What Is Learning? Essay about Learning Importance
- To find inspiration for your paper and overcome writer’s block
- As a source of information (ensure proper referencing)
- As a template for you assignment
What Is learning? 👨🎓️ Why is learning important? Find the answers here! 🔤 This essay on learning describes its outcomes and importance in one’s life.
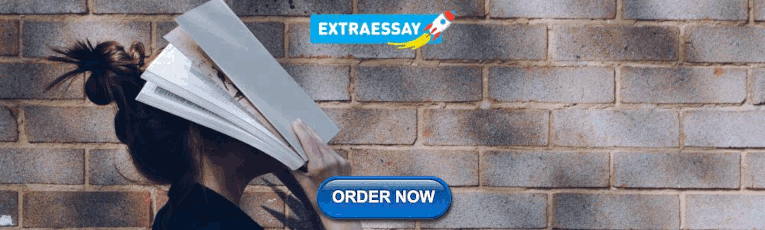
Introduction
- The Key Concepts
Learning is a continuous process that involves the transformation of information and experience into abilities and knowledge. Learning, according to me, is a two way process that involves the learner and the educator leading to knowledge acquisition as well as capability.
It informs my educational sector by making sure that both the students and the teacher participate during the learning process to make it more real and enjoyable so that the learners can clearly understand. There are many and different learning concepts held by students and ways in which the different views affect teaching and learning.
What Is Learning? The Key Concepts
One of the learning concept held by students is, presentation of learning material that is precise. This means that any material that is meant for learning should be very clear put in a language that the learners comprehend (Blackman & Benson 2003). The material should also be detailed with many examples that are relevant to the prior knowledge of the learner.
This means that the learner must have pertinent prior knowledge. This can be obtained by the teacher explaining new ideas and words that are to be encountered in a certain field or topic that might take more consecutive lessons. Different examples assist the students in approaching ideas in many perspectives.
The learner is able to get similarities from the many examples given thus leading to a better understanding of a concept since the ideas are related and linked.
Secondly, new meanings should be incorporated into the students’ prior knowledge, instead of remembering only the definitions or procedures. Therefore, to promote expressive learning, instructional methods that relate new information to the learner’s prior knowledge should be used.
Moreover, significant learning involves the use of evaluation methods that inspire learners to relate their existing knowledge with new ideas. For the students to comprehend complex ideas, they must be combined with the simple ideas they know.
Teaching becomes very easy when a lesson starts with simple concepts that the students are familiar with. The students should start by understanding what they know so that they can use the ideas in comprehending complex concepts. This makes learning smooth and easy for both the learner and the educator (Chermak& Weiss 1999).
Thirdly, acquisition of the basic concepts is very essential for the student to understand the threshold concepts. This is because; the basic concepts act as a foundation in learning a certain topic or procedure. So, the basic concepts must be comprehended first before proceeding to the incorporation of the threshold concepts.
This makes the student to have a clear understanding of each stage due to the possession of initial knowledge (Felder &Brent 1996). A deeper foundation of the study may also be achieved through getting the differences between various concepts clearly and by knowing the necessary as well as the unnecessary aspects. Basic concepts are normally taught in the lower classes of each level.
They include defining terms in each discipline. These terms aid in teaching in all the levels because they act as a foundation. The stage of acquiring the basics determines the students’ success in the rest of their studies.
This is because lack of basics leads to failure since the students can not understand the rest of the context in that discipline, which depends mostly on the basics. For learning to become effective to the students, the basics must be well understood as well as their applications.
Learning by use of models to explain certain procedures or ideas in a certain discipline is also another learning concept held by students. Models are helpful in explaining complex procedures and they assist the students in understanding better (Blackman & Benson 2003).
For instance, in economics, there are many models that are used by the students so that they can comprehend the essential interrelationships in that discipline. A model known as comparative static is used by the students who do economics to understand how equilibrium is used in economic reason as well as the forces that bring back equilibrium after it has been moved.
The students must know the importance of using such kind of models, the main aspect in the model and its relationship with the visual representation. A model is one of the important devices that must be used by a learner to acquire knowledge. They are mainly presented in a diagram form using symbols or arrows.
It simplifies teaching especially to the slow learners who get the concept slowly but clearly. It is the easiest and most effective method of learning complex procedures or directions. Most models are in form of flowcharts.
Learners should get used to learning incomplete ideas so that they can make more complete ideas available to them and enjoy going ahead. This is because, in the process of acquiring the threshold concepts, the prior knowledge acquired previously might be transformed.
So, the students must be ready to admit that every stage in the learning process they get an understanding that is temporary. This problem intensifies when the understanding of an idea acquired currently changes the understanding of an idea that had been taught previously.
This leads to confusion that can make the weak students lose hope. That is why the teacher should always state clear similarities as well as differences of various concepts. On the other hand, the student should be able to compare different concepts and stating their similarities as well as differences (Watkins & Regmy 1992).
The student should also be careful when dealing with concepts that seem similar and must always be attentive to get the first hand information from the teacher. Teaching and learning becomes very hard when learners do not concentrate by paying attention to what the teacher is explaining. For the serious students, learning becomes enjoyable and they do not get confused.
According to Chemkar and Weiss (1999), learners must not just sit down and listen, but they must involve themselves in some other activities such as reading, writing, discussing or solving problems. Basically, they must be very active and concentrate on what they are doing. These techniques are very essential because they have a great impact to the learners.
Students always support learning that is active than the traditional lecture methods because they master the content well and aids in the development of most skills such as writing and reading. So methods that enhance active learning motivate the learners since they also get more information from their fellow learners through discussions.
Students engage themselves in discussion groups or class presentations to break the monotony of lecture method of learning. Learning is a two way process and so both the teacher and the student must be involved.
Active learning removes boredom in the class and the students get so much involved thus improving understanding. This arouses the mind of the student leading to more concentration. During a lecture, the student should write down some of the important points that can later be expounded on.
Involvement in challenging tasks by the learners is so much important. The task should not be very difficult but rather it should just be slightly above the learner’s level of mastery. This makes the learner to get motivated and instills confidence. It leads to success of the learner due to the self confidence that aids in problem solving.
For instance, when a learner tackles a question that deemed hard and gets the answer correct, it becomes the best kind of encouragement ever. The learner gets the confidence that he can make it and this motivates him to achieve even more.
This kind of encouragement mostly occurs to the quick learners because the slow learners fail in most cases. This makes the slow learners fear tackling many problems. So, the concept might not apply to all the learners but for the slow learners who are determined, they can always seek for help incase of such a problem.
Moreover, another concept held by students is repetition because, the most essential factor in learning is efficient time in a task. For a student to study well he or she should consider repetition, that is, looking at the same material over and over again.
For instance, before a teacher comes for the lesson, the student can review notes and then review the same notes after the teacher gets out of class. So, the student reviews the notes many times thus improving the understanding level (Felder & Brent 1996). This simplifies revising for an exam because the student does not need to cram for it.
Reviewing the same material makes teaching very easy since the teacher does not need to go back to the previous material and start explaining again. It becomes very hard for those students who do not review their work at all because they do not understand the teacher well and are faced by a hard time when preparing for examinations.
Basically, learning requires quite enough time so that it can be effective. It also becomes a very big problem for those who do not sacrifice their time in reviews.
Acquisition of the main points improves understanding of the material to the student. Everything that is learnt or taught may not be of importance. Therefore, the student must be very keen to identify the main points when learning. These points should be written down or underlined because they become useful when reviewing notes before doing an exam. It helps in saving time and leads to success.
For those students who do not pay attention, it becomes very difficult for them to highlight the main points. They read for the sake of it and make the teacher undergo a very hard time during teaching. To overcome this problem, the students must be taught how to study so that learning can be effective.
Cooperative learning is also another concept held by the students. It is more detailed than a group work because when used properly, it leads to remarkable results. This is very encouraging in teaching and the learning environment as well.
The students should not work with their friends so that learning can be productive, instead every group should have at least one top level student who can assist the weak students. The groups assist them in achieving academic as well as social abilities due to the interaction. This learning concept benefits the students more because, a fellow student can explain a concept in a better way than how the teacher can explain in class.
Assignments are then given to these groups through a selected group leader (Felder& Brent 1996). Every member must be active in contributing ideas and respect of one’s ideas is necessary. It becomes very easy for the teacher to mark such kind of assignments since they are fewer than marking for each individual.
Learning becomes enjoyable because every student is given a chance to express his or her ideas freely and in a constructive manner. Teaching is also easier because the students encounter very many new ideas during the discussions. Some students deem it as time wastage but it is necessary in every discipline.
Every group member should be given a chance to become the group’s facilitator whose work is to distribute and collect assignments. Dormant students are forced to become active because every group member must contribute his or her points. Cooperative learning is a concept that requires proper planning and organization.
Completion of assignments is another student held learning concept. Its main aim is to assist the student in knowing whether the main concepts in a certain topic were understood. This acts as a kind of self evaluation to the student and also assists the teacher to know whether the students understood a certain topic. The assignments must be submitted to the respective teacher for marking.
Those students who are focused follow the teacher after the assignments have been marked for clarification purposes. This enhances learning and the student understands better. Many students differ with this idea because they do not like relating with the teacher (Marton &Beaty 1993). This leads to very poor grades since communication is a very essential factor in learning.
Teaching becomes easier and enjoyable when there is a student- teacher relationship. Assignment corrections are necessary to both the student and the teacher since the student comprehends the right method of solving a certain problem that he or she could not before.
Lazy students who do not do corrections make teaching hard for the teacher because they make the other students to lag behind. Learning may also become ineffective for them due to low levels of understanding.
Acquisition of facts is still another student held concept that aims at understanding reality. Students capture the essential facts so that they can understand how they suit in another context. Many students fail to obtain the facts because they think that they can get everything taught in class or read from books.
When studying, the student must clearly understand the topic so that he or she can develop a theme. This helps in making short notes by eliminating unnecessary information. So, the facts must always be identified and well understood in order to apply them where necessary. Teaching becomes easier when the facts are well comprehended by the students because it enhances effective learning.
Effective learning occurs when a student possesses strong emotions. A strong memory that lasts for long is linked with the emotional condition of the learner. This means that the learners will always remember well when learning is incorporated with strong emotions. Emotions develop when the students have a positive attitude towards learning (Marton& Beaty 1993).
This is because they will find learning enjoyable and exciting unlike those with a negative attitude who will find learning boring and of no use to them. Emotions affect teaching since a teacher will like to teach those students with a positive attitude towards what he is teaching rather than those with a negative attitude.
The positive attitude leads to effective learning because the students get interested in what they are learning and eventually leads to success. Learning does not become effective where students portray a negative attitude since they are not interested thus leading to failure.
Furthermore, learning through hearing is another student held concept. This concept enables them to understand what they hear thus calling for more attention and concentration. They prefer instructions that are given orally and are very keen but they also participate by speaking. Teaching becomes very enjoyable since the students contribute a lot through talking and interviewing.
Learning occurs effectively because the students involve themselves in oral reading as well as listening to recorded information. In this concept, learning is mostly enhanced by debating, presenting reports orally and interviewing people. Those students who do not prefer this concept as a method of learning do not involve themselves in debates or oral discussions but use other learning concepts.
Learners may also use the concept of seeing to understand better. This makes them remember what they saw and most of them prefer using written materials (Van Rosum & Schenk 1984). Unlike the auditory learners who grasp the concept through hearing, visual learners understand better by seeing.
They use their sight to learn and do it quietly. They prefer watching things like videos and learn from what they see. Learning occurs effectively since the memory is usually connected with visual images. Teaching becomes very easy when visual images are incorporated. They include such things like pictures, objects, graphs.
A teacher can use charts during instruction thus improving the students’ understanding level or present a demonstration for the students to see. Diagrams are also necessary because most students learn through seeing.
Use of visual images makes learning to look real and the student gets the concept better than those who learn through imaginations. This concept makes the students to use text that has got many pictures, diagrams, graphics, maps and graphs.
In learning students may also use the tactile concept whereby they gain knowledge and skills through touching. They gain knowledge mostly through manipulative. Teaching becomes more effective when students are left to handle equipments for themselves for instance in a laboratory practical. Students tend to understand better because they are able to follow instructions (Watkins & Regmy 1992).
After applying this concept, the students are able to engage themselves in making perfect drawings, making models and following procedures to make something. Learning may not take place effectively to those students who do not like manipulating because it arouses the memory and the students comprehends the concept in a better way.
Learning through analysis is also another concept held by students because they are able to plan their work in an organized manner which is based on logic ideas only. It requires individual learning and effective learning occurs when information is given in steps. This makes the teacher to structure the lessons properly and the goals should be clear.
This method of organizing ideas makes learning to become effective thus leading to success and achievement of the objectives. Analysis improves understanding of concepts to the learners (Watkins & Regmy 1992). They also understand certain procedures used in various topics because they are sequential.
Teaching and learning becomes very hard for those students who do not know how to analyze their work. Such students learn in a haphazard way thus leading to failure.
If all the learning concepts held by students are incorporated, then remarkable results can be obtained. A lot information and knowledge can be obtained through learning as long as the learner uses the best concepts for learning. Learners are also different because there are those who understand better by seeing while others understand through listening or touching.
So, it is necessary for each learner to understand the best concept to use in order to improve the understanding level. For the slow learners, extra time should be taken while studying and explanations must be clear to avoid confusion. There are also those who follow written instructions better than those instructions that are given orally. Basically, learners are not the same and so require different techniques.
Reference List
Benson, A., & Blackman, D., 2003. Can research methods ever be interesting? Active Learning in Higher Education, Vol. 4, No. 1, 39-55.
Chermak, S., & Weiss, A., 1999. Activity-based learning of statistics: Using practical applications to improve students’ learning. Journal of Criminal Justice Education , Vol. 10, No. 2, pp. 361-371.
Felder, R., & Brent, R., 1996. Navigating the bumpy road to student-centered instruction. College Teaching , Vol. 44, No. 2, pp. 43-47.
Marton, F. & Beaty, E., 1993. Conceptions of learning. International Journal of Educational Research , Vol. 19, pp. 277-300.
Van Rossum, E., & Schenk, S., 1984. The relationship between learning conception, study strategy and learning outcome. British Journal of Educational Psychology , Vol. 54, No.1, pp. 73-85.
Watkins, D., & Regmy, M., 1992. How universal are student conceptions of learning? A Nepalese investigation. Psychologia , Vol. 25, No. 2, pp. 101-110.
What Is Learning? FAQ
- Why Is Learning Important? Learning means gaining new knowledge, skills, and values, both in a group or on one’s own. It helps a person to develop, maintain their interest in life, and adapt to changes.
- Why Is Online Learning Good? Online learning has a number of advantages over traditional learning. First, it allows you to collaborate with top experts in your area of interest, no matter where you are located geographically. Secondly, it encourages independence and helps you develop time management skills. Last but not least, it saves time on transport.
- How to Overcome Challenges in Online Learning? The most challenging aspects of distant learning are the lack of face-to-face communication and the lack of feedback. The key to overcoming these challenges is effective communication with teachers and classmates through videoconferencing, email, and chats.
- The Benefits and Issues in Bilingual Education
- Benefits of Online College
- Apple’s iBook Using in Schools
- Students in School: Importance of Assessment Essay
- Instructional Plan in Writing for Learners With Disabilities
- Concept of Learning Geometry in School
- Distance Learning OL and Interactive Video in Higher Education
- Taxonomy of Learning Objectives
- Importance of social interaction to learning
- Comparing learning theories
- Chicago (A-D)
- Chicago (N-B)
IvyPanda. (2019, May 2). What Is Learning? Essay about Learning Importance. https://ivypanda.com/essays/what-is-learning-essay/
"What Is Learning? Essay about Learning Importance." IvyPanda , 2 May 2019, ivypanda.com/essays/what-is-learning-essay/.
IvyPanda . (2019) 'What Is Learning? Essay about Learning Importance'. 2 May.
IvyPanda . 2019. "What Is Learning? Essay about Learning Importance." May 2, 2019. https://ivypanda.com/essays/what-is-learning-essay/.
1. IvyPanda . "What Is Learning? Essay about Learning Importance." May 2, 2019. https://ivypanda.com/essays/what-is-learning-essay/.
Bibliography
IvyPanda . "What Is Learning? Essay about Learning Importance." May 2, 2019. https://ivypanda.com/essays/what-is-learning-essay/.
How Effective Is Online Learning? What the Research Does and Doesn’t Tell Us

- Share article
Editor’s Note: This is part of a series on the practical takeaways from research.
The times have dictated school closings and the rapid expansion of online education. Can online lessons replace in-school time?
Clearly online time cannot provide many of the informal social interactions students have at school, but how will online courses do in terms of moving student learning forward? Research to date gives us some clues and also points us to what we could be doing to support students who are most likely to struggle in the online setting.
The use of virtual courses among K-12 students has grown rapidly in recent years. Florida, for example, requires all high school students to take at least one online course. Online learning can take a number of different forms. Often people think of Massive Open Online Courses, or MOOCs, where thousands of students watch a video online and fill out questionnaires or take exams based on those lectures.
In the online setting, students may have more distractions and less oversight, which can reduce their motivation.
Most online courses, however, particularly those serving K-12 students, have a format much more similar to in-person courses. The teacher helps to run virtual discussion among the students, assigns homework, and follows up with individual students. Sometimes these courses are synchronous (teachers and students all meet at the same time) and sometimes they are asynchronous (non-concurrent). In both cases, the teacher is supposed to provide opportunities for students to engage thoughtfully with subject matter, and students, in most cases, are required to interact with each other virtually.
Coronavirus and Schools
Online courses provide opportunities for students. Students in a school that doesn’t offer statistics classes may be able to learn statistics with virtual lessons. If students fail algebra, they may be able to catch up during evenings or summer using online classes, and not disrupt their math trajectory at school. So, almost certainly, online classes sometimes benefit students.
