive statistics as the name implies is used to describe a dataset. It helps understand the details of your data by summarizing it and finding patterns from the specific data sample. They provide absolute numbers obtained from a sample but do not necessarily explain the rationale behind the numbers and are mostly used for analyzing single variables. The methods used in descriptive statistics include:
- Mean: This calculates the numerical average of a set of values.
- Median: This is used to get the midpoint of a set of values when the numbers are arranged in numerical order.
- Mode: This is used to find the most commonly occurring value in a dataset.
- Percentage: This is used to express how a value or group of respondents within the data relates to a larger group of respondents.
- Frequency: This indicates the number of times a value is found.
- Range: This shows the highest and lowest values in a dataset.
- Standard Deviation: This is used to indicate how dispersed a range of numbers is, meaning, it shows how close all the numbers are to the mean.
- Skewness: It indicates how symmetrical a range of numbers is, showing if they cluster into a smooth bell curve shape in the middle of the graph or if they skew towards the left or right.

2) Inferential Statistics
In quantitative analysis, the expectation is to turn raw numbers into meaningful insight using numerical values, and descriptive statistics is all about explaining details of a specific dataset using numbers, but it does not explain the motives behind the numbers; hence, a need for further analysis using inferential statistics.
Inferential statistics aim to make predictions or highlight possible outcomes from the analyzed data obtained from descriptive statistics. They are used to generalize results and make predictions between groups, show relationships that exist between multiple variables, and are used for hypothesis testing that predicts changes or differences.
There are various statistical analysis methods used within inferential statistics; a few are discussed below.
- Cross Tabulations: Cross tabulation or crosstab is used to show the relationship that exists between two variables and is often used to compare results by demographic groups. It uses a basic tabular form to draw inferences between different data sets and contains data that is mutually exclusive or has some connection with each other. Crosstabs help understand the nuances of a dataset and factors that may influence a data point.
- Regression Analysis: Regression analysis estimates the relationship between a set of variables. It shows the correlation between a dependent variable (the variable or outcome you want to measure or predict) and any number of independent variables (factors that may impact the dependent variable). Therefore, the purpose of the regression analysis is to estimate how one or more variables might affect a dependent variable to identify trends and patterns to make predictions and forecast possible future trends. There are many types of regression analysis, and the model you choose will be determined by the type of data you have for the dependent variable. The types of regression analysis include linear regression, non-linear regression, binary logistic regression, etc.
- Monte Carlo Simulation: Monte Carlo simulation, also known as the Monte Carlo method, is a computerized technique of generating models of possible outcomes and showing their probability distributions. It considers a range of possible outcomes and then tries to calculate how likely each outcome will occur. Data analysts use it to perform advanced risk analyses to help forecast future events and make decisions accordingly.
- Analysis of Variance (ANOVA): This is used to test the extent to which two or more groups differ from each other. It compares the mean of various groups and allows the analysis of multiple groups.
- Factor Analysis: A large number of variables can be reduced into a smaller number of factors using the factor analysis technique. It works on the principle that multiple separate observable variables correlate with each other because they are all associated with an underlying construct. It helps in reducing large datasets into smaller, more manageable samples.
- Cohort Analysis: Cohort analysis can be defined as a subset of behavioral analytics that operates from data taken from a given dataset. Rather than looking at all users as one unit, cohort analysis breaks down data into related groups for analysis, where these groups or cohorts usually have common characteristics or similarities within a defined period.
- MaxDiff Analysis: This is a quantitative data analysis method that is used to gauge customers’ preferences for purchase and what parameters rank higher than the others in the process.
- Cluster Analysis: Cluster analysis is a technique used to identify structures within a dataset. Cluster analysis aims to be able to sort different data points into groups that are internally similar and externally different; that is, data points within a cluster will look like each other and different from data points in other clusters.
- Time Series Analysis: This is a statistical analytic technique used to identify trends and cycles over time. It is simply the measurement of the same variables at different times, like weekly and monthly email sign-ups, to uncover trends, seasonality, and cyclic patterns. By doing this, the data analyst can forecast how variables of interest may fluctuate in the future.
- SWOT analysis: This is a quantitative data analysis method that assigns numerical values to indicate strengths, weaknesses, opportunities, and threats of an organization, product, or service to show a clearer picture of competition to foster better business strategies
How to Choose the Right Method for your Analysis?
Choosing between Descriptive Statistics or Inferential Statistics can be often confusing. You should consider the following factors before choosing the right method for your quantitative data analysis:
1. Type of Data
The first consideration in data analysis is understanding the type of data you have. Different statistical methods have specific requirements based on these data types, and using the wrong method can render results meaningless. The choice of statistical method should align with the nature and distribution of your data to ensure meaningful and accurate analysis.
2. Your Research Questions
When deciding on statistical methods, it’s crucial to align them with your specific research questions and hypotheses. The nature of your questions will influence whether descriptive statistics alone, which reveal sample attributes, are sufficient or if you need both descriptive and inferential statistics to understand group differences or relationships between variables and make population inferences.
Pros and Cons of Quantitative Data Analysis
1. Objectivity and Generalizability:
- Quantitative data analysis offers objective, numerical measurements, minimizing bias and personal interpretation.
- Results can often be generalized to larger populations, making them applicable to broader contexts.
Example: A study using quantitative data analysis to measure student test scores can objectively compare performance across different schools and demographics, leading to generalizable insights about educational strategies.
2. Precision and Efficiency:
- Statistical methods provide precise numerical results, allowing for accurate comparisons and prediction.
- Large datasets can be analyzed efficiently with the help of computer software, saving time and resources.
Example: A marketing team can use quantitative data analysis to precisely track click-through rates and conversion rates on different ad campaigns, quickly identifying the most effective strategies for maximizing customer engagement.
3. Identification of Patterns and Relationships:
- Statistical techniques reveal hidden patterns and relationships between variables that might not be apparent through observation alone.
- This can lead to new insights and understanding of complex phenomena.
Example: A medical researcher can use quantitative analysis to pinpoint correlations between lifestyle factors and disease risk, aiding in the development of prevention strategies.
1. Limited Scope:
- Quantitative analysis focuses on quantifiable aspects of a phenomenon , potentially overlooking important qualitative nuances, such as emotions, motivations, or cultural contexts.
Example: A survey measuring customer satisfaction with numerical ratings might miss key insights about the underlying reasons for their satisfaction or dissatisfaction, which could be better captured through open-ended feedback.
2. Oversimplification:
- Reducing complex phenomena to numerical data can lead to oversimplification and a loss of richness in understanding.
Example: Analyzing employee productivity solely through quantitative metrics like hours worked or tasks completed might not account for factors like creativity, collaboration, or problem-solving skills, which are crucial for overall performance.
3. Potential for Misinterpretation:
- Statistical results can be misinterpreted if not analyzed carefully and with appropriate expertise.
- The choice of statistical methods and assumptions can significantly influence results.
This blog discusses the steps, methods, and techniques of quantitative data analysis. It also gives insights into the methods of data collection, the type of data one should work with, and the pros and cons of such analysis.
Gain a better understanding of data analysis with these essential reads:
- Data Analysis and Modeling: 4 Critical Differences
- Exploratory Data Analysis Simplified 101
- 25 Best Data Analysis Tools in 2024
Carrying out successful data analysis requires prepping the data and making it analysis-ready. That is where Hevo steps in.
Want to give Hevo a try? Sign Up for a 14-day free trial and experience the feature-rich Hevo suite first hand. You may also have a look at the amazing Hevo price , which will assist you in selecting the best plan for your requirements.
Share your experience of understanding Quantitative Data Analysis in the comment section below! We would love to hear your thoughts.
Ofem Eteng is a seasoned technical content writer with over 12 years of experience. He has held pivotal roles such as System Analyst (DevOps) at Dagbs Nigeria Limited and Full-Stack Developer at Pedoquasphere International Limited. He specializes in data science, data analytics and cutting-edge technologies, making him an expert in the data industry.
No-code Data Pipeline for your Data Warehouse
- Data Analysis
- Data Warehouse
- Quantitative Data Analysis
Continue Reading
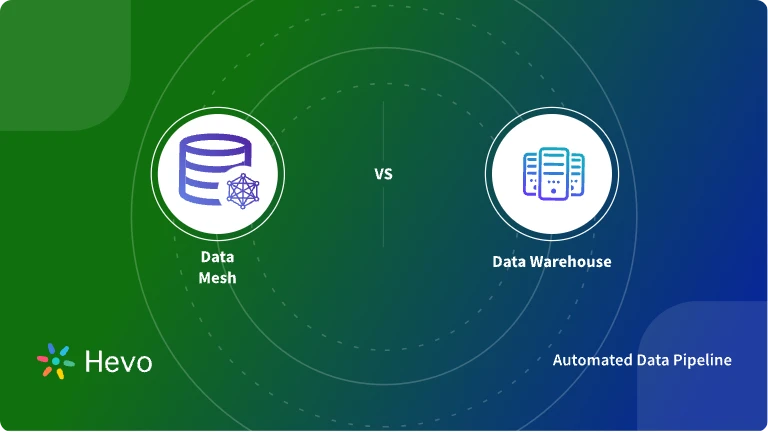
Data Mesh vs Data Warehouse: A Guide to Choosing the Right Data Architecture
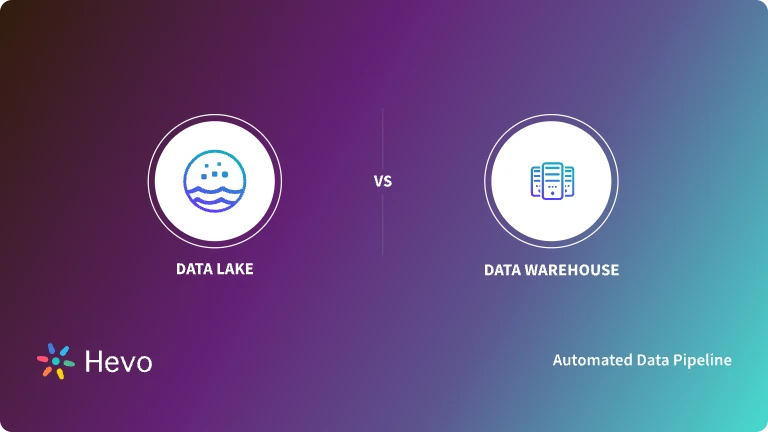
Vinita Mittal
Data Lake vs Data Warehouse: How to choose?
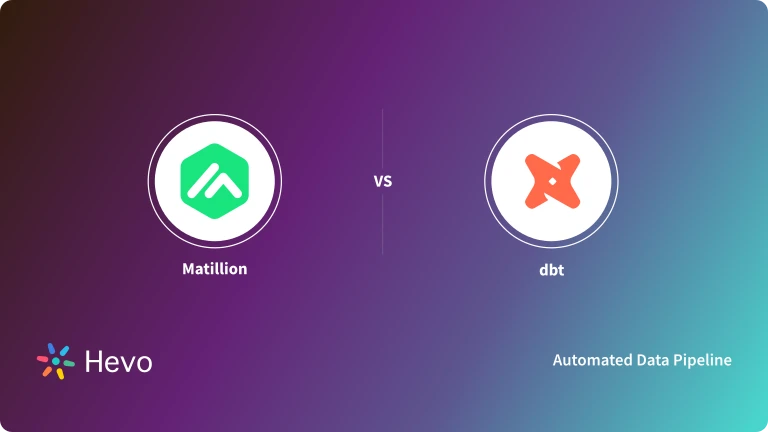
Rashmi Joshi
Matillion vs dbt: 5 Key Differences
I want to read this e-book.

Quantitative Data Analysis 101
The lingo, methods and techniques, explained simply.
By: Derek Jansen (MBA) and Kerryn Warren (PhD) | December 2020
Quantitative data analysis is one of those things that often strikes fear in students. It’s totally understandable – quantitative analysis is a complex topic, full of daunting lingo , like medians, modes, correlation and regression. Suddenly we’re all wishing we’d paid a little more attention in math class…
The good news is that while quantitative data analysis is a mammoth topic, gaining a working understanding of the basics isn’t that hard , even for those of us who avoid numbers and math . In this post, we’ll break quantitative analysis down into simple , bite-sized chunks so you can approach your research with confidence.
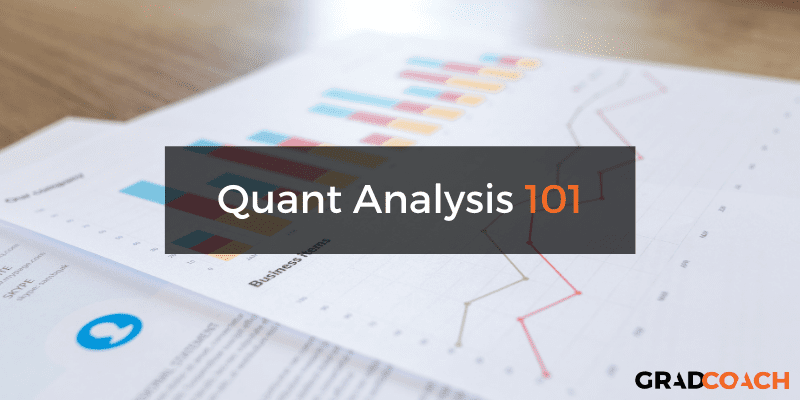
Overview: Quantitative Data Analysis 101
- What (exactly) is quantitative data analysis?
- When to use quantitative analysis
- How quantitative analysis works
The two “branches” of quantitative analysis
- Descriptive statistics 101
- Inferential statistics 101
- How to choose the right quantitative methods
- Recap & summary
What is quantitative data analysis?
Despite being a mouthful, quantitative data analysis simply means analysing data that is numbers-based – or data that can be easily “converted” into numbers without losing any meaning.
For example, category-based variables like gender, ethnicity, or native language could all be “converted” into numbers without losing meaning – for example, English could equal 1, French 2, etc.
This contrasts against qualitative data analysis, where the focus is on words, phrases and expressions that can’t be reduced to numbers. If you’re interested in learning about qualitative analysis, check out our post and video here .
What is quantitative analysis used for?
Quantitative analysis is generally used for three purposes.
- Firstly, it’s used to measure differences between groups . For example, the popularity of different clothing colours or brands.
- Secondly, it’s used to assess relationships between variables . For example, the relationship between weather temperature and voter turnout.
- And third, it’s used to test hypotheses in a scientifically rigorous way. For example, a hypothesis about the impact of a certain vaccine.
Again, this contrasts with qualitative analysis , which can be used to analyse people’s perceptions and feelings about an event or situation. In other words, things that can’t be reduced to numbers.
How does quantitative analysis work?
Well, since quantitative data analysis is all about analysing numbers , it’s no surprise that it involves statistics . Statistical analysis methods form the engine that powers quantitative analysis, and these methods can vary from pretty basic calculations (for example, averages and medians) to more sophisticated analyses (for example, correlations and regressions).
Sounds like gibberish? Don’t worry. We’ll explain all of that in this post. Importantly, you don’t need to be a statistician or math wiz to pull off a good quantitative analysis. We’ll break down all the technical mumbo jumbo in this post.
Need a helping hand?
As I mentioned, quantitative analysis is powered by statistical analysis methods . There are two main “branches” of statistical methods that are used – descriptive statistics and inferential statistics . In your research, you might only use descriptive statistics, or you might use a mix of both , depending on what you’re trying to figure out. In other words, depending on your research questions, aims and objectives . I’ll explain how to choose your methods later.
So, what are descriptive and inferential statistics?
Well, before I can explain that, we need to take a quick detour to explain some lingo. To understand the difference between these two branches of statistics, you need to understand two important words. These words are population and sample .
First up, population . In statistics, the population is the entire group of people (or animals or organisations or whatever) that you’re interested in researching. For example, if you were interested in researching Tesla owners in the US, then the population would be all Tesla owners in the US.
However, it’s extremely unlikely that you’re going to be able to interview or survey every single Tesla owner in the US. Realistically, you’ll likely only get access to a few hundred, or maybe a few thousand owners using an online survey. This smaller group of accessible people whose data you actually collect is called your sample .
So, to recap – the population is the entire group of people you’re interested in, and the sample is the subset of the population that you can actually get access to. In other words, the population is the full chocolate cake , whereas the sample is a slice of that cake.
So, why is this sample-population thing important?
Well, descriptive statistics focus on describing the sample , while inferential statistics aim to make predictions about the population, based on the findings within the sample. In other words, we use one group of statistical methods – descriptive statistics – to investigate the slice of cake, and another group of methods – inferential statistics – to draw conclusions about the entire cake. There I go with the cake analogy again…
With that out the way, let’s take a closer look at each of these branches in more detail.
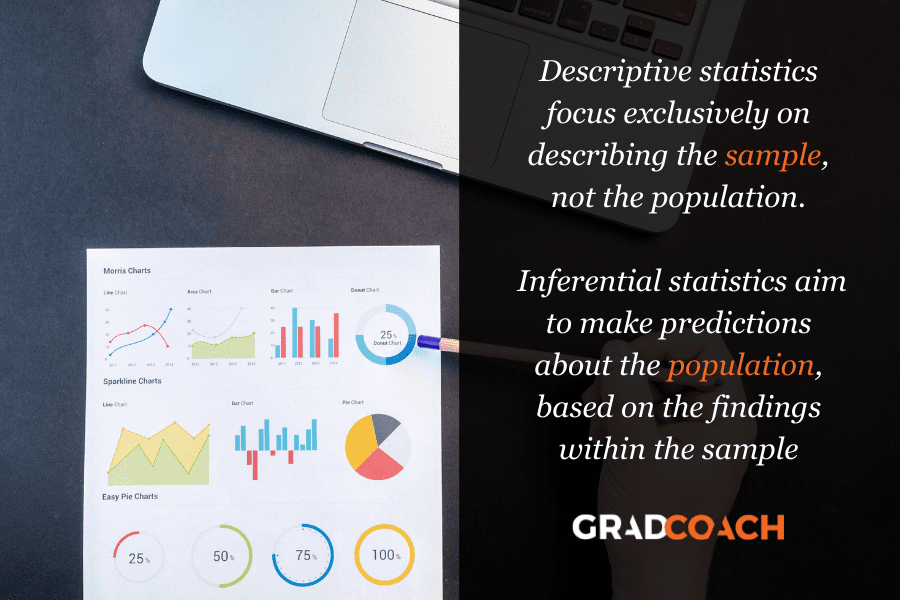
Branch 1: Descriptive Statistics
Descriptive statistics serve a simple but critically important role in your research – to describe your data set – hence the name. In other words, they help you understand the details of your sample . Unlike inferential statistics (which we’ll get to soon), descriptive statistics don’t aim to make inferences or predictions about the entire population – they’re purely interested in the details of your specific sample .
When you’re writing up your analysis, descriptive statistics are the first set of stats you’ll cover, before moving on to inferential statistics. But, that said, depending on your research objectives and research questions , they may be the only type of statistics you use. We’ll explore that a little later.
So, what kind of statistics are usually covered in this section?
Some common statistical tests used in this branch include the following:
- Mean – this is simply the mathematical average of a range of numbers.
- Median – this is the midpoint in a range of numbers when the numbers are arranged in numerical order. If the data set makes up an odd number, then the median is the number right in the middle of the set. If the data set makes up an even number, then the median is the midpoint between the two middle numbers.
- Mode – this is simply the most commonly occurring number in the data set.
- In cases where most of the numbers are quite close to the average, the standard deviation will be relatively low.
- Conversely, in cases where the numbers are scattered all over the place, the standard deviation will be relatively high.
- Skewness . As the name suggests, skewness indicates how symmetrical a range of numbers is. In other words, do they tend to cluster into a smooth bell curve shape in the middle of the graph, or do they skew to the left or right?
Feeling a bit confused? Let’s look at a practical example using a small data set.
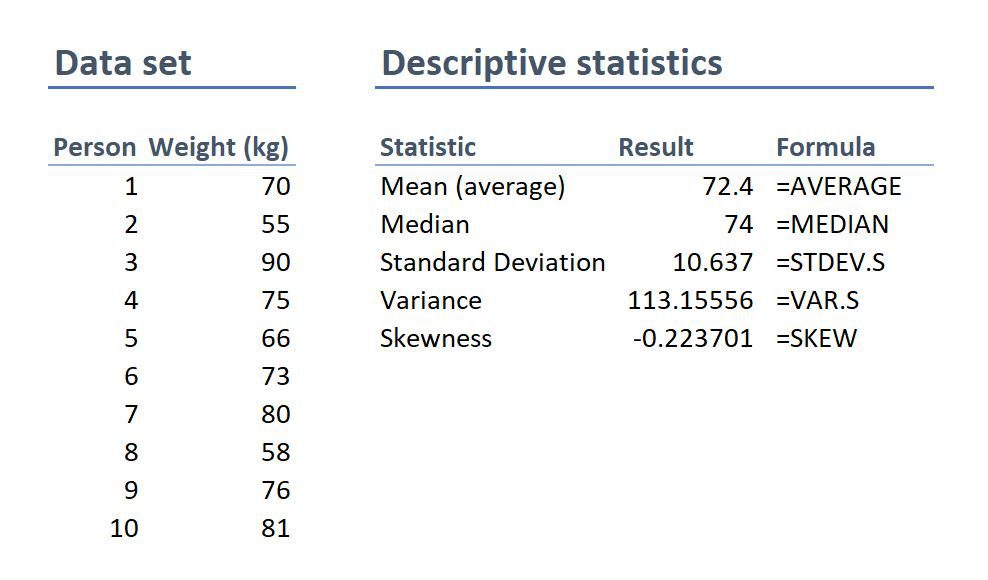
On the left-hand side is the data set. This details the bodyweight of a sample of 10 people. On the right-hand side, we have the descriptive statistics. Let’s take a look at each of them.
First, we can see that the mean weight is 72.4 kilograms. In other words, the average weight across the sample is 72.4 kilograms. Straightforward.
Next, we can see that the median is very similar to the mean (the average). This suggests that this data set has a reasonably symmetrical distribution (in other words, a relatively smooth, centred distribution of weights, clustered towards the centre).
In terms of the mode , there is no mode in this data set. This is because each number is present only once and so there cannot be a “most common number”. If there were two people who were both 65 kilograms, for example, then the mode would be 65.
Next up is the standard deviation . 10.6 indicates that there’s quite a wide spread of numbers. We can see this quite easily by looking at the numbers themselves, which range from 55 to 90, which is quite a stretch from the mean of 72.4.
And lastly, the skewness of -0.2 tells us that the data is very slightly negatively skewed. This makes sense since the mean and the median are slightly different.
As you can see, these descriptive statistics give us some useful insight into the data set. Of course, this is a very small data set (only 10 records), so we can’t read into these statistics too much. Also, keep in mind that this is not a list of all possible descriptive statistics – just the most common ones.
But why do all of these numbers matter?
While these descriptive statistics are all fairly basic, they’re important for a few reasons:
- Firstly, they help you get both a macro and micro-level view of your data. In other words, they help you understand both the big picture and the finer details.
- Secondly, they help you spot potential errors in the data – for example, if an average is way higher than you’d expect, or responses to a question are highly varied, this can act as a warning sign that you need to double-check the data.
- And lastly, these descriptive statistics help inform which inferential statistical techniques you can use, as those techniques depend on the skewness (in other words, the symmetry and normality) of the data.
Simply put, descriptive statistics are really important , even though the statistical techniques used are fairly basic. All too often at Grad Coach, we see students skimming over the descriptives in their eagerness to get to the more exciting inferential methods, and then landing up with some very flawed results.
Don’t be a sucker – give your descriptive statistics the love and attention they deserve!
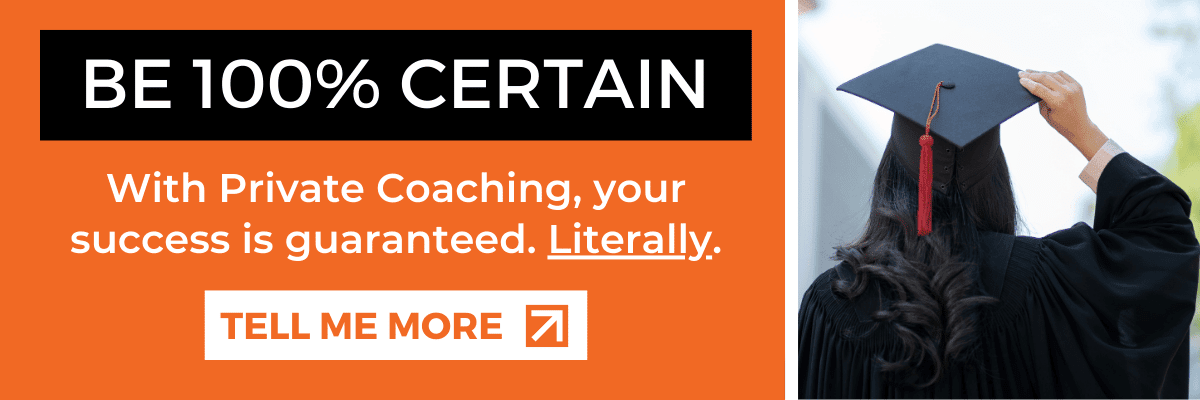
Branch 2: Inferential Statistics
As I mentioned, while descriptive statistics are all about the details of your specific data set – your sample – inferential statistics aim to make inferences about the population . In other words, you’ll use inferential statistics to make predictions about what you’d expect to find in the full population.
What kind of predictions, you ask? Well, there are two common types of predictions that researchers try to make using inferential stats:
- Firstly, predictions about differences between groups – for example, height differences between children grouped by their favourite meal or gender.
- And secondly, relationships between variables – for example, the relationship between body weight and the number of hours a week a person does yoga.
In other words, inferential statistics (when done correctly), allow you to connect the dots and make predictions about what you expect to see in the real world population, based on what you observe in your sample data. For this reason, inferential statistics are used for hypothesis testing – in other words, to test hypotheses that predict changes or differences.
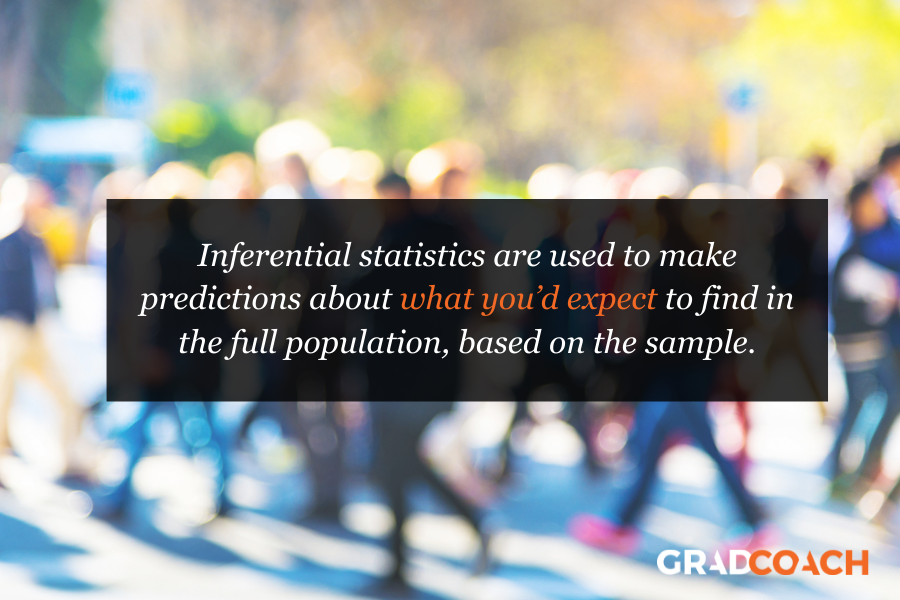
Of course, when you’re working with inferential statistics, the composition of your sample is really important. In other words, if your sample doesn’t accurately represent the population you’re researching, then your findings won’t necessarily be very useful.
For example, if your population of interest is a mix of 50% male and 50% female , but your sample is 80% male , you can’t make inferences about the population based on your sample, since it’s not representative. This area of statistics is called sampling, but we won’t go down that rabbit hole here (it’s a deep one!) – we’ll save that for another post .
What statistics are usually used in this branch?
There are many, many different statistical analysis methods within the inferential branch and it’d be impossible for us to discuss them all here. So we’ll just take a look at some of the most common inferential statistical methods so that you have a solid starting point.
First up are T-Tests . T-tests compare the means (the averages) of two groups of data to assess whether they’re statistically significantly different. In other words, do they have significantly different means, standard deviations and skewness.
This type of testing is very useful for understanding just how similar or different two groups of data are. For example, you might want to compare the mean blood pressure between two groups of people – one that has taken a new medication and one that hasn’t – to assess whether they are significantly different.
Kicking things up a level, we have ANOVA, which stands for “analysis of variance”. This test is similar to a T-test in that it compares the means of various groups, but ANOVA allows you to analyse multiple groups , not just two groups So it’s basically a t-test on steroids…
Next, we have correlation analysis . This type of analysis assesses the relationship between two variables. In other words, if one variable increases, does the other variable also increase, decrease or stay the same. For example, if the average temperature goes up, do average ice creams sales increase too? We’d expect some sort of relationship between these two variables intuitively , but correlation analysis allows us to measure that relationship scientifically .
Lastly, we have regression analysis – this is quite similar to correlation in that it assesses the relationship between variables, but it goes a step further to understand cause and effect between variables, not just whether they move together. In other words, does the one variable actually cause the other one to move, or do they just happen to move together naturally thanks to another force? Just because two variables correlate doesn’t necessarily mean that one causes the other.
Stats overload…
I hear you. To make this all a little more tangible, let’s take a look at an example of a correlation in action.
Here’s a scatter plot demonstrating the correlation (relationship) between weight and height. Intuitively, we’d expect there to be some relationship between these two variables, which is what we see in this scatter plot. In other words, the results tend to cluster together in a diagonal line from bottom left to top right.
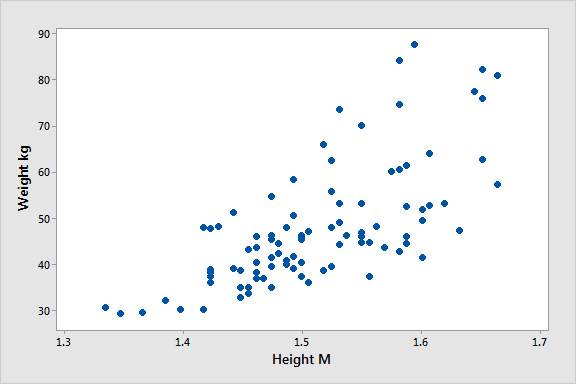
As I mentioned, these are are just a handful of inferential techniques – there are many, many more. Importantly, each statistical method has its own assumptions and limitations .
For example, some methods only work with normally distributed (parametric) data, while other methods are designed specifically for non-parametric data. And that’s exactly why descriptive statistics are so important – they’re the first step to knowing which inferential techniques you can and can’t use.
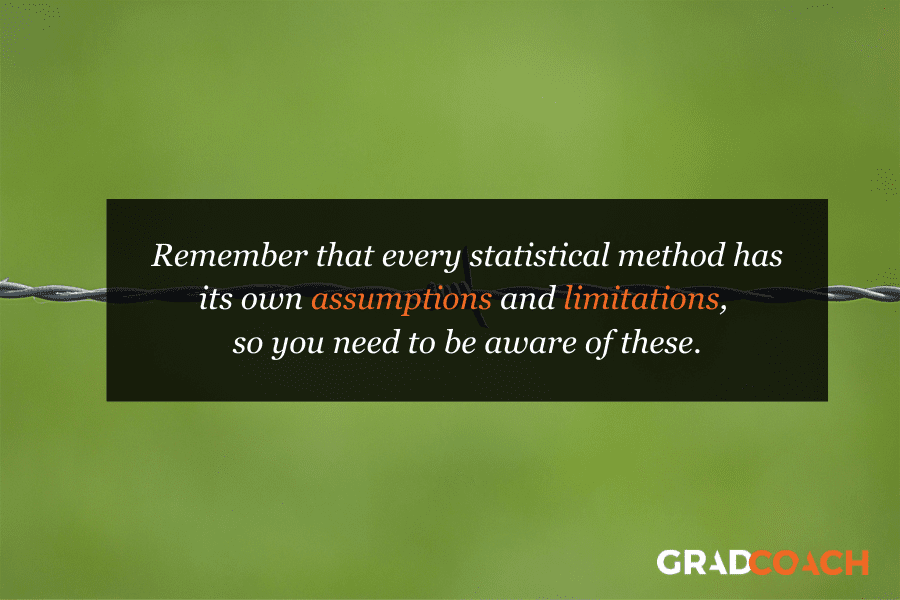
How to choose the right analysis method
To choose the right statistical methods, you need to think about two important factors :
- The type of quantitative data you have (specifically, level of measurement and the shape of the data). And,
- Your research questions and hypotheses
Let’s take a closer look at each of these.
Factor 1 – Data type
The first thing you need to consider is the type of data you’ve collected (or the type of data you will collect). By data types, I’m referring to the four levels of measurement – namely, nominal, ordinal, interval and ratio. If you’re not familiar with this lingo, check out the video below.
Why does this matter?
Well, because different statistical methods and techniques require different types of data. This is one of the “assumptions” I mentioned earlier – every method has its assumptions regarding the type of data.
For example, some techniques work with categorical data (for example, yes/no type questions, or gender or ethnicity), while others work with continuous numerical data (for example, age, weight or income) – and, of course, some work with multiple data types.
If you try to use a statistical method that doesn’t support the data type you have, your results will be largely meaningless . So, make sure that you have a clear understanding of what types of data you’ve collected (or will collect). Once you have this, you can then check which statistical methods would support your data types here .
If you haven’t collected your data yet, you can work in reverse and look at which statistical method would give you the most useful insights, and then design your data collection strategy to collect the correct data types.
Another important factor to consider is the shape of your data . Specifically, does it have a normal distribution (in other words, is it a bell-shaped curve, centred in the middle) or is it very skewed to the left or the right? Again, different statistical techniques work for different shapes of data – some are designed for symmetrical data while others are designed for skewed data.
This is another reminder of why descriptive statistics are so important – they tell you all about the shape of your data.
Factor 2: Your research questions
The next thing you need to consider is your specific research questions, as well as your hypotheses (if you have some). The nature of your research questions and research hypotheses will heavily influence which statistical methods and techniques you should use.
If you’re just interested in understanding the attributes of your sample (as opposed to the entire population), then descriptive statistics are probably all you need. For example, if you just want to assess the means (averages) and medians (centre points) of variables in a group of people.
On the other hand, if you aim to understand differences between groups or relationships between variables and to infer or predict outcomes in the population, then you’ll likely need both descriptive statistics and inferential statistics.
So, it’s really important to get very clear about your research aims and research questions, as well your hypotheses – before you start looking at which statistical techniques to use.
Never shoehorn a specific statistical technique into your research just because you like it or have some experience with it. Your choice of methods must align with all the factors we’ve covered here.
Time to recap…
You’re still with me? That’s impressive. We’ve covered a lot of ground here, so let’s recap on the key points:
- Quantitative data analysis is all about analysing number-based data (which includes categorical and numerical data) using various statistical techniques.
- The two main branches of statistics are descriptive statistics and inferential statistics . Descriptives describe your sample, whereas inferentials make predictions about what you’ll find in the population.
- Common descriptive statistical methods include mean (average), median , standard deviation and skewness .
- Common inferential statistical methods include t-tests , ANOVA , correlation and regression analysis.
- To choose the right statistical methods and techniques, you need to consider the type of data you’re working with , as well as your research questions and hypotheses.
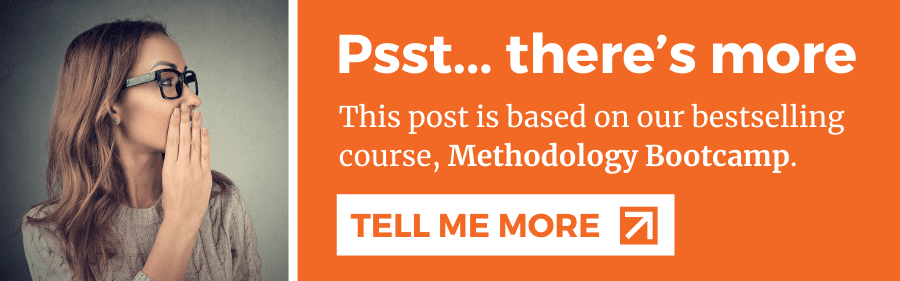
77 Comments
Hi, I have read your article. Such a brilliant post you have created.
Thank you for the feedback. Good luck with your quantitative analysis.
Thank you so much.
Thank you so much. I learnt much well. I love your summaries of the concepts. I had love you to explain how to input data using SPSS
Very useful, I have got the concept
Amazing and simple way of breaking down quantitative methods.
This is beautiful….especially for non-statisticians. I have skimmed through but I wish to read again. and please include me in other articles of the same nature when you do post. I am interested. I am sure, I could easily learn from you and get off the fear that I have had in the past. Thank you sincerely.
Send me every new information you might have.
i need every new information
Thank you for the blog. It is quite informative. Dr Peter Nemaenzhe PhD
It is wonderful. l’ve understood some of the concepts in a more compréhensive manner
Your article is so good! However, I am still a bit lost. I am doing a secondary research on Gun control in the US and increase in crime rates and I am not sure which analysis method I should use?
Based on the given learning points, this is inferential analysis, thus, use ‘t-tests, ANOVA, correlation and regression analysis’
Well explained notes. Am an MPH student and currently working on my thesis proposal, this has really helped me understand some of the things I didn’t know.
I like your page..helpful
wonderful i got my concept crystal clear. thankyou!!
This is really helpful , thank you
Thank you so much this helped
Wonderfully explained
thank u so much, it was so informative
THANKYOU, this was very informative and very helpful
This is great GRADACOACH I am not a statistician but I require more of this in my thesis
Include me in your posts.
This is so great and fully useful. I would like to thank you again and again.
Glad to read this article. I’ve read lot of articles but this article is clear on all concepts. Thanks for sharing.
Thank you so much. This is a very good foundation and intro into quantitative data analysis. Appreciate!
You have a very impressive, simple but concise explanation of data analysis for Quantitative Research here. This is a God-send link for me to appreciate research more. Thank you so much!
Avery good presentation followed by the write up. yes you simplified statistics to make sense even to a layman like me. Thank so much keep it up. The presenter did ell too. i would like more of this for Qualitative and exhaust more of the test example like the Anova.
This is a very helpful article, couldn’t have been clearer. Thank you.
Awesome and phenomenal information.Well done
The video with the accompanying article is super helpful to demystify this topic. Very well done. Thank you so much.
thank you so much, your presentation helped me a lot
I don’t know how should I express that ur article is saviour for me 🥺😍
It is well defined information and thanks for sharing. It helps me a lot in understanding the statistical data.
I gain a lot and thanks for sharing brilliant ideas, so wish to be linked on your email update.
Very helpful and clear .Thank you Gradcoach.
Thank for sharing this article, well organized and information presented are very clear.
VERY INTERESTING AND SUPPORTIVE TO NEW RESEARCHERS LIKE ME. AT LEAST SOME BASICS ABOUT QUANTITATIVE.
An outstanding, well explained and helpful article. This will help me so much with my data analysis for my research project. Thank you!
wow this has just simplified everything i was scared of how i am gonna analyse my data but thanks to you i will be able to do so
simple and constant direction to research. thanks
This is helpful
Great writing!! Comprehensive and very helpful.
Do you provide any assistance for other steps of research methodology like making research problem testing hypothesis report and thesis writing?
Thank you so much for such useful article!
Amazing article. So nicely explained. Wow
Very insightfull. Thanks
I am doing a quality improvement project to determine if the implementation of a protocol will change prescribing habits. Would this be a t-test?
The is a very helpful blog, however, I’m still not sure how to analyze my data collected. I’m doing a research on “Free Education at the University of Guyana”
tnx. fruitful blog!
So I am writing exams and would like to know how do establish which method of data analysis to use from the below research questions: I am a bit lost as to how I determine the data analysis method from the research questions.
Do female employees report higher job satisfaction than male employees with similar job descriptions across the South African telecommunications sector? – I though that maybe Chi Square could be used here. – Is there a gender difference in talented employees’ actual turnover decisions across the South African telecommunications sector? T-tests or Correlation in this one. – Is there a gender difference in the cost of actual turnover decisions across the South African telecommunications sector? T-tests or Correlation in this one. – What practical recommendations can be made to the management of South African telecommunications companies on leveraging gender to mitigate employee turnover decisions?
Your assistance will be appreciated if I could get a response as early as possible tomorrow
This was quite helpful. Thank you so much.
wow I got a lot from this article, thank you very much, keep it up
Thanks for yhe guidance. Can you send me this guidance on my email? To enable offline reading?
Thank you very much, this service is very helpful.
Every novice researcher needs to read this article as it puts things so clear and easy to follow. Its been very helpful.
Wonderful!!!! you explained everything in a way that anyone can learn. Thank you!!
I really enjoyed reading though this. Very easy to follow. Thank you
Many thanks for your useful lecture, I would be really appreciated if you could possibly share with me the PPT of presentation related to Data type?
Thank you very much for sharing, I got much from this article
This is a very informative write-up. Kindly include me in your latest posts.
Very interesting mostly for social scientists
Thank you so much, very helpfull
You’re welcome 🙂
woow, its great, its very informative and well understood because of your way of writing like teaching in front of me in simple languages.
I have been struggling to understand a lot of these concepts. Thank you for the informative piece which is written with outstanding clarity.
very informative article. Easy to understand
Beautiful read, much needed.
Always greet intro and summary. I learn so much from GradCoach
Quite informative. Simple and clear summary.
I thoroughly enjoyed reading your informative and inspiring piece. Your profound insights into this topic truly provide a better understanding of its complexity. I agree with the points you raised, especially when you delved into the specifics of the article. In my opinion, that aspect is often overlooked and deserves further attention.
Absolutely!!! Thank you
Thank you very much for this post. It made me to understand how to do my data analysis.
its nice work and excellent job ,you have made my work easier
Wow! So explicit. Well done.
Submit a Comment Cancel reply
Your email address will not be published. Required fields are marked *
Save my name, email, and website in this browser for the next time I comment.
- Print Friendly
- Databank Solution
- Credit Risk Solution
- Valuation Analytics Solution
- ESG Sustainability Solution
- Quantitative Finance Solution
- Regulatory Technology Solution
- Product Introduction
- Service Provide Method
- Learning Video
Quantitative Data Analysis Guide: Methods, Examples & Uses
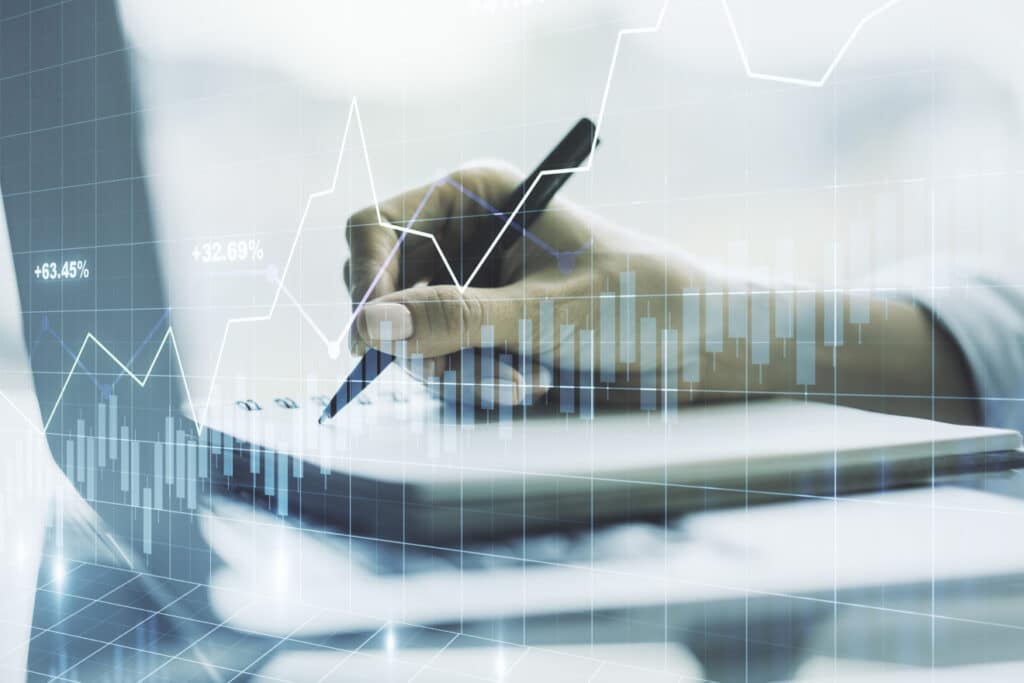
This guide will introduce the types of data analysis used in quantitative research, then discuss relevant examples and applications in the finance industry.
