- Bipolar Disorder
- Therapy Center
- When To See a Therapist
- Types of Therapy
- Best Online Therapy
- Best Couples Therapy
- Managing Stress
- Sleep and Dreaming
- Understanding Emotions
- Self-Improvement
- Healthy Relationships
- Student Resources
- Personality Types
- Guided Meditations
- Verywell Mind Insights
- 2024 Verywell Mind 25
- Mental Health in the Classroom
- Editorial Process
- Meet Our Review Board
- Crisis Support
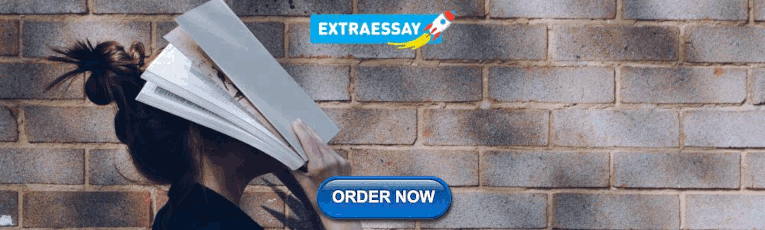
What Is a Control Group?
Control Groups vs. Experimental Groups in Psychology Research
Kendra Cherry, MS, is a psychosocial rehabilitation specialist, psychology educator, and author of the "Everything Psychology Book."
:max_bytes(150000):strip_icc():format(webp)/IMG_9791-89504ab694d54b66bbd72cb84ffb860e.jpg)
Emily Swaim is a board-certified science editor who has worked with top digital publishing brands like Voices for Biodiversity, Study.com, GoodTherapy, and Vox.
:max_bytes(150000):strip_icc():format(webp)/Emily-Swaim-1000-0f3197de18f74329aeffb690a177160c.jpg)
Doug Corrance/The Image Bank/Getty Images
Control Group vs. Experimental Group
Types of control groups.
In simple terms, the control group comprises participants who do not receive the experimental treatment. When conducting an experiment, these people are randomly assigned to this group. They also closely resemble the participants who are in the experimental group or the individuals who receive the treatment.
Experimenters utilize variables to make comparisons between an experimental group and a control group. A variable is something that researchers can manipulate, measure, and control in an experiment. The independent variable is the aspect of the experiment that the researchers manipulate (or the treatment). The dependent variable is what the researchers measure to see if the independent variable had an effect.
While they do not receive the treatment, the control group does play a vital role in the research process. Experimenters compare the experimental group to the control group to determine if the treatment had an effect.
By serving as a comparison group, researchers can isolate the independent variable and look at the impact it had.
The simplest way to determine the difference between a control group and an experimental group is to determine which group receives the treatment and which does not. To ensure that the results can then be compared accurately, the two groups should be otherwise identical.
Not exposed to the treatment (the independent variable)
Used to provide a baseline to compare results against
May receive a placebo treatment
Exposed to the treatment
Used to measure the effects of the independent variable
Identical to the control group aside from their exposure to the treatment
Why a Control Group Is Important
While the control group does not receive treatment, it does play a critical role in the experimental process. This group serves as a benchmark, allowing researchers to compare the experimental group to the control group to see what sort of impact changes to the independent variable produced.
Because participants have been randomly assigned to either the control group or the experimental group, it can be assumed that the groups are comparable.
Any differences between the two groups are, therefore, the result of the manipulations of the independent variable. The experimenters carry out the exact same procedures with both groups with the exception of the manipulation of the independent variable in the experimental group.
There are a number of different types of control groups that might be utilized in psychology research. Some of these include:
- Positive control groups : In this case, researchers already know that a treatment is effective but want to learn more about the impact of variations of the treatment. In this case, the control group receives the treatment that is known to work, while the experimental group receives the variation so that researchers can learn more about how it performs and compares to the control.
- Negative control group : In this type of control group, the participants are not given a treatment. The experimental group can then be compared to the group that did not experience any change or results.
- Placebo control group : This type of control group receives a placebo treatment that they believe will have an effect. This control group allows researchers to examine the impact of the placebo effect and how the experimental treatment compared to the placebo treatment.
- Randomized control group : This type of control group involves using random selection to help ensure that the participants in the control group accurately reflect the demographics of the larger population.
- Natural control group : This type of control group is naturally selected, often by situational factors. For example, researchers might compare people who have experienced trauma due to war to people who have not experienced war. The people who have not experienced war-related trauma would be the control group.
Examples of Control Groups
Control groups can be used in a variety of situations. For example, imagine a study in which researchers example how distractions during an exam influence test results. The control group would take an exam in a setting with no distractions, while the experimental groups would be exposed to different distractions. The results of the exam would then be compared to see the effects that distractions had on test scores.
Experiments that look at the effects of medications on certain conditions are also examples of how a control group can be used in research. For example, researchers looking at the effectiveness of a new antidepressant might use a control group that receives a placebo and an experimental group that receives the new medication. At the end of the study, researchers would compare measures of depression for both groups to determine what impact the new medication had.
After the experiment is complete, researchers can then look at the test results and start making comparisons between the control group and the experimental group.
Uses for Control Groups
Researchers utilize control groups to conduct research in a range of different fields. Some common uses include:
- Psychology : Researchers utilize control groups to learn more about mental health, behaviors, and treatments.
- Medicine : Control groups can be used to learn more about certain health conditions, assess how well medications work to treat these conditions, and assess potential side effects that may result.
- Education : Educational researchers utilize control groups to learn more about how different curriculums, programs, or instructional methods impact student outcomes.
- Marketing : Researchers utilize control groups to learn more about how consumers respond to advertising and marketing efforts.
Malay S, Chung KC. The choice of controls for providing validity and evidence in clinical research . Plast Reconstr Surg. 2012 Oct;130(4):959-965. doi:10.1097/PRS.0b013e318262f4c8
National Cancer Institute. Control group.
Pithon MM. Importance of the control group in scientific research . Dental Press J Orthod. 2013;18(6):13-14. doi:10.1590/s2176-94512013000600003
Karlsson P, Bergmark A. Compared with what? An analysis of control-group types in Cochrane and Campbell reviews of psychosocial treatment efficacy with substance use disorders . Addiction . 2015;110(3):420-8. doi:10.1111/add.12799
Myers A, Hansen C. Experimental Psychology . Belmont, CA: Cengage Learning; 2012.
By Kendra Cherry, MSEd Kendra Cherry, MS, is a psychosocial rehabilitation specialist, psychology educator, and author of the "Everything Psychology Book."
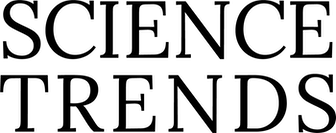
Home » experimental control important
What An Experimental Control Is And Why It’s So Important
Daniel Nelson
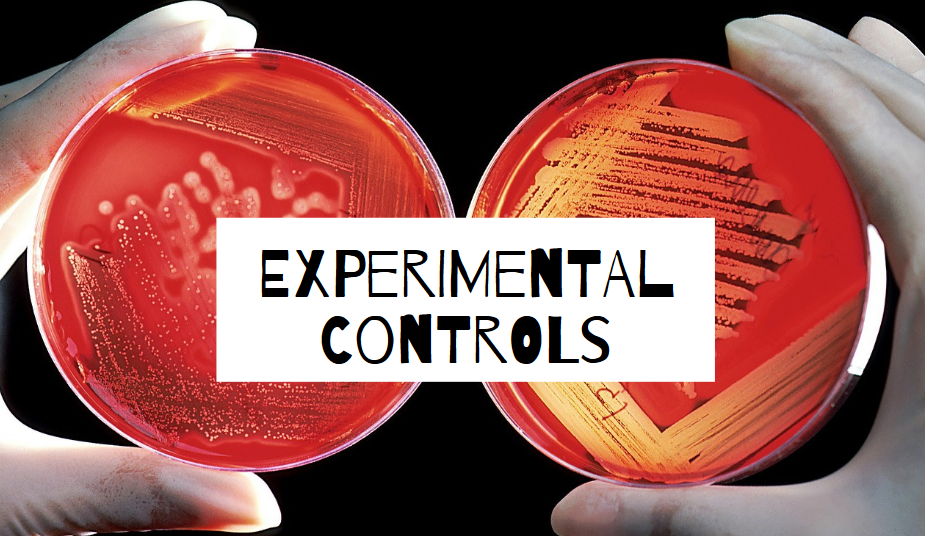
An experimental control is used in scientific experiments to minimize the effect of variables which are not the interest of the study. The control can be an object, population, or any other variable which a scientist would like to “control.”
