8.1 Technology Today
Learning objectives.
By the end of this section, you should be able to:
- Define technology and describe its evolution
- Explain technological inequality and issues related to unequal access to technology
- Describe the role of planned obsolescence in technological development
It is easy to look at the latest virtual reality headset and think technology is a recent addition to our world. But from the steam engine to the most cutting-edge robotic surgery tools, technology has described the application of science to address the problems of daily life. We might roll our eyes at enormous and clunky computers of the 1970s that had far less storage than a free thumb drive. But chances are, twenty years from now our skinny laptops and pocket-filling phones will look just as archaic.
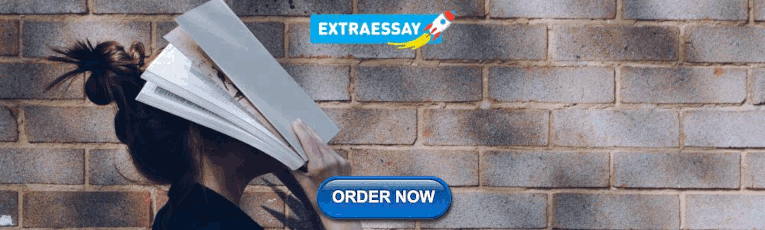
What Is Technology?
If someone asked your instructor what instructional technology they used, your instructor would likely assume the questioner was referring to courseware platforms, classroom response offerings, or presentation software. But if your instructor simply responded with, “pencil and paper,” they’d still be accurately describing technology. Modern paper and writing devices would have been considered fantastical creations in ancient times. And the fact that they endure, even as many other potential replacements have come into play, shows how effective those technologies are.
Just as the availability of digital technology shapes how we live today, the creation of stone tools changed how premodern humans lived and how well they ate. From the first calculator, invented in 2400 BCE Babylon in the form of an abacus, to the predecessor of the modern computer, created in 1882 by Charles Babbage, all of our technological innovations are advancements on previous iterations. And indeed, all aspects of our lives are influenced by technology. In agriculture, the introduction of machines that can till, thresh, plant, and harvest greatly reduced the need for manual labor, which in turn meant there were fewer rural jobs. This led to the urbanization of society, as well as lowered birth rates because there was less need for large families to work the farms. In the criminal justice system, the ability to ascertain innocence through DNA testing has saved the lives of people on death row. The examples are endless: technology plays a role in absolutely every aspect of our lives.
Technological Inequality
As with any improvement to human society, not everyone has equal access. Technology, in particular, often creates changes that lead to ever greater inequalities. In short, the gap gets wider faster. This technological stratification has led to a new focus on ensuring better access for all.
There are two forms of technological stratification. The first is differential class-based access to technology in the form of the digital divide. This digital divide has led to the second form, a knowledge gap , which is, as it sounds, an ongoing and increasing gap in information for those who have less access to technology. Simply put, students in well-funded schools receive more exposure to technology than students in poorly funded schools. Those students with more exposure gain more proficiency, which makes them far more marketable in an increasingly technology-based job market and leaves our society divided into those with technological knowledge and those without. Even as we improve access, we have failed to address an increasingly evident gap in e-readiness —the ability to sort through, interpret, and process knowledge (Sciadas 2003).
Since the beginning of the millennium, social science researchers have tried to bring attention to the digital divide , the uneven access to technology among different races, classes, and geographic areas. The term became part of the common lexicon in 1996, when then Vice President Al Gore used it in a speech. This was the point when personal computer use shifted dramatically, from 300,000 users in 1991 to more than 10 million users by 1996 (Rappaport 2009). In part, the issue of the digital divide had to do with communities that received infrastructure upgrades that enabled high-speed Internet access, upgrades that largely went to affluent urban and suburban areas, leaving out large swaths of the country.
At the end of the twentieth century, technology access was also a big part of the school experience for those whose communities could afford it. Early in the millennium, poorer communities had little or no technology access, while well-off families had personal computers at home and wired classrooms in their schools. In the 2000s, however, the prices for low-end computers dropped considerably, and it appeared the digital divide was naturally ending. Research demonstrates that technology use and Internet access still vary a great deal by race, class, and age in the United States, though most studies agree that there is minimal difference in Internet use by adult men and adult women.
Data from the Pew Research Center (Perrin 2019) suggests the emergence of yet another divide. Larger percentages of groups such as Latinos and African Americans use their phones rather than traditional computers to connect to the Internet and undertake related activities. Roughly eight in ten White people reported owning computers, in contrast to roughly six in ten Black and Hispanic people owning them. White people were also more likely to have broadband (high-speed Internet) in their homes. But approximately one in four Black and Hispanic people reported being smartphone-only Internet users, a number that far outpaces White people's reliance on the devices. While it might seem that the Internet is the Internet, regardless of how you get there, there’s a notable difference. Tasks like updating a résumé or filling out a job application are much harder on a cell phone than on a large-screen computer in the home. As a result, the digital divide might mean no access to computers or the Internet, but could mean access to the kind of online technology that allows for empowerment, not just entertainment (Washington 2011).
Another aspect of the digital divide is present in the type of community one lives in. Census data released in 2018 showed that in the study period of 2013 to 2017, 78 percent of U.S. households had Internet access, but that homes in rural and low-income areas were below that national average by 13 percent. The data was collected by county, and showed that "mostly urban" counties significantly outpaced "mostly rural" counties. "Completely rural," lower-income counties had the lowest rates of home Internet adoption, at about 60 percent (Martin 2019).
One potential outcome of reduced home Internet and computer access can be the relatively low representation of certain populations in computing courses, computing majors, and computing careers. Some school districts, often with the help of government grants or corporate sponsorships, aim to address this aspect of the digital divide by providing computers to those who need them, either at a low cost or at no charge. A number of organizations, such as Code.org, Black Girls Code, and Black Boys Code, work to overcome the disparity by offering computer science education programs and camps, collaborative instruction programs with local school districts, and (perhaps most impactful in the long term) teacher training programs. As a result, the number of Black and Hispanic students in courses like Advanced Placement Computer Science has increased dramatically in recent years, as has the number of college majors from the same populations.
As a whole, the digital divide brings some level of controversy. Some question why it still exists after having been identified more than twenty years ago. Others question whether or not it exists at all, and offer data to support the claim that it does not exist (American Press Institute 2015). However, most experts agree that the COVID-19 pandemic revealed that the digital divide has persisted, particularly in education. While millions of students were confined to home and remote instruction, they were divided by their Internet access, their familiarity with computer hardware and software, and their ability to solve their own technology issues (PRB 2020). Even when governments and educational institutions implemented improvements to the access and technology situation, there remained the qualitative aspect of unplanned remote education: Many instructors and students are not as effective while communicating only through computer screens. When considering education, policymakers faced arduous decision-making processes and contentious debates as they tried the balance the issues of safety, educational quality, teacher safety, student mental health, and the overall changing landscape of the pandemic.
Constant Contact and Replaced Relationships
How often do you check your phone for new messages or alerts? If you're typical, it might be over 100 times a day. (The number is difficult to cite with confidence, because every few months, organizations or companies release new studies claiming to have updated statistics.) What happens to your phone when you are sleeping? In 2012, researchers reported that “44% of cell phone owners have slept with their phone next to their bed because they wanted to make sure they didn’t miss any calls, text messages, or other updates during the night, and 29% of cell owners describe their cell phone as ‘something they can’t imagine living without’” (Smith 2012). Just three years later, a frequently cited report by Bank of America indicated the number of phone-accompanied sleepers was at 71 percent (Kooser 2015). A more recent survey of 500 people found it to be 66 percent, but that survey only included adults (Abbott 2020). However, these surveys and the reaction to them might be a factor of selective memory: Prior to the rise of cell phones, many people had telephones in their rooms, often within arm's reach of their bed.
While people report that cell phones make it easier to stay in touch, simplify planning, and increase their productivity, those are not the only impacts of constant device usage in the United States. Smith also reports that “roughly one in five cell owners say that their phone has made it at least somewhat harder to forget about work at home or on the weekends; to give people their undivided attention; or to focus on a single task without being distracted” (Smith 2012). As mentioned in the opening of this chapter, even celebrities who have perhaps benefitted the most from increased communication and social media report stress and concern about their online presence and its related outcomes.
With so many people using social media both in the United States and abroad, it is no surprise that social media is a powerful force for social change or political expression. For example, McKenna Pope, a thirteen-year-old girl, used the Internet to successfully petition Hasbro to fight gender stereotypes by creating a gender-neutral Easy-Bake Oven instead of using only the traditional pink color (Kumar 2014). Movements such as MeToo and Black Lives Matter gained prominence partly through what is sometimes referred to as "hashtag activism." More recently, TikTok users who actively opposed Donald Trump's re-election registered for nearly all the seats at a major rally. When they did not attend, the arena was nearly empty, after the campaign had predicted it would be overflowing. Later, Trump supporters used the social media site Parler in their own rally planning and coordination.
Such consistent and impactful usage leads to unavoidable results: Newer communication methods are replacing older ones. Speaking by phone seems archaic and almost intrusive for some people, who greatly prefer non-voice messaging apps or texts. Media observers, etiquette commentators, and friends and family may lament people beginning and ending relationships by text message, but those methods have proven more comfortable, especially for young people.
What are the effects? There have not been studies on every type of relationship, but research into romantic relationships shows interesting results. First, consider the elements of a relationship. One is attachment, or the bond that people form with each other. Research has shown that constant communication via messaging significantly increases the level of attachment. That fact seems intuitive: people who continually check in on each other, report their whereabouts, and offer support or affirmation will build a stronger bond. The same study, however, found that the respondents rated the overall quality of the relationship as weaker or less satisfying when it was dominated by text messaging instead of voice conversation (Luo 2014).
Many of us have experienced another aspect of relationships: reliance or imbalance. Researchers have found that close friends who are heavily reliant on mobile devices and associated messaging may have more issues regarding overdependence on the relationship and differing expectations regarding communication. Friendships that do not rely on mobile devices may have far less frequent contact. Research found elements of guilt and pressure to respond (called entrapment) in mobile-dependent relationships, which led to overall dissatisfaction (Hall 2012).
Online Privacy, Security, and Control
As we increase our footprints on the web by going online more often to connect socially, share material, conduct business, and store information, we also increase our vulnerability to those with negative intent. Most Americans seem to accept that increased usage of online and related tools brings risks, but their perceptions of those risks are evolving. For example, people have different viewpoints on risks associated with individuals, companies, and the government. The Pew Research Center conducts frequent surveys on these topics. A recent publication indicated the following:
- 81 percent of people felt they had little control over the data collected by companies; 84 percent felt they had little control over data collected by the government.
- 62 percent felt that it was not possible to go through the day without having data collected about them by companies; 63% felt it wasn't possible to go through a day without data collection by the government.
- 79 percent were concerned about that data use by companies; 64 percent were concerned about data use by the government.
Other elements of the research demonstrate that older Americans felt more concern than younger ones, and that Black and Hispanic people were more likely than White people to believe the government was tracking them (Auxier 2019).
These attitudes may be revealed by practices or attitudes toward privacy efforts and safeguards. One person may be annoyed every time a privacy notice interrupts them, and they may simply sign the statement without thinking much about it. Another person may read every word of the agreement and carefully deliberate over whether to proceed.
Online privacy concerns also extend from individuals to their dependents. In accordance with the Child Online Privacy Protection Act, school districts must consider and control certain elements of privacy on behalf of students, meaning they cannot require or encourage students under age thirteen to provide personal information. Likewise, online platforms such as Instagram do not let children under the age of thirteen register for their sites. And where children are registered by their parents, sites like YouTube and, more recently, TikTok issue controls to prevent inappropriate portrayals by children or inappropriate behavior by other members. For example, YouTube often disables comments on videos produced by children (Moreno 2020). TikTok added privacy and protection methods in 2020, but in early 2021 was hit with allegations of violating child safety and privacy guidelines.
Although schools and companies are required to take steps to lower risks to children, parents and guardians are free to make their own choices on behalf of their children. Some parents avoid showing their children on social media; they do not post pictures, and ask family members to refrain from doing so (Levy, 2019). On the other end of the spectrum, some parents run social media accounts for their children. Sometimes referred to as "sharents," they may share entertaining videos, promote products through demos or try-ons, or post professionally produced photos on behalf of clothing companies or equipment makers. A child's (even a toddler's) role as an influencer can be financially lucrative, and companies making everything from helmets to dancewear have taken notice (Allchin 2012).
Net Neutrality
The issue of net neutrality , the principle that all Internet data should be treated equally by Internet service providers, is part of the national debate about Internet access and the digital divide. On one side of this debate is the belief that those who provide Internet service, like those who provide electricity and water, should be treated as common carriers, legally prohibited from discriminating based on the customer or nature of the goods. Supporters of net neutrality suggest that without such legal protections, the Internet could be divided into “fast” and “slow” lanes. A conflict perspective theorist might suggest that this discrimination would allow bigger corporations, such as Amazon, to pay Internet providers a premium for faster service, which could lead to gaining an advantage that would drive small, local competitors out of business.
The other side of the debate holds the belief that designating Internet service providers as common carriers would constitute an unreasonable regulatory burden and limit the ability of telecommunication companies to operate profitably. A functional perspective theorist might point out that, without profits, companies would not invest in making improvements to their Internet service or expanding those services to underserved areas. The final decision rests with the Federal Communications Commission and the federal government, which must decide how to fairly regulate broadband providers without dividing the Internet into haves and have-nots.
As an Amazon Associate we earn from qualifying purchases.
This book may not be used in the training of large language models or otherwise be ingested into large language models or generative AI offerings without OpenStax's permission.
Want to cite, share, or modify this book? This book uses the Creative Commons Attribution License and you must attribute OpenStax.
Access for free at https://openstax.org/books/introduction-sociology-3e/pages/1-introduction
- Authors: Tonja R. Conerly, Kathleen Holmes, Asha Lal Tamang
- Publisher/website: OpenStax
- Book title: Introduction to Sociology 3e
- Publication date: Jun 3, 2021
- Location: Houston, Texas
- Book URL: https://openstax.org/books/introduction-sociology-3e/pages/1-introduction
- Section URL: https://openstax.org/books/introduction-sociology-3e/pages/8-1-technology-today
© Jan 18, 2024 OpenStax. Textbook content produced by OpenStax is licensed under a Creative Commons Attribution License . The OpenStax name, OpenStax logo, OpenStax book covers, OpenStax CNX name, and OpenStax CNX logo are not subject to the Creative Commons license and may not be reproduced without the prior and express written consent of Rice University.
- Skip to main content
- Keyboard shortcuts for audio player
How Tech Has Changed Our Lives In The Last 10 Years
Several tech experts weigh in on the technologies of the past decade that had the greatest impact on society.
Copyright © 2019 NPR. All rights reserved. Visit our website terms of use and permissions pages at www.npr.org for further information.
NPR transcripts are created on a rush deadline by an NPR contractor. This text may not be in its final form and may be updated or revised in the future. Accuracy and availability may vary. The authoritative record of NPR’s programming is the audio record.
Module 18: Social Movements and Social Change
Social change, technology, and modernization, learning outcomes.
- Describe the influence of media and technology on social change and modernization
We know that technology is one of the major factors that leads to social change. Let’s take a deeper look at how technology and the media play a major role in the accelerated rate of globalization, which in turn, affects social change.
Social Movements and Technology
So what is the real impact of this technology on the world? Did Twitter bring down Mubarak in Egypt? Author Malcolm Gladwell (2010) doesn’t think so. In an article in The New Yorker , Gladwell tackles what he considers the myth that social media gets people more engaged. He points out that most of the tweets relating to the Iran protests were in English and sent from Western accounts (instead of people on the ground). Rather than increasing engagement, he contends that social media only increases participation; after all, the cost of participation is so much lower than the cost of engagement. Instead of risking being arrested, shot with rubber bullets, or sprayed with fire hoses, social media activists can click “like” or retweet a message from the comfort and safety of their desk (Gladwell 2010).
There are, though, good cases to be made for the power of social media in propelling social movements. In the article, “Parrhesia and Democracy: Truth-telling, WikiLeaks and the Arab Spring,” Theresa Sauter and Gavin Kendall (2011) described the importance of social media in the Arab Spring uprisings. Parrhesia means “the practice of truth-telling,” which describes the protestors’ use of social media to make up for the lack of coverage and even misrepresentation of events by state-controlled media. The Tunisian blogger Lina Ben Mhenni posted photographs and videos on Facebook and Twitter of events exposing the violence committed by the government. In Egypt, the journalist Asmaa Mahfouz used Facebook to gather large numbers of people in Tahrir Square in the capital city of Cairo. Sauter and Kendall maintain that it was the use of Web 2.0 technologies that allowed activists not only to share events with the world but also to organize the actions.
When the Egyptian government shut down the Internet to stop the use of social media, the group Anonymous, a hacking organization noted for online acts of civil disobedience initiated “Operation Egypt” and sent thousands of faxes to keep the public informed of their government’s activities (CBS Interactive Inc. 2014) as well as attacking the government’s web site (Wagensiel 2011). In its Facebook press release the group stated the following: “Anonymous wants you to offer free access to uncensored media in your entire country. When you ignore this message, not only will we attack your government websites, Anonymous will also make sure that the international media sees the horrid reality you impose upon your people.”
Sociologists have identified high-risk activism, such as the civil rights movement, as a “strong-tie” phenomenon, meaning that people are far more likely to stay engaged and not run home to safety if they have close friends who are also engaged. The people who dropped out of the movement––who went home after the danger got too great––did not display any less ideological commitment. But they lacked the strong-tie connection to other people who were staying. Social media, by its very makeup, is “weak-tie” (McAdam and Paulsen 1993). People follow or friend people they have never met. But while these online acquaintances are a source of information and inspiration, the lack of engaged personal contact limits the level of risk we’ll take on their behalf.