Table of Contents
An Overview of Quantitative Data Analysis
What is quantitative data analysis and what is it for .
Quantitative data analysis is the process of interpreting meaning and extracting insights from numerical data , which involves mathematical calculations and statistical reviews to uncover patterns, trends, and relationships between variables.
Beyond academic and statistical research, this approach is particularly useful in the finance industry. Financial data, such as stock prices, interest rates, and economic indicators, can all be quantified with statistics and metrics to offer crucial insights for informed investment decisions. To illustrate this, here are some examples of what quantitative data is usually used for:
- Measuring Differences between Groups: For instance, analyzing historical stock prices of different companies or asset classes can reveal which companies consistently outperform the market average.
- Assessing Relationships between Variables: An investor could analyze the relationship between a company’s price-to-earnings ratio (P/E ratio) and relevant factors, like industry performance, inflation rates, interests, etc, allowing them to predict future stock price growth.
- Testing Hypotheses: For example, an investor might hypothesize that companies with strong ESG (Environment, Social, and Governance) practices outperform those without. By categorizing these companies into two groups (strong ESG vs. weak ESG practices), they can compare the average return on investment (ROI) between the groups while assessing relevant factors to find evidence for the hypothesis.
Ultimately, quantitative data analysis helps investors navigate the complex financial landscape and pursue profitable opportunities.
Quantitative Data Analysis VS. Qualitative Data Analysis
Although quantitative data analysis is a powerful tool, it cannot be used to provide context for your research, so this is where qualitative analysis comes in. Qualitative analysis is another common research method that focuses on collecting and analyzing non-numerical data , like text, images, or audio recordings to gain a deeper understanding of experiences, opinions, and motivations. Here’s a table summarizing its key differences between quantitative data analysis:
Types of Data Used | Numerical data: numbers, percentages, etc. | Non-numerical data: text, images, audio, narratives, etc |
Perspective | More objective and less prone to bias | More subjective as it may be influenced by the researcher’s interpretation |
Data Collection | Closed-ended questions, surveys, polls | Open-ended questions, interviews, observations |
Data Analysis | Statistical methods, numbers, graphs, charts | Categorization, thematic analysis, verbal communication |
Focus | and | and |
Best Use Case | Measuring trends, comparing groups, testing hypotheses | Understanding user experience, exploring consumer motivations, uncovering new ideas |
Due to their characteristics, quantitative analysis allows you to measure and compare large datasets; while qualitative analysis helps you understand the context behind the data. In some cases, researchers might even use both methods together for a more comprehensive understanding, but we’ll mainly focus on quantitative analysis for this article.
The 2 Main Quantitative Data Analysis Methods
Once you have your data collected, you have to use descriptive statistics or inferential statistics analysis to draw summaries and conclusions from your raw numbers.
As its name suggests, the purpose of descriptive statistics is to describe your sample . It provides the groundwork for understanding your data by focusing on the details and characteristics of the specific group you’ve collected data from.
On the other hand, inferential statistics act as bridges that connect your sample data to the broader population you’re truly interested in, helping you to draw conclusions in your research. Moreover, choosing the right inferential technique for your specific data and research questions is dependent on the initial insights from descriptive statistics, so both of these methods usually go hand-in-hand.
Descriptive Statistics Analysis
With sophisticated descriptive statistics, you can detect potential errors in your data by highlighting inconsistencies and outliers that might otherwise go unnoticed. Additionally, the characteristics revealed by descriptive statistics will help determine which inferential techniques are suitable for further analysis.
Measures in Descriptive Statistics
One of the key statistical tests used for descriptive statistics is central tendency . It consists of mean, median, and mode, telling you where most of your data points cluster:
- Mean: It refers to the “average” and is calculated by adding all the values in your data set and dividing by the number of values.
- Median: The middle value when your data is arranged in ascending or descending order. If you have an odd number of data points, the median is the exact middle value; with even numbers, it’s the average of the two middle values.
- Mode: This refers to the most frequently occurring value in your data set, indicating the most common response or observation. Some data can have multiple modes (bimodal) or no mode at all.
Another statistic to test in descriptive analysis is the measures of dispersion , which involves range and standard deviation, revealing how spread out your data is relative to the central tendency measures:
- Range: It refers to the difference between the highest and lowest values in your data set.
- Standard Deviation (SD): This tells you how the data is distributed within the range, revealing how much, on average, each data point deviates from the mean. Lower standard deviations indicate data points clustered closer to the mean, while higher standard deviations suggest a wider spread.
The shape of the distribution will then be measured through skewness.
- Skewness: A statistic that indicates whether your data leans to one side (positive or negative) or is symmetrical (normal distribution). A positive skew suggests more data points concentrated on the lower end, while a negative skew indicates more data points on the higher end.
While the core measures mentioned above are fundamental, there are additional descriptive statistics used in specific contexts, including percentiles and interquartile range.
- Percentiles: This divides your data into 100 equal parts, revealing what percentage of data falls below a specific value. The 25th percentile (Q1) is the first quartile, the 50th percentile (Q2) is the median, and the 75th percentile (Q3) is the third quartile. Knowing these quartiles can help visualize the spread of your data.
- Interquartile Range (IQR): This measures the difference between Q3 and Q1, representing the middle 50% of your data.
Example of Descriptive Quantitative Data Analysis
Let’s illustrate these concepts with a real-world example. Imagine a financial advisor analyzing a client’s portfolio. They have data on the client’s various holdings, including stock prices over the past year. With descriptive statistics they can obtain the following information:
- Central Tendency: The mean price for each stock reveals its average price over the year. The median price can further highlight if there were any significant price spikes or dips that skewed the mean.
- Measures of Dispersion: The standard deviation for each stock indicates its price volatility. A high standard deviation suggests the stock’s price fluctuated considerably, while a low standard deviation implies a more stable price history. This helps the advisor assess each stock’s risk profile.
- Shape of the Distribution: If data allows, analyzing skewness can be informative. A positive skew for a stock might suggest more frequent price drops, while a negative skew might indicate more frequent price increases.
By calculating these descriptive statistics, the advisor gains a quick understanding of the client’s portfolio performance and risk distribution. For instance, they could use correlation analysis to see if certain stock prices tend to move together, helping them identify expansion opportunities within the portfolio.
While descriptive statistics provide a foundational understanding, they should be followed by inferential analysis to uncover deeper insights that are crucial for making investment decisions.
Inferential Statistics Analysis
Inferential statistics analysis is particularly useful for hypothesis testing , as you can formulate predictions about group differences or potential relationships between variables , then use statistical tests to see if your sample data supports those hypotheses.
However, the power of inferential statistics hinges on one crucial factor: sample representativeness . If your sample doesn’t accurately reflect the population, your predictions won’t be very reliable.
Statistical Tests for Inferential Statistics
Here are some of the commonly used tests for inferential statistics in commerce and finance, which can also be integrated to most analysis software:
- T-Tests: This compares the means, standard deviation, or skewness of two groups to assess if they’re statistically different, helping you determine if the observed difference is just a quirk within the sample or a significant reflection of the population.
- ANOVA (Analysis of Variance): While T-Tests handle comparisons between two groups, ANOVA focuses on comparisons across multiple groups, allowing you to identify potential variations and trends within the population.
- Correlation Analysis: This technique tests the relationship between two variables, assessing if one variable increases or decreases with the other. However, it’s important to note that just because two financial variables are correlated and move together, doesn’t necessarily mean one directly influences the other.
- Regression Analysis: Building on correlation, regression analysis goes a step further to verify the cause-and-effect relationships between the tested variables, allowing you to investigate if one variable actually influences the other.
- Cross-Tabulation: This breaks down the relationship between two categorical variables by displaying the frequency counts in a table format, helping you to understand how different groups within your data set might behave. The data in cross-tabulation can be mutually exclusive or have several connections with each other.
- Trend Analysis: This examines how a variable in quantitative data changes over time, revealing upward or downward trends, as well as seasonal fluctuations. This can help you forecast future trends, and also lets you assess the effectiveness of the interventions in your marketing or investment strategy.
- MaxDiff Analysis: This is also known as the “best-worst” method. It evaluates customer preferences by asking respondents to choose the most and least preferred options from a set of products or services, allowing stakeholders to optimize product development or marketing strategies.
- Conjoint Analysis: Similar to MaxDiff, conjoint analysis gauges customer preferences, but it goes a step further by allowing researchers to see how changes in different product features (price, size, brand) influence overall preference.
- TURF Analysis (Total Unduplicated Reach and Frequency Analysis): This assesses a marketing campaign’s reach and frequency of exposure in different channels, helping businesses identify the most efficient channels to reach target audiences.
- Gap Analysis: This compares current performance metrics against established goals or benchmarks, using numerical data to represent the factors involved. This helps identify areas where performance falls short of expectations, serving as a springboard for developing strategies to bridge the gap and achieve those desired outcomes.
- SWOT Analysis (Strengths, Weaknesses, Opportunities, and Threats): This uses ratings or rankings to represent an organization’s internal strengths and weaknesses, along with external opportunities and threats. Based on this analysis, organizations can create strategic plans to capitalize on opportunities while minimizing risks.
- Text Analysis: This is an advanced method that uses specialized software to categorize and quantify themes, sentiment (positive, negative, neutral), and topics within textual data, allowing companies to obtain structured quantitative data from surveys, social media posts, or customer reviews.
Example of Inferential Quantitative Data Analysis
If you’re a financial analyst studying the historical performance of a particular stock, here are some predictions you can make with inferential statistics:
- The Differences between Groups: You can conduct T-Tests to compare the average returns of stocks in the technology sector with those in the healthcare sector. It can help assess if the observed difference in returns between these two sectors is simply due to random chance or if it’s statistically significant due to a significant difference in their performance.
- The Relationships between Variables: If you’re curious about the connection between a company’s price-to-earnings ratio (P/E ratios) and its future stock price movements, conducting correlation analysis can let you measure the strength and direction of this relationship. Is there a negative correlation, suggesting that higher P/E ratios might be associated with lower future stock prices? Or is there no significant correlation at all?
Understanding these inferential analysis techniques can help you uncover potential relationships and group differences that might not be readily apparent from descriptive statistics alone. Nonetheless, it’s important to remember that each technique has its own set of assumptions and limitations . Some methods are designed for parametric data with a normal distribution, while others are suitable for non-parametric data.
Guide to Conduct Data Analysis in Quantitative Research
Now that we have discussed the types of data analysis techniques used in quantitative research, here’s a quick guide to help you choose the right method and grasp the essential steps of quantitative data analysis.
How to Choose the Right Quantitative Analysis Method?
Choosing between all these quantitative analysis methods may seem like a complicated task, but if you consider the 2 following factors, you can definitely choose the right technique:
Factor 1: Data Type
The data used in quantitative analysis can be categorized into two types, discrete data and continuous data, based on how they’re measured. They can also be further differentiated by their measurement scale. The four main types of measurement scales include: nominal, ordinal, interval or ratio. Understanding the distinctions between them is essential for choosing the appropriate statistical methods to interpret the results of your quantitative data analysis accurately.
Discrete data , which is also known as attribute data, represents whole numbers that can be easily counted and separated into distinct categories. It is often visualized using bar charts or pie charts, making it easy to see the frequency of each value. In the financial world, examples of discrete quantitative data include:
- The number of shares owned by an investor in a particular company
- The number of customer transactions processed by a bank per day
- Bond ratings (AAA, BBB, etc.) that represent discrete categories indicating the creditworthiness of a bond issuer
- The number of customers with different account types (checking, savings, investment) as seen in the pie chart below:
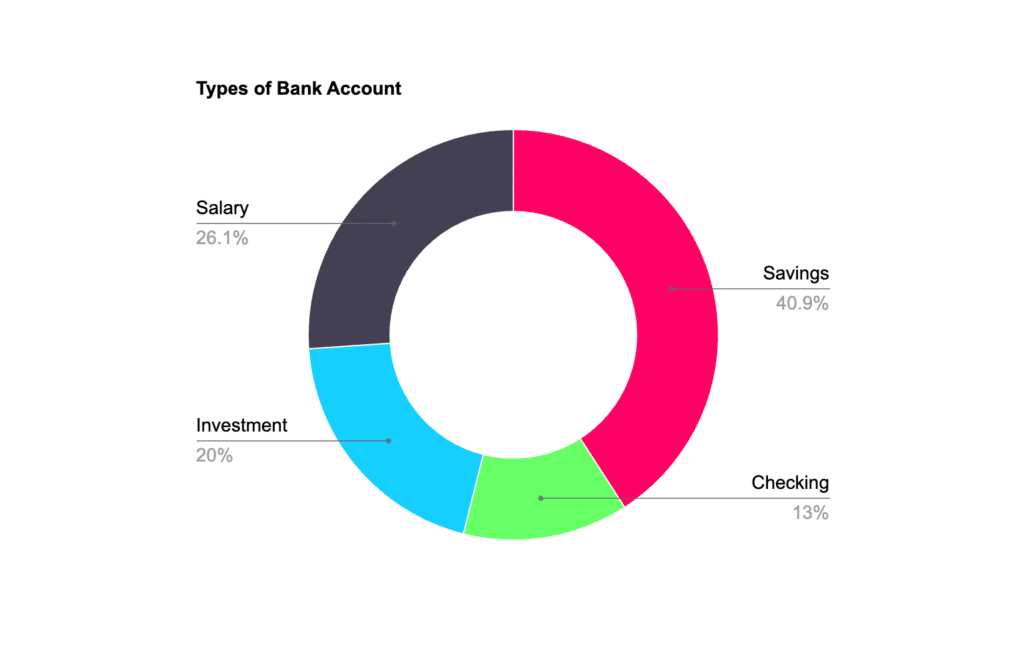
Discrete data usually use nominal or ordinal measurement scales, which can be then quantified to calculate their mode or median. Here are some examples:
- Nominal: This scale categorizes data into distinct groups with no inherent order. For instance, data on bank account types can be considered nominal data as it classifies customers in distinct categories which are independent of each other, either checking, savings, or investment accounts. and no inherent order or ranking implied by these account types.
- Ordinal: Ordinal data establishes a rank or order among categories. For example, investment risk ratings (low, medium, high) are ordered based on their perceived risk of loss, making it a type or ordinal data.
Conversely, continuous data can take on any value and fluctuate over time. It is usually visualized using line graphs, effectively showcasing how the values can change within a specific time frame. Examples of continuous data in the financial industry include:
- Interest rates set by central banks or offered by banks on loans and deposits
- Currency exchange rates which also fluctuate constantly throughout the day
- Daily trading volume of a particular stock on a specific day
- Stock prices that fluctuate throughout the day, as seen in the line graph below:
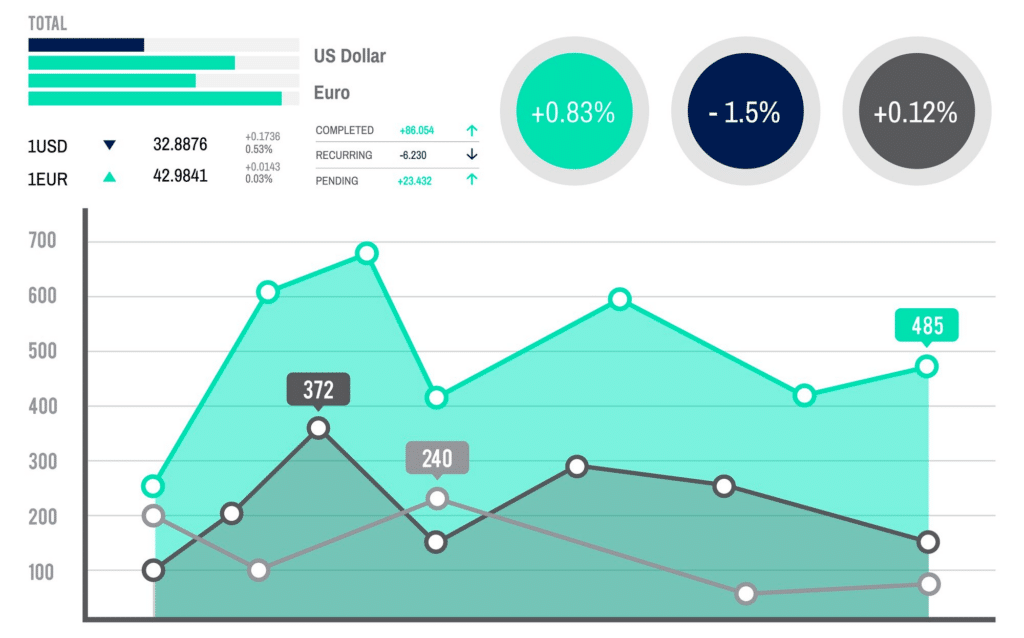
Source: Freepik
The measurement scale for continuous data is usually interval or ratio . Here is breakdown of their differences:
- Interval: This builds upon ordinal data by having consistent intervals between each unit, and its zero point doesn’t represent a complete absence of the variable. Let’s use credit score as an example. While the scale ranges from 300 to 850, the interval between each score rating is consistent (50 points), and a score of zero wouldn’t indicate an absence of credit history, but rather no credit score available.
- Ratio: This scale has all the same characteristics of interval data but also has a true zero point, indicating a complete absence of the variable. Interest rates expressed as percentages are a classic example of ratio data. A 0% interest rate signifies the complete absence of any interest charged or earned, making it a true zero point.
Factor 2: Research Question
You also need to make sure that the analysis method aligns with your specific research questions. If you merely want to focus on understanding the characteristics of your data set, descriptive statistics might be all you need; if you need to analyze the connection between variables, then you have to include inferential statistics as well.
How to Analyze Quantitative Data
Step 1: data collection .
Depending on your research question, you might choose to conduct surveys or interviews. Distributing online or paper surveys can reach a broad audience, while interviews allow for deeper exploration of specific topics. You can also choose to source existing datasets from government agencies or industry reports.
Step 2: Data Cleaning
Raw data might contain errors, inconsistencies, or missing values, so data cleaning has to be done meticulously to ensure accuracy and consistency. This might involve removing duplicates, correcting typos, and handling missing information.
Furthermore, you should also identify the nature of your variables and assign them appropriate measurement scales , it could be nominal, ordinal, interval or ratio. This is important because it determines the types of descriptive statistics and analysis methods you can employ later. Once you categorize your data based on these measurement scales, you can arrange the data of each category in a proper order and organize it in a format that is convenient for you.
Step 3: Data Analysis
Based on the measurement scales of your variables, calculate relevant descriptive statistics to summarize your data. This might include measures of central tendency (mean, median, mode) and dispersion (range, standard deviation, variance). With these statistics, you can identify the pattern within your raw data.
Then, these patterns can be analyzed further with inferential methods to test out the hypotheses you have developed. You may choose any of the statistical tests mentioned above, as long as they are compatible with the characteristics of your data.
Step 4. Data Interpretation and Communication
Now that you have the results from your statistical analysis, you may draw conclusions based on the findings and incorporate them into your business strategies. Additionally, you should also transform your findings into clear and shareable information to facilitate discussion among stakeholders. Visualization techniques like tables, charts, or graphs can make complex data more digestible so that you can communicate your findings efficiently.
Useful Quantitative Data Analysis Tools and Software
We’ve compiled some commonly used quantitative data analysis tools and software. Choosing the right one depends on your experience level, project needs, and budget. Here’s a brief comparison:
Easiest | Beginners & basic analysis | One-time purchase with Microsoft Office Suite | |
Easy | Social scientists & researchers | Paid commercial license | |
Easy | Students & researchers | Paid commercial license or student discounts | |
Moderate | Businesses & advanced research | Paid commercial license | |
Moderate | Researchers & statisticians | Paid commercial license | |
Moderate (Coding optional) | Programmers & data scientists | Free & Open-Source | |
Steep (Coding required) | Experienced users & programmers | Free & Open-Source | |
Steep (Coding required) | Scientists & engineers | Paid commercial license | |
Steep (Coding required) | Scientists & engineers | Paid commercial license |
Quantitative Data in Finance and Investment
So how does this all affect the finance industry? Quantitative finance (or quant finance) has become a growing trend, with the quant fund market valued at $16,008.69 billion in 2023. This value is expected to increase at the compound annual growth rate of 10.09% and reach $31,365.94 billion by 2031, signifying its expanding role in the industry.
What is Quant Finance?
Quant finance is the process of using massive financial data and mathematical models to identify market behavior, financial trends, movements, and economic indicators, so that they can predict future trends.These calculated probabilities can be leveraged to find potential investment opportunities and maximize returns while minimizing risks.
Common Quantitative Investment Strategies
There are several common quantitative strategies, each offering unique approaches to help stakeholders navigate the market:
1. Statistical Arbitrage
This strategy aims for high returns with low volatility. It employs sophisticated algorithms to identify minuscule price discrepancies across the market, then capitalize on them at lightning speed, often generating short-term profits. However, its reliance on market efficiency makes it vulnerable to sudden market shifts, posing a risk of disrupting the calculations.
2. Factor Investing
This strategy identifies and invests in assets based on factors like value, momentum, or quality. By analyzing these factors in quantitative databases , investors can construct portfolios designed to outperform the broader market. Overall, this method offers diversification and potentially higher returns than passive investing, but its success relies on the historical validity of these factors, which can evolve over time.
3. Risk Parity
This approach prioritizes portfolio balance above all else. Instead of allocating assets based on their market value, risk parity distributes them based on their risk contribution to achieve a desired level of overall portfolio risk, regardless of individual asset volatility. Although it is efficient in managing risks while potentially offering positive returns, it is important to note that this strategy’s complex calculations can be sensitive to unexpected market events.
4. Machine Learning & Artificial Intelligence (AI)
Quant analysts are beginning to incorporate these cutting-edge technologies into their strategies. Machine learning algorithms can act as data sifters, identifying complex patterns within massive datasets; whereas AI goes a step further, leveraging these insights to make investment decisions, essentially mimicking human-like decision-making with added adaptability. Despite the hefty development and implementation costs, its superior risk-adjusted returns and uncovering hidden patterns make this strategy a valuable asset.
Pros and Cons of Quantitative Data Analysis
Advantages of quantitative data analysis, minimum bias for reliable results.
Quantitative data analysis relies on objective, numerical data. This minimizes bias and human error, allowing stakeholders to make investment decisions without emotional intuitions that can cloud judgment. In turn, this offers reliable and consistent results for investment strategies.
Precise Calculations for Data-Driven Decisions
Quantitative analysis generates precise numerical results through statistical methods. This allows accurate comparisons between investment options and even predictions of future market behavior, helping investors make informed decisions about where to allocate their capital while managing potential risks.
Generalizability for Broader Insights
By analyzing large datasets and identifying patterns, stakeholders can generalize the findings from quantitative analysis into broader populations, applying them to a wider range of investments for better portfolio construction and risk management
Efficiency for Extensive Research
Quantitative research is more suited to analyze large datasets efficiently, letting companies save valuable time and resources. The softwares used for quantitative analysis can automate the process of sifting through extensive financial data, facilitating quicker decision-making in the fast-paced financial environment.
Disadvantages of Quantitative Data Analysis
Limited scope .
By focusing on numerical data, quantitative analysis may provide a limited scope, as it can’t capture qualitative context such as emotions, motivations, or cultural factors. Although quantitative analysis provides a strong starting point, neglecting qualitative factors can lead to incomplete insights in the financial industry, impacting areas like customer relationship management and targeted marketing strategies.
Oversimplification
Breaking down complex phenomena into numerical data could cause analysts to overlook the richness of the data, leading to the issue of oversimplification. Stakeholders who fail to understand the complexity of economic factors or market trends could face flawed investment decisions and missed opportunities.
Reliable Quantitative Data Solution
In conclusion, quantitative data analysis offers a deeper insight into market trends and patterns, empowering you to make well-informed financial decisions. However, collecting comprehensive data and analyzing them can be a complex task that may divert resources from core investment activity.
As a reliable provider, TEJ understands these concerns. Our TEJ Quantitative Investment Database offers high-quality financial and economic data for rigorous quantitative analysis. This data captures the true market conditions at specific points in time, enabling accurate backtesting of investment strategies.
Furthermore, TEJ offers diverse data sets that go beyond basic stock prices, encompassing various financial metrics, company risk attributes, and even broker trading information, all designed to empower your analysis and strategy development. Save resources and unlock the full potential of quantitative finance with TEJ’s data solutions today!
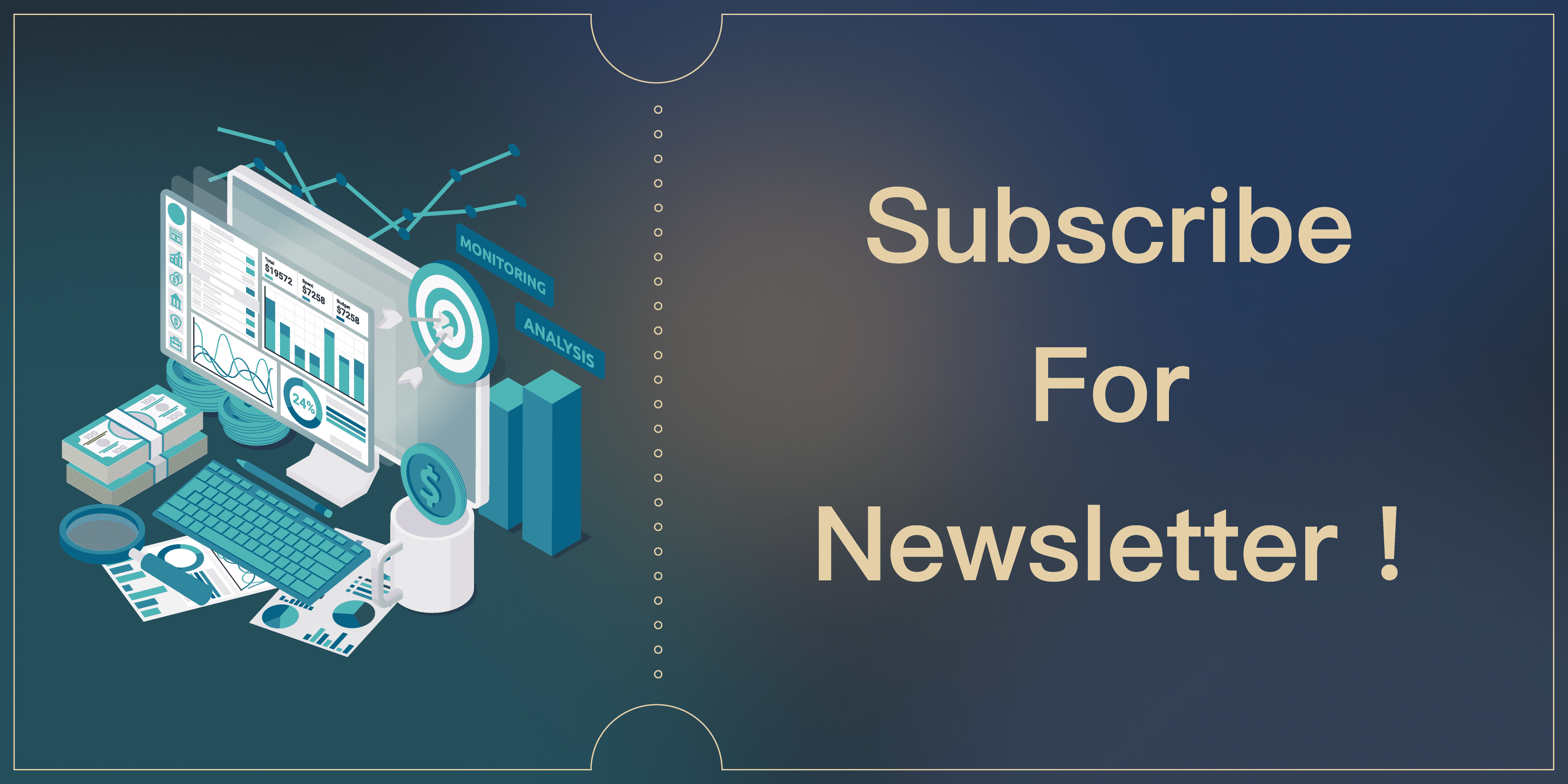
Subscribe to newsletter
- Data Analysis 107
- Market Research 48
- TQuant Lab 26
- Solution&DataSets
- Privacy Policy Statement
- Personal Data Policy
- Copyright Information
- Website licensing terms
- Information Security Policy

- 11th Floor, No. 57, DongXing Road, Taipei 11070, Taiwan(R.O.C.)
- +886-2-8768-1088
- +886-2-8768-1336
- [email protected]
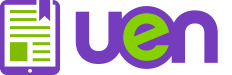
Part II: Data Analysis Methods in Quantitative Research
Data analysis methods in quantitative research.
We started this module with levels of measurement as a way to categorize our data. Data analysis is directed toward answering the original research question and achieving the study purpose (or aim). Now, we are going to delve into two main statistical analyses to describe our data and make inferences about our data:
Descriptive Statistics and Inferential Statistics.
Descriptive Statistics:
Before you panic, we will not be going into statistical analyses very deeply. We want to simply get a good overview of some of the types of general statistical analyses so that it makes some sense to us when we read results in published research articles.
Descriptive statistics summarize or describe the characteristics of a data set. This is a method of simply organizing and describing our data. Why? Because data that are not organized in some fashion are super difficult to interpret.
Let’s say our sample is golden retrievers (population “canines”). Our descriptive statistics tell us more about the same.
- 37% of our sample is male, 43% female
- The mean age is 4 years
- Mode is 6 years
- Median age is 5.5 years
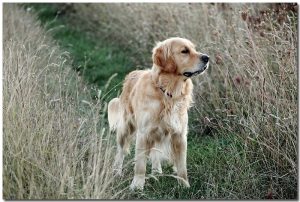
Let’s explore some of the types of descriptive statistics.
Frequency Distributions : A frequency distribution describes the number of observations for each possible value of a measured variable. The numbers are arranged from lowest to highest and features a count of how many times each value occurred.
For example, if 18 students have pet dogs, dog ownership has a frequency of 18.
We might see what other types of pets that students have. Maybe cats, fish, and hamsters. We find that 2 students have hamsters, 9 have fish, 1 has a cat.
You can see that it is very difficult to interpret the various pets into any meaningful interpretation, yes?
Now, let’s take those same pets and place them in a frequency distribution table.
Type of Pet | Frequency |
Dog | 18 |
Fish | 9 |
Hamsters | 2 |
Cat | 1 |
As we can now see, this is much easier to interpret.
Let’s say that we want to know how many books our sample population of students have read in the last year. We collect our data and find this:
Number of Books | Frequency (How many students read that number of books) |
13 | 1 |
12 | 6 |
11 | 18 |
10 | 58 |
9 | 99 |
8 | 138 |
7 | 99 |
6 | 56 |
5 | 21 |
4 | 8 |
3 | 2 |
2 | 1 |
1 | 0 |
We can then take that table and plot it out on a frequency distribution graph. This makes it much easier to see how the numbers are disbursed. Easier on the eyes, yes?
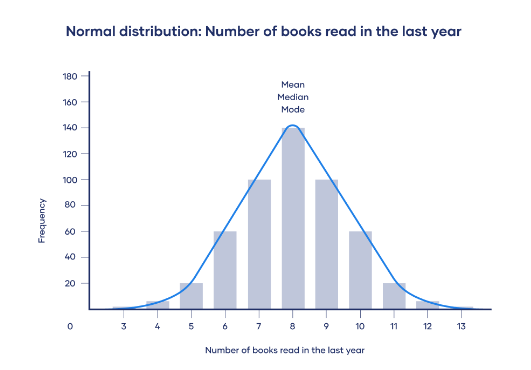
Here’s another example of symmetrical, positive skew, and negative skew:
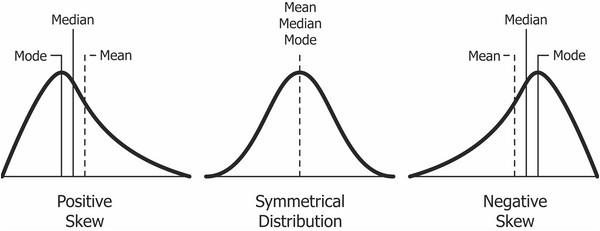
Correlation : Relationships between two research variables are called correlations . Remember, correlation is not cause-and-effect. Correlations simply measure the extent of relationship between two variables. To measure correlation in descriptive statistics, the statistical analysis called Pearson’s correlation coefficient I is often used. You do not need to know how to calculate this for this course. But, do remember that analysis test because you will often see this in published research articles. There really are no set guidelines on what measurement constitutes a “strong” or “weak” correlation, as it really depends on the variables being measured.
However, possible values for correlation coefficients range from -1.00 through .00 to +1.00. A value of +1 means that the two variables are positively correlated, as one variable goes up, the other goes up. A value of r = 0 means that the two variables are not linearly related.
Often, the data will be presented on a scatter plot. Here, we can view the data and there appears to be a straight line (linear) trend between height and weight. The association (or correlation) is positive. That means, that there is a weight increase with height. The Pearson correlation coefficient in this case was r = 0.56.
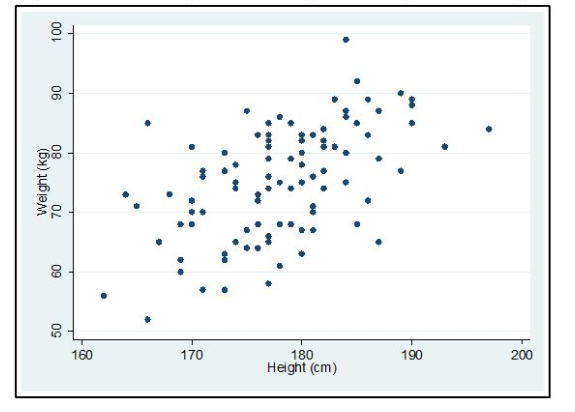
A type I error is made by rejecting a null hypothesis that is true. This means that there was no difference but the researcher concluded that the hypothesis was true.
A type II error is made by accepting that the null hypothesis is true when, in fact, it was false. Meaning there was actually a difference but the researcher did not think their hypothesis was supported.
Hypothesis Testing Procedures : In a general sense, the overall testing of a hypothesis has a systematic methodology. Remember, a hypothesis is an educated guess about the outcome. If we guess wrong, we might set up the tests incorrectly and might get results that are invalid. Sometimes, this is super difficult to get right. The main purpose of statistics is to test a hypothesis.
- Selecting a statistical test. Lots of factors go into this, including levels of measurement of the variables.
- Specifying the level of significance. Usually 0.05 is chosen.
- Computing a test statistic. Lots of software programs to help with this.
- Determining degrees of freedom ( df ). This refers to the number of observations free to vary about a parameter. Computing this is easy (but you don’t need to know how for this course).
- Comparing the test statistic to a theoretical value. Theoretical values exist for all test statistics, which is compared to the study statistics to help establish significance.
Some of the common inferential statistics you will see include:
Comparison tests: Comparison tests look for differences among group means. They can be used to test the effect of a categorical variable on the mean value of some other characteristic.
T-tests are used when comparing the means of precisely two groups (e.g., the average heights of men and women). ANOVA and MANOVA tests are used when comparing the means of more than two groups (e.g., the average heights of children, teenagers, and adults).
- t -tests (compares differences in two groups) – either paired t-test (example: What is the effect of two different test prep programs on the average exam scores for students from the same class?) or independent t-test (example: What is the difference in average exam scores for students from two different schools?)
- analysis of variance (ANOVA, which compares differences in three or more groups) (example: What is the difference in average pain levels among post-surgical patients given three different painkillers?) or MANOVA (compares differences in three or more groups, and 2 or more outcomes) (example: What is the effect of flower species on petal length, petal width, and stem length?)
Correlation tests: Correlation tests check whether variables are related without hypothesizing a cause-and-effect relationship.
- Pearson r (measures the strength and direction of the relationship between two variables) (example: How are latitude and temperature related?)
Nonparametric tests: Non-parametric tests don’t make as many assumptions about the data, and are useful when one or more of the common statistical assumptions are violated. However, the inferences they make aren’t as strong as with parametric tests.
- chi-squared ( X 2 ) test (measures differences in proportions). Chi-square tests are often used to test hypotheses. The chi-square statistic compares the size of any discrepancies between the expected results and the actual results, given the size of the sample and the number of variables in the relationship. For example, the results of tossing a fair coin meet these criteria. We can apply a chi-square test to determine which type of candy is most popular and make sure that our shelves are well stocked. Or maybe you’re a scientist studying the offspring of cats to determine the likelihood of certain genetic traits being passed to a litter of kittens.
Inferential Versus Descriptive Statistics Summary Table
Inferential Statistics | Descriptive Statistics |
Used to make conclusions about the population by using analytical tools on the sample data. | Used to qualify the characteristics of the data. |
Hypothesis testing. | Measures of central tendency and measures of dispersion are the important tools used. |
Is used to make inferences about an unknown population. | Used to describe the characteristics of a known sample or population. |
Measures of inferential statistics include t-tests, ANOVA, chi-squared test, etc. | Measures of descriptive statistics are variances, range, mean, median, etc. |
Statistical Significance Versus Clinical Significance
Finally, when it comes to statistical significance in hypothesis testing, the normal probability value in nursing is <0.05. A p=value (probability) is a statistical measurement used to validate a hypothesis against measured data in the study. Meaning, it measures the likelihood that the results were actually observed due to the intervention, or if the results were just due by chance. The p-value, in measuring the probability of obtaining the observed results, assumes the null hypothesis is true.
The lower the p-value, the greater the statistical significance of the observed difference.
In the example earlier about our diabetic patients receiving online diet education, let’s say we had p = 0.05. Would that be a statistically significant result?
If you answered yes, you are correct!
What if our result was p = 0.8?
Not significant. Good job!
That’s pretty straightforward, right? Below 0.05, significant. Over 0.05 not significant.
Could we have significance clinically even if we do not have statistically significant results? Yes. Let’s explore this a bit.
Statistical hypothesis testing provides little information for interpretation purposes. It’s pretty mathematical and we can still get it wrong. Additionally, attaining statistical significance does not really state whether a finding is clinically meaningful. With a large enough sample, even a small very tiny relationship may be statistically significant. But, clinical significance is the practical importance of research. Meaning, we need to ask what the palpable effects may be on the lives of patients or healthcare decisions.
Remember, hypothesis testing cannot prove. It also cannot tell us much other than “yeah, it’s probably likely that there would be some change with this intervention”. Hypothesis testing tells us the likelihood that the outcome was due to an intervention or influence and not just by chance. Also, as nurses and clinicians, we are not concerned with a group of people – we are concerned at the individual, holistic level. The goal of evidence-based practice is to use best evidence for decisions about specific individual needs.
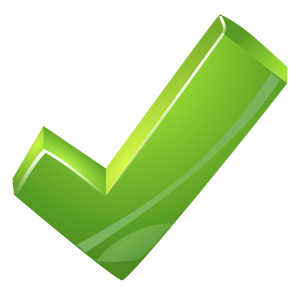
Additionally, begin your Discussion section. What are the implications to practice? Is there little evidence or a lot? Would you recommend additional studies? If so, what type of study would you recommend, and why?
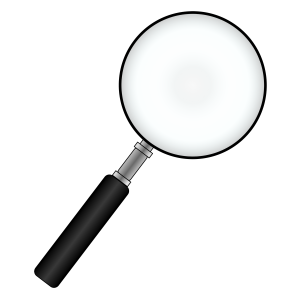
- Were all the important results discussed?
- Did the researchers discuss any study limitations and their possible effects on the credibility of the findings? In discussing limitations, were key threats to the study’s validity and possible biases reviewed? Did the interpretations take limitations into account?
- What types of evidence were offered in support of the interpretation, and was that evidence persuasive? Were results interpreted in light of findings from other studies?
- Did the researchers make any unjustifiable causal inferences? Were alternative explanations for the findings considered? Were the rationales for rejecting these alternatives convincing?
- Did the interpretation consider the precision of the results and/or the magnitude of effects?
- Did the researchers draw any unwarranted conclusions about the generalizability of the results?
- Did the researchers discuss the study’s implications for clinical practice or future nursing research? Did they make specific recommendations?
- If yes, are the stated implications appropriate, given the study’s limitations and the magnitude of the effects as well as evidence from other studies? Are there important implications that the report neglected to include?
- Did the researchers mention or assess clinical significance? Did they make a distinction between statistical and clinical significance?
- If clinical significance was examined, was it assessed in terms of group-level information (e.g., effect sizes) or individual-level results? How was clinical significance operationalized?
References & Attribution
“ Green check mark ” by rawpixel licensed CC0 .
“ Magnifying glass ” by rawpixel licensed CC0
“ Orange flame ” by rawpixel licensed CC0 .
Polit, D. & Beck, C. (2021). Lippincott CoursePoint Enhanced for Polit’s Essentials of Nursing Research (10th ed.). Wolters Kluwer Health
Vaid, N. K. (2019) Statistical performance measures. Medium. https://neeraj-kumar-vaid.medium.com/statistical-performance-measures-12bad66694b7
Evidence-Based Practice & Research Methodologies Copyright © by Tracy Fawns is licensed under a Creative Commons Attribution-NonCommercial-ShareAlike 4.0 International License , except where otherwise noted.
Share This Book
Have a language expert improve your writing
Run a free plagiarism check in 10 minutes, generate accurate citations for free.
- Knowledge Base
The Beginner's Guide to Statistical Analysis | 5 Steps & Examples
Statistical analysis means investigating trends, patterns, and relationships using quantitative data . It is an important research tool used by scientists, governments, businesses, and other organizations.
To draw valid conclusions, statistical analysis requires careful planning from the very start of the research process . You need to specify your hypotheses and make decisions about your research design, sample size, and sampling procedure.
After collecting data from your sample, you can organize and summarize the data using descriptive statistics . Then, you can use inferential statistics to formally test hypotheses and make estimates about the population. Finally, you can interpret and generalize your findings.
This article is a practical introduction to statistical analysis for students and researchers. We’ll walk you through the steps using two research examples. The first investigates a potential cause-and-effect relationship, while the second investigates a potential correlation between variables.
Table of contents
Step 1: write your hypotheses and plan your research design, step 2: collect data from a sample, step 3: summarize your data with descriptive statistics, step 4: test hypotheses or make estimates with inferential statistics, step 5: interpret your results, other interesting articles.
To collect valid data for statistical analysis, you first need to specify your hypotheses and plan out your research design.
Writing statistical hypotheses
The goal of research is often to investigate a relationship between variables within a population . You start with a prediction, and use statistical analysis to test that prediction.
A statistical hypothesis is a formal way of writing a prediction about a population. Every research prediction is rephrased into null and alternative hypotheses that can be tested using sample data.
While the null hypothesis always predicts no effect or no relationship between variables, the alternative hypothesis states your research prediction of an effect or relationship.
- Null hypothesis: A 5-minute meditation exercise will have no effect on math test scores in teenagers.
- Alternative hypothesis: A 5-minute meditation exercise will improve math test scores in teenagers.
- Null hypothesis: Parental income and GPA have no relationship with each other in college students.
- Alternative hypothesis: Parental income and GPA are positively correlated in college students.
Planning your research design
A research design is your overall strategy for data collection and analysis. It determines the statistical tests you can use to test your hypothesis later on.
First, decide whether your research will use a descriptive, correlational, or experimental design. Experiments directly influence variables, whereas descriptive and correlational studies only measure variables.
- In an experimental design , you can assess a cause-and-effect relationship (e.g., the effect of meditation on test scores) using statistical tests of comparison or regression.
- In a correlational design , you can explore relationships between variables (e.g., parental income and GPA) without any assumption of causality using correlation coefficients and significance tests.
- In a descriptive design , you can study the characteristics of a population or phenomenon (e.g., the prevalence of anxiety in U.S. college students) using statistical tests to draw inferences from sample data.
Your research design also concerns whether you’ll compare participants at the group level or individual level, or both.
- In a between-subjects design , you compare the group-level outcomes of participants who have been exposed to different treatments (e.g., those who performed a meditation exercise vs those who didn’t).