You may have heard of experimental control, but what is it? Why is an experimental control important? The function of an experimental control is to hold constant the variables that an experimenter isn’t interested in measuring.
This helps scientists ensure that there have been no deviations in the environment of the experiment that could end up influencing the outcome of the experiment, besides the variable they are investigating. Let’s take a closer look at what this means.
You may have ended up here to understand why a control is important in an experiment. A control is important for an experiment because it allows the experiment to minimize the changes in all other variables except the one being tested.
To start with, it is important to define some terminology.
Terminology Of A Scientific Experiment
Randomization is important as it allows for more non-biased results in experiments. Random numbers generators are often used both in scientific studies as well as on 지노 사이트 to make outcomes fairer.
Scientists use the scientific method to ask questions and come to conclusions about the nature of the world. After making an observation about some sort of phenomena they would like to investigate, a scientist asks what the cause of that phenomena could be. The scientist creates a hypothesis, a proposed explanation that answers the question they asked. A hypothesis doesn’t need to be correct, it just has to be testable.
The hypothesis is a prediction about what will happen during the experiment, and if the hypothesis is correct then the results of the experiment should align with the scientist’s prediction. If the results of the experiment do not align with the hypothesis, then a good scientist will take this data into consideration and form a new hypothesis that can better explain the phenomenon in question.
Independent and Dependent Variables
In order to form an effective hypothesis and do meaningful research, the researcher must define the experiment’s independent and dependent variables . The independent variable is the variable which the experimenter either manipulates or controls in an experiment to test the effects of this manipulation on the dependent variable. A dependent variable is a variable being measured to see if the manipulation has any effect.
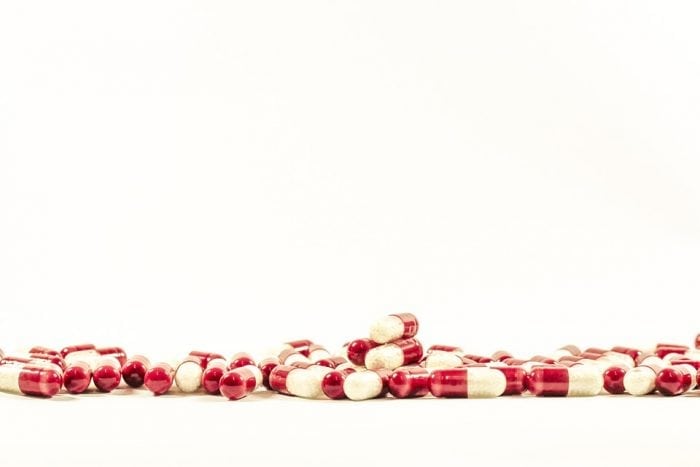
Photo: frolicsomepl via Pixabay, CC0
For instance, if a researcher wanted to see how temperature impacts the behavior of a certain gas, the temperature they adjust would be the independent variable and the behavior of the gas the dependent variable.
Control Groups and Experimental Groups
There will frequently be two groups under observation in an experiment, the experimental group, and the control group . The control group is used to establish a baseline that the behavior of the experimental group can be compared to. If two groups of people were receiving an experimental treatment for a medical condition, one would be given the actual treatment (the experimental group) and one would typically be given a placebo or sugar pill (the control group).
Without an experimental control group, it is difficult to determine the effects of the independent variable on the dependent variable in an experiment. This is because there can always be outside factors that are influencing the behavior of the experimental group. The function of a control group is to act as a point of comparison, by attempting to ensure that the variable under examination (the impact of the medicine) is the thing responsible for creating the results of an experiment. The control group is holding other possible variables constant, such as the act of seeing a doctor and taking a pill, so only the medicine itself is being tested.
Why Are Experimental Controls So Important?
Experimental controls allow scientists to eliminate varying amounts of uncertainty in their experiments. Whenever a researcher does an experiment and wants to ensure that only the variable they are interested in changing is changing, they need to utilize experimental controls.
Experimental controls have been dubbed “controls” precisely because they allow researchers to control the variables they think might have an impact on the results of the study. If a researcher believes that some outside variables could influence the results of their research, they’ll use a control group to try and hold that thing constant and measure any possible influence it has on the results. It is important to note that there may be many different controls for an experiment, and the more complex a phenomenon under investigation is, the more controls it is likely to have.
Not only do controls establish a baseline that the results of an experiment can be compared to, they also allow researchers to correct for possible errors. If something goes wrong in the experiment, a scientist can check on the controls of the experiment to see if the error had to do with the controls. If so, they can correct this next time the experiment is done.
A Practical Example
Let’s take a look at a concrete example of experimental control. If an experimenter wanted to determine how different soil types impacted the germination period of seeds , they could set up four different pots. Each pot would be filled with a different soil type, planted with seeds, then watered and exposed to sunlight. Measurements would be taken regarding how long it took for the seeds to sprout in the different soil types.
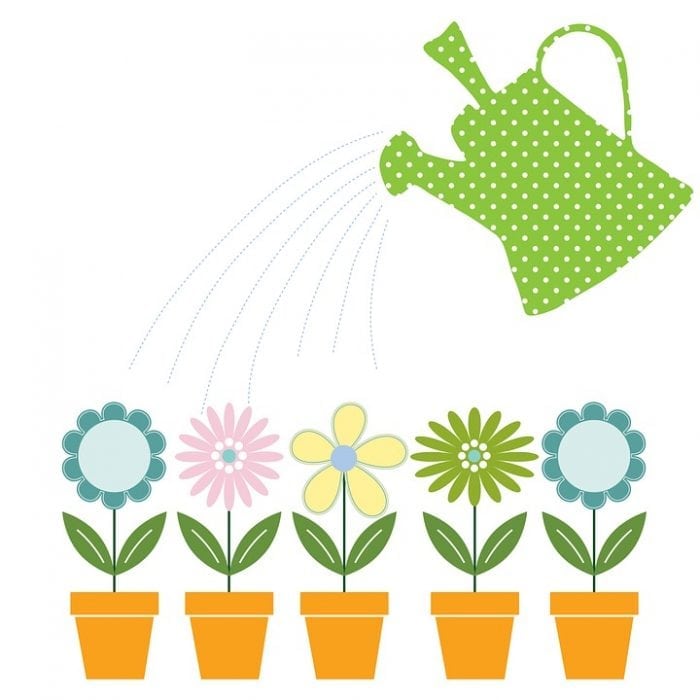
Photo: Kaz via Pixabay, CC0
A control for this experiment might be to fill more pots with just the different types of soil and no seeds or to set aside some seeds in a pot with no soil. The goal is to try and determine that it isn’t something else other than the soil, like the nature of the seeds themselves, the amount of sun they were exposed to, or how much water they are given, that affected how quickly the seeds sprouted. The more variables a researcher controlled for, the surer they could be that it was the type of soil having an impact on the germination period.
Not All Experiments Are Controlled
“It doesn’t matter how beautiful your theory is, it doesn’t matter how smart you are. If it doesn’t agree with experiment, it’s wrong.” — Richard P. Feynman
While experimental controls are important , it is also important to remember that not all experiments are controlled. In the real world, there are going to be limitations on what variables a researcher can control for, and scientists often try to record as much data as they can during an experiment so they can compare factors and variables with one another to see if any variables they didn’t control for might have influenced the outcome. It’s still possible to draw useful data from experiments that don’t have controls, but it is much more difficult to draw meaningful conclusions based on uncontrolled data.
Though it is often impossible in the real world to control for every possible variable, experimental controls are an invaluable part of the scientific process and the more controls an experiment has the better off it is.
← Previous post
Next post →
Related Posts
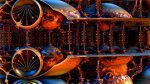
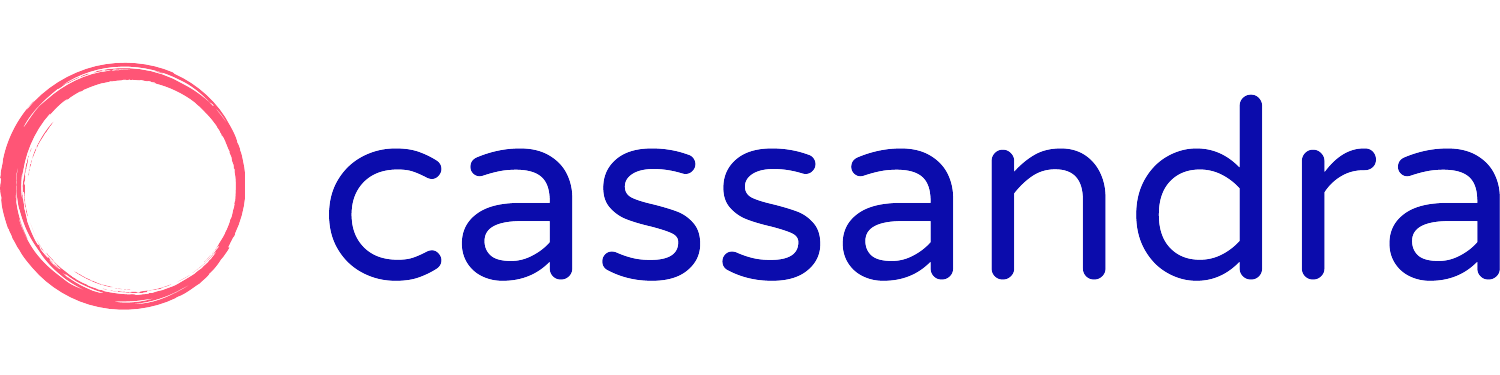
The Importance of Control Group Analysis in Scientific Research
Explore the crucial role of control groups in scientific research, enhancing validity and ensuring accurate results.