In comparisons of online and in-person classes, however, online classes aren’t as effective as in-person classes for most students. Only a little research has assessed the effects of online lessons for elementary and high school students, and even less has used the “gold standard” method of comparing the results for students assigned randomly to online or in-person courses. Jessica Heppen and colleagues at the American Institutes for Research and the University of Chicago Consortium on School Research randomly assigned students who had failed second semester Algebra I to either face-to-face or online credit recovery courses over the summer. Students’ credit-recovery success rates and algebra test scores were lower in the online setting. Students assigned to the online option also rated their class as more difficult than did their peers assigned to the face-to-face option.
Most of the research on online courses for K-12 students has used large-scale administrative data, looking at otherwise similar students in the two settings. One of these studies, by June Ahn of New York University and Andrew McEachin of the RAND Corp., examined Ohio charter schools; I did another with colleagues looking at Florida public school coursework. Both studies found evidence that online coursetaking was less effective.
About this series

This essay is the fifth in a series that aims to put the pieces of research together so that education decisionmakers can evaluate which policies and practices to implement.
The conveners of this project—Susanna Loeb, the director of Brown University’s Annenberg Institute for School Reform, and Harvard education professor Heather Hill—have received grant support from the Annenberg Institute for this series.
To suggest other topics for this series or join in the conversation, use #EdResearchtoPractice on Twitter.
Read the full series here .
It is not surprising that in-person courses are, on average, more effective. Being in person with teachers and other students creates social pressures and benefits that can help motivate students to engage. Some students do as well in online courses as in in-person courses, some may actually do better, but, on average, students do worse in the online setting, and this is particularly true for students with weaker academic backgrounds.
Students who struggle in in-person classes are likely to struggle even more online. While the research on virtual schools in K-12 education doesn’t address these differences directly, a study of college students that I worked on with Stanford colleagues found very little difference in learning for high-performing students in the online and in-person settings. On the other hand, lower performing students performed meaningfully worse in online courses than in in-person courses.
But just because students who struggle in in-person classes are even more likely to struggle online doesn’t mean that’s inevitable. Online teachers will need to consider the needs of less-engaged students and work to engage them. Online courses might be made to work for these students on average, even if they have not in the past.
Just like in brick-and-mortar classrooms, online courses need a strong curriculum and strong pedagogical practices. Teachers need to understand what students know and what they don’t know, as well as how to help them learn new material. What is different in the online setting is that students may have more distractions and less oversight, which can reduce their motivation. The teacher will need to set norms for engagement—such as requiring students to regularly ask questions and respond to their peers—that are different than the norms in the in-person setting.
Online courses are generally not as effective as in-person classes, but they are certainly better than no classes. A substantial research base developed by Karl Alexander at Johns Hopkins University and many others shows that students, especially students with fewer resources at home, learn less when they are not in school. Right now, virtual courses are allowing students to access lessons and exercises and interact with teachers in ways that would have been impossible if an epidemic had closed schools even a decade or two earlier. So we may be skeptical of online learning, but it is also time to embrace and improve it.
A version of this article appeared in the April 01, 2020 edition of Education Week as How Effective Is Online Learning?
Sign Up for EdWeek Tech Leader
Edweek top school jobs.

Sign Up & Sign In


Essay on Importance of Learning
Students are often asked to write an essay on Importance of Learning in their schools and colleges. And if you’re also looking for the same, we have created 100-word, 250-word, and 500-word essays on the topic.
Let’s take a look…
100 Words Essay on Importance of Learning
The value of learning.
Learning is a crucial part of our lives. It helps us understand the world, make informed decisions, and grow as individuals.
Knowledge and Skills
Learning equips us with knowledge and skills. It makes us competent and confident, enabling us to face challenges and solve problems.
Personal Growth
Learning stimulates personal growth. It encourages curiosity and creativity, fostering a lifelong love for exploration and discovery.
Building Connections
Learning helps us connect with others. It promotes empathy and understanding, strengthening our relationships and communities.
In conclusion, learning is essential for our development, success, and happiness.
250 Words Essay on Importance of Learning
The power of learning.
Learning is an integral part of human existence. It is a process that starts at birth and continues throughout our lives, shaping our understanding of the world and our place within it. Learning is not confined to the acquisition of knowledge in a formal education setting. It extends to our daily interactions, experiences, and the continuous process of personal and professional development.
Learning and Personal Growth
Learning is the cornerstone of personal growth and self-improvement. It broadens our perspectives, fosters curiosity, and encourages us to question the status quo. Our ability to learn and adapt is what has allowed humankind to evolve and progress over time. Learning cultivates critical thinking skills, enabling us to analyze situations, solve problems, and make informed decisions.
The Socio-economic Impact of Learning
Learning also plays a significant role in socio-economic development. Education equips individuals with the skills needed to contribute to the workforce effectively, thereby driving economic growth. Moreover, it promotes social cohesion by fostering a shared understanding of societal values and norms.
Learning and Technological Advancements
In the era of rapid technological advancements, the importance of learning cannot be overstated. With the advent of AI and automation, the job market is in a constant state of flux, and the ability to learn new skills is more crucial than ever. Lifelong learning is now a necessity, not a luxury.
In conclusion, learning is a powerful tool that not only enriches our personal lives but also contributes to societal progress. It is the key to unlocking our potential and adapting to the ever-changing world around us.
500 Words Essay on Importance of Learning
The essence of learning, personal growth and development.
Learning is essential for personal growth and development. It equips us with the knowledge and skills necessary to navigate life effectively. Through learning, we acquire the ability to think critically, solve problems, and make informed decisions. It fosters creativity and innovation, enabling us to generate new ideas and solutions. Learning also facilitates emotional growth, helping us understand ourselves better, manage our emotions, and build strong interpersonal relationships.
Contributions to Society
On a societal level, learning plays a crucial role in driving progress and development. It is through learning that we gain an understanding of societal norms, values, and structures, enabling us to function effectively within our communities. Additionally, learning fosters social cohesion by promoting mutual understanding and respect among diverse groups. It equips us with the tools to challenge societal injustices and contribute to social change.
Global Impact
The role of lifelong learning.
In an era marked by rapid technological advancement and societal change, lifelong learning has become increasingly important. It enables us to stay relevant and competitive in the evolving job market, adapt to technological advancements, and navigate societal changes effectively. Lifelong learning fosters resilience, adaptability, and a growth mindset, qualities that are crucial for success in the 21st century.
In conclusion, learning is a vital aspect of human life that shapes our personal growth, contributes to societal development, and drives global progress. It equips us with the knowledge, skills, and values necessary to navigate life effectively, foster social cohesion, and address global challenges. In the face of rapid technological and societal change, lifelong learning has emerged as a critical component of learning, enabling us to adapt and thrive in the evolving world. Therefore, it is essential that we embrace learning as a lifelong pursuit, striving to expand our knowledge, enhance our skills, and deepen our understanding of the world around us.
Apart from these, you can look at all the essays by clicking here .
Happy studying!
Leave a Reply Cancel reply

How People Learn: Brain, Mind, Experience, and School: Expanded Edition (2000)
Chapter: 10 conclusions, 10 conclusions.
The pace at which science proceeds sometimes seems alarmingly slow, and impatience and hopes both run high when discussions turn to issues of learning and education. In the field of learning, the past quarter century has been a period of major research advances. Because of the many new developments, the studies that resulted in this volume were conducted to appraise the scientific knowledge base on human learning and its application to education. We evaluated the best and most current scientific data on learning, teaching, and learning environments. The objective of the analysis was to ascertain what is required for learners to reach deep understanding, to determine what leads to effective teaching, and to evaluate the conditions that lead to supportive environments for teaching and learning.
A scientific understanding of learning includes understanding about learning processes, learning environments, teaching, sociocultural processes, and the many other factors that contribute to learning. Research on all of these topics, both in the field and in laboratories, provides the fundamental knowledge base for understanding and implementing changes in education.
This volume discusses research in six areas that are relevant to a deeper understanding of students’ learning processes: the role of prior knowledge in learning, plasticity and related issues of early experience upon brain development, learning as an active process, learning for understanding, adaptive expertise, and learning as a time-consuming endeavor. It reviews research in five additional areas that are relevant to teaching and environments that support effective learning: the importance of social and cultural contexts, transfer and the conditions for wide application of learning, subject matter uniqueness, assessment to support learning, and the new educational technologies.
LEARNERS AND LEARNING
Development and learning competencies.
Children are born with certain biological capacities for learning. They can recognize human sounds; can distinguish animate from inanimate objects; and have an inherent sense of space, motion, number, and causality. These raw capacities of the human infant are actualized by the environment surrounding a newborn. The environment supplies information, and equally important, provides structure to the information, as when parents draw an infant’s attention to the sounds of her or his native language.
Thus, developmental processes involve interactions between children’s early competencies and their environmental and interpersonal supports. These supports serve to strengthen the capacities that are relevant to a child’s surroundings and to prune those that are not. Learning is promoted and regulated by the children’s biology and their environments. The brain of a developing child is a product, at the molecular level, of interactions between biological and ecological factors. Mind is created in this process.
The term “development” is critical to understanding the changes in children’s conceptual growth. Cognitive changes do not result from mere accretion of information, but are due to processes involved in conceptual reorganization. Research from many fields has supplied the key findings about how early cognitive abilities relate to learning. These include the following:
“Privileged domains:” Young children actively engage in making sense of their worlds. In some domains, most obviously language, but also for biological and physical causality and number, they seem predisposed to learn.
Children are ignorant but not stupid: Young children lack knowledge, but they do have abilities to reason with the knowledge they understand.
Children are problem solvers and, through curiosity, generate questions and problems: Children attempt to solve problems presented to them, and they also seek novel challenges. They persist because success and understanding are motivating in their own right.
Children develop knowledge of their own learning capacities— metacognition—very early. This metacognitive capacity gives them the ability to plan and monitor their success and to correct errors when necessary.
Children’ natural capabilities require assistance for learning: Children’s early capacities are dependent on catalysts and mediation. Adults play a critical role in promoting children’s curiosity and persistence by directing children’s attention, structuring their experiences, supporting their
learning attempts, and regulating the complexity and difficulty of levels of information for them.
Neurocognitive research has contributed evidence that both the developing and the mature brain are structurally altered during learning. For example, the weight and thickness of the cerebral cortex of rats is altered when they have direct contact with a stimulating physical environment and an interactive social group. The structure of the nerve cells themselves is correspondingly altered: under some conditions, both the cells that provide support to the neurons and the capillaries that supply blood to the nerve cells may be altered as well. Learning specific tasks appears to alter the specific regions of the brain appropriate to the task. In humans, for example, brain reorganization has been demonstrated in the language functions of deaf individuals, in rehabilitated stroke patients, and in the visual cortex of people who are blind from birth. These findings suggest that the brain is a dynamic organ, shaped to a great extent by experience and by what a living being does.
Transfer of Learning
A major goal of schooling is to prepare students for flexible adaptation to new problems and settings. Students’ abilities to transfer what they have learned to new situations provides an important index of adaptive, flexible learning; seeing how well they do this can help educators evaluate and improve their instruction. Many approaches to instruction look equivalent when the only measure of learning is memory for facts that were specifically presented. Instructional differences become more apparent when evaluated from the perspective of how well the learning transfers to new problems and settings. Transfer can be explored at a variety of levels, including transfer from one set of concepts to another, one school subject to another, one year of school to another, and across school and everyday, nonschool activities.
People’s abilitiy to transfer what they have learned depends upon a number of factors:
People must achieve a threshold of initial learning that is sufficient to support transfer. This obvious point is often overlooked and can lead to erroneous conclusions about the effectiveness of various instructional approaches. It takes time to learn complex subject matter, and assessments of transfer must take into account the degree to which original learning with understanding was accomplished.
Spending a lot of time (“time on task”) in and of itself is not sufficient to ensure effective learning. Practice and getting familiar with subject matter take time, but most important is how people use their time while
learning. Concepts such as “deliberate practice” emphasize the importance of helping students monitor their learning so that they seek feedback and actively evaluate their strategies and current levels of understanding. Such activities are very different from simply reading and rereading a text.
Learning with understanding is more likely to promote transfer than simply memorizing information from a text or a lecture. Many classroom activities stress the importance of memorization over learning with understanding. Many, as well, focus on facts and details rather than larger themes of causes and consequences of events. The shortfalls of these approaches are not apparent if the only test of learning involves tests of memory, but when the transfer of learning is measured, the advantages of learning with understanding are likely to be revealed.
Knowledge that is taught in a variety of contexts is more likely to support flexible transfer than knowledge that is taught in a single context. Information can become “context-bound” when taught with context-specific examples. When material is taught in multiple contexts, people are more likely to extract the relevant features of the concepts and develop a more flexible representation of knowledge that can be used more generally.
Students develop flexible understanding of when, where, why, and how to use their knowledge to solve new problems if they learn how to extract underlying themes and principles from their learning exercises. Understanding how and when to put knowledge to use—known as conditions of applicability—is an important characteristic of expertise. Learning in multiple contexts most likely affects this aspect of transfer.
Transfer of learning is an active process. Learning and transfer should not be evaluated by “one-shot” tests of transfer. An alternative assessment approach is to consider how learning affects subsequent learning, such as increased speed of learning in a new domain. Often, evidence for positive transfer does not appear until people have had a chance to learn about the new domain—and then transfer occurs and is evident in the learner’s ability to grasp the new information more quickly.
All learning involves transfer from previous experiences. Even initial learning involves transfer that is based on previous experiences and prior knowledge. Transfer is not simply something that may or may not appear after initial learning has occurred. For example, knowledge relevant to a particular task may not automatically be activated by learners and may not serve as a source of positive transfer for learning new information. Effective teachers attempt to support positive transfer by actively identifying the strengths that students bring to a learning situation and building on them, thereby building bridges between students’ knowledge and the learning objectives set out by the teacher.
Sometimes the knowledge that people bring to a new situation impedes subsequent learning because it guides thinking in wrong directions.
For example, young children’s knowledge of everyday counting-based arithmetic can make it difficult for them to deal with rational numbers (a larger number in the numerator of a fraction does not mean the same thing as a larger number in the denominator); assumptions based on everyday physical experiences can make it difficult for students to understand physics concepts (they think a rock falls faster than a leaf because everyday experiences include other variables, such as resistance, that are not present in the vacuum conditions that physicists study), and so forth. In these kinds of situations, teachers must help students change their original conceptions rather than simply use the misconceptions as a basis for further understanding or leaving new material unconnected to current understanding.
Competent and Expert Performance
Cognitive science research has helped us understand how learners develop a knowledge base as they learn. An individual moves from being a novice in a subject area toward developing competency in that area through a series of learning processes. An understanding of the structure of knowledge provides guidelines for ways to assist learners acquire a knowledge base effectively and efficiently. Eight factors affect the development of expertise and competent performance:
Relevant knowledge helps people organize information in ways that support their abilities to remember.
Learners do not always relate the knowledge they possess to new tasks, despite its potential relevance. This “disconnect” has important implications for understanding differences between usable knowledge (which is the kind of knowledge that experts have developed) and less-organized knowledge, which tends to remain “inert.”
Relevant knowledge helps people to go beyond the information given and to think in problem representations, to engage in the mental work of making inferences, and to relate various kinds of information for the purpose of drawing conclusions.
An important way that knowledge affects performances is through its influences on people’s representations of problems and situations. Different representations of the same problem can make it easy, difficult, or impossible to solve.
The sophisticated problem representations of experts are the result of well-organized knowledge structures. Experts know the conditions of applicability of their knowledge, and they are able to access the relevant knowledge with considerable ease.
Different domains of knowledge, such as science, mathematics, and history, have different organizing properties. It follows, therefore, that to
have an in-depth grasp of an area requires knowledge about both the content of the subject and the broader structural organization of the subject.
Competent learners and problem solvers monitor and regulate their own processing and change their strategies as necessary. They are able to make estimates and “educated guesses.”
The study of ordinary people under everyday cognition provides valuable information about competent cognitive performances in routine settings. Like the work of experts, everyday competencies are supported by sets of tools and social norms that allow people to perform tasks in specific contexts that they often cannot perform elsewhere.
Conclusions
Everyone has understanding, resources, and interests on which to build. Learning a topic does not begin from knowing nothing to learning that is based on entirely new information. Many kinds of learning require transforming existing understanding, especially when one’s understanding needs to be applied in new situations. Teachers have a critical role in assisting learners to engage their understanding, building on learners’ understandings, correcting misconceptions, and observing and engaging with learners during the processes of learning.
This view of the interactions of learners with one another and with teachers derives from generalizations about learning mechanisms and the conditions that promote understanding. It begins with the obvious: learning is embedded in many contexts. The most effective learning occurs when learners transport what they have learned to various and diverse new situations. This view of learning also includes the not so obvious: young learners arrive at school with prior knowledge that can facilitate or impede learning. The implications for schooling are many, not the least of which is that teachers must address the multiple levels of knowledge and perspectives of children’s prior knowledge, with all of its inaccuracies and misconceptions.
Effective comprehension and thinking require a coherent understanding of the organizing principles in any subject matter; understanding the essential features of the problems of various school subjects will lead to better reasoning and problem solving; early competencies are foundational to later complex learning; self-regulatory processes enable self-monitoring and control of learning processes by learners themselves.
Transfer and wide application of learning are most likely to occur when learners achieve an organized and coherent understanding of the material; when the situations for transfer share the structure of the original
learning; when the subject matter has been mastered and practiced; when subject domains overlap and share cognitive elements; when instruction includes specific attention to underlying principles; and when instruction explicitly and directly emphasizes transfer.
Learning and understanding can be facilitated in learners by emphasizing organized, coherent bodies of knowledge (in which specific facts and details are embedded), by helping learners learn how to transfer their learning, and by helping them use what they learn.
In-depth understanding requires detailed knowledge of the facts within a domain. The key attribute of expertise is a detailed and organized understanding of the important facts within a specific domain. Education needs to provide children with sufficient mastery of the details of particular subject matters so that they have a foundation for further exploration within those domains.