- In a within-subjects design , you compare repeated measures from participants who have participated in all treatments of a study (e.g., scores from before and after performing a meditation exercise).
- In a mixed (factorial) design , one variable is altered between subjects and another is altered within subjects (e.g., pretest and posttest scores from participants who either did or didn’t do a meditation exercise).
- Experimental
- Correlational
First, you’ll take baseline test scores from participants. Then, your participants will undergo a 5-minute meditation exercise. Finally, you’ll record participants’ scores from a second math test.
In this experiment, the independent variable is the 5-minute meditation exercise, and the dependent variable is the math test score from before and after the intervention. Example: Correlational research design In a correlational study, you test whether there is a relationship between parental income and GPA in graduating college students. To collect your data, you will ask participants to fill in a survey and self-report their parents’ incomes and their own GPA.
Measuring variables
When planning a research design, you should operationalize your variables and decide exactly how you will measure them.
For statistical analysis, it’s important to consider the level of measurement of your variables, which tells you what kind of data they contain:
- Categorical data represents groupings. These may be nominal (e.g., gender) or ordinal (e.g. level of language ability).
- Quantitative data represents amounts. These may be on an interval scale (e.g. test score) or a ratio scale (e.g. age).
Many variables can be measured at different levels of precision. For example, age data can be quantitative (8 years old) or categorical (young). If a variable is coded numerically (e.g., level of agreement from 1–5), it doesn’t automatically mean that it’s quantitative instead of categorical.
Identifying the measurement level is important for choosing appropriate statistics and hypothesis tests. For example, you can calculate a mean score with quantitative data, but not with categorical data.
In a research study, along with measures of your variables of interest, you’ll often collect data on relevant participant characteristics.
Variable | Type of data |
---|---|
Age | Quantitative (ratio) |
Gender | Categorical (nominal) |
Race or ethnicity | Categorical (nominal) |
Baseline test scores | Quantitative (interval) |
Final test scores | Quantitative (interval) |
Parental income | Quantitative (ratio) |
---|---|
GPA | Quantitative (interval) |
Here's why students love Scribbr's proofreading services
Discover proofreading & editing
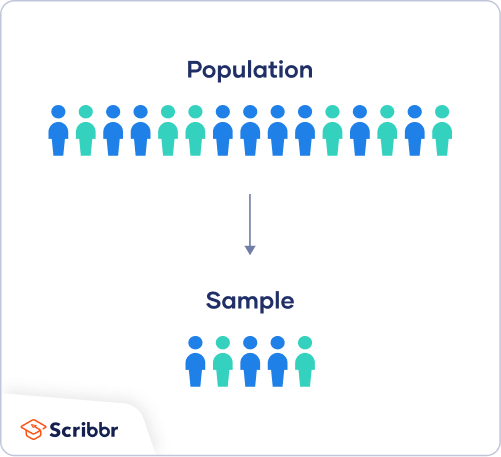
In most cases, it’s too difficult or expensive to collect data from every member of the population you’re interested in studying. Instead, you’ll collect data from a sample.
Statistical analysis allows you to apply your findings beyond your own sample as long as you use appropriate sampling procedures . You should aim for a sample that is representative of the population.
Sampling for statistical analysis
There are two main approaches to selecting a sample.
- Probability sampling: every member of the population has a chance of being selected for the study through random selection.
- Non-probability sampling: some members of the population are more likely than others to be selected for the study because of criteria such as convenience or voluntary self-selection.
In theory, for highly generalizable findings, you should use a probability sampling method. Random selection reduces several types of research bias , like sampling bias , and ensures that data from your sample is actually typical of the population. Parametric tests can be used to make strong statistical inferences when data are collected using probability sampling.
But in practice, it’s rarely possible to gather the ideal sample. While non-probability samples are more likely to at risk for biases like self-selection bias , they are much easier to recruit and collect data from. Non-parametric tests are more appropriate for non-probability samples, but they result in weaker inferences about the population.
If you want to use parametric tests for non-probability samples, you have to make the case that:
- your sample is representative of the population you’re generalizing your findings to.
- your sample lacks systematic bias.
Keep in mind that external validity means that you can only generalize your conclusions to others who share the characteristics of your sample. For instance, results from Western, Educated, Industrialized, Rich and Democratic samples (e.g., college students in the US) aren’t automatically applicable to all non-WEIRD populations.
If you apply parametric tests to data from non-probability samples, be sure to elaborate on the limitations of how far your results can be generalized in your discussion section .
Create an appropriate sampling procedure
Based on the resources available for your research, decide on how you’ll recruit participants.
- Will you have resources to advertise your study widely, including outside of your university setting?
- Will you have the means to recruit a diverse sample that represents a broad population?
- Do you have time to contact and follow up with members of hard-to-reach groups?
Your participants are self-selected by their schools. Although you’re using a non-probability sample, you aim for a diverse and representative sample. Example: Sampling (correlational study) Your main population of interest is male college students in the US. Using social media advertising, you recruit senior-year male college students from a smaller subpopulation: seven universities in the Boston area.
Calculate sufficient sample size
Before recruiting participants, decide on your sample size either by looking at other studies in your field or using statistics. A sample that’s too small may be unrepresentative of the sample, while a sample that’s too large will be more costly than necessary.
There are many sample size calculators online. Different formulas are used depending on whether you have subgroups or how rigorous your study should be (e.g., in clinical research). As a rule of thumb, a minimum of 30 units or more per subgroup is necessary.
To use these calculators, you have to understand and input these key components:
- Significance level (alpha): the risk of rejecting a true null hypothesis that you are willing to take, usually set at 5%.
- Statistical power : the probability of your study detecting an effect of a certain size if there is one, usually 80% or higher.
- Expected effect size : a standardized indication of how large the expected result of your study will be, usually based on other similar studies.
- Population standard deviation: an estimate of the population parameter based on a previous study or a pilot study of your own.
Once you’ve collected all of your data, you can inspect them and calculate descriptive statistics that summarize them.
Inspect your data
There are various ways to inspect your data, including the following:
- Organizing data from each variable in frequency distribution tables .
- Displaying data from a key variable in a bar chart to view the distribution of responses.
- Visualizing the relationship between two variables using a scatter plot .
By visualizing your data in tables and graphs, you can assess whether your data follow a skewed or normal distribution and whether there are any outliers or missing data.
A normal distribution means that your data are symmetrically distributed around a center where most values lie, with the values tapering off at the tail ends.
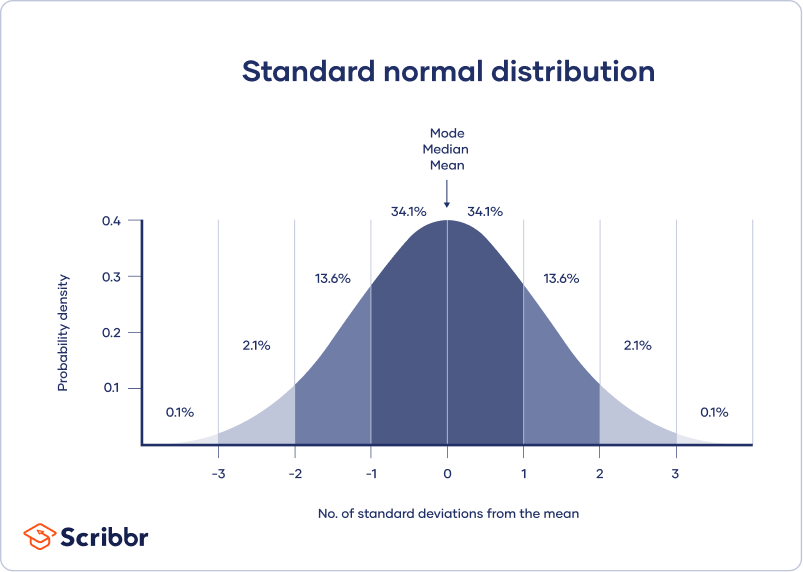
In contrast, a skewed distribution is asymmetric and has more values on one end than the other. The shape of the distribution is important to keep in mind because only some descriptive statistics should be used with skewed distributions.
Extreme outliers can also produce misleading statistics, so you may need a systematic approach to dealing with these values.
Calculate measures of central tendency
Measures of central tendency describe where most of the values in a data set lie. Three main measures of central tendency are often reported:
- Mode : the most popular response or value in the data set.
- Median : the value in the exact middle of the data set when ordered from low to high.
- Mean : the sum of all values divided by the number of values.
However, depending on the shape of the distribution and level of measurement, only one or two of these measures may be appropriate. For example, many demographic characteristics can only be described using the mode or proportions, while a variable like reaction time may not have a mode at all.
Calculate measures of variability
Measures of variability tell you how spread out the values in a data set are. Four main measures of variability are often reported:
- Range : the highest value minus the lowest value of the data set.
- Interquartile range : the range of the middle half of the data set.
- Standard deviation : the average distance between each value in your data set and the mean.
- Variance : the square of the standard deviation.
Once again, the shape of the distribution and level of measurement should guide your choice of variability statistics. The interquartile range is the best measure for skewed distributions, while standard deviation and variance provide the best information for normal distributions.
Using your table, you should check whether the units of the descriptive statistics are comparable for pretest and posttest scores. For example, are the variance levels similar across the groups? Are there any extreme values? If there are, you may need to identify and remove extreme outliers in your data set or transform your data before performing a statistical test.
Pretest scores | Posttest scores | |
---|---|---|
Mean | 68.44 | 75.25 |
Standard deviation | 9.43 | 9.88 |
Variance | 88.96 | 97.96 |
Range | 36.25 | 45.12 |
30 |
From this table, we can see that the mean score increased after the meditation exercise, and the variances of the two scores are comparable. Next, we can perform a statistical test to find out if this improvement in test scores is statistically significant in the population. Example: Descriptive statistics (correlational study) After collecting data from 653 students, you tabulate descriptive statistics for annual parental income and GPA.
It’s important to check whether you have a broad range of data points. If you don’t, your data may be skewed towards some groups more than others (e.g., high academic achievers), and only limited inferences can be made about a relationship.
Parental income (USD) | GPA | |
---|---|---|
Mean | 62,100 | 3.12 |
Standard deviation | 15,000 | 0.45 |
Variance | 225,000,000 | 0.16 |
Range | 8,000–378,000 | 2.64–4.00 |
653 |
A number that describes a sample is called a statistic , while a number describing a population is called a parameter . Using inferential statistics , you can make conclusions about population parameters based on sample statistics.
Researchers often use two main methods (simultaneously) to make inferences in statistics.
- Estimation: calculating population parameters based on sample statistics.
- Hypothesis testing: a formal process for testing research predictions about the population using samples.
You can make two types of estimates of population parameters from sample statistics:
- A point estimate : a value that represents your best guess of the exact parameter.
- An interval estimate : a range of values that represent your best guess of where the parameter lies.
If your aim is to infer and report population characteristics from sample data, it’s best to use both point and interval estimates in your paper.
You can consider a sample statistic a point estimate for the population parameter when you have a representative sample (e.g., in a wide public opinion poll, the proportion of a sample that supports the current government is taken as the population proportion of government supporters).
There’s always error involved in estimation, so you should also provide a confidence interval as an interval estimate to show the variability around a point estimate.
A confidence interval uses the standard error and the z score from the standard normal distribution to convey where you’d generally expect to find the population parameter most of the time.
Hypothesis testing
Using data from a sample, you can test hypotheses about relationships between variables in the population. Hypothesis testing starts with the assumption that the null hypothesis is true in the population, and you use statistical tests to assess whether the null hypothesis can be rejected or not.
Statistical tests determine where your sample data would lie on an expected distribution of sample data if the null hypothesis were true. These tests give two main outputs:
- A test statistic tells you how much your data differs from the null hypothesis of the test.
- A p value tells you the likelihood of obtaining your results if the null hypothesis is actually true in the population.
Statistical tests come in three main varieties:
- Comparison tests assess group differences in outcomes.
- Regression tests assess cause-and-effect relationships between variables.
- Correlation tests assess relationships between variables without assuming causation.
Your choice of statistical test depends on your research questions, research design, sampling method, and data characteristics.
Parametric tests
Parametric tests make powerful inferences about the population based on sample data. But to use them, some assumptions must be met, and only some types of variables can be used. If your data violate these assumptions, you can perform appropriate data transformations or use alternative non-parametric tests instead.
A regression models the extent to which changes in a predictor variable results in changes in outcome variable(s).
- A simple linear regression includes one predictor variable and one outcome variable.
- A multiple linear regression includes two or more predictor variables and one outcome variable.
Comparison tests usually compare the means of groups. These may be the means of different groups within a sample (e.g., a treatment and control group), the means of one sample group taken at different times (e.g., pretest and posttest scores), or a sample mean and a population mean.
- A t test is for exactly 1 or 2 groups when the sample is small (30 or less).
- A z test is for exactly 1 or 2 groups when the sample is large.
- An ANOVA is for 3 or more groups.
The z and t tests have subtypes based on the number and types of samples and the hypotheses:
- If you have only one sample that you want to compare to a population mean, use a one-sample test .
- If you have paired measurements (within-subjects design), use a dependent (paired) samples test .
- If you have completely separate measurements from two unmatched groups (between-subjects design), use an independent (unpaired) samples test .
- If you expect a difference between groups in a specific direction, use a one-tailed test .
- If you don’t have any expectations for the direction of a difference between groups, use a two-tailed test .
The only parametric correlation test is Pearson’s r . The correlation coefficient ( r ) tells you the strength of a linear relationship between two quantitative variables.
However, to test whether the correlation in the sample is strong enough to be important in the population, you also need to perform a significance test of the correlation coefficient, usually a t test, to obtain a p value. This test uses your sample size to calculate how much the correlation coefficient differs from zero in the population.
You use a dependent-samples, one-tailed t test to assess whether the meditation exercise significantly improved math test scores. The test gives you:
- a t value (test statistic) of 3.00
- a p value of 0.0028
Although Pearson’s r is a test statistic, it doesn’t tell you anything about how significant the correlation is in the population. You also need to test whether this sample correlation coefficient is large enough to demonstrate a correlation in the population.
A t test can also determine how significantly a correlation coefficient differs from zero based on sample size. Since you expect a positive correlation between parental income and GPA, you use a one-sample, one-tailed t test. The t test gives you:
- a t value of 3.08
- a p value of 0.001
The final step of statistical analysis is interpreting your results.
Statistical significance
In hypothesis testing, statistical significance is the main criterion for forming conclusions. You compare your p value to a set significance level (usually 0.05) to decide whether your results are statistically significant or non-significant.
Statistically significant results are considered unlikely to have arisen solely due to chance. There is only a very low chance of such a result occurring if the null hypothesis is true in the population.
This means that you believe the meditation intervention, rather than random factors, directly caused the increase in test scores. Example: Interpret your results (correlational study) You compare your p value of 0.001 to your significance threshold of 0.05. With a p value under this threshold, you can reject the null hypothesis. This indicates a statistically significant correlation between parental income and GPA in male college students.
Note that correlation doesn’t always mean causation, because there are often many underlying factors contributing to a complex variable like GPA. Even if one variable is related to another, this may be because of a third variable influencing both of them, or indirect links between the two variables.
Effect size
A statistically significant result doesn’t necessarily mean that there are important real life applications or clinical outcomes for a finding.
In contrast, the effect size indicates the practical significance of your results. It’s important to report effect sizes along with your inferential statistics for a complete picture of your results. You should also report interval estimates of effect sizes if you’re writing an APA style paper .
With a Cohen’s d of 0.72, there’s medium to high practical significance to your finding that the meditation exercise improved test scores. Example: Effect size (correlational study) To determine the effect size of the correlation coefficient, you compare your Pearson’s r value to Cohen’s effect size criteria.
Decision errors
Type I and Type II errors are mistakes made in research conclusions. A Type I error means rejecting the null hypothesis when it’s actually true, while a Type II error means failing to reject the null hypothesis when it’s false.
You can aim to minimize the risk of these errors by selecting an optimal significance level and ensuring high power . However, there’s a trade-off between the two errors, so a fine balance is necessary.
Frequentist versus Bayesian statistics
Traditionally, frequentist statistics emphasizes null hypothesis significance testing and always starts with the assumption of a true null hypothesis.
However, Bayesian statistics has grown in popularity as an alternative approach in the last few decades. In this approach, you use previous research to continually update your hypotheses based on your expectations and observations.
Bayes factor compares the relative strength of evidence for the null versus the alternative hypothesis rather than making a conclusion about rejecting the null hypothesis or not.
If you want to know more about statistics , methodology , or research bias , make sure to check out some of our other articles with explanations and examples.
- Student’s t -distribution
- Normal distribution
- Null and Alternative Hypotheses
- Chi square tests
- Confidence interval
Methodology
- Cluster sampling
- Stratified sampling
- Data cleansing
- Reproducibility vs Replicability
- Peer review
- Likert scale
Research bias
- Implicit bias
- Framing effect
- Cognitive bias
- Placebo effect
- Hawthorne effect
- Hostile attribution bias
- Affect heuristic
Is this article helpful?
Other students also liked.
- Descriptive Statistics | Definitions, Types, Examples
- Inferential Statistics | An Easy Introduction & Examples
- Choosing the Right Statistical Test | Types & Examples
More interesting articles
- Akaike Information Criterion | When & How to Use It (Example)
- An Easy Introduction to Statistical Significance (With Examples)
- An Introduction to t Tests | Definitions, Formula and Examples
- ANOVA in R | A Complete Step-by-Step Guide with Examples
- Central Limit Theorem | Formula, Definition & Examples
- Central Tendency | Understanding the Mean, Median & Mode
- Chi-Square (Χ²) Distributions | Definition & Examples
- Chi-Square (Χ²) Table | Examples & Downloadable Table
- Chi-Square (Χ²) Tests | Types, Formula & Examples
- Chi-Square Goodness of Fit Test | Formula, Guide & Examples
- Chi-Square Test of Independence | Formula, Guide & Examples
- Coefficient of Determination (R²) | Calculation & Interpretation
- Correlation Coefficient | Types, Formulas & Examples
- Frequency Distribution | Tables, Types & Examples
- How to Calculate Standard Deviation (Guide) | Calculator & Examples
- How to Calculate Variance | Calculator, Analysis & Examples
- How to Find Degrees of Freedom | Definition & Formula
- How to Find Interquartile Range (IQR) | Calculator & Examples
- How to Find Outliers | 4 Ways with Examples & Explanation
- How to Find the Geometric Mean | Calculator & Formula
- How to Find the Mean | Definition, Examples & Calculator
- How to Find the Median | Definition, Examples & Calculator
- How to Find the Mode | Definition, Examples & Calculator
- How to Find the Range of a Data Set | Calculator & Formula
- Hypothesis Testing | A Step-by-Step Guide with Easy Examples
- Interval Data and How to Analyze It | Definitions & Examples
- Levels of Measurement | Nominal, Ordinal, Interval and Ratio
- Linear Regression in R | A Step-by-Step Guide & Examples
- Missing Data | Types, Explanation, & Imputation
- Multiple Linear Regression | A Quick Guide (Examples)
- Nominal Data | Definition, Examples, Data Collection & Analysis
- Normal Distribution | Examples, Formulas, & Uses
- Null and Alternative Hypotheses | Definitions & Examples
- One-way ANOVA | When and How to Use It (With Examples)
- Ordinal Data | Definition, Examples, Data Collection & Analysis
- Parameter vs Statistic | Definitions, Differences & Examples
- Pearson Correlation Coefficient (r) | Guide & Examples
- Poisson Distributions | Definition, Formula & Examples
- Probability Distribution | Formula, Types, & Examples
- Quartiles & Quantiles | Calculation, Definition & Interpretation
- Ratio Scales | Definition, Examples, & Data Analysis
- Simple Linear Regression | An Easy Introduction & Examples
- Skewness | Definition, Examples & Formula
- Statistical Power and Why It Matters | A Simple Introduction
- Student's t Table (Free Download) | Guide & Examples
- T-distribution: What it is and how to use it
- Test statistics | Definition, Interpretation, and Examples
- The Standard Normal Distribution | Calculator, Examples & Uses
- Two-Way ANOVA | Examples & When To Use It
- Type I & Type II Errors | Differences, Examples, Visualizations
- Understanding Confidence Intervals | Easy Examples & Formulas
- Understanding P values | Definition and Examples
- Variability | Calculating Range, IQR, Variance, Standard Deviation
- What is Effect Size and Why Does It Matter? (Examples)
- What Is Kurtosis? | Definition, Examples & Formula
- What Is Standard Error? | How to Calculate (Guide with Examples)
What is your plagiarism score?
Are you an agency specialized in UX, digital marketing, or growth? Join our Partner Program
Learn / Guides / Quantitative data analysis guide
Back to guides
8 quantitative data analysis methods to turn numbers into insights
Setting up a few new customer surveys or creating a fresh Google Analytics dashboard feels exciting…until the numbers start rolling in. You want to turn responses into a plan to present to your team and leaders—but which quantitative data analysis method do you use to make sense of the facts and figures?
Last updated
Reading time.
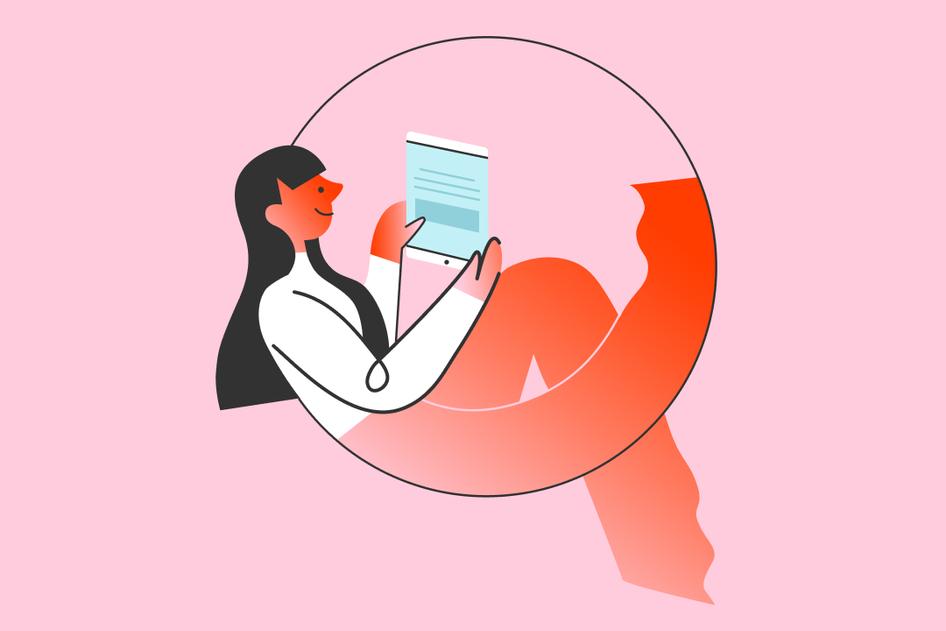
This guide lists eight quantitative research data analysis techniques to help you turn numeric feedback into actionable insights to share with your team and make customer-centric decisions.
To pick the right technique that helps you bridge the gap between data and decision-making, you first need to collect quantitative data from sources like:
Google Analytics
Survey results
On-page feedback scores
Fuel your quantitative analysis with real-time data
Use Hotjar’s tools to collect quantitative data that helps you stay close to customers.
Then, choose an analysis method based on the type of data and how you want to use it.
Descriptive data analysis summarizes results—like measuring website traffic—that help you learn about a problem or opportunity. The descriptive analysis methods we’ll review are:
Multiple choice response rates
Response volume over time
Net Promoter Score®
Inferential data analyzes the relationship between data—like which customer segment has the highest average order value—to help you make hypotheses about product decisions. Inferential analysis methods include:
Cross-tabulation
Weighted customer feedback
You don’t need to worry too much about these specific terms since each quantitative data analysis method listed below explains when and how to use them. Let’s dive in!
1. Compare multiple-choice response rates
The simplest way to analyze survey data is by comparing the percentage of your users who chose each response, which summarizes opinions within your audience.
To do this, divide the number of people who chose a specific response by the total respondents for your multiple-choice survey. Imagine 100 customers respond to a survey about what product category they want to see. If 25 people said ‘snacks’, 25% of your audience favors that category, so you know that adding a snacks category to your list of filters or drop-down menu will make the purchasing process easier for them.
💡Pro tip: ask open-ended survey questions to dig deeper into customer motivations.
A multiple-choice survey measures your audience’s opinions, but numbers don’t tell you why they think the way they do—you need to combine quantitative and qualitative data to learn that.
One research method to learn about customer motivations is through an open-ended survey question. Giving customers space to express their thoughts in their own words—unrestricted by your pre-written multiple-choice questions—prevents you from making assumptions.
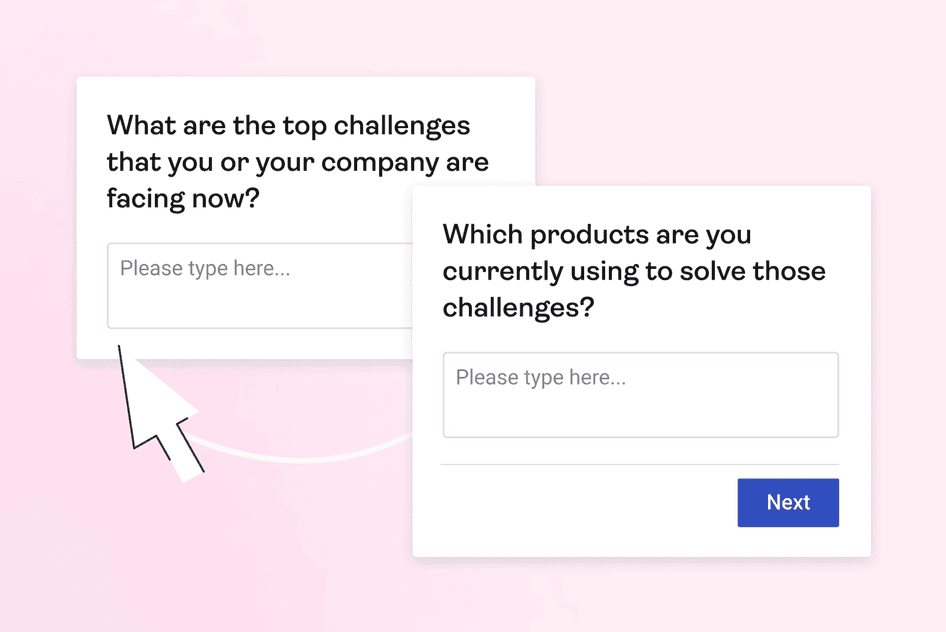
Hotjar’s open-ended surveys have a text box for customers to type a response
2. Cross-tabulate to compare responses between groups
To understand how responses and behavior vary within your audience, compare your quantitative data by group. Use raw numbers, like the number of website visitors, or percentages, like questionnaire responses, across categories like traffic sources or customer segments.
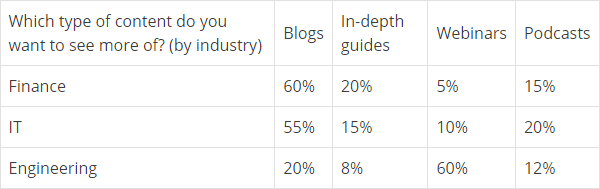
Let’s say you ask your audience what their most-used feature is because you want to know what to highlight on your pricing page. Comparing the most common response for free trial users vs. established customers lets you strategically introduce features at the right point in the customer journey .
💡Pro tip: get some face-to-face time to discover nuances in customer feedback.
Rather than treating your customers as a monolith, use Hotjar to conduct interviews to learn about individuals and subgroups. If you aren’t sure what to ask, start with your quantitative data results. If you notice competing trends between customer segments, have a few conversations with individuals from each group to dig into their unique motivations.
Hotjar Engage lets you identify specific customer segments you want to talk to
Mode is the most common answer in a data set, which means you use it to discover the most popular response for questions with numeric answer options. Mode and median (that's next on the list) are useful to compare to the average in case responses on extreme ends of the scale (outliers) skew the outcome.
Let’s say you want to know how most customers feel about your website, so you use an on-page feedback widget to collect ratings on a scale of one to five.
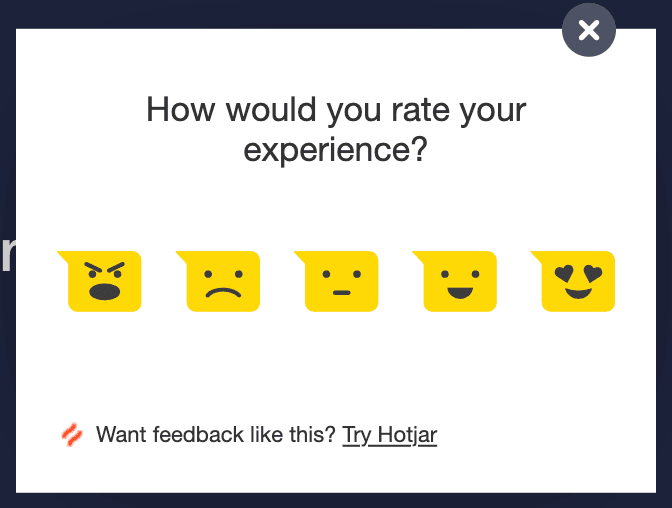
If the mode, or most common response, is a three, you can assume most people feel somewhat positive. But suppose the second-most common response is a one (which would bring the average down). In that case, you need to investigate why so many customers are unhappy.
💡Pro tip: watch recordings to understand how customers interact with your website.
So you used on-page feedback to learn how customers feel about your website, and the mode was two out of five. Ouch. Use Hotjar Recordings to see how customers move around on and interact with your pages to find the source of frustration.
Hotjar Recordings lets you watch individual visitors interact with your site, like how they scroll, hover, and click
Median reveals the middle of the road of your quantitative data by lining up all numeric values in ascending order and then looking at the data point in the middle. Use the median method when you notice a few outliers that bring the average up or down and compare the analysis outcomes.
For example, if your price sensitivity survey has outlandish responses and you want to identify a reasonable middle ground of what customers are willing to pay—calculate the median.
💡Pro-tip: review and clean your data before analysis.
Take a few minutes to familiarize yourself with quantitative data results before you push them through analysis methods. Inaccurate or missing information can complicate your calculations, and it’s less frustrating to resolve issues at the start instead of problem-solving later.
Here are a few data-cleaning tips to keep in mind:
Remove or separate irrelevant data, like responses from a customer segment or time frame you aren’t reviewing right now
Standardize data from multiple sources, like a survey that let customers indicate they use your product ‘daily’ vs. on-page feedback that used the phrasing ‘more than once a week’
Acknowledge missing data, like some customers not answering every question. Just note that your totals between research questions might not match.
Ensure you have enough responses to have a statistically significant result
Decide if you want to keep or remove outlying data. For example, maybe there’s evidence to support a high-price tier, and you shouldn’t dismiss less price-sensitive respondents. Other times, you might want to get rid of obviously trolling responses.
5. Mean (AKA average)
Finding the average of a dataset is an essential quantitative data analysis method and an easy task. First, add all your quantitative data points, like numeric survey responses or daily sales revenue. Then, divide the sum of your data points by the number of responses to get a single number representing the entire dataset.
Use the average of your quant data when you want a summary, like the average order value of your transactions between different sales pages. Then, use your average to benchmark performance, compare over time, or uncover winners across segments—like which sales page design produces the most value.
💡Pro tip: use heatmaps to find attention-catching details numbers can’t give you.
Calculating the average of your quant data set reveals the outcome of customer interactions. However, you need qualitative data like a heatmap to learn about everything that led to that moment. A heatmap uses colors to illustrate where most customers look and click on a page to reveal what drives (or drops) momentum.
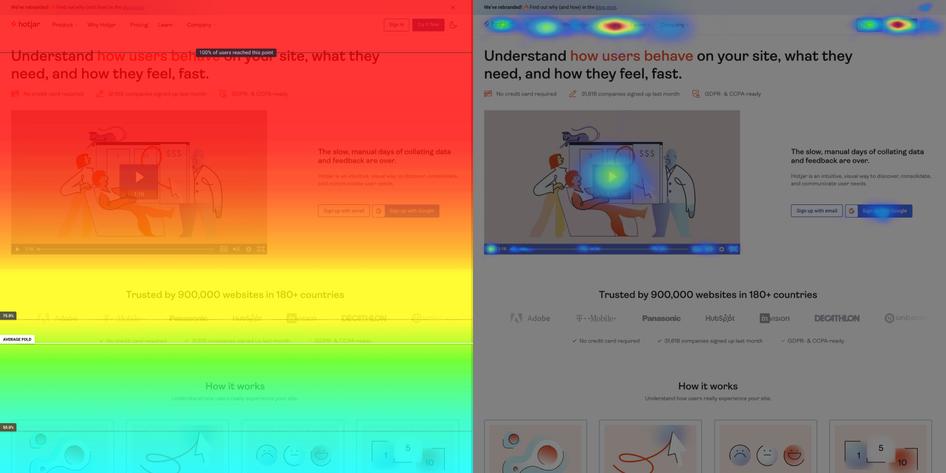
Hotjar Heatmaps uses color to visualize what most visitors see, ignore, and click on
6. Measure the volume of responses over time
Some quantitative data analysis methods are an ongoing project, like comparing top website referral sources by month to gauge the effectiveness of new channels. Analyzing the same metric at regular intervals lets you compare trends and changes.
Look at quantitative survey results, website sessions, sales, cart abandons, or clicks regularly to spot trouble early or monitor the impact of a new initiative.
Here are a few areas you can measure over time (and how to use qualitative research methods listed above to add context to your results):
7. Net Promoter Score®
Net Promoter Score® ( NPS ®) is a popular customer loyalty and satisfaction measurement that also serves as a quantitative data analysis method.
NPS surveys ask customers to rate how likely they are to recommend you on a scale of zero to ten. Calculate it by subtracting the percentage of customers who answer the NPS question with a six or lower (known as ‘detractors’) from those who respond with a nine or ten (known as ‘promoters’). Your NPS score will fall between -100 and 100, and you want a positive number indicating more promoters than detractors.
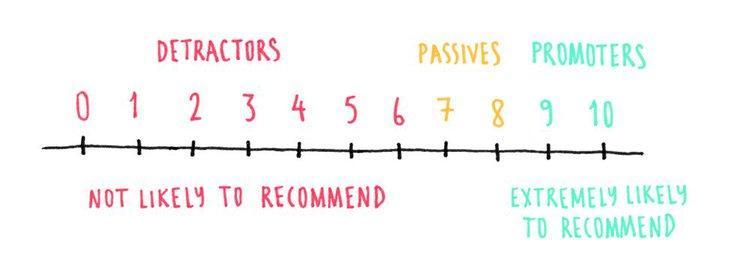
💡Pro tip : like other quantitative data analysis methods, you can review NPS scores over time as a satisfaction benchmark. You can also use it to understand which customer segment is most satisfied or which customers may be willing to share their stories for promotional materials.
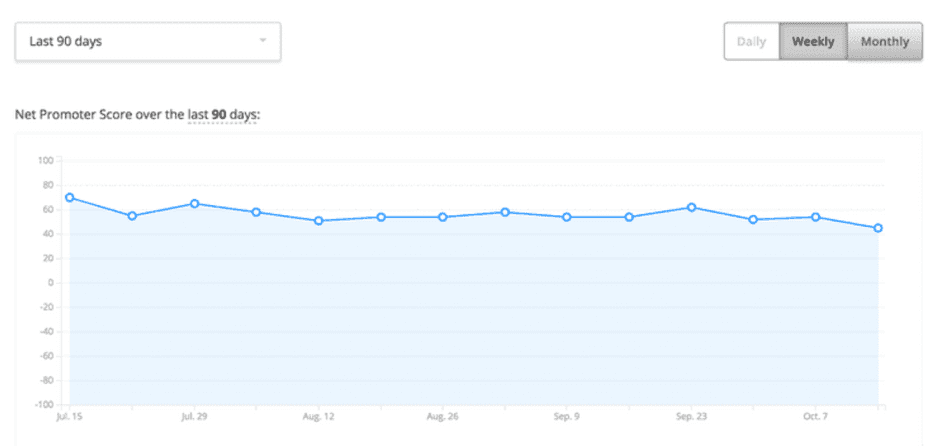
Review NPS score trends with Hotjar to spot any sudden spikes and benchmark performance over time
8. Weight customer feedback
So far, the quantitative data analysis methods on this list have leveraged numeric data only. However, there are ways to turn qualitative data into quantifiable feedback and to mix and match data sources. For example, you might need to analyze user feedback from multiple surveys.
To leverage multiple data points, create a prioritization matrix that assigns ‘weight’ to customer feedback data and company priorities and then multiply them to reveal the highest-scoring option.
Let’s say you identify the top four responses to your churn survey . Rate the most common issue as a four and work down the list until one—these are your customer priorities. Then, rate the ease of fixing each problem with a maximum score of four for the easy wins down to one for difficult tasks—these are your company priorities. Finally, multiply the score of each customer priority with its coordinating company priority scores and lead with the highest scoring idea.
💡Pro-tip: use a product prioritization framework to make decisions.
Try a product prioritization framework when the pressure is on to make high-impact decisions with limited time and budget. These repeatable decision-making tools take the guesswork out of balancing goals, customer priorities, and team resources. Four popular frameworks are:
RICE: weighs four factors—reach, impact, confidence, and effort—to weigh initiatives differently
MoSCoW: considers stakeholder opinions on 'must-have', 'should-have', 'could-have', and 'won't-have' criteria
Kano: ranks ideas based on how likely they are to satisfy customer needs
Cost of delay analysis: determines potential revenue loss by not working on a product or initiative
Share what you learn with data visuals
Data visualization through charts and graphs gives you a new perspective on your results. Plus, removing the clutter of the analysis process helps you and stakeholders focus on the insight over the method.
Data visualization helps you:
Get buy-in with impactful charts that summarize your results
Increase customer empathy and awareness across your company with digestible insights
Use these four data visualization types to illustrate what you learned from your quantitative data analysis:
Bar charts reveal response distribution across multiple options
Line graphs compare data points over time
Scatter plots showcase how two variables interact
Matrices contrast data between categories like customer segments, product types, or traffic source
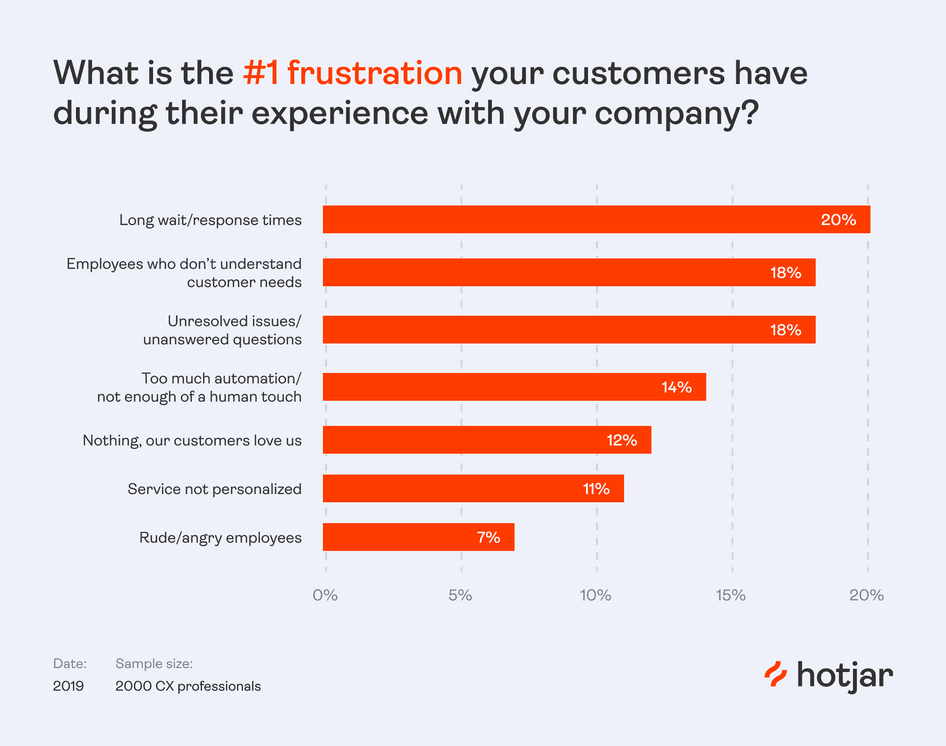
Use a variety of customer feedback types to get the whole picture
Quantitative data analysis pulls the story out of raw numbers—but you shouldn’t take a single result from your data collection and run with it. Instead, combine numbers-based quantitative data with descriptive qualitative research to learn the what, why, and how of customer experiences.
Looking at an opportunity from multiple angles helps you make more customer-centric decisions with less guesswork.
Stay close to customers with Hotjar
Hotjar’s tools offer quantitative and qualitative insights you can use to make customer-centric decisions, get buy-in, and highlight your team’s impact.
Frequently asked questions about quantitative data analysis
What is quantitative data.
Quantitative data is numeric feedback and information that you can count and measure. For example, you can calculate multiple-choice response rates, but you can’t tally a customer’s open-ended product feedback response. You have to use qualitative data analysis methods for non-numeric feedback.
What are quantitative data analysis methods?
Quantitative data analysis either summarizes or finds connections between numerical data feedback. Here are eight ways to analyze your online business’s quantitative data:
Compare multiple-choice response rates
Cross-tabulate to compare responses between groups
Measure the volume of response over time
Net Promoter Score
Weight customer feedback
How do you visualize quantitative data?
Data visualization makes it easier to spot trends and share your analysis with stakeholders. Bar charts, line graphs, scatter plots, and matrices are ways to visualize quantitative data.
What are the two types of statistical analysis for online businesses?
Quantitative data analysis is broken down into two analysis technique types:
Descriptive statistics summarize your collected data, like the number of website visitors this month
Inferential statistics compare relationships between multiple types of quantitative data, like survey responses between different customer segments
Quantitative data analysis process
Previous chapter
Quantitative data analysis software
Next chapter
Have a language expert improve your writing
Run a free plagiarism check in 10 minutes, automatically generate references for free.
- Knowledge Base
- Methodology
- What Is Quantitative Research? | Definition & Methods
What Is Quantitative Research? | Definition & Methods
Published on 4 April 2022 by Pritha Bhandari . Revised on 10 October 2022.
Quantitative research is the process of collecting and analysing numerical data. It can be used to find patterns and averages, make predictions, test causal relationships, and generalise results to wider populations.
Quantitative research is the opposite of qualitative research , which involves collecting and analysing non-numerical data (e.g. text, video, or audio).
Quantitative research is widely used in the natural and social sciences: biology, chemistry, psychology, economics, sociology, marketing, etc.
- What is the demographic makeup of Singapore in 2020?
- How has the average temperature changed globally over the last century?
- Does environmental pollution affect the prevalence of honey bees?
- Does working from home increase productivity for people with long commutes?
Table of contents
Quantitative research methods, quantitative data analysis, advantages of quantitative research, disadvantages of quantitative research, frequently asked questions about quantitative research.
You can use quantitative research methods for descriptive, correlational or experimental research.
- In descriptive research , you simply seek an overall summary of your study variables.
- In correlational research , you investigate relationships between your study variables.
- In experimental research , you systematically examine whether there is a cause-and-effect relationship between variables.
Correlational and experimental research can both be used to formally test hypotheses , or predictions, using statistics. The results may be generalised to broader populations based on the sampling method used.
To collect quantitative data, you will often need to use operational definitions that translate abstract concepts (e.g., mood) into observable and quantifiable measures (e.g., self-ratings of feelings and energy levels).
Research method | How to use | Example |
---|---|---|
Control or manipulate an to measure its effect on a dependent variable. | To test whether an intervention can reduce procrastination in college students, you give equal-sized groups either a procrastination intervention or a comparable task. You compare self-ratings of procrastination behaviors between the groups after the intervention. | |
Ask questions of a group of people in-person, over-the-phone or online. | You distribute with rating scales to first-year international college students to investigate their experiences of culture shock. | |
(Systematic) observation | Identify a behavior or occurrence of interest and monitor it in its natural setting. | To study college classroom participation, you sit in on classes to observe them, counting and recording the prevalence of active and passive behaviors by students from different backgrounds. |
Secondary research | Collect data that has been gathered for other purposes e.g., national surveys or historical records. | To assess whether attitudes towards climate change have changed since the 1980s, you collect relevant questionnaire data from widely available . |
Prevent plagiarism, run a free check.