Control groups are a fundamental component of scientific research, serving as a benchmark to measure the effects of experimental treatments. By comparing outcomes between the control group and the experimental group, researchers can attribute changes in the dependent variable to the independent variable, thus ensuring the internal validity of the study. Without control groups, it becomes challenging to draw accurate conclusions and determine the true efficacy of a treatment or intervention.
Key Takeaways
- Control groups are essential for ensuring the internal validity of scientific research.
- They serve as a baseline to compare the effects of the independent variable on the dependent variable.
- Control groups help in avoiding research biases and confounding variables.
- Different types of control groups, such as positive, negative, and placebo, are used depending on the study design.
- Properly designed control groups enhance the reproducibility and reliability of research findings.
The Role of Control Groups in Ensuring Internal Validity
Control groups are critical to the scientific method as they help ensure the internal validity of a study. Without a control group, it’s harder to be certain that the outcome was caused by the experimental treatment and not by other variables. This is essential for drawing accurate conclusions and avoiding research bias.
Defining Internal Validity
Internal validity refers to the extent to which a study can demonstrate a causal relationship between the treatment and the observed outcome. It ensures that the results are due to the independent variable and not other factors. Control groups play a pivotal role in maintaining this validity by providing a baseline for comparison.
How Control Groups Enhance Validity
Control groups help account for the placebo effect, where participants’ beliefs about the treatment can influence their behavior or responses. By comparing the treatment group to the control group, researchers can isolate the effect of the treatment itself. This increases the internal validity of the results and the confidence we can have in the conclusions.
Examples of Validity in Research
Consider a study testing a new medication for ADHD. One group receives the new medication, while the other group receives a placebo. The placebo group serves as the control group, allowing researchers to determine if changes in the treatment group are due to the medication or other variables. This method is crucial for the future of measurement: triangulating MTA, MMM, and incrementality testing . Triangulation offers a holistic view of marketing effectiveness, optimizing resource allocation for brands.
Types of Control Groups in Scientific Research
Control groups are critical to the scientific method as they help ensure the internal validity of a study. Using a control group means that any change in the dependent variable can be attributed to the independent variable. This helps avoid extraneous variables or confounding variables from impacting your work, as well as a few types of research bias, like omitted variable bias.
Positive Control Groups
Positive control groups are used to ensure that the experimental setup is capable of producing results. For example, if you are testing a new drug, a positive control group might receive a treatment that is already known to produce a certain effect. This helps to confirm that the experimental conditions are working as expected.
Negative Control Groups
Negative control groups are used to ensure that no confounding variable has affected the results. In a drug trial, a negative control group might receive a placebo, which is a treatment that has no therapeutic effect. This helps to show that any changes in the experimental group are due to the treatment itself and not some other factor.
Placebo Control Groups
Placebo control groups are a specific type of negative control group used in clinical trials. Participants in the placebo group receive a treatment that looks identical to the experimental treatment but has no active ingredient. This helps to account for the placebo effect, where participants experience changes simply because they believe they are receiving a treatment.
In clinical trials, the use of placebo control groups is essential for determining the true efficacy of a new treatment. Without this control, it would be difficult to distinguish between the actual effects of the treatment and the psychological impact of believing one is being treated.
Designing Experiments with Control Groups
Designing experiments with control groups is a critical aspect of scientific research. It ensures that the results are reliable and can be attributed to the variables being tested. Here, we will discuss the key elements involved in this process.
Random Assignment
Random assignment is the process of assigning participants to different groups using randomization. This method ensures that each participant has an equal chance of being placed in any group, thereby eliminating selection bias. Random assignment is crucial for maintaining the internal validity of an experiment. For example, in a marketing experiment design, participants might be randomly assigned to either a control group or an experimental group to test the effectiveness of a new advertising strategy.
Blinding and Control Groups
Blinding is a technique used to prevent bias in research. In a single-blind experiment, the participants do not know whether they are in the control group or the experimental group. In a double-blind experiment, neither the participants nor the researchers know who is in which group. This method is particularly useful in medical research, where the placebo effect can influence results. For instance, in a study testing a new drug, blinding ensures that neither the patients nor the doctors know who is receiving the actual medication and who is receiving a placebo.
Maintaining Consistency
Maintaining consistency across all groups in an experiment is essential for obtaining valid results. This means that all conditions, except for the variable being tested, should be kept the same for both the control and experimental groups. For example, in geo experiments, researchers might implement geo-based incrementality testing to measure the real impact of a marketing campaign. By keeping all other variables constant, they can accurately determine the effectiveness of the campaign.
In any well-designed experiment, the control group serves as a benchmark, allowing researchers to measure the true effect of the independent variable. This is especially important in fields like marketing budget planning, where understanding the actual impact of different strategies can lead to more informed decisions.
Challenges and Limitations of Control Group Analysis
Ethical considerations.
When conducting Control Group Analysis , researchers must navigate various ethical dilemmas. For instance, withholding a potentially beneficial treatment from the control group can raise ethical concerns. Balancing the need for rigorous scientific methods with ethical responsibilities is crucial. Researchers often use alternative methodologies to address these challenges, such as crossover designs where participants receive both the treatment and control conditions at different times.
Practical Limitations
Implementing control groups can be resource-intensive. Researchers may face constraints related to time, budget, and participant availability. These limitations can impact the scope and scale of the study. Additionally, maintaining consistency across control and treatment groups can be challenging, especially in long-term studies. Practical solutions include using automated systems for data collection and employing robust randomization techniques.
Addressing Confounding Variables
Confounding variables can significantly impact the validity of a study. These are variables that the researcher failed to control or eliminate, which can cause a false association between the treatment and the outcome. To mitigate this, researchers can use techniques like stratified randomization and matching. Identifying and addressing confounding variables is essential for enhancing the reliability of the results.
Ensuring the internal validity of your research often hinges on how well you manage these challenges. By addressing ethical considerations, practical limitations, and confounding variables, you can significantly improve the robustness of your Control Group Analysis.
Case Studies Highlighting the Importance of Control Groups
Medical research examples.
In medical research, control groups are indispensable for determining the effectiveness of new treatments . For instance, in a clinical trial for a new drug, one group receives the drug while the control group receives a placebo. This setup helps in measuring the Incremental Lift in patient recovery rates attributable to the drug, rather than other factors.
Control groups in medical research ensure that the observed effects are due to the treatment and not external variables.
Psychological Studies
Psychological studies often use control groups to understand the impact of various interventions. For example, a study on the effects of cognitive-behavioral therapy (CBT) for depression might have one group undergo CBT while the control group receives no treatment. This helps in isolating the Incremental Contribution of CBT to improvements in mental health.
Social Science Research
In social science research, control groups help in understanding societal trends and behaviors. For example, a study on the impact of educational programs on student performance might have a control group that does not participate in the program. This allows researchers to measure the Conversion Lift in academic performance due to the educational intervention.
Without control groups, it would be challenging to attribute changes in the dependent variable to the independent variable accurately.
Measuring the Effectiveness of Control Groups
Baseline comparisons.
An important factor when measuring the effectiveness of a control group is the uniformity of samples. Ensuring the control group is both random and representative of the entire population will lead to more dependable results. The control group serves as a baseline , enabling researchers to see what impact changes to the independent variable produce and strengthening researchers’ ability to draw conclusions from a study.
Without the presence of a control group, a researcher cannot determine whether a particular treatment truly has an effect on an experimental group.
Statistical Methods
A chi-squared statistic can reveal differences between the observed results and the results you would expect if there was no relationship in the data. For example, the expectation of variations to have zero impact on conversion rate can be tested using this method. Here are some steps to execute this analysis:
- Define the null hypothesis that there is no difference between the control and test groups.