Expertise can be promoted in learners. The predominant indicator of expert status is the amount of time spent learning and working in a subject area to gain mastery of the content. Secondarily, the more one knows about a subject, the easier it is to learn additional knowledge.
TEACHERS AND TEACHING
The portrait we have sketched of human learning and cognition emphasizes learning for in-depth comprehension. The major ideas that have transformed understanding of learning also have implications for teaching.
Teaching for In-Depth Learning
Traditional education has tended to emphasize memorization and mastery of text. Research on the development of expertise, however, indicates that more than a set of general problem-solving skills or memory for an array of facts is necessary to achieve deep understanding. Expertise requires well-organized knowledge of concepts, principles, and procedures of inquiry. Various subject disciplines are organized differently and require an array of approaches to inquiry. We presented a discussion of the three subject areas of history, mathematics, and science learning to illustrate how the structure of the knowledge domain guides both learning and teaching.
Proponents of the new approaches to teaching engage students in a variety of different activities for constructing a knowledge base in the subject domain. Such approaches involve both a set of facts and clearly defined principles. The teacher’s goal is to develop students’ understanding of a given topic, as well as to help them develop into independent and thoughtful problem solvers. One way to do this is by showing students that they already have relevant knowledge. As students work through different prob-
lems that a teacher presents, they develop their understanding into principles that govern the topic.
In mathematics for younger (first- and second-grade) students, for example, cognitively guided instruction uses a variety of classroom activities to bring number and counting principles into students’ awareness, including snack-time sharing for fractions, lunch count for number, and attendance for part-whole relationships. Through these activities, a teacher has many opportunities to observe what students know and how they approach solutions to problems, to introduce common misconceptions to challenge students’ thinking, and to present more advanced discussions when the students are ready.
For older students, model-based reasoning in mathematics is an effective approach. Beginning with the building of physical models, this approach develops abstract symbol system-based models, such as algebraic equations or geometry-based solutions. Model-based approaches entail selecting and exploring the properties of a model and then applying the model to answer a question that interests the student. This important approach emphasizes understanding over routine memorization and provides students with a learning tool that enables them to figure out new solutions as old ones become obsolete.
These new approaches to mathematics operate from knowledge that learning involves extending understanding to new situations, a guiding principle of transfer ( Chapter 3 ); that young children come to school with early mathematics concepts ( Chapter 4 ); that learners cannot always identify and call up relevant knowledge (Chapters 2 , 3 , and 4 ); and that learning is promoted by encouraging children to try out the ideas and strategies they bring with them to school-based learning ( Chapter 6 ). Students in classes that use the new approaches do not begin learning mathematics by sitting at desks and only doing computational problems. Rather, they are encouraged to explore their own knowledge and to invent strategies for solving problems and to discuss with others why their strategies work or do not work.
A key aspect of the new ways of teaching science is to focus on helping students overcome deeply rooted misconceptions that interfere with learning. Especially in people’s knowledge of the physical, it is clear that prior knowledge, constructed out of personal experiences and observations— such as the conception that heavy objects fall faster than light objects—can conflict with new learning. Casual observations are useful for explaining why a rock falls faster than a leaf, but they can lead to misconceptions that are difficult to overcome. Misconceptions, however, are also the starting point for new approaches to teaching scientific thinking. By probing students’ beliefs and helping them develop ways to resolve conflicting views, teachers can guide students to construct coherent and broad understandings of scientific concepts. This and other new approaches are major break-
throughs in teaching science. Students can often answer fact-based questions on tests that imply understanding, but misconceptions will surface as the students are questioned about scientific concepts.
Chèche Konnen (“search for knowledge” in Haitian Creole) was presented as an example of new approaches to science learning for grade school children. The approach focuses upon students’ personal knowledge as the foundations of sense-making. Further, the approach emphasizes the role of the specialized functions of language, including the students’ own language for communication when it is other than English; the role of language in developing skills of how to “argue” the scientific “evidence” they arrive at; the role of dialogue in sharing information and learning from others; and finally, how the specialized, scientific language of the subject matter, including technical terms and definitions, promote deep understanding of the concepts.
Teaching history for depth of understanding has generated new approaches that recognize that students need to learn about the assumptions any historian makes for connecting events and schemes into a narrative. The process involves learning that any historical account is a history and not the history. A core concept guiding history learning is how to determine, from all of the events possible to enumerate, the ones to single out as significant. The “rules for determining historical significance” become a lightening rod for class discussions in one innovative approach to teaching history. Through this process, students learn to understand the interpretative nature of history and to understand history as an evidentiary form of knowledge. Such an approach runs counter to the image of history as clusters of fixed names and dates that students need to memorize. As with the Chèche Konnen example of science learning, mastering the concepts of historical analysis, developing an evidentiary base, and debating the evidence all become tools in the history toolbox that students carry with them to analyze and solve new problems.
Expert Teachers
Expert teachers know the structure of the knowledge in their disciplines. This knowledge provides them with cognitive roadmaps to guide the assignments they give students, the assessments they use to gauge student progress, and the questions they ask in the give-and-take of classroom life. Expert teachers are sensitive to the aspects of the subject matter that are especially difficult and easy for students to grasp: they know the conceptual barriers that are likely to hinder learning, so they watch for these tell-tale signs of students’ misconceptions. In this way, both students’ prior knowledge and teachers’ knowledge of subject content become critical components of learners’ growth.
Subject-matter expertise requires well-organized knowledge of concepts and inquiry procedures. Similarly, studies of teaching conclude that expertise consists of more than a set of general methods that can be applied across all subject matter. These two sets of research-based findings contradict the common misconception about what teachers need to know in order to design effective learning environments for students. Both subject-matter knowledge and pedagogical knowledge are important for expert teaching because knowledge domains have unique structures and methods of inquiry associated with them.
Accomplished teachers also assess their own effectiveness with their students. They reflect on what goes on in the classroom and modify their teaching plans accordingly. Thinking about teaching is not an abstract or esoteric activity. It is a disciplined, systematic approach to professional development. By reflecting on and evaluating one’s own practices, either alone or in the company of a critical colleague, teachers develop ways to change and improve their practices, like any other opportunity for learning with feedback.
Teachers need expertise in both subject matter content and in teaching.
Teachers need to develop understanding of the theories of knowledge (epistemologies) that guide the subject-matter disciplines in which they work.
Teachers need to develop an understanding of pedagogy as an intellectual discipline that reflects theories of learning, including knowledge of how cultural beliefs and the personal characteristics of learners influence learning.
Teachers are learners and the principles of learning and transfer for student learners apply to teachers.
Teachers need opportunities to learn about children’s cognitive development and children’s development of thought (children’s epistemologies) in order to know how teaching practices build on learners’ prior knowledge.
Teachers need to develop models of their own professional development that are based on lifelong learning, rather than on an “updating” model of learning, in order to have frameworks to guide their career planning.
LEARNING ENVIRONMENTS
Tools of technology.
Technology has become an important instrument in education. Computer-based technologies hold great promise both for increasing access to knowledge and as a means of promoting learning. The public imagination has been captured by the capacity of information technologies to centralize and organize large bodies of knowledge; people are excited by the prospect of information networks, such as the Internet, for linking students around the globe into communities of learners.
There are five ways that technology can be used to help meet the challenges of establishing effective learning environments:
Bringing real-world problems into classrooms through the use of videos, demonstrations, simulations, and Internet connections to concrete data and working scientists.
Providing “scaffolding” support to augment what learners can do and reason about on their path to understanding. Scaffolding allows learners to participate in complex cognitive performances, such as scientific visualization and model-based learning, that is more difficult or impossible without technical support.
Increasing opportunities for learners to receive feedback from software tutors, teachers, and peers; to engage in reflection on their own learning processes; and to receive guidance toward progressive revisions that improve their learning and reasoning.
Building local and global communities of teachers, administrators, students, parents, and other interested learners.
Expanding opportunities for teachers’ learning.
An important function of some of the new technologies is their use as tools of representation. Representational thinking is central to in-depth understanding and problem representation is one of the skills that distinguish subject experts from novices. Many of the tools also have the potential to provide multiple contexts and opportunities for learning and transfer, for both student-learners and teacher-learners. Technologies can be used as learning and problem-solving tools to promote both independent learning and collaborative networks of learners and practitioners.
The use of new technologies in classrooms, or the use of any learning aid for that matter, is never solely a technical matter. The new electronic technologies, like any other educational resource, are used in a social environment and are, therefore, mediated by the dialogues that students have with each other and the teacher.
Educational software needs to be developed and implemented with a full understanding of the principles of learning and developmental psychology. Many new issues arise when one considers how to educate teachers to use new technologies effectively: What do they need to know about learning processes? What do they need to know about the technologies? What kinds of training are most effective for helping teachers use high-quality instructional programs? Understanding the issues that affect teachers who will be using new technologies is just as pressing as questions of the learning potential and developmental appropriateness of the technologies for children.
Assessment to Support Learning
Assessment and feedback are crucial for helping people learn. Assessment that is consistent with principles of learning and understanding should:
Mirror good instruction.
Happen continuously, but not intrusively, as a part of instruction.
Provide information (to teachers, students, and parents) about the levels of understanding that students are reaching.
Assessment should reflect the quality of students’ thinking, as well as what specific content they have learned. For this purpose, achievement measurement must consider cognitive theories of performance. Frameworks that integrate cognition and context in assessing achievement in science, for example, describe performance in terms of the content and process task demands of the subject matter and the nature and extent of cognitive activities likely to be observed in a particular assessment situation. The frameworks provide a basis for examining performance assessments that are designed to measure reasoning, understanding, and complex problem solving.
The nature and purposes of an assessment also influence the specific cognitive activities that are expressed by the student. Some assessment tasks emphasize a particular performance, such as explanation, but deemphasize others, such as self-monitoring. The kind and quality of cognitive activities observed in an assessment situation are functions of the content and process demands of the tasks involved. Similarly, the task demands for process skills can be conceived along a continuum from constrained to open. In open situations, explicit directions are minimized in order to see how students generate and carry out appropriate process skills as they solve problems. Characterizing assessments in terms of components of competence and the content and process demands of the subject matter brings specificity to assessment objectives, such as “higher level thinking” and “deep understanding.” This approach links specific content with the
underlying cognitive processes and the performance objectives that the teacher has in mind. With articulated objectives and an understanding of the correspondence between task features and cognitive activities, the content and process demands of tasks are brought into alignment with the performance objectives.
Effective teachers see assessment opportunities in ongoing classroom learning situations. They continually attempt to learn about students’ thinking and understanding and make it relevant to current learning tasks. They do a great deal of on-line monitoring of both group work and individual performances, and they attempt to link current activities to other parts of the curriculum and to students’ daily life experiences.
Students at all levels, but increasingly so as they progress through the grades, focus their learning attention and energies on the parts of the curriculum that are assessed. In fact, the art of being a good student, at least in the sense of getting good grades, is tied to being able to anticipate what will be tested. This means that the information to be tested has the greatest influence on guiding students’ learning. If teachers stress the importance of understanding but then test for memory of facts and procedures, it is the latter that students will focus on. Many assessments developed by teachers overemphasize memory for procedures and facts; expert teachers, by contrast, align their assessment practices with their instructional goals of depth-of-understanding.
Learning and Connections to Community
Outside of formal school settings, children participate in many institutions that foster their learning. For some of these institutions, promoting learning is part of their goals, including after-school programs, as in such organizations as Boy and Girl Scout Associations and 4–H Clubs, museums, and religious education. In other institutions or activities, learning is more incidental, but learning takes place nevertheless. These learning experiences are fundamental to children’s—and adults’ —lives since they are embedded in the culture and the social structures that organize their daily activities. None of the following points about the importance of out-of-school learning institutions, however, should be taken to deemphasize the central role of schools and the kinds of information that can be most efficiently and effectively taught there.
A key environment for learning is the family. In the United States, many families hold a learning agenda for their children and seek opportunities for their children to engage with the skills, ideas, and information in their communities. Even when family members do not focus consciously on instructional roles, they provide resources for children’s learning that are relevant to school and out-of-school ideas through family activities, the funds of
knowledge available within extended families and their communities, and the attitudes that family members display toward the skills and values of schooling.
The success of the family as a learning environment, especially in the early years, has provided inspiration and guidance for some of the changes recommended in schools. The rapid development of children from birth to ages 4 or 5 is generally supported by family interactions in which children learn by observing and interacting with others in shared endeavors. Conversations and other interactions that occur around events of interest with trusted and skilled adults and child companions are especially powerful environments for learning. Many of the recommendations for changes in schools can be seen as extensions of the learning activities that occur within families. In addition, recommendations to include families in classroom activities and educational planning hold promise of bringing together two powerful systems for supporting children’s learning.
Classroom environments are positively influenced by opportunities to interact with parents and community members who take interest in what they are doing. Teachers and students more easily develop a sense of community as they prepare to discuss their projects with people who come from outside the school and its routines. Outsiders can help students appreciate similarities and differences between classroom environments and everyday environments; such experiences promote transfer of learning by illustrating the many contexts for applying what they know.
Parents and business leaders represent examples of outside people who can have a major impact on student learning. Broad-scale participation in school-based learning rarely happens by accident. It requires clear goals and schedules and relevant curricula that permit and guide adults in ways to help children learn.
Designing effective learning environments includes considering the goals for learning and goals for students. This comparison highlights the fact that there are various means for approaching goals of learning, and furthermore, that goals for students change over time. As goals and objectives have changed, so has the research base on effective learning and the tools that students use. Student populations have also shifted over the years. Given these many changes in student populations, tools of technology, and society’s requirements, different curricula have emerged along with needs for new pedagogical approaches that are more child-centered and more culturally sensitive, all with the objectives of promoting effective learning and adaptation (transfer). The requirement for teachers to meet such a diversity of challenges also illustrates why assessment needs to be a tool to help teach-
ers determine if they have achieved their objectives. Assessment can guide teachers in tailoring their instruction to individual students’ learning needs and, collaterally, inform parents of their children’s progress.
Supportive learning environments, which are the social and organizational structures in which students and teachers operate, need to focus on the characteristics of classroom environments that affect learning; the environments as created by teachers for learning and feedback; and the range of learning environments in which students participate, both in and out of school.
Classroom environments can be positively influenced by opportunities to interact with others who affect learners, particularly families and community members, around school-based learning goals.
New tools of technology have the potential of enhancing learning in many ways. The tools of technology are creating new learning environments, which need to be assessed carefully, including how their use can facilitate learning, the types of assistance that teachers need in order to incorporate the tools into their classroom practices, the changes in classroom organization that are necessary for using technologies, and the cognitive, social, and learning consequences of using these new tools.
First released in the Spring of 1999, How People Learn has been expanded to show how the theories and insights from the original book can translate into actions and practice, now making a real connection between classroom activities and learning behavior. This edition includes far-reaching suggestions for research that could increase the impact that classroom teaching has on actual learning.
Like the original edition, this book offers exciting new research about the mind and the brain that provides answers to a number of compelling questions. When do infants begin to learn? How do experts learn and how is this different from non-experts? What can teachers and schools do-with curricula, classroom settings, and teaching methods—to help children learn most effectively? New evidence from many branches of science has significantly added to our understanding of what it means to know, from the neural processes that occur during learning to the influence of culture on what people see and absorb.
How People Learn examines these findings and their implications for what we teach, how we teach it, and how we assess what our children learn. The book uses exemplary teaching to illustrate how approaches based on what we now know result in in-depth learning. This new knowledge calls into question concepts and practices firmly entrenched in our current education system.
Topics include:
- How learning actually changes the physical structure of the brain.
- How existing knowledge affects what people notice and how they learn.
- What the thought processes of experts tell us about how to teach.
- The amazing learning potential of infants.
- The relationship of classroom learning and everyday settings of community and workplace.
- Learning needs and opportunities for teachers.
- A realistic look at the role of technology in education.
READ FREE ONLINE
Welcome to OpenBook!
You're looking at OpenBook, NAP.edu's online reading room since 1999. Based on feedback from you, our users, we've made some improvements that make it easier than ever to read thousands of publications on our website.
Do you want to take a quick tour of the OpenBook's features?
Show this book's table of contents , where you can jump to any chapter by name.
...or use these buttons to go back to the previous chapter or skip to the next one.
Jump up to the previous page or down to the next one. Also, you can type in a page number and press Enter to go directly to that page in the book.
Switch between the Original Pages , where you can read the report as it appeared in print, and Text Pages for the web version, where you can highlight and search the text.
To search the entire text of this book, type in your search term here and press Enter .
Share a link to this book page on your preferred social network or via email.
View our suggested citation for this chapter.
Ready to take your reading offline? Click here to buy this book in print or download it as a free PDF, if available.
Get Email Updates
Do you enjoy reading reports from the Academies online for free ? Sign up for email notifications and we'll let you know about new publications in your areas of interest when they're released.
Learning Strategies That Work
Dr. Mark A. McDaniel shares effective, evidence-based strategies about learning to replace less effective but widely accepted practices.
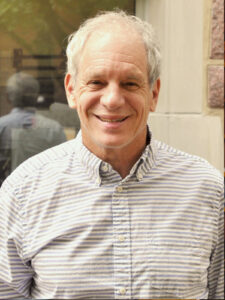
How do we learn and absorb new information? Which learning strategies actually work and which are mere myths?
Such questions are at the center of the work of Mark McDaniel , professor of psychology and the director of the Center for Integrative Research on Cognition, Learning, and Education at Washington University in St. Louis. McDaniel coauthored the book Make it Stick: The Science of Successful Learning .
In this Q&A adapted from a Career & Academic Resource Center podcast episode , McDaniel discusses his research on human learning and memory, including the most effective strategies for learning throughout a lifetime.
Harvard Extension: In your book, you talk about strategies to help students be better learners in and outside of the classroom. You write, “We harbor deep convictions that we learn better through single-minded focus and dogged repetition. And these beliefs are validated time and again by the visible improvement that comes during practice, practice, practice.”