Once data is collected, you may need to process it before it can be analysed. For example, survey and test data may need to be transformed from words to numbers. Then, you can use statistical analysis to answer your research questions .
Descriptive statistics will give you a summary of your data and include measures of averages and variability. You can also use graphs, scatter plots and frequency tables to visualise your data and check for any trends or outliers.
Using inferential statistics , you can make predictions or generalisations based on your data. You can test your hypothesis or use your sample data to estimate the population parameter .
You can also assess the reliability and validity of your data collection methods to indicate how consistently and accurately your methods actually measured what you wanted them to.
Quantitative research is often used to standardise data collection and generalise findings . Strengths of this approach include:
- Replication
Repeating the study is possible because of standardised data collection protocols and tangible definitions of abstract concepts.
- Direct comparisons of results
The study can be reproduced in other cultural settings, times or with different groups of participants. Results can be compared statistically.
- Large samples
Data from large samples can be processed and analysed using reliable and consistent procedures through quantitative data analysis.
- Hypothesis testing
Using formalised and established hypothesis testing procedures means that you have to carefully consider and report your research variables, predictions, data collection and testing methods before coming to a conclusion.
Despite the benefits of quantitative research, it is sometimes inadequate in explaining complex research topics. Its limitations include:
- Superficiality
Using precise and restrictive operational definitions may inadequately represent complex concepts. For example, the concept of mood may be represented with just a number in quantitative research, but explained with elaboration in qualitative research.
- Narrow focus
Predetermined variables and measurement procedures can mean that you ignore other relevant observations.
- Structural bias
Despite standardised procedures, structural biases can still affect quantitative research. Missing data , imprecise measurements or inappropriate sampling methods are biases that can lead to the wrong conclusions.
- Lack of context
Quantitative research often uses unnatural settings like laboratories or fails to consider historical and cultural contexts that may affect data collection and results.
Quantitative research deals with numbers and statistics, while qualitative research deals with words and meanings.
Quantitative methods allow you to test a hypothesis by systematically collecting and analysing data, while qualitative methods allow you to explore ideas and experiences in depth.
In mixed methods research , you use both qualitative and quantitative data collection and analysis methods to answer your research question .
Data collection is the systematic process by which observations or measurements are gathered in research. It is used in many different contexts by academics, governments, businesses, and other organisations.
Operationalisation means turning abstract conceptual ideas into measurable observations.
For example, the concept of social anxiety isn’t directly observable, but it can be operationally defined in terms of self-rating scores, behavioural avoidance of crowded places, or physical anxiety symptoms in social situations.
Before collecting data , it’s important to consider how you will operationalise the variables that you want to measure.
Reliability and validity are both about how well a method measures something:
- Reliability refers to the consistency of a measure (whether the results can be reproduced under the same conditions).
- Validity refers to the accuracy of a measure (whether the results really do represent what they are supposed to measure).
If you are doing experimental research , you also have to consider the internal and external validity of your experiment.
Hypothesis testing is a formal procedure for investigating our ideas about the world using statistics. It is used by scientists to test specific predictions, called hypotheses , by calculating how likely it is that a pattern or relationship between variables could have arisen by chance.
Cite this Scribbr article
If you want to cite this source, you can copy and paste the citation or click the ‘Cite this Scribbr article’ button to automatically add the citation to our free Reference Generator.
Bhandari, P. (2022, October 10). What Is Quantitative Research? | Definition & Methods. Scribbr. Retrieved 9 September 2024, from https://www.scribbr.co.uk/research-methods/introduction-to-quantitative-research/
Is this article helpful?
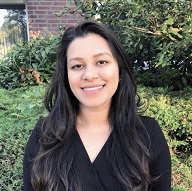
Pritha Bhandari
- Skip to main content
- Skip to primary sidebar
- Skip to footer
- QuestionPro

- Solutions Industries Gaming Automotive Sports and events Education Government Travel & Hospitality Financial Services Healthcare Cannabis Technology Use Case AskWhy Communities Audience Contactless surveys Mobile LivePolls Member Experience GDPR Positive People Science 360 Feedback Surveys
- Resources Blog eBooks Survey Templates Case Studies Training Help center

Home Market Research
Data Analysis in Research: Types & Methods
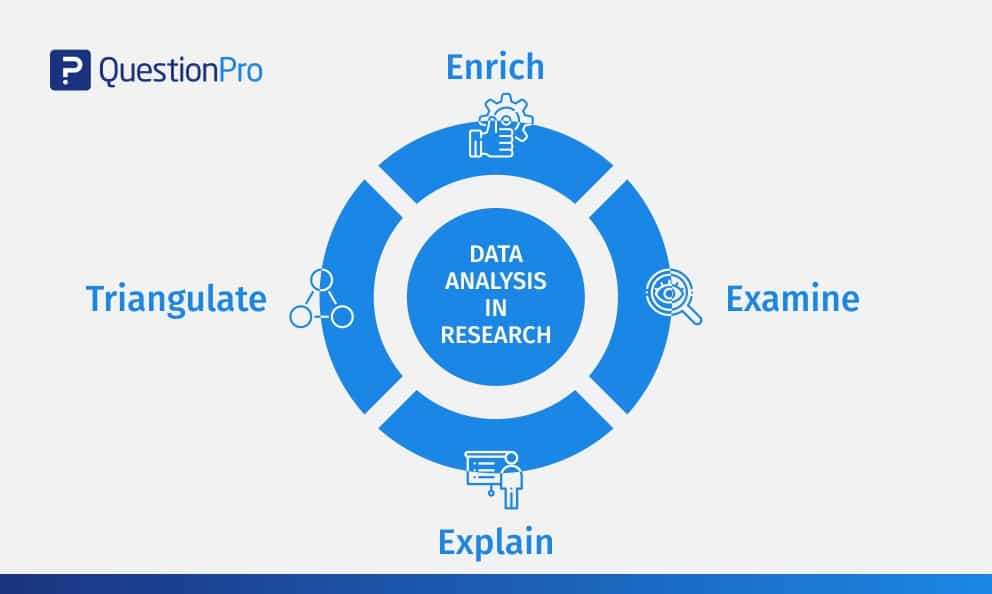
Content Index
Why analyze data in research?
Types of data in research, finding patterns in the qualitative data, methods used for data analysis in qualitative research, preparing data for analysis, methods used for data analysis in quantitative research, considerations in research data analysis, what is data analysis in research.
Definition of research in data analysis: According to LeCompte and Schensul, research data analysis is a process used by researchers to reduce data to a story and interpret it to derive insights. The data analysis process helps reduce a large chunk of data into smaller fragments, which makes sense.
Three essential things occur during the data analysis process — the first is data organization . Summarization and categorization together contribute to becoming the second known method used for data reduction. It helps find patterns and themes in the data for easy identification and linking. The third and last way is data analysis – researchers do it in both top-down and bottom-up fashion.
LEARN ABOUT: Research Process Steps
On the other hand, Marshall and Rossman describe data analysis as a messy, ambiguous, and time-consuming but creative and fascinating process through which a mass of collected data is brought to order, structure and meaning.
We can say that “the data analysis and data interpretation is a process representing the application of deductive and inductive logic to the research and data analysis.”
Researchers rely heavily on data as they have a story to tell or research problems to solve. It starts with a question, and data is nothing but an answer to that question. But, what if there is no question to ask? Well! It is possible to explore data even without a problem – we call it ‘Data Mining’, which often reveals some interesting patterns within the data that are worth exploring.
Irrelevant to the type of data researchers explore, their mission and audiences’ vision guide them to find the patterns to shape the story they want to tell. One of the essential things expected from researchers while analyzing data is to stay open and remain unbiased toward unexpected patterns, expressions, and results. Remember, sometimes, data analysis tells the most unforeseen yet exciting stories that were not expected when initiating data analysis. Therefore, rely on the data you have at hand and enjoy the journey of exploratory research.
Create a Free Account
Every kind of data has a rare quality of describing things after assigning a specific value to it. For analysis, you need to organize these values, processed and presented in a given context, to make it useful. Data can be in different forms; here are the primary data types.
- Qualitative data: When the data presented has words and descriptions, then we call it qualitative data . Although you can observe this data, it is subjective and harder to analyze data in research, especially for comparison. Example: Quality data represents everything describing taste, experience, texture, or an opinion that is considered quality data. This type of data is usually collected through focus groups, personal qualitative interviews , qualitative observation or using open-ended questions in surveys.
- Quantitative data: Any data expressed in numbers of numerical figures are called quantitative data . This type of data can be distinguished into categories, grouped, measured, calculated, or ranked. Example: questions such as age, rank, cost, length, weight, scores, etc. everything comes under this type of data. You can present such data in graphical format, charts, or apply statistical analysis methods to this data. The (Outcomes Measurement Systems) OMS questionnaires in surveys are a significant source of collecting numeric data.
- Categorical data: It is data presented in groups. However, an item included in the categorical data cannot belong to more than one group. Example: A person responding to a survey by telling his living style, marital status, smoking habit, or drinking habit comes under the categorical data. A chi-square test is a standard method used to analyze this data.
Learn More : Examples of Qualitative Data in Education
Data analysis in qualitative research
Data analysis and qualitative data research work a little differently from the numerical data as the quality data is made up of words, descriptions, images, objects, and sometimes symbols. Getting insight from such complicated information is a complicated process. Hence it is typically used for exploratory research and data analysis .
Although there are several ways to find patterns in the textual information, a word-based method is the most relied and widely used global technique for research and data analysis. Notably, the data analysis process in qualitative research is manual. Here the researchers usually read the available data and find repetitive or commonly used words.
For example, while studying data collected from African countries to understand the most pressing issues people face, researchers might find “food” and “hunger” are the most commonly used words and will highlight them for further analysis.
LEARN ABOUT: Level of Analysis
The keyword context is another widely used word-based technique. In this method, the researcher tries to understand the concept by analyzing the context in which the participants use a particular keyword.
For example , researchers conducting research and data analysis for studying the concept of ‘diabetes’ amongst respondents might analyze the context of when and how the respondent has used or referred to the word ‘diabetes.’
The scrutiny-based technique is also one of the highly recommended text analysis methods used to identify a quality data pattern. Compare and contrast is the widely used method under this technique to differentiate how a specific text is similar or different from each other.
For example: To find out the “importance of resident doctor in a company,” the collected data is divided into people who think it is necessary to hire a resident doctor and those who think it is unnecessary. Compare and contrast is the best method that can be used to analyze the polls having single-answer questions types .
Metaphors can be used to reduce the data pile and find patterns in it so that it becomes easier to connect data with theory.
Variable Partitioning is another technique used to split variables so that researchers can find more coherent descriptions and explanations from the enormous data.
LEARN ABOUT: Qualitative Research Questions and Questionnaires
There are several techniques to analyze the data in qualitative research, but here are some commonly used methods,
- Content Analysis: It is widely accepted and the most frequently employed technique for data analysis in research methodology. It can be used to analyze the documented information from text, images, and sometimes from the physical items. It depends on the research questions to predict when and where to use this method.
- Narrative Analysis: This method is used to analyze content gathered from various sources such as personal interviews, field observation, and surveys . The majority of times, stories, or opinions shared by people are focused on finding answers to the research questions.
- Discourse Analysis: Similar to narrative analysis, discourse analysis is used to analyze the interactions with people. Nevertheless, this particular method considers the social context under which or within which the communication between the researcher and respondent takes place. In addition to that, discourse analysis also focuses on the lifestyle and day-to-day environment while deriving any conclusion.
- Grounded Theory: When you want to explain why a particular phenomenon happened, then using grounded theory for analyzing quality data is the best resort. Grounded theory is applied to study data about the host of similar cases occurring in different settings. When researchers are using this method, they might alter explanations or produce new ones until they arrive at some conclusion.
LEARN ABOUT: 12 Best Tools for Researchers
Data analysis in quantitative research
The first stage in research and data analysis is to make it for the analysis so that the nominal data can be converted into something meaningful. Data preparation consists of the below phases.
Phase I: Data Validation
Data validation is done to understand if the collected data sample is per the pre-set standards, or it is a biased data sample again divided into four different stages
- Fraud: To ensure an actual human being records each response to the survey or the questionnaire
- Screening: To make sure each participant or respondent is selected or chosen in compliance with the research criteria
- Procedure: To ensure ethical standards were maintained while collecting the data sample
- Completeness: To ensure that the respondent has answered all the questions in an online survey. Else, the interviewer had asked all the questions devised in the questionnaire.
Phase II: Data Editing
More often, an extensive research data sample comes loaded with errors. Respondents sometimes fill in some fields incorrectly or sometimes skip them accidentally. Data editing is a process wherein the researchers have to confirm that the provided data is free of such errors. They need to conduct necessary checks and outlier checks to edit the raw edit and make it ready for analysis.
Phase III: Data Coding
Out of all three, this is the most critical phase of data preparation associated with grouping and assigning values to the survey responses . If a survey is completed with a 1000 sample size, the researcher will create an age bracket to distinguish the respondents based on their age. Thus, it becomes easier to analyze small data buckets rather than deal with the massive data pile.
LEARN ABOUT: Steps in Qualitative Research
After the data is prepared for analysis, researchers are open to using different research and data analysis methods to derive meaningful insights. For sure, statistical analysis plans are the most favored to analyze numerical data. In statistical analysis, distinguishing between categorical data and numerical data is essential, as categorical data involves distinct categories or labels, while numerical data consists of measurable quantities. The method is again classified into two groups. First, ‘Descriptive Statistics’ used to describe data. Second, ‘Inferential statistics’ that helps in comparing the data .
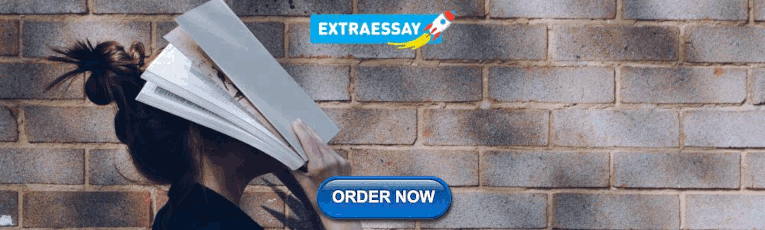
Descriptive statistics
This method is used to describe the basic features of versatile types of data in research. It presents the data in such a meaningful way that pattern in the data starts making sense. Nevertheless, the descriptive analysis does not go beyond making conclusions. The conclusions are again based on the hypothesis researchers have formulated so far. Here are a few major types of descriptive analysis methods.
Measures of Frequency
- Count, Percent, Frequency
- It is used to denote home often a particular event occurs.
- Researchers use it when they want to showcase how often a response is given.
Measures of Central Tendency
- Mean, Median, Mode
- The method is widely used to demonstrate distribution by various points.
- Researchers use this method when they want to showcase the most commonly or averagely indicated response.
Measures of Dispersion or Variation
- Range, Variance, Standard deviation
- Here the field equals high/low points.
- Variance standard deviation = difference between the observed score and mean
- It is used to identify the spread of scores by stating intervals.
- Researchers use this method to showcase data spread out. It helps them identify the depth until which the data is spread out that it directly affects the mean.
Measures of Position
- Percentile ranks, Quartile ranks
- It relies on standardized scores helping researchers to identify the relationship between different scores.
- It is often used when researchers want to compare scores with the average count.
For quantitative research use of descriptive analysis often give absolute numbers, but the in-depth analysis is never sufficient to demonstrate the rationale behind those numbers. Nevertheless, it is necessary to think of the best method for research and data analysis suiting your survey questionnaire and what story researchers want to tell. For example, the mean is the best way to demonstrate the students’ average scores in schools. It is better to rely on the descriptive statistics when the researchers intend to keep the research or outcome limited to the provided sample without generalizing it. For example, when you want to compare average voting done in two different cities, differential statistics are enough.
Descriptive analysis is also called a ‘univariate analysis’ since it is commonly used to analyze a single variable.
Inferential statistics
Inferential statistics are used to make predictions about a larger population after research and data analysis of the representing population’s collected sample. For example, you can ask some odd 100 audiences at a movie theater if they like the movie they are watching. Researchers then use inferential statistics on the collected sample to reason that about 80-90% of people like the movie.
Here are two significant areas of inferential statistics.
- Estimating parameters: It takes statistics from the sample research data and demonstrates something about the population parameter.
- Hypothesis test: I t’s about sampling research data to answer the survey research questions. For example, researchers might be interested to understand if the new shade of lipstick recently launched is good or not, or if the multivitamin capsules help children to perform better at games.
These are sophisticated analysis methods used to showcase the relationship between different variables instead of describing a single variable. It is often used when researchers want something beyond absolute numbers to understand the relationship between variables.
Here are some of the commonly used methods for data analysis in research.
- Correlation: When researchers are not conducting experimental research or quasi-experimental research wherein the researchers are interested to understand the relationship between two or more variables, they opt for correlational research methods.
- Cross-tabulation: Also called contingency tables, cross-tabulation is used to analyze the relationship between multiple variables. Suppose provided data has age and gender categories presented in rows and columns. A two-dimensional cross-tabulation helps for seamless data analysis and research by showing the number of males and females in each age category.
- Regression analysis: For understanding the strong relationship between two variables, researchers do not look beyond the primary and commonly used regression analysis method, which is also a type of predictive analysis used. In this method, you have an essential factor called the dependent variable. You also have multiple independent variables in regression analysis. You undertake efforts to find out the impact of independent variables on the dependent variable. The values of both independent and dependent variables are assumed as being ascertained in an error-free random manner.
- Frequency tables: The statistical procedure is used for testing the degree to which two or more vary or differ in an experiment. A considerable degree of variation means research findings were significant. In many contexts, ANOVA testing and variance analysis are similar.
- Analysis of variance: The statistical procedure is used for testing the degree to which two or more vary or differ in an experiment. A considerable degree of variation means research findings were significant. In many contexts, ANOVA testing and variance analysis are similar.
- Researchers must have the necessary research skills to analyze and manipulation the data , Getting trained to demonstrate a high standard of research practice. Ideally, researchers must possess more than a basic understanding of the rationale of selecting one statistical method over the other to obtain better data insights.
- Usually, research and data analytics projects differ by scientific discipline; therefore, getting statistical advice at the beginning of analysis helps design a survey questionnaire, select data collection methods , and choose samples.
LEARN ABOUT: Best Data Collection Tools
- The primary aim of data research and analysis is to derive ultimate insights that are unbiased. Any mistake in or keeping a biased mind to collect data, selecting an analysis method, or choosing audience sample il to draw a biased inference.
- Irrelevant to the sophistication used in research data and analysis is enough to rectify the poorly defined objective outcome measurements. It does not matter if the design is at fault or intentions are not clear, but lack of clarity might mislead readers, so avoid the practice.
- The motive behind data analysis in research is to present accurate and reliable data. As far as possible, avoid statistical errors, and find a way to deal with everyday challenges like outliers, missing data, data altering, data mining , or developing graphical representation.
LEARN MORE: Descriptive Research vs Correlational Research The sheer amount of data generated daily is frightening. Especially when data analysis has taken center stage. in 2018. In last year, the total data supply amounted to 2.8 trillion gigabytes. Hence, it is clear that the enterprises willing to survive in the hypercompetitive world must possess an excellent capability to analyze complex research data, derive actionable insights, and adapt to the new market needs.
LEARN ABOUT: Average Order Value
QuestionPro is an online survey platform that empowers organizations in data analysis and research and provides them a medium to collect data by creating appealing surveys.
MORE LIKE THIS
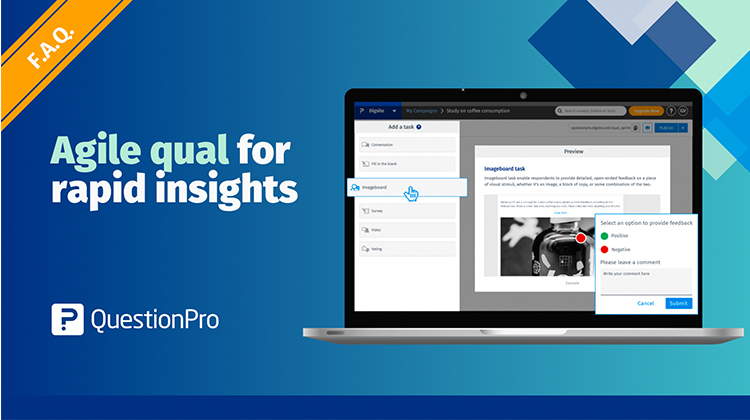
A guide to conducting agile qualitative research for rapid insights with Digsite
Sep 11, 2024
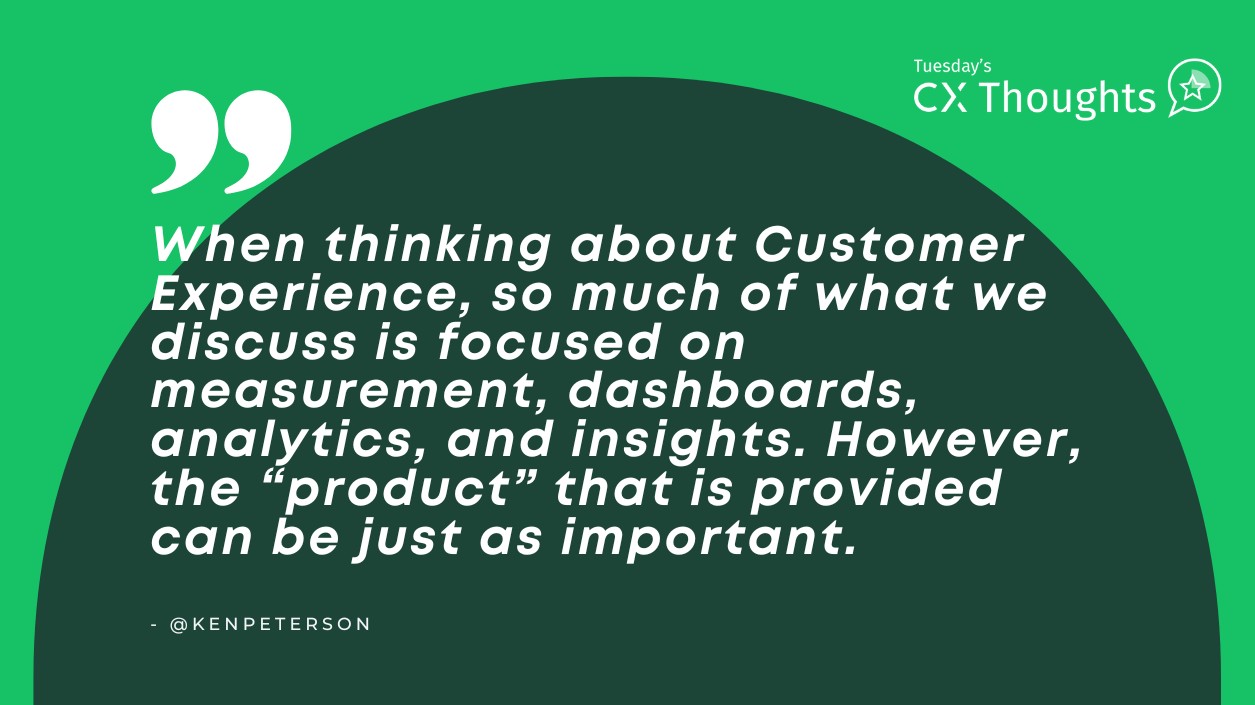
Was The Experience Memorable? — Tuesday CX Thoughts
Sep 10, 2024
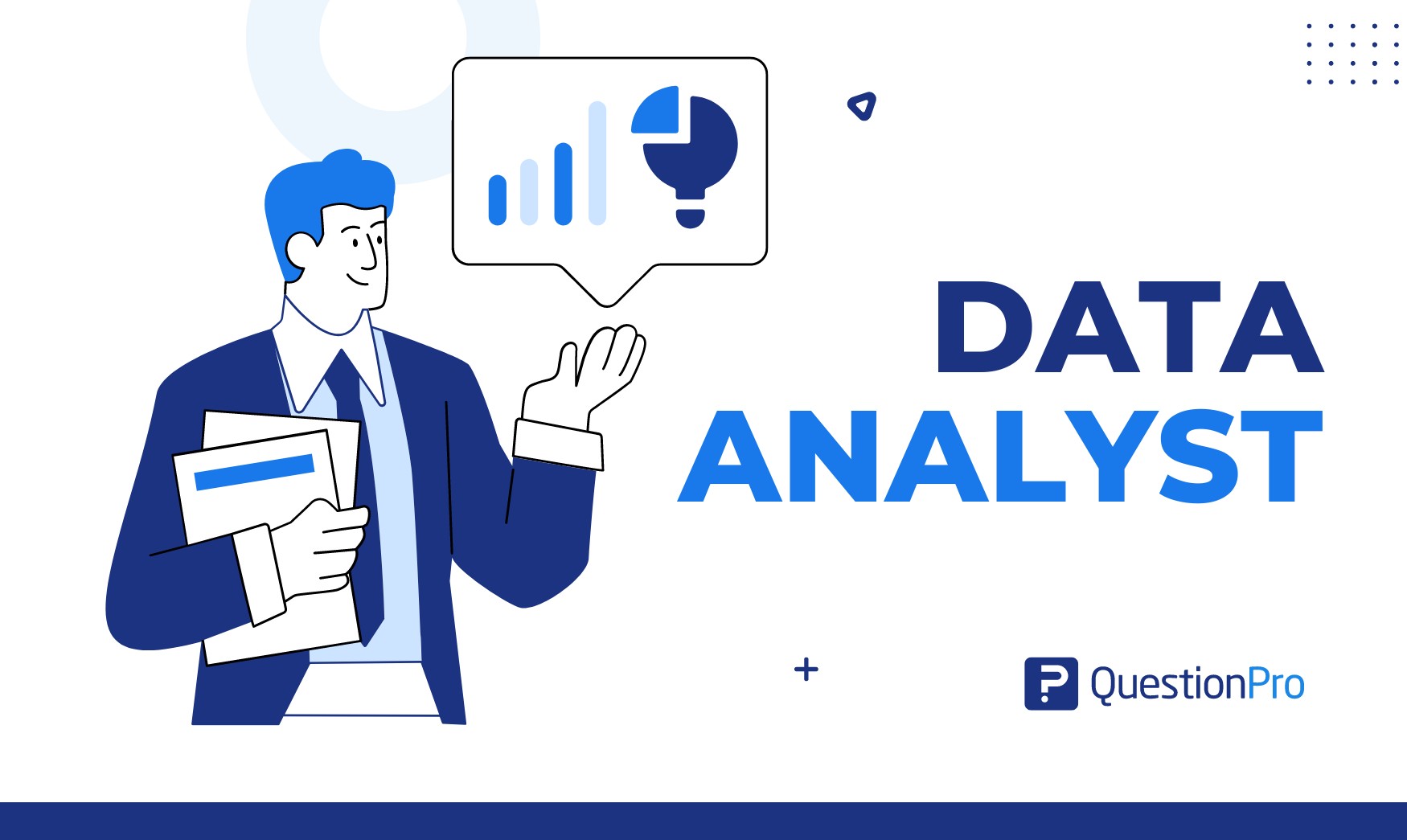
What Does a Data Analyst Do? Skills, Tools & Tips
Sep 9, 2024
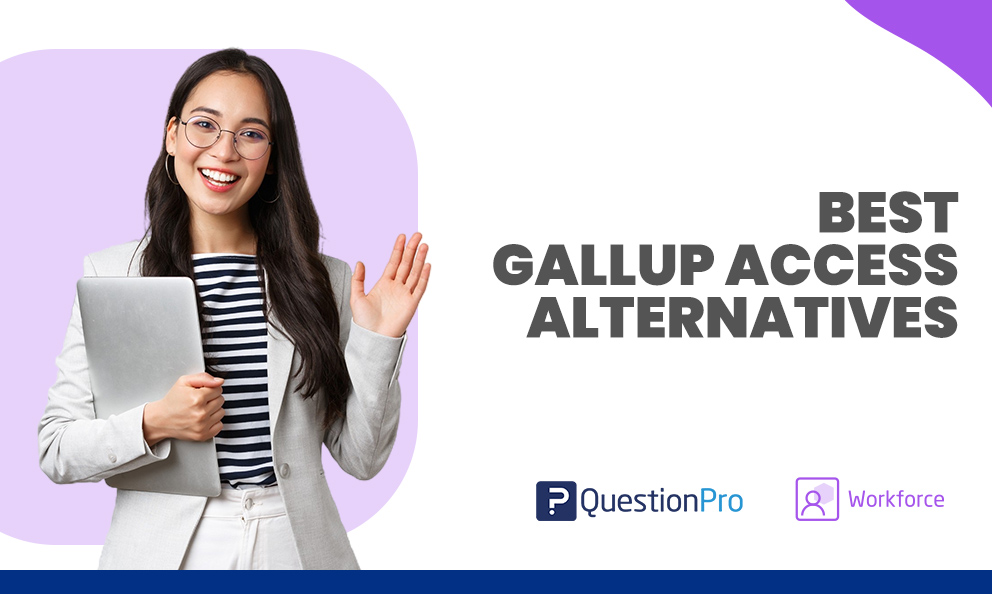
Best Gallup Access Alternatives & Competitors in 2024
Sep 6, 2024
Other categories
- Academic Research
- Artificial Intelligence
- Assessments
- Brand Awareness
- Case Studies
- Communities
- Consumer Insights
- Customer effort score
- Customer Engagement
- Customer Experience
- Customer Loyalty
- Customer Research
- Customer Satisfaction
- Employee Benefits
- Employee Engagement
- Employee Retention
- Friday Five
- General Data Protection Regulation
- Insights Hub
- Life@QuestionPro
- Market Research
- Mobile diaries
- Mobile Surveys
- New Features
- Online Communities
- Question Types
- Questionnaire
- QuestionPro Products
- Release Notes
- Research Tools and Apps
- Revenue at Risk
- Survey Templates
- Training Tips
- Tuesday CX Thoughts (TCXT)
- Uncategorized
- What’s Coming Up
- Workforce Intelligence
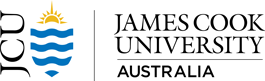
Want to create or adapt books like this? Learn more about how Pressbooks supports open publishing practices.
3.6 Quantitative Data Analysis
Remember that quantitative research explains phenomena by collecting numerical data that are analysed using statistics. 1 Statistics is a scientific method of collecting, processing, analysing, presenting and interpreting data in numerical form. 44 This section discusses how quantitative data is analysed and the choice of test statistics based on the variables (data). First, it is important to understand the different types of variables before delving into the statistical tests.
Types of variables
A variable is an item (data) that can be quantified or measured. There are two main types of variables – numerical variables and categorical variables. 45 Numerical variables describe a measurable quantity and are subdivided into two groups – discrete and continuous data. Discrete variables are finite and are based on a set of whole values or numbers such as 0, 1, 2, 3,… (integer). These data cannot be broken into fractions or decimals. 45 Examples of discrete variables include the number of students in a class and the total number of states in Australia. Continuous variables can assume any value between a certain set of real numbers e.g. height and serum glucose levels. In other words, these are variables that are in between points (101.01 to 101.99 is between 101 and 102) and can be broken down into different parts, fractions and decimals. 45
On the other hand, categorical variables are qualitative and describe characteristics or properties of the data. This type of data may be represented by a name, symbol or number code. 46 There are two types- nominal and ordinal variables. Nominal data are variables having two or more categories without any intrinsic order to the categories. 46 For example, the colour of eyes (blue, brown, and black) and gender (male, female) have no specific order and are nominal categorical variables. Ordinal variables are similar to nominal variables with regard to describing characteristics or properties, but these variables have a clear, logical order or rank in the data. 46 The level of education (primary, secondary and tertiary) is an example of ordinal data.
Now that you understand the different types of variables, identify the variables in the scenario in the Padlet below.
Statistics can be broadly classified into descriptive and inferential statistics. Descriptive statistics explain how different variables in a sample or population relate to one another. 60 Inferential statistics draw conclusions or inferences about a whole population from a random sample of data. 45
Descriptive statistics
This is a summary description of measurements (variables) from a given data set, e.g., a group of study participants. It provides a meaningful interpretation of the data. It has two main measures – central tendency and dispersion measures. 45
The measures of central tendency describe the centre of data and provide a summary of data in the form of mean, median and mode. Mean is the average distribution, the median is the middle value (skewed distribution), and mode is the most frequently occurring variable. 4

- Descriptive statistics for continuous variables
An example is a study conducted among 145 students where their height and weight were obtained. The summary statistics (a measure of central tendency and dispersion) have been presented below in table 3.2.
Table 3.2 Descriptive statistics for continuous variables
Height (cm) | 169.8 | 169.0 | 164.0 | 60.4 | 7.8 | 89.0 | 151.0 | 190.0 |
Weight (kg) | 68.9 | 66.0 | 60.0 | 163.1 | 12.8 | 74.0 | 46.0 | 120.0 |
- Descriptive statistics for categorical variables
Categorical variables are presented using frequencies and percentages or proportions. For example, a hypothetical scenario is a study on smoking history by gender in a population of 4609 people. Below is the summary statistic of the study (Table 3.3).
Table 3.3 Descriptive statistics for categorical variables
Smoker | 636 | 28.7% | 196 | 8.2% |
Non-smoker | 1577 | 71.3% | 2200 | 91.8% |
Normality of data
Before proceeding to inferential statistics, it is important to assess the normality of the data. A normality test evaluates whether or not a sample was selected from a normally distributed population. 47 It is typically used to determine if the data used in the study has a normal distribution. Many statistical techniques, notably parametric tests, such as correlation, regression, t-tests, and ANOVA, are predicated on normal data distribution. 47 There are several methods for assessing whether data are normally distributed. They include graphical or visual tests such as histograms and Q-Q probability plots and analytical tests such as the Shapiro-Wilk test and the Kolmogrorov-Smirnov test. 47 The most useful visual method is visualizing the normality distribution via a histogram, as shown in Figure 3.12. On the other hand, the analytical tests (Shapiro-Wilk test and the Kolmogrorov-Smirnov) determine if the data distribution deviates considerably from the normal distribution by using criteria such as the p-value. 47 If the p-value is < 0.05, the data is not normally distributed. 47 These analytical tests can be conducted using statistical software like SPSS and R. However, when the sample size is > 30, the violation of the normality test is not an issue and the sample is considered to be normally distributed. According to the central limit theorem, in large samples of > 30 or 40, the sampling distribution is normal regardless of the shape of the data. 47, 48 Normally distributed data are also known as parametric data, while non-normally distributed data are known as non-parametric data.
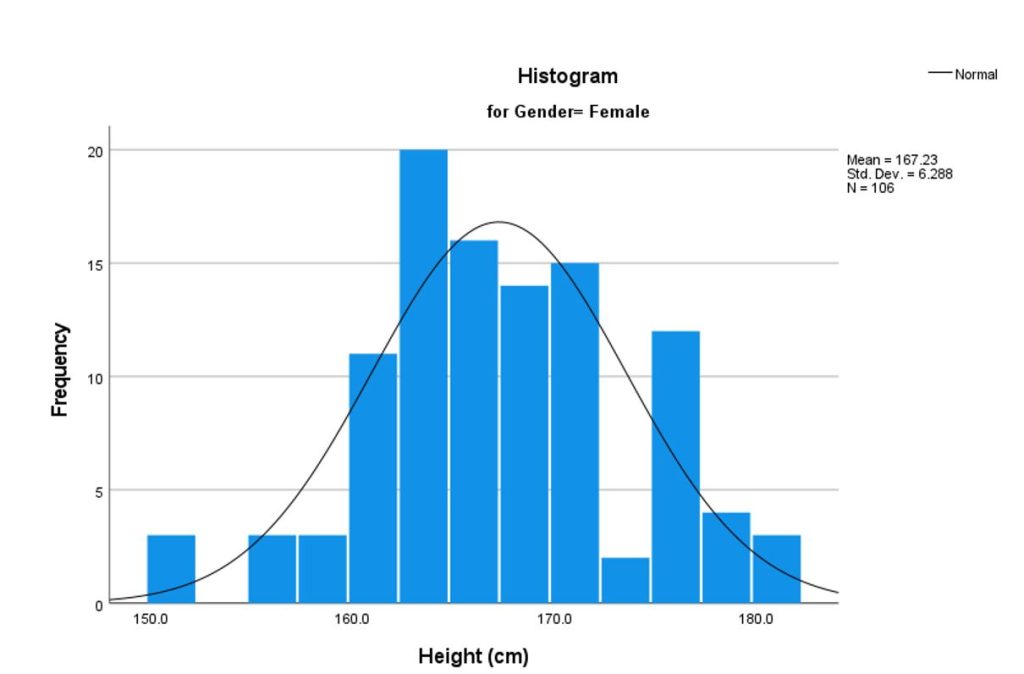
Table 3.4 Tests of normality for height by gender
Male | 0.059 | 39 | 0.200 | 0.983 | 39 | 0.814 | |
Female | 0.082 | 106 | 0.076 | 0.981 | 106 | 0.146 |
Inferential statistics
This statistical analysis involves the analysis of data from a sample to make inferences about the target population. 45 The goal is to test hypotheses. Statistical techniques include parametric and non-parametric tests depending on the normality of the data. 45 Conducting a statistical analysis requires choosing the right test to answer the research question.
Steps in a statistical test
The choice of the statistical test is based on the research question to be answered and the data. There are steps to take before choosing a test and conducting an analysis. 49
- State the research question/aim
- State the null and alternative hypothesis
The null hypothesis states that there is no statistical difference exists between two variables or in a set of given observations. The alternative hypothesis contradicts the null and states that there is a statistical difference between the variables.
- Decide on a suitable statistical test based on the type of variables.
Is the data normally distributed? Are the variables continuous, discrete or categorical data? The identification of the data type will aid the appropriate selection of the right test.
- Specify the level of significance (α -for example, 0.05). The level of significance is the probability of rejecting the null hypothesis when the null is true. The hypothesis is tested by calculating the probability (P value) of observing a difference between the variables, and the value of p ranges from zero to one. The more common cut-off for statistical significance is 0.05. 50
- Conduct the statistical test analysis- calculate the p-value
- p<0.05 leads to rejection of the null hypothesis
- p>0.05 leads to retention of the null hypothesis
- Interpret the results
In the next section, we have provided an overview of the statistical tests. The step-by-step conduct of the test using statistical software is beyond the scope of this book. We have provided the theoretical basis for the test. Other books, like Pallant’s SPSS survival manual: A step-by-step guide to data analysis using IBM SPSS, is a good resource if you wish to learn how to run the different tests. 48
Types of Statistical tests
A distinction is always made based on the data type (categorical or numerical) and if the data is paired or unpaired. Paired data refers to data arising from the same individual at different time points, such as before and after or pre and post-test designs. In contrast, unpaired data are data from separate individuals. Inferential statistics can be grouped into the following categories:
Comparing two categorical variables
- o Two sample groups (one numerical variable and one categorical variable in two groups)
- o Three sample groups (one numerical variable and one categorical variable in three groups)
Comparing two numerical variables
Deciding on the choice of test with two categorical variables involves checking if the data is nominal or ordinal and paired versus unpaired. The figure below (Figure 3.13) shows a decision tree for categorical variables.
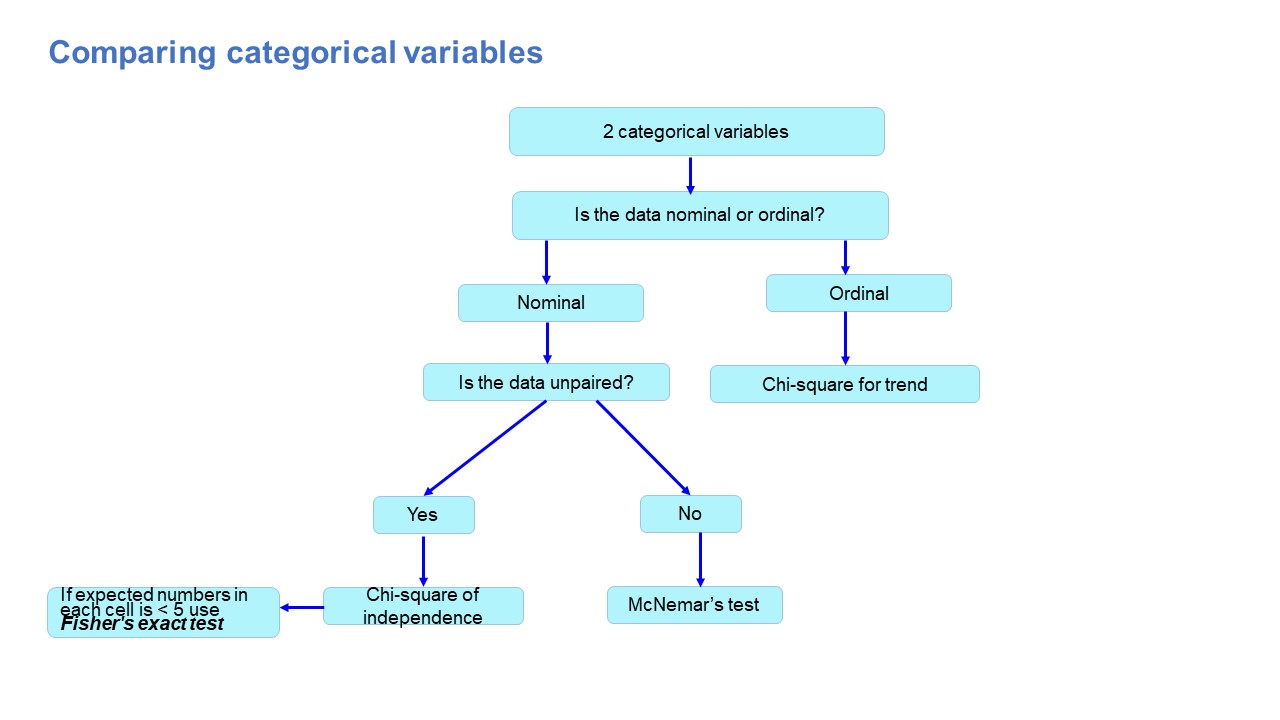
- Chi-square test of independence
The chi-square test of independence compares the distribution of two or more independent data sets. 44 The chi-square value increases when the distributions are found to be increasingly similar, indicating a stronger relationship between them. A value of χ2 = 0 means that there is no relationship between the variables. 44 There are preconditions for the Chi-square test, which include a sample size > 60, and the expected number in each field should not be less than 5. Fisher’s exact test is used if the conditions are not met.
- McNemar’s test
Unlike the Chi-square test, Mcnemar’s test is designed to assess if there is a difference between two related or paired groups (categorical variables). 51
- Chi-square for trend
The chi-square test for trend tests the relationship between one variable that is binary and the other is ordered categorical. 52 The test assesses whether the association between the variables follows a trend. For example, the association between the frequency of mouth rinse (once a week, twice a week and seven days a week) and the presence of dental gingivitis (yes vs no) can be assessed to observe a dose-response effect between mouth rinse usage and dental gingivitis. 52
Tests involving one numerical and one categorical variable
The variables involved in this group of tests are one numerical variable and one categorical variable. These tests have two broad groups – two sample groups and three or more sample groups, as shown in Figures 3.14 and 3.15.
Two sample groups
The parametric two-sample group of tests (independent samples t-test and paired t-test) compare the means of the two samples. On the other hand, the non-parametric tests (Mann-Whitney U test and Wilcoxon Signed Rank test) compare medians of the samples.