- Collect data from both groups.
- Calculate the chi-squared statistic.
- Compare the calculated value with the critical value from the chi-squared distribution table.
- Draw conclusions based on the comparison.
Interpreting Results
When interpreting results, it is crucial to consider the size of the control group. The tradeoff between confidence levels in the results and the opportunity cost of implementing a more successful variation should not be taken lightly. For instance, if the experiment is run on a population size of only 100 participants, a 5% control group would be only 5 individuals, which would certainly diminish the significance of the results. Therefore, maintaining an adequately sized control group is essential for reliable conclusions.
The Impact of Control Groups on Research Outcomes
Drawing accurate conclusions.
Control groups are essential for drawing accurate conclusions in scientific research. By comparing the treatment group to the control group, researchers can isolate the effect of the independent variable. This helps in determining whether the observed changes are due to the treatment or other external factors. For instance, in medical research , a control group receiving a placebo can help identify the true efficacy of a new drug.
Avoiding Research Bias
Control groups play a crucial role in avoiding research bias. They help mitigate the impact of confounding variables and ensure that the results are not skewed by external influences. This is particularly important in psychological studies , where participant expectations can influence outcomes. By using control groups, researchers can ensure that any observed effects are due to the treatment itself and not other factors.
Enhancing Reproducibility
The use of control groups enhances the reproducibility of research findings. When other researchers can replicate the study and achieve similar results, it strengthens the validity of the original findings. This is vital for the advancement of scientific knowledge. For example, in social science research , control groups help in verifying the impact of interventions across different populations and settings.
Control groups are the backbone of rigorous scientific research, ensuring that findings are both valid and reliable.
- isolate the effect
- medical research
- psychological studies
- social science research
In conclusion, control group analysis is indispensable in scientific research. Control groups serve as a baseline, allowing researchers to attribute changes in the dependent variable directly to the independent variable, thereby ensuring the internal validity of the study. Without control groups, it becomes challenging to determine whether observed changes are due to the treatment or other extraneous variables. By providing a clear comparison, control groups enhance the reliability and credibility of research findings, making them a cornerstone of the scientific method. Therefore, the inclusion of control groups in experimental design is not just beneficial but essential for drawing accurate and meaningful conclusions.
Frequently Asked Questions
What is a control group in scientific research.
A control group is a group of participants in an experiment who do not receive the experimental treatment. They serve as a baseline to compare the results of the experimental group against.
Why are control groups important in scientific research?
Control groups help ensure the internal validity of research by providing a baseline. This allows researchers to determine if changes in the dependent variable are due to the independent variable or other factors.
What are the different types of control groups?
There are several types of control groups, including positive control groups, negative control groups, and placebo control groups. Each type serves a different purpose in validating the results of an experiment.
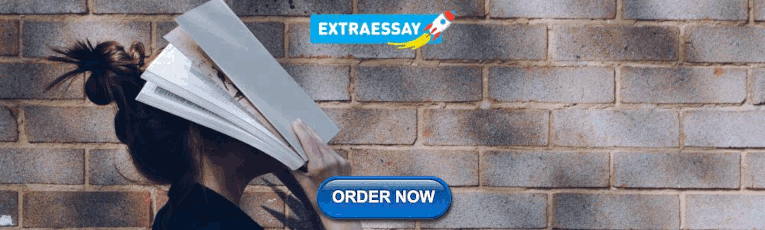
How do control groups enhance the validity of an experiment?
Control groups enhance validity by isolating the effect of the independent variable. This helps to avoid confounding variables and research biases, ensuring that the observed effects are due to the treatment.
What are some challenges associated with using control groups?
Challenges include ethical considerations, practical limitations, and the need to address confounding variables. Researchers must design their studies carefully to mitigate these issues.
Can you provide an example of a control group in research?
In medical research, a control group might receive a placebo while the experimental group receives the actual medication. This allows researchers to determine if the medication has a real effect compared to no treatment.
Related articles
Measure and optimize your media.

- Science Notes Posts
- Contact Science Notes
- Todd Helmenstine Biography
- Anne Helmenstine Biography
- Free Printable Periodic Tables (PDF and PNG)
- Periodic Table Wallpapers
- Interactive Periodic Table
- Periodic Table Posters
- Science Experiments for Kids
- How to Grow Crystals
- Chemistry Projects
- Fire and Flames Projects
- Holiday Science
- Chemistry Problems With Answers
- Physics Problems
- Unit Conversion Example Problems
- Chemistry Worksheets
- Biology Worksheets
- Periodic Table Worksheets
- Physical Science Worksheets
- Science Lab Worksheets
- My Amazon Books
Control Group Definition and Examples

The control group is the set of subjects that does not receive the treatment in a study. In other words, it is the group where the independent variable is held constant. This is important because the control group is a baseline for measuring the effects of a treatment in an experiment or study. A controlled experiment is one which includes one or more control groups.
- The experimental group experiences a treatment or change in the independent variable. In contrast, the independent variable is constant in the control group.
- A control group is important because it allows meaningful comparison. The researcher compares the experimental group to it to assess whether or not there is a relationship between the independent and dependent variable and the magnitude of the effect.
- There are different types of control groups. A controlled experiment has one more control group.
Control Group vs Experimental Group
The only difference between the control group and experimental group is that subjects in the experimental group receive the treatment being studied, while participants in the control group do not. Otherwise, all other variables between the two groups are the same.
Control Group vs Control Variable
A control group is not the same thing as a control variable. A control variable or controlled variable is any factor that is held constant during an experiment. Examples of common control variables include temperature, duration, and sample size. The control variables are the same for both the control and experimental groups.
Types of Control Groups
There are different types of control groups:
- Placebo group : A placebo group receives a placebo , which is a fake treatment that resembles the treatment in every respect except for the active ingredient. Both the placebo and treatment may contain inactive ingredients that produce side effects. Without a placebo group, these effects might be attributed to the treatment.
- Positive control group : A positive control group has conditions that guarantee a positive test result. The positive control group demonstrates an experiment is capable of producing a positive result. Positive controls help researchers identify problems with an experiment.
- Negative control group : A negative control group consists of subjects that are not exposed to a treatment. For example, in an experiment looking at the effect of fertilizer on plant growth, the negative control group receives no fertilizer.
- Natural control group : A natural control group usually is a set of subjects who naturally differ from the experimental group. For example, if you compare the effects of a treatment on women who have had children, the natural control group includes women who have not had children. Non-smokers are a natural control group in comparison to smokers.
- Randomized control group : The subjects in a randomized control group are randomly selected from a larger pool of subjects. Often, subjects are randomly assigned to either the control or experimental group. Randomization reduces bias in an experiment. There are different methods of randomly assigning test subjects.
Control Group Examples
Here are some examples of different control groups in action:
Negative Control and Placebo Group
For example, consider a study of a new cancer drug. The experimental group receives the drug. The placebo group receives a placebo, which contains the same ingredients as the drug formulation, minus the active ingredient. The negative control group receives no treatment. The reason for including the negative group is because the placebo group experiences some level of placebo effect, which is a response to experiencing some form of false treatment.
Positive and Negative Controls
For example, consider an experiment looking at whether a new drug kills bacteria. The experimental group exposes bacterial cultures to the drug. If the group survives, the drug is ineffective. If the group dies, the drug is effective.
The positive control group has a culture of bacteria that carry a drug resistance gene. If the bacteria survive drug exposure (as intended), then it shows the growth medium and conditions allow bacterial growth. If the positive control group dies, it indicates a problem with the experimental conditions. A negative control group of bacteria lacking drug resistance should die. If the negative control group survives, something is wrong with the experimental conditions.
- Bailey, R. A. (2008). Design of Comparative Experiments . Cambridge University Press. ISBN 978-0-521-68357-9.
- Chaplin, S. (2006). “The placebo response: an important part of treatment”. Prescriber . 17 (5): 16–22. doi: 10.1002/psb.344
- Hinkelmann, Klaus; Kempthorne, Oscar (2008). Design and Analysis of Experiments, Volume I: Introduction to Experimental Design (2nd ed.). Wiley. ISBN 978-0-471-72756-9.