McDaniel: This judgment that repetition is effective is hard to shake. There are cues present that your brain picks up when you’re rereading, when you’re repeating something that give you the metacognitive, that is your judgment about your own cognition, give you the misimpression that you really have learned this stuff well.
Older learners shouldn’t feel that they’re at a definitive disadvantage, because they’re not. Older learners really want to try to leverage their prior knowledge and use that as a basis to structure and frame and understand new information coming in.
And two of the primary cues are familiarity. So as you keep rereading, the material becomes more familiar to you. And we mistakenly judge familiarity as meaning robust learning.
And the second cue is fluency. It’s very clear from much work in reading and cognitive processes during reading that when you reread something at every level, the processes are more fluent. Word identification is more fluent. Parsing the structure of the sentence is more fluent. Extracting the ideas is more fluent. Everything is more fluent. And we misinterpret these fluency cues that the brain is getting. And these are accurate cues. It is more fluent. But we misinterpret that as meaning, I’ve really got this. I’ve really learned this. I’m not going to forget this. And that’s really misleading.
So let me give you another example. It’s not just rereading. It’s situations in, say, the STEM fields or any place where you’ve got to learn how to solve certain kinds of problems. One of the standard ways that instructors present homework is to present the same kind of problem in block fashion. You may have encountered this in your own math courses, your own physics courses.
So for example, in a physics course, you might get a particular type of work problem. And the parameters on it, the numbers might change, but in your homework, you’re trying to solve two or three or four of these work problems in a row. Well, it gets more and more fluid because exactly what formula you have to use. You know exactly what the problem is about. And as you get more fluid, and as we say in the book, it looks like you’re getting better. You are getting better at these problems.
But the issue is that can you remember how to identify which kinds of problems go with which kinds of solutions a week later when you’re asked to do a test where you have all different kinds of problems? And the answer is no, you cannot when you’ve done this block practice. So even though instructors who feel like their students are doing great with block practice and students will feel like they’re doing great, they are doing great on that kind of block practice, but they’re not at all good now at retaining information about what distinguishing features or problems are signaling certain kinds of approaches.
What you want to do is interleave practice in these problems. You want to randomly have a problem of one type and then solve a problem of another type and then a problem of another type. And in doing that, it feels difficult and it doesn’t feel fluent. And the signals to your brain are, I’m not getting this. I’m not doing very well. But in fact, that effort to try to figure out what kinds of approaches do I need for each problem as I encounter a different kind of problem, that’s producing learning. That’s producing robust skills that stick with you.
So this is a seductive thing that we have to, instructors and students alike, have to understand and have to move beyond those initial judgments, I haven’t learned very much, and trust that the more difficult practice schedule really is the better learning.
And I’ve written more on this since Make It Stick . And one of my strong theoretical tenets now is that in order for students to really embrace these techniques, they have to believe that they work for them. Each student has to believe it works for them. So I prepare demonstrations to show students these techniques work for them.
The net result of adopting these strategies is that students aren’t spending more time. Instead they’re spending more effective time. They’re working better. They’re working smarter.
When students take an exam after doing lots of retrieval practice, they see how well they’ve done. The classroom becomes very exciting. There’s lots of buy-in from the students. There’s lots of energy. There’s lots of stimulation to want to do more of this retrieval practice, more of this difficulty. Because trying to retrieve information is a lot more difficult than rereading it. But it produces robust learning for a number of reasons.
I think students have to trust that these techniques, and I think they also have to observe that these techniques work for them. It’s creating better learning. And then as a learner, you are more motivated to replace these ineffective techniques with more effective techniques.
Harvard Extension: You talk about tips for learners , how to make it stick. And there are several methods or tips that you share: elaboration, generation, reflection, calibration, among others. Which of these techniques is best?
McDaniel: It depends on the learning challenges that are faced. So retrieval practice, which is practicing trying to recall information from memory is really super effective if the requirements of your course require you to reproduce factual information.
For other things, it may be that you want to try something like generating understanding, creating mental models. So if your exams require you to draw inferences and work with new kinds of problems that are illustrative of the principles, but they’re new problems you haven’t seen before, a good technique is to try to connect the information into what I would call mental models. This is your representation of how the parts and the aspects fit together, relate together.
It’s not that one technique is better than the other. It’s that different techniques produce certain kinds of outcomes. And depending on the outcome you want, you might select one technique or the other.
I really firmly believe that to the extent that you can make learning fun and to the extent that one technique really seems more fun to you, that may be your go to technique. I teach a learning strategy course and I make it very clear to students. You don’t need to use all of these techniques. Find a couple that really work for you and then put those in your toolbox and replace rereading with these techniques.
Harvard Extension: You reference lifelong learning and lifelong learners. You talk about the brain being plastic, mutability of the brain in some ways, and give examples of how some lifelong learners approach their learning.
McDaniel: In some sense, more mature learners, older learners, have an advantage because they have more knowledge. And part of learning involves relating new information that’s coming into your prior knowledge, relating it to your knowledge structures, relating it to your schemas for how you think about certain kinds of content.
And so older adults have the advantage of having this richer knowledge base with which they can try to integrate new material. So older learners shouldn’t feel that they’re at a definitive disadvantage, because they’re not. Older learners really want to try to leverage their prior knowledge and use that as a basis to structure and frame and understand new information coming in.
Our challenges as older learners is that we do have these habits of learning that are not very effective. We turn to these habits. And if these aren’t such effective habits, we maybe attribute our failures to learn to age or a lack of native ability or so on and so forth. And in fact, that’s not it at all. In fact, if you adopt more effective strategies at any age, you’re going to find that your learning is more robust, it’s more successful, it falls into place.
You can learn these strategies at any age. Successful lifelong learning is getting these effective strategies in place, trusting them, and having them become a habit for how you’re going to approach your learning challenges.
6 Benefits of Connecting with an Enrollment Coach
Thinking about pursuing a degree or certificate at Harvard Extension School? Learn more about how working with an enrollment coach will get you off to a great start.
Harvard Division of Continuing Education
The Division of Continuing Education (DCE) at Harvard University is dedicated to bringing rigorous academics and innovative teaching capabilities to those seeking to improve their lives through education. We make Harvard education accessible to lifelong learners from high school to retirement.
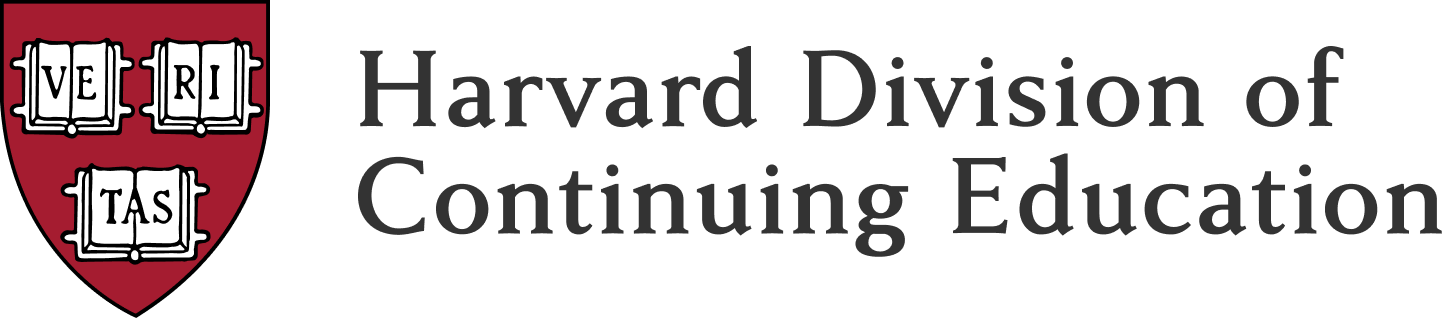
- Our Mission
7 Research Findings About Technology and Education
Here’s what research shows about the effectiveness of technology for learning and when less tech can be more productive.
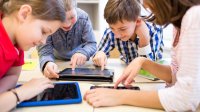
Do students perform better on digital or paper assessments? Does the amount of time spent on an app correlate to learning growth? How much valid and reliable research is typically behind an educational application? These are questions that busy educators often wonder about, yet they may not have an easy way to find answers. Fortunately, there is research on education apps and devices as well as learning growth and outcomes in the research journals. Below are seven things that educators should know about the research on the effectiveness of technology for learning—note that research findings can evolve over time, and the points below are not definitively settled.
Advantages and disadvantages of Tech
1. When screens are present but not being used for learning, students tend to learn less. Whether it’s a laptop or a smartphone , studies have found that the mere presence of these devices reduces available cognitive capacity in college students. Long-term recall and retention of information decreases when students at the university level have screens present during direct instructional time . Just having a laptop screen open or a cell phone next to a student (but not being used) is enough to distract their brain from fully focusing on the class activities.
Further, studies found that students in college who send off-task text or IM messages during class or engage with social media on their devices typically take lower-quality notes, and their overall academic performance is worse than that of those who didn’t engage in those activities during class. It’s important to note that when a student doesn’t have a device but is near another student who is using a device during class, both students’ grades will likely be negatively affected.
2. Literacy applications often have little valid and reliable research associated with them. A number of applications in the app stores (such as Google Play) do not have much, if any, valid and reliable research associated with them. According to a study looking at the top-rated early literacy applications , 77 percent of the applications have zero reliable research behind them. And the few apps that did have research only considered the look and feel of the application (such as ease of navigation or visual appeal), rather than if the child was likely to learn foundational literacy skills from the app.
There are apps that are effective , but finding them in the sea of all available apps—many of them poorly designed, with inadequate backing evidence—is a daunting task.
3. Neither the amount of time spent on an app nor the number of sessions in an app correlates with effectiveness. A recent study found that the “dosage” of the app, such as the number of sessions, time spent per session, and duration of the study, did not predict effectiveness of the app . Thus, learning outcomes did not change if a student spent more or less time in an application. The quality of the application matters more in determining learning growth or outcomes than the amount of time or number of times an application is used.
4. Students who read online tend to comprehend less than those who read via paper. Studies have shown that when it comes to comprehension and reading online versus on paper , the type of text matters. One study discovered that when it comes to leisure reading , the more complex the text, the more likely students will comprehend the content better when reading on paper.
Print reading over a long period of time could boost comprehension skills by six to eight times more than digital reading. The same study found that younger children (ages 6–12) seem to benefit the most from print reading over online. Further, another recent study found that university students tend to annotate more when reading on paper versus digital text, though this does not improve their subsequent memory of the text.
5. Students tend to perform worse when testing online compared with those who test on paper. While many standardized tests have moved online, there’s research that doesn’t support this as the best medium for optimal outcomes. A 2018 study determined that students tend to score worse when testing online versus paper in both math and English language arts. In particular, English language learners, children from lower-income homes, and students on individualized education programs perform worse online than on paper.
Some studies are finding that the use of computers in formal assessments creates an obstacle for students who need special accommodations like text-to-speech readers or language translators. For example, students with visual impairments tended to perform worse on computer-based tests that provided a digital reader, compared with similar students who took paper tests with a human reader.
6. Online classes are best for students who can self-regulate and are independent learners. The Brookings Institution’s Executive Summary on online learning finds that online learning is best suited for students who are high achievers and self-motivated. The research they reviewed found that academically strong students can benefit from fully online courses, while students who are not academically strong tend to do worse in online courses than they would in in-person classes.
One example is the Back on Track study, which looked at ninth-grade students taking credit recovery algebra. The study compared students in a fully online algebra credit recovery course with students in an in-person credit recovery algebra course; the fully online students had worse overall academic outcomes and were less likely to recover credit. Additionally, students in fully online courses with no face-to-face instructor interaction typically fared worse than students in face-to-face classes. The good news is that students in blended courses (part online and part in-person) appear to do about the same as those in fully in-person classes.
7. The type of device matters. While schools often shop for the least expensive option for student devices, it is important to note that a recent study looking at remote learning found that the type and quality of student devices matters in learning outcomes. Students who used devices that were older and had slower processors had a worse quality of learning experiences than those who had newer devices with stronger specifications.
These are some highlights from recent studies that can inform teachers and school districts when it comes to decision-making with purchasing technology, creating policies, or devising alternative academic offerings. It is important to understand the evidence behind any edtech-related decisions that could impact many students.
What are your chances of acceptance?
Calculate for all schools, your chance of acceptance.
Your chancing factors
Extracurriculars.
How to Write Stanford’s “Excited About Learning” Essay
This article was written based on the information and opinions presented by Johnathan Patin-Sauls and Vinay Bhaskara in a CollegeVine livestream. You can watch the full livestream for more info.
What’s Covered:
Choosing an idea vs. an experience, learning for the sake of learning, learning as a means to other ends, be specific.
Stanford University’s first essay prompt asks you to respond to the following:
“ The Stanford community is deeply curious and driven to learn in and out of the classroom. Reflect on an idea or experience that makes you genuinely excited about learning. (100-250 words)”
For this short answer question, your response is limited to a maximum of 250 words. In this article, we will discuss considerations for choosing to write about an idea or experience, ways to demonstrate a love or enthusiasm for learning, and why you should be as specific. For more information and guidance on writing the application essays for Stanford University, check out our post on how to write the Stanford University essays .
Regardless of if you choose either an idea or experience that makes you genuinely excited about learning as a topic, there are a few considerations for each.
Most people gravitate towards writing about an idea. One challenge that arises with an idea-focused essay is that applicants who are passionate about an idea often become hyper focused on explaining the idea but neglect to connect this idea to who they are as a person and why this idea excites them.
When writing about an experience, it is important to strike a balance between describing the experience and analyzing the impact of the experience on you, your goals, and your commitment to learning.
This essay question allows you to expand on your joy for learning and your genuine curiosity. Stanford is searching for students who are naturally curious and enjoy the process of learning and educating themselves. For example, a compelling essay could begin with a riveting story of getting lost while hiking the Appalachian Trail and describing how this experience led to a lifelong passion for studying primitive forms of navigation.
There is a strong tendency among applicants to write about formal academic coursework, however, the most compelling essays will subvert expectations by taking the concept of learning beyond the classroom and demonstrating how learning manifests itself in unique contexts in your life.
If you’re someone for whom learning is a means to other ends, it is important that you convey a sense of genuine enthusiasm and purpose beyond, “I want to go to X school because it will help me get Y job for Z purpose.” You may be motivated to attend college to obtain a certain position and make a comfortable income, however these answers are not necessarily what admissions officers are looking for. Instead, it can be helpful to relate an idea or experience to something more personal to you.
Academic & Professional Trajectory
Consider relating the idea or experience you choose to a major, degree program, research initiative, or professor that interests you at Stanford. Then go beyond the academic context to explain how the idea or experience ties into your future career.
For instance, if you are interested in the concept of universal health care, then you might describe your interest in applying to public health programs with faculty that specialize in national health care systems. You might then describe your long term career aspirations to work in the United States Senate on crafting and passing health care policy.
Personal Values & Experiences
Another way to tie the ideas in this essay back to a more personal topic is to discuss how the idea or experience informs who you are, how you treat others, or how you experience the world around you.
You could also focus on an idea or experience that has challenged, frustrated, or even offended you, thereby reinforcing and further justifying the values you hold and your worldview.
Community Building & Social Connectedness
You may also explore how this idea or experience connects you to a particular community by helping you understand, build, and support members of the community. Stanford is looking to find students who will be engaged members of the student body and carry out the community’s core mission, values, and projects, so this essay can be an opportunity to highlight how you would contribute to Stanford.
Be specific in your choice of idea or the way in which you describe an experience. For example, a response that focuses on the joys of learning philosophy is too broad to be particularly memorable or impactful. However, the mind-body problem looking at the debate concerning the relationship between thought and consciousness is a specific philosophical idea that lends itself to a rich discussion.
Related CollegeVine Blog Posts
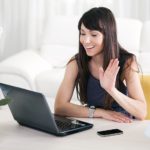
Constructivist Learning Theory and Creating Effective Learning Environments
- First Online: 30 October 2021
Cite this chapter
- Joseph Zajda 36
Part of the book series: Globalisation, Comparative Education and Policy Research ((GCEP,volume 25))
5108 Accesses
18 Citations
This chapter analyses constructivism and the use of constructivist learning theory in schools, in order to create effective learning environments for all students. It discusses various conceptual approaches to constructivist pedagogy. The key idea of constructivism is that meaningful knowledge and critical thinking are actively constructed, in a cognitive, cultural, emotional, and social sense, and that individual learning is an active process, involving engagement and participation in the classroom. This idea is most relevant to the process of creating effective learning environments in schools globally. It is argued that the effectiveness of constructivist learning and teaching is dependent on students’ characteristics, cognitive, social and emotional development, individual differences, cultural diversity, motivational atmosphere and teachers’ classroom strategies, school’s location, and the quality of teachers. The chapter offers some insights as to why and how constructivist learning theory and constructivist pedagogy could be useful in supporting other popular and effective approaches to improve learning, performance, standards and teaching. Suggestions are made on how to apply constructivist learning theory and how to develop constructivist pedagogy, with a range of effective strategies for enhancing meaningful learning and critical thinking in the classroom, and improving academic standards.
The unexamined life is not worth living (Socrates, 399 BCE).
This is a preview of subscription content, log in via an institution to check access.
Access this chapter
Subscribe and save.
- Get 10 units per month
- Download Article/Chapter or eBook
- 1 Unit = 1 Article or 1 Chapter
- Cancel anytime
- Available as PDF
- Read on any device
- Instant download
- Own it forever
- Available as EPUB and PDF
- Compact, lightweight edition
- Dispatched in 3 to 5 business days
- Free shipping worldwide - see info
- Durable hardcover edition
Tax calculation will be finalised at checkout
Purchases are for personal use only
Institutional subscriptions
Abdullah Alwaqassi, S. (2017). The use of multisensory in schools . Indiana University. https://scholarworks.iu.edu/dspace/bitstream/handle/2022/21663/Master%20Thesis%20in%
Acton, G., & Schroeder, D. (2001). Sensory discrimination as related to general intelligence. Intelligence, 29 , 263–271.