These weak-tie connections can still affect change, especially as online activism becomes the norm. For example, look at this Wikipedia page explaining Hashtag Activism , to learn more about movements that gained popularity through Twitter hashtags.
Use of Technology and Social Media in Society by Individuals
Research conducted in 2018 showed that 95% of Americans own a cell phone, and 77% of Americans own a smartphone. [1] Access to the internet is often within arm’s reach—26% of people surveyed in 2018 said they were online almost constantly, while 43% said they got online several times a day. [2]
While people report that cell phones make it easier to stay in touch, simplify planning and scheduling their daily activities, and increase their productivity, that’s not the only impact of increased cell phone ownership in the United States. Smith also reports that “roughly one in five cell owners say that their phone has made it at least somewhat harder to forget about work at home or on the weekends; to give people their undivided attention; or to focus on a single task without being distracted” (Smith 2012).
Planned Obsolescence: Technology That’s Built to Crash
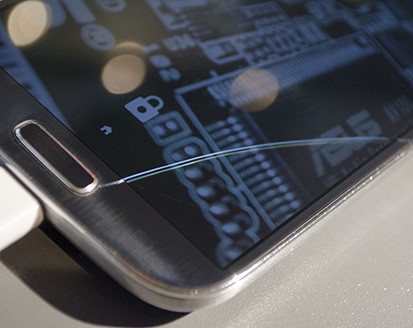
Figure 1. People have trouble keeping up with technological innovation. But people may not be to blame, as manufacturers intentionally develop products with short life spans. (Photo courtesy of Mathias F. Svendsen/flickr)
Chances are your mobile phone company, as well as the makers of your laptop and your household appliances, are all counting on their products to fail. Not too quickly, of course, or consumers wouldn’t stand for it—but frequently enough that you might find that it costs far more to fix a device than to replace it with a newer model. Or you find the phone company e-mails you saying that you’re eligible for a free new phone, because yours is a whopping two years old. And appliance repair people say that while they might be fixing some machines that are twenty years old, they generally aren’t fixing those that are seven years old; newer models are built to be thrown out. This strategy is called planned obsolescence , and it is the business practice of planning for a product to be obsolete or unusable from the time it is created.
To some extent, planned obsolescence is a natural extension of new and emerging technologies. After all, who is going to cling to an enormous and slow desktop computer from 2000 when a few hundred dollars can buy one that is significantly faster and better? But the practice is not always so benign. The classic example of planned obsolescence is the nylon stocking. Women’s stockings—once an everyday staple of women’s lives––get “runs” or “ladders” after only a few wearings. This requires the stockings to be discarded and new ones purchased. Not surprisingly, the garment industry did not invest heavily in finding a rip-proof fabric; it was in manufacturers’ best interest that their product be regularly replaced.
Those who use Microsoft Windows might feel that like the women who purchased endless pairs of stockings, they are victims of planned obsolescence. Every time Windows releases a new operating system, there are typically not many innovations in it that consumers feel they must have. However, the software programs are upwardly compatible only. This means that while the new versions can read older files, the old version cannot read the newer ones. In short order, those who have not upgraded right away find themselves unable to open files sent by colleagues or friends, and they usually wind up upgrading as well.
Ultimately, whether you are getting rid of your old product because you are being offered a shiny new free one (like the latest smartphone model), or because it costs more to fix than to replace (like the iPod model), or because not doing so leaves you out of the loop (like the Windows model), the result is the same. It might just make you nostalgic for your old Sony Discman and simple DVD player.
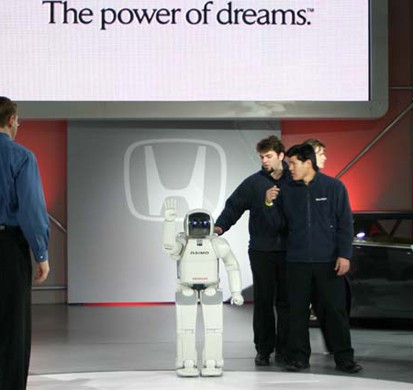
Figure 2. In the coming future, there is no doubt that robots are going to play a large role in all aspects of our lives. (Photo courtesy of shay sowden/flickr)
With so many people using social media both in the United States and abroad, it is no surprise that social media is a powerful force for social change. Spreading democracy, as evidenced in the Arab Spring example, is just the tip of the iceberg when it comes to using social media to incite change. For example, McKenna Pope, a thirteen-year-old girl, used the Internet to successfully petition Hasbro to fight gender stereotypes by creating a gender-neutral Easy-Bake Oven instead of using only the traditional pink color (Kumar 2014).
Product Advertising
Companies use advertising to sell to us, but the way they reach us is changing. Naomi Klein identified the destructive impact of corporate branding her 1999 text, No Logo , an anti-globalization treatise that focused on sweatshops, corporate power, and anti-consumerist social movements. In the post-millennial society, synergistic advertising practices ensure you are receiving the same message from a variety of sources and on a variety of platforms. For example, you may see billboards for Miller beer on your way to a stadium, sit down to watch a game preceded by a Miller commercial on the big screen, and watch a halftime ad in which people are shown holding up the trademark bottles. Chances are you can guess which brand of beer is for sale at the concession stand.
Advertising has changed, as technology and media have allowed consumers to bypass traditional advertising venues. From the invention of the remote control, which allows us to skip television advertising without leaving our seats, to recording devices that let us watch programs but skip the ads, conventional television advertising is on the wane. And print media is no different. Advertising revenue in newspapers and on television fell significantly in 2009, which shows that companies need new ways of getting their messages to consumers.
One model companies are considering to address this advertising downturn uses the same philosophy as celebrity endorsements, just on a different scale. Companies are hiring college students to be their on-campus representatives, and they are looking for popular students engaged in high-profile activities like sports, fraternities, and music. The marketing team is betting that if we buy perfume because Beyoncé tells us to, we’ll also choose our cell phone or smoothie brand if a popular student encourages that choice. According to an article in the New York Times , fall semester 2011 saw an estimated 10,000 U.S. college students working on campus as brand ambassadors for products from Red Bull energy drinks to Hewlett-Packard computers (Singer 2011). As the companies figure it, college students will trust one source of information above all: other students.
Technological Concerns
With all of the new media (social networking sites, blogs, podcasts, wikis, and virtual worlds, etc.) available to us, there are also some new concerns regarding privacy, security, equality, and polarization. Additionally, there is no guarantee that the information offered by new media is accurate. In fact, the immediacy of new media coupled with the lack of oversight means we must be more careful than ever to ensure our news is coming from accurate sources.
Online Privacy and Security
As we increase our footprints on the web by going online more often to connect socially, share material, conduct business, and store information, we also increase our vulnerability to those with criminal intent. The Pew Research Center recently published a report that indicated the number of Internet users who express concern over the extent of personal information about them available online jumped 17 percent between 2009 and 2013. In that same survey, 12 percent of respondents indicated they had been harassed online, and 11 percent indicated that personal information, such as their Social Security number, had been stolen (Rainie, Kiesler, Kang, and Madden 2013).
Online privacy and security is a key organizational concern as well. Recent large-scale data breaches at retailers such as Target, financial powerhouses such as JP Morgan, the government health insurance site Healthcare.gov, and cell phone providers such as Verizon, exposed millions of people to the threat of identity theft when hackers got access to personal information by compromising website security.
For example, in late August 2014, hackers breached the iCloud data storage site and promptly leaked wave after wave of nude photos from the private accounts of actors such as Jennifer Lawrence and Kirsten Dunst (Lewis 2014). While large-scale data breaches that affect corporations and celebrities are more likely to make the news, individuals may put their personal information at risk simply by clicking a suspect link in an official sounding e-mail.
How can individuals protect their data? Numerous facts sheets available through the government, nonprofits, and the private sector outline common safety measures, including the following: become familiar with privacy rights; read privacy policies when making a purchase (rather than simply clicking “accept”); give out only the minimum information requested by any source; ask why information is being collected, how it is going to be used, and who will have access it; and monitor your credit history for red flags that indicate your identity has been compromised.
Net Neutrality
The issue of net neutrality , the principle that all Internet data should be treated equally by Internet service providers, is part of the national debate about Internet access and the digital divide. On one side of this debate is the belief that those who provide Internet service, like those who provide electricity and water, should be treated as common carriers, legally prohibited from discriminating based on the customer or nature of the goods. Supporters of net neutrality suggest that without such legal protections, the Internet could be divided into “fast” and “slow” lanes. A conflict perspective theorist might suggest that this discrimination would allow bigger corporations, such as Amazon, to pay Internet providers a premium for faster service, which could lead to gaining an advantage that would drive small, local competitors out of business.
The other side of the debate holds the belief that designating Internet service providers as common carriers would constitute an unreasonable regulatory burden and limit the ability of telecommunication companies to operate profitably. A functional perspective theorist might point out that, without profits, companies would not invest in making improvements to their Internet service or expanding those services to underserved areas. The final decision rests with the Federal Communications Commission and the federal government, which must decide how to fairly regulate broadband providers without dividing the Internet into haves and have-nots.
Violence in Media and Video Games: Does It Matter?
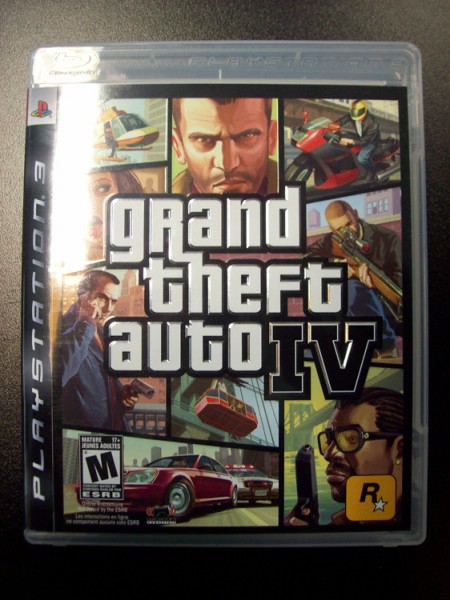
Figure 3. One of the most popular video games, Grand Theft Auto, has frequently been at the center of debate about gratuitous violence in the gaming world. (Photo courtesy of Meddy Garnet/flickr)
A glance through popular video game and movie titles geared toward children and teens shows the vast spectrum of violence that is displayed, condoned, and acted out.
As a way to guide parents in their programming choices, the motion picture industry put a rating system in place in the 1960s. But new media—video games in particular—proved to be uncharted territory. In 1994, the Entertainment Software Rating Board (ERSB) set a ratings system for games that addressed issues of violence, sexuality, drug use, and the like. California took it a step further by making it illegal to sell video games to underage buyers. The case led to a heated debate about personal freedoms and child protection, and in 2011, the U.S. Supreme Court ruled against the California law, stating it violated freedom of speech (ProCon 2012).
Children’s play has often involved games of aggression—from cowboys and Indians, to cops and robbers, to fake sword fights. Many articles report on the controversy surrounding the suggested link between violent video games and violent behavior. Is the link real? Psychologists Anderson and Bushman (2001) reviewed forty-plus years of research on the subject and, in 2003, determined that there are causal linkages between violent video game use and aggression. They found that children who had just played a violent video game demonstrated an immediate increase in hostile or aggressive thoughts, an increase in aggressive emotions, and physiological arousal that increased the chances of acting out aggressive behavior (Anderson 2003).
Ultimately, repeated exposure to this kind of violence leads to increased expectations that violence is a solution, increased violent behavioral scripts, and an increased cognitive accessibility to violent behavior (Anderson 2003). In short, people who play a lot of these games find it easier to imagine and access violent solutions than nonviolent ones, and they are less socialized to see violence as a negative. While these facts do not mean there is no role for video games, it should give players pause. In 2013, The American Psychological Association began an expansive meta-analysis of peer-reviewed research analyzing the effect of media violence. Results are expected in 2014.
Homogenization and Fragmentation
Despite the variety of media at hand, the mainstream news and entertainment you enjoy are increasingly homogenized. Research by McManus (1995) suggests that different news outlets all tell the same stories, using the same sources, resulting in the same message, presented with only slight variations. So whether you are reading the New York Times or the CNN’s web site, the coverage of national events like a major court case or political issue will likely be the same.
Simultaneously with this homogenization among the major news outlets, the opposite process is occurring in the newer media streams. With so many choices, people increasingly customize their news experience, minimizing their opportunity to encounter information that does not jive with their worldview (Prior 2005). For instance, those who are staunchly Republican can avoid centrist or liberal-leaning cable news shows and web sites that would show Democrats in a favorable light. They know to seek out Fox News over MSNBC, just as Democrats know to do the opposite. Further, people who want to avoid politics completely can choose to visit web sites that deal only with entertainment or that will keep them up to date on sports scores. They have an easy way to avoid information they do not wish to hear.
Further Research
- To learn more about new media, visit newmedia.org .
- To understand how independent media coverage differs from major corporate affiliated news outlets, review material from the Democracy Now! website.
- Watch this RSA Animate video “The Internet in Society: Empowering or Censoring Citizens?” to learn more about the power of the internet and its role in global politics.
Modernization
Modernization describes the processes that increase the amount of specialization and differentiation of structure in societies resulting in the move from an undeveloped society to a developed, technologically driven society (Irwin 1975). By this definition, the level of modernity within a society is judged by the sophistication of its technology, particularly as it relates to infrastructure, industry, and the like. However, it is important to note the inherent ethnocentric bias of such assessment. Why do we assume that those living in semi-peripheral and peripheral nations would find it so wonderful to become more like the core nations? Is modernization always positive?
One contradiction of all kinds of technology is that they often promise time-saving benefits, but somehow fail to deliver. How many times have you ground your teeth in frustration at an Internet site that refused to load or at a dropped call on your cell phone? Despite time-saving devices such as dishwashers, washing machines, and, now, remote control vacuum cleaners, the average amount of time spent on housework is the same today as it was fifty years ago. And the dubious benefits of 24/7 e-mail and immediate information have simply increased the amount of time employees are expected to be responsive and available. While once businesses had to travel at the speed of the U.S. postal system, sending something off and waiting until it was received before the next stage, today the immediacy of information transfer means there are no such breaks.
Further, the Internet bought us information, but at a cost. The morass of information means that there is as much poor information available as trustworthy sources. There is a delicate line to walk when core nations seek to bring the assumed benefits of modernization to more traditional cultures. For one, there are obvious pro-capitalist biases that go into such attempts, and it is short-sighted for western governments and social scientists to assume all other countries aspire to follow in their footsteps. Additionally, there can be a kind of neo-liberal defense of rural cultures, ignoring the often crushing poverty and diseases that exist in peripheral nations and focusing only on a nostalgic mythology of the happy peasant. It takes a very careful hand to understand both the need for cultural identity and preservation as well as the hopes for future growth.
Think It Over
- Where and how do you get your news? Do you watch network television? Read the newspaper? Go online? How about your parents or grandparents? Do you think it matters where you seek out information? Why, or why not?
- Document your screen time usage for one 24-hour period. Some phones have built in trackers and there are a variety of free apps to track cell phone screen time. Challenge yourself by documenting how many times you touch your phone and/or look at your phone. Does it also serve as your timepiece? Do you “check” your phone just to see what is there? Do you fiddle with your phone (in a pocket or bag) even when you are not actively using it? How often do you check social media? How many different types of alerts do you receive? Share your responses with your classmates. Were you surprised by the results? Did your habits change because you knew they would be tracked?
- If you are up for another challenge, go for a 24-hour period with essential phone use only. Since most of us use our cell phones as our only means of communication, it might be unreasonable to shut your phone down for the day, especially if you have family or work matters that might require your immediate attention. Other than “emergency-type” situations (you could set a special ring tone), try to not touch or look at your phone for 24 hours. Instead of documenting usage, document each time you thought about “checking something” on your phone. Share your responses with your classmates. Were you surprised by the results? Did your habits change because you knew they would be tracked?
- Can you think of people in your own life who support or defy the premise that access to technology leads to greater opportunities? How have you noticed technology use and opportunity to be linked, or does your experience contradict this idea?
- Should the U.S. government be responsible for providing all citizens with access to the Internet? Or is gaining Internet access an individual responsibility?
How have digital media changed social interactions? Do you believe it has deepened or weakened human connections? Defend your answer.
Conduct sociological research. Google yourself. How much information about you is available to the public? How many and what types of companies offer private information about you for a fee? Compile the data and statistics you find. Write a paragraph or two about the social issues and behaviors you notice.
- Mobile Fact Sheet (February 2018). Pew Research Center. Retrieved from https://www.pewinternet.org/fact-sheet/mobile/ ↵
- Perrin, Andrew and Jiang JingJing (March 2018). Pew Research Center. About a quarter of U.S. adults say they are ‘almost constantly’ online. Retrieved from https://www.pewresearch.org/fact-tank/2019/07/25/americans-going-online-almost-constantly/ . ↵
- Social Change. Authored by : OpenStax CNX. Located at : https://cnx.org/contents/[email protected]:vi4eB2eh@5/Social-Change . License : CC BY: Attribution . License Terms : Download for free at http://cnx.org/contents/[email protected]
- Modernization. Provided by : OpenStax College. Located at : https://cnx.org/contents/[email protected]:vi4eB2eh@9/21-3-Social-Change . License : CC BY: Attribution . License Terms : Download for free at http://cnx.org/contents/[email protected].
- Technology Today. Provided by : OpenStax College. Located at : https://cnx.org/contents/[email protected]:_5jASD-H@12/8-1-Technology-Today . License : CC BY: Attribution . License Terms : Download for free at http://cnx.org/contents/[email protected].
- Media and Technology in Society. Provided by : OpenStax College. Located at : https://cnx.org/contents/[email protected]:_U5AN6pQ@11/8-2-Media-and-Technology-in-Society . License : CC BY: Attribution . License Terms : Download for free at http://cnx.org/contents/[email protected].
