User Preferences
Content preview.
Arcu felis bibendum ut tristique et egestas quis:
- Ut enim ad minim veniam, quis nostrud exercitation ullamco laboris
- Duis aute irure dolor in reprehenderit in voluptate
- Excepteur sint occaecat cupidatat non proident
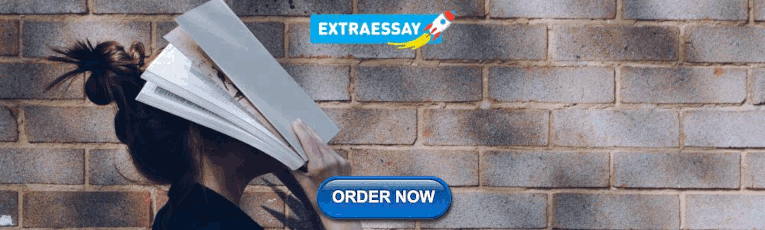
Keyboard Shortcuts
Lesson 5: introduction to factorial designs, introduction section .
Factorial designs are the basis for another important principle besides blocking - examining several factors simultaneously. We will start by looking at just two factors and then generalize to more than two factors. Investigating multiple factors in the same design automatically gives us replication for each of the factors.
- Factorial Designs as among the most common experimental designs
- Two factor Factorial Design and its extension to the General Factorial Designs
- Sample size determination in Factorial Designs
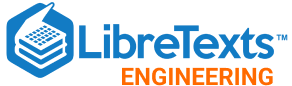
- school Campus Bookshelves
- menu_book Bookshelves
- perm_media Learning Objects
- login Login
- how_to_reg Request Instructor Account
- hub Instructor Commons
Margin Size
- Download Page (PDF)
- Download Full Book (PDF)
- Periodic Table
- Physics Constants
- Scientific Calculator
- Reference & Cite
- Tools expand_more
- Readability
selected template will load here
This action is not available.
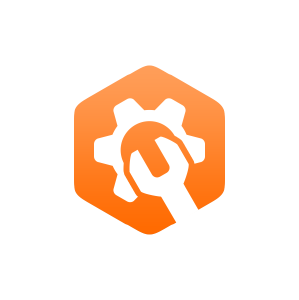
14.2: Design of experiments via factorial designs
- Last updated
- Save as PDF
- Page ID 22537
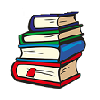
- Jocelyn Anleitner, Stephanie Combs, Diane Feldkamp, Heeral Sheth, Jason Bourgeois, Michael Kravchenko, Nicholas Parsons, & Andrew Wang
- University of Michigan
\( \newcommand{\vecs}[1]{\overset { \scriptstyle \rightharpoonup} {\mathbf{#1}} } \)
\( \newcommand{\vecd}[1]{\overset{-\!-\!\rightharpoonup}{\vphantom{a}\smash {#1}}} \)
\( \newcommand{\id}{\mathrm{id}}\) \( \newcommand{\Span}{\mathrm{span}}\)
( \newcommand{\kernel}{\mathrm{null}\,}\) \( \newcommand{\range}{\mathrm{range}\,}\)
\( \newcommand{\RealPart}{\mathrm{Re}}\) \( \newcommand{\ImaginaryPart}{\mathrm{Im}}\)
\( \newcommand{\Argument}{\mathrm{Arg}}\) \( \newcommand{\norm}[1]{\| #1 \|}\)
\( \newcommand{\inner}[2]{\langle #1, #2 \rangle}\)
\( \newcommand{\Span}{\mathrm{span}}\)
\( \newcommand{\id}{\mathrm{id}}\)
\( \newcommand{\kernel}{\mathrm{null}\,}\)
\( \newcommand{\range}{\mathrm{range}\,}\)
\( \newcommand{\RealPart}{\mathrm{Re}}\)
\( \newcommand{\ImaginaryPart}{\mathrm{Im}}\)
\( \newcommand{\Argument}{\mathrm{Arg}}\)
\( \newcommand{\norm}[1]{\| #1 \|}\)
\( \newcommand{\Span}{\mathrm{span}}\) \( \newcommand{\AA}{\unicode[.8,0]{x212B}}\)
\( \newcommand{\vectorA}[1]{\vec{#1}} % arrow\)
\( \newcommand{\vectorAt}[1]{\vec{\text{#1}}} % arrow\)
\( \newcommand{\vectorB}[1]{\overset { \scriptstyle \rightharpoonup} {\mathbf{#1}} } \)
\( \newcommand{\vectorC}[1]{\textbf{#1}} \)
\( \newcommand{\vectorD}[1]{\overrightarrow{#1}} \)
\( \newcommand{\vectorDt}[1]{\overrightarrow{\text{#1}}} \)
\( \newcommand{\vectE}[1]{\overset{-\!-\!\rightharpoonup}{\vphantom{a}\smash{\mathbf {#1}}}} \)
Factorial design is an important method to determine the effects of multiple variables on a response. Traditionally, experiments are designed to determine the effect of ONE variable upon ONE response. R.A. Fisher showed that there are advantages by combining the study of multiple variables in the same factorial experiment. Factorial design can reduce the number of experiments one has to perform by studying multiple factors simultaneously. Additionally, it can be used to find both main effects (from each independent factor) and interaction effects (when both factors must be used to explain the outcome). However, factorial design can only give relative values, and to achieve actual numerical values the math becomes difficult, as regressions (which require minimizing a sum of values) need to be performed. Regardless, factorial design is a useful method to design experiments in both laboratory and industrial settings.
Factorial design tests all possible conditions. Because factorial design can lead to a large number of trials, which can become expensive and time-consuming, factorial design is best used for a small number of variables with few states (1 to 3). Factorial design works well when interactions between variables are strong and important and where every variable contributes significantly.
What is Factorial Design?
Factorial design example.
The easiest way to understand how factorial design works is to read an example. Suppose that you, a scientist working for the FDA, would like to study and measure the probability of patients suffering from seizures after taking a new pharmaceutical drug called CureAll. CureAll is a novel drug on the market and can cure nearly any ailment of the body. You along with your co-workers at the FDA have decided to test two dosage levels: 5 mg and 10 mg. You are also interested in determining whether the drug side-effects differ between younger adults (age 20) and older adults (age 40). Based on the given information, you see that there are two factors: dosage and age. Factors are the main categories to explore when determining the cause of seizures in patients. Under each of these factors, there are different levels: 5 and 10 mg for the dosage; 20 and 40 years for age. A level is basically one of the subdivisions that make up a factor. From this information, we can see that we have a 2 x 2 factorial design, which means that we will have 2 * 2 = 4 groups. A group is set of conditions that will make up that particular experiment.
Null Outcome
A null outcome situation is when the outcome of your experiment is the same regardless of how the levels within your experiment were combined. From the example above, a null outcome would exist if you received the same percentage of seizures occurring in patients with varying dose and age. The graphs below illustrate no change in the percentage of seizures for all factors, so you can conclude that the chance of suffering from a seizure is not affected by the dosage of the drug or the age of the patient.
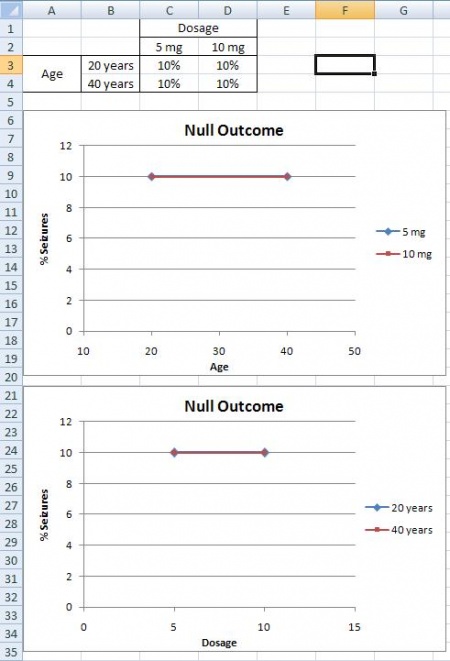
Main Effects
A main effects situation is when there exists a consistent trend among the different levels of a factor. From the example above, suppose you find that as dosage increases, the percentage of people who suffer from seizures increases as well. You also notice that age does not play a role; both 20 and 40 year olds suffer the same percentage of seizures for a given amount of CureAll. From this information, you can conclude that the chance of a patient suffering a seizure is minimized at lower dosages of the drug (5 mg). The second graph illustrates that with increased drug dosage there is an increased percentage of seizures, while the first graph illustrates that with increased age there is no change in the percentage of seizures. Both of these graphs only contain one main effect, since only dose has an effect the percentage of seizures. Whereas, graphs three and four have two main effects, since dose and age both have an effect on the percentage of seizures.
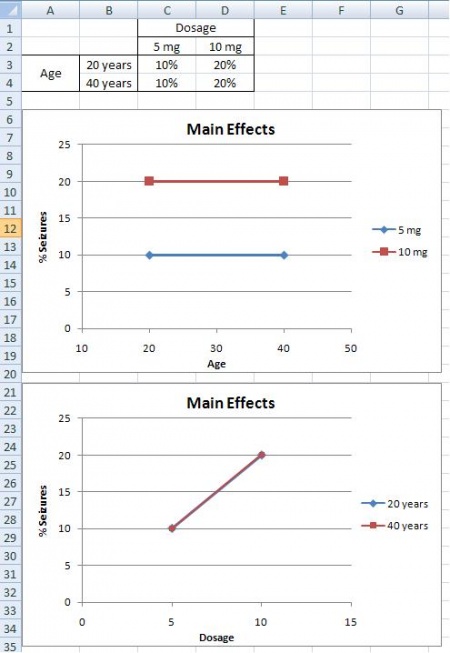
Interaction Effects
The interaction effects situation is the last outcome that can be detected using factorial design. From the example above, suppose you find that 20 year olds will suffer from seizures 10% of the time when given a 5 mg CureAll pill, while 20 year olds will suffer 25% of the time when given a 10 mg CureAll pill. When 40 year olds, however, are given a 5 mg pill or a 10 mg pill, 15% suffer from seizures at both of these dosages. This correlation can be seen in the graphs below. There is an increasing chance of suffering from a seizure at higher doses for 20 year olds, but no difference in suffering from seizures for 40 year olds. Thus, there must be an interaction effect between the dosage of CureAll, and the age of the patient taking the drug. When you have an interaction effect it is impossible to describe your results accurately without mentioning both factors. You can always spot an interaction in the graphs because when there are lines that are not parallel an interaction is present. If you observe the main effect graphs above, you will notice that all of the lines within a graph are parallel. In contrast, for interaction effect graphs, you will see that the lines are not parallel.
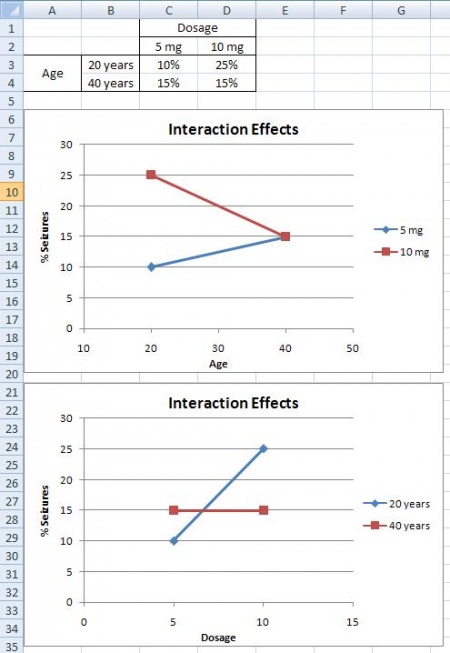
Mathematical Analysis Approach
In the previous section, we looked at a qualitative approach to determining the effects of different factors using factorial design. Now we are going to shift gears and look at factorial design in a quantitative approach in order to determine how much influence the factors in an experiment have on the outcome.
How to Deal with a 2 n Factorial Design
Suppose you have two variables \(A\) and \(B\) and each have two levels a 1 , a 2 and b 1 , b 2 . You would measure combination effects of \(A\) and \(B\) (a 1 b 1 , a 1 b 2 , a 2 b 1 , a 2 b 2 ). Since we have two factors, each of which has two levels, we say that we have a 2 x 2 or a 2 2 factorial design. Typically, when performing factorial design, there will be two levels, and n different factors. Thus, the general form of factorial design is 2 n .
In order to find the main effect of \(A\), we use the following equation:
\[A = (a_2b_1 - a_1b_1) + (a_2b_2 - a_1b_2) \nonumber \]
Similarly, the main effect of B is given by:
\[B = (b_2a_1 - b_1a_1) + (b_2a_2 - b_1a_2) \nonumber \]
By the traditional experimentation, each experiment would have to be isolated separately to fully find the effect on B. This would have resulted in 8 different experiments being performed. Note that only four experiments were required in factorial designs to solve for the eight values in A and B. This shows how factorial design is a timesaver.
By taking the coefficients in A and B, the table below was created.
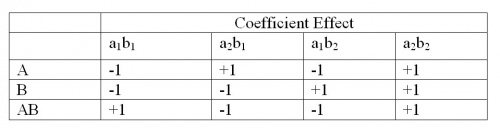
AB is found by multiplying the coefficients of a x b x to get the new coefficient effect.
The additional complication is the fact that more than one trial/replication is required for accuracy, so this requires adding up each sub-effect (e.g adding up the three trials of a 1 b 1 ). By adding up the coefficient effects with the sub-effects (multiply coefficient with sub-effect), a total factorial effect can be found. This value will determine if the factor has a significant effect on the outcome. For larger numbers, the factor can be considered extremely important and for smaller numbers, the factor can be considered less important. The sign of the number also has a direct correlation to the effect being positive or negative.
To get a mean factorial effect, the totals needs to be divided by 2 times the number of replicates , where a replicate is a repeated experiment.
\[\text {mean factorial effect} = \dfrac{\text{total factorial effect}}{2r} \nonumber \]
By adding a third variable (\(C\)), the process of obtaining the coefficients becomes significantly complicated. The main factorial effect for \(A\):
\[A=\left(a_{2} b_{1} c_{1}-a_{1} b_{1} c_{1}\right)+\left(a_{2} b_{2} c_{1}-a_{1} b_{2} c_{1}\right)+\left(a_{2} b_{1} c_{2}-a_{1} b_{1} c_{2}\right)+\left(a_{2} b_{2} c_{2}-a_{1} b_{2} c_{2}\right) \nonumber \]
The table of coefficients is listed below
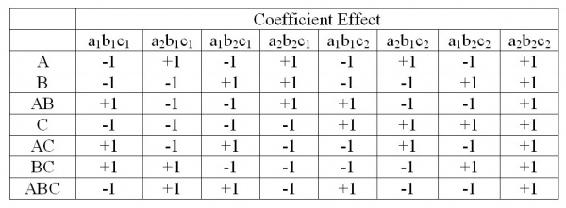
It is clear that in order to find the total factorial effects, you would have to find the main effects of the variable and then the coefficients. Yates Algorithm can be used to simplify the process.
Yates Algorithm
Frank Yates created an algorithm to easily find the total factorial effects in a 2 n factorial that is easily programmable in Excel. While this algorithm is fairly straightforward, it is also quite tedious and is limited to 2 n factorial designs. Thus, modern technology has allowed for this analysis to be done using statistical software programs through regression.
- In the first column, list all the individual experimental combinations
According to the yates order, such as follows for a 2 3 factorial design
- In the second column, list all the totals for each combination
- The 1 st four entries in the third column (Stage 1) are obtained by adding pairs together from the "totals list" (previous column). The next four numbers are obtained by subtracting the top number from the bottom number of each pair.
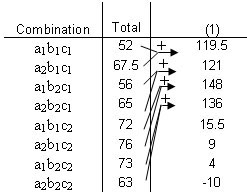
- The fourth column (Stage 2) is obtained in the same fashion, but this time adding and subtracting pairs from Stage 1.
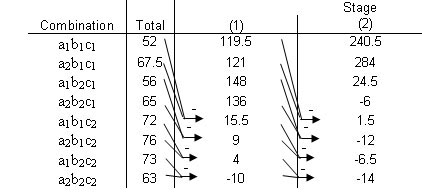
- The fifth column (Stage 3) is obtained in the same fashion, but this time adding and subtracting pairs from Stage 2.