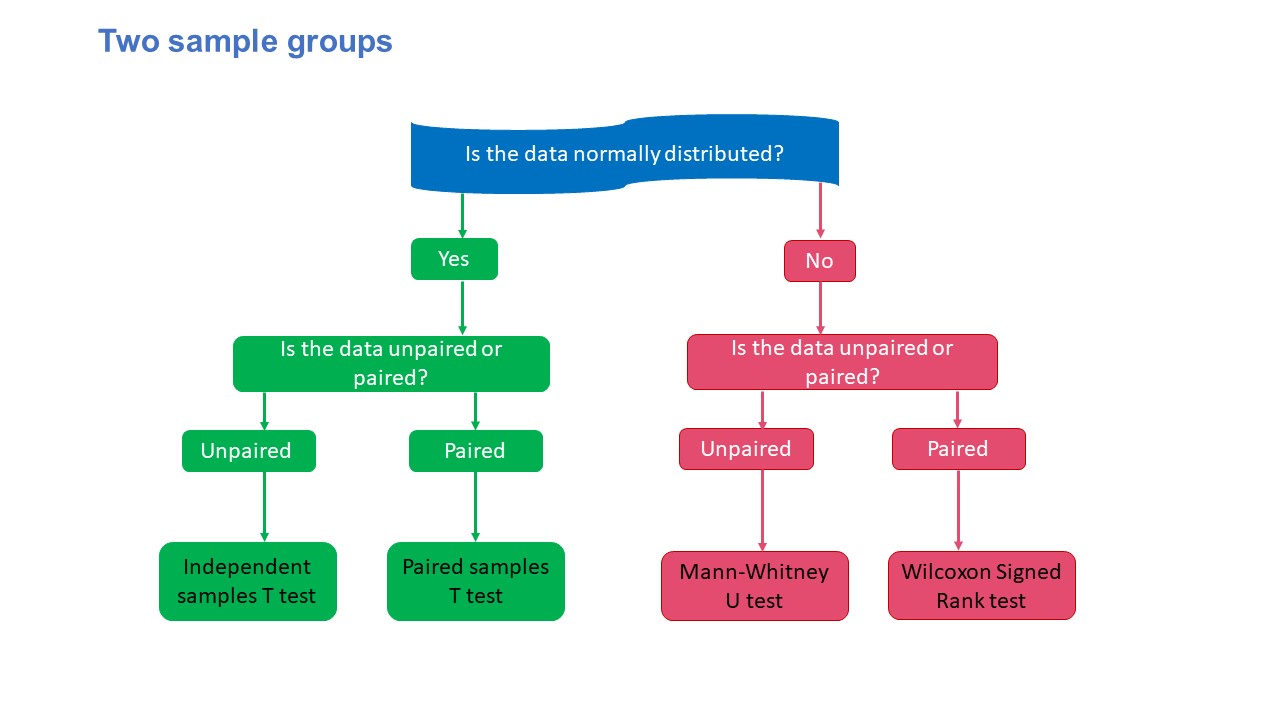
- Parametric: Independent samples T-test and Paired Samples t-test
The independent or unpaired t-test is used when the participants in both groups are independent of one another (those in the first group are distinct from those in the second group) and when the parameters are typically distributed and continuous. 44 On the other hand, the paired t-test is used to test two paired sets of normally distributed continuous data. A paired test involves measuring the same item twice on each subject. 44 For instance, you would wish to compare the differences in each subject’s heart rates before and after an exercise. The tests compare the mean values between the groups.
- Non-parametric: Mann-Whitney U test and Wilcoxon Signed Rank test
The nonparametric equivalents of the paired and independent sample t-tests are the Wilcoxon signed-rank test and the Mann-Whitney U test. 44 These tests examine if the two data sets’ medians are equal and whether the sample sets are representative of the same population. 44 They have less power than their parametric counterparts, as is the case with all nonparametric tests but can be applied to data that is not normally distributed or small samples. 44
Three samples group
The t-tests and their non-parametric counterparts cannot be used for comparing three or more groups. Thus, three or more sample groups of the test are used. The parametric three samples group of tests are one-way ANOVA (Analysis of variance) and repeated measures ANOVA. In contrast, the non-parametric tests are the Kruskal-Wallis test and the Friedman test.
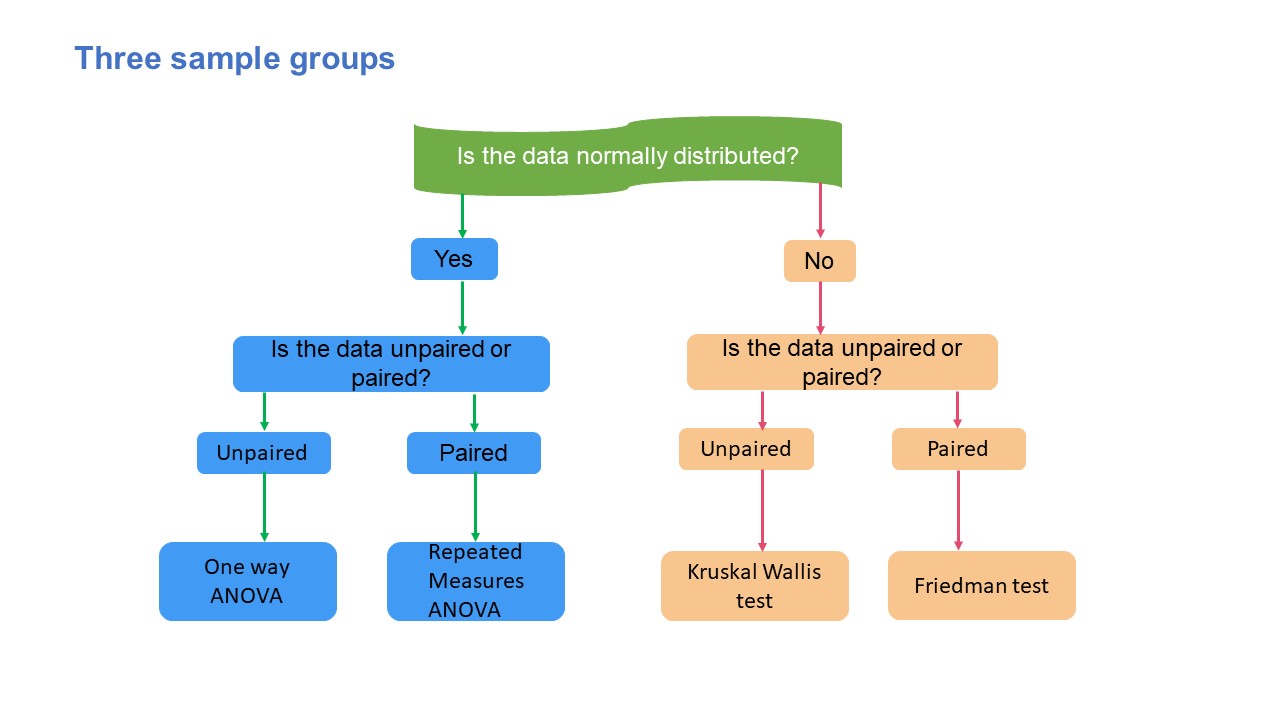
- Parametric: One-way ANOVA and Repeated measures ANOVA
ANOVA is used to determine whether there are appreciable differences between the means of three or more groups. 45 Within-group and between-group variability are the two variances examined in a one-way ANOVA test. The repeated measures ANOVA examines whether the means of three or more groups are identical. 45 When all the variables in a sample are tested under various circumstances or at various times, a repeated measure ANOVA is utilized. 45 The dependent variable is measured repeatedly as the variables are determined from samples at various times. The data don’t conform to the ANOVA premise of independence; thus, using a typical ANOVA in this situation is inappropriate. 45
- Non-parametric: Kuskal Wallis test and Friedman test
The non-parametric test to analyse variance is the Kruskal-Wallis test. It examines if the median values of three or more independent samples differ in any way. 45 The test statistic is produced after the rank sums of the data values, which are ranked in ascending order. On the other hand, the Friedman test is the non-parametric test for comparing the differences between related samples. When the same parameter is assessed repeatedly or under different conditions on the same participants, the Friedman test can be used as an alternative to repeated measures ANOVA. 45
Pearson’s correlation and regression tests are used to compare two numerical variables.
- Pearson’s Correlation and Regression
Pearson’s correlation (r) indicates a relationship between two numerical variables assuming that the relationship is linear. 53 This implies that for every unit rise or reduction in one variable, the other increases or decreases by a constant amount. The values of the correlation coefficient vary from -1 to + 1. Negative correlation coefficient values suggest a rise in one variable will lead to a fall in the other variable and vice versa. 53 Positive correlation coefficient values indicate a propensity for one variable to rise or decrease in tandem with another. Pearson’s correlation also quantifies the strength of the relationship between the two variables. Correlation coefficient values close to zero suggest a weak linear relationship between two variables, whereas those close to -1 or +1 indicate a robust linear relationship between two variables. 53 It is important to note that correlation does not imply causation. The Spearman rank correlation coefficient test (rs) is the nonparametric equivalent of the Pearson coefficient. It is useful when the conditions for calculating a meaningful r value cannot be satisfied and numerical data is being analysed. 44
Regression measures the connection between two correlated variables. The variables are usually labelled as dependent or independent. An independent variable is a factor that influences a dependent variable (which can also be called an outcome). 54 Regression analyses describe, estimate, predict and control the effect of one or more independent variables while investigating the relationship between the independent and dependent variables. 54 There are three common types of regression analyses – linear, logistic and multiple regression. 54
- Linear regression examines the relationship between one continuous dependent and one continuous independent variable. For example, the effect of age on shoe size can be analysed using linear regression. 54
- Logistic regression estimates an event’s likelihood with binary outcomes (present or absent). It involves one categorical dependent variable and two or more continuous or categorical predictor (independent) variables. 54
- Multiple regression is an extension of simple linear regression and investigates one continuous dependent and two or more continuous independent variables. 54
An Introduction to Research Methods for Undergraduate Health Profession Students Copyright © 2023 by Faith Alele and Bunmi Malau-Aduli is licensed under a Creative Commons Attribution-NonCommercial 4.0 International License , except where otherwise noted.

An official website of the United States government
The .gov means it’s official. Federal government websites often end in .gov or .mil. Before sharing sensitive information, make sure you’re on a federal government site.
The site is secure. The https:// ensures that you are connecting to the official website and that any information you provide is encrypted and transmitted securely.
- Publications
- Account settings
Preview improvements coming to the PMC website in October 2024. Learn More or Try it out now .
- Advanced Search
- Journal List
- HCA Healthc J Med
- v.1(2); 2020
- PMC10324782

Introduction to Research Statistical Analysis: An Overview of the Basics
Christian vandever.
1 HCA Healthcare Graduate Medical Education
Description
This article covers many statistical ideas essential to research statistical analysis. Sample size is explained through the concepts of statistical significance level and power. Variable types and definitions are included to clarify necessities for how the analysis will be interpreted. Categorical and quantitative variable types are defined, as well as response and predictor variables. Statistical tests described include t-tests, ANOVA and chi-square tests. Multiple regression is also explored for both logistic and linear regression. Finally, the most common statistics produced by these methods are explored.
Introduction
Statistical analysis is necessary for any research project seeking to make quantitative conclusions. The following is a primer for research-based statistical analysis. It is intended to be a high-level overview of appropriate statistical testing, while not diving too deep into any specific methodology. Some of the information is more applicable to retrospective projects, where analysis is performed on data that has already been collected, but most of it will be suitable to any type of research. This primer will help the reader understand research results in coordination with a statistician, not to perform the actual analysis. Analysis is commonly performed using statistical programming software such as R, SAS or SPSS. These allow for analysis to be replicated while minimizing the risk for an error. Resources are listed later for those working on analysis without a statistician.
After coming up with a hypothesis for a study, including any variables to be used, one of the first steps is to think about the patient population to apply the question. Results are only relevant to the population that the underlying data represents. Since it is impractical to include everyone with a certain condition, a subset of the population of interest should be taken. This subset should be large enough to have power, which means there is enough data to deliver significant results and accurately reflect the study’s population.
The first statistics of interest are related to significance level and power, alpha and beta. Alpha (α) is the significance level and probability of a type I error, the rejection of the null hypothesis when it is true. The null hypothesis is generally that there is no difference between the groups compared. A type I error is also known as a false positive. An example would be an analysis that finds one medication statistically better than another, when in reality there is no difference in efficacy between the two. Beta (β) is the probability of a type II error, the failure to reject the null hypothesis when it is actually false. A type II error is also known as a false negative. This occurs when the analysis finds there is no difference in two medications when in reality one works better than the other. Power is defined as 1-β and should be calculated prior to running any sort of statistical testing. Ideally, alpha should be as small as possible while power should be as large as possible. Power generally increases with a larger sample size, but so does cost and the effect of any bias in the study design. Additionally, as the sample size gets bigger, the chance for a statistically significant result goes up even though these results can be small differences that do not matter practically. Power calculators include the magnitude of the effect in order to combat the potential for exaggeration and only give significant results that have an actual impact. The calculators take inputs like the mean, effect size and desired power, and output the required minimum sample size for analysis. Effect size is calculated using statistical information on the variables of interest. If that information is not available, most tests have commonly used values for small, medium or large effect sizes.
When the desired patient population is decided, the next step is to define the variables previously chosen to be included. Variables come in different types that determine which statistical methods are appropriate and useful. One way variables can be split is into categorical and quantitative variables. ( Table 1 ) Categorical variables place patients into groups, such as gender, race and smoking status. Quantitative variables measure or count some quantity of interest. Common quantitative variables in research include age and weight. An important note is that there can often be a choice for whether to treat a variable as quantitative or categorical. For example, in a study looking at body mass index (BMI), BMI could be defined as a quantitative variable or as a categorical variable, with each patient’s BMI listed as a category (underweight, normal, overweight, and obese) rather than the discrete value. The decision whether a variable is quantitative or categorical will affect what conclusions can be made when interpreting results from statistical tests. Keep in mind that since quantitative variables are treated on a continuous scale it would be inappropriate to transform a variable like which medication was given into a quantitative variable with values 1, 2 and 3.
Categorical vs. Quantitative Variables
Categorical Variables | Quantitative Variables |
---|---|
Categorize patients into discrete groups | Continuous values that measure a variable |
Patient categories are mutually exclusive | For time based studies, there would be a new variable for each measurement at each time |
Examples: race, smoking status, demographic group | Examples: age, weight, heart rate, white blood cell count |
Both of these types of variables can also be split into response and predictor variables. ( Table 2 ) Predictor variables are explanatory, or independent, variables that help explain changes in a response variable. Conversely, response variables are outcome, or dependent, variables whose changes can be partially explained by the predictor variables.
Response vs. Predictor Variables
Response Variables | Predictor Variables |
---|---|
Outcome variables | Explanatory variables |
Should be the result of the predictor variables | Should help explain changes in the response variables |
One variable per statistical test | Can be multiple variables that may have an impact on the response variable |
Can be categorical or quantitative | Can be categorical or quantitative |
Choosing the correct statistical test depends on the types of variables defined and the question being answered. The appropriate test is determined by the variables being compared. Some common statistical tests include t-tests, ANOVA and chi-square tests.
T-tests compare whether there are differences in a quantitative variable between two values of a categorical variable. For example, a t-test could be useful to compare the length of stay for knee replacement surgery patients between those that took apixaban and those that took rivaroxaban. A t-test could examine whether there is a statistically significant difference in the length of stay between the two groups. The t-test will output a p-value, a number between zero and one, which represents the probability that the two groups could be as different as they are in the data, if they were actually the same. A value closer to zero suggests that the difference, in this case for length of stay, is more statistically significant than a number closer to one. Prior to collecting the data, set a significance level, the previously defined alpha. Alpha is typically set at 0.05, but is commonly reduced in order to limit the chance of a type I error, or false positive. Going back to the example above, if alpha is set at 0.05 and the analysis gives a p-value of 0.039, then a statistically significant difference in length of stay is observed between apixaban and rivaroxaban patients. If the analysis gives a p-value of 0.91, then there was no statistical evidence of a difference in length of stay between the two medications. Other statistical summaries or methods examine how big of a difference that might be. These other summaries are known as post-hoc analysis since they are performed after the original test to provide additional context to the results.
Analysis of variance, or ANOVA, tests can observe mean differences in a quantitative variable between values of a categorical variable, typically with three or more values to distinguish from a t-test. ANOVA could add patients given dabigatran to the previous population and evaluate whether the length of stay was significantly different across the three medications. If the p-value is lower than the designated significance level then the hypothesis that length of stay was the same across the three medications is rejected. Summaries and post-hoc tests also could be performed to look at the differences between length of stay and which individual medications may have observed statistically significant differences in length of stay from the other medications. A chi-square test examines the association between two categorical variables. An example would be to consider whether the rate of having a post-operative bleed is the same across patients provided with apixaban, rivaroxaban and dabigatran. A chi-square test can compute a p-value determining whether the bleeding rates were significantly different or not. Post-hoc tests could then give the bleeding rate for each medication, as well as a breakdown as to which specific medications may have a significantly different bleeding rate from each other.
A slightly more advanced way of examining a question can come through multiple regression. Regression allows more predictor variables to be analyzed and can act as a control when looking at associations between variables. Common control variables are age, sex and any comorbidities likely to affect the outcome variable that are not closely related to the other explanatory variables. Control variables can be especially important in reducing the effect of bias in a retrospective population. Since retrospective data was not built with the research question in mind, it is important to eliminate threats to the validity of the analysis. Testing that controls for confounding variables, such as regression, is often more valuable with retrospective data because it can ease these concerns. The two main types of regression are linear and logistic. Linear regression is used to predict differences in a quantitative, continuous response variable, such as length of stay. Logistic regression predicts differences in a dichotomous, categorical response variable, such as 90-day readmission. So whether the outcome variable is categorical or quantitative, regression can be appropriate. An example for each of these types could be found in two similar cases. For both examples define the predictor variables as age, gender and anticoagulant usage. In the first, use the predictor variables in a linear regression to evaluate their individual effects on length of stay, a quantitative variable. For the second, use the same predictor variables in a logistic regression to evaluate their individual effects on whether the patient had a 90-day readmission, a dichotomous categorical variable. Analysis can compute a p-value for each included predictor variable to determine whether they are significantly associated. The statistical tests in this article generate an associated test statistic which determines the probability the results could be acquired given that there is no association between the compared variables. These results often come with coefficients which can give the degree of the association and the degree to which one variable changes with another. Most tests, including all listed in this article, also have confidence intervals, which give a range for the correlation with a specified level of confidence. Even if these tests do not give statistically significant results, the results are still important. Not reporting statistically insignificant findings creates a bias in research. Ideas can be repeated enough times that eventually statistically significant results are reached, even though there is no true significance. In some cases with very large sample sizes, p-values will almost always be significant. In this case the effect size is critical as even the smallest, meaningless differences can be found to be statistically significant.
These variables and tests are just some things to keep in mind before, during and after the analysis process in order to make sure that the statistical reports are supporting the questions being answered. The patient population, types of variables and statistical tests are all important things to consider in the process of statistical analysis. Any results are only as useful as the process used to obtain them. This primer can be used as a reference to help ensure appropriate statistical analysis.
Alpha (α) | the significance level and probability of a type I error, the probability of a false positive |
Analysis of variance/ANOVA | test observing mean differences in a quantitative variable between values of a categorical variable, typically with three or more values to distinguish from a t-test |
Beta (β) | the probability of a type II error, the probability of a false negative |
Categorical variable | place patients into groups, such as gender, race or smoking status |
Chi-square test | examines association between two categorical variables |
Confidence interval | a range for the correlation with a specified level of confidence, 95% for example |
Control variables | variables likely to affect the outcome variable that are not closely related to the other explanatory variables |
Hypothesis | the idea being tested by statistical analysis |
Linear regression | regression used to predict differences in a quantitative, continuous response variable, such as length of stay |
Logistic regression | regression used to predict differences in a dichotomous, categorical response variable, such as 90-day readmission |
Multiple regression | regression utilizing more than one predictor variable |
Null hypothesis | the hypothesis that there are no significant differences for the variable(s) being tested |
Patient population | the population the data is collected to represent |
Post-hoc analysis | analysis performed after the original test to provide additional context to the results |
Power | 1-beta, the probability of avoiding a type II error, avoiding a false negative |
Predictor variable | explanatory, or independent, variables that help explain changes in a response variable |
p-value | a value between zero and one, which represents the probability that the null hypothesis is true, usually compared against a significance level to judge statistical significance |
Quantitative variable | variable measuring or counting some quantity of interest |
Response variable | outcome, or dependent, variables whose changes can be partially explained by the predictor variables |
Retrospective study | a study using previously existing data that was not originally collected for the purposes of the study |
Sample size | the number of patients or observations used for the study |
Significance level | alpha, the probability of a type I error, usually compared to a p-value to determine statistical significance |
Statistical analysis | analysis of data using statistical testing to examine a research hypothesis |
Statistical testing | testing used to examine the validity of a hypothesis using statistical calculations |
Statistical significance | determine whether to reject the null hypothesis, whether the p-value is below the threshold of a predetermined significance level |
T-test | test comparing whether there are differences in a quantitative variable between two values of a categorical variable |
Funding Statement
This research was supported (in whole or in part) by HCA Healthcare and/or an HCA Healthcare affiliated entity.
Conflicts of Interest
The author declares he has no conflicts of interest.
Christian Vandever is an employee of HCA Healthcare Graduate Medical Education, an organization affiliated with the journal’s publisher.
This research was supported (in whole or in part) by HCA Healthcare and/or an HCA Healthcare affiliated entity. The views expressed in this publication represent those of the author(s) and do not necessarily represent the official views of HCA Healthcare or any of its affiliated entities.
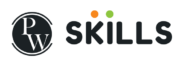
Data Analysis Techniques in Research – Methods, Tools & Examples
Varun Saharawat is a seasoned professional in the fields of SEO and content writing. With a profound knowledge of the intricate aspects of these disciplines, Varun has established himself as a valuable asset in the world of digital marketing and online content creation.
Data analysis techniques in research are essential because they allow researchers to derive meaningful insights from data sets to support their hypotheses or research objectives.
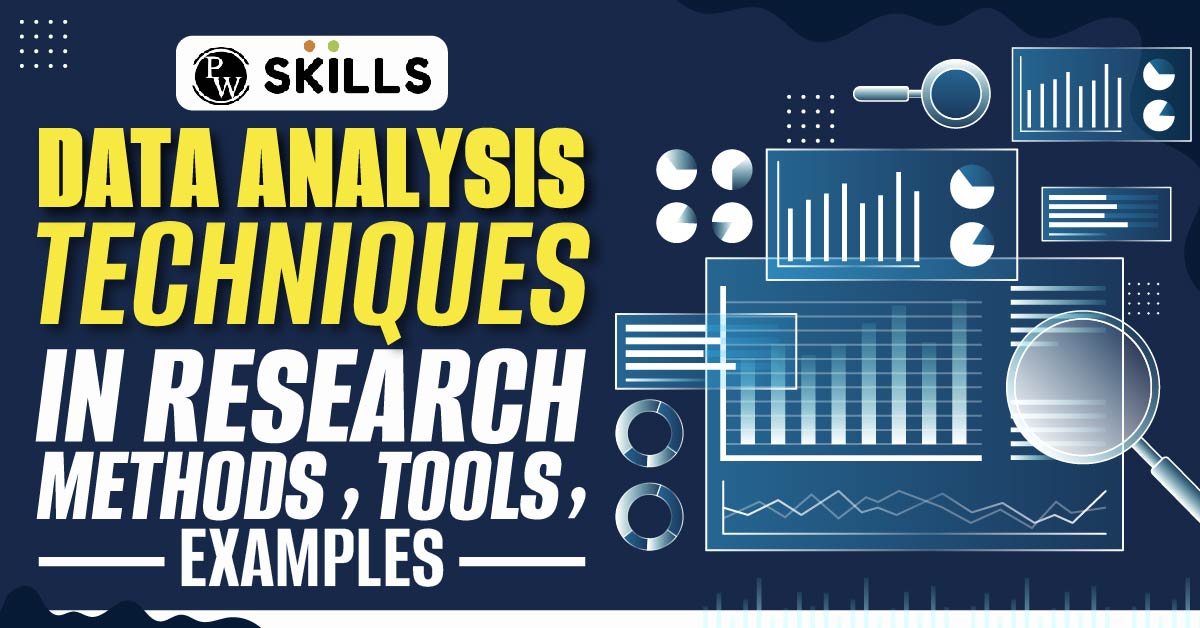
Data Analysis Techniques in Research : While various groups, institutions, and professionals may have diverse approaches to data analysis, a universal definition captures its essence. Data analysis involves refining, transforming, and interpreting raw data to derive actionable insights that guide informed decision-making for businesses.
A straightforward illustration of data analysis emerges when we make everyday decisions, basing our choices on past experiences or predictions of potential outcomes.
If you want to learn more about this topic and acquire valuable skills that will set you apart in today’s data-driven world, we highly recommend enrolling in the Data Analytics Course by Physics Wallah . And as a special offer for our readers, use the coupon code “READER” to get a discount on this course.
Table of Contents
What is Data Analysis?
Data analysis is the systematic process of inspecting, cleaning, transforming, and interpreting data with the objective of discovering valuable insights and drawing meaningful conclusions. This process involves several steps:
- Inspecting : Initial examination of data to understand its structure, quality, and completeness.
- Cleaning : Removing errors, inconsistencies, or irrelevant information to ensure accurate analysis.
- Transforming : Converting data into a format suitable for analysis, such as normalization or aggregation.
- Interpreting : Analyzing the transformed data to identify patterns, trends, and relationships.
Types of Data Analysis Techniques in Research
Data analysis techniques in research are categorized into qualitative and quantitative methods, each with its specific approaches and tools. These techniques are instrumental in extracting meaningful insights, patterns, and relationships from data to support informed decision-making, validate hypotheses, and derive actionable recommendations. Below is an in-depth exploration of the various types of data analysis techniques commonly employed in research:
1) Qualitative Analysis:
Definition: Qualitative analysis focuses on understanding non-numerical data, such as opinions, concepts, or experiences, to derive insights into human behavior, attitudes, and perceptions.
- Content Analysis: Examines textual data, such as interview transcripts, articles, or open-ended survey responses, to identify themes, patterns, or trends.
- Narrative Analysis: Analyzes personal stories or narratives to understand individuals’ experiences, emotions, or perspectives.
- Ethnographic Studies: Involves observing and analyzing cultural practices, behaviors, and norms within specific communities or settings.
2) Quantitative Analysis:
Quantitative analysis emphasizes numerical data and employs statistical methods to explore relationships, patterns, and trends. It encompasses several approaches:
Descriptive Analysis:
- Frequency Distribution: Represents the number of occurrences of distinct values within a dataset.
- Central Tendency: Measures such as mean, median, and mode provide insights into the central values of a dataset.
- Dispersion: Techniques like variance and standard deviation indicate the spread or variability of data.
Diagnostic Analysis:
- Regression Analysis: Assesses the relationship between dependent and independent variables, enabling prediction or understanding causality.
- ANOVA (Analysis of Variance): Examines differences between groups to identify significant variations or effects.
Predictive Analysis:
- Time Series Forecasting: Uses historical data points to predict future trends or outcomes.
- Machine Learning Algorithms: Techniques like decision trees, random forests, and neural networks predict outcomes based on patterns in data.
Prescriptive Analysis:
- Optimization Models: Utilizes linear programming, integer programming, or other optimization techniques to identify the best solutions or strategies.
- Simulation: Mimics real-world scenarios to evaluate various strategies or decisions and determine optimal outcomes.
Specific Techniques:
- Monte Carlo Simulation: Models probabilistic outcomes to assess risk and uncertainty.
- Factor Analysis: Reduces the dimensionality of data by identifying underlying factors or components.
- Cohort Analysis: Studies specific groups or cohorts over time to understand trends, behaviors, or patterns within these groups.
- Cluster Analysis: Classifies objects or individuals into homogeneous groups or clusters based on similarities or attributes.
- Sentiment Analysis: Uses natural language processing and machine learning techniques to determine sentiment, emotions, or opinions from textual data.
Also Read: AI and Predictive Analytics: Examples, Tools, Uses, Ai Vs Predictive Analytics
Data Analysis Techniques in Research Examples
To provide a clearer understanding of how data analysis techniques are applied in research, let’s consider a hypothetical research study focused on evaluating the impact of online learning platforms on students’ academic performance.
Research Objective:
Determine if students using online learning platforms achieve higher academic performance compared to those relying solely on traditional classroom instruction.
Data Collection:
- Quantitative Data: Academic scores (grades) of students using online platforms and those using traditional classroom methods.
- Qualitative Data: Feedback from students regarding their learning experiences, challenges faced, and preferences.
Data Analysis Techniques Applied:
1) Descriptive Analysis:
- Calculate the mean, median, and mode of academic scores for both groups.
- Create frequency distributions to represent the distribution of grades in each group.
2) Diagnostic Analysis:
- Conduct an Analysis of Variance (ANOVA) to determine if there’s a statistically significant difference in academic scores between the two groups.
- Perform Regression Analysis to assess the relationship between the time spent on online platforms and academic performance.
3) Predictive Analysis:
- Utilize Time Series Forecasting to predict future academic performance trends based on historical data.
- Implement Machine Learning algorithms to develop a predictive model that identifies factors contributing to academic success on online platforms.
4) Prescriptive Analysis:
- Apply Optimization Models to identify the optimal combination of online learning resources (e.g., video lectures, interactive quizzes) that maximize academic performance.
- Use Simulation Techniques to evaluate different scenarios, such as varying student engagement levels with online resources, to determine the most effective strategies for improving learning outcomes.
5) Specific Techniques:
- Conduct Factor Analysis on qualitative feedback to identify common themes or factors influencing students’ perceptions and experiences with online learning.
- Perform Cluster Analysis to segment students based on their engagement levels, preferences, or academic outcomes, enabling targeted interventions or personalized learning strategies.
- Apply Sentiment Analysis on textual feedback to categorize students’ sentiments as positive, negative, or neutral regarding online learning experiences.
By applying a combination of qualitative and quantitative data analysis techniques, this research example aims to provide comprehensive insights into the effectiveness of online learning platforms.
Also Read: Learning Path to Become a Data Analyst in 2024
Data Analysis Techniques in Quantitative Research
Quantitative research involves collecting numerical data to examine relationships, test hypotheses, and make predictions. Various data analysis techniques are employed to interpret and draw conclusions from quantitative data. Here are some key data analysis techniques commonly used in quantitative research:
1) Descriptive Statistics:
- Description: Descriptive statistics are used to summarize and describe the main aspects of a dataset, such as central tendency (mean, median, mode), variability (range, variance, standard deviation), and distribution (skewness, kurtosis).
- Applications: Summarizing data, identifying patterns, and providing initial insights into the dataset.
2) Inferential Statistics:
- Description: Inferential statistics involve making predictions or inferences about a population based on a sample of data. This technique includes hypothesis testing, confidence intervals, t-tests, chi-square tests, analysis of variance (ANOVA), regression analysis, and correlation analysis.
- Applications: Testing hypotheses, making predictions, and generalizing findings from a sample to a larger population.
3) Regression Analysis:
- Description: Regression analysis is a statistical technique used to model and examine the relationship between a dependent variable and one or more independent variables. Linear regression, multiple regression, logistic regression, and nonlinear regression are common types of regression analysis .
- Applications: Predicting outcomes, identifying relationships between variables, and understanding the impact of independent variables on the dependent variable.
4) Correlation Analysis:
- Description: Correlation analysis is used to measure and assess the strength and direction of the relationship between two or more variables. The Pearson correlation coefficient, Spearman rank correlation coefficient, and Kendall’s tau are commonly used measures of correlation.
- Applications: Identifying associations between variables and assessing the degree and nature of the relationship.
5) Factor Analysis:
- Description: Factor analysis is a multivariate statistical technique used to identify and analyze underlying relationships or factors among a set of observed variables. It helps in reducing the dimensionality of data and identifying latent variables or constructs.
- Applications: Identifying underlying factors or constructs, simplifying data structures, and understanding the underlying relationships among variables.
6) Time Series Analysis:
- Description: Time series analysis involves analyzing data collected or recorded over a specific period at regular intervals to identify patterns, trends, and seasonality. Techniques such as moving averages, exponential smoothing, autoregressive integrated moving average (ARIMA), and Fourier analysis are used.
- Applications: Forecasting future trends, analyzing seasonal patterns, and understanding time-dependent relationships in data.
7) ANOVA (Analysis of Variance):
- Description: Analysis of variance (ANOVA) is a statistical technique used to analyze and compare the means of two or more groups or treatments to determine if they are statistically different from each other. One-way ANOVA, two-way ANOVA, and MANOVA (Multivariate Analysis of Variance) are common types of ANOVA.
- Applications: Comparing group means, testing hypotheses, and determining the effects of categorical independent variables on a continuous dependent variable.
8) Chi-Square Tests:
- Description: Chi-square tests are non-parametric statistical tests used to assess the association between categorical variables in a contingency table. The Chi-square test of independence, goodness-of-fit test, and test of homogeneity are common chi-square tests.
- Applications: Testing relationships between categorical variables, assessing goodness-of-fit, and evaluating independence.
These quantitative data analysis techniques provide researchers with valuable tools and methods to analyze, interpret, and derive meaningful insights from numerical data. The selection of a specific technique often depends on the research objectives, the nature of the data, and the underlying assumptions of the statistical methods being used.
Also Read: Analysis vs. Analytics: How Are They Different?
Data Analysis Methods
Data analysis methods refer to the techniques and procedures used to analyze, interpret, and draw conclusions from data. These methods are essential for transforming raw data into meaningful insights, facilitating decision-making processes, and driving strategies across various fields. Here are some common data analysis methods:
- Description: Descriptive statistics summarize and organize data to provide a clear and concise overview of the dataset. Measures such as mean, median, mode, range, variance, and standard deviation are commonly used.
- Description: Inferential statistics involve making predictions or inferences about a population based on a sample of data. Techniques such as hypothesis testing, confidence intervals, and regression analysis are used.
3) Exploratory Data Analysis (EDA):
- Description: EDA techniques involve visually exploring and analyzing data to discover patterns, relationships, anomalies, and insights. Methods such as scatter plots, histograms, box plots, and correlation matrices are utilized.
- Applications: Identifying trends, patterns, outliers, and relationships within the dataset.
4) Predictive Analytics:
- Description: Predictive analytics use statistical algorithms and machine learning techniques to analyze historical data and make predictions about future events or outcomes. Techniques such as regression analysis, time series forecasting, and machine learning algorithms (e.g., decision trees, random forests, neural networks) are employed.
- Applications: Forecasting future trends, predicting outcomes, and identifying potential risks or opportunities.
5) Prescriptive Analytics:
- Description: Prescriptive analytics involve analyzing data to recommend actions or strategies that optimize specific objectives or outcomes. Optimization techniques, simulation models, and decision-making algorithms are utilized.
- Applications: Recommending optimal strategies, decision-making support, and resource allocation.
6) Qualitative Data Analysis:
- Description: Qualitative data analysis involves analyzing non-numerical data, such as text, images, videos, or audio, to identify themes, patterns, and insights. Methods such as content analysis, thematic analysis, and narrative analysis are used.
- Applications: Understanding human behavior, attitudes, perceptions, and experiences.
7) Big Data Analytics:
- Description: Big data analytics methods are designed to analyze large volumes of structured and unstructured data to extract valuable insights. Technologies such as Hadoop, Spark, and NoSQL databases are used to process and analyze big data.
- Applications: Analyzing large datasets, identifying trends, patterns, and insights from big data sources.
8) Text Analytics:
- Description: Text analytics methods involve analyzing textual data, such as customer reviews, social media posts, emails, and documents, to extract meaningful information and insights. Techniques such as sentiment analysis, text mining, and natural language processing (NLP) are used.
- Applications: Analyzing customer feedback, monitoring brand reputation, and extracting insights from textual data sources.
These data analysis methods are instrumental in transforming data into actionable insights, informing decision-making processes, and driving organizational success across various sectors, including business, healthcare, finance, marketing, and research. The selection of a specific method often depends on the nature of the data, the research objectives, and the analytical requirements of the project or organization.
Also Read: Quantitative Data Analysis: Types, Analysis & Examples
Data Analysis Tools
Data analysis tools are essential instruments that facilitate the process of examining, cleaning, transforming, and modeling data to uncover useful information, make informed decisions, and drive strategies. Here are some prominent data analysis tools widely used across various industries:
1) Microsoft Excel:
- Description: A spreadsheet software that offers basic to advanced data analysis features, including pivot tables, data visualization tools, and statistical functions.
- Applications: Data cleaning, basic statistical analysis, visualization, and reporting.
2) R Programming Language :
- Description: An open-source programming language specifically designed for statistical computing and data visualization.
- Applications: Advanced statistical analysis, data manipulation, visualization, and machine learning.
3) Python (with Libraries like Pandas, NumPy, Matplotlib, and Seaborn):
- Description: A versatile programming language with libraries that support data manipulation, analysis, and visualization.
- Applications: Data cleaning, statistical analysis, machine learning, and data visualization.
4) SPSS (Statistical Package for the Social Sciences):
- Description: A comprehensive statistical software suite used for data analysis, data mining, and predictive analytics.
- Applications: Descriptive statistics, hypothesis testing, regression analysis, and advanced analytics.
5) SAS (Statistical Analysis System):
- Description: A software suite used for advanced analytics, multivariate analysis, and predictive modeling.
- Applications: Data management, statistical analysis, predictive modeling, and business intelligence.
6) Tableau:
- Description: A data visualization tool that allows users to create interactive and shareable dashboards and reports.
- Applications: Data visualization , business intelligence , and interactive dashboard creation.
7) Power BI:
- Description: A business analytics tool developed by Microsoft that provides interactive visualizations and business intelligence capabilities.
- Applications: Data visualization, business intelligence, reporting, and dashboard creation.
8) SQL (Structured Query Language) Databases (e.g., MySQL, PostgreSQL, Microsoft SQL Server):
- Description: Database management systems that support data storage, retrieval, and manipulation using SQL queries.
- Applications: Data retrieval, data cleaning, data transformation, and database management.
9) Apache Spark:
- Description: A fast and general-purpose distributed computing system designed for big data processing and analytics.
- Applications: Big data processing, machine learning, data streaming, and real-time analytics.
10) IBM SPSS Modeler:
- Description: A data mining software application used for building predictive models and conducting advanced analytics.
- Applications: Predictive modeling, data mining, statistical analysis, and decision optimization.
These tools serve various purposes and cater to different data analysis needs, from basic statistical analysis and data visualization to advanced analytics, machine learning, and big data processing. The choice of a specific tool often depends on the nature of the data, the complexity of the analysis, and the specific requirements of the project or organization.
Also Read: How to Analyze Survey Data: Methods & Examples
Importance of Data Analysis in Research
The importance of data analysis in research cannot be overstated; it serves as the backbone of any scientific investigation or study. Here are several key reasons why data analysis is crucial in the research process:
- Data analysis helps ensure that the results obtained are valid and reliable. By systematically examining the data, researchers can identify any inconsistencies or anomalies that may affect the credibility of the findings.
- Effective data analysis provides researchers with the necessary information to make informed decisions. By interpreting the collected data, researchers can draw conclusions, make predictions, or formulate recommendations based on evidence rather than intuition or guesswork.
- Data analysis allows researchers to identify patterns, trends, and relationships within the data. This can lead to a deeper understanding of the research topic, enabling researchers to uncover insights that may not be immediately apparent.
- In empirical research, data analysis plays a critical role in testing hypotheses. Researchers collect data to either support or refute their hypotheses, and data analysis provides the tools and techniques to evaluate these hypotheses rigorously.
- Transparent and well-executed data analysis enhances the credibility of research findings. By clearly documenting the data analysis methods and procedures, researchers allow others to replicate the study, thereby contributing to the reproducibility of research findings.
- In fields such as business or healthcare, data analysis helps organizations allocate resources more efficiently. By analyzing data on consumer behavior, market trends, or patient outcomes, organizations can make strategic decisions about resource allocation, budgeting, and planning.
- In public policy and social sciences, data analysis is instrumental in developing and evaluating policies and interventions. By analyzing data on social, economic, or environmental factors, policymakers can assess the effectiveness of existing policies and inform the development of new ones.
- Data analysis allows for continuous improvement in research methods and practices. By analyzing past research projects, identifying areas for improvement, and implementing changes based on data-driven insights, researchers can refine their approaches and enhance the quality of future research endeavors.
However, it is important to remember that mastering these techniques requires practice and continuous learning. That’s why we highly recommend the Data Analytics Course by Physics Wallah . Not only does it cover all the fundamentals of data analysis, but it also provides hands-on experience with various tools such as Excel, Python, and Tableau. Plus, if you use the “ READER ” coupon code at checkout, you can get a special discount on the course.
For Latest Tech Related Information, Join Our Official Free Telegram Group : PW Skills Telegram Group
Data Analysis Techniques in Research FAQs
What are the 5 techniques for data analysis.
The five techniques for data analysis include: Descriptive Analysis Diagnostic Analysis Predictive Analysis Prescriptive Analysis Qualitative Analysis
What are techniques of data analysis in research?
Techniques of data analysis in research encompass both qualitative and quantitative methods. These techniques involve processes like summarizing raw data, investigating causes of events, forecasting future outcomes, offering recommendations based on predictions, and examining non-numerical data to understand concepts or experiences.
What are the 3 methods of data analysis?
The three primary methods of data analysis are: Qualitative Analysis Quantitative Analysis Mixed-Methods Analysis
What are the four types of data analysis techniques?