- Pithon, M.M. (2013). “Importance of the control group in scientific research.” Dental Press J Orthod . 18 (6):13-14. doi: 10.1590/s2176-94512013000600003
- Stigler, Stephen M. (1992). “A Historical View of Statistical Concepts in Psychology and Educational Research”. American Journal of Education . 101 (1): 60–70. doi: 10.1086/444032
Related Posts
Control Group: The Key Elements In Experimental Research
Understand the design and interpretation of control group in research experiments for powerful conclusions
The control group constitutes a baseline for comparison, enabling researchers to assess the true effects of independent variables. Researchers can effectively assess the impact of independent variables and discern causation from correlation, by comparing the results of experimental groups to those of control groups. This article will highlight the significance and implementation of control groups in research experiments, and explain their role in ensuring scientific methodology and reliable findings. We will explore the fundamental principles of control groups, examine their types, and discuss their importance in minimizing biases and confounding factors.
What Is A Control Group?
A control group is a fundamental component of scientific experiments designed to compare and evaluate the effects of an intervention or treatment. It serves as a baseline against which the experimental group is measured. The control group consists of individuals or subjects who do not receive the experimental treatment but are otherwise subjected to the same conditions and procedures as the experimental group. Working with a control group, researchers can assess the specific impact of the intervention by comparing the outcomes between the experimental and control groups.
Related article: The Role Of Experimental Groups In Research
The Role Of A Control Group In Scientific Experiments
A control group plays a crucial role in scientific experiments as it enables researchers to establish a valid cause-and-effect relationship between the experimental treatment and the observed outcomes. By comparing the experimental group’s results with those of the control group, researchers can determine whether any observed effects are due to the treatment or other factors. The control group serves as a standard for comparison, helping to isolate the specific influence of the intervention being tested. It provides a baseline against which experimental group outcomes can be evaluated and allows researchers to draw accurate conclusions about the treatment’s efficacy or the impact of other variables being studied.
Why Is A Control Group Necessary?
Including a control group in scientific experiments is essential for ensuring the reliability and validity of the findings. Without a control group, it becomes challenging to determine whether any observed changes or effects are truly attributable to the intervention or simply a result of chance or other factors. The control group allows researchers to differentiate between the effects of the experimental treatment and background noise or confounding variables because it provides a reference point. A well-designed control group is crucial for generating reliable and meaningful results, intensifying the scientific rigor of the study, and supporting evidence-based decision-making in various fields of research.
Types Of Control Groups
In scientific experiments, different types of control groups are used to ensure accurate and meaningful results. These control groups help researchers compare the effects of an intervention or treatment against a reference point. Four common types of control groups are negative controls, positive controls, placebo controls, and randomized control groups.
Negative Controls
Negative controls are an integral part of scientific experiments, serving as a reference to establish the absence of a specific effect. In these control groups, no treatment is administered, allowing researchers to compare the outcomes with the experimental group. Researchers can identify and account for confounding variables and background effects that may influence the results when they include negative control groups. This ensures the specificity of the treatment and enhances the validity of the study. Negative controls can take various forms, such as placebos or control groups receiving no treatment, depending on the research question.
Positive controls
Positive controls are references to validate the reliability and sensitivity of the experimental setup. In these control groups, a known treatment or condition is applied to generate an expected response or outcome. By including positive controls, researchers can assess whether the experimental conditions and methodology are capable of detecting the desired effect. Positive controls act as a benchmark, providing evidence that the experimental system is functioning properly and capable of producing the anticipated results. This helps researchers ensure the validity and accuracy of their findings by confirming that the experimental conditions are conducive to detecting the intended response.
Placebo controls
Placebo controls play a significant role in medical and clinical research by providing a baseline for comparison and evaluating the effectiveness of a new treatment or intervention. In a placebo control group, participants receive an inactive substance or sham procedure that is indistinguishable from the active treatment being tested. The purpose of the placebo control is to assess the specific effects of the treatment by comparing it to the effects observed in the placebo group. By administering a placebo, researchers can account for the psychological and physiological responses that may occur simply due to the participants’ belief in receiving treatment. This helps determine the true efficacy of the active treatment, as any observed improvements in the treatment group can be attributed to the treatment itself, beyond the placebo effect. Placebo controls are essential in clinical trials and other studies to minimize bias, establish the true therapeutic benefits of treatment, and ensure the reliability of the results.
Randomized Control Group
Randomized control groups are an essential component of research studies as they introduce unpredictability to control factors. By randomly assigning participants to either the control or treatment group, researchers ensure that the variables not specifically tested are evenly distributed. This randomization helps eliminate bias and allows for accurate analysis of the independent variable. By using randomized control groups, researchers can draw reliable conclusions about the impact of the variables being studied.
Quasi-Experimental Designs And Their Role In Social Policy Studies
Quasi-experimental designs in social policy studies often utilize control groups to assess the impact of interventions or policies on a target population. While these designs do not involve random assignment of participants to groups, they still incorporate a control group to establish a baseline for comparison. The control group consists of individuals who do not receive the intervention or policy being studied, allowing researchers to evaluate the effects of the intervention by comparing outcomes between the treatment group and the control group. This helps control for confounding variables and provides insights into a causal relationship between the intervention and the observed outcomes.
Implementing Control Groups In Experimental Design And Analysis
Control groups serve as a reference point against which the effects of experimental interventions can be measured. They provide a baseline to compare with the treatment group, allowing researchers to determine the true impact of the variables under investigation. This approach helps establish causal relationships and increases the internal validity of the research.
Randomized Controlled Experiments (RCTs) For Public Policy Studies
Randomized controlled experiments are widely used in public policy studies. RCTs involve randomly assigning participants to either a treatment group or a control group. The treatment group receives the intervention or policy being tested, while the control group does not. RCTs help ensure that any observed differences between the groups are not due to pre-existing factors, increasing the reliability of the study’s findings. RCTs are particularly valuable in evaluating the impact of public policies and interventions on a large scale.
Non-Experimental Research Vs. Actual Experimentation
When determining the baseline for comparison in research, researchers must consider whether to use non-experimental research or actual experimentation. Non-experimental research involves observing and analyzing existing data without manipulating any variables. This approach is helpful in situations where it is not feasible or ethical to conduct an experiment. On the other hand, actual experimentation involves actively manipulating variables and comparing groups with and without the intervention. While actual experimentation provides stronger causal evidence, non-experimental research can still provide valuable insights when experiments are not possible.
Identifying Confounding Variables And Factors
Confounding variables and factors are extraneous variables that can influence the relationship between the independent and dependent variables in a study. Identifying and controlling for confounding variables is crucial to ensure accurate and valid results. Researchers employ various techniques to address confounding variables, such as random assignment of participants to groups, matching participants based on relevant characteristics, or statistical techniques like regression analysis. By accounting for confounding variables, researchers can strengthen the internal validity of their studies and draw more accurate conclusions about the relationship between variables.
The Vital Role Of The Control Group In Scientific Methodology And Analysis
In experimental studies, the control group serves as a standard against which the effects of a particular intervention or treatment are measured. By keeping all variables constant except for the one being studied, researchers can isolate the true impact of the intervention. This helps to establish causality and determine whether the observed effects are indeed due to the intervention or simply a result of other factors.
In addition to experimental studies, control groups are also essential in observational and epidemiological research. They help researchers account for potential biases and confounding factors when analyzing the relationship between variables. By comparing a group exposed to a certain risk factor or condition with a similar group that is not exposed, researchers can better understand the true impact of the risk factor or condition on the outcome of interest.
Overall, the control group serves as a guide in scientific methodology and analysis. It allows researchers to draw valid and reliable conclusions, enhance the internal validity of their studies, and provide more robust evidence for decision-making in various fields, including medicine, psychology, biology, and social sciences.
Mind the Graph Has 200+ Pre-Made Beautiful Templates For Professional Infographics
Mind the Graph is a powerful platform that offers valuable assistance to scientists in their research endeavors. With a collection of over 200 pre-made templates, Mind the Graph enables scientists to effortlessly create professional and visually captivating infographics. These templates are the foundation for conveying complex scientific concepts, data, and research findings in a visually engaging manner. Professionals can simply choose from a wide range of visually appealing templates and customize them with their own data, illustrations, and text to effectively communicate their scientific discoveries. Sign up for free and start your first design now.