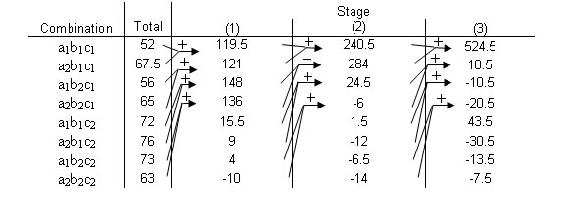
- Continue with Stages until reaching n , or the number of factors. This final column is the Effect Total. A positive value means a positive correlation, and a negative values means a negative correlation. These values are all relative, however, so there is no way to compare different effect totals from different experiments.
Ignoring the first row, look in the last stage and find the variable that has the largest relative number, then that row indicates the MAIN TOTAL EFFECT . The Main Total Effect can be related to input variables by moving along the row and looking at the first column. If the row in the first column is a 2 b 1 c 1 then the main total effect is A. The row for a 1 b 2 c 1 would be for B. The row for a 2 b 1 c 2 would be for AC.
This main total effect value for each variable or variable combination will be some value that signifies the relationship between the output and the variable. For instance, if your value is positive, then there is a positive relationship between the variable and the output (i.e. as you increase the variable, the output increases as well). A negative value would signify a negative relationship. Notice, however, that the values are all relative to one another. So while the largest main total effect value in one set of experiments may have a value of 128, another experiment may have its largest main total effect value be 43. There is no way to determine if a value of 128 in one experiment has more control over its output than a value of 43 does, but for the purposes of comparing variables within an experiment, the main total effect does allow you to see the relative control the variables have on the output.
Factorial Design Example Revisited
Recall the example given in the previous section What is Factorial Design? In the example, there were two factors and two levels, which gave a 2 2 factorial design. The Yates Algorithm can be used in order to quantitatively determine which factor affects the percentage of seizures the most. For the use of the Yates algorithm, we will call age factor A with a 1 = 20 years, and a 2 = 40 years. Likewise, we will call dosage factor B, with b 1 = 5 mg, and b 2 = 10 mg. The data for the three outcomes is taken from the figures given in the example, assuming that the data given resulted from multiple trials.
The following Yates algorithm table using the data for the null outcome was constructed. As seen in the table, the values of the main total factorial effect are 0 for A, B, and AB. This proves that neither dosage or age have any effect on percentage of seizures.
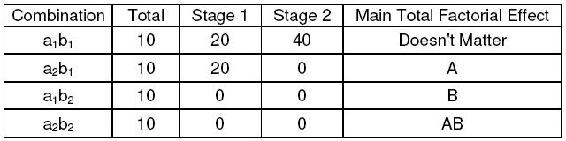
Main Effect
The following Yates algorithm table using the data from the first two graphs of the main effects section was constructed. Besides the first row in the table, the row with the largest main total factorial effect is the B row, while the main total effect for A is 0. This means that dosage (factor B) affects the percentage of seizures, while age (factor A) has no effect, which is also what was seen graphically.
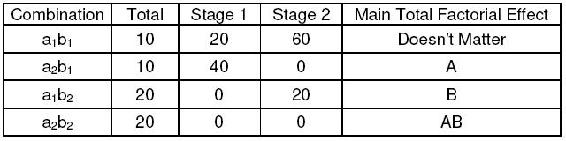
The following Yates algorithm table using the data from second two graphs of the main effects section was constructed. Besides the first row in the table, the main total effect value was 10 for factor A and 20 for factor B. This means that both age and dosage affect percentage seizures. However, since the value for B is larger, dosage has a larger effect on percentage of seizures than age. This is what was seen graphically, since the graph with dosage on the horizontal axis has a slope with larger magnitude than the graph with age on the horizontal axis.
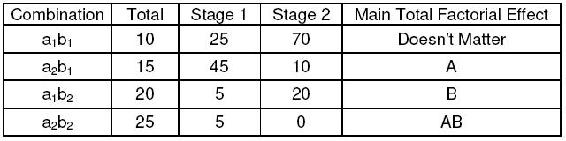
Interaction Effect
The following Yates algorithm table was constructed using the data from the interaction effects section. Since the main total factorial effect for AB is non-zero, there are interaction effects. This means that it is impossible to correlate the results with either one factor or another; both factors must be taken into account.
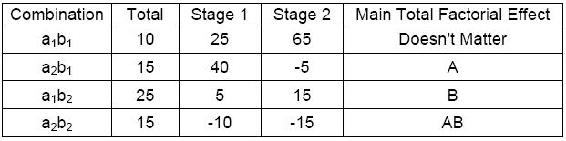
Chemical Engineering Applications
It should be quite clear that factorial design can be easily integrated into a chemical engineering application. Many chemical engineers face problems at their jobs when dealing with how to determine the effects of various factors on their outputs. For example, suppose that you have a reactor and want to study the effect of temperature, concentration and pressure on multiple outputs. In order to minimize the number of experiments that you would have to perform, you can utilize factorial design. This will allow you to determine the effects of temperature and pressure while saving money on performing unnecessary experiments.
A 2007 study on converting wheat straw to fuel utilized factorial design to study the effect of four factors on the composition and susceptibility to enzyme hydrolysis of the final product. (Perez, et.al.). The table below shows the full factorial design for the study. The four factors that were studied all had only two levels and dealt with pretreatment parameters. They were: Water temperature, residence time, solid fraction and overpressure in the reactor. It is not necessary to understand what each of these are to understand the experimental design. As seen in the table below, there were sixteen trials, or 2^4 experiments.
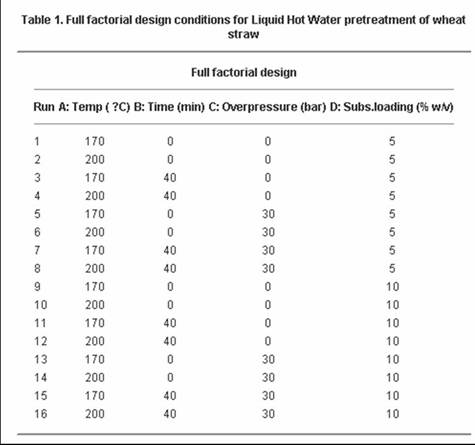
Source: Perez, et. al.
Minitab DOE Example
Minitab 15 Statistical Software is a powerful statistics program capable of performing regressions, ANOVA, control charts, DOE, and much more. Minitab is especially useful for creating and analyzing the results for DOE studies. It is possible to create factorial, response surface, mixture, and taguchi method DOEs in Minitab. The general method for creating factorial DOEs is discussed below.
Creating Factorial DOE
Minitab provides a simple and user-friendly method to design a table of experiments. Additionally, analysis of multiple responses (results obtained from experimentation) to determine which parameters significantly affect the responses is easy to do with Minitab. Minitab 15 Statistical Software can be used via Virtual CAEN Labs by going to Start>All Programs>Math and Numerical Methods>Minitab Solutions>Minitab 15 Statistical Software.
The first step is creating the DOE by specifying the number of levels (typically 2) and number of responses. To do this, go to Stat>DOE>Factorial>Create Factorial Design as shown in the image below.
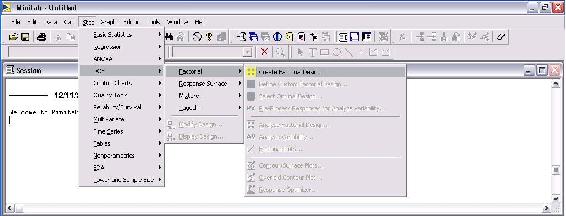
The next image is the "Create Factorial Design" options menu.
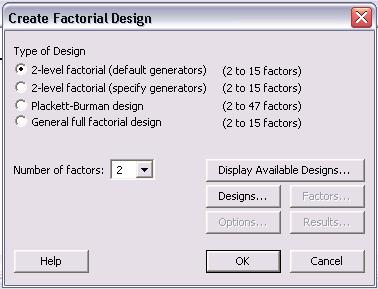
For a 2 level design, click the "2-level factorial (default generators)" radio button. Then specify the number of factors between 2 and 15. Other designs such as Plackett-Burman or a General full factorial design can be chosen. For information about these designs, please refer to the "Help" menu.
After the number of factors is chosen, click on the "Designs..." option to see the following menu.
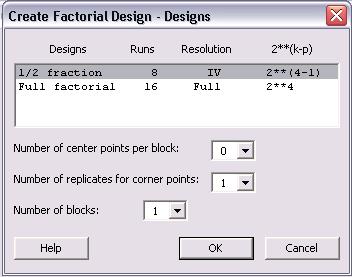
In this menu, a 1/2 fraction or full factorial design can be chosen. Although the full factorial provides better resolution and is a more complete analysis, the 1/2 fraction requires half the number of runs as the full factorial design. In lack of time or to get a general idea of the relationships, the 1/2 fraction design is a good choice. Additionally, the number of center points per block, number of replicates for corner points, and number of blocks can be chosen in this menu. Consult the "Help" menu for details about these options. Click "Ok" once the type of design has been chosen.
Once the design has been chosen, the "Factors...", "Options..." and "Results..." buttons become active in the "Create Factorial Designs" option menu. Click on "Factors..." button to see the following menu.
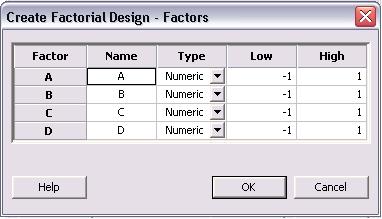
The above image is for a 4 factor design. Factors A - D can be renamed to represent the actual factors of the system. The factors can be numeric or text. Additionally, a low and high value are initially listed as -1 and 1, where -1 is the low and 1 is the high value. The low and high levels for each factor can be changed to their actual values in this menu. Click "OK" once this is completed.
The necessary steps for creating the DOE are complete, but other options for "Results..." and "Options..." can be specified. The menus for "Results..." and "Options..." are shown below.
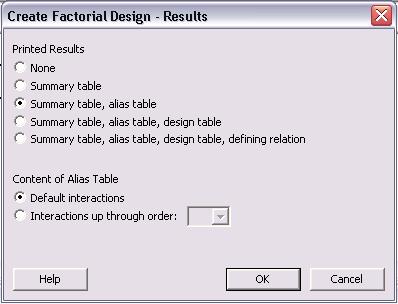
In the main "Create Factorial Design" menu, click "OK" once all specifications are complete. The following table is obtained for a 2-level, 4 factor, full factorial design. None of the levels were specified as they appear as -1 and 1 for low and high levels, respectively.
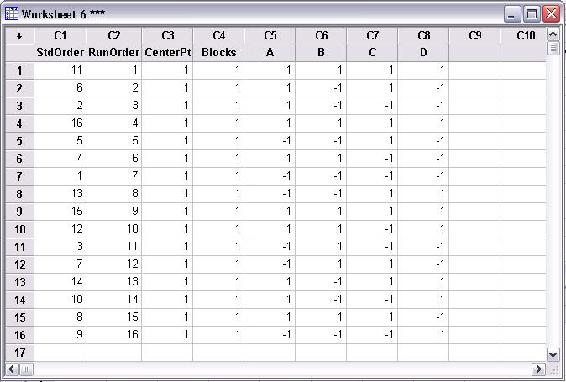
The above table contains all the conditions required for a full factorial DOE. Minitab displays the standard order and randomized run order in columns C1 and C2, respectively. Columns A-D are the factors. The first run (as specified by the random run order) should be performed at the low levels of A and C and the high levels of B and D. A total of 16 runs are required to complete the DOE.
Modifying DOE Table
Once a table of trials for the DOE has been created, additional modifications can be made as needed. Some typical modifications include modifying the name of each factors, specifying the high and low level of each factor, and adding replicates to the design. To being modifications of a current design, go to Stat>DOE>Modify Design... as seen in the figure below.
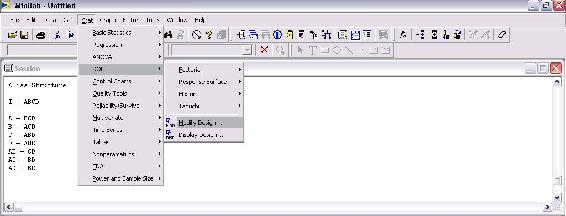
The following menu is displayed for modifying the design.
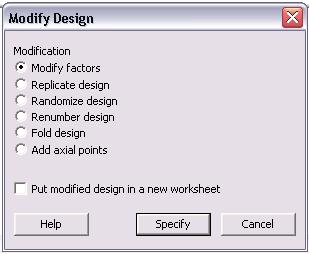
In the "Modify Design" menu, users can modify factors, replicate design, randomize design, renumber design, fold design, and add axial points. Additionally, any changes made can be put into a new worksheet. To change the factors, click the "Modify factors" radio button and then "Specify" to see the following options menu.
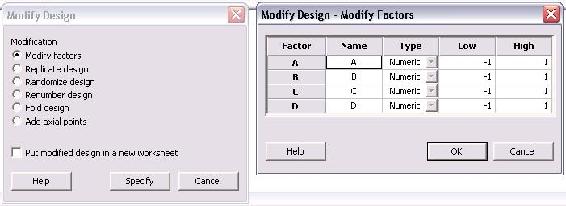
The default factors are named "A", "B", "C", and "D" and have respective high and low levels of 1 and -1. The name of the factors can be changed by simply clicking in the box and typing a new name. Additionally, the low and high levels for each factor can be modified in this menu. Since the high and low levels for each factor may not be known when the design is first created, it is convenient to be able to define them later. Click "OK" after modifications are complete.
Another typical modification is adding replicates to a design. Replicates are repeats of each trial that help determine the reproducibility of the design, thus increasing the number of trials and accuracy of the DOE. To add replicates, click the "Replicate design" radio button in the "Modify Design" menu. The following menu will be displayed.
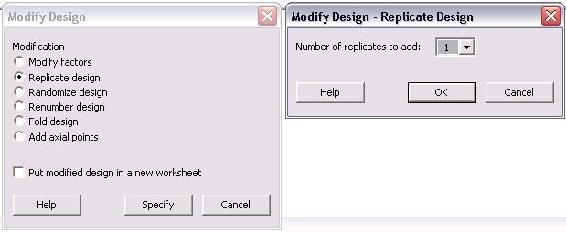
The only option in this menu is the number of replicates to add. The number ranges between 1 and 10. To have a total of 3 trials of each, the user should add 2 replicates in this menu. If 4 replicates are added, there will be a total of 5 trials of each. Typically, if the same experimentation will occur for 3 lab periods, 2 replicates will be added.
Additional modifications to the design include randomizing and renumbering the design. These are very straightforward modifications which affect the ordering of the trials. For information about the "Fold design" and "Add axial points", consult the "Help" menu.
Analyzing DOE Results
After the complete DOE study has been performed, Minitab can be used to analyze the effect of experimental results (referred to as responses) on the factors specified in the design. The first step in analyzing the results is entering the responses into the DOE table. This is done much like adding data into an Excel data sheet. In the columns to the right of the last factor, enter each response as seen in the figure below.
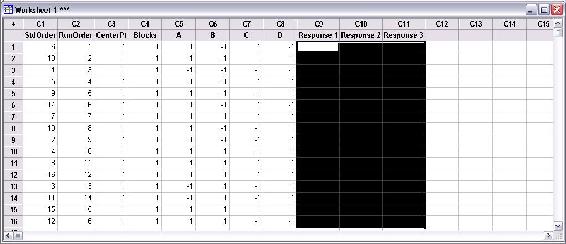
The above figure contains three response columns. The names of each response can be changed by clicking on the column name and entering the desired name. In the figure, the area selected in black is where the responses will be inputted. For instance, if the purity, yield, and residual amount of catalyst was measured in the DOE study, the values of these for each trial would be entered in the columns.
Once the responses are entered, statistical analysis on the data can be performed. Go to Stat>DOE>Factorial>Analyze Factorial Design... as seen in the following image.
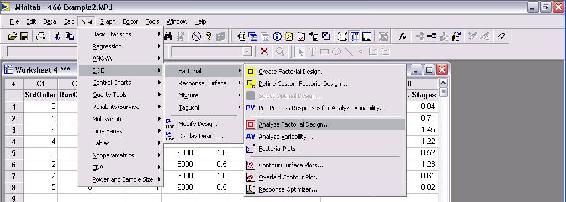
The menu that appears for analyzing factorial design is shown below.