- RSA ANIMATE: The Internet in Society: Empowering or Censoring Citizens?. Authored by : The RSA. Located at : https://www.youtube.com/watch?v=Uk8x3V-sUgU . License : All Rights Reserved
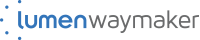
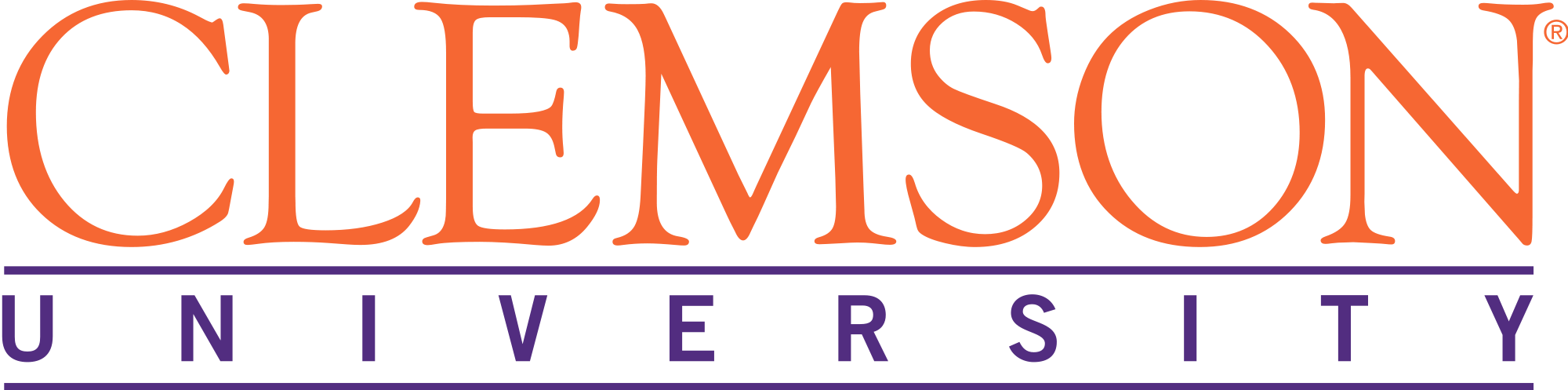
Want to create or adapt books like this? Learn more about how Pressbooks supports open publishing practices.
Part 1: Theories and Concepts of STS
5 Technological Determinism
Belle Salsone; Peter Sebastian Stein; Kaleb Gage Parsons; Thomas Kent; Krystal Nielsen; and David Thomas Nitz
We all know technology as an important part of our daily lives. Humans use tools everyday in order to make our lives easier. We use language to communicate with others, and we drive cars in order to get us from place to place, and as such a prevalent aspect of human life, it makes us question, “what exactly is technology? Where did it come from? and does technology drive history?” The theory of technological determinism states that societal growth does in fact follow an inevitable course that is propelled by technological innovation. It brings light to the positive effects STS has on our lives
A simple example of this concept is the development of the wheel. It “revolutionized human mobility, allowing humans to travel greater distances and carry greater loads with them” (Drew 2021). From this one can conclude that this technological advancement heavily impacted the course of human history, thus substantiating the concept of technological determinism. In this chapter, more examples and concepts surrounding this idea that technology drives history will be discussed. www.communicationtheory.org
There are those who feel as though technology hasn’t influenced change to occur in society or believe that technology isn’t the main factor that causes society to progress. Many times people disagree with technological determinism because they focus on other factors that prompted the societal change being discussed instead of just focusing on the technology driven change. Some may even disagree with the theory because they interpret it as saying technology controls their lives, not them.
One of the more outspoken critics of technological determinism is Daniel Chandler. He believes that while technology does contribute to societal change, it isn’t the only factor causing the change. He states that many technological determinists have a reductionist interpretation, meaning they only see technology being the main factor of change, being the only cause. Chandler explains his theory in which societal change is multicausal rather than monocausal, meaning society’s progression is driven by multiple causes like economics, politics and education (Chandler, 2000). He goes on to say that technological determinism puts technology above society, which makes people feel hopeless to change society by themselves, thus causing a ‘self-fulfilling prophecy’ (Harrison, 2013).
Another position people have taken against technological determinism is that technology isn’t the causation factor, but rather a tool that could be used to assist in the evolution of society. As the American historian Lynn White writes “a new device merely opens a door; it does not compel one to enter” (White, 1971), meaning technology produces advancement if one allows it to. Another American academic, Ithiel de Sola Pool, states “Technology shapes the structure of the battle but not every outcome” (Finnegan et al., 1987), which implies that technology is a factor of change, but there are other factors as well that can prompt progression.
Relationship to STS
Which is more influential – technology over society or society over technology?
Many people believe that technology has a negative impact on society. Others believe that technology has no impact on society, and it is humans who take the technology and create negative impacts. For that reason, Technological Determinism is often called a “critics term.” To elaborate, “technological determinism” is predominantly employed as a critic’s term, used to dismiss certain classes of theoretical and empirical claims.”
The central issue here is agency. To what extent of control do we have? Is technology thrust upon us with some internal implications controlling our path dependency?
One critique is that “technology never forces itself on members of the society. Man creates technology and choses to use them…There is no imposition on the part of the technology to be used rather technology requires people to participate or involve themselves at some point or another to use a car or a microwave. The choice of using technology and experiencing its effects therefore lies in the hands of a human being (Communication Theory, 2018).”
Many technology scholars believed that technology developments would become out of control and shape society in drastic ways. They based this belief on the fact that their studies of technology showed that over time, technology would impact society in ways that creators did not intend (Drinkwater, 2018).
In short, there are two viewpoints when it comes to the debate of whether technology influences society or if society influences technology. While it is clear that both influence each other, scholars debate over which has more influence. The first group called the technological determinists chose the side that technology has the most impact on society, and that society has little to no say as to how technologies evolve. The opposing side of social determinists believe that society drives technological development. This group may claim that “We shape our technology, then technology shapes us (Drinkwater, 2018).”
Japan displays good examples of a social deterministic view of technology. The first example being that their toilets are very elaborate compared to Western-style toilets. Japanese toilets have a control panel with many settings including heated toilet seats, bidet-style functions, and sound masking features. These settings are incorporated into their toilets because of their high societal regard for cleanliness, which is

The technological determinist side of the debate argues that technology has shaped our lives dramatically in small and large ways. Perhaps smartphones can be an example of how technology has drastically influenced our daily habits. While smartphones have connected people from all different corners of the world, it has also been a relentless distraction for most smartphone owners. It’s undeniable that smartphones have benefited our lives greatly. We can conduct bank transactions, rescue workers can pinpoint exactly where help is needed, we can track how well we are sleeping at night, all from the ease of our fingertips. We rely on apps with mapping capabilities to direct us to places over memory. It has now become habitual for many people to use map apps to drive or walk somewhere we know how to get to heart. The question is: at what cost do these benefits come at? A person’s social and mental health can be at risk from the constant use of smartphones. Some people have even gone as far to say that smartphones can be as addictive and seen as somewhat of a drug. The irony is that although smartphones allow us to be constantly connected to each other, they are also constantly distracting us from the real world, and we are getting lost in virtual reality. People are consumed by phones, as you can see from the dozens of bowed heads staring at their phone, anywhere you go (Bhattacharjee, 2019).
At the University of Texas at Austin, psychologist Adrian Ward conducted a study where he challenged 800 participants with mental tasks. The challenge was to solve a math problem while memorizing a random sequence of letters, then selecting an image out of a group of options to make a visual pattern. One group of participants were asked to leave their smartphones in another room. The other group kept their phones in their pockets or in front of them, however, the participants were not allowed to use their phones during the tasks. The group that did not have their phone with them did better on the tasks than the group that had access to their phones. The psychologist concluded that smartphones did cause people to feel distracted, even though they were not using them, and diminished their cognitive abilities (Bhattacharjee, 2019).
Another study was conducted at the University of Columbia, to attest to the fact that smartphones adversely affect social interaction. The researchers gathered 300 participants to have dinner at a restaurant with friends or family. One group was asked to leave their phones on the table and the other group was asked to put them away. The group with their phones felt more distracted, and bored, enjoying their meal less than the group without their phone. Ethan Kross, a psychologist at the University of Michigan explained why something that is supposedly entertaining as a smartphone, would make us enjoy our meal less, “It’s well known that if you want to keep a person dialed into something, give them a reward at variable times. Turns out, that’s exactly what email or social media does– you don’t know when you’ll get another like or receive your next email, and so we keep checking (Bhattacharjee, 2019). The compulsive urge to constantly check your phone can be attributed to the fact that technology companies can carefully orchestrate apps and websites to keep you hooked and coming back for more. These companies create features to conduct positive reinforcement, encouraging smartphone owners to constantly check their favorite apps (Bhattacharjee, 2019).
As a response to these criticisms, there are now apps to track screen time, although there is no proof that this monitoring has users change their habits. A study was conducted of high school students as participants. Of these participants who checked their screen time monitoring apps on an occasional basis and saw they were spending more time than they originally thought, only about half did nothing to change their behavior (Bhattacharjee, 2019).
The question that still remains is the question of agency: what extent of control do we have? There is no doubt that technology influences our lives significantly, however; we do have complete freedom to use or to not use technology. We, as humans, need to actively work to not lose our sense of agency and control over technology. Right now, we are guided by technology. However, this is because we allow it. Literature’s popular trope that a creation can take on a life of its own, and the creator loses control of it, is a narrative that has warned future generations of the power of technology. Frankenstein and his monster is just one literature example of how innovation and endless possibility can lead to loss of control and destruction. We, as humans, need to analyze the complex relationship of humans and technology. From there, we can direct technological innovation as opposed to letting it direct us (Jain, 2021).
To further determine if technology shapes us or if we shape technology, we can examine human sentiment about technological innovation. A survey was conducted in 2017 that asked participants if they felt enthusiastic or worried about, “the increased role of automation in different facts of life, such as self-driving vehicles, robot caretakers, for older adults and, more generally, a future of machines performing jobs previously filled by humans (Jain, 2021).” In every category, there were more people that expressed worriedness than enthusiasm. Another 2019 study showed that, “nearly twice as many Americans believe automation has done more harm than good.” What this tells us is that people do feel as if technology shapes us, not vice versa. Humans feel a sense that they have lost agency, and that technology may have an adverse effect on society in the future (Jain, 2021).
So what can we do to respond to this dilemma? We must examine how new technologies are shaping and accepted into society. A field of study, called the foundation of control systems, has a feedback control system that attempts to limit the effects of newly deployed technology. The system collects measurements and compares them to a desired outcome, then implements an action to achieve that outcome. This template can be applied to analyze how we relate to and accept technology. We can see how individuals, and society are responding to new technologies. Leaders in the world such as engineers, policymakers, and business influencers have to play a role in this and actively participate to leverage their resources to help us mitigate any potential dangers (Jain, 2021).
Social Media Algorithms
Peter – examples of how social media’s algorithms feed off of society for marketing purposes?
Social Media Platforms & Echo Chambers
Examining how social media platforms like instagram have created echo chambers and comparison traps that are degrading valuable human interaction between younger generations.
How much time do you think the average teenager spends on social media every day? Most people would probably guess a few hours at the most, but still within reason. If that is what you think, then you would probably be surprised to hear that the average teenager actually spends around 9 hours a day looking at some form of social media (Fox). The real question now is what is this actual effect on these individuals who are spending an increasing amount of time online? The rise of social media has disconnected younger generations from each other by creating echo chambers and comparison traps which pushes society farther away from reality, and more towards a false utopia that no one will ever reach.
The first question that needs to be answered is how did social media get so ingrained into our society, and what platforms have become the most prominent? The main reason that people have become more engaged with social media is because “there are more layers of publicness available to those using networked media than ever before; as a result, people’s relationship to public life is shifting in ways we have barely begun to understand” (Matthews, 2016, p. 4). This shift has opened the door for many social media platforms to rapidly expand and root themselves into the culture. There are hundreds of platforms available, but the main one that has affected younger generations is Instagram.
[ 1 ] Source: Max J. Coppes
The first problem with social media, and Instagram in particular, is the prominence of echo chambers. There are two parts to echo chambers. The first is selective exposure which is defined as “the tendency to seek out opinion-reinforcing information,” and the second is selective avoidance which is similarly defined as “avoiding opinion-challenging views” (Pamelee, 2020). When these two parts are implemented as algorithms into social media platforms like Instagram, they will funnel very specific information toward an individual. This will then create a fake reality bubble around them where they perceive the world in a very narrow light, which can hurt their ability to communicate with people outside of their bubble.
The second problem with Instagram is the effects of comparison traps. “People are most likely to share peak experiences and flattering news about themselves… The narrow, distorted slice of reality that is displayed on social media is almost perfectly constructed to make viewers feel deficient and discouraged” (Webber, 2017). When individuals – especially younger generations who are easily impressionable – see someone post the best experiences of their lives, they will inevitably compare it to the entirety of their life, with all its ups and downs, and feel as though they are worthless. This cycle is very harmful for people and will affect how they interact with others, because they will feel as though their lives are not exciting enough. In many cases, people will also turn it into a never ending competition of posting the best parts of their lives to try and outdo others on social media. The fake realities that are created from comparison traps on social media have become harmful to society, and reshaped the culture in unexpected ways.
[ 2 ] Source: Lara Antal
When analyzing the effects of echo chambers and comparison traps on individuals, there is a clear correlation between time spent on social media and a degraded mental health and diminished ability to communicate. Research has shown that an increased amount of time on Instagram and other social media platforms has led to increased feelings of depression and low self esteem (Hunt, 47). While analyzing the effects of technological determinism in this chapter, social media has shown to be changing the environment in which people communicate in ways that are not beneficial to our mental and relational health.
Internet as a Utility – Thomas Johnson
Over the past couple of years, schools have shifted towards online educational methods which have allowed us to adapt in this continuously changing world. But, what about those students who don’t have access to the internet? For those students who are not fortunate enough to have fast and reliable internet access, getting an education has only become much more difficult. In today’s world the internet has become almost a necessity to survive in our technologically driven society and many believe that the internet should now be a school-managed public utility. This would allow internet access to students located all over the United States, but would also pose a lot of problems towards getting internet access to everybody such as infrastructure, service and support, and affordability. Ultimately, internet access has become a crucial part of society today and is something that needs to be managed as a public utility so that education can be accessible to all.
In today’s world internet access has become a necessity and Lisa Gilbert, executive vice president of the advocacy group Public Citizen claims, “It’s critical to the functioning of society.” She continues to say that “treating the internet like electricity or water is the way we need to think about it” (Lazarus). The Internet has become the infrastructure of our lives and now more than ever internet access has become crucial to get an education as well as work from home.
Unfortunately, for those less fortunate students, getting an education has only become harder due to the shift towards online classes. Jonathan Browning of Duke University states, “In the past five years, studies have shown that 35% of lower-income households (annual income of under $35,000 a year) with children in school lack broadband access, compared with just 6% of households making upwards of $75,000 a year” (Browning). This shows that a majority of those families who don’t have access to the internet at home have to travel to local public areas that have internet just for their children to get an education. From this many people believe that it is necessary to treat the internet as a utility and that infrastructure must be built in order to reach everyone. Browning also reported that 70% of teachers assign homework that requires broadband access and 65% of students use the Internet to complete assignments ” (Browning). But, obviously without internet access at home, none of this would be possible.
While it is apparent that internet access and infrastructure needs to be increased across the nation, making the internet accessible to a wide range of the population is not such an easy task. There are a lot of challenges that come along with this such as affordability, infrastructure, and service and support. Affordability is one of the biggest issues as many families have numerous different expenses to the point that they can’t afford to spend the extra money on fast and reliable internet access every month. Another big issue is putting together infrastructure that is strong enough to accommodate for everyone to achieve fast and reliable internet access. Achieving this would require a large amount of funding and work as extending fiber networks towards every community would be a difficult task. The last big problem is service and support as when something goes wrong with the internet, someone has to find a solution to the problem. Every community deserves fast and reliable internet, but what happens when internet access gets interrupted or stops functioning? It shows how much work needs to be done in order to get internet access to everyone in our society,
Overall we can see how dependent our society has become on the internet and how many students need access to the internet in order to successfully obtain an education. The internet has played a big role in our everyday lives and ultimately our society needs to make the internet a school-managed public utility. In today’s world we have seen how many classes have shifted towards online educational methods and for those students who don’t have fast and reliable internet access getting an education can be a difficult task. Altogether as the internet has taken control of our everyday lives it is apparent that internet access needs to be developed into a school-managed public utility so that a good education can be accessible to all.
ARTIFICIAL INTELLIGENCE
Artificial Intelligence is not simply a robot invasion. In reality, it applies to a very broad aspect of our lives, everyday taking a more ever present role in societal functioning.
In the near future most, if not all cars will be guided by artificial technology that allows the passenger to sit in the back seat, allowing them to be more efficient and productive than if they actually had to drive. (Coeckelbergh) These cars also allow the roadways to be more safe, as the complex algorithms and exceedingly quick machine reaction times drastically minimize the chances of collisions. Even today, multiple companies such as TESLA have vehicles that are fully autonomous on the freeway and highway, as well as low-traffic country roads.
Algorithms are the pure essence of artificial intelligence as they inform the main computer how to make decisions. As time passes, these algorithms grow more complex and allow for the computers to make more well informed decisions from larger amounts of data. A prime example of this is using the COMPAS algorithm within the criminal justice system. The COMPAS system allows for the court to determine the likelihood of the guilty becoming repeat offenders, which then gives them more information with regards to bail and sentencing. It has been proven to be 63% accurate which is astronomically high given the complexity and variability of the human subconscious and their corresponding actions(Coeckelbergh). Once again, this algorithm gives society the means to be safer and more efficient.