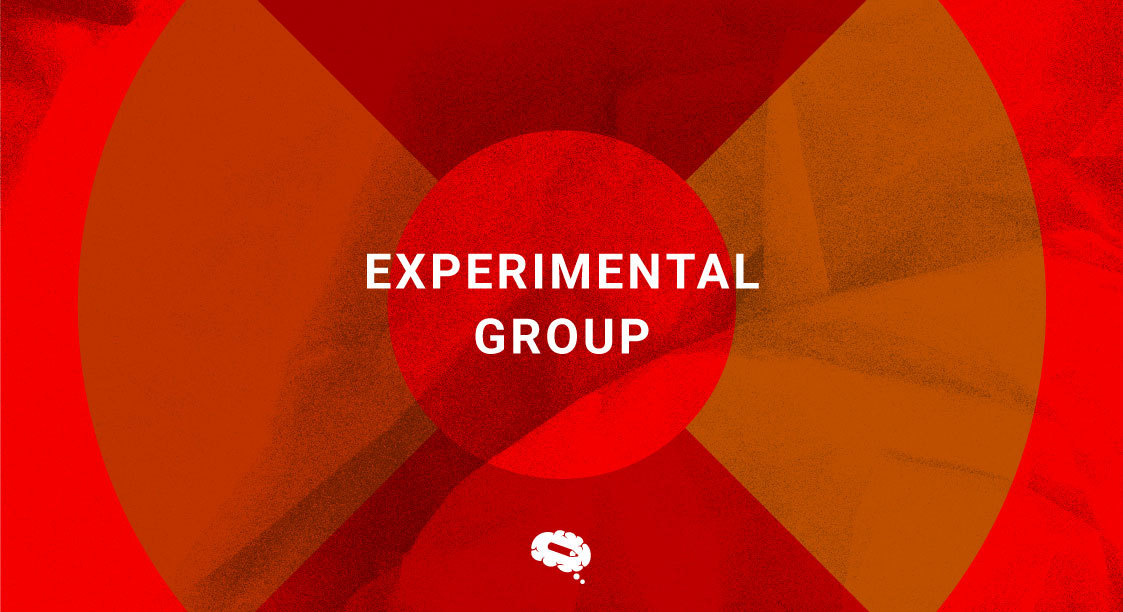
Subscribe to our newsletter
Exclusive high quality content about effective visual communication in science.
Content tags

- Science, Tech, Math ›
- Chemistry ›
What Is a Control Group?
- Chemical Laws
- Periodic Table
- Projects & Experiments
- Scientific Method
- Biochemistry
- Physical Chemistry
- Medical Chemistry
- Chemistry In Everyday Life
- Famous Chemists
- Activities for Kids
- Abbreviations & Acronyms
- Weather & Climate
- Ph.D., Biomedical Sciences, University of Tennessee at Knoxville
- B.A., Physics and Mathematics, Hastings College
A control group in a scientific experiment is a group separated from the rest of the experiment, where the independent variable being tested cannot influence the results. This isolates the independent variable's effects on the experiment and can help rule out alternative explanations of the experimental results.
A control group definition can also be separated into two other types: positive or negative.
Positive control groups are groups where the conditions of the experiment are set to guarantee a positive result. A positive control group can show the experiment is functioning properly as planned.
Negative control groups are groups where the conditions of the experiment are set to cause a negative outcome.
Control groups are not necessary for all scientific experiments. Controls are extremely useful when the experimental conditions are complex and difficult to isolate.
Example of a Negative Control Group
Negative control groups are particularly common in science fair experiments , to teach students how to identify the independent variable . A simple example of a control group can be seen in an experiment in which the researcher tests whether or not a new fertilizer affects plant growth. The negative control group would be the plants grown without fertilizer but under the same conditions as the experimental group. The only difference between the experimental group would be whether or not the fertilizer was used.
Several experimental groups could differ in the fertilizer concentration, application method, etc. The null hypothesis would be that the fertilizer does not affect plant growth. Then, if a difference is seen in the growth rate or the height of plants over time, a strong correlation between fertilizer and growth would be established. Note the fertilizer could have a negative impact on growth rather than positive. Or, for some reason, the plants might not grow at all. The negative control group helps establish the experimental variable is the cause of atypical growth rather than some other (possibly unforeseen) variable.
Example of a Positive Control Group
A positive control demonstrates an experiment is capable of producing a positive result. For example, let's say you are examining bacterial susceptibility to a drug. You might use a positive control to make sure the growth medium is capable of supporting any bacteria. You could culture bacteria known to carry the drug resistance marker, so they should be capable of surviving on a drug-treated medium. If these bacteria grow, you have a positive control that shows other drug-resistant bacteria should be capable of surviving the test.
The experiment could also include a negative control. You could plate bacteria known not to carry a drug-resistant marker. These bacteria should be unable to grow on the drug-laced medium. If they do grow, you know there is a problem with the experiment .
- Understanding Experimental Groups
- The Difference Between Control Group and Experimental Group
- List of Platinum Group Metals or PGMs
- Scientific Hypothesis, Model, Theory, and Law
- What Is an Experiment? Definition and Design
- What Is a Base Metal? Definition and Examples
- What Is a Molecule?
- What Chemistry Is and What Chemists Do
- Acid Dissociation Constant Definition: Ka
- Fatty Acid Definition
- Dissolving Sugar in Water: Chemical or Physical Change?
- Hydroxyl Group Definition in Chemistry
- Heavy Metals in Science
- Is Glass a Liquid or a Solid?
- Examples of Independent and Dependent Variables
- Noble Metals List and Properties

Understanding Control Groups for Research
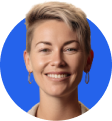
Introduction
What are control groups in research, examples of control groups in research, control group vs. experimental group, types of control groups, control groups in non-experimental research.
A control group is typically thought of as the baseline in an experiment. In an experiment, clinical trial, or other sort of controlled study, there are at least two groups whose results are compared against each other.
The experimental group receives some sort of treatment, and their results are compared against those of the control group, which is not given the treatment. This is important to determine whether there is an identifiable causal relationship between the treatment and the resulting effects.
As intuitive as this may sound, there is an entire methodology that is useful to understanding the role of the control group in experimental research and as part of a broader concept in research. This article will examine the particulars of that methodology so you can design your research more rigorously .
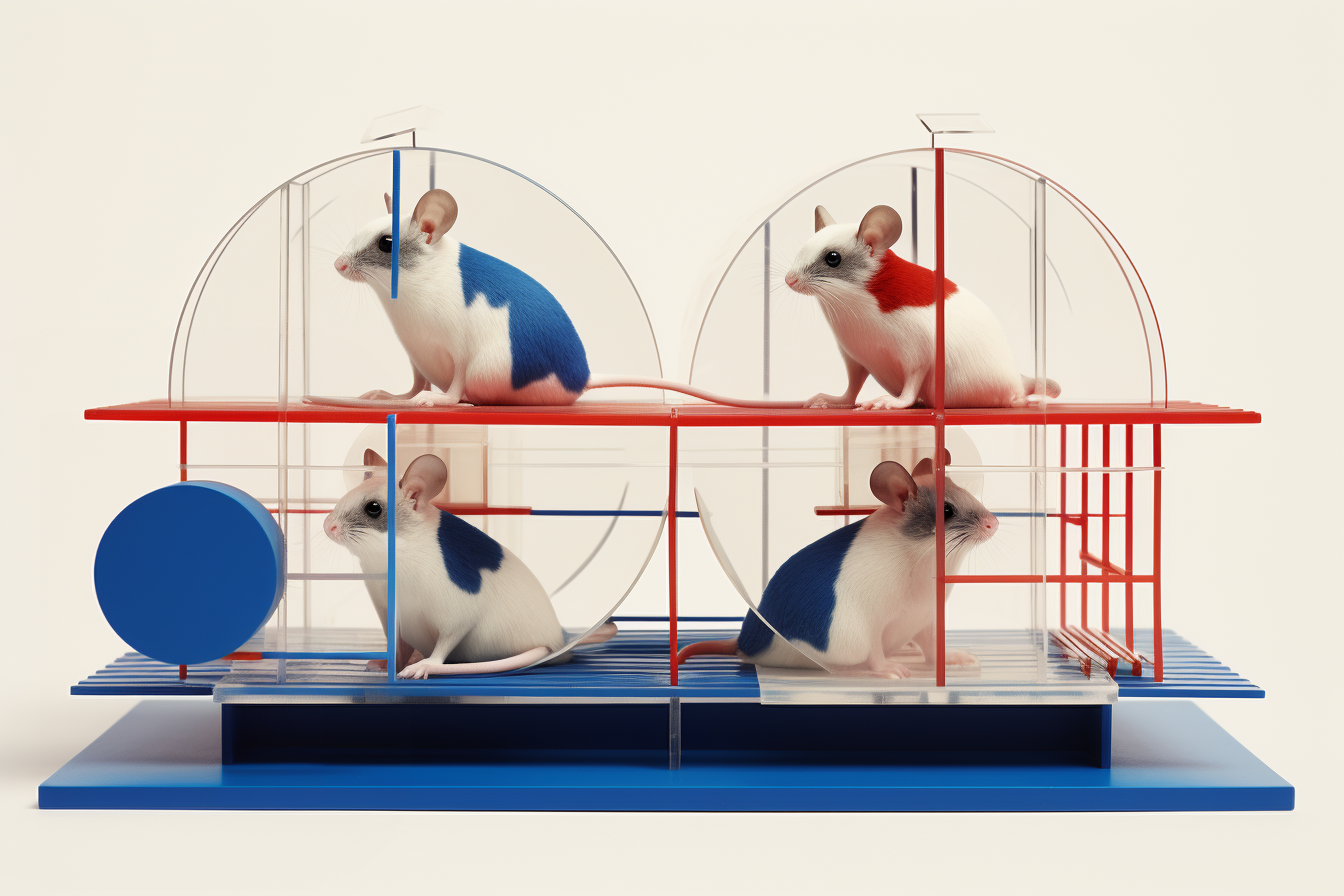
Suppose that a friend or colleague of yours has a headache. You give them some over-the-counter medicine to relieve some of the pain. Shortly after they take the medicine, the pain is gone and they feel better. In casual settings, we can assume that it must be the medicine that was the cause of their headache going away.