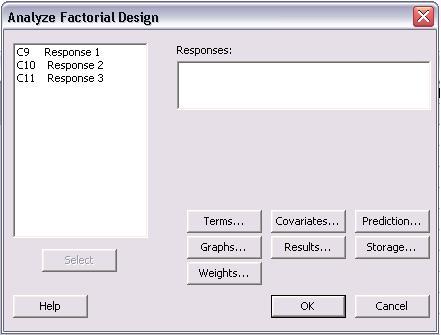
In the "Analyze Factorial Design" menu, the responses are shown on the left of the screen. The first step is to choose the responses to be analyzed. All of the responses can be chosen at once or individually. To choose them, click (or click and drag to select many) and then click "Select" to add them into the "Responses:" section as seen below.
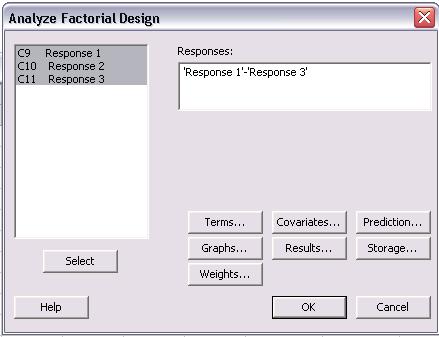
The next step is selecting which terms will be analyzed for the responses. To do this, click on "Terms..." and the following menu will appear.
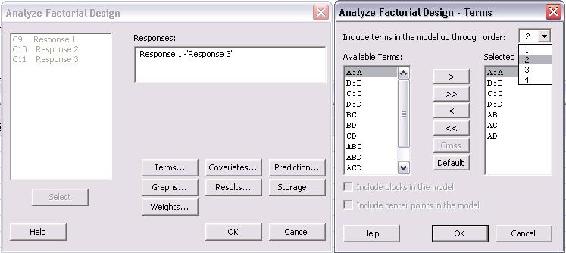
The types of interactions between factors are chosen in this menu. For a first order model which excludes all factor-to-factor interactions, "1" should be chosen from the drop-down menu for "Include terms in the model up through order:". To include higher order terms and account for factor interactions, choose 2, 3, or 4 from the drop-down menu. Unless significant factor-to-factor interactions are expected, it is recommended to use a first order model which is a linear approximation.
Once the terms have been chosen, the next step is determining which graphs should be created. The types of graphs can be selected by clicking on "Graphs..." in the main "Analyze Factorial Design" menu.
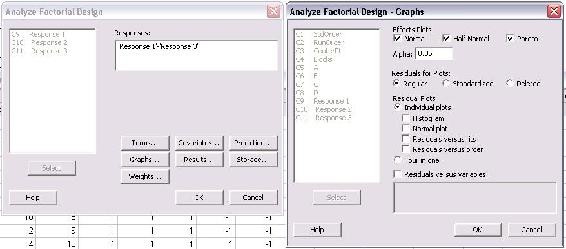
In the Graphs menu shown above, the three effects plots for "Normal", "Half Normal", and "Pareto" were selected. These plots are different ways to present the statistical results of the analysis. Examples of these plots can be found in the Minitab Example for Centrifugal Contactor Analysis. The alpha value, which determines the limit of statistical significance, can be chosen in this menu also. Typically, the alpha value is 0.05. The last type of plots that can be chosen is residual plots. A common one to select is "Residuals versus fits" which shows how the variance between the predicted values from the model and the actual values.
The final option that must be specified is results. Click "Results..." from the "Analyze Factorial Design" menu to see the following screen.
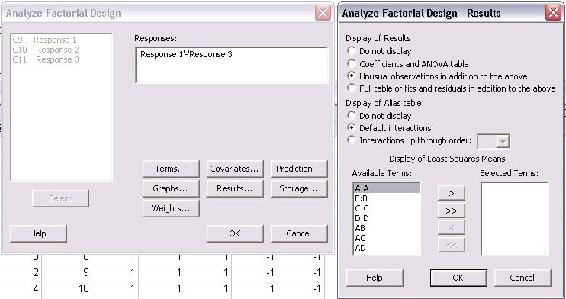
In this menu, select all of the "Available Terms" and click the ">>" button to move them to the "Selected Terms". This will ensure that all the terms will be included in the analysis. Another feature that can be selected from this menu is to display the "Coefficients and ANOVA table" for the DOE study.
Other options can be selected from the "Analyze Factorial Design" menu such as "Covariates...", "Prediction...", "Storage...", and "Weights...". Consult the "Help" menu for descriptions of the other options. Once all desired changes have been made, click "OK" to perform the analysis. All of the plots will pop-up on the screen and a text file of the results will be generated in the session file.
Minitab Example for Centrifugal Contactor Analysis
Centrifugal Contactors, also known as Podbielniak (POD) centrifugal contactors, are used to purify a contaminated stream by counter-current, liquid-liquid extraction. Two immiscible fluids with different specific gravities are contacted counter-currently and the solute from the dirty stream is extracted by the clean stream. A common use for PODs methanol removal from biodiesel by contacting the stream with water. The amount of methanol remaining in the biodiesel (wt% MeOH) after the purification and the number of theoretical stages (No. Theor. Stages) obtained depend on the operating conditions of the POD. The four main operating parameters of the POD are rotational speed (RPM), ratio of biodiesel to water (Ratio), total flow rate of biodiesel and water (Flow Rate), and pressure (Pressure). A DOE study has been performed to determine the effect of the four operating conditions on the responses of wt% MeOH in biodiesel and number of theoretical stages achieved. (NOTE: The actual data for this example was made-up)
A 4-factor, 2-level DOE study was created using Minitab. Because experiments from the POD are time consuming, a half fraction design of 8 trial was used. The figure below contains the table of trials for the DOE.
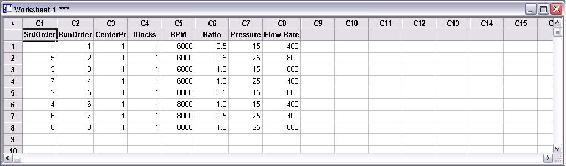
After all the trials were performed, the wt% methanol remaining in the biodiesel and number of theoretical stages achieved were calculated. The figure below contains the DOE table of trials including the two responses.
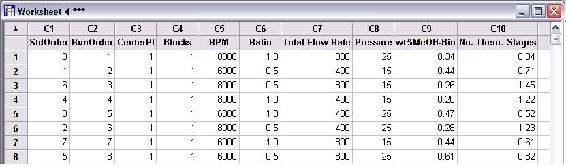
Analysis was performed on the DOE study to determine the effects of each factor on the responses. Only first order terms were included in the analysis to create a linear model. Pareto charts for both wt% MeOH in biodiesel and number of theoretical stages are shown below.
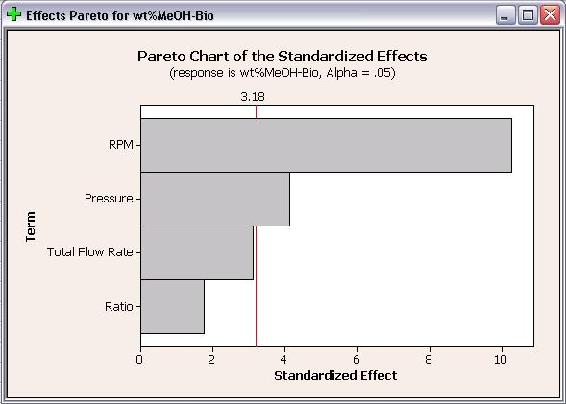
The Pareto charts show which factors have statistically significant effects on the responses. As seen in the above plots, RPM has significant effects for both responses and pressure has a statistically significant effect on wt% methanol in biodiesel. Neither flow rate or ratio have statistically significant effects on either response. The Pareto charts are bar charts which allow users to easily see which factors have significant effects.
Half Normal Plots for wt% methanol in biodiesel and number of theoretical stages are shown below.
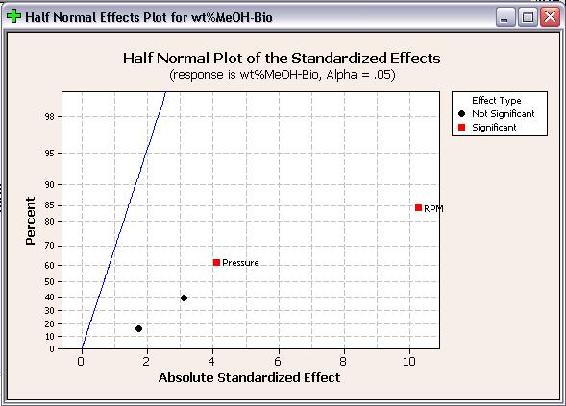
Like Pareto plots, Half Normal plots show which factors have significant effects on the responses. The factors that have significant effects are shown in red and the ones without significant effects are shown in black. The further a factor is from the blue line, the more significant effect it has on the corresponding response. For wt% methanol in biodiesel, RPM is further from the blue line than pressure, which indicates that RPM has a more significant effect on wt% methanol in biodiesel than pressure does.
The final plot created is the Normal Effect Plot. The Normal Plot is similar to the Half Normal plot in design. However, the Normal Plot displays whether the effect of the factor is positive or negative on the response. The Normal Plots for the responses are shown below.
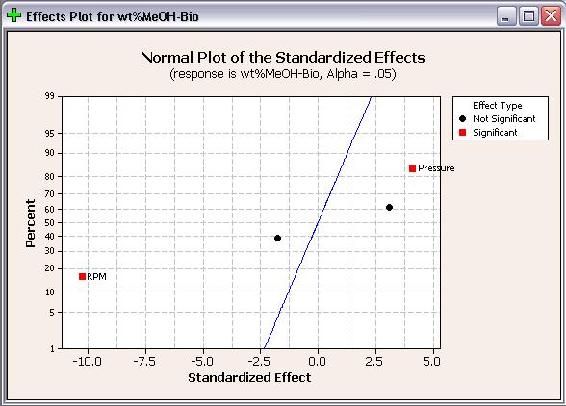
As seen above, RPM is shown with a positive effect for number of theoretical stages, but a negative effect for wt% methanol in biodiesel. A positive effect means that as RPM increases, the number of theoretical stages increases. Whereas a negative effect indicates that as RPM increases, the wt% methanol in biodiesel decreases. Fortunately for operation with the POD, these are desired results. When choosing operating conditions for the POD, RPM should be maximized to minimize the residual methanol in biodiesel and maximize the number of theoretical stages achieved.
In addition to the above effects plots, Minitab calculates the coefficients and constants for response equations. The response equations can be used as models for predicting responses at different operating conditions (factors). The coefficients and constants for wt% methanol in biodiesel and number of theoretical stages are shown below.
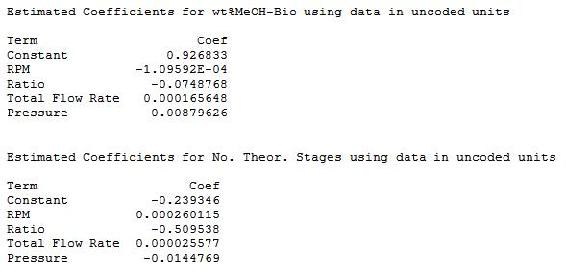
Since this is a first order, linear model, the coefficients can be combined with the operating parameters to determine equations. The equations from this model are shown below.

These equations can be used as a predictive model to determine wt% methanol in biodiesel and number of theoretical stages achieved at different operating conditions without actually performing the experiments. However, the limits of the model should be tested before the model is used to predict responses at many different operating conditions.
Example \(\PageIndex{1}\)
You have been employed by SuperGym, a local personal training gym, who want an engineer's perspective on how to offer the best plans to their clients. SuperGym currently categorizes her clients into 4 body types to help plan for the best possible program.
- Type 1 - Very healthy
- Type 2 - Needs tone
- Type 3 - Needs strength
- Type 4 - Needs tone and strength
In addition, SuperGym offers 4 different workout plans, A through D, none of which are directly catered to any of the different types. Create an experimental factorial design that could be used to test the effects of the different workout plans on the different types of people at the gym.
In order to solve this problem, we need to determine how many different experiments would need to be performed. In order to solve this, we can see that we have two different factors, body type and workout plan. For each factor, there exist four different levels. Thus, we have a 4 2 factorial design, which gives us 16 different experimental groups. Creating a table of all of the different groups, we arrive at the following factorial design:
A1 | B1 | C1 | D1 |
A2 | B2 | C2 | D2 |
A3 | B3 | C3 | D3 |
A4 | B4 | C4 | D4 |
Where A-D is the workout plan and 1-4 is the types
Example \(\PageIndex{2}\)
Suppose that you are looking to study the effects of hours slept (A), hours spent with significant other (B), and hours spent studying (C) on a students exam scores. You are given the following table that relates the combination of these factors and the students scores over the course of a semester. Use the Yates method in order to determine the effect each variable on the students performance in the course.
1 | 17 | 24 | 19 | 21 | 22 | 28 | 25 | 24 |
---|---|---|---|---|---|---|---|---|
2 | 18.5 | 21 | 20 | 19 | 26 | 22 | 27 | 19 |
3 | 16.5 | 22.5 | 22 | 25 | 24 | 26 | 21 | 20 |
Total | 52 | 67.5 | 61 | 65 | 72 | 76 | 73 | 63 |
Using the approach introduced earlier in this article, we arrive at the following Yates solution.
Stage | Main Total | ||||
Combination | Total | 1 | 2 | 3 | Factorial Effect |
a b c | 52 | 119.5 | 245.5 | 529.9 | Doesn't matter |
a b c | 67.5 | 126 | 284 | 13.5 | A |
a b c | 61 | 148 | 19.5 | -5.5 | B |
a b c | 65 | 136 | -6 | -25.5 | AB |
a b c | 72 | 15.5 | 6.5 | 38.5 | C |
a b c | 76 | 4 | -12 | -25.5 | AC |
a b c | 73 | 4 | -11.5 | -18.5 | BC |
a b c | 63 | -10 | -14 | -2.5 | ABC |
From this table, we can see that there is positive correlation for factors A and C, meaning that more sleep and more studying leads to a better test grade in the class. Factor B, however, has a negative effect, which means that spending time with your significant other leads to a worse test score. The lesson here, therefore, is to spend more time sleeping and studying, and less time with your boyfriend or girlfriend.
Example \(\PageIndex{3}\)
Your mom is growing a garden for the state fair and has done some experiments to find the ideal growing condition for her vegetables. She asks you for help interpreting the results and shows you the following data:
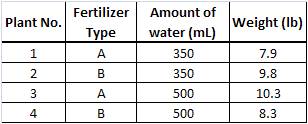
Make plots to determine the main or interaction effects of each factor.
Here is the plot you should have gotten for the given data.
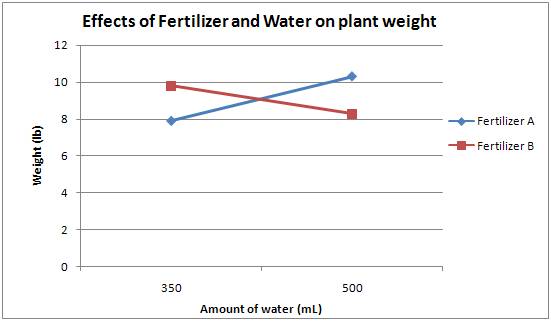
From this one can see that there is an interaction effect since the lines cross. One cannot discuss the results without speaking about both the type of fertilizer and the amount of water used. Using fertilizer A and 500 mL of water resulted in the largest plant, while fertilizer A and 350 mL gave the smallest plant. Fertilizer B and 350 mL gave the second largest plant, and fertilizer B and 500 mL gave the second smallest plant. There is clearly an interaction due to the amount of water used and the fertilizer present. Perhaps each fertilizer is most effective with a certain amount of water. In any case, your mom has to consider both the fertilizer type and amount of water provided to the plants when determining the proper growing conditions.
Exercise \(\PageIndex{1}\)
Which of the following is not an advantage of the use of factorial design over one factor design?
- More time efficient
- Provides how each factor effects the response
- Does not require explicit testing
- Does not require regression
Exercise \(\PageIndex{2}\)
In a 2 2 factorial design experiment, a total main effect value of -5 is obtained. This means that
- there is a relative positive correlation between the two factors
- there is no correlation between the two factors
- there is a relative negative correlation between the two factors
- there is either a positive or negative relative correlation between the two factors
- Box, George E.P., et. al. "Statistics for Engineers: An Introduction to Design, Data Analysis, and Model Building." New York: John Wiley & Sons.