The beneficial effect of artificial intelligence even goes beyond safety; in fact, it has the necessary capability to greatly restore the quality of life in the medical sector. Those with the disease ALS gradually lose all muscular control, including the ability to speak, while maintaining the same quality and functioning of their mind. However, due to AI developments, programs have been created to track the users eye movements which then synthesize the words allowing the user to regain the ability to speak again (Hamet). Before AI these people lost their ability to speak for good, but now, they still have the ability to communicate with their peers and family which greatly improves their quality of life.
Missing Voices
Boyd and Ellison define social media as an internet platform that allows people to interact and develop social relationships through private or public online profiles (Boyd & Ellison, 2007). Despite the usefulness of Social Media, researchers claim it to be widely manipulative. The 2020 Netflix hit “The Social Dilemma” introduces the public to this manipulation and exposes the dark business models. Platforms take advantage of users’ behavioral vulnerabilities and social rewards for revenue, even with the cost of social media addiction and the escalation of mental health issues (Roy, 2020). Notably, the companies use unethical algorithms to profit off the missing voices of college students.
[ 3 ] Source: Oladimeji Ajegbile
Social media companies commonly profit off users through the Attention Economic business model. Within a Cambridge article, Bhargava & Velasquez summarize that the model is an ad-based practice. The user’s attention is the product, and businesses sell this attention to the advertisers or other buyers. The longer a user spends on their platform, the more that companies profit. Platforms retain attention through rewarding mechanisms like infinite scrolling, “likes,” and intermittent variable rewards.
Infinite scrolling entices users to continue scrolling because it inhibits stopping cues. Platforms also integrate social validation through likes, encouraging individuals to spend more time online. Additionally, companies like Twitter and Pinterest use intermittent variable signals, which keep users’ attention by reducing content predictability. For example, Twitter uses the blue loading screen
[ 4 ] Source: Ron Lach
to hide tweets, and Pinterest only shows portions of images at the bottom of the screen. These platform elements increase the amount of time that users spend online, increasing the amount of collectible data. This data is thereby used through algorithms to keep users on social media. Furthermore, this fact is concerning, given that most news is on social media (Bhargava & Velasquez, 2020).
In a 2020 study about the impacts of social media on the social comparison of women in college, Engeln and others determined that women spend significantly more time looking at people on Instagram than on Facebook. Women online are often portrayed with unrealistic body types, contributing to comparison. Given the study results, companies should rethink business practices (Engeln et al., 2020).
Algorithmic content retains attention through trending posts, friends’ posts or comments, and clickbait, using personalized ads for profit. In an article about recommender systems, Bojić and others define this content in more detail. Specifically, algorithms use artificial intelligence to provide systems with harvested user data (Bojić et al., 2021).
It is problematic that businesses utilize this model, because social media platforms are addictive. In the Cambridge article, they define internet addiction as “a behavioral addiction.” Specifically, it encompasses several terms, including social media addiction (Bhargava & Velasquez, 2020). Further, the impact of addiction on individuals is concerning, especially considering the prevalence of internet addiction among college students. Setty and others reported the majority of internet addiction among college students in Jodhpur, India. The researchers discovered that “51%” of the 2,035 students suffered from addictive symptoms (Setty & Rajasekhar et al., 2021).
Despite internet addiction’s broad definition, there is controversy over how to describe it. In the Cambridge article, they counter the discussion with supportive evidence for internet addiction. They cite the supportive material of internet overuse and connections between people with internet and substance addiction.
Additionally, the article explains how companies exploit the users’ vulnerabilities, to keep them addicted to the platforms. Two of the discussed vulnerabilities include an individual’s need or desire for the rewards and the importance of the internet to daily life. (Bhargava & Velasquez, 2020). Boer and others attempt to explain the relationship between social-media-use intensity and adolescent wellbeing in another article. They conclude that “the higher adolescents’ average intensity of certain” social media usage “activities, the lower their average level of life satisfaction” (Boer & Stevens et al., 2022). This information is concerning, given that Undergraduate Freshmen and Sophomores are at the end of the adolescent age range from 18-19 years old.
To measure the consequences and risk factors of social media misuse, researchers Zhang and Rau analyze 12 questionnaires in a 2020 article. They conclude that social-media-misuse (SMM) of Facebook leads to a deterioration in self-control ability. Additionally, they analyze a positive relationship between the combination of perceived envy through social comparison tendencies and depression, which poor self-esteem may exacerbate (Zhang & Rau, 2020). These behavioral tendencies may also harm interpersonal relationships. Further, those with emotional instability have shown an elevated risk of developing SMM (McCrae & Gealish, 2019).
There are several proposed solutions to the business models with platforms that contribute to poor mental health and social media misuse. Sarah Brown proposes two solutions in an MIT Management Sloan School article. The first is a subscription-based model, which would, “allow businesses to own the relationship with customers.” The company Neeva uses this model by directing individuals to the most helpful content instead of prioritizing profit from searches. The alternative to the subscription model is Identity Verification, which requires accounts to access feeds. LinkedIn uses this system, limiting the number of uncredible users (Brown, 2021). Overall, these solutions may produce profitable platforms that are healthy for users.
Here you will provide an infographic that sums up your theory or concept that includes a brief definition, its relationship to STS, and brief examples.
Chapter Questions
- After reading this chapter, what do you think the most important effect technology has on our lives?
- What is one direct way that technology has made our lives safer or more functional?
- What technological advances do you foresee within the near future?
- In what ways do computer algorithms advance society?
[1] https://med.unr.edu/news/archive/2019/coppes-teens-and-social-media
[2] https://www.verywellfamily.com/ways-social-media-affects-teen-mental-health-4144769
[3] https://www.pexels.com/photo/man-in-white-crew-neck-top-reaching-for-the-like-3314294/
[4] https://www.pexels.com/photo/boy-beside-two-screens-9783827/
“ japanese vending machine ” by Melosh is marked with CC BY-NC-ND 2.0 .
Bhargava, V., & Velasquez, M. (2021). Ethics of the Attention Economy: The Problem of Social Media Addiction. Business Ethics Quarterly, 31(3), 321-359. https://www.cambridge.org/core/journals/business-ethics-quarterly/article/ethics-of-the-attention-economy-the-problem-of-social-media-addiction/1CC67609A12E9A912BB8A291FDFFE799
Bhattacharjee, Y. (2021, May 3). Smartphones revolutionize our lives-but at what cost? Science. Retrieved April 26, 2022, from https://www.nationalgeographic.com/science/article/smartphones-revolutionize-our-lives-but-at-what-cost#:~:text=It’s%20incontrovertible%20that%20smartphone%20technology,help%20is%20needed%20most%20urgently.
Boer, Maartje & Stevens, Gonneke & Finkenauer, Catrin & Eijnden, Regina. (2021). The complex association between social media use intensity and adolescent wellbeing: A longitudinal investigation of five factors that may affect the association. Computers in Human Behavior. https://www.researchgate.net/publication/355704059_The_complex_association_between_social_media_use_intensity_and_adolescent_wellbeing_A_longitudinal_investigation_of_five_factors_that_may_affect_the_association
Bojic, L., Zaric, M., Zikic, S., (2021). Worrying Impact of Artificial Intelligence and Big Data Through the Prism of Recommender Systems. https://www.eap-iea.org/index.php/eap/article/view/207/200
Brown, S. (2021, June 16). The case for new social media business models. MIT Sloan School of Management. https://mitsloan.mit.edu/ideas-made-to-matter/case-new-social-media-business-models
Browning, J. (2021, March 22). Broadband as a utility is a matter of ethics, not economy. Ethical Tech. Retrieved April 5, 2022, from https://ethicaltech.duke.edu/2021/03/22/broadband-as-a-utility-is-a-matter-of-ethics-not-economy/
Chandler, D. (2000). Technological or media determinism. Technological Determinism: Theoretical Stances. Retrieved March 31, 2022, from http://visual-memory.co.uk/daniel//Documents/tecdet/tdet11.htm
Coeckelbergh, M. (2020). Ai Ethics . Google Books. Retrieved December 6, 2022, from https://books.google.com/bookshl=en&lr=&id=Gs_XDwAAQBAJ&oi=fnd&pg=PR9&dq=ai%2Bals%2Btechnology&ots=EZ0c8BTyJT&sig=CR5hdz8_LjxxhCNROsHdc1GdQk#v=onepage&q=ai%20als%20technology&f=false
Dafoe. (2015). On Technological Determinism: A Typology, Scope Conditions, and a Mechanism. Science, Technology, & Human Values, 40(6), 1047–1076. https://doi.org/10.1177/0162243915579283
Danah M. Boyd, Nicole B. Ellison, Social Network Sites: Definition, History, and Scholarship, Journal of Computer-Mediated Communication, Volume 13, Issue 1, 1 October 2007, Pages 210–230, https://doi.org/10.1111/j.1083-6101.2007.00393.x
Drew, C. (2022, October 23). Technological determinism theory (5 examples, Pros & Cons) . Helpful Professor. Retrieved November 3, 2022, from https://helpfulprofessor.com/technological-determinism-theory/
Drinkwater, S. (2018, December 10). "we shape our technology; then technology shapes us.". Medium. Retrieved April 26, 2022, from https://medium.com/doteveryone/https-medium-com-doteveryoneuk-we-shape-our-technology-then-technology-shapes-us-36d16251176e
Dullaert, S. (2020, July 31). Covid-19 crisis shows the internet should be a public utility. Socialist Alternative. Retrieved April 5, 2022, from https://www.socialistalternative.org/2020/07/31/covid-19-crisis-shows-internet-should-be-public-utility/
Engeln, R. et al. (2020, June 4). Compared to Facebook, Instagram use causes more appearance comparison and lower body satisfaction in college women. ELSEVIER. https://www.sciencedirect.com/science/article/abs/pii/S1740144519303754?via%3Dihub
Finnegan, R., Salaman, G., & Thompson, K. (1987). Information Technology: Social issues: reader. Hodder and Stoughton/The Open University.
Fox, M., & Edwards, E. (n.d.). Teens Spend 'Astounding' Nine Hours a Day in Front of Screens: Researchers. WVEA. Retrieved April 14, 2022, from https://www.wvea.org/content/teens-spend-astounding-nine-hours-day-front-screens-researchers
Fuller, J. (2017, December 8). Does Society Influence Tech, or vice versa? Engineering360. Retrieved April 10, 2022, from https://insights.globalspec.com/article/7343/does-society-influence-tech-or-vice-versa
Greene, P. (2020, November 10). Curmudgucation: Is it time for the internet to be a school-managed public utility? National Education Policy Center. Retrieved April 5, 2022, from https://nepc.colorado.edu/blog/it-time-internet
Hamet, P. (2017, January 11). Artificial Intelligence in medicine . Artificial Intelligence in Medecine. Retrieved December 6, 2022, from https://www.sciencedirect.com/science/article/pii/S002604951730015X
Harrison, N. (2013, October 25). Technological determinism: A Critique based on several readings in adult education. Learning Tech. Retrieved March 31, 2022, from https://sites.psu.edu/natalieharp/writings/technological-determinism-a-critique-based-on-several-readings-in-adult-education/
Hunt, M., All, K., Burns, B., & Li, K. (2021). Too much of a good thing: Who we follow, what we do, and how much time we spend on social media affects well-being. Journal of Social and Clinical Psychology, 40(1), 46–68. https://doi.org/10.1521/jscp.2021.40.1.46
Jain, N. (2021, April 30). Who is in control: Humans or tech? Forbes. Retrieved April 26, 2022, from https://www.forbes.com/sites/neerajain/2021/04/30/who-is-in-control-humans-or-tech/?sh=511d6547689c
Lazarus, D. (2020, October 23). Column: The pandemic makes clear it's time to treat the internet as a utility. Los Angeles Times. Retrieved April 5, 2022, from https://www.latimes.com/business/story/2020-10-23/coronavirus-internet-is-a-utility
Matthews, P. (2016). Social Media, Community Development and Social Capital. Community Development Journal, 51(3), 419–435. https://doi.org/https://academic.oup.com/cdj/issue
Betul Keles, Niall McCrae & Annmarie Grealish (2020) A systematic review: the influence of social media on depression, anxiety and psychological distress in adolescents, International Journal of Adolescence and Youth, 25:1, 79-93, DOI: 10.1080/02673843.2019.1590851
Parmelee, J. H., & Roman, N. (2020). Insta-echoes: Selective exposure and selective avoidance on Instagram. Telematics and Informatics, 52, 101432. https://doi.org/10.1016/j.tele.2020.101432
Priyanka Roy. (September 14, 2020 Monday). Social media is a drug that controls and manipulates us: The Social Dilemma. The Telegraph (India). https://advance-lexis-com.libproxy.clemson.edu/api/document?collection=news&id=urn:contentItem:60VC-18G1-DXMP-K4JH-00000-00&context=1516831 .
Setty, N. K. H., Rajasekhar, T., Raghav, P., Aneja, J., Gupta, M. K., Sharma, P. P., Goel, A. D., Srinivasan, S., & T, P. (2021). 722 Prevalence and predictors of internet addiction among college students in Jodhpur city, India. International Journal of Epidemiology, 50, 1–2. https://doi-org.libproxy.clemson.edu/10.1093/ije/dyab168.346
Technological determinism. (2018, April 23) Communication Theory. Retrieved April 10, 2022, from https://www.communicationtheory.org/technological-determinism/
Webber, R. (2017, November 7). The comparison trap. Psychology Today. Retrieved April 16, 2022, from https://www.psychologytoday.com/us/articles/201711/the-comparison-trap
White, L. J. (1971). Medieval technology and Social Change. Oxford University Press.
Zhang, A., & Rau, P-L., (2020). A Review and Reappraisal of Social Media Misuse:
Measurements, Consequences, and Predictors. International Journal of Human-Computer Interaction, 37(1), 1-14. https://www-tandfonline-com.libproxy.clemson.edu/doi/full/10.1080/10447318.2020.1807281
To the extent possible under law, Belle Salsone; Peter Sebastian Stein; Kaleb Gage Parsons; Thomas Kent; Krystal Nielsen; and David Thomas Nitz have waived all copyright and related or neighboring rights to Science Technology and Society a Student Led Exploration , except where otherwise noted.
Share This Book

An official website of the United States government
The .gov means it’s official. Federal government websites often end in .gov or .mil. Before sharing sensitive information, make sure you’re on a federal government site.
The site is secure. The https:// ensures that you are connecting to the official website and that any information you provide is encrypted and transmitted securely.
- Publications
- Account settings
Preview improvements coming to the PMC website in October 2024. Learn More or Try it out now .
- Advanced Search
- Journal List
- Innov Aging

Social Relations and Technology: Continuity, Context, and Change
Toni c antonucci.
1 Department of Psychology and Institute for Social Research, University of Michigan
Kristine J Ajrouch
3 Department of Sociology, Anthropology and Criminology, Eastern Michigan University
Jasmine A Manalel
2 Department of Psychology, University of Michigan
Social relations, although basic to human nature, health and well-being, have become increasingly complicated as a result of changing population demography and technology. In this essay, we provide a historical overview of social relations, especially as they affect older people. We briefly review the evolution of theory and measurement surrounding social relations as well as early empirical evidence. We consider how social relations have changed over time as well as continuity and change regarding basic characteristics of social relations. Of special interest is the emerging influence of technology on how people maintain contact, especially the changing ways people can use technology to increase, decrease, maintain, or avoid social relations. We consider both negative and positive aspects of these new technologies and their influence on health and well-being. Finally, we conclude that new and emerging technologies hold great promise for the future by overcoming traditional barriers to maintaining social contact, support exchange, and information acquisition. Nevertheless, we caution that these new technologies can have the dehumanizing effect of distance thus creating the potential for insensitivity and increased negativity. In sum, we are cautiously optimistic about the promise of technology to expand, but not replace, traditional forms of social contact.
Translational Significance
Incorporating technology into our study of social relations will be informative for our understanding of how communication modalities influence or are an expression of closeness and/or conflict. Further, technology has important potential for keeping social networks linked and for delivering potential interventions from telehealth to caregiving.
Social relations are a fundamental aspect of human life. This has been advocated early in the history of social science by luminaries such as Cooley (1902) , Durkheim (1915) , and Mead (1913) , and continues to be of significance today as scholars document this point both theoretically and empirically, see Antonucci, Ajrouch, & Birditt (2014) , for a review. Context also matters, in that the ways in which social relations evolve and influence well-being have been shown to vary across time and place ( Ajrouch, Fuller, Akiyama & Antonucci, 2017 ; Fiori, Smith & Antonucci, 2007 ). At the same time, technological developments are fundamentally changing the ways in which we experience social relations, and may impact health and well-being accordingly. In this invited essay, we identify the convoy model as our guiding theoretical framework for understanding continuity and change in social relations. We consider how social relations have changed over time, specifically how technological advances engender new modes of contact for older adults. This is followed by a consideration of challenges facing the study of social relations, with particular attention to the need for theoretical and empirical assessments that take into account newly developing characteristics of our society. These include changes in the demography of the family and changes in migration patterns. We then elaborate on how new of experiencing social relations may have both positive and negative effects, thereby differentially influencing health and well-being. An important challenge to the field of social relations is to consider how to incorporate these developments into current and timely research.