The four types of data analysis techniques are: Descriptive Analysis Diagnostic Analysis Predictive Analysis Prescriptive Analysis
- Descriptive Analytics: What It Is and Related Terms
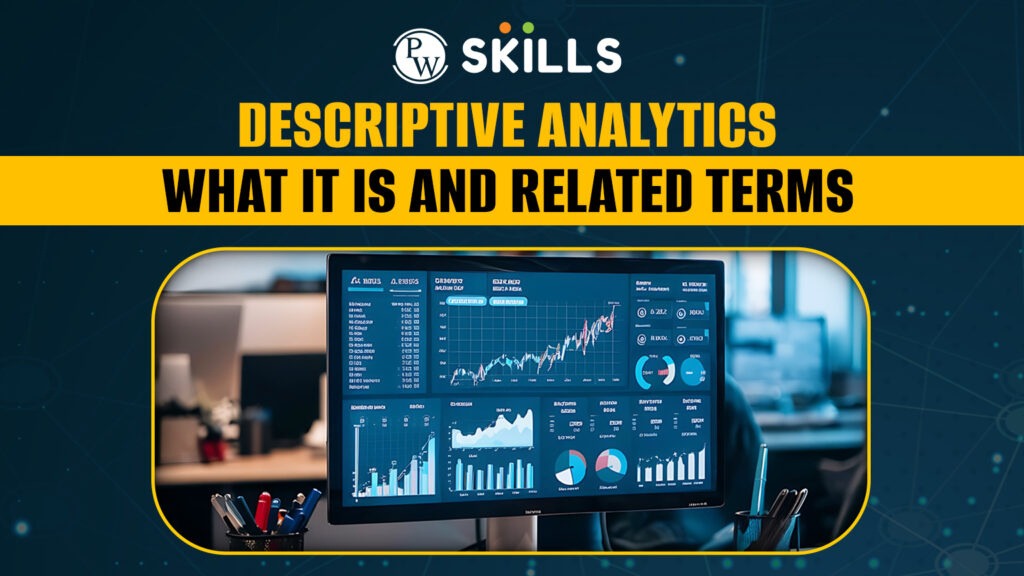
Descriptive analytics is the process of analyzing past data to understand what has happened. Read this article to understand what…
- 10 Best Companies For Data Analysis Internships 2024
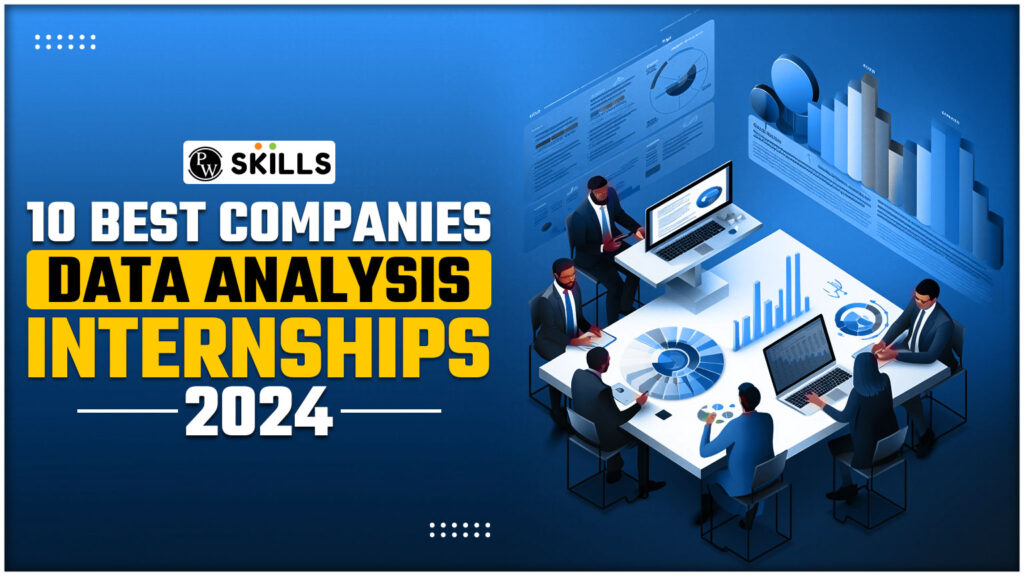
This article will help you provide the top 10 best companies for a Data Analysis Internship which will not only…
- Top Best Big Data Analytics Classes 2024
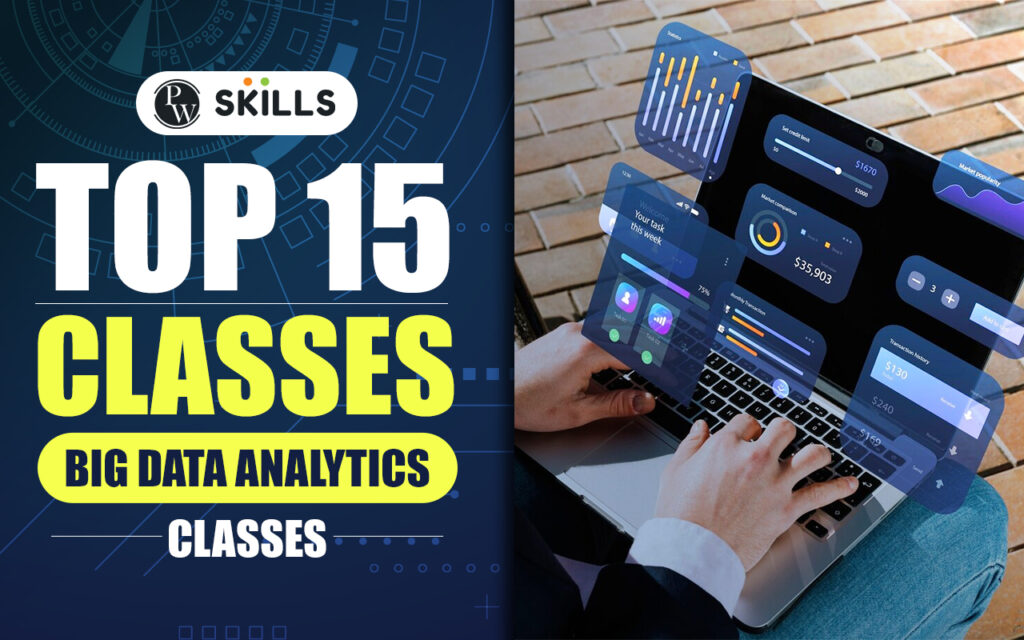
Many websites and institutions provide online remote big data analytics classes to help you learn and also earn certifications for…
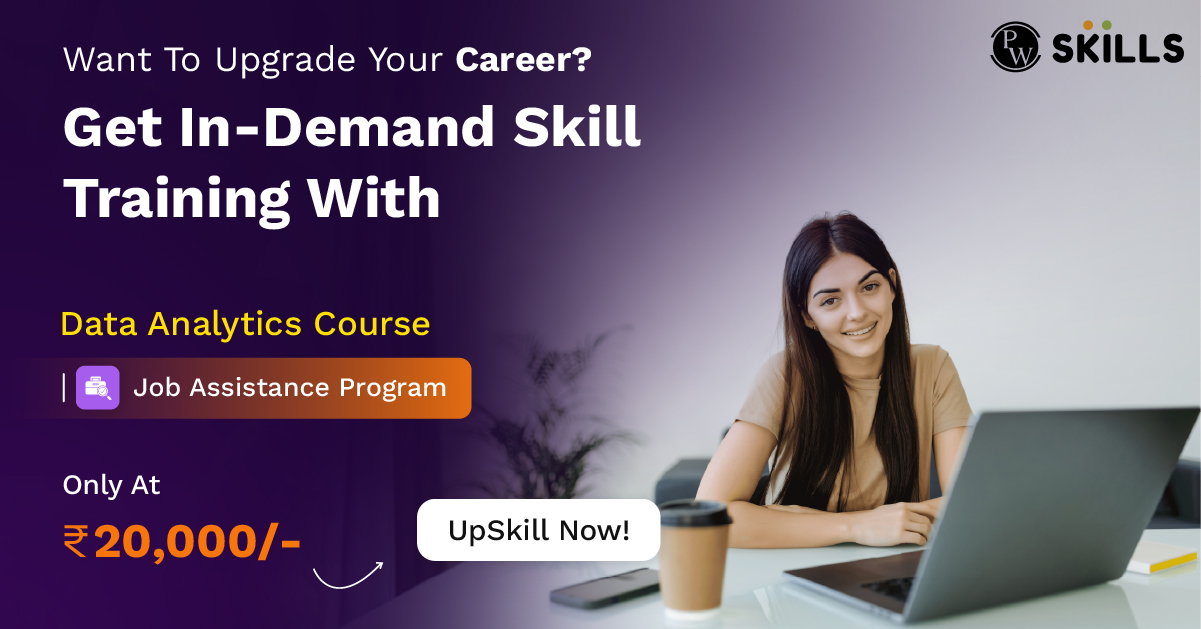
Related Articles
- Data Analyst Roadmap 2024: Responsibilities, Skills Required, Career Path
- The Best Data And Analytics Courses For Beginners
- Best Courses For Data Analytics: Top 10 Courses For Your Career in Trend
- BI & Analytics: What’s The Difference?
- Predictive Analysis: Predicting the Future with Data
- Graph Analytics – What Is it and Why Does It Matter?
- How to Analysis of Survey Data: Methods & Examples
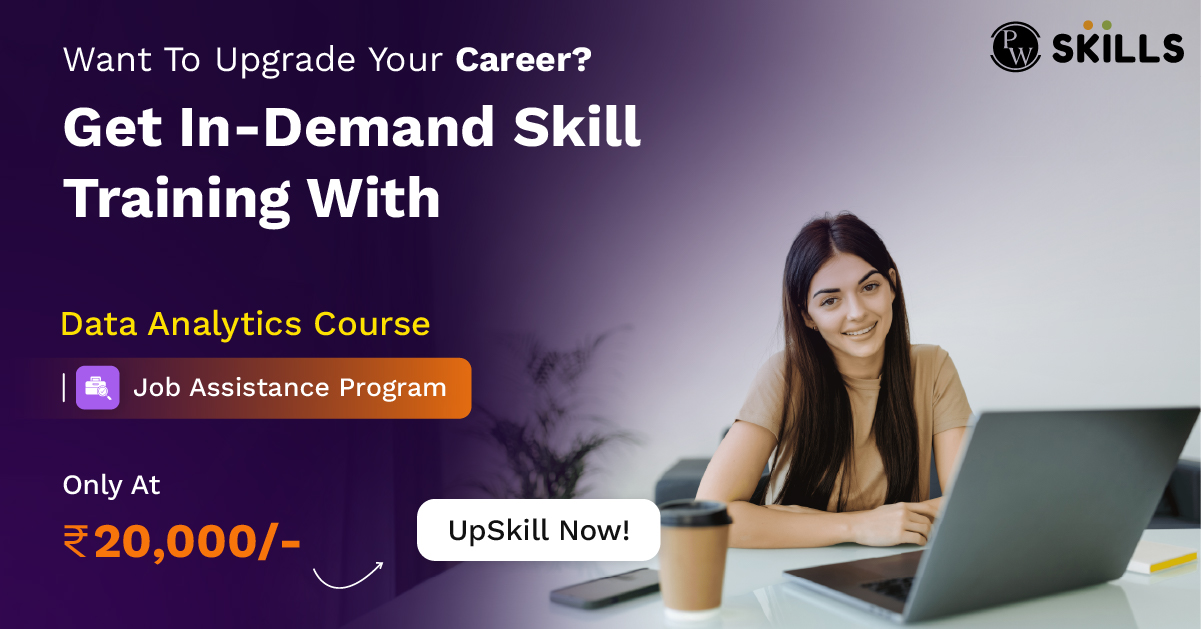
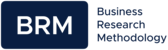
Quantitative Data Analysis
In quantitative data analysis you are expected to turn raw numbers into meaningful data through the application of rational and critical thinking. Quantitative data analysis may include the calculation of frequencies of variables and differences between variables. A quantitative approach is usually associated with finding evidence to either support or reject hypotheses you have formulated at the earlier stages of your research process .
The same figure within data set can be interpreted in many different ways; therefore it is important to apply fair and careful judgement.
For example, questionnaire findings of a research titled “A study into the impacts of informal management-employee communication on the levels of employee motivation: a case study of Agro Bravo Enterprise” may indicate that the majority 52% of respondents assess communication skills of their immediate supervisors as inadequate.
This specific piece of primary data findings needs to be critically analyzed and objectively interpreted through comparing it to other findings within the framework of the same research. For example, organizational culture of Agro Bravo Enterprise, leadership style, the levels of frequency of management-employee communications need to be taken into account during the data analysis.
Moreover, literature review findings conducted at the earlier stages of the research process need to be referred to in order to reflect the viewpoints of other authors regarding the causes of employee dissatisfaction with management communication. Also, secondary data needs to be integrated in data analysis in a logical and unbiased manner.
Let’s take another example. You are writing a dissertation exploring the impacts of foreign direct investment (FDI) on the levels of economic growth in Vietnam using correlation quantitative data analysis method . You have specified FDI and GDP as variables for your research and correlation tests produced correlation coefficient of 0.9.
In this case simply stating that there is a strong positive correlation between FDI and GDP would not suffice; you have to provide explanation about the manners in which the growth on the levels of FDI may contribute to the growth of GDP by referring to the findings of the literature review and applying your own critical and rational reasoning skills.
A set of analytical software can be used to assist with analysis of quantitative data. The following table illustrates the advantages and disadvantages of three popular quantitative data analysis software: Microsoft Excel, Microsoft Access and SPSS.
| Cost effective or Free of Charge Can be sent as e-mail attachments & viewed by most smartphones All in one program Excel files can be secured by a password | Big Excel files may run slowly Numbers of rows and columns are limited Advanced analysis functions are time consuming to be learned by beginners Virus vulnerability through macros
|
One of the cheapest amongst premium programs Flexible information retrieval Ease of use
| Difficult in dealing with large database Low level of interactivity Remote use requires installation of the same version of Microsoft Access | |
Broad coverage of formulas and statistical routines Data files can be imported through other programs Annually updated to increase sophistication | Expensive cost Limited license duration Confusion among the different versions due to regular update |
Advantages and disadvantages of popular quantitative analytical software
Quantitative data analysis with the application of statistical software consists of the following stages [1] :
- Preparing and checking the data. Input of data into computer.
- Selecting the most appropriate tables and diagrams to use according to your research objectives.
- Selecting the most appropriate statistics to describe your data.
- Selecting the most appropriate statistics to examine relationships and trends in your data.
It is important to note that while the application of various statistical software and programs are invaluable to avoid drawing charts by hand or undertake calculations manually, it is easy to use them incorrectly. In other words, quantitative data analysis is “a field where it is not at all difficult to carry out an analysis which is simply wrong, or inappropriate for your data or purposes. And the negative side of readily available specialist statistical software is that it becomes that much easier to generate elegantly presented rubbish” [2] .
Therefore, it is important for you to seek advice from your dissertation supervisor regarding statistical analyses in general and the choice and application of statistical software in particular.
My e-book, The Ultimate Guide to Writing a Dissertation in Business Studies: a step by step approach contains a detailed, yet simple explanation of quantitative data analysis methods . The e-book explains all stages of the research process starting from the selection of the research area to writing personal reflection. Important elements of dissertations such as research philosophy, research approach, research design, methods of data collection and data analysis are explained in simple words. John Dudovskiy
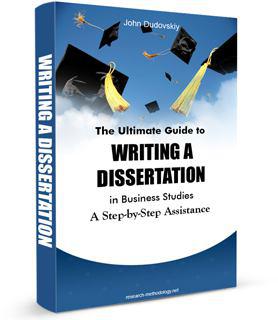
[1] Saunders, M., Lewis, P. & Thornhill, A. (2012) “Research Methods for Business Students” 6th edition, Pearson Education Limited.
[2] Robson, C. (2011) Real World Research: A Resource for Users of Social Research Methods in Applied Settings (3rd edn). Chichester: John Wiley.
Qualitative vs Quantitative Research Methods & Data Analysis
Saul McLeod, PhD
Editor-in-Chief for Simply Psychology
BSc (Hons) Psychology, MRes, PhD, University of Manchester
Saul McLeod, PhD., is a qualified psychology teacher with over 18 years of experience in further and higher education. He has been published in peer-reviewed journals, including the Journal of Clinical Psychology.
Learn about our Editorial Process
Olivia Guy-Evans, MSc
Associate Editor for Simply Psychology
BSc (Hons) Psychology, MSc Psychology of Education
Olivia Guy-Evans is a writer and associate editor for Simply Psychology. She has previously worked in healthcare and educational sectors.
The main difference between quantitative and qualitative research is the type of data they collect and analyze.
Quantitative data is information about quantities, and therefore numbers, and qualitative data is descriptive, and regards phenomenon which can be observed but not measured, such as language.
- Quantitative research collects numerical data and analyzes it using statistical methods. The aim is to produce objective, empirical data that can be measured and expressed numerically. Quantitative research is often used to test hypotheses, identify patterns, and make predictions.
- Qualitative research gathers non-numerical data (words, images, sounds) to explore subjective experiences and attitudes, often via observation and interviews. It aims to produce detailed descriptions and uncover new insights about the studied phenomenon.
On This Page:
What Is Qualitative Research?
Qualitative research is the process of collecting, analyzing, and interpreting non-numerical data, such as language. Qualitative research can be used to understand how an individual subjectively perceives and gives meaning to their social reality.
Qualitative data is non-numerical data, such as text, video, photographs, or audio recordings. This type of data can be collected using diary accounts or in-depth interviews and analyzed using grounded theory or thematic analysis.
Qualitative research is multimethod in focus, involving an interpretive, naturalistic approach to its subject matter. This means that qualitative researchers study things in their natural settings, attempting to make sense of, or interpret, phenomena in terms of the meanings people bring to them. Denzin and Lincoln (1994, p. 2)
Interest in qualitative data came about as the result of the dissatisfaction of some psychologists (e.g., Carl Rogers) with the scientific study of psychologists such as behaviorists (e.g., Skinner ).
Since psychologists study people, the traditional approach to science is not seen as an appropriate way of carrying out research since it fails to capture the totality of human experience and the essence of being human. Exploring participants’ experiences is known as a phenomenological approach (re: Humanism ).
Qualitative research is primarily concerned with meaning, subjectivity, and lived experience. The goal is to understand the quality and texture of people’s experiences, how they make sense of them, and the implications for their lives.
Qualitative research aims to understand the social reality of individuals, groups, and cultures as nearly as possible as participants feel or live it. Thus, people and groups are studied in their natural setting.
Some examples of qualitative research questions are provided, such as what an experience feels like, how people talk about something, how they make sense of an experience, and how events unfold for people.
Research following a qualitative approach is exploratory and seeks to explain ‘how’ and ‘why’ a particular phenomenon, or behavior, operates as it does in a particular context. It can be used to generate hypotheses and theories from the data.
Qualitative Methods
There are different types of qualitative research methods, including diary accounts, in-depth interviews , documents, focus groups , case study research , and ethnography .
The results of qualitative methods provide a deep understanding of how people perceive their social realities and in consequence, how they act within the social world.
The researcher has several methods for collecting empirical materials, ranging from the interview to direct observation, to the analysis of artifacts, documents, and cultural records, to the use of visual materials or personal experience. Denzin and Lincoln (1994, p. 14)
Here are some examples of qualitative data:
Interview transcripts : Verbatim records of what participants said during an interview or focus group. They allow researchers to identify common themes and patterns, and draw conclusions based on the data. Interview transcripts can also be useful in providing direct quotes and examples to support research findings.
Observations : The researcher typically takes detailed notes on what they observe, including any contextual information, nonverbal cues, or other relevant details. The resulting observational data can be analyzed to gain insights into social phenomena, such as human behavior, social interactions, and cultural practices.
Unstructured interviews : generate qualitative data through the use of open questions. This allows the respondent to talk in some depth, choosing their own words. This helps the researcher develop a real sense of a person’s understanding of a situation.
Diaries or journals : Written accounts of personal experiences or reflections.
Notice that qualitative data could be much more than just words or text. Photographs, videos, sound recordings, and so on, can be considered qualitative data. Visual data can be used to understand behaviors, environments, and social interactions.
Qualitative Data Analysis
Qualitative research is endlessly creative and interpretive. The researcher does not just leave the field with mountains of empirical data and then easily write up his or her findings.
Qualitative interpretations are constructed, and various techniques can be used to make sense of the data, such as content analysis, grounded theory (Glaser & Strauss, 1967), thematic analysis (Braun & Clarke, 2006), or discourse analysis .
For example, thematic analysis is a qualitative approach that involves identifying implicit or explicit ideas within the data. Themes will often emerge once the data has been coded .
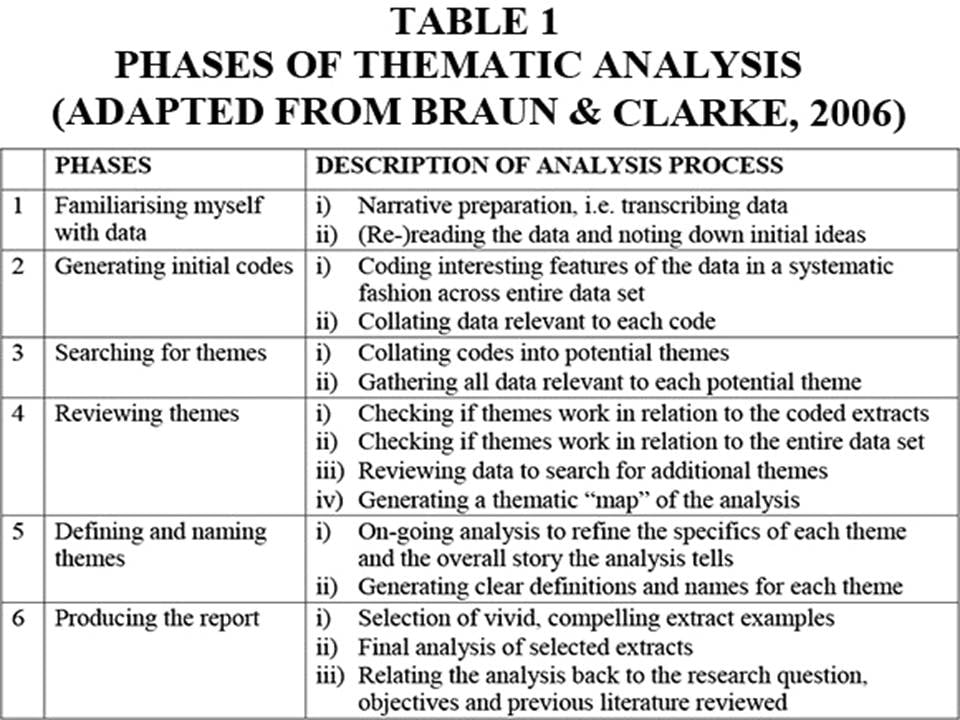
Key Features
- Events can be understood adequately only if they are seen in context. Therefore, a qualitative researcher immerses her/himself in the field, in natural surroundings. The contexts of inquiry are not contrived; they are natural. Nothing is predefined or taken for granted.
- Qualitative researchers want those who are studied to speak for themselves, to provide their perspectives in words and other actions. Therefore, qualitative research is an interactive process in which the persons studied teach the researcher about their lives.
- The qualitative researcher is an integral part of the data; without the active participation of the researcher, no data exists.
- The study’s design evolves during the research and can be adjusted or changed as it progresses. For the qualitative researcher, there is no single reality. It is subjective and exists only in reference to the observer.
- The theory is data-driven and emerges as part of the research process, evolving from the data as they are collected.
Limitations of Qualitative Research
- Because of the time and costs involved, qualitative designs do not generally draw samples from large-scale data sets.
- The problem of adequate validity or reliability is a major criticism. Because of the subjective nature of qualitative data and its origin in single contexts, it is difficult to apply conventional standards of reliability and validity. For example, because of the central role played by the researcher in the generation of data, it is not possible to replicate qualitative studies.
- Also, contexts, situations, events, conditions, and interactions cannot be replicated to any extent, nor can generalizations be made to a wider context than the one studied with confidence.
- The time required for data collection, analysis, and interpretation is lengthy. Analysis of qualitative data is difficult, and expert knowledge of an area is necessary to interpret qualitative data. Great care must be taken when doing so, for example, looking for mental illness symptoms.
Advantages of Qualitative Research
- Because of close researcher involvement, the researcher gains an insider’s view of the field. This allows the researcher to find issues that are often missed (such as subtleties and complexities) by the scientific, more positivistic inquiries.
- Qualitative descriptions can be important in suggesting possible relationships, causes, effects, and dynamic processes.
- Qualitative analysis allows for ambiguities/contradictions in the data, which reflect social reality (Denscombe, 2010).
- Qualitative research uses a descriptive, narrative style; this research might be of particular benefit to the practitioner as she or he could turn to qualitative reports to examine forms of knowledge that might otherwise be unavailable, thereby gaining new insight.
What Is Quantitative Research?
Quantitative research involves the process of objectively collecting and analyzing numerical data to describe, predict, or control variables of interest.
The goals of quantitative research are to test causal relationships between variables , make predictions, and generalize results to wider populations.
Quantitative researchers aim to establish general laws of behavior and phenomenon across different settings/contexts. Research is used to test a theory and ultimately support or reject it.
Quantitative Methods
Experiments typically yield quantitative data, as they are concerned with measuring things. However, other research methods, such as controlled observations and questionnaires , can produce both quantitative information.
For example, a rating scale or closed questions on a questionnaire would generate quantitative data as these produce either numerical data or data that can be put into categories (e.g., “yes,” “no” answers).
Experimental methods limit how research participants react to and express appropriate social behavior.
Findings are, therefore, likely to be context-bound and simply a reflection of the assumptions that the researcher brings to the investigation.
There are numerous examples of quantitative data in psychological research, including mental health. Here are a few examples:
Another example is the Experience in Close Relationships Scale (ECR), a self-report questionnaire widely used to assess adult attachment styles .
The ECR provides quantitative data that can be used to assess attachment styles and predict relationship outcomes.
Neuroimaging data : Neuroimaging techniques, such as MRI and fMRI, provide quantitative data on brain structure and function.
This data can be analyzed to identify brain regions involved in specific mental processes or disorders.
For example, the Beck Depression Inventory (BDI) is a clinician-administered questionnaire widely used to assess the severity of depressive symptoms in individuals.
The BDI consists of 21 questions, each scored on a scale of 0 to 3, with higher scores indicating more severe depressive symptoms.
Quantitative Data Analysis
Statistics help us turn quantitative data into useful information to help with decision-making. We can use statistics to summarize our data, describing patterns, relationships, and connections. Statistics can be descriptive or inferential.
Descriptive statistics help us to summarize our data. In contrast, inferential statistics are used to identify statistically significant differences between groups of data (such as intervention and control groups in a randomized control study).
- Quantitative researchers try to control extraneous variables by conducting their studies in the lab.
- The research aims for objectivity (i.e., without bias) and is separated from the data.
- The design of the study is determined before it begins.
- For the quantitative researcher, the reality is objective, exists separately from the researcher, and can be seen by anyone.
- Research is used to test a theory and ultimately support or reject it.
Limitations of Quantitative Research
- Context: Quantitative experiments do not take place in natural settings. In addition, they do not allow participants to explain their choices or the meaning of the questions they may have for those participants (Carr, 1994).
- Researcher expertise: Poor knowledge of the application of statistical analysis may negatively affect analysis and subsequent interpretation (Black, 1999).
- Variability of data quantity: Large sample sizes are needed for more accurate analysis. Small-scale quantitative studies may be less reliable because of the low quantity of data (Denscombe, 2010). This also affects the ability to generalize study findings to wider populations.
- Confirmation bias: The researcher might miss observing phenomena because of focus on theory or hypothesis testing rather than on the theory of hypothesis generation.
Advantages of Quantitative Research
- Scientific objectivity: Quantitative data can be interpreted with statistical analysis, and since statistics are based on the principles of mathematics, the quantitative approach is viewed as scientifically objective and rational (Carr, 1994; Denscombe, 2010).
- Useful for testing and validating already constructed theories.
- Rapid analysis: Sophisticated software removes much of the need for prolonged data analysis, especially with large volumes of data involved (Antonius, 2003).
- Replication: Quantitative data is based on measured values and can be checked by others because numerical data is less open to ambiguities of interpretation.
- Hypotheses can also be tested because of statistical analysis (Antonius, 2003).
Antonius, R. (2003). Interpreting quantitative data with SPSS . Sage.
Black, T. R. (1999). Doing quantitative research in the social sciences: An integrated approach to research design, measurement and statistics . Sage.
Braun, V. & Clarke, V. (2006). Using thematic analysis in psychology . Qualitative Research in Psychology , 3, 77–101.
Carr, L. T. (1994). The strengths and weaknesses of quantitative and qualitative research : what method for nursing? Journal of advanced nursing, 20(4) , 716-721.
Denscombe, M. (2010). The Good Research Guide: for small-scale social research. McGraw Hill.
Denzin, N., & Lincoln. Y. (1994). Handbook of Qualitative Research. Thousand Oaks, CA, US: Sage Publications Inc.
Glaser, B. G., Strauss, A. L., & Strutzel, E. (1968). The discovery of grounded theory; strategies for qualitative research. Nursing research, 17(4) , 364.
Minichiello, V. (1990). In-Depth Interviewing: Researching People. Longman Cheshire.
Punch, K. (1998). Introduction to Social Research: Quantitative and Qualitative Approaches. London: Sage
Further Information
- Mixed methods research
- Designing qualitative research
- Methods of data collection and analysis
- Introduction to quantitative and qualitative research
- Checklists for improving rigour in qualitative research: a case of the tail wagging the dog?
- Qualitative research in health care: Analysing qualitative data
- Qualitative data analysis: the framework approach
- Using the framework method for the analysis of
- Qualitative data in multi-disciplinary health research
- Content Analysis
- Grounded Theory
- Thematic Analysis

- Harvard Library
- Research Guides
- Faculty of Arts & Sciences Libraries
Library Support for Qualitative Research
- Data Analysis
- Types of Interviews
- Recruiting & Engaging Participants
- Interview Questions
- Conducting Interviews
- Recording & Transcription
QDA Software
Coding and themeing the data, data visualization, testing or generating theories.
- Managing Interview Data
- Finding Extant Interviews
- Past Workshops on Interview Research
- Methodological Resources
- Remote & Virtual Fieldwork
- Data Management & Repositories
- Campus Access
- Free download available for Harvard Faculty of Arts and Sciences (FAS) affiliates
- Desktop access at Lamont Library Media Lab, 3rd floor
- Desktop access at Harvard Kennedy School Library (with HKS ID)
- Remote desktop access for Harvard affiliates from IQSS Computer Labs . Email them at [email protected] and ask for a new lab account and remote desktop access to NVivo.
- Virtual Desktop Infrastructure (VDI) access available to Harvard T.H. Chan School of Public Health affiliates.
Qualitative data analysis methods should flow from, or align with, the methodological paradigm chosen for your study, whether that paradigm is interpretivist, critical, positivist, or participative in nature (or a combination of these). Some established methods include Content Analysis, Critical Analysis, Discourse Analysis, Gestalt Analysis, Grounded Theory Analysis, Interpretive Analysis, Narrative Analysis, Normative Analysis, Phenomenological Analysis, Rhetorical Analysis, and Semiotic Analysis, among others. The following resources should help you navigate your methodological options and put into practice methods for coding, themeing, interpreting, and presenting your data.
- Users can browse content by topic, discipline, or format type (reference works, book chapters, definitions, etc.). SRM offers several research tools as well: a methods map, user-created reading lists, a project planner, and advice on choosing statistical tests.
- Abductive Coding: Theory Building and Qualitative (Re)Analysis by Vila-Henninger, et al. The authors recommend an abductive approach to guide qualitative researchers who are oriented towards theory-building. They outline a set of tactics for abductive analysis, including the generation of an abductive codebook, abductive data reduction through code equations, and in-depth abductive qualitative analysis.
- Analyzing and Interpreting Qualitative Research: After the Interview by Charles F. Vanover, Paul A. Mihas, and Johnny Saldana (Editors) Providing insight into the wide range of approaches available to the qualitative researcher and covering all steps in the research process, the authors utilize a consistent chapter structure that provides novice and seasoned researchers with pragmatic, "how-to" strategies. Each chapter author introduces the method, uses one of their own research projects as a case study of the method described, shows how the specific analytic method can be used in other types of studies, and concludes with three questions/activities to prompt class discussion or personal study.
- "Analyzing Qualitative Data." Theory Into Practice 39, no. 3 (2000): 146-54 by Margaret D. LeCompte This article walks readers though rules for unbiased data analysis and provides guidance for getting organized, finding items, creating stable sets of items, creating patterns, assembling structures, and conducting data validity checks.
- "Coding is Not a Dirty Word" in Chapter 1 (pp. 1–30) of Enhancing Qualitative and Mixed Methods Research with Technology by Shalin Hai-Jew (Editor) Current discourses in qualitative research, especially those situated in postmodernism, represent coding and the technology that assists with coding as reductive, lacking complexity, and detached from theory. In this chapter, the author presents a counter-narrative to this dominant discourse in qualitative research. The author argues that coding is not necessarily devoid of theory, nor does the use of software for data management and analysis automatically render scholarship theoretically lightweight or barren. A lack of deep analytical insight is a consequence not of software but of epistemology. Using examples informed by interpretive and critical approaches, the author demonstrates how NVivo can provide an effective tool for data management and analysis. The author also highlights ideas for critical and deconstructive approaches in qualitative inquiry while using NVivo. By troubling the positivist discourse of coding, the author seeks to create dialogic spaces that integrate theory with technology-driven data management and analysis, while maintaining the depth and rigor of qualitative research.
- The Coding Manual for Qualitative Researchers by Johnny Saldana An in-depth guide to the multiple approaches available for coding qualitative data. Clear, practical and authoritative, the book profiles 32 coding methods that can be applied to a range of research genres from grounded theory to phenomenology to narrative inquiry. For each approach, Saldaña discusses the methods, origins, a description of the method, practical applications, and a clearly illustrated example with analytic follow-up. Essential reading across the social sciences.
- Flexible Coding of In-depth Interviews: A Twenty-first-century Approach by Nicole M. Deterding and Mary C. Waters The authors suggest steps in data organization and analysis to better utilize qualitative data analysis technologies and support rigorous, transparent, and flexible analysis of in-depth interview data.
- From the Editors: What Grounded Theory is Not by Roy Suddaby Walks readers through common misconceptions that hinder grounded theory studies, reinforcing the two key concepts of the grounded theory approach: (1) constant comparison of data gathered throughout the data collection process and (2) the determination of which kinds of data to sample in succession based on emergent themes (i.e., "theoretical sampling").
- “Good enough” methods for life-story analysis, by Wendy Luttrell. In Quinn N. (Ed.), Finding culture in talk (pp. 243–268). Demonstrates for researchers of culture and consciousness who use narrative how to concretely document reflexive processes in terms of where, how and why particular decisions are made at particular stages of the research process.
- The Ethnographic Interview by James P. Spradley “Spradley wrote this book for the professional and student who have never done ethnographic fieldwork (p. 231) and for the professional ethnographer who is interested in adapting the author’s procedures (p. iv) ... Steps 6 and 8 explain lucidly how to construct a domain and a taxonomic analysis” (excerpted from book review by James D. Sexton, 1980). See also: Presentation slides on coding and themeing your data, derived from Saldana, Spradley, and LeCompte Click to request access.
- Qualitative Data Analysis by Matthew B. Miles; A. Michael Huberman A practical sourcebook for researchers who make use of qualitative data, presenting the current state of the craft in the design, testing, and use of qualitative analysis methods. Strong emphasis is placed on data displays matrices and networks that go beyond ordinary narrative text. Each method of data display and analysis is described and illustrated.
- "A Survey of Qualitative Data Analytic Methods" in Chapter 4 (pp. 89–138) of Fundamentals of Qualitative Research by Johnny Saldana Provides an in-depth introduction to coding as a heuristic, particularly focusing on process coding, in vivo coding, descriptive coding, values coding, dramaturgical coding, and versus coding. Includes advice on writing analytic memos, developing categories, and themeing data.
- "Thematic Networks: An Analytic Tool for Qualitative Research." Qualitative Research : QR, 1(3), 385–405 by Jennifer Attride-Stirling Details a technique for conducting thematic analysis of qualitative material, presenting a step-by-step guide of the analytic process, with the aid of an empirical example. The analytic method presented employs established, well-known techniques; the article proposes that thematic analyses can be usefully aided by and presented as thematic networks.
- Using Thematic Analysis in Psychology by Virginia Braun and Victoria Clark Walks readers through the process of reflexive thematic analysis, step by step. The method may be adapted in fields outside of psychology as relevant. Pair this with One Size Fits All? What Counts as Quality Practice in Reflexive Thematic Analysis? by Virginia Braun and Victoria Clark
Data visualization can be employed formatively, to aid your data analysis, or summatively, to present your findings. Many qualitative data analysis (QDA) software platforms, such as NVivo , feature search functionality and data visualization options within them to aid data analysis during the formative stages of your project.
For expert assistance creating data visualizations to present your research, Harvard Library offers Visualization Support . Get help and training with data visualization design and tools—such as Tableau—for the Harvard community. Workshops and one-on-one consultations are also available.
The quality of your data analysis depends on how you situate what you learn within a wider body of knowledge. Consider the following advice:
A good literature review has many obvious virtues. It enables the investigator to define problems and assess data. It provides the concepts on which percepts depend. But the literature review has a special importance for the qualitative researcher. This consists of its ability to sharpen his or her capacity for surprise (Lazarsfeld, 1972b). The investigator who is well versed in the literature now has a set of expectations the data can defy. Counterexpectational data are conspicuous, readable, and highly provocative data. They signal the existence of unfulfilled theoretical assumptions, and these are, as Kuhn (1962) has noted, the very origins of intellectual innovation. A thorough review of the literature is, to this extent, a way to manufacture distance. It is a way to let the data of one's research project take issue with the theory of one's field.
- McCracken, G. (1988), The Long Interview, Sage: Newbury Park, CA, p. 31
Once you have coalesced around a theory, realize that a theory should reveal rather than color your discoveries. Allow your data to guide you to what's most suitable. Grounded theory researchers may develop their own theory where current theories fail to provide insight. This guide on Theoretical Models from Alfaisal University Library provides a helpful overview on using theory.
If you'd like to supplement what you learned about relevant theories through your coursework and literature review, try these sources:
- Annual Reviews Review articles sum up the latest research in many fields, including social sciences, biomedicine, life sciences, and physical sciences. These are timely collections of critical reviews written by leading scientists.
- HOLLIS - search for resources on theories in your field Modify this example search by entering the name of your field in place of "your discipline," then hit search.
- Oxford Bibliographies Written and reviewed by academic experts, every article in this database is an authoritative guide to the current scholarship in a variety of fields, containing original commentary and annotations.
- ProQuest Dissertations & Theses (PQDT) Indexes dissertations and masters' theses from most North American graduate schools as well as some European universities. Provides full text for most indexed dissertations from 1990-present.
- Very Short Introductions Launched by Oxford University Press in 1995, Very Short Introductions offer concise introductions to a diverse range of subjects from Climate to Consciousness, Game Theory to Ancient Warfare, Privacy to Islamic History, Economics to Literary Theory.
- << Previous: Recording & Transcription
- Next: Managing Interview Data >>
Except where otherwise noted, this work is subject to a Creative Commons Attribution 4.0 International License , which allows anyone to share and adapt our material as long as proper attribution is given. For details and exceptions, see the Harvard Library Copyright Policy ©2021 Presidents and Fellows of Harvard College.
We Trust in Human Precision
20,000+ Professional Language Experts Ready to Help. Expertise in a variety of Niches.
API Solutions
- API Pricing
- Cost estimate
- Customer loyalty program
- Educational Discount
- Non-Profit Discount
- Green Initiative Discount1
Value-Driven Pricing
Unmatched expertise at affordable rates tailored for your needs. Our services empower you to boost your productivity.

- Special Discounts
- Enterprise transcription solutions
- Enterprise translation solutions
- Transcription/Caption API
- AI Transcription Proofreading API
Trusted by Global Leaders
GoTranscript is the chosen service for top media organizations, universities, and Fortune 50 companies.
GoTranscript
One of the Largest Online Transcription and Translation Agencies in the World. Founded in 2005.
Speaker 1: Hi, Dr. Eli Lee over here of DEDUCE. My training is as a quantitative psychologist and I've been working in the field for over 20 years. But over the last 15, I really dove into doing mixed methods research. We found out a long time ago that there really weren't any tools to help us do the kinds of work that we wanted to do when we were using methods from psychology, anthropology, sociology, marketing, research. So we came up with a tool. It's evolved over the last 10 years and now we're really happy to show you what DEDUCE can do for qualitative research and mixed methods research. So what are mixed methods? Well, most people are pretty familiar with quantitative research methods. Quantitative methods are great for learning about what kinds of things there are, what kinds of people are they, and how many. So we might ask questions about people's demographics, their gender, age, income level. We might get scores. We might have them rate on a 1 to 10 scale how important to you is education, religion, family. And we might have them fill out other kinds of scales like depression. How happy are you on a scale of 1 to 100 or a bunch of items that we fold together to make scale scores. And so what do we do with these things? We can analyze them as individual variables like age group, 1, 2, 3, how many, what percentage of our population sits in each of those categories. We can do bivariate relationships looking at age by sex, for example. So here's the age group here, but when we break it out by males and females, we see there's a different percentage in each of those groups. And we can do multivariate types of analysis as well. So here's an average rating of how important family is to you broken out by the age group and by sex. There's all kinds of great and powerful things that we can do with quantitative data analysis, and we're not here to talk about that. Qualitative methods seek to understand human behavior and the reasons for those behaviors. A lot of people are less familiar with qualitative research methods than they are with quantitative. But basically qualitative data and the methods that generate them are seeking answers for why people do the things that they do and how they do them. What motivates them? What feelings drive the kinds of decisions that people make? What values? What things about their cultural background are important in understanding why they act the way that they do? So as a simple illustration, let's say we're interested in understanding why people make certain decisions about hotels. We go out to the field and have people just tell us stories about their most recent hotel decision. Typical approach to these data from qualitative research perspective would be to go through those texts and look for the themes that people talk about in a consistent way. So let's say for example after we look at a whole bunch of these stories, we hear people talking about luxury, sophistication, and intimacy on a pretty regular basis. The next thing that we do is go through those stories looking for sections of text where people talk about one or more of these things. We can also apply a weighting system. Here we've got an example of a 1 to 10 weight scale based on how important it is. So let's say in this story here's a piece of text where they're talking about luxury and intimacy. So we'll say 1 and 3 are the codes and they really thought luxury was the most important factor. So we'll give that a weight of 10 and intimacy was moderately important, we'll give that a 7. So we continue going through those texts looking for all the sections where people talk about one or more of these texts. So here's another one. They're talking about sophistication. Sophistication they say, well you know people talk about that but it's really not that important to me. So we'll give that a weight of 2. Here's one more. They're talking about luxury and sophistication. Again sophistication is really low, luxury remains really high. But wouldn't it be cool if we could put all these methods together? Some famous researchers have talked about the fact that all research methods have flaws. So by mixing methods together we seek to capitalize on the strengths of each and avoid some of the weaknesses. Deduce is great just for qualitative data analysis but when people are trying to bring it all together in a true mixed method design that's where deduce really shines. That's why we built it and that's what it was designed to accomplish as efficiently as possible. These studies can be really complex and people are looking for answers as efficiently as they can. With today's technologies we were able to put together a tool that accomplishes all of this and gets you to your answers very quickly. So come check it out. Alright welcome to my computer. I'm going to just log in to deduce but remember as a web application anywhere I have a computer and internet access I can log in to my project. So here's our hotel project. Our resources are here. Here are the tags we've already created. Here's some excerpts that we've already created and tagged. Our descriptor data are over here. Here's the fields that we've defined and here are the data themselves. And right here on our dashboard we get a glimpse of the many data visualizations that deduce produces automatically. Most of them can be modified and all are dynamically linked to the qualitative data that they represent. So if we're interested in drilling down to those excerpts from the 50 plus age group that were tagged with cost, simply click on that bar, pulls those excerpts up and we can go ahead and exam them further. Also deduce is very transparent and allows you to move seamlessly throughout your database. So if we pull up excerpts that we're interested in and we want to drill in a little bit deeper simply clicking on that excerpt takes us to the excerpt itself in the context of its original source. So let's go back home. It's also important to point out that all the charts, graphs, list of excerpts, descriptor data can all be exported from deduce with a simple click to be popped right into presentations or imported into other software. So let me show you one of the other important set up activities, getting your documents or resources into deduce. I'm going to go ahead and just import a document, prompts me to find the resource on my computer. I'll go ahead and give it a title, submit that to the database. You can see that deduce supports images in virtually any language. So here's some example text from the hotel study. So I'm going to go ahead and just block a piece of text to create an excerpt. I'll right click, create excerpt. Now I can go ahead and attach tags to it. This person talks about sexiness factor but that's not particularly important for them. Also sophistication is reasonably important. We'll give that seven. Go ahead and create another excerpt here. Service is high on their list. Let's go ahead and create an excerpt there. Give service a ten. Another thing that we need to do when we put resources into a project is attach it to the appropriate individual so that it's linked to the descriptor data. Let's go ahead and attach that person here. Okay, so we can see that one descriptor has been attached now. Now the really fun part. Let's get over to the analysis center. There are a wide variety of charts, graphs, plots and tables in deduce. There's really too much to show in this brief introduction so let's go after answers to just a couple of questions. You see that there are lots of charts, tables and plots that are available in deduce. Let's go ahead and look at something based on our tagging activity. We'll look at tag co-occurrence for example. What we see here is code by code matrix. This shows where two tags have been used on the same excerpt. The numbers, the tagging activity and all the descriptor data expose the patterns in our data. The qualitative data that sits behind those images is what really gives us the richness in this mixed methods research. We see here that 13 times luxury and cost were used on the same excerpt. If it's meaningful to our research that people talk about cost and luxury at the same time, we might want to go and look at those excerpts. Here we can pull them up. We can explore them. Again we can jump back to the resource and export these as well. Let's go ahead and close that. In terms of exposing patterns, bubble plots are really illuminating. This plot gives us information about the average weights or importance or sentiments that were associated with our tagging activity. I'm going to go ahead and break things out by age group. Let's use the tags luxury, sophistication and intimacy. This plot actually gives us four dimensions to look at our data. The plots themselves can communicate a great deal, can be exported and popped into presentations. We can also learn a lot more about the pattern by drilling down into the excerpts themselves. This bubble here represents the age group 50 plus and this group was relatively low in the importance of luxury but relatively high in sophistication and intimacy in their hotel decisions. If we're putting together a marketing message, we want to come up with something that's really going to resonate with this particular subgroup. We go ahead and open up those excerpts and we can really get a feel for how people are talking about these things and understand the reasons for why they feel the particular characteristics are more or less important when they're making their decisions. There's so much more I'd love to show you about deduce but I think we're out of time. Thanks for checking in. We've got a number of other videos on our website that give you step-by-step instructions about how to set projects up and how to get the best out of deducing your work.