- Trochim, William M.K. 2006. "Factorial Designs." Research Methods Knowledge Base. < http://www.socialresearchmethods.net/kb/expfact.htm >
- Perez, Jose A., et. al. "Effect of process variables on liquid hot water pretreatment of wheat straw for bioconversion to fuel-ethanol in a batch reactor." Journal of Chemical Technology & Biotechnology. Volume 82, Issue 10, Pages 929-938. Published Online Sep 3, 2007.

An official website of the United States government
The .gov means it’s official. Federal government websites often end in .gov or .mil. Before sharing sensitive information, make sure you’re on a federal government site.
The site is secure. The https:// ensures that you are connecting to the official website and that any information you provide is encrypted and transmitted securely.
- Publications
- Account settings
Preview improvements coming to the PMC website in October 2024. Learn More or Try it out now .
- Advanced Search
- Journal List
- HHS Author Manuscripts

Implementing Clinical Research Using Factorial Designs: A Primer
Timothy b. baker.
1 Center for Tobacco Research and Intervention, University of Wisconsin School of Medicine and Public Health, 1930 Monroe St., Suite 200, Madison, WI 53711
Stevens S. Smith
2 University of Wisconsin School of Medicine and Public Health, Department of Medicine, 1025 W. Johnson St., Madison, WI 53706
Daniel M. Bolt
3 University of Wisconsin, Department of Educational Psychology, 1025 W. Johnson St., Madison, WI 53706
Wei-Yin Loh
4 University of Wisconsin, Department of Statistics, 1300 University Ave., Madison, WI 53706
Robin Mermelstein
5 University of Illinois at Chicago, Institute for Health Research and Policy, 544 Westside Research Office Bldg., 1747 West Roosevelt Rd., Chicago, IL 60608
Michael C. Fiore
Megan e. piper, linda m. collins.
6 The Methodology Center and Department of Human Development & Family Studies, The Pennsylvania State University, 404 Health and Human Development Building, University Park, PA 16802
Factorial experiments have rarely been used in the development or evaluation of clinical interventions. However, factorial designs offer advantages over randomized controlled trial designs, the latter being much more frequently used in such research. Factorial designs are highly efficient (permitting evaluation of multiple intervention components with good statistical power) and present the opportunity to detect interactions amongst intervention components. Such advantages have led methodologists to advocate for the greater use of factorial designs in research on clinical interventions ( Collins, Dziak, & Li, 2009 ). However, researchers considering the use of such designs in clinical research face a series of choices that have consequential implications for the interpretability and value of the experimental results. These choices include: whether to use a factorial design, selection of the number and type of factors to include, how to address the compatibility of the different factors included, whether and how to avoid confounds between the type and number of interventions a participant receives, and how to interpret interactions. The use of factorial designs in clinical intervention research poses choices that differ from those typically considered in randomized clinical trial designs. However, the great information yield of the former encourages clinical researchers’ increased and careful execution of such designs.
There is increasing recognition of the need for more efficient research strategies to accelerate progress in the treatment of health and behavioral health problems (e.g., Glasgow, 2013 ; Glasgow, Klesges, Dzewaltowski, Bull, & Estabrooks, 2004 ; Riley, Glasgow, Etheredge, & Abernethy, 2013 ). One promising approach to enhancing research progress is the use of the factorial experiment ( Collins et al., 2009 ; Collins, Kugler, & Gwadz, 2016 ). While factorial experiments have a long history of use in psychology, they have been little used in research on clinical interventions. The factorial experiment is one strategy recommended in the innovative Multiphase Optimization Strategy (MOST: Collins et al., 2009 ), a framework for treatment development and evaluation. MOST holds that such designs be used in screening experiments to evaluate multiple intervention components (ICs) that are candidates for ultimate inclusion in an integrated behavioral or biobehavioral treatment. Factorial experiments are recommended for this screening function since they are highly efficient (using relatively small numbers of participants to screen multiple ICs) and they yield information that shows how different ICs work together (i.e., whether they yield additive or interactive effects). In addition, because they typically experimentally evaluate relatively discrete intervention components, they have the potential to yield especially informative data on the change mechanisms activated by specific treatments. Several recent research studies using factorial designs have now appeared and these attest to the value of such designs ( Cook et al., 2016 ; Fraser et al., 2014 ; McClure et al., 2014 ; Schlam et al., 2016 ).
The vast majority of investigations of treatment efficacy and effectiveness over the past 30–40 years have used the randomized controlled trial (RCT) design. While factorial designs offer some advantages for certain research goals, their use can entail critical decisions regarding design, implementation, analysis, and interpretation. This paper is intended to alert the investigator to such challenges as this may inform decisions about whether to use a factorial design, and how to do so. This paper will use smoking treatment research to illustrate its points, but its content is broadly relevant to the development and evaluation of other types of clinical interventions. Also, it will focus primarily on research design and design implementation rather than on statistical analysis (for relevent discussion of statistical analysis see Box, Hunter, & Hunter, 2005 ; Keppel, 1991 ).
Basic Elements of RCT and Factorial Designs
In an RCT an “active” treatment arm or condition is statistically contrasted with a “control” treatment arm or condition ( Friedman, Furberg, & Demets, 2010 ). The two conditions should be identical except that the control condition lacks one or more ICs or features that are provided to the active condition. The random assignment of participants to the treatment arms means that the two groups of assigned participants should differ systematically only with regard to exposure to those features that are intentionally withheld from the controls. In smoking cessation research a common RCT design is one in which participants are randomly assigned to either an active pharmacotherapy or to placebo, with both groups also receiving the same counseling intervention.
Of course, there are many different types of RCT designs. For instance, RCTs need not have a true placebo or control condition. Thus, two different active treatments might be contrasted with one another in a two-group design, such as a comparison of two different counseling approaches (e.g., skill training vs. supportive counseling), each paired with the same medication. Neither one of these conditions would be a control condition in a strict sense, since each delivers a different form of active treatment. In addition, an RCT might have a control condition, but this might be used in comparisons with many active treatment conditions. For instance, in the comparative treatment design , multiple active treatment conditions are contrasted with a single common control condition (e.g., each of four conditions might receive a different active medication, and each is then compared with a single placebo condition).
A full factorial experiment with k factors, each comprising two levels, contains 2 k unique combinations of factor levels. In this case, a factor is a type or dimension of treatment that the investigator wishes to experimentally evaluate; a “level” is a value that a factor might take on, such as whether an intervention component is of high versus low intensity, or is provided [“on”] or not provided [“off”]. In a full factorial experiment, factors are completely crossed; that is, the factors and their levels are combined so that the design comprises every possible combination of the factor levels. For example, a recent factorial experiment ( Schlam et al., 2016 ) crossed 5 2-level factors, resulting in 32 combinations of factor levels (see Table 1 ). In this case, each of the 32 unique combinations of factor levels could be viewed as constituting a different treatment or treatment condition . In a factorial experiment a person is randomly assigned to a treatment (or treatment condition) from a pool of treatments that collectively comprises all possible combinations of factor levels. Thus, the typical RCT might be viewed as just a single-factor, factorial experiment with that factor comprising two levels: one, an “on” active treatment condition and the other an “off” control treatment condition.
Factors and their levels in Schlam et al. 2016
Cessation Interventions | Adherence Interventions | ||||
---|---|---|---|---|---|
Condition | Medication Duration (8 vs. 26 weeks) | Maintenance Phone Counseling (Intensive vs. none) | Maintenance Medication Adherence Counseling (MMAC vs. none) | Automated Phone Adherence Counseling (Auto phone vs. none) | Electronic Monitoring Adherence Feedback (feedback vs. no feedback) |
1 | Extended | Intensive | MMAC | Auto phone | Feedback |
2 | Extended | Intensive | MMAC | Auto phone | No feedback |
3 | Extended | Intensive | MMAC | None | Feedback |
4 | Extended | Intensive | MMAC | None | No feedback |
5 | Extended | Intensive | None | Auto phone | Feedback |
6 | Extended | Intensive | None | Auto phone | No feedback |
7 | Extended | Intensive | None | None | Feedback |
8 | Extended | Intensive | None | None | No feedback |
9 | Extended | None | MMAC | Auto phone | Feedback |
10 | Extended | None | MMAC | Auto phone | No feedback |
11 | Extended | None | MMAC | None | Feedback |
12 | Extended | None | MMAC | None | No feedback |
13 | Extended | None | None | Auto phone | Feedback |
14 | Extended | None | None | Auto phone | No feedback |
15 | Extended | None | None | None | Feedback |
16 | Extended | None | None | None | No feedback |
17 | Standard | Intensive | MMAC | Auto phone | Feedback |
18 | Standard | Intensive | MMAC | Auto phone | No feedback |
19 | Standard | Intensive | MMAC | None | Feedback |
20 | Standard | Intensive | MMAC | None | No feedback |
21 | Standard | Intensive | None | Auto phone | Feedback |
22 | Standard | Intensive | None | Auto phone | No feedback |
23 | Standard | Intensive | None | None | Feedback |
24 | Standard | Intensive | None | None | No feedback |
25 | Standard | None | MMAC | Auto phone | Feedback |
26 | Standard | None | MMAC | Auto phone | No feedback |
27 | Standard | None | MMAC | None | Feedback |
28 | Standard | None | MMAC | None | No feedback |
29 | Standard | None | None | Auto phone | Feedback |
30 | Standard | None | None | Auto phone | No feedback |
31 | Standard | None | None | None | Feedback |
32 | Standard | None | None | None | No feedback |
Note. This table reflects the combinations of intervention components (conditions) that is generated by the crossing of two levels of five factors in a factorial design ( Schlam et al. 2016 ). The table shows that the crossing of the five factors generates 32 unique combinations of intervention components; participants in the experiment were randomly assigned to one of these conditions so that approximately 1/32 of the N was assigned to each condition.
Note that in the Schlam et al. (2016) experiment ( Table 1 ), all participants in the experiment received one level of each factor. Thus, some participants would receive an “on” or “Hi” level of every factor (an active intervention component); other participants would receive the “off” or “Low” levels of every factor (the “control” levels); and other participants would receive a mixture of the two levels of the various factors. The fact that half of the participants are assigned to one of the two levels of each factor allows the entire N of the experiment to be used to evaluate the effects of each factor. For instance, to evaluate the main effect of Medication Duration, the outcomes of all participants who received Extended Medication (mean of Conditions 1–16: Table 1 ) would be compared to the outcomes of all those who received Standard Medication (mean of Conditions 17–32). Similarly, the entire N of the experiment is used to test interaction effects. A two-way interaction reflects the extent to which the effect of one factor differs depending on the level of another factor. For instance, the interaction of Medication Duration and Maintenance Phone Counseling is computed by examining whether the effect of Medication Duration when Maintenance Phone Counseling is “off” (the mean of Conditions 9–16 compared to the mean of Conditions 25–32) is different from the effect of Medication Duration when Maintenance Phone Counseling is “on” (the mean of Conditions 1–8 compared to the mean of Conditions 17–24). In sum, factorial experiments are efficient because each of the effects in the model is tested with the same N that would alternatively have been used to contrast just the experimental and control conditions in a 2-group RCT (assuming that each factor in the factorial experiment comprises only two levels: Collins et al., 2016 ).
It is possible for a factor to have three or more levels (say “High,” “Medium,” and “Low” levels). However, once a single factor has more than two levels, the experiment loses some of its efficiency since it is no longer the case that every factor level is represented by half of the total N. (If a factor has 4 levels, only 25% of the total N would be exposed to a particular level of that factor.) This means that estimations of effects at a level of that factor will tend to be less reliable, and that tests involving particular factor levels (e.g., when unpacking interaction effects) will have less power if focused comparisons are made with regard to particular factor levels (if factor levels are assumed to be quantitative, as in medication dosages, then a linear term could be used to represent multiple factor levels and conserve power). However, it is important to note that even two-level factors can be used to model intervention intensities at more than two levels. For instance, in Piper et al., (2016) three 2-level factors were used to model counseling intensity as reflected across 8 different experimental conditions (formed by crossing of the three factors: Prequit “Preparation” in-person counseling: On/Off; Postquit In-Person Cessation counseling: Intense/Minimal; and Postquit Phone Cessation Counseling: Intense/Minimal). This design, which broke-up counseling contact into three clinically meaningful factors, resulted in participants getting counseling that ranged from minimal phone and minimal in-person cessation counseling at one extreme, to relatively intense versions of all three counseling components. In theory, if increased counseling intensity as modeled with these three factors significantly enhanced outcomes, then each should yield strong additive effects relative to its control condition. Thus, this design not only roughly captured counseling intensity, but also had the potential to reveal which types of counseling were especially effective relative to their control condition (e.g., phone vs. in-person, prequit vs. post quit). Thus, the use of 2-level factors may not be as restricting as it might seem.
As an example of the statistical power that is available when each factor has two levels, the sample size used in the Schlam study ( N = 544) affords power at ≥ 80% to detect main effect differences in abstinence rates between levels of each of the 5 factors ( Table 1 ): e.g., a difference in abstinence rates of 20% vs. 31% for the two factor levels with α=.05 (two-tailed test). This is the same level of power as would exist for an RCT testing only a single active treatment versus a control treatment. Given a particular N , Type I error rate, and effect size, and regardless of number of factors, a factorial design with each factor comprising two levels, affords the same statistical power for testing the main effects of each factor as does a 2-group RCT that tests a single factor.
Whether to Use an RCT or a Factorial Design
A research design should reflect the goals of the research endeavor. In general, if the major goal of a study is to contrast directly one “treatment” with another treatment (e.g., a control treatment), then an RCT is usually the best choice. Note that here “treatment” is used to connote a set of intervention components (ICs); e.g., a particular type, dose, and duration of medication, type of counseling that is delivered for a particular number of sessions of a particular duration, and so on. Thus, if an investigator were interested, for instance, in the effects of a medication given at a particular dose and duration, and when used with counseling of a particular type, intensity, and delivery system, and how this compares with usual care (which also may contain multiple components), s/he should use an RCT. The statistical analyses would reveal whether the experimental treatment “package” differs in effects from the usual care treatment. However, conducting an RCT that comprises ICs whose joint effects are unknown, poses clear risks. This is because research shows that the effectiveness of a IC can be substantially modulated by the other ICs with which it is used ( Cook et al., 2016 ; Fraser et al., 2014 ; Schlam et al., 2016 ); i.e., they may interact. Thus, in an RCT designed to evaluate a medication, the effect of the medication might be significantly altered by features of the psychosocial intervention that accompanies it (e.g., by the type or intensity of counseling, the number of treatment delivery modalities, and so forth). In other words, if the treatment elements in an RCT have not been experimentally tested in a factorial experiment so that their joint effects are known, the effects of an active IC (e.g., an active medication) might reflect either its main effects or its interaction with another treatment element.
One might use a dismantling or additive treatment strategy as an alternative to a factorial design to try to explore how each IC affects an outcome. For instance, if an RCT shows that a multicomponent treatment is quite effective, the investigator might conduct a series of subsequent RCTs in which elements of the treatment are systematically added or removed in order to discover which IC or set of ICs is especially responsible for the treatment’s effectiveness. Thus, in an additive or “stacked” design, the investigator might start with what he or she identifies as a base intervention IC (e.g., cessation counseling), and then systematically add other ICs that were comprised by the multifactorial treatment, resulting perhaps in the following combinations of ICs to be compared in a single 4-group RCT: 1) cessation counseling alone; 2) cessation counseling + 16 weeks of cessation medication; 3) cessation counseling + cessation medication + prequit counseling, and 4) cessation counseling + cessation medication + prequit counseling + maintenance phone counseling. This strategy would be less efficient than a factorial experiment in that a comparison of just two of the above combinations of ICs would require the same sample size to achieve adequate statistical power as would a factorial comparison of all four ICs. This is because in the additive RCT, each participant would be assigned to only one level of the treatment factor (one of the four combinations). Moreover, such a design would not distinguish between additive and interactive effects of the tested ICs.