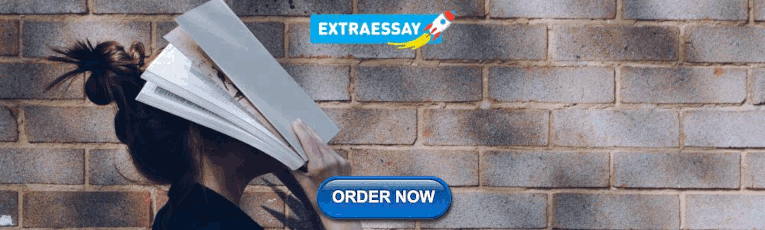
The Convoy Model of Social Relations
The convoy model was developed to specify the scientific study of social relations by detailing the antecedent factors influencing social relations (personal and situational), identifying multiple dimensions of social relations, and illustrating how these factors influence health and well-being ( Antonucci, 2001 ; Kahn & Antonucci, 1980 ). Individual characteristics such as age, gender, race, and religion illustrate the personal characteristics thought to influence social relations, whereas situational characteristics such as roles, norms, organizations, and communities were identified as important external factors influencing social relations. Multiple dimensions of social relations were specified to include convoy structure, support, and satisfaction or relationship quality. The tenets of the convoy model were built on key findings in the literature showing that social relations are an important part of the health and well-being of older people in the United States and around the globe.
Structure refers to characteristics of the people in one’s network such as size, composition, contact frequency, and geographic proximity. Size and composition are important in so far as larger, more diverse networks are associated with positive outcomes. Much evidence has accumulated to indicate that older people enjoy spending leisure time with friends and that these relationships are associated with positive well-being ( Adams & Blieszner, 1989 ; Antonucci & Akiyama, 1995 ). On the other hand, older people also frequently report that they would turn to family, specifically spouse and children when in need. Cantor’s (1979) hierarchy of caregiving clearly designated the order of caregiving preference to be spouse/partner, child, other family, friend\neighbor, and formal caregiver.
A great deal of attention over the last century was given to the “decline of the family” as well as the decreased status of elders in our society. It was argued that older people were increasingly disrespected, alone, and isolated. Yet, classic studies challenged these notions with extensive, empirical investigations. It is now fairly well established, and convoy data continue to empirically support, that older people generally live quite close to and are in regular contact with their children ( Ajrouch et al., 2017 ; Fiori, Antonucci & Akiyama, 2008 ; Shanas et al, 1968 ). Not only do older people receive help, support, and contributions from their children, they also provide these same types of assistance to their children. In fact, older people often provide more than they receive ( Akiyama, Antonucci, & Campbell, 1997 ; Webster et al., 2012 ; Wiemers, Seltzer, Schoen, Hotz & Bianchi, 2016 ). In sum, social support structure includes various elements, all of which are included in the convoy model.
Support refers to the provision and receipt of support, such as aid, affect and affirmation. Lack of social support can have a significant negative impact on health and well-being. One of the most intriguing classic findings is that the single factor most likely to prevent nursing home placement is the report by the older person that they had a confidante, someone with whom they could share their intimate feelings ( Lowenthal & Haven, 1968 ; this finding has been replicated around the world, e.g. in Australia by Giles, Glonek, Luszcz, & Andrews, 2007 ). Social support, and, in particular, protection from isolation and loneliness, are clearly important for the health and well-being of older people. The convoy model recognizes various support types, including instrumental and emotional support, as key predictors of health and well-being.
Satisfaction refers to one’s assessment of one’s social relations, sometimes referred to as adequacy or quality of relationships. It was thought that the existence of a relationship presupposed positive relationship quality and support. These assumptions were increasingly questioned as people began to note that while some families did evidence close, positive relationships, others might better be characterized as negative or ambivalent (both positive and negative) at best. Troll (1971) used the term residential propinquity to note that while many older people wanted to remain close to their family, they actually preferred not to live with them. She suggested that people recognized that it was easier to maintain positive relationships when some distance, privacy, and independence could be maintained. Family relations often include intergenerational relations. Bengtson and his colleagues expanded the family social relations literature by examining intrafamily intergenerational relations and introducing solidarity theory. According to this theory, positive features of adult child–parent ties include contact, emotional bonds, and support exchanges ( Fingerman, Sechrist, & Birditt, 2013 ; Silverstein & Bengtson, 1997 ). In addition, once Bengtson and colleagues expanded this work to investigate the possibility of negativity in intergenerational relations ( Silverstein, Parrott, Angelinni, & Cook, 2000 ), they found that in most families some level of conflict also existed, with younger people reporting more conflict than older people. Bengtson attributed this to differences in intergenerational stake, which referred to the fact that older people were more invested in family links to ensure their legacy, whereas younger people sought to establish independence and create their own legacy ( Bengtson & Kuypers, 1971 ). Empirical evidence has accumulated supporting both these theoretical perspectives ( Suitor, Sechrist, Gilligan, & Pillemer, 2011 ). The convoy model ensures attention to the complexity of relationships quality.
Over the years, evidence has accumulated in support of the convoy model ( Antonucci, 2001 ; Ajrouch et al., 2017 ). Fortunately, the model is designed to incorporate the study of newly emerging developments that might influence social relations. Technological advances, especially with regard to communication technology and social media, offer new ways for enabling older adults to establish social connectedness with family and friends ( Czaja et al., 2017 ; Delello & McWhorter, 2017 ; Leist, 2013 ). Technology can also provide pathways for support in managing health conditions among older adults and those who provide care ( Czaja, 2017 ). Though, as the convoy model posits, use and benefits of technology likely vary according to personal and situational characteristics, and will influence health in unique ways.
Incorporating Technological Developments Into the Study of Social Relations
The nature of social interaction has changed as technological advances have provided new methods of contact. Consider the evolution from in-person contact and letter writing to the telegraph and telephone and, most recently, to ever more individualized and electronic forms of contact such as cell phones, video calls (e.g., Skype, FaceTime), and social media (e.g., Facebook). We know very little about how different forms of communication influence social relations, health, and well-being.
To address the observation that social relations are now experienced in new ways because of technological developments, we recently analyzed a measure of contact frequency that distinguished in-person contact from telephone and electronic contact using the longitudinal Survey of Social Relations ( Antonucci, Birditt & Webster, 2010 ). See Table 1 for a description of participant characteristics. We then examined the degree to which positive and negative relationship quality measured at Time 1, predicted adults’ frequency and use of different forms of communication 10 years later with members of their convoy, namely parents, spouse, child, and friend. We briefly report on our findings in the following paragraphs. For those who might doubt their use, we should note that older adults are increasingly using social media. While over 90% of young people are online and have cell phones, over half of adults age 65 and over are online and 78% own a cell phone ( Anderson, 2015 ; Zickuhr & Madden, 2012 ).
Social Relations Study Wave 3 (Time 2) Sample Descriptives ( N = 557)
( ) | (%) | |
---|---|---|
Age (years) | 59.8 (16.0) | |
Education (years) | 14.2 (2.1) | |
Female | 355 (63.7) | |
Married/living with partner | 346 (62.1) | |
Have children | 468 (84.0) |
Note . Includes nonindependent sample of respondents who completed interviews at both Time 1 and Time 2. M = mean; SD = standard deviation.
Considering different contact modes, as expected, in-person contact was most frequent with spouse (see Table 2 ). Electronic communication was lowest with parents. Interestingly, telephone use was consistent across all relationships.
Descriptive Statistics for Contact Frequency With Network Members via Different Modes a
In person | Telephone | Electronic | ||||
---|---|---|---|---|---|---|
( ) | ( ) | ( ) | ||||
Mother | 3.30 (1.16) | 94 | 3.71 (1.16) | 94 | 2.27 (1.52) | 84 |
Father | 3.31 (1.16) | 52 | 3.31 (1.16) | 52 | 1.98 (1.41) | 50 |
Child | 3.53 (1.12) | 204 | 3.96 (1.00) | 205 | 3.47 (1.42) | 155 |
Spouse | 4.88 (0.50) | 180 | 4.09 (1.35) | 179 | 3.39 (1.75) | 147 |
Friend | 3.02 (1.17) | 187 | 3.62 (1.08) | 187 | 3.11 (1.56) | 150 |
Note. M = mean; SD = standard deviation.
In many cases, links between relationship quality and contact differed between younger and older adults, depending on the mode of contact. Among older respondents, in-person contact frequency with fathers with whom respondents had a highly negative relationship was much lower than among those with a lower negative relationship quality (see Figure 1 ; all graphs plotted at 1 SD above and below the mean for relationship quality and age). On the other hand, there was little difference among younger respondents’ contact frequency across levels of negative relationship quality with father.

Relationship quality by age interaction effect on in-person contact with father.
There was also an age × relationship quality effect on telephone contact with spouse indicating little difference among younger people, but older people with low spousal positive relationship quality reporting significantly less telephone contact with their spouse than those with a high spousal positive relationship quality ( Figure 2 ). The findings with respect to negative relationship quality were somewhat but not completely parallel. Level of negativity in the relationship did not influence frequency of telephone contact with friends among older people but, interestingly, more negativity in the relationship was associated with more telephone contact with friends among younger people ( Figure 3 ).

Relationship quality by age interaction effect on telephone contact with spouse.

Relationship quality by age interaction effect on telephone contact with friend.
Finally, we examined the use of electronic forms of communication such as video chat, Skype, text, Facebook, and email. There were no effects of positive relationship quality across any of these forms of communication, although older people were less likely to use them than younger people. This age effect was also evident for negative relationship quality. Older people with high negativity in their relationship with their child were significantly less likely to communicate with them electronically than those with low negativity in their child relationship ( Figure 4 ). On the other hand, once again there were no differences in electronic communications among young people regardless of the negativity of their relationship with child.

Relationship quality by age interaction effect on electronic contact with child.
In sum, these findings show how new ways of experiencing social relations vary by age and relationship type. These findings do suggest some age differences but perhaps most importantly highlight the role of relationship quality to mode of communication. New ways of engaging in social relations are not evenly experienced across generations, and hence point to new areas for investigating how social relations influence well-being. Next, we present the ways in which new contact forms via technological developments inform the scientific study of social relations in the context of demographic shifts and health.
Population Demographic Shifts
Many technological advances have occurred within the context of broader demographic changes, including shifts in mortality, fertility, mobility, and marital patterns ( Bianchi, 2014 ). Longer life spans provide older adults with more opportunities to build relationships with younger generations. Increased mobility and migration have led to less geographically proximate family networks, posing potential barriers to support exchanges and contact. Changes in marital patterns have resulted in increasing heterogeneity of family structures. Thus, older adults today are embedded within diverse and complex family structures that shape the type and quality of their social ties. It is important to consider these new aging family forms and functions when evaluating the role of technology in the establishment and maintenance of these social ties, and how the social needs of older adults are being met through technological advances in communication. We next discuss how these demographic shifts influence patterns of intergenerational and romantic relationships, the adaptation of immigrants, and the implications that technology has for these patterns.
Intergenerational Relations
Families are changing such that intergenerational ties, especially those across more than two generations, are becoming increasingly salient ( Antonucci, Jackson, & Biggs, 2007 ; Bengtson, 2001 ; Swartz, 2009 ). Although older adults are less likely to adopt new technologies, they may be motivated to do so by intergenerational ties, e.g., to learn to use a smartphone or social media in order to maintain contact with children and grandchildren. A recent cross-national study demonstrated that countries with a higher prevalence of mobile phone subscriptions also had higher levels of maternal contact by adult children, particularly daughters ( Gubernskaya & Treas, 2016 ).
Technology has the unique potential to influence grandparent–grandchild relationships due to younger generations’ faster adoption of new technologies. Although in-person communication continues to be the most frequent type of contact for grandparents, mobile phones, texting, and email are becoming increasingly popular as a means of staying in touch with grandchildren ( Hurme, Westerback, & Quadrello, 2010 ; Quadrello et al., 2005 ). Given the increased mobility of families and the inverse relationship between geographic proximity and in-person contact, newer communication technologies provide a means by which grandparents can overcome barriers of distance to maintain meaningful ties with younger generations.
Increased levels of intergenerational contact via multiple media platforms, including texting and social networking sites, can have both positive and negative implications for the quality of relationships. Increased contact between older and younger generations could foster feelings of solidarity and closeness, leading to more positive evaluations of the relationship. On the other hand, higher levels of telephone and electronic contact could also promote more negative interactions and exchanges, especially when compared to in-person contact which may mute negativity because of the ability to perceive real-time reactions. Similarly, more technologically proficient individuals may feel frustrated with friends or relatives who struggle to communicate with newer technologies, eroding the quality of their relationship. More research is needed to identify the positive and negative implications of contact via newer technologies for intergenerational relationships, especially given the generational disparities in technology use ( Fingerman & Birditt, 2011 ).
Immigrant Aging
New and varied ways to communicate across geographic distances have created a world of possibilities for immigrants. The advent of communication technologies such as Skype, WhatsApp, Viber, and FaceTime (among others), has made the ability to connect with close others who are geographically distant almost effortless. Moreover, smart phones are revolutionizing communication patterns, no longer restricting the ability to connect by having to be at a particular place. New technologies now facilitate connections between individuals wherever they are instead of individuals in specific locations. For older immigrants, these ways of having social relations may be a double-edged sword, as they facilitate relationships with those left behind, but may also make interactions in the host country more segregated. On the other hand, the Internet may simply serve as a buffer, much as ethnic enclaves do, facilitating adaptation and integration to the host society. We review recent findings in the following paragraphs.
Technology can be an outlet for immigrants who are socially isolated. For instance, among older immigrants from the former Soviet Union to Israel, social media became a resource that both reunited families and old friends living in various parts of the world, as well as helped to create new relationships ( Khvorostianov, Elias, & Nimrod, 2012 ). This way of practicing social relations overcame major problems encountered by elderly immigrants—that of loneliness and social isolation. Khvorostianov and colleagues illustrate that such connections served as a source of joy and empowerment, facilitating transnational connections created through the Internet, ultimately supporting social integration. Similar trends have been identified among older Chinese immigrants living in New Zealand ( Zhang, 2016 ).
For immigrants who leave their homeland at a young age, using information and communication technology (ICT) can, in fact, strengthen adaptation in the host country as one grows older. Hunter (2015) found that migrant workers from Africa living in France opted to remain in the host society after retirement given the ease with which they could connect with family back home as well as remain connected to attachments in France that were reinforced through smart phone technology. Evidence is accumulating to suggest that older immigrants’ social relations facilitated through ICT leads to stronger identities, and empowerment, overall enhancing quality of life. Yet, research of this sort is sparse, and generally occurs with small, nonrepresentative samples. The potential advantages and challenges that arise for immigrants through these new types of social relations is an area in need of further study.
Marital Patterns
A population trend that has widespread implications for how older adults use technology is the heterogeneity of marital statuses, including “gray divorce” (i.e., divorce after the age of 50; Brown & Lin, 2013 ) and never married older adults ( Cooney & Dunne, 2001 ). The misconception that older singles are not interested in finding and maintaining romantic relationships is countered by increasing numbers of later life daters ( Brown & Shinohara, 2013 ). The role that the Internet and social media play in establishing new romantic relationships presents a promising opportunity for research on how older adults use technology.
Although growing numbers of older adults turn to the Internet, including social media and dating websites, to find romantic partners, a surprising lack of attention has been paid regarding older adults’ use of technology to establish romantic connections. Online dating has become a popular means of finding romantic partners for people of all ages, including older adults. Some studies suggest that middle-aged and older adults may, in fact, be more likely than younger adults to use the Internet to meet potential partners ( Stephure, Boon, Mackinnon, & Deveau, 2009 ; Valkenburg & Peter, 2007 ). One advantage of online dating is that individuals’ partner preferences can be tailored and expectations can be explicitly stated. Older adults have been found to capitalize on this feature through the content of their online personal ads ( Alterovitz & Mendelsohn, 2009 ; Davis & Fingerman, 2016 ; Wada, Mortenson, & Hurd Clarke, 2016 ).
In response to older adults’ adoption of the Internet in finding romantic partners, dating websites have made a more concerted effort to target this population. Popular dating websites boast large bases of older subscribers, whereas others are solely dedicated to serving adults aged 50 and older (e.g. OurTime.com ). This is one example of how older adults are not simply consumers of new technology, but also influence the creation of new technology aimed to meet their social needs. Future research should consider the evolving bi-directionality of technology use by older individuals and their resulting influence on the development of new technology. Next, we consider how technology may impact social relations in the context of health.
Social Relations and Health
New technologies have been found to directly influence health due to the possibilities they generate to better connect with others. There is concern that the latest forms of contact and communication threaten community in the U.S. ( Althaus & Tewksbury, 2000 ); yet, it appears that using the Internet is associated with higher levels of perceived support among older adults ( Cody et al., 1999 ) and lower levels of isolation and loneliness ( Cotten, Anderson & McCullough, 2013 ). Further, older adults are often motivated to use new technologies so that they may connect with others ( Sims, Reed, & Carr, 2016 ). In sum, opportunities to enhance social relations through new technologies may initiate new ways to think about how social relations influence health and well-being.
New ways to create and sustain social relations may represent viable alternative sources for developing a sense of community in situations where mobility is limited or restricted. Research indicates that technological developments greatly expand communication options for older adults with mobility limitations, resulting in positive effects for well-being ( Jaeger & Xie, 2009 ; Sims et al., 2016 ). Yet, the effects of communication technology are not necessarily always direct. For instance, Elliot and colleagues (2014) found that ill-health was a considerably weaker predictor of depressive symptoms for high ICT users than for non/limited users, but there was no direct effect of ICT on depressive symptoms. Furthermore, limitations in activities of daily living were a stronger predictor of depressive symptoms for high ICT users. Hence, the benefits of ICT for health are still not clear. Yet, the benefit of ICT-mediated social relations for health and well-being suggests multiple avenues to pursue for social support interventions that may address the challenges that older adults face with the onset of chronic illness. Technological innovations have also spawned various new forms of telehealth communications and treatment as well as social support interventions for their caregivers. We present examples of these potential opportunities next.