Emotion Regulation and Academic Burnout Among Youth: a Quantitative Meta-analysis
- META-ANALYSIS
- Open access
- Published: 10 September 2024
- Volume 36 , article number 106 , ( 2024 )
Cite this article
You have full access to this open access article
- Ioana Alexandra Iuga ORCID: orcid.org/0000-0001-9152-2004 1 , 2 &
- Oana Alexandra David ORCID: orcid.org/0000-0001-8706-1778 2 , 3
Emotion regulation (ER) represents an important factor in youth’s academic wellbeing even in contexts that are not characterized by outstanding levels of academic stress. Effective ER not only enhances learning and, consequentially, improves youths’ academic achievement, but can also serve as a protective factor against academic burnout. The relationship between ER and academic burnout is complex and varies across studies. This meta-analysis examines the connection between ER strategies and student burnout, considering a series of influencing factors. Data analysis involved a random effects meta-analytic approach, assessing heterogeneity and employing multiple methods to address publication bias, along with meta-regression for continuous moderating variables (quality, female percentage and mean age) and subgroup analyses for categorical moderating variables (sample grade level). According to our findings, adaptive ER strategies are negatively associated with overall burnout scores, whereas ER difficulties are positively associated with burnout and its dimensions, comprising emotional exhaustion, cynicism, and lack of efficacy. These results suggest the nuanced role of ER in psychopathology and well-being. We also identified moderating factors such as mean age, grade level and gender composition of the sample in shaping these associations. This study highlights the need for the expansion of the body of literature concerning ER and academic burnout, that would allow for particularized analyses, along with context-specific ER research and consistent measurement approaches in understanding academic burnout. Despite methodological limitations, our findings contribute to a deeper understanding of ER's intricate relationship with student burnout, guiding future research in this field.
Similar content being viewed by others
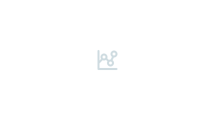
Does Burnout Affect Academic Achievement? A Meta-Analysis of over 100,000 Students
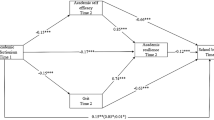
Antecedents of school burnout: A longitudinal mediation study
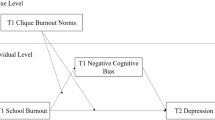
How School Burnout Affects Depression Symptoms Among Chinese Adolescents: Evidence from Individual and Peer Clique Level
Avoid common mistakes on your manuscript.
Introduction
The transitional stages of late adolescence and early adulthood are characterized by significant physiological and psychological changes, including increased stress (Matud et al., 2020 ). Academic stress among students has long been studied in various samples, most of them focusing on university students (Bedewy & Gabriel, 2015 ; Córdova Olivera et al., 2023 ; Hystad et al., 2009 ) and, more recently, high school (Deb et al., 2015 ) and middle school students (Luo et al., 2020 ). Further, studies report an exacerbation of academic stress and mental health difficulties in response to the COVID-19 pandemic (Guessoum et al., 2020 ), with children facing additional challenges that affect their academic well-being, such as increasing workloads, influences from the family, and the issue of decreasing financial income (Ibda et al., 2023 ; Yang et al., 2021 ). For youth to maintain their well-being in stressful academic settings, emotion regulation (ER) has been identified as an important factor (Santos Alves Peixoto et al., 2022 ; Yildiz, 2017 ; Zahniser & Conley, 2018 ).
Emotion regulation, referring to”the process by which individuals influence which emotions they have, when they have them, and how they experience and express their emotions” (Gross, 1998b ), represents an important factor in youth’s academic well-being even in contexts that are not characterized by outstanding levels of stress. Emotion regulation strategies promote more efficient learning and, consequentially, improve youth’s academic achievement and motivation (Asareh et al., 2022 ; Davis & Levine, 2013 ), discourage academic procrastination (Mohammadi Bytamar et al., 2020 ), and decrease the chances of developing emotional problems such as burnout (Narimanj et al., 2021 ) and anxiety (Shahidi et al., 2017 ).
Approaches to Emotion Regulation
Numerous theories have been proposed to elucidate the process underlying the emergence and progression of emotional regulation (Gross, 1998a , 1998b ; Koole, 2009 ; Larsen, 2000 ; Parkinson & Totterdell, 1999 ). One prominent approach, developed by Gross ( 2015 ), refers to the process model of emotion regulation, which lays out the sequential actions people take to regulate their emotions during the emotion-generative process. These steps involve situation selection, situation modification, attentional deployment, cognitive change, and response modulation. The kind and timing of the emotion regulation strategies people use, according to this paradigm, influence the specific emotions people experience and express.
Recent theories of emotion regulation propose two separate, yet interconnected approaches: ER abilities and ER strategies. ER abilities are considered a higher-order process that guides the type of ER strategy an individual uses in the context of an emotion-generative circumstance. Further, ER strategies are considered factors that can also influence ER abilities, forming a bidirectional relationship (Tull & Aldao, 2015 ). Researchers use many definitions and classifications of emotion regulation, however, upon closer inspection, it becomes clear that there are notable similarities across these concepts. While there are many models of emotion regulation, it's important to keep from seeing them as competing or incompatible since each one represents a unique and important aspect of the multifaceted concept of emotion regulation.
Emotion Regulation and Emotional Problems
The connection between ER strategies and psychopathology is intricate and multifaceted. While some researchers propose that ER’s effectiveness is context-dependent (Kobylińska & Kusev, 2019 ; Troy et al., 2013 ), several ER strategies have long been attested as adaptive or maladaptive. This body of work suggests that certain emotion regulation strategies (such as avoidance and expressive suppression) demonstrate, based on findings from experimental studies, inefficacy in altering affect and appear to be linked to higher levels of psychological symptoms. These strategies have been categorized as ER difficulties. In contrast, alternative emotion regulation strategies (such as reappraisal and acceptance) have demonstrated effectiveness in modifying affect within controlled laboratory environments, exhibiting a negative association with clinical symptoms. As a result, these strategies have been characterized as potentially adaptive (Aldao & Nolen-Hoeksema, 2012a , 2012b ; Aldao et al., 2010 ; Gross, 2013 ; Webb et al., 2012 ).
A long line of research highlights the divergent impact of putatively maladaptive and adaptive ER strategies on psychopathology and overall well-being (Gross & Levenson, 1993 ; Gross, 1998a ). Increased negative affect, increased physiological reactivity, memory problems (Richards et al., 2003 ), a decline in functional behavior (Dixon-Gordon et al., 2011 ), and a decline in social support (Séguin & MacDonald, 2018 ) are just a few of the negative effects that have consistently been linked to emotional regulation difficulties, which include but are not limited to the use of avoidance, suppression, rumination, and self-blame strategies. Additionally, a wide range of mental problems, such as depression (Nolen-Hoeksema et al., 2008 ), anxiety disorders (Campbell-Sills et al., 2006a , 2006b ; Mennin et al., 2007 ), eating disorders (Prefit et al., 2019 ), and borderline personality disorder (Lynch et al., 2007 ; Neacsiu et al., 2010 ) are connected to self-reports of using these strategies.
Conversely, putatively adaptive strategies, including acceptance, problem-solving, and cognitive reappraisal, have consistently yielded beneficial outcomes in experimental studies. These outcomes encompass reductions in negative emotional responses, enhancements in interpersonal relationships, increased pain tolerance, reductions in physiological reactivity, and lower levels of psychopathological symptoms (Aldao et al., 2010 ; Goldin et al., 2008 ; Hayes et al., 1999 ; Richards & Gross, 2000 ).
Notably, despite the fact that therapeutic techniquest for enhancing the use of adaptive ER strategies are core elements of many therapeutic approaches, from traditional Cognitive Behavioral Therapy (CBT) to more recent third-wave interventions (Beck, 1976 ; Hofmann & Asmundson, 2008 ; Linehan, 1993 ; Roemer et al., 2008 ; Segal et al., 2002 ), the association between ER difficulties and psychopathology frequently show a stronger positive correlation compared to the inverse negative association with adaptive ER strategies, as highlighted by Aldao and Nolen-Hoeksema ( 2012a ).
Pines & Aronson ( 1988 ) characterize burnout that arises in the workplace context as a state wherein individuals encounter emotional challenges, such as experiencing fatigue and physical exhaustion due to heightened task demands. Recently, driven by the rationale that schools are the environments where students engage in significant work, the concept of burnout has been extended to educational contexts (Salmela-Aro, 2017 ; Salmela-Aro & Tynkkynen, 2012 ; Walburg, 2014 ). Academic burnout is defined as a syndrome comprising three dimensions: exhaustion stemming from school demands, a cynical and detached attitude toward one's academic environment, and feelings of inadequacy as a student (Salmela-Aro et al., 2004 ; Schaufeli et al., 2002 ).
School burnout has quickly garnered international attention, despite its relatively recent emergence, underscoring its relevance across multiple nations (Herrmann et al., 2019 ; May et al., 2015 ; Meylan et al., 2015 ; Yang & Chen, 2016 ). Similar to other emotional difficulties, it has been observed among students from various educational systems and academic policies, suggesting that this phenomenon transcends cultural and geographical boundaries (Walburg, 2014 ).
The link between ER and school burnout can be understood through Gross's ( 1998a ) process model of emotion regulation. This model suggests that an individual's emotional responses are influenced by their ER strategies, which are adaptive or maladaptive reactions to stressors like academic pressure. Given that academic stress greatly influences school burnout (Jiang et al., 2021 ; Nikdel et al., 2019 ), the ER strategies students use to manage this stress may impact their likelihood of experiencing burnout. In essence, whether a student employs efficient ER strategies or encounters ER difficulties could influence their susceptibility to school burnout.
The exploration of ER in relation to student burnout has garnered attention through various studies. However, the existing body of research is not yet robust enough, and its outcomes are not universally congruent. Suppression, defined as efforts to inhibit ongoing emotional expression (Balzarotti et al., 2010 ), has demonstrated a positive and significant correlation with both general and specific burnout dimensions (Chacón-Cuberos et al., 2019 ; Seibert et al., 2017 ), with the exception of the study conducted by Yu et al., ( 2022 ), where there is a negative, but not significant association between suppression and reduced accomplishment. Notably, research by Muchacka-Cymerman and Tomaszek ( 2018 ) indicates that ER strategies, encompassing both dispositional and situational approaches, exhibit a negative relationship with overall burnout. Situational ER, however, displays a negative impact on dimensions like inadequacy and declining interest, particularly concerning the school environment.
Cognitive ER strategies such as reappraisal, positive refocusing, and planning are, generally, negatively associated with burnout, while self-blame, other-blame, rumination, and catastrophizing present a positive association with burnout (Dominguez-Lara, 2018 ; Vinter et al., 2021 ). It's important to note that these relationships have not been consistently replicated across all investigations. Inconsistencies in the findings highlight the complexity of the interactions and the potential influence of various contextual factors. Consequently, there remains a critical need for further research to thoroughly examine these associations and identify the factors contributing to the variability in results.
Existing Research
Although we were unable to identify any reviews or meta-analyses that synthesize the literature concerning emotion regulation strategies and student burnout, recent meta-analyses have identified the role of emotion regulation across pathologies. A recent network meta-analysis identified the role of rumination and non-acceptance of emotions to be closely related to eating disorders (Leppanen et al., 2022 ). Further, compared to healthy controls, people presenting bipolar disorder symptoms reported significantly higher difficulties in emotion regulation (Miola et al., 2022 ). Weiss et al. ( 2022 ) identified a small to medium association between emotion regulation and substance use, and a subsequent meta-analysis conducted by Stellern et al. ( 2023 ) confirmed that individuals with substance use disorders have significantly higher emotion regulation difficulties compared to controls. The study of Dawel et al. ( 2021 ) represents the many research papers asking the question”Cause or symptom” in the context of emotion regulation. The longitudinal study brings forward the bidirectional relationship between ER and depression and anxiety, particularly in the case of suppression, suggesting that suppressing emotions is indicative of and can predict psychological distress.
Despite the increasing research attention to academic burnout in recent years, the current body of literature primarily concentrates on specific groups such as medical students (Almutairi et al., 2022 ; Frajerman et al., 2019 ), educators (Aloe et al., 2014 ; Park & Shin, 2020 ), and students at the secondary and tertiary education levels (Madigan & Curran, 2021 ) in the context of meta-analyses and reviews. A limited number of recent reviews have expanded their focus to include a more diverse range of participants, encompassing middle school, graduate, and university students (Kim et al., 2018 , 2021 ), with a particular emphasis on investigating social support and exploring the demand-control-support model in relation to student burnout.
The significance of managing burnout in educational settings is becoming more widely acknowledged, as seen by the rise in interventions designed to reduce the symptoms of burnout in students. Specific interventions for alleviating burnout symptoms among students continue to proliferate (Madigan et al., 2023 ), with a focus on stress reduction through mindfulness-based strategies (Lo et al., 2021 ; Modrego-Alarcón et al., 2021 ) and rational-emotive behavioral techniques (Ogbuanya et al., 2019 ) to enhance emotion-regulation skills (Charbonnier et al., 2022 ) and foster rational thinking (Bresó et al., 2011 ; Ezeudu et al., 2020 ). This underscores the significance of emotion regulation in addressing burnout.
Despite several randomized clinical trials addressing student burnout and an emerging body of research relating emotion regulation and academic burnout, there's a lack of a systematic examination of how emotion regulation strategies relate to various dimensions of student burnout. This highlights the necessity for a systematic review of existing evidence. The current meta-analysis addresses the association between emotion regulation strategies and student burnout.
A secondary objective is to test the moderating effect of school level and female percentage in the sample, as well as study quality, in order to identify possible sources of heterogeneity among effect sizes. By analyzing the moderating effect of school level and gender, we may determine if the strength of the association between student burnout and emotion regulation is contingent upon the educational setting and participant characteristics. This offers information on the findings' generalizability to all included student demographics, including those in elementary, middle, and secondary education and of different genders. Additionally, the reliability and validity of meta-analytic results rely on the evaluation of research quality, and the inclusion of study quality rating allows us to determine if the observed association between emotion regulation and student burnout differs based on the methodological rigor of the included studies.
Materials and Methods
Study protocol.
The present meta-analysis has been carried out following the Preferred Reporting Items for Systematic Review and Meta-Analyses (PRISMA) statement (Moher et al., 2009 ). The protocol for the meta-analysis was pre-registered in PROSPERO (PROSPERO, 2022 CRD42022325570).
Selection of Studies
A systematic search was performed using relevant databases (PubMed, Web of Science, PsychINFO, and Scopus). The search was carried out on 25 May of 2023 using 25 key terms related to the variables of interest, such as: (a) academic burnout, (b) school burnout, (c) student burnout (d) education burnout, (d) exhaustion, (e) cynicism, (f) inadequacy, (g) emotion regulation, (h) coping, (i) self-blame, (j) acceptance, and (h) problem solving.
Studies of any design published in peer-reviewed journals were eligible for inclusion, provided they used empirical data to assess the relationship between student burnout and emotion regulation strategies. Only studies that employed samples of children, adolescents, and youth were eligible for inclusion. For the purpose of the current paper, we define youth as people aged 18 to 25, based on how it is typically defined in the literature (Westhues & Cohen, 1997 ).
Studies were excluded from the meta-analysis if they: (a) were not a quantitative study, (b) did not explore the relationship between academic burnout and emotion regulation strategies, (c) did not have a sample that can be defined as consisting of children and youth (Scales et al., 2016 ), (e) did not utilize Pearson’s correlation or measures that could be converted to a Pearson’s correlation, (f) include medical school or associated disciplines samples.
Statistical Analysis
For the data analysis, we employed Comprehensive Meta-Analysis 4 software. Anticipating significant heterogeneity in the included studies, we opted for a random effects meta-analytic approach instead of a fixed-effects model, a choice that acknowledges and accounts for potential variations in effect sizes across studies, contributing to a more robust and generalizable synthesis of the results. Heterogeneity among the studies was assessed using the I 2 and Q statistics, adhering to the interpretation thresholds outlined in the Cochrane Handbook (Deeks et al., 2023 ).
Publication bias was assessed through a multi-faceted approach. We first examined the funnel plot for the primary outcome measures, a graphical representation revealing potential asymmetry that might indicate publication bias. Furthermore, we utilized Duval and Tweedie's trim and fill procedure (Duval & Tweedie, 2000 ), as implemented in CMA, to estimate the effect size after accounting for potential publication bias. Additionally, Egger's test of the intercept was conducted to quantify the bias detected by the funnel plot and to determine its statistical significance.
When dealing with continuous moderating variables, we employed meta-regression to evaluate the significance of their effects. For categorical moderating variables, we conducted subgroup analyses to test for significance. To ensure the validity of these analyses, it was essential that there was a minimum of three effect sizes within each subgroup under the same moderating variable, following the guidelines outlined by Junyan and Minqiang ( 2020 ). In accordance with the guidance provided in the Cochrane Handbook (Schmid et al., 2020 ), our application of meta-regression analyses was limited to cases where a minimum of 10 studies were available for each examined covariate. This approach ensures that there is a sufficient number of studies to support meaningful statistical analysis and reliable conclusions when exploring the influence of various covariates on the observed relationships.
Data Extraction and Quality Assessment
In addition to the identification information (i.e., authors, publication year), we extracted data required for the effect size calculation for the variables relevant to burnout and emotion regulation strategies. Where data was unavailable, the authors were contacted via email in order to provide the necessary information. Potential moderator variables were coded in order to examine the sources of variation in study findings. The potential moderators included: (a) participants’ gender, (b), grade level (c) study quality, and (d) mean age.
The full-text articles were independently assessed using the Standard Quality Assessment Criteria for Evaluating Primary Research Papers from a Variety of Fields tool (Kmet et al., 2004 ) by a pair of coders (II and SM), to ensure the reliability of the data, resulting in a substantial level of agreement (Cohen’s k = 0.89). The disagreements and discrepancies between the two coders were resolved through discussion and consensus. If consensus could not be reached, a third researcher (OD) was consulted to resolve the disagreement.
The checklist items focused on evaluating the alignment of the study's design with its stated objectives, the methodology employed, the level of precision in presenting the results, and the accuracy of the drawn conclusions. The assessment criteria were composed of 14 items, which were evaluated using a 3-point Likert scale (with responses of 2 for "yes," 1 for "partly," and 0 for "no"). A cumulative score was computed for each study based on these items. For studies where certain checklist items were not relevant due to their design, those items were marked as "n/a" and were excluded from the cumulative score calculation.
Study Selection
The combined search terms yielded a total of 15,179 results. The duplicate studies were removed using Zotero, and a total of 8,022 studies remained. The initial screening focused on the titles and abstracts of all remaining studies, removing all documents that target irrelevant predictors or outcomes, as well as qualitative studies and reviews. Two assessors (II and SA) independently screened the papers against the inclusion and exclusion criteria. A number of 7,934 records were removed, while the remaining 88 were sought for retrieval. Out of the 88 articles, we were unable to find one, while another has been retracted by the journal. Finally, 86 articles were assessed for eligibility. A total of 20 articles met the inclusion criteria (see Fig. 1 ). Although a specific cutoff criterion for reliability coefficients was not imposed during study selection, the majority of the included studies had alpha Cronbach values for the instruments assessing emotion regulation and school burnout greater than 0.70.
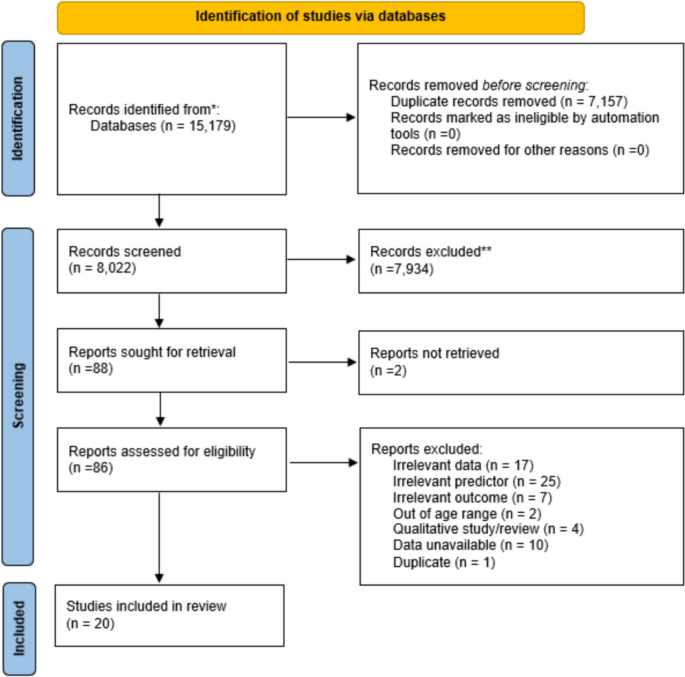
Preferred Reporting Items for Systematic Reviews and Meta-Analyses (PRISMA) flowchart of the study selection process
Data Overview
Among the included studies, four focused on middle school students, two encompassed high school student samples, and the remaining 14 articles involved samples of university students. The majority of the included studies had cross-sectional designs (17), while the rest consisted of 2 longitudinal studies and one non-randomized controlled pilot study. The percentage of females within the samples ranged from 46% to 88.3%, averaging 65%, while the mean age of participants ranged from 10.39 to 25. The investigated emotional regulation strategies within the included studies exhibit variation, encompassing other-blame, self-blame, acceptance, rumination, catastrophizing, putting into perspective, reappraisal, planning, behavioral and mental disengagement, expressive suppression, and others (see Table 1 for a detailed study presentation).
Study Quality
Every study surpasses a quality threshold of 0.60, and 75% of the studies achieve a score above the more conservative threshold indicated by Kmet et al. ( 2004 ). This indicates a minimal risk of bias in these studies. Moreover, 80% of the studies adequately describe their objectives, while the appropriateness of the study design is recognized in 50% of the cases, mostly utilizing cross-sectional designs. While 95% of the studies provide sufficient descriptions of their samples, only 10% employ appropriate sampling methods, with the majority relying on convenience sampling. Notably, there is just one interventional study that lacks random allocation and blinding of investigators or subjects.
In terms of measurement, 85% of the studies employ validated and reliable tools. Adequacy in sample size and well-justified and appropriate analytic methods are observed across all included studies. While approximately 50% of the studies present estimates of variance, a mere 30% of them acknowledge the control of confounding variables. Lastly, 95% of the studies provide results in comprehensive detail, with 60% effectively grounding their discussions in the obtained results. The quality assessment criteria and results can be consulted in Supplementary Material 4 .
Pooled Effects
A sensitivity analysis using standardized residuals was conducted. Provided that the residuals are normally distributed, 95% of them would fall within the range of -2 to 2. Residuals outside this range were considered unusual. We applied this cutoff in our meta-analysis to identify any outliers. The results of the analysis revealed that several relationships had standardized residuals falling outside the specified range. Re-analysis excluding these outliers demonstrated that our initial results were robust and did not significantly change in magnitude or significance. As a result, we have moved on with the analysis for the entire sample.
The calculated overall effects can be consulted in Table 2 . The correlation between ER difficulties and student burnout is a significant one, with significant positive associations between ER difficulties and overall burnout (k = 13), r = 0.25 (95% CI = 0.182; 0.311), p < 0.001, as well as individual burnout dimensions: cynicism (k = 9), r = 0.28 (95% CI = 0.195; 0.353) p < 0.001, lack of efficacy (k = 8), r = 0.17 (95% CI = 0.023; 0.303), p < 0.05 and emotional exhaustion (k = 11), r = 0.27 (95% CI = 0.207; 0.335) p < 0.001. Regarding the relationship between adaptive ER strategies and student burnout, a statistically significant result is observed solely between overall student burnout and adaptive ER (k = 17), r = -14 (95% CI = -0.239; 0.046) p < 0.005. The forest plots can be consulted in Supplementary Material 1 .
Heterogeneity and Publication Bias
Table 3 shows that all Q tests were significant, indicating that there is significant variation among the effect sizes of the individual studies included in the meta-analysis. Further, all I 2 indices are over 75%, ranging from 83.67% to 99.32%, which also indicates high heterogeneity (Borenstein et al., 2017 ). This consistently high level of heterogeneity indicates substantial variation in effect sizes, pointing to influential factors that significantly shape the outcomes of the included studies. Consequentially, subgroup and meta-regression analyses are to be carried out in order to unravel the underlying factors driving this pronounced heterogeneity. The results of the publication bias analysis are presented individually below and, additionally, you can consult the funnel plots included in Supplementary Material 2 .
Adaptive ER and School Burnout
Upon visual examination of the funnel plot, asymmetry to the right of the mean was observed. To validate this observation, a trim-and-fill analysis using Duval and Tweedie’s method was conducted, revealing the absence of three studies on the left side of the mean. The adjusted effect size ( r = -0.17, 95% CI [0.27; 0.68]) resulting from this analysis was found to be higher than the initially observed effect size. Nevertheless, the application of Egger’s test did not yield a significant indication of publication bias ( B = -5.34, 95% CI [-11.85; 1.16], p = 0.10).
Adaptive ER and Cynicism
Following a visual examination of the funnel plot, a symmetrical arrangement of effect sizes around the mean was apparent. This finding was contradicted by the application of Duval and Tweedie's trim-and-fill method, which revealed two missing studies to the right of the mean. The adjusted effect size ( r = 0.04, 95% CI [-0.21; 0.13]) is smaller than the initially observed effect size. The application of Egger’s test did not yield a significant indication of publication bias ( B = -2.187, 95% CI [-8.57; 4.19], p = 0.43).
ER difficulties and Lack of Efficacy
The visual examination of the funnel plot revealed asymmetry to the right of the mean. This finding was validated by the application of Duval and Tweedie's trim-and-fill method, which revealed two missing studies to the left of the mean and a lower adjusted effect size ( r = 0.08, 95% CI [-0.07; 0.23]), the effect becoming statistically non-significant. The application of Egger’s test did not yield a significant indication of publication bias ( B = 7.76, 95% CI [-16.53; 32.05], p = 0.46).
Adaptive ER and Emotional Exhaustion
The visual examination of the funnel plot revealed asymmetry to the left of the mean. The trim-and-fill method also revealed one missing study to the right of the mean and a lower adjusted effect size ( r = 0.00, 95% CI [-0.13; 0.12]). The application of Egger’s test did not yield a significant indication of publication bias ( B = 7.02, 95% CI [-23.05; 9.02], p = 0.46).
Adaptive ER and Lack of Efficacy; ER difficulties and School Burnout, Cynicism, and Exhaustion
Upon visually assessing the funnel plot, a balanced distribution of effect sizes centered around the mean was observed. This observation is corroborated by the application of Duval and Tweedie's trim-and-fill method, which also revealed no indication of missing studies. The adjusted effect size remained consistent, and the intercept signifying publication bias was found to be statistically insignificant.
Moderator Analysis
We performed moderator analyses for the categorical variables, in the case of significant relationships that were uncovered in the initial analysis. These analyses were carried out specifically for cases where there were more than three effect sizes available within each subgroup that fell under the same moderating variable.
Students’ grade level was used as a categorical moderator. Pre-university students included students enrolled in primary and secondary education, while the university student category included tertiary education students. The results, presented in Table 4 , show that the moderating effect of grade level is not significant for the relationship between adaptive ER and overall school burnout Q (1) = 0.20, p = 0.66. At a specific level, the moderating effect is significant for the relationship between ER difficulties and overall burnout Q (1) = 9.81, p = 0.002, cynicism Q (1) = 16.27, p < 0.001, lack of efficacy Q (1) = 15.47 ( p < 0.001), and emotional exhaustion Q (1) = 13.85, p < 0.001. A particularity of the moderator analysis in the relationship between ER difficulties and lack of efficacy is that, once the effect of the moderator is accounted for, the relationship is not statistically significant anymore for the university level, r = -0.01 (95% CI = -0.132; 0.138), but significant for the pre-university level, r = 0.33 (95% CI = 0.217; 0.439).
Meta-regressions
Meta-regression analyses were employed to examine how the effect size or relationship between variables changes based on continuous moderator variables. We included as moderators the female percentage (the proportion of female participants in each study’s sample) and the study quality assessed based on the Standard Quality Assessment Criteria for Evaluating Primary Research Papers from a Variety of Fields tool (Kmet et al., 2004 ).
Results, presented in Table 5 , show that study quality does not significantly influence the relationship between ER and school burnout. The proportion of female participants in the study sample significantly influences the relationship between ER difficulties and overall burnout ( β , -0.0055, SE = 0.001, p < 0.001), as well as the emotional exhaustion dimension ( β , -0.0049, SE = 0.002, p < 0.01). Mean age significantly influences the relationship between ER difficulties and overall burnout ( β , -0.0184, SE = 0.006, p < 0.01). Meta-regression plots can be consulted in detail in Supplementary Material 3 .
A post hoc power analysis was conducted using the metapower package in R. For the pooled effects analysis of the relationship between ER difficulties and overall school burnout, as well as with cynicism and emotional exhaustion, the statistical power was adequate, surpassing the recommended 0.80 cutoff. The analysis of the association between ER difficulties and lack of efficacy, along with the relationship between adaptive ER and school burnout, cynicism, lack of efficacy, and emotional exhaustion were greatly underpowered. In the case of the moderator analysis, the post-hoc power analysis indicates insufficient power. Please consult the coefficients in Table 6 .
The central goal of this meta-analysis was to examine the relationship between emotion-regulation strategies and student burnout dimensions. Additionally, we focused on the possible effects of sample distribution, in particular on participants’ age, education levels they are enrolled in, and the percentage of female participants included in the sample. The study also aimed to determine how research quality influences the overall findings. Taking into consideration the possible moderating effects of sample characteristics and research quality, the study aimed to offer a thorough assessment of the literature concerning the association between emotion regulation strategies and student burnout dimensions. A correlation approach was used as the current literature predominantly consists of cross-sectional studies, with insufficient longitudinal studies or other designs that would allow for causal interpretation of the results.
The study’s main findings indicate that adaptive ER strategies are associated with overall burnout, whereas ER difficulties are associated with both overall burnout and all its dimensions encompassing emotional exhaustion, cynicism, and lack of efficacy.
Prior meta-analyses have similarly observed that adaptive ER strategies tend to exhibit modest negative associations with psychopathology, while ER difficulties generally presented more robust positive associations with psychopathology (Aldao et al., 2010 ; Miu et al., 2022 ). These findings could suggest that the observed variation in the effect of ER strategies on psychopathology, as previously indicated in the literature, can also be considered in the context of academic burnout.
However, it would be an oversimplification to conclude that adaptive ER strategies are less effective in preventing psychopathology than ER difficulties are in creating vulnerability to it. Alternatively, as previously underlined, researchers should consider the frequency, flexibility, and variability in the way ER strategies are applied and how they relate to well-being and psychopathology. Further, it’s important to also address the possible directionality of the relationship. While the few studies that assume a prediction model for academic burnout and ER treat ER as a predictor for burnout and its dimensions (see Seibert et al., 2017 ; Vizoso et al., 2019 ), we were unable to identify studies that assume the role of burnout in the development of ER difficulties. Additionally, the studies identified that relate to academic burnout have a cross-sectional design that makes it even more difficult to pinpoint the ecological directionality of the relationship.
While the focus on the causal role of ER strategies in psychopathology and psychological difficulties is of great importance for psychological interventions, addressing a factor that merely reflects an effect or consequence of psychopathology will not lead to an effective solution. According to Gross ( 2015 ), emotion regulation strategies are employed when there is a discrepancy between a person's current emotional state and their desired emotional state. Consequently, individuals could be likely to also utilize emotion regulation strategies in response to academic burnout. Additionally, studies that have utilized a longitudinal approach have demonstrated that, in the case of spontaneous ER, people with a history of psychopathology attempt to regulate their emotions more when presented with negative stimuli (Campbell-Sills et al., 2006a , 2006b ; Ehring et al., 2010 ; Gruber et al., 2012 ). The results of Dawel et al. ( 2021 ) further solidify a bidirectional model that could and should be also applied to academic burnout research.
Following the moderator analysis, the results indicate that the moderating effect of grade level did not have a substantial impact on the relationship between adaptive ER and school burnout. In the context of this discussion, it is important to note that regarding the relationship between adaptive ER and overall burnout, there is an imbalance in the distribution of studies between the university and pre-university levels, which could potentially present a source of bias or error.
When it comes to the relationship between ER difficulties and burnout, the inclusion of the moderator exhibited notable significance, overall and at the dimensions’ level. Particularly noteworthy is the finding that, within the relationship involving ER difficulties and lack of efficacy, the inclusion of the moderator rendered the association statistically insignificant for university-level students, while maintaining significance for pre-university-level students. The outcomes consistently demonstrate larger effect sizes for the relationship between ER difficulties and burnout at the pre-university level in comparison to the university level. Additionally, the mean age significantly influences the relationship between ER difficulties and overall burnout.
These findings may imply the presence of additional variables that exert a varying influence at the two educational levels and as a function of age. There are several contextual factors that could be framing the current findings, such as parental education anxiety (Wu et al., 2022 ), parenting behaviors, classroom atmosphere (Lin & Yang, 2021 ), and self-efficacy (Naderi et al., 2018 ). As the level of independence drastically increases from pre-university to university, the influence of negative parental behaviors and attitudes can become limited. Furthermore, the university-level learning environment often provides a satisfying and challenging educational experience, with greater opportunities for students to engage in decision-making and take an active role in their learning (Belaineh, 2017 ), which can serve as a protective factor against student’s academic burnout (Grech, 2021 ). At an individual level, many years of experience in navigating the educational environment can increase youths’ self-efficacy in the educational context and offer proper learning tools and techniques, which can further influence various aspects of self-regulated learning, such as monitoring of working time and task persistence (Bouffard-Bouchard et al., 1991 ; Cattelino et al., 2019 ).
The findings of the meta-regression analysis suggest that the association between ER and school burnout is not significantly impacted by study quality. It’s important to interpret these findings in the context of rather homogenous study quality ratings that can limit the detection of significant impacts.
The current results underline that the correlation between ER difficulties and both overall burnout and the emotional exhaustion dimension is significantly influenced by the percentage of female participants in the study sample. Previous research has shown that girls experience higher levels of stress, as well as higher expectations concerning their school performance, which can originate not only intrinsically, but also from external sources such as parents, peers, and educators (Östberg et al., 2015 ). These heightened expectations and stress levels may contribute to the gender differences in how emotion regulation difficulties are associated with school burnout.
The results of this meta-analysis suggest that most of the included studies present an increased level of methodological quality, reaching or surpassing the quality thresholds previously established. These encouraging results indicate a minimal risk of bias in the selected research. Moreover, it’s notable that a sizable proportion of the included studies clearly articulate their research objectives and employ well-established measurement tools, that would accurately capture the constructs of interest. There are still several areas of improvement, especially with regard to variable conceptualization and sampling methods, highlighting the importance of maintaining methodological rigor in this area of research.
Significant Q tests and I 2 identified in the case of several analyses indicate a strong heterogeneity among the effect sizes of individual studies in the meta-analysis's findings. This variability suggests that there is a significant level of diversity and variation among the effects observed in the studies, and it is improbable that this diversity is solely attributable to random chance. Even with as few as 10 studies, with 30 participants in the primary studies, the Q test has been demonstrated to have good power for identifying heterogeneity (Maeda & Harwell, 2016 ). Recent research (Mickenautsch et al., 2024 ), suggests that the I 2 statistic is not influenced by the number of studies and sample sizes included in a meta-analysis. While the relationships between Adaptive ER—cynicism, ER difficulties—cynicism, Adaptive ER—lack of efficacy, and ER difficulties—lack of efficacy are based on a limited number of studies (8–9 studies), it's noteworthy that the primary study sample sizes for these relationships are relatively large, averaging above 300. This suggests that despite the small number of studies, the robustness of the findings may be supported by the substantial sample sizes, which can contribute to the statistical power of the analysis.
However, it's essential to consider potential limitations such as range restriction or measurement error, which could impact the validity of the findings. Despite these considerations, the combination of substantial primary study sample sizes and the robustness of the Q test provides a basis for confidence in the results.
The results obtained when publication bias was examined using funnel plots, trim-and-fill analyses, and Egger's tests were varying across different outcomes. In the case of adaptive emotion regulation (ER) and school burnout, no evidence of publication bias was found, suggesting that the observed effects are likely robust. The trim-and-fill analysis, however, indicated the existence of missing studies for adaptive ER and cynicism, potentially influencing the initial effect size estimate. For ER difficulties and lack of efficacy, the adjustment for missing studies in the trim-and-fill analysis led to a non-significant effect. Additionally, adaptive ER and emotional exhaustion displayed a similar pattern with the trim-and-fill method leading to a lower, non-significant effect size. This indicates the need for additional studies to be included in future meta-analyses. According to the Cochrane Handbook (Higgins et al., 2011 ), the results of Egger’s test and funnel plot asymmetry should be interpreted with caution, when conducted on fewer than 10 studies.
The results of the post-hoc power analysis reveal that the relationship between ER difficulties and cynicism, as well as emotional exhaustion, meets the threshold of 0.80 for statistical power, as suggested by Harrer et al. ( 2022 ). This implies that our study had a high likelihood of detecting significant associations between ER difficulties and these specific outcomes, providing robust evidence for the observed relationships. However, for the relationship between ER difficulties and overall burnout, the power coefficient falls just below the indicated threshold. While our study still demonstrated considerable power to detect effects, the slightly lower coefficient suggests a marginally reduced probability of detecting significant associations between ER difficulties and overall burnout.
The power coefficients for the remaining post-hoc analyses are fairly small, which suggests that there is not enough statistical power to find meaningful relationships. This shows that there might not have been enough power in our investigation to find significant correlations between the variables we sought to investigate. Even if these analyses' power coefficients are lower than ideal, it's important to consider the study's limitations and implications when interpreting the results.
Limitations and Future Directions
One important limitation of our meta-analysis is represented by the small number of studies included in the analysis. Smaller meta-analyses could result in less reliable findings, with estimates that could be significantly influenced by outliers and inclusion of studies with extreme results. The small number of studies also interferes with the interpretation of both Q and I 2 heterogeneity indices (von Hippel, 2015 ). In small sample sizes, it may be challenging to detect true heterogeneity, and the I 2 value may be imprecise or underestimate the actual heterogeneity.
The studies included in the current meta-analysis focused on investigating how individuals generally respond to stressors. However, it's crucial to remember that people commonly use various ER strategies based on particular contexts, or they could even combine ER strategies within a single context. This adaptability in ER strategies reflects the dynamic and context-dependent nature of emotional regulation, where people draw upon various tools and approaches to effectively manage their emotions in different circumstances.
Given the heterogeneity of studies that investigate ER as a context-dependent phenomenon in the context of academic burnout, as well as the diverse nature of these existing studies, it becomes imperative for future research to consider a number of key aspects. First and foremost, future studies should aim to expand the body of literature on this topic by conducting more research specifically focusing on the context-dependent and flexible nature of ER in the context of academic burnout and other psychopathologies. Taking into account the diversity of educational environments, curricula, and student demographics, these research initiatives should also include a wide range of academic contexts.
Furthermore, it is advisable for researchers to implement a uniform methodology for assessing and documenting ER strategies. This consistency in measurement will simplify the process of comparing results among different studies, bolster the reliability of the data, and pave the way for more extensive and comprehensive meta-analyses.
Insufficient research that delves into the connection between burnout and particular emotional regulation (ER) strategies, such as reappraisal or suppression, has made it unfeasible to conduct a meaningful analysis within the scope of the current meta-analysis, that could further bring specificity as to which ER strategies could influence or be affected in the context of academic burnout. Consequentially, the expansion of the inclusion criteria for future meta-analyses should be considered, along with the replication of the current meta-analysis in the context of future publications on this topic.
Future interventions aimed at addressing academic burnout should adopt a tailored approach that takes into consideration age or school-level influences, as well as gender differences. Implementing prevention programs in pre-university educational settings can play a pivotal role in equipping children and adolescents with vital emotion regulation skills and stress management strategies. Additionally, it is essential to provide additional support to girls, recognizing their unique stressors and increased academic expectations.
Implications
Our meta-analysis has several implications, both theoretical and practical. Firstly, the meta-analysis extends the understanding of the relationship between emotion regulation (ER) strategies and student burnout dimensions. Although the correlational and cross-sectional nature of the included studies does not allow for drawing causal implications, the results represent a great stepping stone for future research. Secondly, the results highlight the intricacy of ER strategies and their applicability in educational contexts. Along with the identified differences between preuniversity and university students, this emphasizes the importance of developmental and contextual factors in ER research and the necessity of having an elaborate understanding of the ways in which these strategies are used in various situations and according to individual particularities. The significant impact of the percentage of female participants on the relationship between ER strategies and academic burnout points to the need for gender-sensitive approaches in ER research. On a practical level, our results suggest the need for targeted interventions aimed at the specific needs of different educational levels and age groups, as well as gender-specific strategies to address ER difficulties.
In conclusion, the findings of the current meta-analysis reveal that adaptive ER strategies are associated with overall burnout, while ER difficulties are linked to both overall burnout and its constituent dimensions, including emotional exhaustion, cynicism, and lack of efficacy. These results align with prior research in the domain of psychopathology, suggesting that adaptive ER strategies may be more efficient in preventing psychopathology than ER difficulties are in creating vulnerability to it, or that academic burnout negatively influences the use of adaptive ER strategies in the youth population. As an alternative explanation, it might also be that the association between ER strategies, well-being, and burnout can vary based on the context, frequency, flexibility, and variability of their application. Furthermore, our study identified the moderating role of grade level and the sample’s gender composition in shaping these associations. The academic environment, parental influences, and self-efficacy may contribute to the observed differences between pre-university and university levels and age differences.
Despite some methodological limitations, the current meta-analysis underscores the need for context-dependent ER research and consistent measurement approaches in future investigations of academic burnout and psychopathology. The heterogeneity among studies may suggest variability in the relationship between emotion regulation and student burnout across different contexts. This variability could be explained through methodological differences, assessment methods, and other contextual factors that were not uniformly accounted for in the included studies. The included studies do not provide insights into changes over time as most studies were cross-sectional. Future research should aim to better understand the underlying reasons for the observed differences and to reach more conclusive insights through longitudinal research designs.
Overall, this meta-analysis contributes to a deeper understanding of the intricate relationship between ER strategies and student burnout and serves as a good reference point for future research within the academic burnout field.
Data Availability
The data that support the findings of this study are available from the corresponding author upon reasonable request.
Alarcon, G. M., Edwards, J. M., & Menke, L. E. (2011). Student Burnout and Engagement: A Test of the Conservation of Resources Theory. The Journal of Psychology, 145 (3), 211–227. https://doi.org/10.1080/00223980.2011.555432
Article Google Scholar
Aldao, A., & Nolen-Hoeksema, S. (2012a). The influence of context on the implementation of adaptive emotion regulation strategies. Behaviour Research and Therapy, 50 (7), 493–501. https://doi.org/10.1016/j.brat.2012.04.004
Aldao, A., & Nolen-Hoeksema, S. (2012b). When are adaptive strategies most predictive of psychopathology? Journal of Abnormal Psychology, 121 (1), 276–281. https://doi.org/10.1037/a0023598
Aldao, A., Nolen-Hoeksema, S., & Schweizer, S. (2010). Emotion-regulation strategies across psychopathology: A meta-analytic review. Clinical Psychology Review, 30 (2), 217–237. https://doi.org/10.1016/j.cpr.2009.11.004
Almutairi, H., Alsubaiei, A., Abduljawad, S., Alshatti, A., Fekih-Romdhane, F., Husni, M., & Jahrami, H. (2022). Prevalence of burnout in medical students: A systematic review and meta-analysis. International Journal of Social Psychiatry, 68 (6), 1157–1170. https://doi.org/10.1177/00207640221106691
Aloe, A. M., Amo, L. C., & Shanahan, M. E. (2014). Classroom Management Self-Efficacy and Burnout: A Multivariate Meta-analysis. Educational Psychology Review, 26 (1), 101–126. https://doi.org/10.1007/s10648-013-9244-0
Arias-Gundín, O. (Olga), & Vizoso-Gómez, C. (Carmen). (2018). Relación entre estrategias activas de afrontamiento, burnout y engagement en futuros educadores . https://doi.org/10.15581/004.35.409-427
Asareh, N., Pirani, Z., & Zanganeh, F. (2022). Evaluating the effectiveness of self-help cognitive and emotion regulation training On the psychological capital and academic motivation of female students with anxiety. Journal of School Psychology, 11 (2), 96–110. https://doi.org/10.22098/jsp.2022.1702
Balzarotti, S., John, O. P., & Gross, J. J. (2010). An Italian Adaptation of the Emotion Regulation Questionnaire. European Journal of Psychological Assessment, 26 (1), 61–67. https://doi.org/10.1027/1015-5759/a000009
Beck, A. T. (1976). Cognitive therapy and the emotional disorders. International Universities Press.