Even if one were interested in evaluating multicomponent treatments, it is still possible that factorial designs could prove useful. For instance, it is entirely possible that one factor (Factor 1) in a factorial experiment could comprise active medication plus a collection of 3 counseling components (skill building, support, and motivational intervention) as one level, while the second level would comprise a different medication and different counseling elements. This factor, containing the two different multicomponent treatments, could appear in a factorial experiment alongside additional factors: e.g., Factor 2 could be the provision of an “active” smoking cessation focused website versus no website, and Factor 3 could be a duration factor, which would indicate whether the medication and counseling treatments contrasted in the first factor last either 26 or 8 weeks. Thus, this factorial experiment could provide a direct contrast of two multicomponential treatments such as ones that might be contrasted in an RCT (active medication + counseling versus placebo medication + counseling). In addition, it would explore the effects of other factors (the website or treatment duration) that might affect the outcome (e.g., long-term abstinence) through either main or interactive effects. It is important to note, though, that this strategy does not allow the investigator to determine the separate effects of the individual ICs that make up the multicomponent treatments: the counseling and medication ICs. Nor does it reveal how any of the ICs would work if used as a sole treatment (the main effects of other factors and interaction effects would, no doubt, influence the outcomes associated with any given component.)
In sum, unless the investigator has access to clearly relevant data (preferably from factorial experiments) s/he should have strong concerns about how the elements in a treatment (the ICs) might interact. Should 3 or 6 counseling sessions be used? Should counseling comprise both support and skill training? Should counseling be by phone or in-person? Should sessions be 10 or 30 minutes in length? Such uncertainty favors the use of a factorial design. Only factorial designs permit efficient screening of multiple ICs or dimensions (e.g., length of treatment), revealing their main effects and interactions and permitting the identification of those that are compatible or most promising ( Collins et al., 2016 ). However, factorial experiments do not permit strong inferences about how well a particular grouping of components (occurring as levels of different factors) will work as an integrated treatment as compared to a control. After all, only a small portion of a sample in a factorial experiment will get a particular set of components (e.g., in the design depicted in Table 1 only 1/32 of the N will get a particular combination of components).
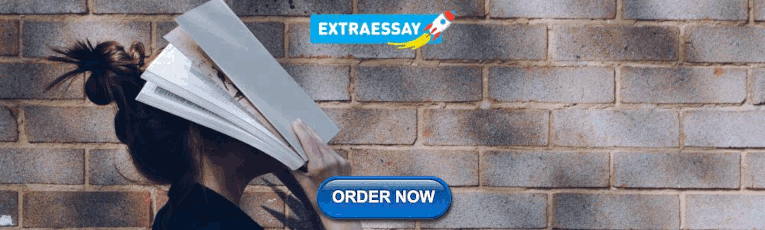
Effect Coding Effects
When taking a general linear model approach to the analysis of data from RCTs and factorial experiments, analysts must decide how to code categorical independent variables. This problem is avoided if an analysis of variance package is used, because such packages typically default to effect coding. However, as noted by Kugler et al. ( Kugler, Trail, Dziak, & Collins, 2012 ), in regression analyses investigators may use either dummy coding (also known as reference cell coding) or effect coding (also known as unweighted effects coding) (cf. Cohen, Cohen, West, & Aiken, 2003 ). In dummy coding, a binary variable, a reference group (e.g., a control group) is assigned a value of zero (0) and the other group (e.g., an active treatment group) is assigned a value of one (1). Effect coding of a binary variable is the same except that the zero for the reference group is replaced with −1.
When a study design has two or more factors and interactions are included in the models, dummy coding and effect coding yield the same overall model fit, but yield different estimates of component effects, which should be interpreted differently; i.e., the parameter estimates, standard errors, and statistical significance for both main and interaction effects may differ for models computed with the two types of coding ( Kugler et al., 2012 ). In general, when dummy coding is used, the effects corresponding to main effects in a standard ANOVA are similar to simple effects, i.e., the effect of a variable when all other variables in the model are set to the level coded as zero. A major concern with dummy coding is that a “main effect” in such models is actually a weighted combination of both the factor’s main effect and its interactions with other components; in other words, it does not correspond to the common interpretation of a main effect as being the effect of manipulating a factor averaged across the other factors. For instance, in the design depicted in Table 1 , the effect of Extended Medication would be reflected by the average effect of all Extended Medication conditions (1–16) versus the average effect of all Standard Medication conditions (17–32). With effect coding, when the experimental conditions have equal (or nearly equal) numbers of participants, the main effect of a factor does not reflect the effects of interaction effects that may be present in the data.
It is worthwhile noting that the presence of other factors (and ICs) in a factorial experiment can affect the level of outcome of any single factor. Thus, the abstinence rate averaged across all Extended Medication “on” conditions would reflect the effects of other components (e.g., Automated Phone Adherence Counseling: see Table 1 ). Investigators should remain cognizant of this and not assume that an IC will yield a similar outcome level (e.g., abstinence rate) when tested with different ICs (or none). (Significance tests of experimental effects [e.g., main effects, interaction effects] are designed to account for the other factors included in the experiment because these other factors contribute to the means of both the “on” and “off” levels of a factor [e.g., to the means of both the Extended and Standard Medication conditions]. However, even significance tests of a given factor can be affected by the other factors included in an experiment: See discussion below.)
Thus, investigators must decide if they wish to directly compare two treatment conditions (and these may be multicomponential) with one another, without the results being affected by the presence of other experimental factors being manipulated. If they wish to do so, they would choose an RCT. Or, if the effectiveness and compatibility of potentially important features of treatment have not been empirically established, and investigators wish to examine the effects of multiple ICs or dimensions simultaneously, allowing for the separate analysis of their main and interactive effects, they would choose a factorial design.
Achieving the Right Component Comparisons
We have stressed that a factorial design typically does not provide direct tests of different combinations of ICs and therefore limits inferences about such combinations. For example, let us assume that an investigator wishes to compare the nicotine patch and varenicline as smoking cessation agents, but also wants to draw conclusions about the effectiveness of the combination of those two agents ( Koegelenberg et al., 2014 ) relative to monotherapy (either agent by itself) and to combination NRT (e.g., patch plus lozenge). The investigator designs an experiment with the following factors: Factor 1: varenicline versus no varenicline; Factor 2: nicotine patch versus no nicotine patch; Factor 3: nicotine lozenge versus no nicotine lozenge. The crossing of the three medication factors means that participants in the experiment would get one of 8 combinations of the medications: no medication, varenicline alone, nicotine patch alone, nicotine lozenge alone, varenicline + patch, varenicline + lozenge, patch + lozenge, or all three agents. Note that this design allows the investigator to study the effects of the three individual agents versus its control (“off” level) , but, it does not directly contrast the various individual medication conditions with one another, and the combination medication conditions (e.g., varenicline + nicotine patch) are not directly contrasted with the other medication conditions nor with a control condition. Rather, the design would test for an interaction between these two agents; i.e., whether they produce effects that are significantly different from the sum of their main effects. One could make meaningful inferences about how well the agents would work together by examining the main effects of each treatment versus its corresponding control condition; positive effects of each in the absence of an interaction between them would allow one to conclude that they exert additive effects, and the size of these effects would convey information about the potential magnitude of their additive effects. Further information relative to their joint effects could be gained by examining the magnitude and direction of their interactive effects. But, it remains the case that the individual medications are not directly juxtaposed to one another in the above design, nor is their combination directly statistically contrasted with another medication condition.
In the above case the investigator might be better served by an RCT that contrasts three separate treatment groups that receive: varenicline, varenicline + the nicotine patch, and combination NRT, with each group receiving the same counseling intervention in addition to medication. Of course, this design would be resource-intensive and would not provide some of the information that would be yielded by a factorial design (e.g., whether NRT and varenicline interact with one another, the effect of the nicotine patch without another medication, whether all three medications produce additive effects). Also, with an RCT, it is possible that any differences observed amongst the contrasted medication conditions would actually reflect interactions between the medications and the features of the counseling intervention, and one would never know this. In a factorial design, counseling (On/Off) could be included as an additional factor.
The above dichotomy (RCT vs. completely crossed factorial design) does not exhaust the options available to investigators (e.g., factorial experiments with factors with more than two levels; which should be considered with care because they tend to be resource intensive: Collins et al., 2009 ). The main point is that in decisions about design choice, the investigator must identify the principal hypotheses and select an experimental design that makes the best use of available resources to test those hypotheses, recognizing that no single experimental design can efficiently address all possible research questions.
Selecting the Right Factors and Components in a Factorial Design: Design and Clinical Considerations
Selecting the right number of factors, challenges of numerous factors.
If an investigator decides to use a factorial design, s/he has numerous choices to make, including choices about the number and types of factors to include. The number of factors is important for several reasons.
Staff burden
It is tempting to take advantage of the efficiency of the factorial experiment and use it to evaluate many components since power is unrelated to the number of factors, and therefore, a single experiment can be used to screen many components. However, the number of factors used and the types and number of levels per factor can certainly affect staff burden. A 5-factor design with 2-levels/factor yields some 32 unique combinations of components ( Table 1 ), and requires that at least five different active or “on” ICs be delivered. Moreover, if instead of “off” or no-treatment conditions, less intensive levels of components are used, then even more ICs must be delivered (albeit some of reduced intensity). While staff can certainly deliver a very large number of components (e.g., Piper et al., 2016 ; Schlam et al., 2016 ), it can present challenges to staff as they must learn to deliver the many different combinations of components and deliver just the right combination to the right patients, at the right times. The extent of this challenge is, of course, affected by the nature of the ICs included; including a factor such as a longer medication duration may result in little additional burden for staff, but including additional counseling interventions might greatly increase the complexity of learning and delivering ICs.
In our experience staff burden tends to be greater in our factorial experiments than in our RCTs, and the burden increases with the number of factors used. In a common form of RCT with two treatment conditions, both conditions might get the same counseling intervention, but one would get active medication and the other placebo medication. In essence, from the perspective of treatment staff, both treatment groups would receive exactly the same treatment. But, as noted above, in a factorial experiment with many factors, the staff must learn to deliver numerous combinations of components, with each combination needing to be well integrated and coherently delivered. This can be challenging since the components in a factorial experiment are not necessarily selected to be especially compatible with one another; they may represent distinct, alternative approaches to intervention, making their integration challenging when they co-occur (see “Selecting Factors: Factor and Intervention Component Compatibility” below).
Patient burden
A large number of ICs can also increase burden on participants. This burden may occur in various ways; increased visits, more assessments (to measure mechanisms of change), more work (e.g., carrying out prescribed activities, homework), a greater learning demand, and simply more time spent in treatment. Of course, this burden might be apparent only in certain conditions of an experiment (those exposed to large numbers of “on” components). With multiple, moderately intense IC’s, the differences in treatment contact, and perhaps burden, amongst treatment conditions can become quite marked. In the Schlam 5-factor experiment (e.g., Schlam et al., 2016 : Table 1 ), at the extremes, some participants were scheduled to receive 30 more intervention contacts than were others (who were assigned to few “on” levels of factors.) Clearly, this burden could increase attrition or noncompliance.
Clinical relevance and generalizability
The number of ICs may affect the clinical relevance and generalizability of the research findings. Increased numbers of ICs and assessments may create nonspecific or attentional effects that distort component effects. For instance, while a real world application of a treatment might involve the administration of only two bundled ICs (counseling + medication), a factorial experiment might involve 6 or more ICs. Such a large number of ICs (admittedly only a portion of all subjects would get 4 or more) could either enhance outcomes with regard to the main effects of some factors (e.g., due to increased contact or involvement with the treatment team) or degrade them (due to overlap/redundancy of component effects, or alternatively fatigue, frustration, or simple inattention to critical therapeutic elements; see ( Fraser et al., 2014 ) for an example of the last). Such effects would be manifest in interactions amongst components (e.g., the effectiveness of a component might be reduced when it is paired with other components) or in increased data missingness. Either of these influences could affect main effects. Moreover, if higher order interactions are not examined in models, researchers will not know if an intervention component is intrinsically weak (or strong) or is meaningfully affected by negative (or positive) interactions with other factors. Of course, burden and interactions amongst components might certainly affect outcomes in an RCT, but the intent of the RCT is to determine whether the treatment works as a whole (not to evaluate promise of individual ICs); thus such effects would merely be factored into the net effects of the treatment.
Finally, including numerous ICs in an experiment could cause staff or counselors to spontaneously adjust their delivery of an intervention component because of their awareness of the total intensity of treatment provided to a participant. This could certainly affect the external validity of the results. Counselors could either reduce the intensity of an intervention component when it is one of many that a participant receives, or they could increase the intensity of an intervention component if the participant is receiving little other treatment. Of course, problems with intervention fidelity may occur in RCTs as well as in factorial experiments, but they may be a greater problem in the latter where differences in treatment intensity can be much more marked and salient (e.g., four “on” counseling components versus one). In short, maintaining treatment delivery fidelity may take more care, training and supervision in a factorial experiment than in an RCT.
Statistical Significance
Including additional factors in an experiment might certainly affect the significance tests of a given factor. For instance, if the added factors have very weak effects (i.e., the outcome is unchanged whether the levels are “on” or “off”), then their presence will reduce power because they reduce the degrees of freedom in the estimate of sigma (part of the denominator of the t statistic). The reduction in power will be greater if we control experiment-wise error, due to the larger number of main and interaction effects. On the other hand, if the additional components have strong effects (i.e., the outcome changes substantially between the “on” and “off” levels of each factor), then their presence should reduce the estimate of sigma (all other things being equal) and hence increase the value of the t-statistic (often overwhelming the loss of degrees of freedom). Including numerous factors might also increase the occurrence of interactions, which might affect the magnitude of a main effect (despite the lack of correlation between main and interaction effects with effect coding). For instance, if the “on” level of Factor A is highly effective at the “off” level of Factor B, but ineffective at the “on” level of Factor B, this will certainly influence the magnitude of the main effect of Factor A versus the situation where Factor B was not included in the experiment and all participants were given instead what was the “off” level of Factor B.
Finally, it is important to note that if investigators include multiple, discrete IC’s in a factorial experiment the effects of the individual ICs may be limited to the extent that the various ICs exert their effects via similar or redundant pathways ( Baker et al., 2016 ). Thus, to the extent that two ICs affect coping execution or withdrawal severity, their co-occurrence in the experiment could reduce estimates of their main effects via negative interaction. One might think of this as interventions “competing” for a limit subset of participants who are actually capable of change or improvement; in a sense this subsample would be spread across multiple active intervention components.
In sum, in a factorial experiment, the effects, relative effects, and statistical significance of ICs will likely change depending upon the number and types of components that co-occur in the experimental design. This arises, in part, from the fact that the effects of any given factor are defined by its average over the levels of the other factors in the experiment. It is important, therefore, for researchers to interpret the effects of a factorial experiment with regard to the context of the other experimental factors, their levels and effects. This does not reflect any sort of problem inherent in factorial designs; rather, it reflects the trade-offs to consider when designing factorial experiments.
Steps to Reduce the Burden of Multiple Components
The staff burden posed by multiple interventions may be addressed somewhat by use of dynamic databases that guide intervention delivery by staff and by using automated ICs (e.g., via automated phone calls) or automated assessments (e.g., automated emails to prompt completion of an online survey).
The choice of control conditions can also affect burden and complexity for both staff and patients. In this regard, “off” conditions (connoting a no-treatment control condition as one level of a factor) have certain advantages. They are relatively easy to implement, they do not add burden to the participants, and they should maximize sensitivity to experimental effects (versus a low-treatment control). Of course, less intensive (versus no-treatment) control conditions might be used for substantive reasons or because they ensure that every participant gets at least some treatment.
In addition, the complexity of delivering multiple combinations of components can be reduced by using a fractional factorial design ( Collins et al., 2009 ), which reduces the number of different component combinations per the number of factors used. While implementing fewer combinations of components can make an experiment easier to conduct, such designs confound some higher order interactions (with main effects and lower order interactions), and so should be used only when higher order interactions are believed to be negligible (i.e., approximately zero). While more research on IC interactions is surely needed, our research has consistently found such interactions ( Cook et al., 2016 ; Fraser et al., 2014 ; Piper et al., 2016 ; Schlam et al., 2016 ). Thus, it might be difficult in many cases to assume conditions that would justify the use of a fractional factorial design.