In scientific research, however, we don't really know if the medicine made a difference or if the headache would have gone away on its own. Maybe in the time it took for the headache to go away, they ate or drank something that might have had an effect. Perhaps they had a quick nap that helped relieve the tension from the headache. Without rigorously exploring this phenomenon , any number of confounding factors exist that can make us question the actual efficacy of any particular treatment.
Experimental research relies on observing differences between the two groups by "controlling" the independent variable , or in the case of our example above, the medicine that is given or not given depending on the group. The dependent variable in this case is the change in how the person suffering the headache feels, and the difference between taking and not taking the medicine is evidence (or lack thereof) that the treatment is effective.
The catch is that, between the control group and other groups (typically called experimental groups), it's important to ensure that all other factors are the same or at least as similar as possible. Things such as age, fitness level, and even occupation can affect the likelihood someone has a headache and whether a certain medication is effective.
Faced with this dynamic, researchers try to make sure that participants in their control group and experimental group are as similar as possible to each other, with the only difference being the treatment they receive.
Experimental research is often associated with scientists in lab coats holding beakers containing liquids with funny colors. Clinical trials that deal with medical treatments rely primarily, if not exclusively, on experimental research designs involving comparisons between control and experimental groups.
However, many studies in the social sciences also employ some sort of experimental design which calls for the use of control groups. This type of research is useful when researchers are trying to confirm or challenge an existing notion or measure the difference in effects.
Workplace efficiency research
How might a company know if an employee training program is effective? They may decide to pilot the program to a small group of their employees before they implement the training to their entire workforce.
If they adopt an experimental design, they could compare results between an experimental group of workers who participate in the training program against a control group who continues as per usual without any additional training.
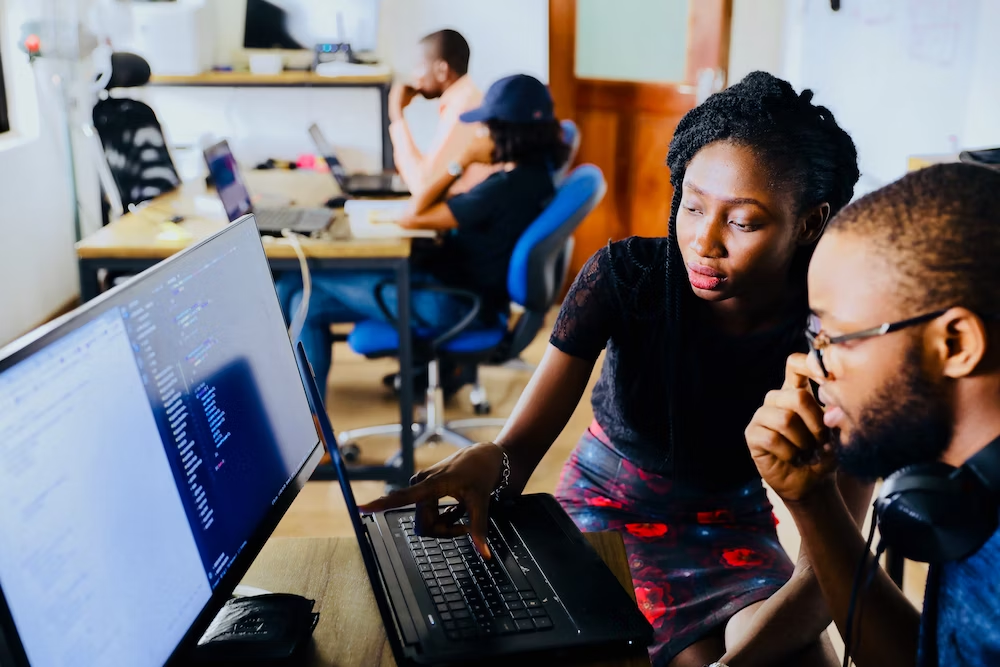
Qualitative data analysis starts with ATLAS.ti
Turn data into rich insights with our powerful data analysis software. Get started with a free trial.
Mental health research
Music certainly has profound effects on psychology, but what kind of music would be most effective for concentration? Here, a researcher might be interested in having participants in a control group perform a series of tasks in an environment with no background music, and participants in multiple experimental groups perform those same tasks with background music of different genres. The subsequent analysis could determine how well people perform with classical music, jazz music, or no music at all in the background.
Educational research
Suppose that you want to improve reading ability among elementary school students, and there is research on a particular teaching method that is associated with facilitating reading comprehension. How do you measure the effects of that teaching method?
A study could be conducted on two groups of otherwise equally proficient students to measure the difference in test scores. The teacher delivers the same instruction to the control group as they have to previous students, but they teach the experimental group using the new technique. A reading test after a certain amount of instruction could determine the extent of effectiveness of the new teaching method.
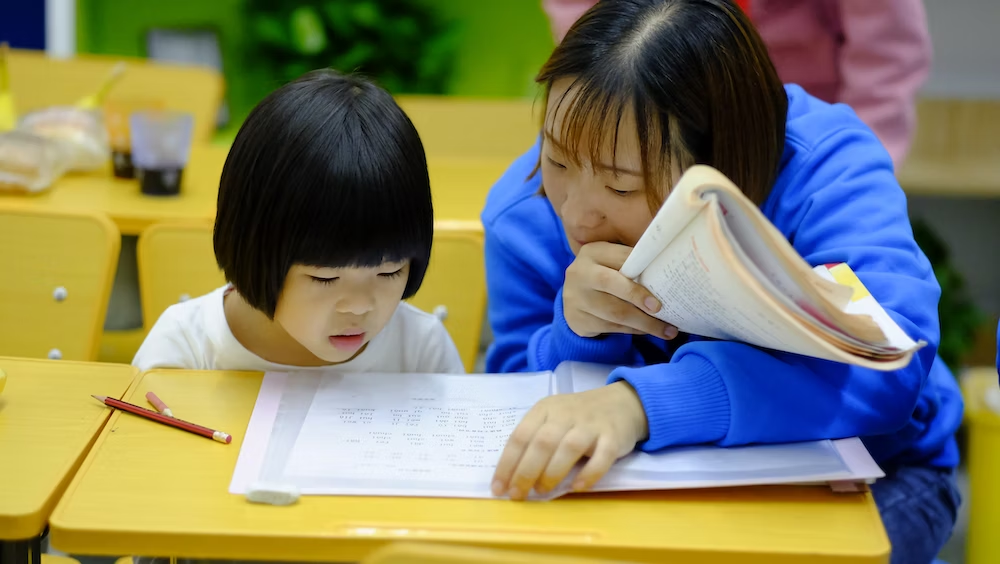
As you can see from the three examples above, experimental groups are the counterbalance to control groups. A control group offers an essential point of comparison. For an experimental study to be considered credible, it must establish a baseline against which novel research is conducted.
Researchers can determine the makeup of their experimental and control groups from their literature review . Remember that the objective of a review is to establish what is known about the object of inquiry and what is not known. Where experimental groups explore the unknown aspects of scientific knowledge, a control group is a sort of simulation of what would happen if the treatment or intervention was not administered. As a result, it will benefit researchers to have a foundational knowledge of the existing research to create a credible control group against which experimental results are compared, especially in terms of remaining sensitive to relevant participant characteristics that could confound the effects of your treatment or intervention so that you can appropriately distribute participants between the experimental and control groups.
There are multiple control groups to consider depending on the study you are looking to conduct. All of them are variations of the basic control group used to establish a baseline for experimental conditions.
No-treatment control group
This kind of control group is common when trying to establish the effects of an experimental treatment against the absence of treatment. This is arguably the most straightforward approach to an experimental design as it aims to directly demonstrate how a certain change in conditions produces an effect.