Bedewy, D., & Gabriel, A. (2015). Examining perceptions of academic stress and its sources among university students: The Perception of Academic Stress Scale. Health Psychology Open, 2 (2), 205510291559671. https://doi.org/10.1177/2055102915596714
Belaineh, M. S. (2017). Students’ Conception of Learning Environment and Their Approach to Learning and Its Implication on Quality Education. Educational Research and Reviews, 12 (14), 695–703.
Boada-Grau, J., Merino-Tejedor, E., Sánchez-García, J.-C., Prizmic-Kuzmica, A.-J., & Vigil-Colet, A. (2015). Adaptation and psychometric properties of the SBI-U scale for Academic Burnout in university students. Anales de Psicología / Annals of Psychology, 31 (1). https://doi.org/10.6018/analesps.31.1.168581
Borenstein, M., Higgins, J., Hedges, L., & Rothstein, H. (2017). Basics of meta-analysis: I(2) is not an absolute measure of heterogeneity. Research synthesis methods, 8. https://doi.org/10.1002/jrsm.1230
Bouffard-Bouchard, T., Parent, S., & Larivee, S. (1991). Influence of Self-Efficacy on Self-Regulation and Performance among Junior and Senior High-School Age Students. International Journal of Behavioral Development, 14 (2), 153–164. https://doi.org/10.1177/016502549101400203
Bresó, E., Schaufeli, W. B., & Salanova, M. (2011). Can a self-efficacy-based intervention decrease burnout, increase engagement, and enhance performance? A Quasi-Experimental Study. Higher Education, 61 (4), 339–355. https://doi.org/10.1007/s10734-010-9334-6
Burić, I., Sorić, I., & Penezić, Z. (2016). Emotion regulation in academic domain: Development and validation of the academic emotion regulation questionnaire (AERQ). Personality and Individual Differences, 96 , 138–147. https://doi.org/10.1016/j.paid.2016.02.074
Campbell-Sills, L., Barlow, D. H., Brown, T. A., & Hofmann, S. G. (2006a). Effects of suppression and acceptance on emotional responses of individuals with anxiety and mood disorders. Behaviour Research and Therapy, 44 (9), 1251–1263. https://doi.org/10.1016/j.brat.2005.10.001
Campbell-Sills, L., Barlow, D. H., Brown, T. A., & Hofmann, S. G. (2006b). Acceptability and suppression of negative emotion in anxiety and mood disorders. Emotion, 6 (4), 587–595. https://doi.org/10.1037/1528-3542.6.4.587
Carver, C. S. (1997). You want to measure coping but your protocol’ too long: Consider the brief cope. International Journal of Behavioral Medicine, 4 (1), 92–100. https://doi.org/10.1207/s15327558ijbm0401_6
Carver, C. S., Scheier, M. F., & Weintraub, J. K. (1989). Assessing coping strategies: A theoretically based approach. Journal of Personality and Social Psychology, 56 (2), 267–283. https://doi.org/10.1037/0022-3514.56.2.267
Cattelino, E., Morelli, M., Baiocco, R., & Chirumbolo, A. (2019). From external regulation to school achievement: The mediation of self-efficacy at school. Journal of Applied Developmental Psychology, 60 , 127–133. https://doi.org/10.1016/j.appdev.2018.09.007
Chacón-Cuberos, R., Martínez-Martínez, A., García-Garnica, M., Pistón-Rodríguez, M. D., & Expósito-López, J. (2019). The Relationship between Emotional Regulation and School Burnout: Structural Equation Model According to Dedication to Tutoring. International Journal of Environmental Research and Public Health, 16 (23), 4703. https://doi.org/10.3390/ijerph16234703
Charbonnier, E., Trémolière, B., Baussard, L., Goncalves, A., Lespiau, F., Philippe, A. G., & Le Vigouroux, S. (2022). Effects of an online self-help intervention on university students’ mental health during COVID-19: A non-randomized controlled pilot study. Computers in Human Behavior Reports, 5 , 100175. https://doi.org/10.1016/j.chbr.2022.100175
Chen, S., Zheng, Q., Pan, J., & Zheng, S. (2000). Preliminary development of the Coping Style Scale for Middle School Students. Chinese Journal of Clinical Psychology, 8 , 211–214, 237.
Córdova Olivera, P., Gasser Gordillo, P., Naranjo Mejía, H., La Fuente Taborga, I., Grajeda Chacón, A., & Sanjinés Unzueta, A. (2023). Academic stress as a predictor of mental health in university students. Cogent Education, 10 (2), 2232686. https://doi.org/10.1080/2331186X.2023.2232686
Davis, E. L., & Levine, L. J. (2013). Emotion Regulation Strategies That Promote Learning: Reappraisal Enhances Children’s Memory for Educational Information: Reappraisal and Memory in Children. Child Development, 84 (1), 361–374. https://doi.org/10.1111/j.1467-8624.2012.01836.x
Dawel, A., Shou, Y., Gulliver, A., Cherbuin, N., Banfield, M., Murray, K., Calear, A. L., Morse, A. R., Farrer, L. M., & Smithson, M. (2021). Cause or symptom? A longitudinal test of bidirectional relationships between emotion regulation strategies and mental health symptoms. Emotion, 21 (7), 1511–1521. https://doi.org/10.1037/emo0001018
Deb, S., Strodl, E., & Sun, H. (2015). Academic stress, parental pressure, anxiety and mental health among Indian high school students. International Journal of Psychology and Behavioral Science, 5 (1), 1.
Google Scholar
Deeks, J. J., Bossuyt, P. M., Leeflang, M. M., & Takwoingi, Y. (2023). Cochrane Handbook for Systematic Reviews of Diagnostic Test Accuracy . John Wiley & Sons.
Book Google Scholar
Dixon-Gordon, K. L., Chapman, A. L., Lovasz, N., & Walters, K. (2011). Too upset to think: The interplay of borderline personality features, negative emotions, and social problem solving in the laboratory. Personality Disorders: Theory, Research, and Treatment, 2 (4), 243–260. https://doi.org/10.1037/a0021799
Dominguez-Lara, S. A. (2018). Agotamiento emocional académico en estudiantes universitarios: ¿cuánto influyen las estrategias cognitivas de regulación emocional? Educación Médica, 19 (2), 96–103. https://doi.org/10.1016/j.edumed.2016.11.010
Duval, S., & Tweedie, R. (2000). Trim and fill: A simple funnel-plot-based method of testing and adjusting for publication bias in meta-analysis. Biometrics, 56 (2), 455–463. https://doi.org/10.1111/j.0006-341x.2000.00455.x
Ehring, T., Tuschen-Caffier, B., Schnülle, J., Fischer, S., & Gross, J. J. (2010). Emotion regulation and vulnerability to depression: Spontaneous versus instructed use of emotion suppression and reappraisal. Emotion, 10 (4), 563–572. https://doi.org/10.1037/a0019010
Ezeudu, F. O., Attah, F. O., Onah, A. E., Nwangwu, T. L., & Nnadi, E. M. (2020). Intervention for burnout among postgraduate chemistry education students. Journal of International Medical Research, 48 (1), 0300060519866279. https://doi.org/10.1177/0300060519866279
Fong, M., & Loi, N. M. (2016). The Mediating Role of Self-compassion in Student Psychological Health. Australian Psychologist, 51 (6), 431–441. https://doi.org/10.1111/ap.12185
Frajerman, A., Morvan, Y., Krebs, M.-O., Gorwood, P., & Chaumette, B. (2019). Burnout in medical students before residency: A systematic review and meta-analysis. European Psychiatry: The Journal of the Association of European Psychiatrists, 55 , 36–42. https://doi.org/10.1016/j.eurpsy.2018.08.006
Garnefski, N., Kraaij, V., & Spinhoven, P. (2001). Negative life events, cognitive emotion regulation and emotional problems. Personality and Individual Differences, 30 (8), 1311–1327. https://doi.org/10.1016/S0191-8869(00)00113-6
Goldin, P. R., McRae, K., Ramel, W., & Gross, J. J. (2008). The neural bases of emotion regulation: Reappraisal and suppression of negative emotion. Biological Psychiatry, 63 (6), 577–586. https://doi.org/10.1016/j.biopsych.2007.05.031
Grech, M. (2021). The Effect of the Educational Environment on the rate of Burnout among Postgraduate Medical Trainees – A Narrative Literature Review. Journal of Medical Education and Curricular Development, 8 , 23821205211018700. https://doi.org/10.1177/23821205211018700
Gross, J. J. (1998a). The emerging field of emotion regulation: An integrative review. Review of General Psychology, 2 (3), 271–299. https://doi.org/10.1037/1089-2680.2.3.271
Gross, J. J. (1998b). Antecedent- and response-focused emotion regulation: Divergent consequences for experience, expression, and physiology. Journal of Personality and Social Psychology, 74 (1), 224–237. https://doi.org/10.1037/0022-3514.74.1.224
Gross, J. J. (2013). Emotion regulation: Taking stock and moving forward. Emotion, 13 (3), 359–365. https://doi.org/10.1037/a0032135
Gross, J. J. (2015). Emotion regulation: Current status and future prospects. Psychological Inquiry, 26 (1), 1–26. https://doi.org/10.1080/1047840X.2014.940781
Gross, J. J., & John, O. P. (2003). Individual differences in two emotion regulation processes: Implications for affect, relationships, and well-being. Journal of Personality and Social Psychology, 85 (2), 348–362. https://doi.org/10.1037/0022-3514.85.2.348
Gross, J. J., & Levenson, R. W. (1993). Emotional suppression: Physiology, self-report, and expressive behavior. Journal of Personality and Social Psychology, 64 (6), 970–986. https://doi.org/10.1037/0022-3514.64.6.970
Gruber, J., Harvey, A. G., & Gross, J. J. (2012). When trying is not enough: Emotion regulation and the effort–success gap in bipolar disorder. Emotion, 12 (5), 997–1003. https://doi.org/10.1037/a0026822
Guessoum, S. B., Lachal, J., Radjack, R., Carretier, E., Minassian, S., Benoit, L., & Moro, M. R. (2020). Adolescent psychiatric disorders during the COVID-19 pandemic and lockdown. Psychiatry Research, 291 , 113264. https://doi.org/10.1016/j.psychres.2020.113264
Harrer, M., Cuijpers, P., Furukawa, T. A., & Ebert, D. D. (2022). Doing meta-analysis with R: A hands-on guide (First edition). CRC Press.
Hayes, S. C., Strosahl, K. D., & Wilson, K. G. (1999). Acceptance and commitment therapy: An experiential approach to behavior change (pp. xvi, 304). Guilford Press.
Herrmann, J., Koeppen, K., & Kessels, U. (2019). Do girls take school too seriously? Investigating gender differences in school burnout from a self-worth perspective. Learning and Individual Differences, 69 , 150–161. https://doi.org/10.1016/j.lindif.2018.11.011
Higgins, J. P. T., & Green, S. (Eds.) (2011.). Cochrane handbook for systematic reviews of interventions Version 5.1.0 [updated March 2011]. The Cochrane Collaboration. Retrieved May 13, 2024 from www.handbook.cochrane.org .
Hofmann, S. G., & Asmundson, G. J. G. (2008). Acceptance and mindfulness-based therapy: New wave or old hat? Clinical Psychology Review, 28 (1), 1–16. https://doi.org/10.1016/j.cpr.2007.09.003
Hystad, S. W., Eid, J., Laberg, J. C., Johnsen, B. H., & Bartone, P. T. (2009). Academic Stress and Health: Exploring the Moderating Role of Personality Hardiness. Scandinavian Journal of Educational Research, 53 (5), 421–429. https://doi.org/10.1080/00313830903180349
Ibda, H., Wulandari, T. S., Abdillah, A., Hastuti, A. P., & Mahsun, M. (2023). Student academic stress during the COVID-19 pandemic: A systematic literature review. International Journal of Public Health Science (IJPHS), 12 (1), 286. https://doi.org/10.11591/ijphs.v12i1.21983
Jiang, S., Ren, Q., Jiang, C., & Wang, L. (2021). Academic stress and depression of Chinese adolescents in junior high schools: Moderated mediation model of school burnout and self-esteem. Journal of Affective Disorders, 295 , 384–389. https://doi.org/10.1016/j.jad.2021.08.085
Junyan, F., & Minqiang, Z. (2020). What is the minimum number of effect sizes required in meta-regression? An estimation based on statistical power and estimation precision. Advances in Psychological Science, 28 (4), 673. https://doi.org/10.3724/SP.J.1042.2020.00673
Kim, B., Jee, S., Lee, J., An, S., & Lee, S. M. (2018). Relationships between social support and student burnout: A meta-analytic approach. Stress and Health, 34 (1), 127–134. https://doi.org/10.1002/smi.2771
Kim, S., Kim, H., Park, E. H., Kim, B., Lee, S. M., & Kim, B. (2021). Applying the demand–control–support model on burnout in students: A meta-analysis. Psychology in the Schools, 58 (11), 2130–2147. https://doi.org/10.1002/pits.22581
Kmet, Leanne M. ; Cook, Linda S. ; Lee, Robert C. (2004). Standard Quality Assessment Criteria for Evaluating Primary Research Papers from a Variety of Fields . https://doi.org/10.7939/R37M04F16
Kobylińska, D., & Kusev, P. (2019). Flexible Emotion Regulation: How Situational Demands and Individual Differences Influence the Effectiveness of Regulatory Strategies. Frontiers in Psychology , 10 . https://doi.org/10.3389/fpsyg.2019.00072
Koole, S. L. (2009). The psychology of emotion regulation: An integrative review. Cognition and Emotion, 23 (1), 4–41. https://doi.org/10.1080/02699930802619031
Kristensen, T. S., Borritz, M., Villadsen, E., & Christensen, K. B. (2005). The copenhagen burnout inventory: A new tool for the assessment of burnout. Work & Stress, 19 (3), 192–207. https://doi.org/10.1080/02678370500297720
Larsen, R. J. (2000). Toward a science of mood regulation. Psychological Inquiry, 11 (3), 129–141. https://doi.org/10.1207/S15327965PLI1103_01
Lau, S. C., Chow, H. J., Wong, S. C., & Lim, C. S. (2020). An empirical study of the influence of individual-related factors on undergraduates’ academic burnout: Malaysian context. Journal of Applied Research in Higher Education, 13 (4), 1181–1197. https://doi.org/10.1108/JARHE-02-2020-0037
Leppanen, J., Brown, D., McLinden, H., Williams, S., & Tchanturia, K. (2022). The Role of Emotion Regulation in Eating Disorders: A Network Meta-Analysis Approach. Frontiers in Psychiatry, 13. https://doi.org/10.3389/fpsyt.2022.793094
Libert, C., Chabrol, H., & Laconi, S. (2019). Exploration du burn-out et du surengagement académique dans un échantillon d’étudiants. Journal De Thérapie Comportementale Et Cognitive, 29 (3), 119–131. https://doi.org/10.1016/j.jtcc.2019.01.001
Lin, F., & Yang, K. (2021). The External and Internal Factors of Academic Burnout: 2021 4th International Conference on Humanities Education and Social Sciences (ICHESS 2021), Xishuangbanna, China. https://doi.org/10.2991/assehr.k.211220.307
Linehan, M. M. (1993). Cognitive-behavioral treatment of borderline personality disorder (pp. xvii, 558). Guilford Press.
Lo, H. H. M., Ngai, S., & Yam, K. (2021). Effects of Mindfulness-Based Stress Reduction on Health and Social Care Education: A Cohort-Controlled Study. Mindfulness, 12 (8), 2050–2058. https://doi.org/10.1007/s12671-021-01663-z
Luszczynska, A., Diehl, M., Gutiérrez-Doña, B., Kuusinen, P., & Schwarzer, R. (2004). Measuring one component of dispositional self-regulation: Attention control in goal pursuit. Personality and Individual Differences, 37 (3), 555–566. https://doi.org/10.1016/j.paid.2003.09.026
Luo, Y., Wang, Z., Zhang, H., Chen, A., & Quan, S. (2016). The effect of perfectionism on school burnout among adolescence: The mediator of self-esteem and coping style. Personality and Individual Differences, 88 , 202–208. https://doi.org/10.1016/j.paid.2015.08.056
Luo, Y., Deng, Y., & Zhang, H. (2020). The influences of parental emotional warmth on the association between perceived teacher–student relationships and academic stress among middle school students in China. Children and Youth Services Review, 114 , 105014. https://doi.org/10.1016/j.childyouth.2020.105014
Lynch, T. R., Trost, W. T., Salsman, N., & Linehan, M. M. (2007). Dialectical behavior therapy for borderline personality disorder. Annual Review of Clinical Psychology, 3 , 181–205. https://doi.org/10.1146/annurev.clinpsy.2.022305.095229
Madigan, D. J., & Curran, T. (2021). Does burnout affect academic achievement? A meta-analysis of over 100,000 students. Educational Psychology Review, 33 (2), 387–405. https://doi.org/10.1007/s10648-020-09533-1
Madigan, D. J., Kim, L. E., & Glandorf, H. L. (2023). Interventions to reduce burnout in students: A systematic review and meta-analysis. European Journal of Psychology of Education . https://doi.org/10.1007/s10212-023-00731-3
Maeda, Y., & Harwell, M. (2016). Guidelines for using the Q Test in Meta-Analysis. Mid-Western Educational Researcher, 28 (1). Retrieved May 22, 2024, from https://scholarworks.bgsu.edu/mwer/vol28/iss1/4
Marques, H., Brites, R., Nunes, O., Hipólito, J., & Brandão, T. (2023). Attachment, emotion regulation, and burnout among university students: A mediational hypothesis. Educational Psychology, 43 (4), 344–362. https://doi.org/10.1080/01443410.2023.2212889
Matud, M. P., Díaz, A., Bethencourt, J. M., & Ibáñez, I. (2020). Stress and Psychological Distress in Emerging Adulthood: A Gender Analysis. Journal of Clinical Medicine, 9 (9), 2859. https://doi.org/10.3390/jcm9092859
May, R. W., Bauer, K. N., & Fincham, F. D. (2015). School burnout: Diminished academic and cognitive performance. Learning and Individual Differences, 42 , 126–131. https://doi.org/10.1016/j.lindif.2015.07.015
Mennin, D. S., Holaway, R. M., Fresco, D. M., Moore, M. T., & Heimberg, R. G. (2007). Delineating components of emotion and its dysregulation in anxiety and mood psychopathology. Behavior Therapy, 38 (3), 284–302. https://doi.org/10.1016/j.beth.2006.09.001
Merino-Tejedor, E., Hontangas, P. M., & Boada-Grau, J. (2016). Career adaptability and its relation to self-regulation, career construction, and academic engagement among Spanish university students. Journal of Vocational Behavior, 93 , 92–102. https://doi.org/10.1016/j.jvb.2016.01.005
Meylan, N., Doudin, P.-A., Curchod-Ruedi, D., & Stephan, P. (2015). Burnout scolaire et soutien social: L’importance du soutien des parents et des enseignants. Psychologie Française, 60 (1), 1–15. https://doi.org/10.1016/j.psfr.2014.01.003
Mickenautsch, S., Yengopal, V., Mickenautsch, S., & Yengopal, V. (2024). Trial Number and Sample Size Do Not Affect the Accuracy of the I2-Point Estimate for Testing Selection Bias Risk in Meta-Analyses. Cureus, 16 , 4. https://doi.org/10.7759/cureus.58961
Midgley, C., Maehr, M., Hruda, L., Anderman, E., Anderman, L., Freeman, K., Gheen, M., Kaplan, A., Kumar, R., Middleton, M., Nelson, J., Roeser, R., & Urdan, T. (2000). The patterns of adaptive learning scales (PALS) 2000 [Dataset].
Miola, A., Cattarinussi, G., Antiga, G., Caiolo, S., Solmi, M., & Sambataro, F. (2022). Difficulties in emotion regulation in bipolar disorder: A systematic review and meta-analysis. Journal of Affective Disorders, 302 , 352–360. https://doi.org/10.1016/j.jad.2022.01.102
Miu, A. C., Szentágotai-Tătar, A., Balázsi, R., Nechita, D., Bunea, I., & Pollak, S. D. (2022). Emotion regulation as mediator between childhood adversity and psychopathology: A meta-analysis. Clinical Psychology Review, 93 , 102141. https://doi.org/10.1016/j.cpr.2022.102141
Modrego-Alarcón, M., López-Del-Hoyo, Y., García-Campayo, J., Pérez-Aranda, A., Navarro-Gil, M., Beltrán-Ruiz, M., Morillo, H., Delgado-Suarez, I., Oliván-Arévalo, R., & Montero-Marin, J. (2021). Efficacy of a mindfulness-based programme with and without virtual reality support to reduce stress in university students: A randomized controlled trial. Behaviour Research and Therapy, 142 , 103866. https://doi.org/10.1016/j.brat.2021.103866
Mohammadi Bytamar, J., Saed, O., & Khakpoor, S. (2020). Emotion Regulation Difficulties and Academic Procrastination. Frontiers in Psychology, 11 , 524588. https://doi.org/10.3389/fpsyg.2020.524588
Moher, D., Liberati, A., Tetzlaff, J., & Altman, D. G. (2009). Preferred reporting items for systematic reviews and meta-analyses: The PRISMA statement. BMJ, 339 , b2535. https://doi.org/10.1136/bmj.b2535
Muchacka-Cymerman, A., & Tomaszek, K. (2018). Polish Adaptation of the ESSBS School-Burnout Scale: Pilot Study Results. Hacettepe University Journal of Education , 1–16. https://doi.org/10.16986/HUJE.2018043462
Naderi, Z., Bakhtiari, S., Momennasab, M., Abootalebi, M., & Mirzaei, T. (2018). Prediction of academic burnout and academic performance based on the need for cognition and general self-efficacy: A cross-sectional analytical study. Revista Latinoamericana De Hipertensión, 13 (6), 584–591.
Narimanj, A., Kazemi, R., & Narimani, M. (2021). Relationship between Cognitive Emotion Regulation, Personal Intelligence and Academic Burnout. Journal of Modern Psychological Researches, 16 (61), 65–74.
Neacsiu, A. D., Rizvi, S. L., & Linehan, M. M. (2010). Dialectical behavior therapy skills use as a mediator and outcome of treatment for borderline personality disorder. Behaviour Research and Therapy, 48 (9), 832–839. https://doi.org/10.1016/j.brat.2010.05.017
Neff, K. D. (2003). The development and validation of a scale to measure self-compassion. Self and Identity, 2 (3), 223–250. https://doi.org/10.1080/15298860309027
Nikdel, F., Hadi, J., & Ali, T. (2019). SOCIAL SCIENCES & HUMANITIES Students’ Academic Stress, Stress Response and Academic Burnout: Mediating Role of Self-Efficacy .
Noh, H., Chang, E., Jang, Y., Lee, J. H., & Lee, S. M. (2016). Suppressor Effects of Positive and Negative Religious Coping on Academic Burnout Among Korean Middle School Students. Journal of Religion and Health, 55 (1), 135–146. https://doi.org/10.1007/s10943-015-0007-8
Nolen-Hoeksema, S., Wisco, B. E., & Lyubomirsky, S. (2008). Rethinking Rumination. Perspectives on Psychological Science, 3 (5), 400–424. https://doi.org/10.1111/j.1745-6924.2008.00088.x
Nyklícek, I., & Temoshok, L. (2004). Emotional expression and health: Advances in theory, assessment and clinical applications . Routledge.
Ogbuanya, T. C., Eseadi, C., Orji, C. T., Omeje, J. C., Anyanwu, J. I., Ugwoke, S. C., & Edeh, N. C. (2019). Effect of Rational-Emotive Behavior Therapy Program on the Symptoms of Burnout Syndrome Among Undergraduate Electronics Work Students in Nigeria. Psychological Reports, 122 (1), 4–22. https://doi.org/10.1177/0033294117748587
Östberg, V., Almquist, Y. B., Folkesson, L., Låftman, S. B., Modin, B., & Lindfors, P. (2015). The Complexity of Stress in Mid-Adolescent Girls and Boys. Child Indicators Research, 8 (2), 403–423. https://doi.org/10.1007/s12187-014-9245-7
Park, E.-Y., & Shin, M. (2020). A Meta-Analysis of Special Education Teachers’ Burnout. SAGE Open, 10 (2), 2158244020918297. https://doi.org/10.1177/2158244020918297
Parkinson, B., & Totterdell, P. (1999). Classifying affect-regulation strategies. Cognition and Emotion, 13 (3), 277–303. https://doi.org/10.1080/026999399379285
Pines, A., & Aronson, E. (1988). Career Burnout: Causes and Cures . Free Press.
Popescu, B., Maricuțoiu, L. P., & De Witte, H. (2023). The student version of the Burnout assessement tool (BAT): Psychometric properties and evidence regarding measurement validity on a romanian sample. Current Psychology . https://doi.org/10.1007/s12144-023-04232-w
Prefit, A.-B., Cândea, D. M., & Szentagotai-Tătar, A. (2019). Emotion regulation across eating pathology: A meta-analysis. Appetite, 143 , 104438. https://doi.org/10.1016/j.appet.2019.104438
Prospero. (2022). Systematic review registration: Emotion regulation and academic burnout in youths: a meta-analysis. Retrieved May 22, 2024, from https://www.crd.york.ac.uk/prospero/display_record.php?RecordID=325570
Ramírez, M. T. G., & Hernández, R. L. (2007). ESCALA DE CANSANCIO EMOCIONAL (ECE) PARA ESTUDIANTES UNIVERSITARIOS: PROPIEDADES PSICOMÉTRICAS EN UNA MUESTRA DE MÉXICO. Anales de Psicología / Annals of Psychology, 23 (2).
Richards, J. M., & Gross, J. J. (2000). Emotion regulation and memory: The cognitive costs of keeping one’s cool. Journal of Personality and Social Psychology, 79 (3), 410–424. https://doi.org/10.1037/0022-3514.79.3.410
Richards, J. M., Butler, E. A., & Gross, J. J. (2003). Emotion regulation in romantic relationships: The cognitive consequences of concealing feelings. Journal of Social and Personal Relationships, 20 (5), 599–620. https://doi.org/10.1177/02654075030205002
Roemer, L., Orsillo, S. M., & Salters-Pedneault, K. (2008). Efficacy of an acceptance-based behavior therapy for generalized anxiety disorder: Evaluation in a randomized controlled trial. Journal of Consulting and Clinical Psychology, 76 (6), 1083–1089. https://doi.org/10.1037/a0012720
Salmela-Aro, K. (2017). Dark and bright sides of thriving – school burnout and engagement in the Finnish context. European Journal of Developmental Psychology, 14 (3), 337–349. https://doi.org/10.1080/17405629.2016.1207517
Salmela-Aro, K., & Tynkkynen, L. (2012). Gendered pathways in school burnout among adolescents. Journal of Adolescence, 35 (4), 929–939. https://doi.org/10.1016/j.adolescence.2012.01.001
Salmela-aro *, K., Näätänen, P., & Nurmi, J. (2004). The role of work-related personal projects during two burnout interventions: A longitudinal study. Work & Stress, 18(3), 208–230. https://doi.org/10.1080/02678370412331317480
Salmela-Aro, K., Kiuru, N., Leskinen, E., & Nurmi, J.-E. (2009). School burnout inventory (SBI). European Journal of Psychological Assessment, 25 (1), 48–57. https://doi.org/10.1027/1015-5759.25.1.48
Santos Alves Peixoto, L., Guedes Gondim, S. M., & Pereira, C. R. (2022). Emotion Regulation, Stress, and Well-Being in Academic Education: Analyzing the Effect of Mindfulness-Based Intervention. Trends in Psychology, 30 (1), 33–57. https://doi.org/10.1007/s43076-021-00092-0
Scales, P. C., Benson, P. L., Oesterle, S., Hill, K. G., Hawkins, J. D., & Pashak, T. J. (2016). The dimensions of successful young adult development: A conceptual and measurement framework. Applied Developmental Science, 20 (3), 150–174. https://doi.org/10.1080/10888691.2015.1082429
Schaufeli, W. B., Salanova, M., González-romá, V., & Bakker, A. B. (2002). The measurement of engagement and burnout: A two sample confirmatory factor analytic approach. Journal of Happiness Studies, 3 (1), 71–92. https://doi.org/10.1023/A:1015630930326
Schaufeli, W. B., Desart, S., & De Witte, H. (2020). Burnout assessment tool (BAT)—development, validity, and reliability. International Journal of Environmental Research and Public Health, 17 (24). https://doi.org/10.3390/ijerph17249495
Schmid, C. H., Stijnen, T., & White, I. (2020). Handbook of Meta-Analysis . CRC Press.
Segal, Z. V., Williams, J. M. G., & Teasdale, J. D. (2002). Mindfulness-based cognitive therapy for depression: A new approach to preventing relapse (pp. xiv, 351). Guilford Press.
Séguin, D. G., & MacDonald, B. (2018). The role of emotion regulation and temperament in the prediction of the quality of social relationships in early childhood. Early Child Development and Care, 188 (8), 1147–1163. https://doi.org/10.1080/03004430.2016.1251678
Seibert, G. S., Bauer, K. N., May, R. W., & Fincham, F. D. (2017). Emotion regulation and academic underperformance: The role of school burnout. Learning and Individual Differences, 60 , 1–9. https://doi.org/10.1016/j.lindif.2017.10.001
Shahidi, S., Akbari, H., & Zargar, F. (2017). Effectiveness of mindfulness-based stress reduction on emotion regulation and test anxiety in female high school students. Journal of Education and Health Promotion, 6 , 87. https://doi.org/10.4103/jehp.jehp_98_16
Shih, S.-S. (2013). The effects of autonomy support versus psychological control and work engagement versus academic burnout on adolescents’ use of avoidance strategies. School Psychology International, 34 (3), 330–347. https://doi.org/10.1177/0143034312466423
Shih, S.-S. (2015a). An Examination of Academic Coping Among Taiwanese Adolescents. The Journal of Educational Research, 108 (3), 175–185. https://doi.org/10.1080/00220671.2013.867473
Shih, S.-S. (2015b). The relationships among Taiwanese adolescents’ perceived classroom environment, academic coping, and burnout. School Psychology Quarterly: The Official Journal of the Division of School Psychology, American Psychological Association, 30 (2), 307–320. https://doi.org/10.1037/spq0000093
Stellern, J., Xiao, K. B., Grennell, E., Sanches, M., Gowin, J. L., & Sloan, M. E. (2023). Emotion regulation in substance use disorders: A systematic review and meta-analysis. Addiction, 118 (1), 30–47. https://doi.org/10.1111/add.16001
Tobin, D. L., Holroyd, K. A., Reynolds, R. V., & Wigal, J. K. (1989). The hierarchical factor structure of the Coping Strategies Inventory. Cognitive Therapy and Research, 13 (4), 343–361. https://doi.org/10.1007/BF01173478
Troy, A. S., Shallcross, A. J., & Mauss, I. B. (2013). A Person-by-Situation Approach to Emotion Regulation: Cognitive Reappraisal Can Either Help or Hurt. Depending on the Context. Psychological Science, 24 (12), 2505–2514. https://doi.org/10.1177/0956797613496434
Tull, M. T., & Aldao, A. (2015). Editorial overview: New directions in the science of emotion regulation. Current Opinion in Psychology, 3 , iv–x. https://doi.org/10.1016/j.copsyc.2015.03.009
Vinter, K., Aus, K., & Arro, G. (2021). Adolescent girls’ and boys’ academic burnout and its associations with cognitive emotion regulation strategies. Educational Psychology, 41 (8), 1061–1077. https://doi.org/10.1080/01443410.2020.1855631
Vizoso, C., Arias-Gundín, O., & Rodríguez, C. (2019). Exploring coping and optimism as predictors of academic burnout and performance among university students. Educational Psychology, 39 (6), 768–783. https://doi.org/10.1080/01443410.2018.1545996
von Hippel, P. T. (2015). The heterogeneity statistic I(2) can be biased in small meta-analyses. BMC Medical Research Methodology, 15 , 35. https://doi.org/10.1186/s12874-015-0024-z
Walburg, V. (2014). Burnout among high school students: A literature review. Children and Youth Services Review, 42 , 28–33. https://doi.org/10.1016/j.childyouth.2014.03.020
Webb, T. L., Miles, E., & Sheeran, P. (2012). Dealing with feeling: A meta-analysis of the effectiveness of strategies derived from the process model of emotion regulation. Psychological Bulletin, 138 (4), 775–808. https://doi.org/10.1037/a0027600
Weiss, N. H., Kiefer, R., Goncharenko, S., Raudales, A. M., Forkus, S. R., Schick, M. R., & Contractor, A. A. (2022). Emotion regulation and substance use: A meta-analysis. Drug and Alcohol Dependence, 230 , 109131. https://doi.org/10.1016/j.drugalcdep.2021.109131
Westhues, A., & Cohen, J. S. (1997). A comparison of the adjustment of adolescent and young adult inter-country adoptees and their siblings. International Journal of Behavioral Development, 20 (1), 47–65. https://doi.org/10.1080/016502597385432
Wu, K., Wang, F., Wang, W., & Li, Y. (2022). Parents’ Education Anxiety and Children’s Academic Burnout: The Role of Parental Burnout and Family Function. Frontiers in Psychology , 12 . https://doi.org/10.3389/fpsyg.2021.764824
Yang, H., & Chen, J. (2016). Learning Perfectionism and Learning Burnout in a Primary School Student Sample: A Test of a Learning-Stress Mediation Model. Journal of Child and Family Studies, 25 (1), 345–353. https://doi.org/10.1007/s10826-015-0213-8
Yang, C., Chen, A., & Chen, Y. (2021). College students’ stress and health in the COVID-19 pandemic: The role of academic workload, separation from school, and fears of contagion. PLoS ONE, 16 (2), e0246676. https://doi.org/10.1371/journal.pone.0246676
Yildiz, M. A. (2017). Pathways to positivity from perceived stress in adolescents: Multiple mediation of emotion regulation and coping strategies. Current Issues in Personality Psychology, 5 (4), 272–284. https://doi.org/10.5114/cipp.2017.67894
Yu, X., Wang, Y., & Liu, F. (2022). Language learning motivation and burnout among english as a foreign language undergraduates: The moderating role of maladaptive emotion regulation strategies. Frontiers in Psychology , 13 . https://www.frontiersin.org/articles/10.3389/fpsyg.2022.808118
Zahniser, E., & Conley, C. S. (2018). Interactions of emotion regulation and perceived stress in predicting emerging adults’ subsequent internalizing symptoms. Motivation and Emotion, 42 (5), 763–773. https://doi.org/10.1007/s11031-018-9696-0
Download references
Acknowledgements
This work was supported by two grants awarded to the corresponding author from the Romanian National Authority for Scientific Research, CNCS—UEFISCDI (Grant number PN-III-P4-ID-PCE-2020-2170 and PN-III-P2-2.1-PED-2021-3882)
Author information
Authors and affiliations.
Evidence-Based Psychological Assessment and Interventions Doctoral School, Babes-Bolyai University of Cluj-Napoca, Cluj-Napoca, Napoca, Romania
Ioana Alexandra Iuga
DATA Lab, The International Institute for the Advanced Studies of Psychotherapy and Applied Mental Health, Babes-Bolyai University Cluj-Napoca, Cluj-Napoca, Romania
Ioana Alexandra Iuga & Oana Alexandra David
Department of Clinical Psychology and Psychotherapy, Babeş-Bolyai University, No 37 Republicii Street, 400015, Cluj-Napoca, Napoca, Romania
Oana Alexandra David
You can also search for this author in PubMed Google Scholar
Corresponding author
Correspondence to Oana Alexandra David .
Ethics declarations
Competing interests.
The authors declare that they have no known competing financial interests or personal relationships that influence the work reported in this paper.
Additional information
Publisher's note.
Springer Nature remains neutral with regard to jurisdictional claims in published maps and institutional affiliations.
Supplementary Information
Below is the link to the electronic supplementary material.
Supplementary file1 (DOCX 26534 KB)
Supplementary file2 (docx 221 kb), supplementary file3 (docx 315 kb), supplementary file4 (docx 16 kb), rights and permissions.
Open Access This article is licensed under a Creative Commons Attribution 4.0 International License, which permits use, sharing, adaptation, distribution and reproduction in any medium or format, as long as you give appropriate credit to the original author(s) and the source, provide a link to the Creative Commons licence, and indicate if changes were made. The images or other third party material in this article are included in the article's Creative Commons licence, unless indicated otherwise in a credit line to the material. If material is not included in the article's Creative Commons licence and your intended use is not permitted by statutory regulation or exceeds the permitted use, you will need to obtain permission directly from the copyright holder. To view a copy of this licence, visit http://creativecommons.org/licenses/by/4.0/ .
Reprints and permissions
About this article
Iuga, I.A., David, O.A. Emotion Regulation and Academic Burnout Among Youth: a Quantitative Meta-analysis. Educ Psychol Rev 36 , 106 (2024). https://doi.org/10.1007/s10648-024-09930-w
Download citation
Accepted : 01 August 2024
Published : 10 September 2024
DOI : https://doi.org/10.1007/s10648-024-09930-w
Share this article
Anyone you share the following link with will be able to read this content:
Sorry, a shareable link is not currently available for this article.
Provided by the Springer Nature SharedIt content-sharing initiative
- Emotion regulation
- Academic burnout
- Meta-analysis
- Find a journal
- Publish with us
- Track your research
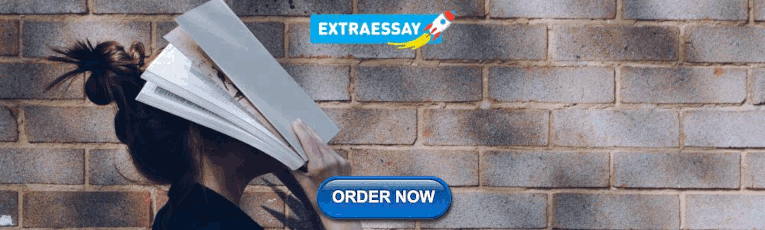
COMMENTS
Below are the steps to prepare a data before quantitative research analysis: Step 1: Data Collection. Before beginning the analysis process, you need data. Data can be collected through rigorous quantitative research, which includes methods such as interviews, focus groups, surveys, and questionnaires. Step 2: Data Cleaning.
The two "branches" of quantitative analysis. As I mentioned, quantitative analysis is powered by statistical analysis methods.There are two main "branches" of statistical methods that are used - descriptive statistics and inferential statistics.In your research, you might only use descriptive statistics, or you might use a mix of both, depending on what you're trying to figure out.
Here is a basic guide for gathering quantitative data: Define the research question: The first step in gathering quantitative data is to clearly define the research question. This will help determine the type of data to be collected, the sample size, and the methods of data analysis.
Revised on June 22, 2023. Quantitative research is the process of collecting and analyzing numerical data. It can be used to find patterns and averages, make predictions, test causal relationships, and generalize results to wider populations. Quantitative research is the opposite of qualitative research, which involves collecting and analyzing ...
It is important to know w hat kind of data you are planning to collect or analyse as this w ill. affect your analysis method. A 12 step approach to quantitative data analysis. Step 1: Start with ...
Although quantitative data analysis is a powerful tool, it cannot be used to provide context for your research, so this is where qualitative analysis comes in. Qualitative analysis is another common research method that focuses on collecting and analyzing non-numerical data, like text, images, or audio recordings to gain a deeper understanding ...
Data Analysis Methods in Quantitative Research. We started this module with levels of measurement as a way to categorize our data. Data analysis is directed toward answering the original research question and achieving the study purpose (or aim). Now, we are going to delve into two main statistical analyses to describe our data and make ...
Statistical analysis means investigating trends, patterns, and relationships using quantitative data. It is an important research tool used by scientists, governments, businesses, and other organizations. To draw valid conclusions, statistical analysis requires careful planning from the very start of the research process. You need to specify ...
Abstract. Quantitative data analysis serves as part of an essential process of evidence-making in health and social sciences. It is adopted for any types of research question and design whether it is descriptive, explanatory, or causal. However, compared with qualitative counterpart, quantitative data analysis has less flexibility.
8. Weight customer feedback. So far, the quantitative data analysis methods on this list have leveraged numeric data only. However, there are ways to turn qualitative data into quantifiable feedback and to mix and match data sources. For example, you might need to analyze user feedback from multiple surveys.
Replicable: Quantitative research aims to be replicable, meaning that other researchers should be able to conduct similar studies and obtain similar results using the same methods. Statistical analysis: Quantitative research involves using statistical tools and techniques to analyze the numerical data collected during the research process ...
Revised on 10 October 2022. Quantitative research is the process of collecting and analysing numerical data. It can be used to find patterns and averages, make predictions, test causal relationships, and generalise results to wider populations. Quantitative research is the opposite of qualitative research, which involves collecting and ...
Methods used for data analysis in qualitative research. There are several techniques to analyze the data in qualitative research, but here are some commonly used methods, Content Analysis: It is widely accepted and the most frequently employed technique for data analysis in research methodology. It can be used to analyze the documented ...
3.6 Quantitative Data Analysis Remember that quantitative research explains phenomena by collecting numerical data that are analysed using statistics. 1 Statistics is a scientific method of collecting, processing, analysing, presenting and interpreting data in numerical form. 44 This section discusses how quantitative data is analysed and the choice of test statistics based on the variables ...
Introduction. Statistical analysis is necessary for any research project seeking to make quantitative conclusions. The following is a primer for research-based statistical analysis. It is intended to be a high-level overview of appropriate statistical testing, while not diving too deep into any specific methodology.
Definition. Quantitative method is the collection and analysis of numerical data to answer scientific research questions. Quantitative method is used to summarize, average, find patterns, make predictions, and test causal associations as well as generalizing results to wider populations.
Here are some commonly used methods for analyzing qualitative data: Thematic Analysis: Identifies recurring themes or patterns in qualitative data by categorizing and coding the data. Content Analysis: Analyzes textual data systematically by categorizing and coding it to identify patterns and concepts.
Abstract. This chapter describes the types of data analysis techniques in quantitative research and sampling strategies suitable for quantitative studies, particularly probability sampling, to produce credible and trustworthy explanations of a phenomenon. Initially, it briefly describes the measurement levels of variables.
Data analysis techniques in research are categorized into qualitative and quantitative methods, each with its specific approaches and tools. These techniques are instrumental in extracting meaningful insights, patterns, and relationships from data to support informed decision-making, validate hypotheses, and derive actionable recommendations.
Quantitative data analysis may include the calculation of frequencies of variables and differences between variables. A quantitative approach is usually associated with finding evidence to either support or reject hypotheses you have formulated at the earlier stages of your research process. The same figure within data set can be interpreted in ...
The main difference between quantitative and qualitative research is the type of data they collect and analyze. Quantitative data is information about quantities, and therefore numbers, and qualitative data is descriptive, and regards phenomenon which can be observed but not measured, such as language. Quantitative research collects numerical ...
Quantitative data analysis is a systematic process of both collecting and evaluating measurable. and verifiable data. It contains a statistical mechanism of assessing or analyzing quantitative ...
Qualitative research is a type of research that aims to gather and analyse non-numerical (descriptive) data in order to gain an understanding of individuals' social reality, including understanding their attitudes, beliefs, and motivation. This type of research typically involves in-depth interviews, focus groups, or observations in order to collect data that is rich in detail and context.
Qualitative Data Analysis by Matthew B. Miles; A. Michael Huberman A practical sourcebook for researchers who make use of qualitative data, presenting the current state of the craft in the design, testing, and use of qualitative analysis methods. Strong emphasis is placed on data displays matrices and networks that go beyond ordinary narrative ...
Rather than presenting an exhaustive overview of the methods or explaining them in detail, the book serves as a starting point for developing data analysis skills: it provides hands-on guidelines for conducting the most common analyses and reporting results, and includes pointers to more extensive resources.
Qualitative methods seek to understand human behavior and the reasons for those behaviors. A lot of people are less familiar with qualitative research methods than they are with quantitative. But basically qualitative data and the methods that generate them are seeking answers for why people do the things that they do and how they do them.
The objective to which this manuscript is oriented to is focused on the analysis of interpersonal communication in sport. The multimodal essence of human nature adopts special characteristics in individual and team sports, given the roles that athletes adopt in different circumstances, depending on the contingencies that characterize each competition or each training session. The mixed methods ...
Data analysis involved a random effects meta-analytic approach, assessing heterogeneity and employing multiple methods to address publication bias, along with meta-regression for continuous moderating variables (quality, female percentage and mean age) and subgroup analyses for categorical moderating variables (sample grade level).