One point that the investigator should keep in mind regarding the increased burden imposed by numerous intervention components is that if the burden is really imposed by requirements of treatment (versus, say assessment related to research needs), then the burden or treatment complexity reflects the nature of the multiple ICs as they would occur in real world circumstances. The different ICs when used in real world settings would entail different amounts of contact or different delivery routes and their net real world effects would reflect these influences. Thus, it is important to recognize that such effects do not really constitute experimental artifacts, but rather presage the costs of complex treatments as used in real world application, presumably something worth knowing.
Advantages and Adaptations to Multiple Factors
Factorial designs can pose challenges, but they offer important advantages that can offset such challenges. Of course, there is increased efficiency as investigators can screen more components at a reduced expenditure of resources. In addition, even if large numbers of ICs produce burden, the investigator can powerfully explore the relation between burden and outcome by examining how outcomes or adherence are related to measures of burden (e.g., total contacts entailed, number of active ICs assigned, or total estimated time required). While burden may affect outcomes in a multicomponent treatment that is evaluated in an RCT, the investigator in an RCT typically has much weaker strategies to investigate it since all participants are typically exposed to very similar levels of burden (counseling + active medication versus counseling + placebo medication).
In addition, the use of a large number of factors allows for built-in evaluations of the robustness of the main effects of the ICs. This is because, as noted earlier, such effects are determined by averaging over the other component effects (with effect coding). As Fisher observed ( Fisher, 1971 ) (also see Collins et al., 2009 ; Finney, 1955 ), if a main effect emerges against a backdrop of the averaged effects of other components, it demonstrates the robustness of such effects across considerable variability, akin to the demonstration of reliability across treatment facets as per generalizability theory ( Brennan, 2001 ). Therefore, main effects that are resilient with regard to the effects of multiple other ICs, might be resilient to variability in real world clinical settings (although experimental factors typically do not resemble the number and types of environmental influences that often reduce external validity ( Couper, Hosking, Cisler, Gastfriend, & Kivlahan, 2005 ). In sum, despite some complexities, factorial experiments remain the most efficient means of determining the relative effectiveness of multiple components, setting their levels of intensity, and determining how well they work together ( Chakraborty, Collins, Strecher, & Murphy, 2009 ).
Selecting Factors: Factor and Intervention Component Compatibility
There are multiple issues to consider when selecting which particular factors and ICs to use in a factorial experiment. In an RCT, one would presumably evaluate a treatment that has been shown to work as a unified whole, or that shows great promise of doing so; its constituents are selected to work well together. In a factorial experiment though, one might select components that reflect distinct alternatives to one another (i.e., that reflect alternative views of change mechanisms or incompatible intervention dimensions: 8 vs. 26 weeks of treatment). Because factorial experiments can be used to screen multiple, potentially divergent components, it is important to consider whether such components will be compatible or “mergeable” with one another. Components that reflect divergent approaches to treatment might produce a combination that makes little sense theoretically or clinically, or that is confusing to participants. For example, counseling strategies that emphasize immediate, absolute abstinence could not be effectively meshed with approaches that emphasize gradual smoking reduction. Similarly, highly directive and nondirective counseling strategies might create a confusing amalgam for participants. One might try to avoid incompatibility by making two conflicting combinations different levels of the same factor, in which case participants would get one or the other, but not both. This means, however, that neither component would be paired with a no-treatment control, which could reduce power (if each component is at least somewhat effective) and compromise inference (e.g., in the case of nonsignificance one could not determine if both or neither component was effective).
If an investigator anticipates severe problems from including a particular factor in an experiment, perhaps due to its nature or the burden entailed, s/he should certainly consider dropping it as an experimental factor. Indeed, the MOST approach to the use of factorial designs holds that such designs be used to decompose a set of compatible ICs, ones that might all fit well in an integrated treatment package (to identify those that are most promising). That is, one should include only those ICs that are thought to be compatible, not competitive.
Adjusting ICs to Achieve Compatibility: Potential Costs
Investigators may wish to adjust ICs to enhance their compatibility with other components. For instance, investigators might choose to reduce the burden of an IC by cutting sessions or contact times. This might reduce the meaning of the factor because it might make the IC unnecessarily ineffective or unrepresentative.
Alternatively, an investigator might modify an intervention when it co-occurs with a particular, second intervention component. For instance, assume that a design has three factors; two are medication factors (e.g., varenicline, on/off, in one factor and NRT product [nicotine patch vs. nicotine lozenge], in a second factor). The third factor is an adherence factor (i.e., an automated medication counter with counseling, on/off). Thus, this experiment would address which type of NRT exerts additive or interactive effects when used with varenicline, and whether the adherence intervention exerts main or interactive effects. The investigator might tailor the adherence factor so that it is appropriate for the different types of medication that the participant is to receive (use instructions, delivery systems, and adverse events are very different for the different types of medication ( Fiore et al., 2008 ). Obviously the investigator must make the intervention relevant to each medication type, but such adjustment raises questions. Is the adherence intervention different enough in its various forms (across medications) so that it no longer constitutes a single, coherent component? If that is true, its effects cannot be interpreted in a straightforward manner. For instance, if an interaction were found between the two medication factors and the adherence component, is it because the adherence intervention was made more or less effective due to the way it was changed, or instead because of differences intrinsic to type of medication (e.g., side effects strongly constrain adherent use for one medication and not the other)? If no adherence main effect is found, is that because this component was inconsistently delivered (adjusted for each medication)? In sum, investigators should be cognizant of the possible effects of such intervention adjustment and consider options for addressing them (e.g., by making only essential adjustments to a component, nesting an adjusted factor in the design).
We have stressed the notion that adjusting ICs can compromise interpretation. However, a failure to integrate ICs can also exact costs. How meaningful would it be to test an adherence IC that did not address the specific or characteristic challenges that pertain to the particular medication being used by a patient? In sum, the manipulation of multiple ICs may require balancing conflicting goals: viz. how to keep ICs consistent and stable each time they are used; how to make them clinically meaningful and distinct, but integrated with the other components with which they might be paired; and how to set their intensity so they are clinically meaningful but not too burdensome. Such balancing is done routinely in developing a single integrated treatment for RCT evaluation; it presents additional challenges when numerous, and perhaps dissimilar, components are evaluated in a factorial experiment yielding 30 or more IC combinations. In short, the investigator must balance the desire for a uniform intervention with its compatibility with co-occurring components, recognizing that a significant change in a factor level, contingent upon its co-occurrence with other factors, challenges the inferences that might be drawn from a factorial design.
Data analysis and Interpretation
Certainly any research evaluation of intervention effectiveness can pose analytic and interpretive challenges. However, some challenges are of particular relevance to factorial designs.
Experimentwise error
Experimentwise error may be more of a problem in factorial designs than in RCTs because multiple main and interactive effects are typically examined. In a 5-factor experiment there are 31 main and interaction effects for a single outcome variable, and more if an outcome is measured at repeated time points and analyzed in a longitudinal model with additional time effects. If more than one outcome variable is used in analyses, the number of models computed and effects tested grow quickly. Various approaches have been suggested for dealing with the challenge posed by so many statistical comparisons being afforded by complex factorial designs ( Couper et al., 2005 ; Green, Liu, & O’Sullivan, 2002 ). However, it is important to note that if a factorial experiment has been conducted for the purpose of screening multiple ICs to identify those that are most promising (as per the MOST approach), then statistical significance should probably be viewed as a secondary criterion. As opposed to an RCT, where the focus is on demonstrating effects that are highly unlikely to be due to chance, the screening experiment is focused on relative promise of the tested ICs.
Mediators and nonfactorial influences
Investigators using factorial designs may wish to pay particular attention to the assessment of two types of dependent measures other that the primary outcomes: 1) mediators, and 2) variables that may potentially index unintended influences on outcomes. Mediators may be particularly informative in factorial designs since mediational influences can be associated precisely to particular, relatively discrete ICs and their interactions. For instance, in an RCT one treatment condition might get active medication, two kinds of cessation counseling (skill training and intratreatment support), and extended maintenance or relapse prevention phone calls. The other arm or condition in the RCT would receive the same intervention but with placebo instead of active medication. Conducting mediation analyses using the RCT data might reveal that increased self-efficacy and decreased craving appear to mediate the beneficial effects of active medication on long-term abstinence. However, it is unclear that it is really the main effect of medication that accounts for long term abstinence. Since medication is bundled with other ICs it is impossible to determine if its effects are due to the medication per se or instead due to its interactions with other ICs (medication may allow patients to benefit more from counseling). Thus, by systematically manipulating the provision of relatively discrete, individual ICs, factorial experiments may allow investigators to achieve a better understanding of how ICs work, an understanding that may be invaluable for combining them so that they activate complementary mechanisms.
Investigators may also wish to include measures in their factorial experiments that assess potential alternative explanations for their findings. We have discussed how the manipulation of multiple treatment factors might create unintended effects due to overall burden, inducement of optimism, apparent incompatibility of components or delivery routes, differential staff delivery, and so on. Investigators should consider using measures that would be sensitive to such effects. For instance, investigators might assess measures of burden (treatment fatigue) and determine if these are especially highly related to particular ICs or to an increasing number of ICs. Indeed, even without the use of special assessments, investigators might correlate the number of ICs a person receives (regardless of type) to outcomes. Interestingly, in our factorial research thus far, the best outcomes (across multiple experiments) tend to be produced by combinations of two ICs, not more ( Cook et al., 2016 ; Fraser et al., 2014 ; Piper et al., 2016 ; Schlam et al., 2016 ), suggesting the possible influence of burden, or ceiling effects (e.g., the ICs produce effects via redundant mechanisms).
Interactions: Challenges to Interpretation
Chakraborty et al., ( Chakraborty et al., 2009 ) noted that factorial designs may not perform optimally for intervention selection in cases where there are weak main effects, but relatively strong interaction effects. Unfortunately, this situation may be a fairly common occurrence in factorial experiments of clinical interventions (e.g., Cook et al., 2016 ; Piper et al., 2016 ; Schlam et al., 2016 ).
Complex interactions can produce several challenges. For instance, some interactions may be due to the overall burden due to subjects receiving large numbers of components. This might result in subadditive or negative interactions in which interventions produce less benefit, or even produce net decreases in benefit, when they co-occur with another intervention(s). This can pose interpretive challenges as it may be difficult to separate the effects of a component per se from the impact of burden. In addition, a higher order interaction may not be due to a single uniquely performing combination of ICs, but rather due to multiple combinations of ICs, some of which may overperform, and others underperform, in relation to what is implied by relevant main effects and lower order interactions.
Figure 1 , (from Cook et al., 2016 ) arises from a 4-factor experiment that illustrates some of the challenges of interpreting interactions for the purpose of identifying especially promising ICs. This figure shows the data patterns associated with a significant interaction amongst factors delivered to smokers who were not yet willing to make a quit attempt, but who were willing to try to reduce their smoking. In theory, smoking reduction should ultimately lead to quit attempts and greater quitting success (cf. Baker et al., 2011 ; Moore et al., 2009 ). The four factors were: Nicotine Gum (on/off), Nicotine Patch (on/off), Behavioral Reduction Counseling (on/off), and Motivational Interviewing (on/off). This interaction was generated by an analysis of covariance on percent reduction in smoking rate (cigarettes smoked/day) that occurred from baseline to 12 Weeks after the start of treatment. Figure 1 shows that it is difficult to definitively identify the most promising combination of ICs. In essence, three 2-component combinations look essentially indistinguishable in terms of smoking reduction: Nicotine Gum + Behavioral Reduction, Nicotine Gum + Nicotine Patch, and Behavioral Reduction + Motivational Interviewing. Because all four of the components are involved in one or more of the best performing combinations, it might be tempting to think that the optimal combination would comprise all four. Figure 1 shows that this is not the case as the combination of all four performed relatively poorly. Thus, interaction effects may not highlight a clear “winner” in terms of the most promising IC(s). (Moreover, interactions do not directly test whether a combination of components is superior to the condition where all factors are set to the “off” level.)

Note. This figure describes the results of a four-factor factorial experiment ( Cook et al., 2016 ) and depicts the data patterns that reflect the significant 4-way interaction found in the experiment. Participants were smokers who were trying to reduce their smoking and the outcome is mean percent smoking reduction 12 weeks after treatment initiation. The four factors were: “Gum” = Nicotine Gum vs. “No Gum”; “Patch” = Nicotine Patch vs. “No Patch”; “BR” = Behavioral Reduction Counseling vs. “No BR”; and “MI” = Motivational Interviewing vs. “No MI”.
It is important to note that interpretation of complex higher order interactions may not be aided by simple effects testing. First, it is highly unlikely that such testing would have distinguished amongst the three leading combinations shown in Figure 1 (the differences in outcomes are too small). Second, such tests would have been grievously underpowered, and increasing the sample size to supply the needed power would have compromised the efficiency of the factorial design ( Green et al., 2002 ). Thus, instead of using simple effects tests, researchers might interpret interaction effects via practices used in engineering; i.e., by inspecting differences in performance of one or more ICs across levels of other relevant ICs, and then relating this information to relevant main effects (cf. Box et al., 2005 ; Cox & Reid, 2000 ). This, of course, has limitations, such as not permitting strong inference regarding the source(s) of the interaction.
Higher order interactions can reflect complex patterns that defy easy interpretation. However, they also reveal information that is unique and of potentially great value. Further, this problem is reduced if factorial designs are used as screening experiments, whose purpose is not to identify the single best combination of ICs ( Collins et al., 2009 ). Rather such experiments are used to identify the ICs that are amongst the best. Therefore, finding that several combinations of ICs yield promising effects is compatible with the goal of a screening experiment, which is to distill the number of ICS to those holding relatively great promise. In keeping with this, the data in Figure 1 suggest that we can winnow potentially promising combinations from 16, to 3. Which one of those three might be deemed most promising might be addressed via other criteria (effects on abstinence, costs, and so on) and in a follow-up RCT.
Privileging Main Effects
The challenges that may arise in interpreting interactions support strategies to select ICs based chiefly on main effects ( Collins et al., 2009 ; Wu & Hamada, 2011 ), which is the approach taken in engineering and other fields that use screening experiments ( Wu & Hamada, 2011 ). This approach has several advantages. For instance, relative to some complex interactions, main effects are more easily interpreted ( Collins et al., 2014 ); a factor’s main effects are interpretable even when it interacts with other factors. When effect coding is used, each effect is orthogonal to every other effect in the analysis model (orthogonal when the n’s are equal in each experimental condition, and nearly orthogonal when the n’s differ by a modest amount). Thus, a significant main effect reflects an experimental effect that occurs on average across all other factors in the model even when the relevant factor is involved in significant interactions ( Chakraborty et al., 2009 ). (Interactions should be considered in interpretations and selection; they just do not invalidate the main effect.) Collins and her colleagues have proposed relatively straightforward steps for identifying promising components, steps that arise from engineering research and that prioritize main effects versus interactions ( Collins et al., 2014 ).
In addition, the efficiency of a factorial experiment depends in part on the extent to which higher order interactions are not found. If interactions are found, and inferential statistics must be used to unpackage such interactions, such simple effects tests would require examining the effects of ICs in only subgroups of the sample. In essence, if it is necessary to follow-up an interaction by identifying which particular subgroups differ from one another, some of the efficiency of the factorial design may be lost. However, it is important to note that interaction effects can be highly informative without simple effects tests ( Baker et al., 2016 ; Box et al., 2005 ).
Conclusions
Ambitious, multifactor, factorial experiments designed to evaluate clinical ICs can and do work for the purpose of intervention component screening ( Baker et al., 2016 ; Collins et al., 2016 ; Collins, Murphy, & Strecher, 2007 ; Fraser et al., 2014 ). We are confident, based upon our conduct of several factorial experiments on clinical interventions ( Baker et al., 2016 ; Cook et al., 2016 ; Fraser et al., 2014 ; Piper et al., 2016 ; Schlam et al., 2016 ), that such experiments can be designed and used to identify ICs that are especially worthy of further investigation with an eye to assembling an optimized treatment package ( Collins et al., 2016 ). We believe that their potential to yield unique data, and to do so efficiently, should make factorial screening experiments a core strategy in the process of developing effective treatments ( Collins et al., 2016 ). For instance, not only do such designs permit the screening of multiple intervention components in a single experiment, but compared with RCT designs, factorial experiments permit more precise estimates of mediational effects. This paper highlights decisions and challenges related to the use of factorial designs, with the expectation that their careful consideration will improve the design, implementation, and interpretation of factorial experiments.