Placebo control group
In this case, the control group receives some sort of treatment under the exact same procedures as those in the experimental group. The only difference in this case is that the treatment in the placebo control group has already been judged to be ineffective, except that the research participants don't know that it is ineffective.
Placebo control groups (or negative control groups) are useful for allowing researchers to account for any psychological or affective factors that might impact the outcomes. The negative control group exists to explicitly eliminate factors other than changes in the independent variable conditions as causes of the effects experienced in the experimental group.
Positive control group
Contrasted with a no-treatment control group, a positive control group employs a treatment against which the treatment in the experimental group is compared. However, unlike in a placebo group, participants in a positive control group receive treatment that is known to have an effect.
If we were to use our first example of headache medicine, a researcher could compare results between medication that is commonly known as effective against the newer medication that the researcher thinks is more effective. Positive control groups are useful for validating experimental results when compared against familiar results.
Historical control group
Rather than study participants in control group conditions, researchers may employ existing data to create historical control groups. This form of control group is useful for examining changing conditions over time, particularly when incorporating past conditions that can't be replicated in the analysis.
Qualitative research more often relies on non-experimental research such as observations and interviews to examine phenomena in their natural environments. This sort of research is more suited for inductive and exploratory inquiries, not confirmatory studies meant to test or measure a phenomenon.
That said, the broader concept of a control group is still present in observational and interview research in the form of a comparison group. Comparison groups are used in qualitative research designs to show differences between phenomena, with the exception being that there is no baseline against which data is analyzed.
Comparison groups are useful when an experimental environment cannot produce results that would be applicable to real-world conditions. Research inquiries examining the social world face challenges of having too many variables to control, making observations and interviews across comparable groups more appropriate for data collection than clinical or sterile environments.
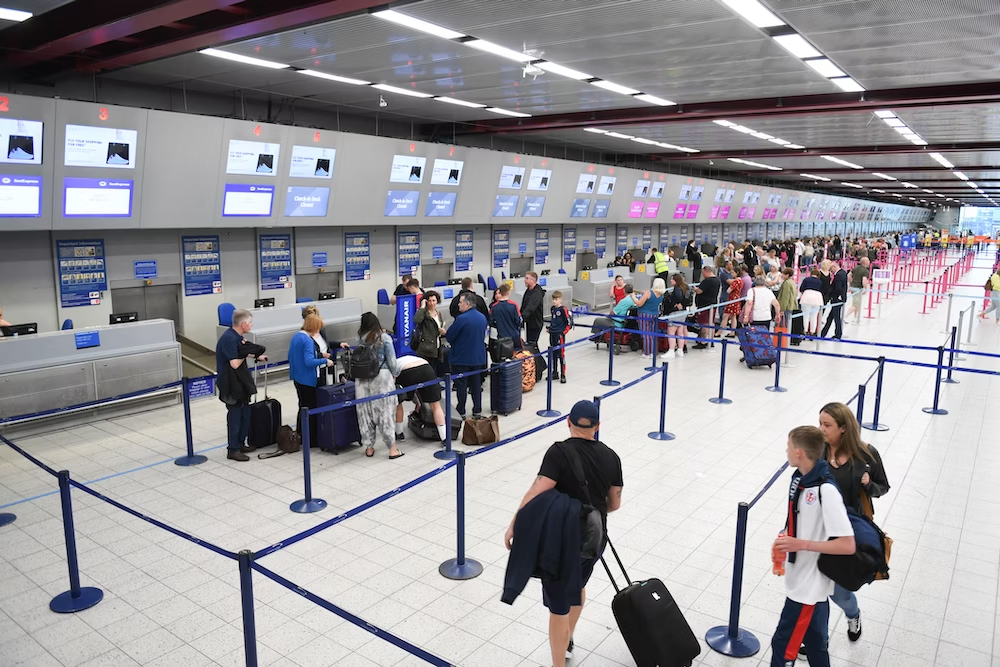
Analyze data and generate rich results with ATLAS.ti
Try out a free trial of ATLAS.ti to see how you can make the most of your qualitative data.
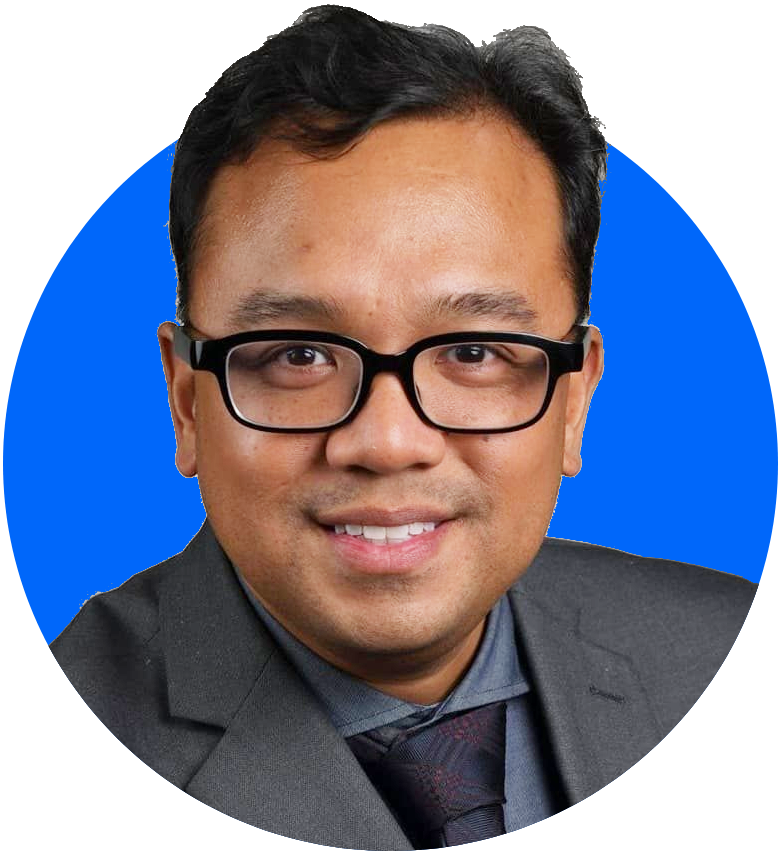
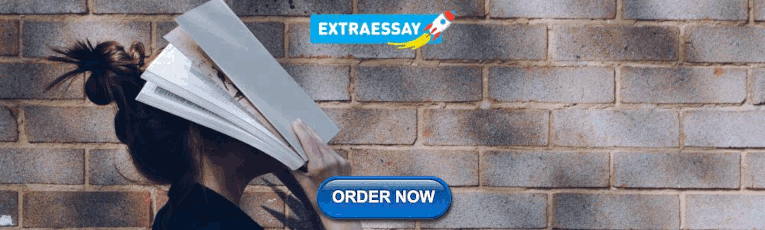
IMAGES
VIDEO
COMMENTS
In a scientific study, a control group is used to establish causality by isolating the effect of an independent variable. Here, researchers change the independent variable in the treatment group and keep it constant in the control group. Then they compare the results of these groups.
While the control group does not receive treatment, it does play a critical role in the experimental process. This group serves as a benchmark, allowing researchers to compare the experimental group to the control group to see what sort of impact changes to the independent variable produced.
The function of a control group is to act as a point of comparison, by attempting to ensure that the variable under examination (the impact of the medicine) is the thing responsible for creating the results of an experiment.
Explore the crucial role of control groups in scientific research, enhancing validity and ensuring accurate results. Control groups are a fundamental component of scientific research, serving as a benchmark to measure the effects of experimental treatments.
A control group is important because it allows meaningful comparison. The researcher compares the experimental group to it to assess whether or not there is a relationship between the independent and dependent variable and the magnitude of the effect.
control group, the standard to which comparisons are made in an experiment. Many experiments are designed to include a control group and one or more experimental groups; in fact, some scholars reserve the term experiment for study designs that include a control group.
Understand the design and interpretation of control group in research experiments for powerful conclusions. The control group constitutes a baseline for comparison, enabling researchers to assess the true effects of independent variables.
A control group in a scientific experiment is a group separated from the rest of the experiment, where the independent variable being tested cannot influence the results. This isolates the independent variable's effects on the experiment and can help rule out alternative explanations of the experimental results.
Controlled experiments require control groups. Control groups allow you to test a comparable treatment, no treatment, or a fake treatment (e.g., a placebo to control for a placebo effect), and compare the outcome with your experimental treatment.
A control group is typically thought of as the baseline in an experiment. In an experiment, clinical trial, or other sort of controlled study, there are at least two groups whose results are compared against each other.