Google Scholar
Adak, S. (2017). Effectiveness of constructivist approach on academic achievement in science at secondary level. Educational Research Review, 12 (22), 1074–1079.
Adler, E. (1997). Seizing the middle ground: Constructivism in world politics. European Journal of International Relations, 3 , 319–363.
Akpan, J., & Beard, B. (2016). Using constructivist teaching strategies to enhance academic outcomes of students with special needs. Journal of Educational Research , 4 (2), 392–398. Retrieved from https://files.eric.ed.gov/fulltext/EJ1089692.pdf
Al Sayyed Obaid, M. (2013). The impact of using multi-sensory approach for teaching students with learning disabilities. Journal of International Education Research , 9 (1), 75–82. Retrieved from https://eric.ed.gov/?id=EJ1010855
Alt, D. (2017).Constructivist learning and openness to diversity and challenge in higher education environments. Learning Environments Research, 20 , 99–119. Retrieved from https://doi.org/10.1007/s10984-016-9223-8
Arends, R. (1998). Learning to teach . Boston: McGraw Hill.
Ayaz, M. F., & Şekerci, H. (2015). The effects of the constructivist learning approach on student’s academic achievement: A meta-analysis study . Retrieved from http://citeseerx.ist.psu.edu/viewdoc/download?doi=10.1.1.1072.4600&rep=rep1&type=pdf
Barlett, F. (1932), Remembering: A study in experimental and social psychology. Cambridge: CUP.
Bandura, A. (1977). Social learning theory . New York: General Learning Press.
Beck, C., & Kosnik, C. (2006). Innovations in teacher education: A social constructivist approach . New York, NY: SUNY Press.
Black, A., & Ammon, P. (1992). A developmental-constructivist approach to teacher education. Journal of Teacher Education, 43 (5), 323–335.
Bowles, S., & Gintis, H. (1976). Schooling in capitalist America . London: Routledge & Kegan Paul.
Brooks, J., & Brooks, M. (1993). In search of understanding: The case for constructivist classrooms . Alexandria, VA: Association of Supervision and Curriculum Development.
Bruner, J. (1963). The process of education . New York: Vintage Books.
Bynum, W. F., & Porter, R. (Eds.). (2005). Oxford dictionary of scientific quotations . Oxford: Oxford University Press.
Carnoy, M. (1999). Globalization and education reforms: What planners need to know . Paris: UNESCO, International Institute for Educational Planning.
Crogman, H., & Trebeau Crogman, M. (2016). Generated questions learning model (GQLM): Beyond learning styles . Retrieved from https://www.cogentoa.com/article/10.1080/2331186X.2016.1202460
Dangel, J. R. (2011). An analysis of research on constructivist teacher education . Retreived from https://ineducation.ca/ineducation/article/view/85/361
Dewey, J. (1938). Experience and education . New York: Collier Books.
Doll, W. (1993). A post-modem perspective on curriculum . New York: Teachers College Press.
Doolittle, P. E., & Hicks, D. E. (2003). Constructivism as a theoretical foundation for the use of technology in social studies. Theory and Research in Social Education, 31 (1), 71–103.
Dunn, R., & Smith, J. B. (1990). Chapter four: Learning styles and library media programs. In J. B. Smith (Ed.), School library media annual (pp. 32–49). Englewood, CO: Libraries Unlimited.
Dunn, R., et al. (2009). Impact of learning-style instructional strategies on students’ achievement and attitudes: Perceptions of educators in diverse institutions. The Clearing House, 82 (3), 135–140. Retrieved from http://www.jstor.org/stable/30181095
Fontana, D. (1995). Psychology for teachers . London: Palgrave Macmillan.
Fosnot, C. T. (Ed.). (1989). Constructivism: Theory, perspectives, and practice . New York: Teacher's College Press.
Fosnot, C. T., & Perry, R. S. (2005). Constructivism: A psychological theory of learning. In C. T. Fosnot (Ed.), Constructivism: Theory, perspectives, and practice . New York: Teacher’s College Press.
Gardner, H. (1983). Frames of mind: The theory of multiple intelligences . New York: Basic Books.
Gardner, H. (1999). Intelligence reframed: Multiple intelligences for the 21st century . New York: Basic Books.
Gredler, M. E. (1997). Learning and instruction: Theory into practice (3rd ed.). Upper Saddle River, NJ: Prentice-Hall.
Gupta, N., & Tyagi, H. K. (2017). Constructivist based pedagogy for academic improvement at elementary level . Retrieved from https://www.researchgate.net/publication/321018062_constructivist_based_pedagogy_for_academic_improvement_at_elementary_level
Guzzini, S. (2000). A reconstruction of constructivism in international relations. European Journal of International Relations, 6 , 147–182.
Hirtle, J. (1996). Social constructivism . English Journal, 85 (1), 91. Retrieved from. https://search.proquest.com/docview/237276544?accountid=8194
Howe, K., & Berv, J. (2000). Constructing constructivism, epistemological and pedagogical. In D. C. Phillips (Ed.), Constructivism in education (pp. 19–40). Illinois: The National Society for the Study of Education.
Hunter, W. (2015). Teaching for engagement: part 1: Constructivist principles, case-based teaching, and active learning . Retrieved from https://www.researchgate.net/publication/301950392_Teaching_for_Engagement_Part_1_Co
Jonassen, D. H. (1994). Thinking technology. Educational Technology, 34 (4), 34–37.
Jonassen, D. H. (2000). Revisiting activity theory as a framework for designing student-centered learning environments. In D. H. Jonassen & S. M. Land (Eds.), Theoretical foundations of learning environments (pp. 89–121). Mahwah, NJ: Lawrence Erlbaum.
Kelly, G. A. (1955/1991). The psychology of personal constructs . Norton (Reprinted by Routledge, London, 1991).
Kharb, P. et al. (2013). The learning styles and the preferred teaching—Learning strategies of first year medical students . Retrieved from https://www.ncbi.nlm.nih.gov/pmc/articles/PMC3708205/
Kim, B. (2001). Social constructivism. In M. Orey (Ed.), Emerging perspectives on learning, teaching, and technology . http://www.coe.uga.edu/epltt/SocialConstructivism.htm
Kim, J. S. (2005). The effects of a constructivist teaching approach on student academic achievement, self-concept, and learning strategies. Asia Pacific Education Review, 6 (1), 7–19.
Kolb, D. A., & Fry, R. (1975). Toward an applied theory of experiential learning. In C. Cooper (Ed.), Theories of group process . London: John Wiley.
Kukla, A. (2000). Social constructivism and the philosophy of science . London: Routledge.
Mahn, H., & John-Steiner, V. (2012). Vygotsky and sociocultural approaches to teaching and learning . https://doi.org/10.1002/9781118133880.hop207006
Martin, J., & Sugarman, J. (1999). The psychology of human possibility and constraint . Albany: SUNY.
Matthews, M. (2000). Constructivism in science and mathematics education. In C. Phillips (Ed.), Constructivism in education, ninety-ninth yearbook of the national society for the study of education, Part 1 (pp. 159–192). Chicago: University of Chicago Press.
Maypole, J., & Davies, T. (2001). Students’ perceptions of constructivist learning in a Community College American History 11 Survey Course . Retrieved from https://doi.org/10.1177/009155210102900205
McInerney, D. M., & McInerney, V. (2018). Educational psychology: Constructing learning (5th ed.). Sydney: Pearson.
McLeod, S. (2019). Constructivism as a theory for teaching and learning . Retrieved from https://www.simplypsychology.org/constructivism.html
OECD. (2007). Equity and quality in education . Paris: OECD.
OECD. (2009a). Key factors in developing effective learning environments: Classroom disciplinary climate and teachers’ self-efficacy. In Creating effective teaching and learning environments . Paris: OECD.
OECD. (2009b). Education at a glance . Paris: OECD.
OECD. (2009c). The schooling for tomorrow . Paris: OECD, Centre for Educational Research and Innovation.
OECD. (2013). Synergies for better learning: An international perspective on evaluation & assessment . Retrieved from // www.oecd.org/edu/school/Evaluation_and_Assessment_Synthesis_Report.pdf
OECD. (2019a). PISA 2018 results (volume III): What school life means for students’ lives . Paris: OECD.
Oldfather, P., West, J., White, J., & Wilmarth, J. (1999). Learning through children’s eyes: Social constructivism and the desire to learn . Washington, DC: American Psychological Association.
O’Loughin, M. (1992). Rethinking science education: Beyond Piagetian constructivism toward a sociocultural model of teaching and learning. Journal of Research in Science Teaching, 2 (8), 791–820.
Onuf, N. (2003). Parsing personal identity: Self, other, agent. In F. Debrix (Ed.), Language, agency and politics in a constructed world (pp. 26–49). Armonk, NY: M.E. Sharpe.
Onuf, N. G. (2013). World of our making . Abingdon, UK: Routledge.
Packer, M., & Goicoechea, J. (2000). Sociocultural and constructivist theories of learning: Ontology, not just epistemology. Educational Psychologist, 35 (4), 227–241.
Phillips, D. (2000). An opinionated account of the constructivist landscape. In D. C. Phillips (Ed.), Constructivism in education, Ninety-ninth yearbook of the national society for the study of education, Part 1 (pp. 1–16). Chicago: University of Chicago Press.
Piaget, J. (1936). Origins of intelligence in the child . London: Routledge & Kegan Paul.
Piaget, J. (1967). Biologie et connaissance (Biology and knowledge). Gallimard.
Piaget, J. (1972). The principles of genetic epistemology (W. Mays, Trans.). Basic Books.
Piaget, J. (1977). The development of thought: Equilibration of cognitive structures . (A. Rosin, Trans.). The Viking Press.
Postman, N., & Weingartner, C. S. (1971). Teaching as a subversive activity . Harmondsworth: Penguin Books.
Puacharearn, P. (2004). The effectiveness of constructivist teaching on improving learning environments in thai secondary school science classrooms . Doctor of Science Education thesis. Curtin University of Technology. Retrieved from https://espace.curtin.edu.au/bitstream/handle/20.500.11937/2329/14877_
Richardson, V. (2003). Constructivist pedagogy. Teachers College Record, 105 (9), 1623–1640.
Sadler-Smith, E. (2001). The relationship between learning style and cognitive style. Personality and Individual Differences, 30 (4), 609–616.
Searle, J. R. (1995). The construction of social reality . New York, NY: Penguin Books.
Shah, R. K. (2019). Effective constructivist teaching learning in the classroom . Retrieved from https://files.eric.ed.gov/fulltext/ED598340.pdf
Sharma, H. L., & Sharma, L. (2012). Effect of constructivist approach on academic achievement of seventh grade learners in Mathematics. International Journal of Scientific Research, 2 (10), 1–2.
Shively, J. (2015). Constructivism in music education. Arts Education Policy Review: Constructivism, Policy, and Arts Education, 116 (3), 128–136.
Shor, I. (1992). Empowering education: Critical teaching for social change . Chicago: University of Chicago Press.
Slavin, R. (1984). Effective classrooms, effective schools: A research base for reform in Latin American education . Retrieved from http://www.educoas.org/Portal/bdigital/contenido/interamer/BkIACD/Interamer/
Slavin, R. E. (2020). Education psychology: theory and practice (12th ed.). Pearson.
Steffe, L., & Gale, J. (Eds.). (1995). Constructivism in education . Hillsdale, NJ.: Erlbaum.
Stoffers, M. (2011). Using a multi-sensory teaching approach to impact learning and community in a second-grade classroom . Retrieved from https://rdw.rowan.edu/cgi/viewcontent.cgi?article=1109&context=etd
Thomas, A., Menon, A., Boruff, J., et al. (2014). Applications of social constructivist learning theories in knowledge translation for healthcare professionals: A scoping review. Implementation Science, 9 , 54. https://doi.org/10.1186/1748-5908-9-54 .
Article Google Scholar
Thompson, P. (2000). Radical constructivism: Reflections and directions. In L. P. Steffe & P. W. Thompson (Eds.), Radical constructivism in action: Building on the pioneering work of Ernst von Glasersfeld (pp. 412–448). London: Falmer Press.
von Glaserfeld, E. (1995). Radical constructivism: A way of knowing and learning . London: The Falmer Press.
Vygotsky, L. S. (1934a). Myshlenie i rech (Thought and language). State Socio-Economic Publishing House (translated in 1986 by Alex Kozulin, MIT).
Vygotsky. (1934b). Thought and language . Cambridge, Mass.: The MIT Press.
Vygotsky, L. (1968). The psychology of art . Moscow: Art.
Vygotsky, L. (1973). Thought and language (A. Kozulin, Trans. and Ed.). The MIT Press. (Originally published in Russian in 1934.)
Vygotsky, L. S. (1978). In M. Cole, V. John-Steiner, S. Scribner, & E. Souberman (Eds.), Mind in society: The development of higher psychological processes . Cambridge, MA: Harvard University Press.
Watson, J. (2003). Social constructivism in the classroom . Retrieved from https://onlinelibrary.wiley.com/doi/abs/10.1111/1467-9604.00206
Wertsch, J. V. (1991). Voices of the mind: A sociocultural approach to mediated action . Cambridge, MA: Harvard University Press.
Zajda, J. (Ed.). (2008a). Learning and teaching (2nd ed.). Melbourne: James Nicholas Publishers.
Zajda, J. (2008b). Aptitude. In G. McCulloch & D. Crook (Eds.), The international encyclopedia of education . London: Routledge.
Zajda, J. (2008c). Globalisation, education and social stratification. In J. Zajda, B. Biraimah, & W. Gaudelli (Eds.), Education and social inequality in the global culture (pp. 1–15). Dordrecht: Springer.
Zajda, J. (2018a). Motivation in the classroom: Creating effective learning environments. Educational Practice & Theory, 40 (2), 85–103.
Zajda, J. (2018b). Effective constructivist pedagogy for quality learning in schools. Educational Practice & Theory, 40 (1), 67–80.
Zajda, J. (Ed.). (2020a). Globalisation, ideology and education reforms: Emerging paradigms . Dordrecht: Springer.
Zajda, J. (Ed.). (2021). 3rd international handbook of globalisation, education and policy research . Dordrecht: Springer.
Zajda, J., & Majhanovich, S. (Eds.). (2021). Globalisation, cultural identity and nation-building: The changing paradigms . Dordrecht: Springer.
Zaphir, L. (2019). Can schools implement constructivism as an education philosophy? Retrieved from https://www.studyinternational.com/news/constructivism-education/
Download references
Author information
Authors and affiliations.
Faculty of Education & Arts, School of Education, Australian Catholic University, East Melbourne, VIC, Australia
Joseph Zajda
You can also search for this author in PubMed Google Scholar
Rights and permissions
Reprints and permissions
Copyright information
© 2021 Springer Nature Switzerland AG
About this chapter
Zajda, J. (2021). Constructivist Learning Theory and Creating Effective Learning Environments. In: Globalisation and Education Reforms. Globalisation, Comparative Education and Policy Research, vol 25. Springer, Cham. https://doi.org/10.1007/978-3-030-71575-5_3
Download citation
DOI : https://doi.org/10.1007/978-3-030-71575-5_3
Published : 30 October 2021
Publisher Name : Springer, Cham
Print ISBN : 978-3-030-71574-8
Online ISBN : 978-3-030-71575-5
eBook Packages : Education Education (R0)
Share this chapter
Anyone you share the following link with will be able to read this content:
Sorry, a shareable link is not currently available for this article.
Provided by the Springer Nature SharedIt content-sharing initiative
- Publish with us
Policies and ethics
- Find a journal
- Track your research

An official website of the United States government
The .gov means it’s official. Federal government websites often end in .gov or .mil. Before sharing sensitive information, make sure you’re on a federal government site.
The site is secure. The https:// ensures that you are connecting to the official website and that any information you provide is encrypted and transmitted securely.
- Publications
- Account settings
Preview improvements coming to the PMC website in October 2024. Learn More or Try it out now .
- Advanced Search
- Journal List
- Springer Nature - PMC COVID-19 Collection

What are the key elements of a positive learning environment? Perspectives from students and faculty
Shayna a. rusticus.
Department of Psychology, Kwantlen Polytechnic University, 12666 72 Ave, Surrey, BC V3W 2M8 Canada
Tina Pashootan
The learning environment comprises the psychological, social, cultural and physical setting in which learning occurs and has an influence on student motivation and success. The purpose of the present study was to explore qualitatively, from the perspectives of both students and faculty, the key elements of the learning environment that supported and hindered student learning. We recruited a total of 22 students and 9 faculty to participate in either a focus group or an individual interview session about their perceptions of the learning environment at their university. We analyzed the data using a directed content analysis and organized the themes around the three key dimensions of personal development, relationships, and institutional culture. Within each of these dimensions, we identified subthemes that facilitated or impeded student learning and faculty work. We also identified and discussed similarities in subthemes identified by students and faculty.
Introduction
The learning environment (LE) comprises the psychological, social, cultural, and physical setting in which learning occurs and in which experiences and expectations are co-created among its participants (Rusticus et al., 2020 ; Shochet et al., 2013 ). These individuals, who are primarily students, faculty and staff, engage in this environment and the learning process as they navigate through their personal motivations and emotions and various interpersonal interactions. This all takes place within a physical setting that consists of various cultural and administrative norms (e.g. school policies).
While many studies of the LE have focused on student perspectives (e.g. Cayubit, 2021 ; Schussler et al., 2021 ; Tharani et al., 2017 ), few studies have jointly incorporated the perspectives of students and faculty. Both groups are key players within the educational learning environment. Some exceptions include researchers who have used both instructor and student informants to examine features of the LE in elementary schools (Fraser & O’Brien, 1985 ; Monsen et al., 2014 ) and in virtual learning and technology engaged environments in college (Annansingh, 2019 ; Downie et al., 2021 ) Other researchers have examined perceptions of both groups, but in ways that are not focused on understanding the LE (e.g. Bolliger & Martin, 2018 ; Gorham & Millette, 1997 ; Midgly et al., 1989 ).