- Factorial designs are highly efficient but offer special challenges
- Review of issues that determine whether a factorial design is appropriate
- Review of problems may arise when using factorial designs (e.g., interaction effects)
- Strategies for addressing the challenges that may arise from factorial designs
Acknowledgments
This research was supported by grants 9P50CA143188 and 1K05CA139871, and R35 CA197573, from the National Cancer Institute to the University of Wisconsin Center for Tobacco Research and Intervention and by the Wisconsin Partnership Program. Dr. Collins is also supported by NIH grants P50DA039838, R01DK097364, and R01AA022931. Dr. Loh is also supported by NSF grant DMS-1305725.
The authors, other than Dr. Loh, have received no direct or indirect funding from, nor do they have a connection with, the tobacco, alcohol, pharmaceutical or gaming industries or anybody substantially funded by one of these organizations. Dr. Loh conducts research and consults for the pharmaceutical industry on statistical methodology, but the activities are unrelated to smoking or tobacco dependence treatment.
Publisher's Disclaimer: This is a PDF file of an unedited manuscript that has been accepted for publication. As a service to our customers we are providing this early version of the manuscript. The manuscript will undergo copyediting, typesetting, and review of the resulting proof before it is published in its final citable form. Please note that during the production process errors may be discovered which could affect the content, and all legal disclaimers that apply to the journal pertain.
- Baker TB, Collins LM, Mermelstein R, Piper ME, Schlam TR, Cook JW, Fiore MC. Enhancing the effectiveness of smoking treatment research: conceptual bases and progress. Addiction. 2016; 111 (1):107–116. doi: 10.1111/add.13154. [ PMC free article ] [ PubMed ] [ CrossRef ] [ Google Scholar ]
- Baker TB, Mermelstein R, Collins LM, Piper ME, Jorenby DE, Smith SS, Fiore MC. New methods for tobacco dependence treatment research. Annals of Behavioral Medicine. 2011; 41 (2):192–207. doi: 10.1007/s12160-010-9252-y. [ PMC free article ] [ PubMed ] [ CrossRef ] [ Google Scholar ]
- Box GEP, Hunter WG, Hunter JS. Statistics for experiementers: design, innovation and discovery. 2nd. Hoboken, NJ: Wiley-Interscience; 2005. [ Google Scholar ]
- Brennan RL. Generalizability theory. New York, NY: Springer-Verlag; 2001. [ Google Scholar ]
- Chakraborty B, Collins LM, Strecher VJ, Murphy SA. Developing multicomponent interventions using fractional factorial designs. Statistics in Medicine. 2009; 28 (21):2687–2708. doi: 10.1002/sim.3643. [ PMC free article ] [ PubMed ] [ CrossRef ] [ Google Scholar ]
- Cohen J, Cohen P, West SG, Aiken LS. Applied multiple regression/correlation analysis in the behavioral sciences. 3rd. Malwah, NJ: Lawrence Erlbaum Associates; 2003. [ Google Scholar ]
- Collins LM, Dziak JJ, Li R. Design of experiments with multiple independent variables: a resource management perspective on complete and reduced factorial designs. Psychological Methods. 2009; 14 (3):202–224. doi: 10.1037/a0015826. [ PMC free article ] [ PubMed ] [ CrossRef ] [ Google Scholar ]
- Collins LM, Kugler KC, Gwadz MV. Optimization of multicomponent behavioral and biobehavioral interventions for the prevention and treatment of HIV/AIDS. AIDS and Behavior. 2016; 20 (Suppl 1):197–214. doi: 10.1007/s10461-015-1145-4. [ PMC free article ] [ PubMed ] [ CrossRef ] [ Google Scholar ]
- Collins LM, Murphy SA, Strecher V. The multiphase optimization strategy (MOST) and the sequential multiple assignment randomized trial (SMART): new methods for more potent eHealth interventions. American Journal of Preventive Medicine. 2007; 32 (5 Suppl):S112–118. doi: 10.1016/j.amepre.2007.01.022. [ PMC free article ] [ PubMed ] [ CrossRef ] [ Google Scholar ]
- Collins LM, Trail JB, Kugler KC, Baker TB, Piper ME, Mermelstein RJ. Evaluating individual intervention components: making decisions based on the results of a factorial screening experiment. Translational Behavioral Medicine. 2014; 4 (3):238–251. doi: 10.1007/s13142-013-0239-7. [ PMC free article ] [ PubMed ] [ CrossRef ] [ Google Scholar ]
- Cook JW, Collins LM, Fiore MC, Smith SS, Fraser D, Bolt DM, Mermelstein R. Comparative effectiveness of motivation phase intervention components for use with smokers unwilling to quit: a factorial screening experiment. Addiction. 2016; 111 (1):117–128. doi: 10.1111/add.13161. [ PMC free article ] [ PubMed ] [ CrossRef ] [ Google Scholar ]
- Couper DJ, Hosking JD, Cisler RA, Gastfriend DR, Kivlahan DR. Factorial designs in clinical trials: options for combination treatment studies. Journal of Studies on Alcohol. 2005; Supplement (15):24–32. discussion 26–27. Retrieved from http://www.ncbi.nlm.nih.gov/pubmed/16223053 . [ PubMed ] [ Google Scholar ]
- Cox DR, Reid N. The theory of the design of experiments. Boca Raton, FL: Chapman & Hall/CRC; 2000. [ Google Scholar ]
- Finney DJ. Experimental design and its statistical basis. Chicago, IL: Univ. of Chicago Press; 1955. [ Google Scholar ]
- Fiore MC, Jaen CR, Baker TB, Bailey WC, Benowitz N, Curry SJ, Wewers ME. Treating tobacco use and dependence: 2008 update. Rockville, MD: U.S. Department of Health and Human Services, U.S. Public Health Service; 2008. [ Google Scholar ]
- Fisher JO. The design of experiments. New York, NY: Hafner; 1971. [ Google Scholar ]
- Fraser D, Kobinsky K, Smith SS, Kramer J, Theobald WE, Baker TB. Five population-based interventions for smoking cessation: a MOST trial. Translational Behavioral Medicine. 2014; 4 (4):382–390. doi: 10.1007/s13142-014-0278-8. [ PMC free article ] [ PubMed ] [ CrossRef ] [ Google Scholar ]
- Friedman L, Furberg C, Demets D. Fundamentals of clinical trials. 4th. New York, NY: Springer; 2010. [ Google Scholar ]
- Glasgow RE. What does it mean to be pragmatic? Pragmatic methods, measures, and models to facilitate research translation. Health Education and Behavior. 2013; 40 (3):257–265. doi: 10.1177/1090198113486805. [ PubMed ] [ CrossRef ] [ Google Scholar ]
- Glasgow RE, Klesges LM, Dzewaltowski DA, Bull SS, Estabrooks P. The future of health behavior change research: what is needed to improve translation of research into health promotion practice? Annals of Behavioral Medicine. 2004; 27 (1):3–12. Retrieved from http://www.ncbi.nlm.nih.gov/entrez/query.fcgi?cmd=Retrieve&db=PubMed&dopt=Citation&list_uids=14979858 . [ PubMed ] [ Google Scholar ]
- Green S, Liu PY, O’Sullivan J. Factorial design considerations. Journal of Clinical Oncology. 2002; 20 (16):3424–3430. Retrieved from http://www.ncbi.nlm.nih.gov/pubmed/12177102 . [ PubMed ] [ Google Scholar ]
- Hernandez-Lopez M, Luciano MC, Bricker JB, Roales-Nieto JG, Montesinos F. Acceptance and commitment therapy for smoking cessation: a preliminary study of its effectiveness in comparison with cognitive behavioral therapy. Psychology of Addictive Behaviors. 2009; 23 (4):723–730. doi: 10.1037/a0017632. [ PubMed ] [ CrossRef ] [ Google Scholar ]
- Keppel G. Design and analysis: A researcher’s handbook. 3rd. Englewood Cliffs, NJ: Prentice-Hall, Inc; 1991. [ Google Scholar ]
- Koegelenberg CF, Noor F, Bateman ED, van Zyl-Smit RN, Bruning A, O’Brien JA, Irusen EM. Efficacy of varenicline combined with nicotine replacement therapy vs varenicline alone for smoking cessation: a randomized clinical trial. JAMA. 2014; 312 (2):155–161. doi: 10.1001/jama.2014.7195. [ PubMed ] [ CrossRef ] [ Google Scholar ]
- Kugler KC, Trail JB, Dziak JJ, Collins LM. Effect coding versus dummy coding in analysis of data from factorial experiments. University Park, PA: The Methodology Center, Pennsylvania State University; 2012. [ Google Scholar ]
- McClure JB, Peterson D, Derry H, Riggs K, Saint-Johnson J, Nair V, Shortreed SM. Exploring the “active ingredients” of an online smoking intervention: a randomized factorial trial. Nicotine & Tobacco Research. 2014; 16 (8):1129–1139. doi: 10.1093/ntr/ntu057. [ PMC free article ] [ PubMed ] [ CrossRef ] [ Google Scholar ]
- Moore D, Aveyard P, Connock M, Wang D, Fry-Smith A, Barton P. Effectiveness and safety of nicotine replacement therapy assisted reduction to stop smoking: systematic review and meta-analysis. British Medical Journal. 2009; 338 :b1024. doi: 10.1136/bmj.b1024. [ PMC free article ] [ PubMed ] [ CrossRef ] [ Google Scholar ]
- Piper ME, Fiore MC, Smith SS, Fraser D, Bolt DM, Collins LM, Baker TB. Identifying effective intervention components for smoking cessation: a factorial screening experiment. Addiction. 2016; 111 (1):129–141. doi: 10.1111/add.13162. [ PMC free article ] [ PubMed ] [ CrossRef ] [ Google Scholar ]
- Riley WT, Glasgow RE, Etheredge L, Abernethy AP. Rapid, responsive, relevant (R3) research: a call for a rapid learning health research enterprise. Clinical and TranslationalMedicine. 2013; 2 (1):10. doi: 10.1186/2001-1326-2-10. [ PMC free article ] [ PubMed ] [ CrossRef ] [ Google Scholar ]
- Schlam TR, Fiore MC, Smith SS, Fraser D, Bolt DM, Collins LM, Baker TB. Comparative effectiveness of intervention components for producing long-term abstinence from smoking: a factorial screening experiment. Addiction. 2016; 111 (1):142–155. doi: 10.1111/add.13153. [ PMC free article ] [ PubMed ] [ CrossRef ] [ Google Scholar ]
- Wu CF, Hamada M. Experiments: planning, analysis, and optimization. 2nd. New York: Wiley; 2011. [ Google Scholar ]
Academia.edu no longer supports Internet Explorer.
To browse Academia.edu and the wider internet faster and more securely, please take a few seconds to upgrade your browser .
Enter the email address you signed up with and we'll email you a reset link.
- We're Hiring!
- Help Center
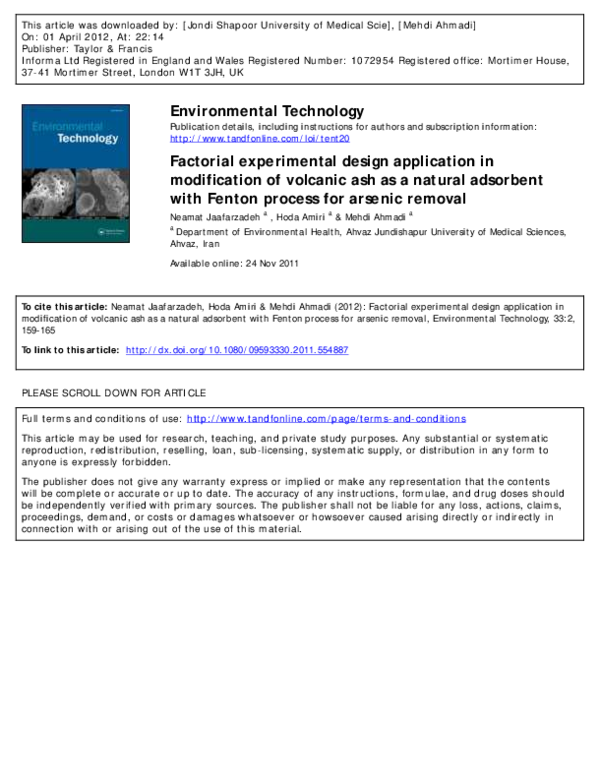
Factorial experimental design application in.pdf

Related Papers
Asian Journal of Chemistry
Dosu Malomo
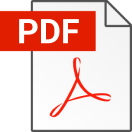
Applied Geochemistry
Zsolt A Berner
Yacouba SANOU
Arsenic pollution is one of issues for drinkable water supply in rural areas of Burkina Faso. The objective of this study was to look for a cheap technology for a better treatment of enriched arsenic water up to the admissible value (10 µg/L) in drinking water. To fulfil this objective, two mixed materials were prepared using a solid / solid mixture between laterite soil and granular ferric hydroxide for arsenic adsorption. Chemical analysis of laterite soil indicated a high amount of iron, aluminum and silicon. Batch experiments were conducted for As(V) adsorption using aqueous solutions. Results showed that the adsorption of arsenic (V) was strongly influenced by contact time, initial pH, adsorbent amount and initial As(V) concentration requiring their optimization. Indeed, the increase of the contact time between 5 and 90 min involved an increase of adsorption capacity up to 49.47µg/g while a change of initial pH caused a variation of adsorption capacity from 49 to 42.38 µg/g. An...
Iranica Journal of energy and environment
rahim yusoff
Journal of Chemistry
Branislava i Zoran Lekic
In this study, removal of arsenic ions using two industrial by-products as adsorbents is represented. Removal of As(III) and As(V) from water was carried out with industrial by-products: residual from the groundwater treatment process, iron-manganese oxide coated sand (IMOCS), and blast furnace slag from steel production (BFS), both inexpensive and locally available. In addition, the BFS was modified in order to minimise its deteriorating impact on the initial water quality. Kinetic and equilibrium studies were carried out using batch and fixed-bed column adsorption techniques under the conditions that are likely to occur in real water treatment systems. To evaluate the application for real groundwater treatment, the capacities of the selected materials were further compared to those exhibited by commercial sorbents, which were examined under the same experimental conditions. IMOCS was found to be a good and inexpensive sorbent for arsenic, while BFS and modified slag showed the hig...
Arsenic pollution is one of issues for drinkable water supply in rural areas of Burkina Faso. The objective of this study was to look for a cheap technology for a better treatment of enriched arsenic water up to the admissible value (10 μg/L) in drinking water. To fulfil this objective, two mixed materials were prepared using a solid / solid mixture between laterite soil and granular ferric hydroxide for arsenic adsorption. Chemical analysis of laterite soil indicated a high amount of iron, aluminum and silicon. Batch experiments were conducted for As(V) adsorption using aqueous solutions. Results showed that the adsorption of arsenic (V) was strongly influenced by contact time, initial pH, adsorbent amount and initial As(V) concentration requiring their optimization. Indeed, the increase of the contact time between 5 and 90 min involved an increase of adsorption capacity up to 49.47μg/g while a change of initial pH caused a variation of adsorption capacity from 49 to 42.38 μg/g. An...
Indian Journal of Chemical Technology
Kshipra Misra
Present study is carried out for the removal of As(III) from water using commonly available adsorbents such as sand, from Yamuna river ( Delhi ), as well as from Ganga river (Kolkata), activated carbon, Hametite ore and sand -iron scrap mixture. All these adsorbents are used as received but sand and activated carbon which do not show much adsorption for As(III) are modified by treating with different metal ions in order to improve their adsorption efficiency. Results of the laboratory experiments under static conditions have confirmed that iron impregnated granular activated carbon (GAC), spherical activated carbon (SAC) as well as sand - iron scrap mixture have much promise as a medium for the removal of As(III) in drinking water. Various parameters like adsorbent dose, contact time, pH and arsenic concentration are optimized. A simple and economical domestic arsenic removal kit has been designed and successfully evaluated in the laboratory using sand-iron scrap mixture as media fo...