Social Support, Intervention, and Technology
Innovative uses of technology have been applied to create social support interventions that maximize good health and well-being. Use of the Internet has opened new avenues for enhancing social support for older adults, especially to address the threat of social isolation and loneliness. One such intervention is the Personal Reminder Information and Social Management (PRISM) system ( Czaja et al., 2017 ). According to Czaja and colleagues, PRISM is a software application designed to support connectivity and resource access among older adults. In a randomized control trial, they showed that access to technology applications, especially email, Internet and games, facilitated social engagement, and provided an effective means of promoting social interactions and connections. Likewise, Delello and McWhorter (2017) showed in a mixed-method study among older adults living in a retirement community that iPads can be used to facilitate closer family relationships and greater overall connection to wider society. Moreover, both studies challenge myths that older adults avoid new technology. Instead, older adults can and will learn new skills to use technology successfully, even if they have never been exposed to it before.
Beyond the issue of social isolation, new communication technology creates unique ways for those with chronic disease to receive support that helps older adults meet the demands of managing illness. One promising mode involves interactive voice response technology (IVR). IVR provides an opportunity to use technology to schedule automated telephone assessment and self-care support calls ( Heisler & Piette, 2005 ). In the case of diabetes, Heilser and Piette used IVR to facilitate connections between peers with the same disease. Findings showed that the technology facilitates an opportunity for reciprocity, where each peer receives and as well as provides support. Moreover, the support experience appears to generate increased self-efficacy, ultimately contributing to better management of diabetes. The IVR technology has also been extended to create the notion of CarePartners as a means to address the health and well-being of informal caregivers ( Piette et al., 2015 ). Piette and colleagues conducted a randomized trial of mobile health support for heart failure patients and their informal caregivers. A CarePartner was identified by measuring the elements of closeness, support type, and quality of key individuals identified by the patient. That person then became the caregiver. The identified caregiver received weekly emails about their loved one’s status and suggestions for how to support self-management. In sum, the provision of informal support was facilitated by IVR and Internet technology. Technological innovations suggest several potential opportunities to leverage the benefits of social support for health and well-being.
Summary and Conclusion
New forms of communication have created unique challenges for understanding relationships. Electronic communication, such as Facebook, instant messaging, Snapchat, Skype, FaceTime, and have all created new opportunities to maintain contacts with close others. Cell phones and email have fundamentally changed how and how often people communicate. The reduced cost of these forms of contact has resulted in almost universal adaptation of some, if not all, of these tools of communication to maintain contact with friends and family. However, we know very little about the effect of these new forms of communication. On the plus side, increased communication can lead to less likelihood of isolation, with easy opportunity to share good news, seek advice about problems, manage health conditions, and generally enjoy exchanges with people we love ( Czaja, 2017 ; Delello & McWhorter, 2017 ; Leist, 2013 ). But is there a minus side? It is also possible that people are losing the art of face-to-face contact, that people are more negative in less personal forms of communication, witness the rise in cyberbullying, because they do not see another’s reactions. Although it is recognized that these public forms of communication can be hurtful, little is currently being done to restrict such negativity. These are challenges that clearly must be addressed. Incorporating new ways of having social relations into theory, recognizing that the use and benefits of technology likely vary according to personal and situational characteristics, and that these new social relations will influence health in unique ways represent important future directions. The convoy model provides a helpful framework for thinking about the ways in which new technologies create new forms of social relations. It is quite clear that the advent and evolution of new communication technologies provide exciting and promising new directions for how we develop, use, and experience social relations.
Conflict of Interest
None reported.
This work was supported by the National Institutes of Health 1R01AG045423-01.
- Adams R. G. & Blieszner R (Eds.). (1989). Older adult friendship: Structure and process . Newbury Park, CA: Sage. [ Google Scholar ]
- Akiyama H., Antonucci T. C., & Campbell R (1997). Exchange and reciprocity among two generations of Japanese and American women . In Sokolovsky J. (Ed.), Cultural Context of aging: Worldwide perspectives (2nd ed, pp. 127–138). Westfort, CT: Greenwood Press. [ Google Scholar ]
- Alterovitz S. S.-R., & Mendelsohn G. A (2009). Partner preferences across the life span: Online dating by older adults . Psychology and Aging , 24 , 513–517. doi:10.1037/a0015897 [ PubMed ] [ Google Scholar ]
- Althaus S. L., & Tewksbury D (2000). Patterns of Internet and traditional news media use in a networked community . Political Communication , 17 , 21–45. doi:10.1080/105846000198495 [ Google Scholar ]
- Anderson M. (2015). Technology Device Ownership: 2015 Retreived August 11, 2017, from http://www.pewinternet.org/2015/10/29/technology-device-ownership-2015/
- Antonucci T. (2001). Social relations: An examination of social networks, social support, and sense of control . In Birren J., & Schaie K. (Eds.), Handbook of the psychology of aging (5th ed). San Diego: Academic Press. [ Google Scholar ]
- Antonucci T. C., Ajrouch K. J., & Birditt K. S (2014). The convoy model: Explaining social relations from a multidisciplinary perspective . The Gerontologist , 54 , 82–92. doi:10.1093/geront/gnt118 [ PMC free article ] [ PubMed ] [ Google Scholar ]
- Antonucci T. C., & Akiyama H (1995). Convoys of social relations: Family and friendships within a life span context . In Blieszner R. & Bedford V. H. (Eds.), Handbook of aging and the family (pp. 355–372). Westport, CT: Greenwood Press. [ Google Scholar ]
- Antonucci T. C., Birditt K. S., & Webster N. J (2010). Social relations and mortality: A more nuanced approach . Journal of Health Psychology , 15 , 649–659. doi:10.1177/1359105310368189 [ PubMed ] [ Google Scholar ]
- Antonucci T. C., Jackson J. S., & Biggs S (2007). Intergenerational relations: Theory, research, and policy . Journal of Social Issues , 63 , 679–693. doi:10.1111/j.1540-4560.2007.00530.x [ Google Scholar ]
- Ajrouch K. J., Fuller H. R., Akiyama H., & Antonucci T. C (2017). Convoys of social relations in cross-national context . The Gerontologist . Epub ahead of print. doi:10.1093/geront/gnw204 [ PMC free article ] [ PubMed ] [ Google Scholar ]
- Bengtson V. L. (2001). Beyond the nuclear family: The increasing importance of multigenerational bonds . Journal of Marriage and Family , 63 , 1–16. Retrieved from http://onlinelibrary.wiley.com/doi/10.1111/j.1741-3737.2001.00001.x/full [ Google Scholar ]
- Bengtson V. L., & Kuypers J. A (1971). Generational difference and the “developmental stake.” Aging and Human Development , 2 , 249–260. doi:10.2190/AG.2.4.b [ Google Scholar ]
- Bianchi S. M. (2014). A demographic perspective on family change . Journal of Family Theory and Review , 6 , 35–44. doi:10.1111/jftr.12029 [ PMC free article ] [ PubMed ] [ Google Scholar ]
- Brown S. L., & Lin I.-F (2013). The gray divorce revolution: Rising divorce among middle-aged and older adults, 1990–2010 . Journals of Gerontology Series B Psychological Sciences and Social Sciences , 67 , 731–741. doi:10.1093/geronb/gbs089 [ PMC free article ] [ PubMed ] [ Google Scholar ]
- Brown S. L., & Shinohara S. K (2013). Dating relationships in older adulthood: A national portrait susan . Journal of Marriage and Family , 75 , 1194–1202. doi:10.1021/nl061786n.Core-Shell [ PMC free article ] [ PubMed ] [ Google Scholar ]
- Cantor M. H. (1979). Neighbors and friends: An overlooked resource in the informal support system . Research on Aging , 1 , 434–463. doi:10.1177/016402757914002 [ Google Scholar ]
- Cody M. J., Dunn D., Hoppin S., & Wendt P (1999). Silver surfers: Training and evaluating Internet use among older adult learners . Communication Education , 48 , 269–286. doi:10.1080/03634529909379178 [ Google Scholar ]
- Conney T. M., & Dunne K (2001). Intimate relationships in later life: Current realities, future prospects . Journal of Family Issues , 22 , 838–858. doi:10.1177/019251301022007003 [ Google Scholar ]
- Cooley C. H. (1902). Human nature and the social order . New York: Scribner’s. [ Google Scholar ]
- Cotten S. R., Anderson W. A., & McCullough B. M (2013). Impact of internet use on loneliness and contact with others among older adults: Cross-sectional analysis . Journal of Medical Internet Research , 15 , e39 doi:10.2196/jmir.2306 [ PMC free article ] [ PubMed ] [ Google Scholar ]
- Czaja S. J. (2017). The potential role of technology in supporting older adults . Public Policy and Aging Report , 27 , 44–48. doi:10.1093/ppar/prx006 [ Google Scholar ]
- Czaja S. J., Boot W. R., Charness N., Rogers W. A., & Sharit J (2017). Improving social support for older adults through technology: Findings from the PRISM randomized controlled trial . The Gerontologist , gnw249 [Epub ahead of print]. doi:10.1093/geront/gnw249 [ PMC free article ] [ PubMed ] [ Google Scholar ]
- Davis E. M., & Fingerman K. L (2016). Digital dating: Online profile content of older and younger adults . Journals of Gerontology, Series B: Psychological Sciences and Social Sciences , 71 , 959–967. doi:10.1093/geronb/gbv042 [ PMC free article ] [ PubMed ] [ Google Scholar ]
- Delello J. A., & McWhorter R. R (2017). Reducing the digital divide: Connecting older adults to ipad technology . Journal of Applied Gerontology: The Official Journal of the Southern Gerontological Society , 36 , 3–28. doi:10.1177/0733464815589985 [ PubMed ] [ Google Scholar ]
- Durkheim É. (1915). Elementary forms of the religious life . New York, Free Press. [ Google Scholar ]
- Elliot A. J., Mooney C. J., Douthit K. Z., & Lynch M. F (2014). Predictors of older adults’ technology use and its relationship to depressive symptoms and well-being . The Journals of Gerontology, Series B: Psychological Sciences and Social Sciences , 69 , 667–677. doi:10.1093/geronb/gbt109 [ PMC free article ] [ PubMed ] [ Google Scholar ]
- Fingerman K. L., & Birditt K. S (2011). Relationships between adults and their aging parents . In Schaie K. W., & Willis S. L. (Eds.), Handbook of the Psychology of Aging (7th ed, pp. 219–232). Elsevier Inc; 10.1016/B978-0-12-380882-0.00014-0 [ CrossRef ] [ Google Scholar ]
- Fingerman K. L., Sechrist J., & Birditt K (2013). Changing views on intergenerational ties . Gerontology , 59 , 64–70. doi:10.1159/000342211 [ PMC free article ] [ PubMed ] [ Google Scholar ]
- Fiori K. L., Antonucci T. C., & Akiyama H (2008). Profiles of social relations among older adults: A cross-cultural approach . Ageing and Society , 28 , 203–231. doi:10.1017/S0144686X07006472 [ Google Scholar ]
- Fiori K. L., Smith J., & Antonucci T. C (2007). Social network types among older adults: A multidimensional approach . The Journals of Gerontology, Series B: Psychological Sciences and Social Sciences , 62 , P322–P330. doi:10.1093/geronb/62.6.P322 [ PubMed ] [ Google Scholar ]
- Giles L. C., Glonek G. F., Luszcz M. A., & Andrews G. R (2007). Do social networks affect the use of residential aged care among older Australians ? BMC Geriatrics , 7 , 24 doi:10.1186/1471-2318-7-24 [ PMC free article ] [ PubMed ] [ Google Scholar ]
- Gubernskaya Z., & Treas J (2016). Call home? Mobile phones and contacts with mother in 24 countries . Journal of Marriage and Family , 78 , 1237–1249. doi:10.1111/jomf.12342 [ PMC free article ] [ PubMed ] [ Google Scholar ]
- Heisler M. & Piette J. D (2005). “I help you, and you help me”: facilitated telephone peer support among patients with diabetes . The Diabetes Educator , 31 , 869–879. doi:10.1177/0145721705283247 [ PubMed ] [ Google Scholar ]
- Hunter A. (2015). Empowering or impeding return migration? ICT, mobile phones, and older migrants’ communications with home . Global Networks , 15 , 485–502. doi:10.1111/glob.12091 [ Google Scholar ]
- Hurme H., Westerback S., & Quadrello T (2010). Traditional and new forms of contact between grandparents and grandchildren . Journal of Intergenerational Relationships , 8 , 264–280. doi:10.1080/15350770.2010.498739 [ Google Scholar ]
- Jaeger P. T., & Xie B (2009). Developing online community accessibility guidelines for persons with disabilities and older adults . Journal of Disability Policy Studies , 20 , 55–63. doi:10.1177/1044207308325997 [ Google Scholar ]
- Kahn R. L., & Antonucci T. C (1980). Convoys over the life course: Attachment, roles and social support . In Baltes P. B. & Brim O. G. (Eds.), Life-span development and behavior (pp. 253–286). New York: Academic Press. [ Google Scholar ]
- Khvorostianov N., Elias N., & Nimrod G (2012). ‘Without it I am nothing’: The internet in the lives of older immigrants . New Media and Society , 14 , 583–599. doi:10.1177/1461444811421599 [ Google Scholar ]
- Leist A. K. (2013). Social media use of older adults: A mini-review . Gerontology , 59 , 378–384. doi:10.1159/000346818 [ PubMed ] [ Google Scholar ]
- Lowenthal M. F. & Haven C (1968). Interaction and adaptation: Intimacy as a critical variable . American Sociological Review , 33 , 20–30. doi:10.2307/2092237 [ PubMed ] [ Google Scholar ]
- Mead G. H. (1913). The social self . Journal of Philosophy, Psychology and Scientific Methods , 10 , 374–380. doi:10.2307/2012910 [ Google Scholar ]
- Piette J. D., Striplin D., Marinec N., Chen J., & Aikens J. E (2015). A randomized trial of mobile health support for heart failure patients and their informal caregivers: Impacts on caregiver-reported outcomes . Medical Care , 53 , 692–699. doi:10.1097/MLR.0000000000000378 [ PMC free article ] [ PubMed ] [ Google Scholar ]
- Quadrello T., Hurme H., Menzinger J., Smith P. K., Veisson M., Vidal S., & Westerback S (2005). Grandparents use of new communication technologies in a European perspective . European Journal of Ageing , 2 , 200–207. doi:10.1007/s10433-005-0004-y [ PMC free article ] [ PubMed ] [ Google Scholar ]
- Shanas E., Townsend P., Wedderburn D., Friis H., Milhoj P., & Stehouwer J (Eds.) (1968). Old people in three industrial societies . New York: Atherton Press. [ Google Scholar ]
- Silverstein M., & Bengtson V. L (1997). Intergenerational solidarity and the structure of adult child-parent relationships in American families . American Journal of Sociology , 103 , 429–460. doi:10.1086/231213 [ Google Scholar ]
- Silverstein M., Parrott T., Angelinni J. J., & Cook F. L (2000). Solidarity and tension between age groups in the United States: Challenge for an aging America in the 21st century . International Journal of Social Welfare , 9 , 270–284. doi:10.1111/1468-2397.00139 [ Google Scholar ]
- Sims T., Reed A. E., & Carr D. C (2016). Information and communication technology use is related to higher well-being among the oldest-old . The Journals of Gerontology, Series B: Psychological Sciences and Social Sciences , gbw130. doi:10.1093/geronb/gbw130 [ PubMed ] [ Google Scholar ]
- Stephure R. J., Boon S. D., Mackinnon S. L., & Deveau V. L (2009). Internet initiated relationships: Associations between age and involvement in online dating . Journal of Computer-Mediated Communication , 14 , 658–681. doi:10.1111/j.1083-6101.2009.01457.x [ Google Scholar ]
- Suitor J. J., Sechrist J., Gilligan M., & Pillemer K (2011). Intergenerational relations in later-life families . In Settersten R., and Angel J. (Eds.) Handbook of Sociology of Aging (pp. 161–178). New York: Springer; 10.1007/978-1-4419-7374-0_11 [ CrossRef ] [ Google Scholar ]
- Swartz T. T. (2009). Intergenerational family relations in adulthood: Patterns, variations, and implications in the contemporary United States . Annual Review of Sociology , 35 , 191–212. doi:10.1146/annurev.soc.34.040507.134615 [ Google Scholar ]
- Troll L. E. (1971) The family of later life: A decade review . Journal of Marriage and the Family , 33 , 263–290. doi:10.2307/349414 [ Google Scholar ]
- Valkenburg P. M., & Peter J (2007). Who visits online dating sites? Exploring some characteristics of online daters . CyberPsychology and Behavior , 10 , 849–852. doi:10.1089/cpb.2007.9941 [ PubMed ] [ Google Scholar ]
- Wada M., Mortenson W. B., & Hurd Clarke L (2016). Older adults’ online dating profiles and successful aging . Canadian Journal on Aging/La Revue Canadienne Du Vieillissement , 35 , 479–490. doi:10.1017/S0714980816000507 [ PubMed ] [ Google Scholar ]
- Webster N. J., Antonucci T. C., Yoon C., McCullough W. R., Fin D. N., & Hartsell D. L (2012). Older adults as consumers: An examination of differences by birth cohort . In Preston S., Kringelback M., & Knutson B. (Eds.) Interdisciplinary Science of Consumption (pp. 281–298). Cambridge, MA: MIT Press. [ Google Scholar ]
- Wiemers E. E., Seltzer J. A., Schoen R. F., Hotz V. J. & Bianchi S. M (2016). The Generational Structure of U.S. Families and Their Intergenerational Transfers . PSC Research Report No. 16–867. 7 2016. [ Google Scholar ]
- UCLA CCPR Population Working Papers . Retrieved from http://papers.ccpr.ucla.edu/index.php/pwp/article/view/PWP-CCPR-2016-036 10.1186/s40711-016-0045-y . [ Google Scholar ]
- Zhang J. (2016). Aging in cyberspace: Internet use and quality of life of older Chinese migrants . The Journal of Chinese Sociology , 3 , 26. doi:10.1186/s40711-016-0045-y [ Google Scholar ]
- Zickuhr K. & Madden M (2012). Older adults and Internet use. For the first time, half of adults aged 65 and older are online Retrieved from http://pewinter- net.org/Reports/2012/Older-adults-and-in- ternet-use.aspx
How technology use is changing adolescents’ behaviors and their social, physical, and cognitive development
- Published: 19 January 2023
- Volume 42 , pages 16466–16469, ( 2023 )
Cite this article
- Martina Benvenuti ORCID: orcid.org/0000-0001-8575-5047 1 ,
- Michelle Wright ORCID: orcid.org/0000-0002-6703-8586 2 ,
- John Naslund ORCID: orcid.org/0000-0001-6777-0104 3 &
- Anne C. Miers ORCID: orcid.org/0000-0001-5077-4731 4
34k Accesses
7 Citations
Explore all metrics
Avoid common mistakes on your manuscript.