In past work, LEs have been evaluated on the basis of a variety of factors, such as students’ perceptions of the LE have been operationalized as their course experiences and evaluations of teaching (Guo et al., 2021 ); level of academic engagement, skill development, and satisfaction with learning experience (Lu et al., 2014 ); teacher–student and student–peer interactions and curriculum (Bolliger & Martin, 2018 ; Vermeulen & Schmidt, 2008 ); perceptions of classroom personalization, involvement, opportunities for and quality of interactions with classmates, organization of the course, and how much instructors make use of more unique methods of teaching and working (Cayubit, 2021 ). In general, high-quality learning environments are associated with positive outcomes for students at all levels. For example, ratings of high-quality LEs have been correlated with outcomes such as increased satisfaction and motivation (Lin et al., 2018 ; Rusticus et al., 2014 ; Vermeulen & Schmidt, 2008 ), higher academic performance (Lizzio et al., 2002 ; Rusticus et al., 2014 ), emotional well-being (Tharani et al., 2017 ), better career outcomes such as satisfaction, job competencies, and retention (Vermeulen & Schmidt, 2008 ) and less stress and burnout (Dyrbye et al., 2009 ). From teacher perspectives, high-quality LEs have been defined in terms of the same concepts and features as those used to evaluate student perspective and outcomes. For example, in one quantitative study, LEs were rated as better by students and teachers when they were seen as more inclusive (Monsen et al., 2014 ).
However, LEs are diverse and can vary depending on context and, although many elements of the LE that have been identified, there has been neither a consistent nor clear use of theory in assessing those key elements (Schönrock-Adema et al., 2012 ). One theory that has been recommended by Schönrock-Adema et al. ( 2012 ) to understand the LE is Moos’ framework of human environments (Insel & Moos, 1974 ; Moos, 1973 , 1991 ). Through his study of a variety of human environments (e.g. classrooms, psychiatric wards, correctional institutions, military organizations, families), Moos proposed that all environments have three key dimensions: (1) personal development/goal direction, (2) relationships, and (3) system maintenance/change. The personal development dimension encompasses the potential in the environment for personal growth, as well as reflecting the emotional climate of the environment and contributing to the development of self-esteem. The relationship dimension encompasses the types and quality of social interactions that occur within the environment, and it reflects the extent to which individuals are involved in the environment and the degree to which they interact with, and support, each other. The system maintenance/change dimension encompasses the degree of structure, clarity and openness to change that characterizes the environment, as well as reflecting physical aspects of the environment.
We used this framework to guide our research question: What do post-secondary students and faculty identify as the positive and negative aspects of the learning environment? Through the use of a qualitative methodology to explore the LE, over the more-typical survey-based approaches, we were able to explore this topic in greater depth, to understand not only the what, but also the how and the why of what impacts the LE. Furthermore, in exploring the LE from both the student and faculty perspectives, we highlight similarities and differences across these two groups and garner an understanding of how both student and faculty experience the LE.
Participants
All participants were recruited from a single Canadian university with three main campuses where students can attend classes to obtain credentials, ranging from a one-year certificate to a four-year undergraduate degree. Approximately 20,000 students attend each year. The student sample was recruited through the university’s subject pool within the psychology department. The faculty sample was recruited through emails sent out through the arts faculty list-serve and through direct recruitment from the first author.
The student sample was comprised of 22 participants, with the majority being psychology majors ( n = 10), followed by science majors ( n = 4) and criminology majors ( n = 3). Students spanned all years of study with seven in their first year, three in second year, five in third year, six in fourth year, and one unclassified. The faculty sample consisted of nine participants (6 male, 3 female). Seven of these participants were from the psychology department, one was from the criminology department and one was from educational studies. The teaching experience of faculty ranged from 6 to 20 years.
Interview schedule and procedure
We collected student data through five focus groups and two individual interviews. The focus groups ranged in size from two to six participants. All sessions occurred in a private meeting room on campus and participants were provided with food and beverages, as well as bonus credit. Each focus group/interview ranged from 30 to 60 min. We collected all faculty data through individual interviews ranging from 30 to 75 min. Faculty did not receive any incentives for their participation. All sessions were conducted by the first author, with the second author assisting with each of the student focus groups.
With the consent of each participant, we audio-recorded each session and transcribed them verbatim. For both samples, we used a semi-structured interview format involving a set of eight open-ended questions about participants’ overall perceptions of the LE at their institution (see Appendix for interview guide). These questions were adapted from a previous study conducted by the first author (Rusticus et al., 2020 ) and focused on how participants defined the LE, what they considered to be important elements of the LE, and their positive and negative experiences within their environment. Example questions were: “Can you describe a [negative/positive] learning [students]/teaching [faculty] experience that you have had?”.
We analyzed the data using a directed content analysis approach (Hsieh & Shannon, 2005 ) that used existing theory to develop the initial coding scheme. We used Moos’s (Insel & Moos, 1974 ; Moos, 1973 , 1991 ) framework and its three dimensions of personal development, relationships, and system maintenance/change to guide our analysis. During the analysis phase, we renamed the system maintenance/change dimension to ‘institutional setting’ as we felt it was more descriptive of, and better represented, the content of this theme.
We analyzed student and faculty data separately, starting with the student data, but used the same process for both. First, we randomly selected two transcripts. We each independently coded the first transcript using the broad themes of personal development, relationships and institutional setting, and developed subcodes within each of these themes, as needed. We then reviewed and discussed our codes, reaching consensus on any differences in coding. We then repeated this process for the second transcript and, through group discussions, created a codebook. The first author then coded the remaining transcripts.
When coding the faculty data, we aimed to maintain subcodes similar to the student data while allowing for flexibility when needed. For instance, within the personal development theme, a subcode for the student data was ‘engagement with learning’, whereas a parallel subcode for the faculty data was ‘engagement with teaching’.
We present the results of the student and faculty data separately. For both, we have organized our analysis around the three overarching themes of personal development, relationships and institutional setting.
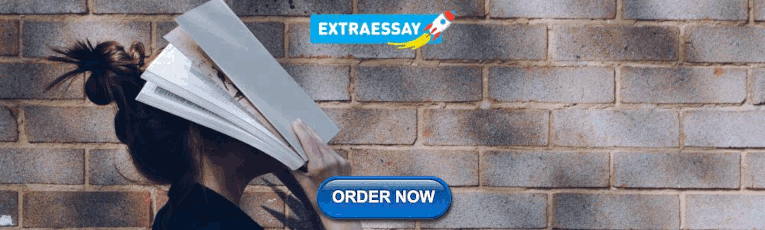
Student perspectives of the learning environment
Personal development.
Personal development was defined as any motivation either within or outside the LE that provide students with encouragement, drive, and direction for their personal growth and achievement. Within this theme, there were two subthemes: engaging with learning and work-life balance.
Engagement with learning reflected a student’s desire and ability to participate in their learning, as opposed to a passive-learning approach. Students felt more engaged when they were active learners, as well as when they perceived the material to be relevant to their career goals or real-world applications. Students also said that having opportunities to apply their learning helped them to better understand their own career paths:
I had two different instructors and both of them were just so open and engaging and they shared so many personal stories and they just seemed so interested in what they were doing and what I was like. Wow, I want to be that. I want to be interested in what I’m learning. (G6P1)
A common complaint that negatively impacted student motivation was that instructors would lecture for the entire class without supporting materials or opportunities for students to participate.
I’ve had a couple professors who just don’t have any visuals at all. All he does is talk. So, for the whole three hours, we would just be scrambling to write down the notes. It’s brutal...(G7P2)
Trying to establish a healthy work-life balance and managing the demands of their courses, often in parallel with managing work and family demands, were key challenges for students and were often sources of stress and anxiety. For instance, one student spoke about her struggles in meeting expectations:
It was a tough semester. For the expectations that I had placed on myself, I wasn’t meeting them and it took a toll on me. But now I know that I can exceed my expectations, but you really have to try and work hard for it. (G6P1)
Achieving a good work-life balance and adjusting to university life takes time. Many students commented that, as they reached their third year of study, they felt more comfortable in the school environment. Unfortunately, students also noted that the mental and emotional toll of university life can lead to doubt about the future and a desire to leave. One student suggested more support for students to help with this adjustment:
I think school should give students more service to help them to overcome the pressure and make integration into the first year and second year quicker and faster. Maybe it’s very helpful for the new students. (G7P4)
Relationships
Relationships was the second dimension of the LE. Subthemes within this dimension included: faculty support, peer interaction, and group work. Most students commented on the impact that faculty had on their learning. Faculty support included creating a safe or unsafe space in the classroom (i.e. ability to ask questions without judgement, fostering a respectful atmosphere), providing additional learning material, accommodating requests, or simply listening to students. Students generally indicated that faculty at this university were very willing to offer extra support and genuinely cared for them and their education. Faculty were described as friendly and approachable, and their relationships with students were perceived as “egalitarian”.
I think feeling that you’re safe in that environment, that anything you pose or any questions that you may have, you’re free to ask. And without being judged. And you’ll get an answer that actually helps you. (G1P4)
While most students felt welcome and comfortable in their classes, a few students spoke about negative experiences that they had because of lack of faculty support. Students cited examples of professors “shutting down” questions, saying that a question was “stupid”, refusing requests for additional help, or interrupting them while speaking. Another student felt that the inaction of faculty sometimes contributed to a negative atmosphere:
I’ve had bad professors that just don't listen to any comment or, if you suggest something to improve it which may seem empirically better, they still shut you down! That’s insane. (G2P2)
The peer interactions subtheme referred to any instances when students could interact with other students; this occurred both in and out of the classroom. Most often, students interacted with their peers during a class or because of an assignment:
I think the way the class is structured really helps you build relationships with your peers. For example, I met S, we had several classes with each other. Those classes were more proactive and so it allowed us to build a relationship… I think that’s very important because we’re going to be in the same facility for a long time and to have somebody to back you up, or to have someone to study with…”. (G1P4)
However, other students felt that they lacked opportunities to interact with peers in class. Although a few participants stated that they felt the purpose of going to school was to get a degree, rather than to socialize with others, students wanted more opportunities to interact with peers.
The final subtheme, group work, was a very common activity at this school. The types of group work in which students engaged included classroom discussions, assignments/projects, and presentations. Many students had enjoyable experiences working in groups, noting that working together helped them to solve problems and create something that was better than one individual’s work. Even though sometimes doing the work itself was a negative experience, people still saw value in group work:
Some of the best memories I’ve ever had was group work and the struggles we've had. (G2P2) I don’t like group work but it taught me a lot, I’ve been able to stay friends and be able to connect with people that I’ve had a class with in 2nd year psych all the way up till now. I think that’s very valuable. (G6P1)
Almost all students who spoke about group work also talked about negative aspects or experiences they had. When the work of a group made up a large proportion of the final grade, students sometimes would have preferred to be evaluated individually. Students disliked when they worked in groups when members were irresponsible or work was not shared equally, and they were forced to undertake work that other students were not completing.
A lot of people don’t really care, or they don’t take as much responsibility as you. I think people have different goals and different ways of working, so sometimes I find that challenging. (G7P2)
Institutional setting
The third overarching theme was the institutional setting. Broadly, this theme refers to the physical structure, expectations, and the overall culture of the environment and was composed of two key subthemes: importance of small class sizes; and the lack of a sense of community.
Small class sizes, with a maximum of 35 students, were a key reason why many students chose to come to this institution. The small classes created an environment in which students and faculty were able to get to know one another more personally; students felt that they were known as individuals, not just as numbers. They also noted that this promoted greater feelings of connectedness to the class environment, more personalized attention, and opportunities to request reference letters in the future:
My professors know my name. Not all of them that I’m having for the first time ever, but they try… That means a lot to me. (G6P4)
Several students also said that having smaller class sizes helped them to do well in their courses. The extra attention encouraged them to perform better academically, increased their engagement with their material, and made them feel more comfortable in asking for help.
Having a sense of belonging was a key feature of the environment and discussions around a sense of community (or lack thereof) was a prominent theme among the students. Students generally agreed that the overall climate of the school is warm and friendly. However, many students referred to the institution as a “commuter school”, because there are no residencies on campus and students must commute to the school. This often resulted in students attending their classes and then leaving immediately after, contributing to a lack of community life on campus.
What [other schools] have is that people live on campus. I think that plays a huge role. We can’t ignore that we are a commuter school… They have these events and people go because they’re already there and you look at that and it seems to be fun and engaging. (G4P1)
Furthermore, students commented on a lack of campus areas that supported socialization and encouraged students to remain on campus. While there were events and activities that were regularly hosted at the school, students had mixed opinions about them. Some students attended the events and found them personally beneficial. Other students stated that, although many events and activities were available, turnout was often low:
There isn’t any hanging out after campus and you can even see in-events and in-event turnout for different events… It is like pulling teeth to get people to come out to an event… There are free food and fun music and really cool stuff. But, no one’s going to go. It’s sad. (G5P1)
Faculty perspectives on the learning environment
Similar to the student findings, faculty data were coded within the three overarching themes of personal development, relationships, and institutional setting.
Personal development reflected any motivation either within or outside the LE that provided faculty with the encouragement, drive, and direction for their personal growth and engagement with teaching. Within this dimension, there were two main subthemes: motivation to teach and emotional well-being.
As with any career, there are many positive and negative motivating factors that contribute to one’s involvement in their work. Faculty generally reported feeling passionate about their work, and recounted positive experiences they have had while teaching, both personally and professionally. While recollecting positive drives throughout their career, one instructor shared:
It’s [teaching in a speciality program] allowed me to teach in a very different way than the traditional classroom… I’ve been able to translate those experiences into conferences, into papers, into connections, conversations with others that have opened up really interesting dialogues…. (8M)
Faculty also reported that receiving positive feedback from students or getting to see their students grow over time was highly motivating:
I take my teaching evaluations very seriously and I keep hearing that feedback time and time again they feel safe. They feel connected, they feel listened too, they feel like I'm there for them. I think, you know, those are the things that let me know what I'm doing is achieving the goals that I have as an educator. (1F) Being able to watch [students] grow over time is very important to me… I always try to have a few people I work with and see over the course of their degree. So, when they graduate, you know I have a reason to be all misty-eyed. (2M)
Emotional well-being related to how different interactions, primarily with students, affected instructors’ mental states. Sometimes the emotional well-being of faculty was negatively affected by the behaviour of students. One instructor spoke about being concerned when students drop out of a class:
A student just this last semester was doing so well, but then dropped off the face of the earth… I felt such a disappointing loss… So, when that happens, I'm always left with those questions about what I could have done differently. Maybe, at the end of the day, there is nothing I could've done, nothing. It's a tragedy or something's happened in their life or I don't know. But those unanswered questions do concern-- they cause me some stress or concern. (1F)
Another instructor said that, while initially they had let the students’ behaviour negatively affect their well-being, over time, they had eventually become more apathetic.
There are some who come, leave after the break. Or they do not come, right, or come off and on. Previously I was motivated to ask them ‘what is your problem?’ Now I do not care. That is the difference which has happened. I do not care. (4M)
This dimension included comments related to interactions with other faculty and with students and consisted of three subthemes: faculty supporting faculty; faculty supporting students; and creating meaningful experiences for students.
Most faculty felt that it was important to be supported by, and supportive to, their colleagues. For instance, one instructor reported that their colleagues’ helpfulness inspired them to be supportive of others:
If I was teaching a new course, without me having to go and beg for resources or just plead and hope that someone might be willing to share, my experience was that the person who last taught the course messaged me and said let me know if anything I have will be useful to you… When people are willing to do that for you, then you’re willing to do that for someone else….(7M)
Many faculty members also spoke about the importance of having supportive relationships with students, and that this would lead to better learning outcomes:
If you don't connect with your students, you're not going to get them learning much. They're not; they're just going to tune out. So, I think, I think connection is critical to having a student not only trust in the learning environment, but also want to learn from the learning environment. (3M)
Facilitating an open, inviting space in the classroom and during their office hours, where students were comfortable asking questions, was one way that faculty tried to help students succeed. Faculty also spoke about the value of having close mentorship relationships with students:
I work with them a lot and intensively…and their growth into publishing, presenting, and seeing them get recognized and get jobs on their way out and so forth are extraordinary. So, being able to watch them grow over time is very important to me. (2M)
Faculty also noted that occasionally there were instances when students wanted exceptions to be made for them which can create tensions in the environment. One instructor spoke about the unfairness of those requests arguing that students need to be accountable to themselves:
The failure rate, …it was 43%. I do not know if there is any other course in which there is a 43% failure rate. So, I do not want to fail these students, why? Instructors want these students to pass, these are my efforts […], and there are also the efforts of these students and their money, right? But, if a student doesn’t want to pass himself or herself, I cannot pass this student, that’s it. (4M)
Faculty were generally motivated to provide memorable and engaging experiences for students. These included providing practical knowledge and opportunities to apply knowledge in real-world settings, field schools, laboratory activities, group discussions, guest speakers, field trips, videos and group activities. They were often willing to put in extra effort if it meant that students would have a better educational experience.
Creating meaningful experiences for students was also meaningful for faculty. One faculty member said that faculty felt amazing when the methods that they used in their courses were appreciated by students. Another faculty member noted:
This student who was in my social psychology class, who was really bright and kind of quirky, would come to my office, twice a week, and just want to talk about psychology … That was like a really satisfying experience for me to see someone get so sparked by the content. (9F)
This third theme refers to the physical structure, expectation, and overall culture of the environment and it consisted of two subthemes: the importance of small class sizes, and the lack of a sense of community.
The majority of the faculty indicated that the small class sizes are an integral feature of the LE. The key advantage of the small classes was that they allowed greater connection with students.