Jorge Salinas C
Heat treatment and acid treatment methods have been tested on red mud to increase its arsenic adsorption capability. The results indicate that the adsorptive capacity of red mud can be increased by acid treatment. This treatment causes sodalite compounds to leach out. As(III) and As(V) adsorption characteristics of activated red mud have similar tendencies with raw red mud. Batch adsorption studies have shown that activated red mud in dosages ranging from 20 to 100 g l À1 can be used effectively to remove arsenic from aqueous solutions. The process is pH dependent, the optimum range being 5.8–7.5 for As(III) and 1.8–3.5 for As(V). The maximum removals are 96.52% for As(V) and 87.54% for As(III) for solutions with a final pH of 7.25 and 3.50, respectively, for the initial arsenic concentration of 133.5 mmol l À1 (10 mg l À1), activated red mud dosage of 20 g l À1 , contact time of 60 min and temperature of 25 C. The adsorption data obtained follow a first-order rate expression and fit the Langmuir isotherm well. Iso-therms have been used to obtain the thermodynamic parameters. It was found that the adsorption of As(III) was exothermic, whereas As(V) adsorption was endothermic. #
Water quality research …
Sushil Kanel
Loading Preview
Sorry, preview is currently unavailable. You can download the paper by clicking the button above.
RELATED PAPERS
Waste Management
HAMDİ SONER ALTUNDOĞAN
Asian Journal of Chemical Sciences
Hatem A Al-Aoh
Mehdi Ahmadi
International Journal of Phytoremediation
Azra Yasmin
Chemical Engineering Journal
Rainer Wennrich
Journal of Environmental Sciences
Maohong Fan
Polish Journal of Chemical Technology
Zygmunt Sadowski
Journal of Hazardous Materials
Hiroshi Kubo
Applied Sciences
Shin Dong Kim
The Canadian Journal of Chemical Engineering
Shahnawaz Sinha
Editor IJRET
Earth & Environmental Science Research & Reviews
Abdu H U S S E N Ali
Jorge Meichtry
Mohamed CHIBAN
Chemical Engineering Communications
Vladana Rajaković-ognjanović
TJPRC Publication
International Journal of ChemTech Research
Lokendra Singh Thakur
Tribhuvan University Journal
Megh Raj Pokhrel
Meththika Vithanage
Water Research
Anjali Gupta
Journal of the Chemical Society of Pakistan
Tahseen Aslam
- We're Hiring!
- Help Center
- Find new research papers in:
- Health Sciences
- Earth Sciences
- Cognitive Science
- Mathematics
- Computer Science
- Academia ©2024
Sequential good lattice point sets for computer experiments
- Published: 13 June 2024
Cite this article
- Xue-Ru Zhang 1 ,
- Yong-Dao Zhou 1 ,
- Min-Qian Liu 1 &
- Dennis K. J. Lin 2
1 Altmetric
Sequential Latin hypercube designs (SLHDs) have recently received great attention for computer experiments, with much of the research restricted to invariant spaces. The related systematic construction methods are inflexible, and algorithmic methods are ineffective for large designs. For designs in contracting spaces, systematic construction methods have not been investigated yet. This paper proposes a new method for constructing SLHDs via good lattice point sets in various experimental spaces. These designs are called sequential good lattice point (SGLP) sets. Moreover, we provide efficient approaches for identifying the (nearly) optimal SGLP sets under a given criterion. Combining the linear level permutation technique, we obtain a class of asymptotically optimal SLHDs in invariant spaces, where the L 1 -distance in each stage is either optimal or asymptotically optimal. Numerical results demonstrate that the SGLP set has a better space-filling property than the existing SLHDs in invariant spaces. It is also shown that SGLP sets have less computational complexity and more adaptability.
This is a preview of subscription content, log in via an institution to check access.
Access this article
Price includes VAT (Russian Federation)
Instant access to the full article PDF.
Rent this article via DeepDyve
Institutional subscriptions
Chen D J, Xiong S F. Flexible nested Latin hypercube designs for computer experiments. J Qual Technol, 2021, 49: 337–353
Article Google Scholar
Fang K T, Liu M Q, Qin H, et al. Theory and Application of Uniform Experimental Designs. Singapore: Springer/Beijing: Science Press, 2018
Book Google Scholar
Fang K T, Wang Y. A sequential algorithm for optimization and its application to regression analysis. In: Lecture Notes in Contemporary Mathematics. Beijing: Science Press, 1990, 17–28
Google Scholar
Hou R Z, Lu L. MOLHD: Multiple objective Latin hypercube design. R package version 0.2, http://CRAN.R-project.org/package=MOLHD
Hua L K, Wang Y. Application of Number Theory to Numberial Analysis. Berlin: Springer/Beijing: Science Press, 1981
Huang C M, Lee Y J, Lin D K J, et al. Model selection for support vector machines via uniform design. Comput Statist Data Anal, 2007, 52: 335–346
Article MathSciNet Google Scholar
Johnson M E, Moore L M, Ylvisaker D. Minimax and maximin distance designs. J Statist Plann Inference, 1990, 26: 131–148
Joseph V R. Space-filling designs for computer experiments: A review. Qual Engrg, 2016, 28: 28–35
Kong X, Ai M, Tsui K L. Flexible sliced designs for computer experiments. Ann Inst Statist Math, 2018, 70: 1–16
Korobov N M. The approximate computation of multiple integrals. Dokl Akad Nauk SSSR, 1959, 124: 1207–1210
MathSciNet Google Scholar
Lam C Q, Notz W I. Sequential adaptive designs in computer experiments for response surface model fit. Statist Appl, 2008, 6: 207–233
Liu H, Xu S, Wang X. Sequential sampling designs based on space reduction. Eng Optim, 2015, 47: 867–884
Loeppky J L, Moore L M, Williams B J. Batch sequential designs for computer experiments. J Statist Plann Inference, 2010, 140: 1452–1464
McKay M D, Beckman R J, Conover W J. Comparison of three methods for selecting values of input variables in the analysis of output from a computer code. Technometrics, 1979, 33: 239–245
Niederreiter H, Peart P. Localization of search in quasi-Monte Carlo methods for global optimization. SIAM J Sci Stat Comput, 1986, 7: 660–664
Qian P Z G, Ai M, Wu C F J. Construction of nested space-filling designs. Ann Statist, 2009, 37: 3616–3643
Qian P Z G, Tang B, Wu C F J. Nested space-filling designs for computer experiments with two levels of accuracy. Statist Sinica, 2009, 19: 287–300
Qian P Z G, Wu C F J. Bayesian hierarchical modeling for integrating low-accuracy and high-accuracy experiments. Technometrics, 2008, 50: 192–204
Ranjan P, Bingham D, Michailidis G. Sequential experiment design for contour estimation from complex computer codes. Technometrics, 2008, 50: 527–541
Rennen G, Husslage B, Van Dam E R, et al. Nested maximin Latin hypercube designs. Struct Multidiscip Optim, 2010, 41: 371–395
Santner T J, Williams B J, Notz W I. The Design and Analysis of Computer Experiments, 2nd ed. New York: Springer, 2018
Sun F S, Liu M Q, Qian P Z G. On the construction of nested space-filling designs. Ann Statist, 2014, 42: 1394–1425
Wang G G. Adaptive response surface method using inherited Latin hypercube design points. J Mech Des, 2003, 125: 210–220
Williams B J, Santner T J, Notz W I. Sequential design of computer experiments to minimize integrated response functions. Statist Sinica, 2000, 10: 1133–1152
Yang J Y, Liu M Q, Lin D K J. Construction of nested orthogonal Latin hypercube designs. Statist Sinica, 2014, 24: 211–219
Zhou X J, Lin D K J, Hu X L, et al. Sequential Latin hypercube design with both space-filling and projective properties. Qual Reliab Eng Int, 2019, 35: 1941–1951
Zhou Y D, Fang K T, Ning J H. Mixture discrepancy for quasi-random point sets. J Complexity, 2013, 29: 283–301
Zhou Y D, Xu H. Space-filling fractional factorial designs. J Amer Statist Assoc, 2014, 109: 1134–1144
Zhou Y D, Xu H. Space-filling properties of good lattice point sets. Biometrika, 2015, 102: 959–966
Download references
Acknowledgements
This work was supported by National Natural Science Foundation of China (Grant Nos. 11871288, 12131001, and 12226343), National Ten Thousand Talents Program, Fundamental Research Funds for the Central Universities, China Scholarship Council and U.S. National Science Foundation (Grant No. DMS-18102925). The authors thank the referees for their helpful comments and suggestions. The first two authors contributed equally to this work.
Author information
Authors and affiliations.
NITFID, LPMC & KLMDASR, School of Statistics and Data Science, Nankai University, Tianjin, 300071, China
Xue-Ru Zhang, Yong-Dao Zhou & Min-Qian Liu
Department of Statistics, Purdue University, West Lafayette, IN, 47907, USA
Dennis K. J. Lin
You can also search for this author in PubMed Google Scholar
Corresponding author
Correspondence to Min-Qian Liu .
Rights and permissions
Reprints and permissions
About this article
Zhang, XR., Zhou, YD., Liu, MQ. et al. Sequential good lattice point sets for computer experiments. Sci. China Math. (2024). https://doi.org/10.1007/s11425-021-2087-2
Download citation
Received : 10 November 2021
Accepted : 11 January 2023
Published : 13 June 2024
DOI : https://doi.org/10.1007/s11425-021-2087-2
Share this article
Anyone you share the following link with will be able to read this content:
Sorry, a shareable link is not currently available for this article.
Provided by the Springer Nature SharedIt content-sharing initiative
- contracting space
- maximin distance
- nested Latin hypercube design
- sequential design
- space-filling design
- Find a journal
- Publish with us
- Track your research
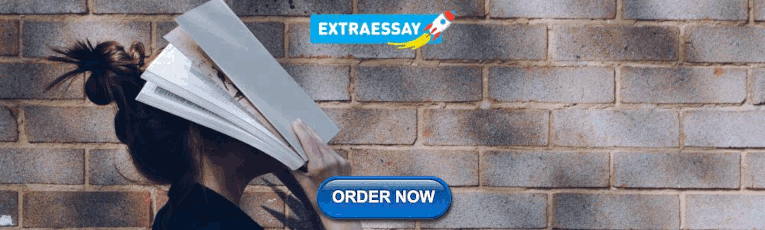
IMAGES
VIDEO
COMMENTS
The factorial analysis implies that the exp. units assigned to pH1 have something in common that differentiate them from the e.u. assigned to pH2. In the nested design batch 1 in S1 is equally related to batches 1 and 2 in S2. 9. 7. The two-way factorial analysis 9. 7. 1. The linear model for two-way factorial experiments
2n. factorial experiment, the analysis of variance involves the partitioning of treatment sum of squares so as to obtain sum of squares due to main and interaction effects of factors. These sum of squares are mutually orthogonal, so Treatment SS = Total of SS due to main and interaction effects.
Contents Preface xvii 1 Introduction 1 1.1 Why Experiment? . . . . . . . . . . . . . . . . . . . . . . 1 1.2 Components of an Experiment . . . . . . . . . . . . . . . 4
4 FACTORIAL DESIGNS 4.1 Two Factor Factorial Designs A two-factor factorial design is an experimental design in which data is collected for all possible combinations of the levels of the two factors of interest. If equal sample sizes are taken for each of the possible factor combinations then the design is a balanced two-factor factorial design.
2k Factorial Models Design of Experiments - Montgomery Chapter 6 23 2k Factorial Design † Each factor has two levels (often labeled + and ¡) † Very useful design for preliminary analysis † Can \weed out" unimportant factors † Also allows initial study of interactions † For general two-factor factorial model y ijk = „ + fi i + fl j +(fifl) ij + † ijk † Have 1+(a ¡ 1) + (b ...
A factorial design examines the effects of two independent variables on a single, continuous dependent variable. ... PDF/ePub View PDF/ePub. Get access. Access options. ... Experimental Design and the Analysis of Variance. 1997. SAGE Research Methods. Book chapter . Factorial Analysis of Variance.
It has been argued that factorial designs epitomize the true beginning of modern behavioral research and have caused a significant paradigm shift in the way social scientists conceptualize their research questions and produce objective outcomes (Kerlinger & Lee, 2000).Factorial design can be categorized as an experimental methodology which goes beyond common single-variable experimentation.
A full factorial design (also known as complete factorial design) is the most complete of the design options, meaning that each factor and level are combined to test every possible combination condition. Let us expand upon the theoretical ERAS factorial experiment as an illustrative example. We designed our own ERAS protocol for Whipple procedures, and our objective is to test which components ...
- Orthogonality: An experimental design is orthogonal if each factor can be evaluated independently at all the other factors. It is achieved by matching each level of each factor with an equal number of each level of the other factors. 4.1.3 Factorial Experiments A full factorial experiment is an experiment whose design consists of two or more
which act as a crucial tool for optimization. Factorial design is more efficient for the type of. experiments having effect of two or more factors. Factorial design is more adaptable and. provides ...
Factorial Experiments. Analysis of variance for a factorial experiment allows investigation into the effect of two or more variables on the mean value of a response variable. Various combinations of factor 'levels' can be examined. It is normal to replicate a factorial experiment at least 2 times. The analysis of variance for a factorial ...
Experiments of factorial design offer a highly efficient method to evaluate multiple component interventions. The main effect of multiple components can be measured with the same number of participants as a classic two-arm randomized controlled trial (RCT) while maintaining adequate statistical power. In addition, interactions between components can be estimated.
A three factor factorial experiment with n= 2 replicates was run. The order of data collection was completely randomized. We assume all three factors are xed. The experimental data are in the table below. Cooking time 3.0 hours Cooking time 4.0 hours Hardwood Pressure Pressure Concentration 400 500 650 400 500 650 2 196.6 197.7 199.8 198.4 199. ...
Factorial design is an experimental setup that consists of multiple factors, independent variables, and is a study of both their separate and conjoint effects on the dependent variable.The factorial design enables a clinical trial to evaluate two or more interventions simultaneously. Factors have subdivisions called levels and factorial design is usually expressed in number notation to signify ...
Introduction. Factorial designs are the basis for another important principle besides blocking - examining several factors simultaneously. We will start by looking at just two factors and then generalize to more than two factors. Investigating multiple factors in the same design automatically gives us replication for each of the factors.
Figure 2. Example of the process of research. A designed experiment must satisfy all requirements of the objectives of a study but is also subject to the limitations of available resources. Below we will give examples of how the objective and hypothesis of a study influences the design of an experiment. 1.
Regardless, factorial design is a useful method to design experiments in both laboratory and industrial settings. Factorial design tests all possible conditions. Because factorial design can lead to a large number of trials, which can become expensive and time-consuming, factorial design is best used for a small number of variables with few ...
Factorial experiments have rarely been used in the development or evaluation of clinical interventions. However, factorial designs offer advantages over randomized controlled trial designs, the latter being much more frequently used in such research. Factorial designs are highly efficient (permitting evaluation of multiple intervention ...
Abstract and Figures. In this paper we will describe design of experiment by factorial analysis of variance (ANOVA) method. Experiments where the effects of more then one one factors are ...
In two-level factorial designs, can incorporate Boolean variables. i.e. x = -1 if no catalyst. 3 used. = +1 if catalyst used. With three variables, the most general polynomial model that can be generated from a full 2 level factorial design is. y = β o + β 1x 1 + β 2x 2 + β 3x 3 + β 12x 1x + β.
The simplest factorial design involves two factors, each at two levels. The top part of Figure 3-1 shows the layout of this two-by-two design, which forms the square "X-space" on the left. The equivalent one-factor-at-a-time (OFAT) experiment is shown at the upper right. Figure 3-1: Two-level factorial versus one-factor-at-a-time (OFAT) The ...
Pareto chart of standardized effects on As (V) removal. 4. Conclusion The factorial experiment design method is certainly a good technique for studying the influence of major process parameters on response factors, by significantly reducing the number of experiments and, in future, saving time, energy and money.
Title: A Factorial Design of An Active Impedance Threshold Valve versus Sham Valve and Analyze Later versus Analyze Early Abbreviations commonly used in this protocol: ACLS advanced cardiac life support AED automated external defibrillator CPR cardiopulmonary resuscitation DCC data coordinating center
maximizing the diesel and crude oil adsorptivity, were found by means of factorial experimental design. Diesel and crude oil spills were formed on seawater (two. Ports), stream water and lake ...
Sequential Latin hypercube designs (SLHDs) have recently received great attention for computer experiments, with much of the research restricted to invariant spaces. The related systematic construction methods are inflexible, and algorithmic methods are ineffective for large designs. For designs in contracting spaces, systematic construction methods have not been investigated yet. This paper ...