The rapid spread of Information and Communication Technologies (ICTs) in recent decades has pushed the use of smartphones, tablets, and their applications to become pervasive tools used in everyday life, especially among adolescents (Khang et al., 2013 ; Odaci & Çıkrıkçı, 2014 ). The possibility of being constantly connected significantly increases the amount of time adolescents spend online, and Web and mobile applications are ubiquitous in many adolescents’ lives (Durak, 2018 ). Research has shown that adolescents use the Internet more frequently (compared to adults) than other generations and in a more multipurpose context (Cain & Gradisar, 2010 ; Livingstone et al., 2011 ; O’Keeffe et al., 2011 ; Tzavela et al., 2015 , Casaló & Escario, 2018 ). The use of digital applications has also dramatically changed the way adolescents relate to their peers, access information, and engage in social relationships, and has also had a profound influence on their health, including their well-being (e.g., the impact of smartphone uses on the sleep-waking cycle), and on their cognitive development (e.g., level of attention in carrying out a task). Given the importance of considering technology use as having a profound role in adolescent development, one key question many scholars are now attempting to answer concerns how adolescents’ online presence shapes their offline lives; this question could be encapsulated as follows: “ Is technology use changing adolescents’ behaviors, their social, physical, and cognitive development? ”
Our principal focus in this special issue is on adolescence and digital natives. In this regard, we consider adolescence as the transition between childhood and adulthood, characterized by biological, cognitive, social, and psychological changes (Berk, 2017 ). Adolescents are also commonly referred to as “Digital Natives” (Prensky, 2001 ), meaning that they were born into a world where technology is central to daily life, they are learning and adopting technology from infancy, and as a result they think and process information in fundamentally different ways when compared to their predecessors, referred it as “digital immigrants”. Considering this definition, it is also important to note that the duration of adolescence, its demands, and pressures vary substantially between cultures (Berk, 2017 ). Most tribal and village societies have only a brief intervening phase between childhood and the full assumption of an adult role (Berk, 2017 ). On the other hand, young people in Western societies (e.g., Europe and North America) face a prolonged dependence on their parents and the postponement of sexual gratification while they prepare for a productive working life. As a result, adolescence is often greatly extended in these countries. In this regard, according to Berk ( 2017 ), for this special issue, we consider a wide range of the meaning of adolescence, which is reflected in the included papers that considered the following groups: Early Adolescence (11–12 to 14 years): this is a period of rapid pubertal change; Middle Adolescence (14 to 16 years): pubertal changes are now almost complete; Late Adolescence (16 to 18 years): the young person achieves their full adult appearance and begins to take on more adult roles. Then, considering all these aspects, the main goal of this special issue is to include papers on the impact of technology on adolescent development. Papers published consider different fields of research (e.g., psychology, sociology, health, data science, cognitive science) that describe the impact of technology on behavioral, social, physical, and cognitive changes during adolescence, and how technology may influence these changes in both positive or negative ways throughout adolescent development (psychological, sociological, physical, and cognitive). With this wide range of topics described across the included contributions, then we can ideally divide these papers into three macro sections.
Section 1: Behavioral and psychological changes during adolescence using technology
Around 71% of young people worldwide are internet-users compared to 48% of the world’s population. The highest reported percentage of internet users in this age group is in Europe where 97% of 15 to 24 year-olds are internet users.
Around 71% of young people worldwide are internet-users compared to 48% of the world’s population. The highest reported percentage of internet users in this age group is in Europe where 97% of 15- to 24-year-olds are internet users Footnote 1 . Similarly, in many lower resource countries globally, use of technology among adolescents is increasing rapidly, with smartphones, mobile apps, and social media platforms featuring prominently in the daily lives of many adolescents. Therefore, the internet provides teens with various opportunities, including the formation and maintenance of peer relationships, entertainment (e.g., watching videos and movies), access to information for schoolwork, and fast communication with parents, friends, and other individuals (Lenhart, 2015 ). Complicating the “picture” of teens’ online behaviors is that they often coordinate this activity simultaneously with spending time with their friends online and offline (Lenhart et al., 2015 ). Research suggests that many teens “hang out” with their friends online and “hangout” with their friends in person too (Lenhart et al., 2015 ). Despite these opportunities, teens are potentially exposed to harmful or disturbing content, experience internet victimization or online hate, and are exposed to identity theft, unwanted sexual solicitation, and sexual predation (Kuss et al., 2013 ; Weaver et al., 2011 ; Yakovlev & Kinney, 2008 ). Teens who are low-income, have a history of maltreatment, and disclose more personal information online are more likely to experience these online risks (Noll et al., 2013 ). Researchers, educators, and clinicians are also particularly concerned with the psychological risks and addictive potential of excessive internet use among teens. Prevention efforts have focused attention on promoting digital literacy skills, training parents and educators on teens’ exposure to online risks and promoting kindness online through various social awareness campaigns. Papers that refer to this section come from different cultures and social contexts and analyze the behavior of adolescents in the use of technologies. Summarizing the results of these research, it is highlighted how the time and way of using the internet (in general) and specific technologies influence adolescents’ behavior (development and application), and how this affects their personality, their social relationships, and their relationship with family and with peers.
Section 2: Cognitive and physiological development as well as impacts on health, mental health, and wellbeing
Having near constant access to technology and internet might affect teens’ cognitive development. The constant access to the internet might hinder teens’ memory abilities or the ability to engage in effortful thinking (Nasi & Koivusilta, 2012 ). Research indicates that near-constant access to the internet influences the kind of information people choose to remember (Sparrow et al., 2011 ). Such a finding might suggest an adaptation to the online environment where it is more efficient to remember where to access information easily than remembering specific information. Another concern is that teens’ ability to multitask between various activities, those online and offline, could shorten their attention spans. Furthermore, multitasking might include simultaneously accessing digital media and the internet while also engaging in other activities, like household chores (Common Sense Media, 2015 ). Social interactions online might also occur simultaneously with socializing offline, as teens might play consoles or mobile phone games, surf social media, and video chat others while a friend is present. Findings from one study revealed that heavy media users were much better at switching between tasks than light media users (Alzahabi & Becker, 2013 ). By focusing attention on the function for which a technology is built and reasoning from the adolescent development perspective, it is possible to say that when the use of a technology supports an adolescent activity (or compensates for its shortcomings) to reach a goal or to facilitate its achievement, then this technology is functional to the adolescent development. On the contrary, when this does not happen the technology is dysfunctional and can lead to problematic use. Papers in this section highlight the results of research that show the effects and potential of technologies in the development of adolescent well-being, with a focus also on risk prevention, gender differences and gender equality.
Section 3: Explores potential for digital health interventions (DHIs) to have positive effects on promoting adolescent health/wellbeing, adoption of positive health behaviors, cognitive development, and positive impact on other important aspects of adolescent development
Digital health interventions (DHIs), involving computer-assisted therapy, smartphone apps, online interventions, and wearable technologies, have been developed for a wide variety of adolescent mental health outcomes such as anxiety, depression, loneliness, autism, psychosis, attention deficit hyperactivity disorder, eating disorders, and post-traumatic stress disorder (Alvarez-Jimenez., et al. 2014 ; Hollis et al., 2017 ; Lim et al., 2019 ). According to a systematic and meta-review, modest clinical benefit of DHIs was found mainly for depression and anxiety and particularly for computerized cognitive behavioral therapy (cCBT; Hollis et al., 2017 ). In short, anxiety and depression symptoms were found to improve through cCBT. However, the evidence was mainly relevant to late adolescents (16–18 years old) with mild to moderate symptoms, though for other mental health outcomes (e.g., psychosis, ADHD, eating disorders) the evidence is limited. Moreover, the review concluded that DHIs were not necessarily favored by adolescents’ over face-to-face contact. This suggests that the commonly held assumption that DHIs are preferred among adolescents may not accurately portray their views or perceptions, and explorative qualitative research could inform this issue (Hollis et al., 2017 ). Methodological issues abound in this field: there is great heterogeneity among DHIs, and a taxonomy does not exist to specify components of DHIs making comparison across studies difficult; studies are generally under-powered and poorly controlled. Hollis et al. ( 2017 ) suggested that more research should be conducted on how DHIs should be tailored to personal requirements, what the active components or ingredients in DHIs are (i.e., what is driving change?), cost-effectiveness, investigation of how much human facilitation/support is necessary, DHI effects in the offline world, and the use and effectiveness of mHealth smartphone/tablet apps. Additionally, most studies rely on self-reported data from subjects, making it difficult to fully explore the effects of technology use on different changes in physiology and behaviors (George & Odgers, 2015 ). Results described in the papers referring to this section show how DHIs are extremely relevant for adolescents, and how they can positively support the changes that occur in this age, considering the uncertainties, changes and physical exploration and different stages of adolescence.
In this special issue we have tried to collect the latest research that addresses the most relevant issues for adolescents, taking into consideration a broad spectrum of cultures and populations of adolescents. Future research on these issues should take into consideration some aspects that may be relevant in the future for adolescents, who will later become adults in the future. A very relevant aspect is certainly their well-being, an in-depth study on the use of technologies and well-being could lead to results and the formation of educational and psychological paths, that can help adolescents to face the challenges that this difficult phase of development offers. A second aspect concerns the importance of monitoring the development of changes in interpersonal relationships, both at the family level and with peers. The relevance of building relationships in adolescence is fundamental for a positive physical and psychological development. The significance of online relationships is linked to this aspect. In the teenagers of tomorrow, now digital natives, there is no longer the difference between online relationships and offline relationships, there is a concept of onlife: their relationships merge into a unique dimension. The value of focusing on managing these relationships is also fundamental for handling the use of social networking sites (SNSs) and adolescents’ online reputation (What place and with whom). Finally, we want to emphasize the meaning of research that can include multiple scientific, interdisciplinary sectors that can create ideas for the new challenges of the future, and that can keep pace with the social, cultural, and behavioral changes of future adolescents worldwide.
https://www.wish.org.qa/wp-content/uploads/2022/04/WISH-Digital-Technology-and-Child-Wellbeing.12.04.22-V06.pdf .
References
Alvarez-Jimenez, M., Alcazar-Corcoles, M. A., Gonzalez-Blanch, C., Bendall, S., McGorry, P. D., & Gleeson, J. F. (2014). Online, social media and mobile technologies for psychosis treatment: a systematic review on novel user-led interventions. Schizophrenia research , 156 (1), 96–106.
Article PubMed Google Scholar
Alzahabi, R., & Becker, M. W. (2013). The association between media multitasking, task-switching, and dual-task performance. Journal of Experimental Psychology: Human Perception and Performance , 39 (5), 1485–1495. https://doi.org/10.1037/a0031208
Berk, L. E. (2017). Exploring lifespan development . Pearson.
Cain, N., & Gradisar, M. (2010). Electronic media use and sleep in school-aged children and adolescents: a review. Sleep Medicine , 11 (8), 735–742. https://doi.org/10.1016/j.sleep.2010.02.006
Casalo, L. V., & Escario, J. (2018). Predictors of excessive internet use among adolescents in Spain: the relevance of the relationship between parents and their children. Computers in Human Behavior , 92 , 344–351. https://doi.org/10.1016/j.chb.2018.11.042
Article Google Scholar
Common Sense Media (2015). The common sense census: Media use by tweens and teens. Retrieved from: https://www.commonsensemedia.org/research/the-common-sense-census-media-use-by-tweens-and-teens-2015
Durak, H. Y. (2018). Digitial story design activities used for teaching programming effect on learning of programming concepts, programming self-efficacy, and participation and analysis of student experiences. Journal of Computer Assisted Learning , 34 (6), 740–752. https://doi.org/10.1111/jcal.12281
George, M. J., & Odgers, C. L. (2015). Seven fears and the science of how mobile technologies may be influencing adolescents in the digital age. Perspectives on psychological science , 10 (6), 832–851. Retrieve from: https://www.ncbi.nlm.nih.gov/pmc/articles/PMC4654691/pdf/nihms704598.pdf
Article PubMed PubMed Central Google Scholar
Hollis, C., Falconer, C. J., Martin, J. L., Whittington, C., Stockton, S., Glazebrook, C., & Davies, E. B. (2017). Annual Research Review: Digital health interventions for children and young people with mental health problems–a systematic and meta-review. Journal of Child Psychology and Psychiatry , 58 (4), 474–503.
Keeley, B., & Little, C. (2017). The State of the Worlds Children 2017: Children in a Digital World . UNICEF. 3 United Nations Plaza, New York, NY 10017. Retrive from: https://www.unicef.org/publications/files/SOWC_2017_ENG_WEB.pdf
Khang, H., Kim, J. K., & Kim, Y. (2013). Self-traits and motivations as antecedents of digital media flow and addiction: the internet, mobile phones, and video games. Computers in Human Behavior , 29 (6), 2416–2424. https://doi.org/10.1016/j.chb.2013.05.027
Krantz, L., & Jaffray, P. (2017). Taking stock with teens – spring 2017 . A collaborative consumer insights project. Retrieved from: http://www.piperjaffray.com/3col.aspx?id=4359
Kuss, D. J., van Rooij, A. J., Shorter, G. W., Griffiths, M. D., & van de Mheen, D. (2013). Internet addiction in adolescents: prevalence and risk factors. Computers in Human Behavior , 29 (5), 1987–1996. https://doi.org/10.1016/j.chb.2013.04.002
Lenhart, A. (2015). Teens, social media & technoloy: Overview 2015. Retrieved from: https://www.pewresearch.org/internet/2015/04/09/teens-social-media-technology-2015/
Lenhart, A., Smith, A., Anderson, M., Duggan, M., & Perrin, A. (2015). Teens, Technology and Friendships. Pew Research Center. Retrieved from: https://www.pewresearch.org/internet/2015/08/06/teens-technology-and-friendships/
Lim, M. H., Rodebaugh, T. L., Eres, R., Long, K., Penn, D. L., & Gleeson, J. F. (2019). A pilot digital intervention targeting loneliness in youth mental health. Frontiers in psychiatry , 10 , 604.
Livingstone, S., Haddon, L., Görzig, A., & Ólafsson, K. (2011). Risks and safety on the internet: the perspective of European children: full findings and policy implications from the EU Kids Online survey of 9–16 year olds and their parents in 25 countries. Retrieved from: http://eprints.lse.ac.uk/33731/1/Risks%20and%20safety%20on%20the%20internet(lsero).pdf
Livingstone, S., Nandi, A., Banaji, S., & Stoilova, M. (2017). Young adolescents and digital media: uses, risks and opportunities in low-and middle-income countries: a rapid evidence review. Retrieved from http://eprints.lse.ac.uk/83753/1/Livingstone_Young_Adolescents_Digital_Media.pdf
Munafò, M. R., Nosek, B. A., Bishop, D. V., Button, K. S., Chambers, C. D., Sert, D., & Ioannidis, N. P., J. P (2017). A manifesto for reproducible science. Nature human behaviour , 1 (1), 1–9.
Nasi, M., & Koivusilta, L. (2013). Internet and everyday life: the perceived implications of internet use on memory and ability to concentrate. CyberPsychology Behavior & Social Networking , 16 (2), 88–93. https://doi.org/10.1089/cyber.2012.0058
Noll, J. G., Shenk, C. E., Barnes, J. E., & Haralson, K. J. (2013). Association of maltreatment with high-risk internet behaviors and offline encounters. Pediatrics , 131 (2), e510–e517. https://doi.org/10.1542/peds.2012-1281
O’Keeffe, G. S., Clarke-Pearson, K., & Council on Communications and Media. (2011). The impact of social media on children, adolescents, and families. Pediatrics , 127 (4), 800–804. https://doi.org/10.1542/peds.2011-0054
Open Science Collaboration (2015). Estimating the reproducibility of psychological science. Science , 349 (6251).
Odaci, H., & Cikrikci, O. (2014). Problematic internet use in terms of gender, attachment styles and subjective well-being in university tudents. Computers in Human Behavior , 32 (C), 61–66.
Prensky, M. (2001). Digital natives, digital immigrants part 1. On the horizon , 9 (5), 1–6.
Sparrow, B., Liu, J., & Wegner, D. M. (2011). Google effects on memory: Cognitive consequences of having information at our fingertips . Science, 333, 776–778.
Tsitsika, A. K., Tzavela, E. C., Janikian, M., Olafsson, K., Iordache, A., Schoenmakers, T. M., & Richardson, C. (2014). Online social networking in adolescence: patterns of use in six european countires and links with psychosocial funcitonin. Journal of Adolescent Health , 55 (1), 141–147. https://doi.org/10.1016/j.jadohealth.2013.11.010
Tzavela, E. C., Karakitsou, C., Dreier, M., Mavromati, F., W?lfling, K., Halapi, E., Macarie, G., Wójcik, .S, Veldhuisgh, L., & Tsitsika, A. K. (2015). Processes discriminating adaptive and maladaptive Internet use among European adolescents highly engaged online. Journal of adolescence , 40 , 34–47.