Your professor knows your name. That’s a huge difference from other schools. It’s a small classroom benefit. (6F)
Similar to the students, nearly all the faculty indicated that a sense of community at the institution was an important part of the environment, and something that was desired, but it currently was lacking. They spoke about various barriers which prevent a sense of community, such as the lack of residences, a dearth of events and activities at the university, the busy schedules of faculty and students, the commuter nature of the school, and characteristics of the student population:
When I complain about the commuter campus feeling that occurs with students, we suffer from that too at a faculty level… People are just not in their offices because we work from home… And that really also affects the culture… We come in. We do our thing. We meet with students. And then we leave… I encounter so many students in the hallway who are looking for instructors and they can’t find them. (9F)
These findings have provided insight into the perspectives of both students and faculty on the LE of a Canadian undergraduate university. We found that framing our analysis and results within Moos’ framework of human environments (Insel & Moos, 1974 ; Moos, 1973 , 1991 ) was an appropriate lens for the data and that the data fit well within these three themes. This provides support for the use of this theory to characterize the educational LE. Within each of these dimensions, we discuss subthemes that both facilitated and hindered student learning and commonalities among student and faculty perspectives.
Within the personal development dimension, both students and faculty discussed the importance of engagement and/or motivation as a facilitator of a positive LE. When students were engaged with their learning, most often by being an active participant or seeing the relevance of what they were learning, they saw it as a key strength. Other studies have also identified engagement as a feature of positive LEs for populations such as high-school students (Seidel, 2006 ), nursing students (D’Souza et al., 2013 ) and college students taking online courses (e.g. Holley & Dobson, 2008 ; O’Shea et al., 2015 ). Faculty who reported being motivated to teach, often felt that this motivation was fueled by the reactions of their students; when students were engaged, they felt more motivated. This creates a positive cyclic pattern in which one group feeds into the motivation and engagement levels of the other. However, this can also hinder the LE when a lack of engagement in one group can bring down the motivation of the other group (such as students paying more attention to their phones than to a lecture or faculty lecturing for the entire class period).
Emotional climate was another subtheme within the personal development dimension that was shared by both students and faculty although, for students, this was focused more on the stress and anxiety that they felt trying to manage their school workloads with their work and family commitments. The overall emotional climate of the school was generally considered to be positive, which was largely driven by the supportive and welcoming environment provided by the faculty. However, it was the negative emotions of stress and anxiety that often surfaced as a challenging aspect in the environment for students. Past research suggests that some types of stress, such as from a challenge, can improve learning and motivation, but negative stress, such as that reported by our participants, is associated with worsened performance and greater fatigue (LePine et al., 2004 ).
For faculty, their emotional state was often influenced by their students. When things were going well for their students, faculty often shared in the joy; however, when students would disappear without notice from a class, it was a source of disappointment and self-doubt. For other faculty, the accumulation of negative experiences resulted in them being more distant and less affected emotionally than they had been earlier in their career. This diminishing concern could have implications for how engaged faculty are in their teaching, which could in turn influence student engagement and harm the LE.
The relationships dimension was the most influential aspect of the environment for both students and faculty. While both groups felt that the relationships that they formed were generally positive, they also reported a desire for more peer connections (i.e. students with other students and faculty with other faculty). Students commented that it was a typical experience for them to come to campus to attend their classes and then leave afterwards, often to work or study at home. Many of the students at this school attend on a part-time basis while they work part- or full-time and/or attend to family commitments. While this is a benefit to these students to have the flexibility to work and further their education, it comes at loss of the social aspect of post-secondary education.
The one way in which student–peer relationships were fostered was through group work. However, students held both positive and negative views on this: the positive aspect was the opportunity to get to know other students and being able to share the burden of the workload, and the negative aspect was being unfair workloads among team members. When group dynamics are poor, such as unfair work distribution, having different goals and motivations, or not communicating effectively with their groups, it has been shown to lead to negative experiences (Rusticus & Justus, 2019 ).
Faculty also commented that it was typical for them and other faculty to come up to campus only to teach their classes and then leave afterwards. They noted that their office block was often empty and noted instances when students have come looking for faculty only to find a locked office. Overall, faculty did report feeling congenial with, and supported by, their peers. They also desired a greater connection with their peers, but noted that it would require effort to build, which many were not willing to make.
Finally, student–faculty relationships were the most-rewarding experience for both groups. Students saw these experiences as highly encouraging and felt that they created a safe and welcoming environment where they could approach faculty to ask questions and get extra support. However, in some cases, students had negative experiences with faculty and these had an impact on their self-esteem, motivation and willingness to participate in class. Students’ negative experiences and feedback have been shown to result in declined levels of intrinsic motivation, even if their performance ability is not low (Weidinger et al., 2016 ).
Within the third dimension, institutional setting, a key strength was the small class sizes. With a maximum class size of 35 students, this created a more personal and welcoming environment for students. Students felt that their instructors got to know their names and this promoted more opportunities for interactions. Faculty concurred with this, indicating that the small classes provided greater opportunities for interactions with their students. This enabled more class discussions and grouped-based activities which contributed to a more engaging and interactive educational experience for students and faculty. For students, not being able to hide in the crowd of a large lecture hall, as is common in other university settings, encouraged them to work harder on their studies and to seek help from their instructor if needed.
Finally, both students and faculty commented that the lack of a sense of community was a negative aspect of the LE. This institution is known as a commuter school and both groups reported that they would often attend campus only for school/work and would leave as soon as their commitments were done. This limits opportunities to interact with others and could also potentially impact one’s identity as a member of this community. While both groups expressed a desire for more of a community life, neither group was willing to put in much effort to make this happen. Others have also found that sense of community, including opportunities to engage and interact with others, is important in LEs (e.g. Sadera et al., 2009 ). Schools with more activities and opportunities for student involvement have reports of higher satisfaction for both academic and social experiences (Charles et al., 2016 ).
Limitations
Because this study is based on a relatively small sample at a single university, there is a question of whether the findings can be applied to other departments, universities or contexts. However, it is a strength of this study that both student and faculty perceptions were included, because few past studies have jointly looked at these two groups together using qualitative methods. The use of focus groups among the student groups might have limited the openness of some participants. We also acknowledge that the analysis of qualitative data is inevitably influenced by our roles, life experiences and backgrounds. (The first author is a faculty member and the second and third authors were fourth year students at the time of the study.) This might have impacted our approach to the interpretation of the data compared with how others might approach the data and analysis (Denzin & Lincoln, 2008 ). However, the analysis involved consultation among the research team to identify and refine the themes, and the findings are presented with quotes to support the interpretation. Finally, because experiences were self-reported in this study, they have the associated limitations of self-report data. Despite these limitations, we believe that our findings add to what is known about LEs by capturing multiple perspectives within the same environment.
Future directions
Because our sample was comprised of students across multiple years of their program, some of our findings suggest that upper-level students might have different perceptions of the LE from lower-level students (e.g. work/life balance, access to resources, and overall familiarity with the environment and resources available). However, because the small sample sizes within these subgroups prevent any strong conclusions being made, future researchers might want to explore year-of-study differences in the LE. Additionally, the data collected for this study occurred prior to the COVID-19 pandemic and the mandatory switch to online teaching and learning. Future researchers might want to consider how this has impacted student and faculty perceptions of the LE regarding their personal motivation, the nature and quality of the relationships that have formed with peers and faculty, and the culture and norms of their institution.
This study increases our understanding of LEs by incorporating data collected from both students and faculty working in the same context. Across both groups, we identified important aspects of the LE as being high levels of engagement and motivation, a positive emotional climate, support among peers, strong faculty–student relationships, meaningful experiences, and small class sizes. Students identified negative aspects of the LE, such as certain characteristics of group work and struggles with work–life balance. Both faculty and students identified a lack of a sense of community as something that could detract from the LE. These findings identify important elements that educators and researchers might want to consider as they strive to promote more-positive LEs and learning experiences for students.
Appendix: Interview guide
[Faculty] Tell me a little bit about yourself. For instance, what department you are in, how long you have been teaching at KPU, what courses you teach, why you were interested in this study
- When I say the word learning environment, what does that mean to you?
- Probe for specific examples
- Relate to goal development, relationships, KPU culture
- Probe for factors that made it a positive environment
- Probe for factors that made it a negative environment
- How would you describe an ideal environment?
- Probe for reasons why
- Probe for how KPU could be made more ideal
- What recommendations would you give to the Dean of Arts regarding the learning environment? This could be changes you would recommend or things you recommend should stay the same.
- Do you have any final comments? Or feel there is anything about the learning environment that we have not addressed?
Publisher's Note
Springer Nature remains neutral with regard to jurisdictional claims in published maps and institutional affiliations.
- Annansingh F. Mind the gap: Cognitive active learning in virtual learning environment perception of instructors and students. Education and Information Technologies. 2019; 24 (6):3669–3688. doi: 10.1007/s10639-019-09949-5. [ CrossRef ] [ Google Scholar ]
- Bolliger DU, Martin F. Instructor and student perceptions of online student engagement strategies. Distance Education. 2018; 39 (4):568–583. doi: 10.1080/01587919.2018.1520041. [ CrossRef ] [ Google Scholar ]
- Cayubit RFO. Why learning environment matters? An analysis on how the learning environment influences the academic motivation, learning strategies and engagement of college students. Learning Environments Research. 2021 doi: 10.1007/s10984-021-09382-x. [ CrossRef ] [ Google Scholar ]
- Charles CZ, Fischer MJ, Mooney MA, Massey DS. Taming the river: Negotiating the academic, financial, and social currents in selective colleges and universities. Princeton University Press; 2016. [ Google Scholar ]
- D’Souza MA, Venkatesaperumal R, Radhakrishnan J, Balachandran S. Engagement in clinical learning environment among nursing students: Role of nurse educators. Open Journal of Nursing. 2013; 31 (1):25–32. doi: 10.4236/ojn.2013.31004. [ CrossRef ] [ Google Scholar ]
- Denzin NK, Lincoln YS. Collecting and interpreting qualitative materials. Sage; 2008. [ Google Scholar ]
- Downie S, Gao X, Bedford S, Bell K, Kuit T. Technology enhanced learning environments in higher education: A cross-discipline study on teacher and student perceptions. Journal of University Teaching & Learning Practice. 2021; 18 (4):12–21. doi: 10.53761/1.18.4.12. [ CrossRef ] [ Google Scholar ]
- Dyrbye LN, Thomas MR, Harper W, Massie FS, Jr, Power DV, Eacker A, Szydlo DW, Novotny PJ, Sloan JA, Shanafelt TD. The learning environment and medical student burnout: A multicentre study. Medical Education. 2009; 43 (3):274–282. doi: 10.1111/j.1365-2923.2008.03282.x. [ PubMed ] [ CrossRef ] [ Google Scholar ]
- Fraser BJ, O'Brien P. Student and teacher perceptions of the environment of elementary school classrooms. The Elementary School Journal. 1985; 85 (5):567–580. doi: 10.1086/461422. [ CrossRef ] [ Google Scholar ]
- Gorham J, Millette DM. A comparative analysis of teacher and student perceptions of sources of motivation and demotivation in college classes. Communication Education. 1997; 46 (4):245–261. doi: 10.1080/03634529709379099. [ CrossRef ] [ Google Scholar ]
- Guo JP, Yang LY, Zhang J, Gan YJ. Academic self-concept, perceptions of the learning environment, engagement, and learning outcomes of university students: Relationships and causal ordering. Higher Education. 2021 doi: 10.1007/s10734-021-00705-8. [ CrossRef ] [ Google Scholar ]
- Holley D, Dobson C. Encouraging student engagement in a blended learning environment: The use of contemporary learning spaces. Learning, Media and Technology. 2008; 33 (2):139–150. doi: 10.1080/17439880802097683. [ CrossRef ] [ Google Scholar ]
- Hsieh H-F, Shannon SE. Three approaches to qualitative content analysis. Qualitative Health Research. 2005; 15 :1277–1288. doi: 10.1177/1049732305276687. [ PubMed ] [ CrossRef ] [ Google Scholar ]
- Insel PM, Moos RH. Psychological environments: Expanding the scope of human ecology. American Psychologist. 1974; 29 :179–188. doi: 10.1037/h0035994. [ CrossRef ] [ Google Scholar ]
- LePine JA, LePine MA, Jackson CL. Challenge and hindrance stress: Relationships with exhaustion, motivation to learn, and learning performance. Journal of Applied Psychology. 2004; 89 (5):883–891. doi: 10.1037/0021-9010.89.5.883. [ PubMed ] [ CrossRef ] [ Google Scholar ]
- Lin S, Salazar TR, Wu S. Impact of academic experience and school climate of diversity on student satisfaction. Learning Environments Research. 2018; 22 :25–41. doi: 10.1007/s10984-018-9265-1. [ CrossRef ] [ Google Scholar ]
- Lizzio A, Wilson K, Simons R. University students’ perceptions of the learning environment and academic outcomes: Implications for theory and practice. Studies in Higher Education. 2002; 27 :27–52. doi: 10.1080/03075070120099359. [ CrossRef ] [ Google Scholar ]
- Lu G, Hu W, Peng Z, Kang H. The influence of undergraduate students’ academic involvement and learning environment on learning outcomes. International Journal of Chinese Education. 2014; 2 (2):265–288. doi: 10.1163/22125868-12340024. [ CrossRef ] [ Google Scholar ]
- Midgley C, Feldlaufer H, Eccles JS. Student/teacher relations and attitudes toward mathematics before and after the transition to junior high school. Child Development. 1989; 60 (4):981–992. doi: 10.2307/1131038. [ PubMed ] [ CrossRef ] [ Google Scholar ]
- Monsen JJ, Ewing DL, Kwoka M. Teachers’ attitudes towards inclusion, perceived adequacy of support and classroom learning environment. Learning Environments Research. 2014; 17 :113–126. doi: 10.1007/s10984-013-9144-8. [ CrossRef ] [ Google Scholar ]
- Moos RH. Conceptualizations of human environments. American Psychologist. 1973; 28 :652–665. doi: 10.1037/h0035722. [ CrossRef ] [ Google Scholar ]
- Moos RH. Connections between school, work and family settings. In: Fraser BJ, Walberg HJ, editors. Educational environments: Evaluation, antecedents and consequences. Pergamon Press; 1991. pp. 29–53. [ Google Scholar ]
- O’Shea S, Stone C, Delahunty J. “I ‘feel’ like I am at university even though I am online.” Exploring how students narrate their engagement with higher education institutions in an online learning environment. Distance Education. 2015; 36 (1):41–58. doi: 10.1080/01587919.2015.1019970. [ CrossRef ] [ Google Scholar ]
- Rusticus SA, Justus B. Comparing student- and teacher-formed teams on group dynamics, satisfaction and performance. Small Group Research. 2019; 50 (4):443–457. doi: 10.1177/1046496419854520. [ CrossRef ] [ Google Scholar ]
- Rusticus, S. A., Wilson, D., Casiro, O., & Lovato, C. (2020). Evaluating the quality of health professions learning environments: development and validation of the health education learning environment survey (HELES). Evaluation & the health professions, 43 (3), 162–168. 10.1177/0163278719834339 [ PubMed ]
- Rusticus SA, Worthington A, Wilson DC, Joughin K. The Medical School Learning Environment Survey: An examination of its factor structure and relationship to student performance and satisfaction. Learning Environments Research. 2014; 17 :423–435. doi: 10.1007/s10984-014-9167-9. [ CrossRef ] [ Google Scholar ]
- Sadera WA, Robertson J, Song L, Midon MN. The role of community in online learning success. Journal of Online Learning and Teaching. 2009; 5 (2):277–284. [ Google Scholar ]
- Schönrock-Adema J, Bouwkamp-Timmer T, van Hell EA, Cohen-Schotanus J. Key elements in assessing the educational environment: Where is the theory? Advances in Health Sciences Education. 2012; 17 :727–742. doi: 10.1007/s10459-011-9346-8. [ PMC free article ] [ PubMed ] [ CrossRef ] [ Google Scholar ]
- Schussler, E. E., Weatherton, M., Chen Musgrove, M. M., Brigati, J. R., & England, B. J. (2021). Student perceptions of instructor supportiveness: What characteristics make a difference? CBE—Life Sciences Education , 20 (2), Article 29. [ PMC free article ] [ PubMed ]
- Seidel T. The role of student characteristics in studying micro teaching–learning environments. Learning Environments Research. 2006; 9 (3):253–271. doi: 10.1016/j.learninstruc.2005.08.004. [ CrossRef ] [ Google Scholar ]
- Shochet RB, Colbert-Getz JM, Levine RB, Wright SM. Gauging events that influence students’ perceptions of the medical school learning environment: Findings from one institution. Academic Medicine. 2013; 88 :246–252. doi: 10.1097/ACM.0b013e31827bfa14. [ PubMed ] [ CrossRef ] [ Google Scholar ]
- Tharani A, Husain Y, Warwick I. Learning environment and emotional well-being: A qualitative study of undergraduate nursing students. Nurse Education Today. 2017; 59 :82–87. doi: 10.1016/j.nedt.2017.09.008. [ PubMed ] [ CrossRef ] [ Google Scholar ]
- Vermeulen L, Schmidt HG. Learning environment, learning process, academic outcomes and career success of university graduates. Studies in Higher Education. 2008; 33 (4):431–451. doi: 10.1080/03075070802211810. [ CrossRef ] [ Google Scholar ]
- Weidinger AF, Spinath B, Steinmayr R. Why does intrinsic motivation decline following negative feedback? The mediating role of ability self-concept and its moderation by goal orientations. Learning and Individual Differences. 2016; 47 :117–128. doi: 10.1016/j.lindif.2016.01.003. [ CrossRef ] [ Google Scholar ]
Reflective Essay on Learning and Teaching
- October 2019

Discover the world's research
- 25+ million members
- 160+ million publication pages
- 2.3+ billion citations

- Susulawati Susilawati

- Vachira Jantarach

- Shenggao Wang

- Thomas J. Shuell
- D W Johnson
- R T Johnson
- F C Lunenburg
- Recruit researchers
- Join for free
- Login Email Tip: Most researchers use their institutional email address as their ResearchGate login Password Forgot password? Keep me logged in Log in or Continue with Google Welcome back! Please log in. Email · Hint Tip: Most researchers use their institutional email address as their ResearchGate login Password Forgot password? Keep me logged in Log in or Continue with Google No account? Sign up
Home — Essay Samples — Life — Personal Growth and Development — Reflective My Learning Experience

Reflective My Learning Experience
- Categories: Personal Growth and Development
About this sample

Words: 595 |
Published: Mar 19, 2024
Words: 595 | Page: 1 | 3 min read
Table of contents
Introduction.