Weaver, J. B., Weaver, S. S., Mays, D., Hopkins, G. L., Kannenberg, W., & McBride, D. (2011). Mental- and physical-health indicators and sexually explicit media use behavior by adults. The Journal of Sexual Medicine , 8 (3), 764–771. https://doi.org/10.1111/J.1743-6109.2010.02030.X
Yakovlev, P., & Kinney, L. (2008). Additional evidence on the effect of class attendance on academic performance. Atlantic Economics Journal , 36 (4), 493–494. https://doi.org/10.1007/s11293-008-9142-x
Download references
Author information
Authors and affiliations.
Department of Psychology, University of Bologna, Bologna, Italy
Martina Benvenuti
DePaul University, Chicago, USA
Michelle Wright
Department of Global Health and Social Medicine, Harvard Medical School, Boston, USA
John Naslund
Developmental and Educational Psychology Unit, Institute of Psychology, Leiden University, Leiden, Netherlands
Anne C. Miers
You can also search for this author in PubMed Google Scholar
Corresponding author
Correspondence to Martina Benvenuti .
Additional information
Publisher’s note.
Springer Nature remains neutral with regard to jurisdictional claims in published maps and institutional affiliations.
Rights and permissions
Reprints and permissions
About this article
Benvenuti, M., Wright, M., Naslund, J. et al. How technology use is changing adolescents’ behaviors and their social, physical, and cognitive development. Curr Psychol 42 , 16466–16469 (2023). https://doi.org/10.1007/s12144-023-04254-4
Download citation
Published : 19 January 2023
Issue Date : July 2023
DOI : https://doi.org/10.1007/s12144-023-04254-4
Share this article
Anyone you share the following link with will be able to read this content:
Sorry, a shareable link is not currently available for this article.
Provided by the Springer Nature SharedIt content-sharing initiative
- Find a journal
- Publish with us
- Track your research
- Ethical Theory
Comparative Hypotheses for Technology Analysis
- In book: Global Encyclopedia of Public Administration, Public Policy, and Governance (pp.1-8)
- Publisher: Springer Nature Switzerland

- Italian National Research Council
Discover the world's research
- 25+ million members
- 160+ million publication pages
- 2.3+ billion citations

- SENSORS-BASEL

- J ENG TECHNOL MANAGE
- TECHNOL FORECAST SOC

- Recruit researchers
- Join for free
- Login Email Tip: Most researchers use their institutional email address as their ResearchGate login Password Forgot password? Keep me logged in Log in or Continue with Google Welcome back! Please log in. Email · Hint Tip: Most researchers use their institutional email address as their ResearchGate login Password Forgot password? Keep me logged in Log in or Continue with Google No account? Sign up
More From Forbes
How we can shape the technology we need for the world we want.
- Share to Facebook
- Share to Twitter
- Share to Linkedin
Our aspirations and actions influence the environment that we are part of, including the technology ... [+] that we interact with.
In an era where AI can compose symphonies and drive cars , we stand at a crossroads. The choices we make today will shape the contours of tomorrow's world. But how can we ensure the expanding technological tsunami propels us towards a brighter future rather than sweeping away our humanity?
The Internet of Things (IoT) is rapidly evolving into the Internet of Everything. Our devices aren't just connected; they're becoming extensions of ourselves. The line between user and tool blurs with each passing innovation. Whether the trend of this innovative dynamic is in the interest of people and planet, or pure profit depends on us. Our choices matter.
From Code to Conscience: The Ripple Effect
Every interaction with AI, from a simple Google search to a complex financial algorithm, leaves an indelible mark on the digital landscape. When millions choose privacy-centric platforms like DuckDuckGo over data-hungry alternatives, it sends shockwaves through Silicon Valley.
Consider the AI for Good movement. What started as a niche initiative has blossomed into a global phenomenon, with tech giants and startups alike vying to showcase their ethical AI credentials. This shift isn't just changing products; it's reshaping entire business philosophies. It may seem far out to draw the connect between the individual consumer and gigantic tech companies such as OpenAI or Google, yet ultimately every person matters in the kaleidoscopic ‘reality’ of society.
Every app we choose, every AI-driven service we use, and every tech company we (in)directly support is a vote for the future we do (not) want to see.
What To Know About Olympics Gender Debate As Imane Khelif Prepares For Next Women’s Boxing Match
Today’s nyt mini crossword clues and answers for friday, august 2, jam-proof fiber optic drone testing in ukraine, augmenting humanity: ai as the great enabler.
A lot has been said about the threat that AI poses to jobs – and a lot of up-skilling, down-skilling, cross-skilling is happening already. But whether the expansion of this new general purpose tool into ever more areas of life and work helps or harms us is yet to be seen. An optimistic angle may serve us best during this transition. Because whereas the avoidance of negative outcomes undermines our creativity, a focus on opportunities unleashes our creativity in making the best out of a situation, as shown by research long before the onset of generative AI. It is up to us how we distribute our tasks, and which of them we delegate to our 24/7 assistants.
Perhaps the true power of AI lies not in mimicking humans, but in amplifying our unique capabilities. Conscious complementarity may take us closer to solving certain lingering social challenges; for example in precision medicine where IBM's Watson for Oncology is partnering with doctors to create personalized cancer treatment plans, potentially saving countless lives. Or take tools like DALL-E that are transforming anyone with an idea into a visual artist, democratizing creativity in unprecedented ways. Another example of prosocial AI is Microsoft's AI for Earth which is tailored for the use of AI to track endangered species, predict natural disasters, and optimize renewable energy systems. The scope and direction of each of these examples was decided upon by people, individuals like you and me.
Take the LEAD: Architecting an Ethical AI Future
To steer this AI revolution towards the greater good, each of us can embrace:
- Leadership : Visionaries like Fei-Fei Li , co-director of Stanford's Human-Centered AI Institute, are championing an AI future that enhances rather than replaces human capabilities. No matter the arena we evolve in, we can adopt a human-first perspective in our interaction with AI; as business leaders, our vision influences our teams.
- Ethics : Initiatives like the IEEE Global Initiative on Ethics of Autonomous and Intelligent Systems are setting standards for responsible AI development. The respect and promotion of these standards depends on individuals, and the institutions that they are part of.
- Accessibility : Projects such as AI Commons are working to make AI tools available to solve global challenges, regardless of economic barriers. For those of us who are fortunate to benefit from AI, it matters to remember that we are a tiny fraction of this world's population. Our voice can serve to expand access to others.
- Development : Amid the pursuit of technological prowess, we can seek to push the boundaries of prosocial AI capabilities, while positioning safety and ethical considerations as prime concerns. Whether we are technological experts or informed consumers, the next generation of AI is shaped under our watch.
Crafting Tomorrow, Today
As we navigate this AI-augmented world, each of us holds the power to shape its trajectory. Our daily choices ripple outward, influencing the development and application of AI technologies. By making conscious decisions that prioritize people and planet, we can steer the AI revolution towards a more equitable and sustainable future.
Consider the impact of your digital footprint. When you opt for privacy-respecting services, support ethical AI initiatives, or choose products from companies with strong environmental commitments, you're casting a vote for the future you want to see. Individual actions, multiplied across millions of users, send a message to tech giants and startups alike.
Moreover, our choices extend beyond the digital realm. Supporting local businesses that use AI to reduce waste, participating in community AI literacy programs, or advocating for AI ethics in the workplace all contribute to a more thoughtful integration of AI into our society. By aligning our personal values with our technological choices, we can ensure that AI serves as a tool for collective progress rather than a force that exacerbates existing inequalities.
To make informed choices, it's useful to have basic algorithmic literacy.
- Learn : Exploring AI ethics courses is one step towards informed consumption.
- Expand : Beyond the range of usual platforms, deliberately supporting companies prioritizing responsible AI , can make a difference.
- Act: Contributing to open-source AI projects can be a way to expand the range of models.
- Dare : Envisioning the future that we want to build, and that we want our children to grow up in is the first step toward making that reality happen. If we do not know where we want to go, someone else can easily take us for a ride.
LEAD ership does not depend on status or hierarchy. It is the result of personal choices that each of us can take.
If you are interested in the topic of Agency Amid AI for All (A4), please join us at the United Nations Science Summit in September 2024.
And check my previous articles in this series:
Why AI Inclusion Is A Matter Of Life And Death
How To Use AI As A Creative Sparring Partner
Building Hybrid Resilience In A Tech-Dependent World: Lessons Learned
How Can AI Compensate For Age-Related Cognitive Decline.
Harnessing Human Intelligence In An AI-Driven World
If you have comments or ideas please reach out via LinkedIn
Thank you !
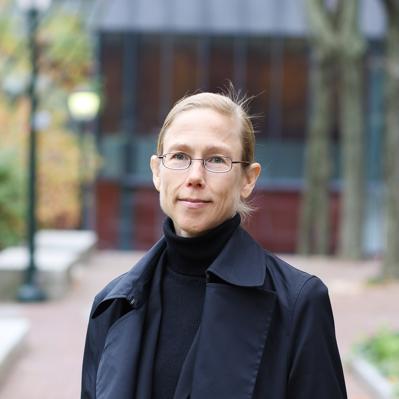
- Editorial Standards
- Reprints & Permissions
Join The Conversation
One Community. Many Voices. Create a free account to share your thoughts.
Forbes Community Guidelines
Our community is about connecting people through open and thoughtful conversations. We want our readers to share their views and exchange ideas and facts in a safe space.
In order to do so, please follow the posting rules in our site's Terms of Service. We've summarized some of those key rules below. Simply put, keep it civil.
Your post will be rejected if we notice that it seems to contain:
- False or intentionally out-of-context or misleading information
- Insults, profanity, incoherent, obscene or inflammatory language or threats of any kind
- Attacks on the identity of other commenters or the article's author
- Content that otherwise violates our site's terms.
User accounts will be blocked if we notice or believe that users are engaged in:
- Continuous attempts to re-post comments that have been previously moderated/rejected
- Racist, sexist, homophobic or other discriminatory comments
- Attempts or tactics that put the site security at risk
- Actions that otherwise violate our site's terms.
So, how can you be a power user?
- Stay on topic and share your insights
- Feel free to be clear and thoughtful to get your point across
- ‘Like’ or ‘Dislike’ to show your point of view.
- Protect your community.
- Use the report tool to alert us when someone breaks the rules.
Thanks for reading our community guidelines. Please read the full list of posting rules found in our site's Terms of Service.
- High School
Part C Use what you've learned in the lesson to develop a hypothesis about how changes in technology affect society. Descril your hypothesis and provide evidence for it in one paragraph. Your response should include a reference to changes in food production and the effects they had on population.
Aidudieo is waiting for your help., expert-verified answer.
- 33K answers
- 15.7M people helped
The advancement of technology has a significant impact on society because it provides people with the means to control their environment and make decisions about their surroundings.
Which development in technology has had the biggest impact on life in this nation?
- The development of the Internet has had a significant impact on both American life and that of the rest of the globe.
- Everywhere in the United States—at work, at home, in hospitals, at school, etc.—Internet usage is having an influence.
What impact does technical advancement have on society?
Among many other things, technology has made it easier to travel, establish cities, and cultivate crops, effectively connecting all nations on earth.
What effects does technology have on people's lives?
- Communication, transportation, education and learning, health care, and many other infrastructure business areas have all benefited from technology .
- Engineering is thought to be the main driver of technological advances in society because it is the practice of developing technology.
Learn more about advancement of technology
brainly.com/question/13743788
Still have questions?
Get more answers for free, expand your knowledge with related topics.
- Tech & Society
You might be interested in
New questions in history.
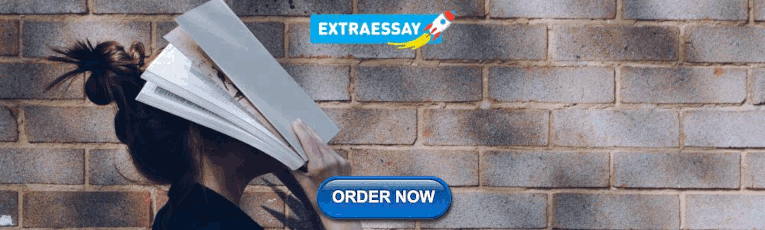
IMAGES
VIDEO
COMMENTS
A crucial part of understanding how technology has created global change and, in turn, how global changes have influenced the development of new technologies is understanding the technologies themselves in all their richness and complexity—how they work, the limits of what they can do, what they were designed to do, how they are actually used.
The societal impacts of technological change can be seen in many domains, from messenger RNA vaccines and automation to drones and climate change. The pace of that technological change can affect its impact, and how quickly a technology improves in performance can be an indicator of its future importance.
by Simon Pratico September 5, 2020. To understand science, technology and society one must read through theorists work and examine the evolution in both science and technology and how it coincides with society. While reading works by Karl Popper, Thomas Kuhn and David Nye it is evident that both science and technology are always changing.
Technology may have had an effect on social behavior due to the online environment, and. to-face communi. ation (Diamanduros, Downs &Jenkins, 2008). People seem to be less politica. ly correct when they are online and are notdealing directly with people (Diamanduros et al.
As with any improvement to human society, not everyone has equal access. Technology, in particular, often creates changes that lead to ever greater inequalities. In short, the gap gets wider faster. This technological stratification has led to a new focus on ensuring better access for all. There are two forms of technological stratification.
In a minute, we'll look ahead to the next decade in tech. Before we do, let's revisit this one. We asked three experts to pick what they see as the most significant ways tech has changed our lives ...
Technological determinism theorises the relationship between technological and social change that informs academic, policy and popular accounts about the place of technology in everyday life ...
by Mary Ann Liebert, Inc. Credit: Unsplash/CC0 Public Domain. A new study published in Cyberpsychology, Behavior and Social Networking introduces a novel hypothesis that could reshape our ...
The majority of human actions today are impacted by modern technologies. Different technical trends have emerged with the development of technology. Social media's growth, cloud computing, and mobile computing are a few examples. The world is becoming smarter thanks to the Internet of Things (IoT), a network of gadgets that may be connected ...
In developing our framework, we considered four important dimensions relevant to socioeconomic development: society, technology, business, and policy. These four dimensions represent independent or explanatory variables, which explain changes in the dependent or response variable, socioeconomic development. The framework is summarized in Figure ...
Describe the influence of media and technology on social change and modernization. We know that technology is one of the major factors that leads to social change. Let's take a deeper look at how technology and the media play a major role in the accelerated rate of globalization, which in turn, affects social change.
While it is clear that both influence each other, scholars debate over which has more influence. The first group called the technological determinists chose the side that technology has the most impact on society, and that society has little to no say as to how technologies evolve. The opposing side of social determinists believe that society ...
New hypothesis rethinks tech's impact on social interaction. July 10 2024. Credit: Unsplash/CC0 Public Domain. A new study published in Cyberpsychology, Behavior and Social Networking introduces a ...
The impact of technology on society is extensive. Karl Marx claimed that technology is necessary for the development of social relationships as well as mental concepts and attitudes. Karl Marx, Thorstein Veblen, and a few others believed that technology was the only factor contributing to social change (Ogburn, Citation 1922).
Abstract. Social relations, although basic to human nature, health and well-being, have become increasingly complicated as a result of changing population demography and technology. In this essay, we provide a historical overview of social relations, especially as they affect older people. We briefly review the evolution of theory and ...
European cohesion and social and political change.The social foundation of technology is a newly established interest area of sociol. gy and contributions have only recently been made. Increasing interest and research in information technology—and its euphoric assumptions—.
Having near constant access to technology and internet might affect teens' cognitive development. The constant access to the internet might hinder teens' memory abilities or the ability to engage in effortful thinking (Nasi & Koivusilta, 2012).Research indicates that near-constant access to the internet influences the kind of information people choose to remember (Sparrow et al., 2011).
4 Comparative Hypotheses for Technology Analysis. tire cords, telephone versus telegraph usage, etc. Overall, then, a predator-prey interaction has. an emerging technology in the role of predator ...
Summary. Technology and society are mutually dependent: technology is shaped by the society it shapes in turn. This chapter is structured around the idea of a joint structuring of technology and society, in tune with the chosen anthropotechnical perspective. It approaches technological change from the perspective of a set of societal dimensions ...
ECHNOLOGY changes society by. changing our environment to which. we, in turn, adapt. This change is usu- ally in the material environment, and. the adjustment we make to the changes often modifies customs and social insti-. tutions. Everywhere there is evidence of the stimulus of war in developing. an unprecedented number of inventions.
The technological development affects societies at a very large scale as technology is the tools and services by which one can make and take charge of the situation and the surroundings.. The technological revolution affected society at a very larger extent and also impacted the green revolution.; The change in the food production and the impact it had on the riding population.
Development: Amid the pursuit of technological prowess, we can seek to push the boundaries of prosocial AI capabilities, while positioning safety and ethical considerations as prime concerns ...
My hypothesis is that changes in technology generally lead to a profound impact on society by modifying how individuals interact, work, and live, ultimately influencing societal structures and population dynamics. An illustrative example can be drawn from advancements in agricultural technology, which have directly affected food production.
My hypothesis is that changes in technology have significant impacts on society, including changes in social behavior, economic activity, and cultural practices. One example of this is the impact of technological advances on food production, which has had a profound effect on global population growth. In the past, food production was limited by ...
The advancement of technology has a significant impact on society because it provides people with the means to control their environment and make decisions about their surroundings.. Which development in technology has had the biggest impact on life in this nation? The development of the Internet has had a significant impact on both American life and that of the rest of the globe.