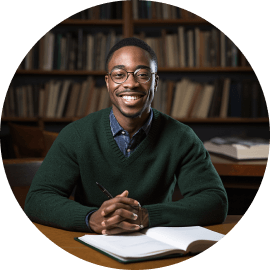
Cite this Essay
To export a reference to this article please select a referencing style below:
Let us write you an essay from scratch
- 450+ experts on 30 subjects ready to help
- Custom essay delivered in as few as 3 hours
Get high-quality help
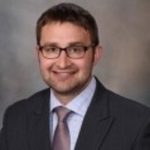
Dr. Heisenberg
Verified writer
- Expert in: Life
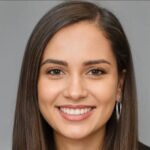
+ 120 experts online
By clicking “Check Writers’ Offers”, you agree to our terms of service and privacy policy . We’ll occasionally send you promo and account related email
No need to pay just yet!
Related Essays
1 pages / 647 words
3 pages / 1206 words
1 pages / 673 words
1 pages / 649 words
Remember! This is just a sample.
You can get your custom paper by one of our expert writers.
121 writers online
Still can’t find what you need?
Browse our vast selection of original essay samples, each expertly formatted and styled
Related Essays on Personal Growth and Development
Throughout life, individuals encounter a myriad of unique opportunities and challenges that can profoundly shape their journeys. These experiences, whether they involve overcoming adversity, pursuing a passion, or taking on a [...]
The concept of coming of age is a universal and timeless theme that has captured the imaginations of storytellers and readers alike. This essay delves into the multifaceted nature of coming of age and its profound relevance to [...]
Self-evaluation is a reflective process that holds the key to personal and professional growth. This essay explores the significance of self-evaluation as a tool for enhancing one's capabilities and achieving goals. Drawing from [...]
Personal responsibility is an essential aspect of individual growth and development as it enables individuals to take ownership of their actions and make decisions that align with their values and goals. In this essay, I will [...]
Dealing with an abusive father, vicious dogs, being chased by a crowd of angry southerners are among the many obstacles Huck Finn faces in his journey to personal salvation, but more explicitly, the saving of his friend Jim. [...]
I am writing this statement of purpose in support of my application for admission to MBA program at University Canada West. I have chosen Canada for my study destination for many reasons including reasonable living and education [...]
Related Topics
By clicking “Send”, you agree to our Terms of service and Privacy statement . We will occasionally send you account related emails.
Where do you want us to send this sample?
By clicking “Continue”, you agree to our terms of service and privacy policy.
Be careful. This essay is not unique
This essay was donated by a student and is likely to have been used and submitted before
Download this Sample
Free samples may contain mistakes and not unique parts
Sorry, we could not paraphrase this essay. Our professional writers can rewrite it and get you a unique paper.
Please check your inbox.
We can write you a custom essay that will follow your exact instructions and meet the deadlines. Let's fix your grades together!
Get Your Personalized Essay in 3 Hours or Less!
We use cookies to personalyze your web-site experience. By continuing we’ll assume you board with our cookie policy .
- Instructions Followed To The Letter
- Deadlines Met At Every Stage
- Unique And Plagiarism Free
- Tutorial Review
- Open access
- Published: 24 January 2018
Teaching the science of learning
- Yana Weinstein ORCID: orcid.org/0000-0002-5144-968X 1 ,
- Christopher R. Madan 2 , 3 &
- Megan A. Sumeracki 4
Cognitive Research: Principles and Implications volume 3 , Article number: 2 ( 2018 ) Cite this article
267k Accesses
101 Citations
756 Altmetric
Metrics details
The science of learning has made a considerable contribution to our understanding of effective teaching and learning strategies. However, few instructors outside of the field are privy to this research. In this tutorial review, we focus on six specific cognitive strategies that have received robust support from decades of research: spaced practice, interleaving, retrieval practice, elaboration, concrete examples, and dual coding. We describe the basic research behind each strategy and relevant applied research, present examples of existing and suggested implementation, and make recommendations for further research that would broaden the reach of these strategies.
Significance
Education does not currently adhere to the medical model of evidence-based practice (Roediger, 2013 ). However, over the past few decades, our field has made significant advances in applying cognitive processes to education. From this work, specific recommendations can be made for students to maximize their learning efficiency (Dunlosky, Rawson, Marsh, Nathan, & Willingham, 2013 ; Roediger, Finn, & Weinstein, 2012 ). In particular, a review published 10 years ago identified a limited number of study techniques that have received solid evidence from multiple replications testing their effectiveness in and out of the classroom (Pashler et al., 2007 ). A recent textbook analysis (Pomerance, Greenberg, & Walsh, 2016 ) took the six key learning strategies from this report by Pashler and colleagues, and found that very few teacher-training textbooks cover any of these six principles – and none cover them all, suggesting that these strategies are not systematically making their way into the classroom. This is the case in spite of multiple recent academic (e.g., Dunlosky et al., 2013 ) and general audience (e.g., Dunlosky, 2013 ) publications about these strategies. In this tutorial review, we present the basic science behind each of these six key principles, along with more recent research on their effectiveness in live classrooms, and suggest ideas for pedagogical implementation. The target audience of this review is (a) educators who might be interested in integrating the strategies into their teaching practice, (b) science of learning researchers who are looking for open questions to help determine future research priorities, and (c) researchers in other subfields who are interested in the ways that principles from cognitive psychology have been applied to education.
While the typical teacher may not be exposed to this research during teacher training, a small cohort of teachers intensely interested in cognitive psychology has recently emerged. These teachers are mainly based in the UK, and, anecdotally (e.g., Dennis (2016), personal communication), appear to have taken an interest in the science of learning after reading Make it Stick (Brown, Roediger, & McDaniel, 2014 ; see Clark ( 2016 ) for an enthusiastic review of this book on a teacher’s blog, and “Learning Scientists” ( 2016c ) for a collection). In addition, a grassroots teacher movement has led to the creation of “researchED” – a series of conferences on evidence-based education (researchED, 2013 ). The teachers who form part of this network frequently discuss cognitive psychology techniques and their applications to education on social media (mainly Twitter; e.g., Fordham, 2016 ; Penfound, 2016 ) and on their blogs, such as Evidence Into Practice ( https://evidenceintopractice.wordpress.com/ ), My Learning Journey ( http://reflectionsofmyteaching.blogspot.com/ ), and The Effortful Educator ( https://theeffortfuleducator.com/ ). In general, the teachers who write about these issues pay careful attention to the relevant literature, often citing some of the work described in this review.
These informal writings, while allowing teachers to explore their approach to teaching practice (Luehmann, 2008 ), give us a unique window into the application of the science of learning to the classroom. By examining these blogs, we can not only observe how basic cognitive research is being applied in the classroom by teachers who are reading it, but also how it is being misapplied, and what questions teachers may be posing that have gone unaddressed in the scientific literature. Throughout this review, we illustrate each strategy with examples of how it can be implemented (see Table 1 and Figs. 1 , 2 , 3 , 4 , 5 , 6 and 7 ), as well as with relevant teacher blog posts that reflect on its application, and draw upon this work to pin-point fruitful avenues for further basic and applied research.
Spaced practice schedule for one week. This schedule is designed to represent a typical timetable of a high-school student. The schedule includes four one-hour study sessions, one longer study session on the weekend, and one rest day. Notice that each subject is studied one day after it is covered in school, to create spacing between classes and study sessions. Copyright note: this image was produced by the authors
a Blocked practice and interleaved practice with fraction problems. In the blocked version, students answer four multiplication problems consecutively. In the interleaved version, students answer a multiplication problem followed by a division problem and then an addition problem, before returning to multiplication. For an experiment with a similar setup, see Patel et al. ( 2016 ). Copyright note: this image was produced by the authors. b Illustration of interleaving and spacing. Each color represents a different homework topic. Interleaving involves alternating between topics, rather than blocking. Spacing involves distributing practice over time, rather than massing. Interleaving inherently involves spacing as other tasks naturally “fill” the spaces between interleaved sessions. Copyright note: this image was produced by the authors, adapted from Rohrer ( 2012 )
Concept map illustrating the process and resulting benefits of retrieval practice. Retrieval practice involves the process of withdrawing learned information from long-term memory into working memory, which requires effort. This produces direct benefits via the consolidation of learned information, making it easier to remember later and causing improvements in memory, transfer, and inferences. Retrieval practice also produces indirect benefits of feedback to students and teachers, which in turn can lead to more effective study and teaching practices, with a focus on information that was not accurately retrieved. Copyright note: this figure originally appeared in a blog post by the first and third authors ( http://www.learningscientists.org/blog/2016/4/1-1 )
Illustration of “how” and “why” questions (i.e., elaborative interrogation questions) students might ask while studying the physics of flight. To help figure out how physics explains flight, students might ask themselves the following questions: “How does a plane take off?”; “Why does a plane need an engine?”; “How does the upward force (lift) work?”; “Why do the wings have a curved upper surface and a flat lower surface?”; and “Why is there a downwash behind the wings?”. Copyright note: the image of the plane was downloaded from Pixabay.com and is free to use, modify, and share
Three examples of physics problems that would be categorized differently by novices and experts. The problems in ( a ) and ( c ) look similar on the surface, so novices would group them together into one category. Experts, however, will recognize that the problems in ( b ) and ( c ) both relate to the principle of energy conservation, and so will group those two problems into one category instead. Copyright note: the figure was produced by the authors, based on figures in Chi et al. ( 1981 )
Example of how to enhance learning through use of a visual example. Students might view this visual representation of neural communications with the words provided, or they could draw a similar visual representation themselves. Copyright note: this figure was produced by the authors
Example of word properties associated with visual, verbal, and motor coding for the word “SPOON”. A word can evoke multiple types of representation (“codes” in dual coding theory). Viewing a word will automatically evoke verbal representations related to its component letters and phonemes. Words representing objects (i.e., concrete nouns) will also evoke visual representations, including information about similar objects, component parts of the object, and information about where the object is typically found. In some cases, additional codes can also be evoked, such as motor-related properties of the represented object, where contextual information related to the object’s functional intention and manipulation action may also be processed automatically when reading the word. Copyright note: this figure was produced by the authors and is based on Aylwin ( 1990 ; Fig. 2 ) and Madan and Singhal ( 2012a , Fig. 3 )
Spaced practice
The benefits of spaced (or distributed) practice to learning are arguably one of the strongest contributions that cognitive psychology has made to education (Kang, 2016 ). The effect is simple: the same amount of repeated studying of the same information spaced out over time will lead to greater retention of that information in the long run, compared with repeated studying of the same information for the same amount of time in one study session. The benefits of distributed practice were first empirically demonstrated in the 19 th century. As part of his extensive investigation into his own memory, Ebbinghaus ( 1885/1913 ) found that when he spaced out repetitions across 3 days, he could almost halve the number of repetitions necessary to relearn a series of 12 syllables in one day (Chapter 8). He thus concluded that “a suitable distribution of [repetitions] over a space of time is decidedly more advantageous than the massing of them at a single time” (Section 34). For those who want to read more about Ebbinghaus’s contribution to memory research, Roediger ( 1985 ) provides an excellent summary.
Since then, hundreds of studies have examined spacing effects both in the laboratory and in the classroom (Kang, 2016 ). Spaced practice appears to be particularly useful at large retention intervals: in the meta-analysis by Cepeda, Pashler, Vul, Wixted, and Rohrer ( 2006 ), all studies with a retention interval longer than a month showed a clear benefit of distributed practice. The “new theory of disuse” (Bjork & Bjork, 1992 ) provides a helpful mechanistic explanation for the benefits of spacing to learning. This theory posits that memories have both retrieval strength and storage strength. Whereas retrieval strength is thought to measure the ease with which a memory can be recalled at a given moment, storage strength (which cannot be measured directly) represents the extent to which a memory is truly embedded in the mind. When studying is taking place, both retrieval strength and storage strength receive a boost. However, the extent to which storage strength is boosted depends upon retrieval strength, and the relationship is negative: the greater the current retrieval strength, the smaller the gains in storage strength. Thus, the information learned through “cramming” will be rapidly forgotten due to high retrieval strength and low storage strength (Bjork & Bjork, 2011 ), whereas spacing out learning increases storage strength by allowing retrieval strength to wane before restudy.
Teachers can introduce spacing to their students in two broad ways. One involves creating opportunities to revisit information throughout the semester, or even in future semesters. This does involve some up-front planning, and can be difficult to achieve, given time constraints and the need to cover a set curriculum. However, spacing can be achieved with no great costs if teachers set aside a few minutes per class to review information from previous lessons. The second method involves putting the onus to space on the students themselves. Of course, this would work best with older students – high school and above. Because spacing requires advance planning, it is crucial that the teacher helps students plan their studying. For example, teachers could suggest that students schedule study sessions on days that alternate with the days on which a particular class meets (e.g., schedule review sessions for Tuesday and Thursday when the class meets Monday and Wednesday; see Fig. 1 for a more complete weekly spaced practice schedule). It important to note that the spacing effect refers to information that is repeated multiple times, rather than the idea of studying different material in one long session versus spaced out in small study sessions over time. However, for teachers and particularly for students planning a study schedule, the subtle difference between the two situations (spacing out restudy opportunities, versus spacing out studying of different information over time) may be lost. Future research should address the effects of spacing out studying of different information over time, whether the same considerations apply in this situation as compared to spacing out restudy opportunities, and how important it is for teachers and students to understand the difference between these two types of spaced practice.
It is important to note that students may feel less confident when they space their learning (Bjork, 1999 ) than when they cram. This is because spaced learning is harder – but it is this “desirable difficulty” that helps learning in the long term (Bjork, 1994 ). Students tend to cram for exams rather than space out their learning. One explanation for this is that cramming does “work”, if the goal is only to pass an exam. In order to change students’ minds about how they schedule their studying, it might be important to emphasize the value of retaining information beyond a final exam in one course.
Ideas for how to apply spaced practice in teaching have appeared in numerous teacher blogs (e.g., Fawcett, 2013 ; Kraft, 2015 ; Picciotto, 2009 ). In England in particular, as of 2013, high-school students need to be able to remember content from up to 3 years back on cumulative exams (General Certificate of Secondary Education (GCSE) and A-level exams; see CIFE, 2012 ). A-levels in particular determine what subject students study in university and which programs they are accepted into, and thus shape the path of their academic career. A common approach for dealing with these exams has been to include a “revision” (i.e., studying or cramming) period of a few weeks leading up to the high-stakes cumulative exams. Now, teachers who follow cognitive psychology are advocating a shift of priorities to spacing learning over time across the 3 years, rather than teaching a topic once and then intensely reviewing it weeks before the exam (Cox, 2016a ; Wood, 2017 ). For example, some teachers have suggested using homework assignments as an opportunity for spaced practice by giving students homework on previous topics (Rose, 2014 ). However, questions remain, such as whether spaced practice can ever be effective enough to completely alleviate the need or utility of a cramming period (Cox, 2016b ), and how one can possibly figure out the optimal lag for spacing (Benney, 2016 ; Firth, 2016 ).
There has been considerable research on the question of optimal lag, and much of it is quite complex; two sessions neither too close together (i.e., cramming) nor too far apart are ideal for retention. In a large-scale study, Cepeda, Vul, Rohrer, Wixted, and Pashler ( 2008 ) examined the effects of the gap between study sessions and the interval between study and test across long periods, and found that the optimal gap between study sessions was contingent on the retention interval. Thus, it is not clear how teachers can apply the complex findings on lag to their own classrooms.
A useful avenue of research would be to simplify the research paradigms that are used to study optimal lag, with the goal of creating a flexible, spaced-practice framework that teachers could apply and tailor to their own teaching needs. For example, an Excel macro spreadsheet was recently produced to help teachers plan for lagged lessons (Weinstein-Jones & Weinstein, 2017 ; see Weinstein & Weinstein-Jones ( 2017 ) for a description of the algorithm used in the spreadsheet), and has been used by teachers to plan their lessons (Penfound, 2017 ). However, one teacher who found this tool helpful also wondered whether the more sophisticated plan was any better than his own method of manually selecting poorly understood material from previous classes for later review (Lovell, 2017 ). This direction is being actively explored within personalized online learning environments (Kornell & Finn, 2016 ; Lindsey, Shroyer, Pashler, & Mozer, 2014 ), but teachers in physical classrooms might need less technologically-driven solutions to teach cohorts of students.
It seems teachers would greatly appreciate a set of guidelines for how to implement spacing in the curriculum in the most effective, but also the most efficient manner. While the cognitive field has made great advances in terms of understanding the mechanisms behind spacing, what teachers need more of are concrete evidence-based tools and guidelines for direct implementation in the classroom. These could include more sophisticated and experimentally tested versions of the software described above (Weinstein-Jones & Weinstein, 2017 ), or adaptable templates of spaced curricula. Moreover, researchers need to evaluate the effectiveness of these tools in a real classroom environment, over a semester or academic year, in order to give pedagogically relevant evidence-based recommendations to teachers.
Interleaving
Another scheduling technique that has been shown to increase learning is interleaving. Interleaving occurs when different ideas or problem types are tackled in a sequence, as opposed to the more common method of attempting multiple versions of the same problem in a given study session (known as blocking). Interleaving as a principle can be applied in many different ways. One such way involves interleaving different types of problems during learning, which is particularly applicable to subjects such as math and physics (see Fig. 2 a for an example with fractions, based on a study by Patel, Liu, & Koedinger, 2016 ). For example, in a study with college students, Rohrer and Taylor ( 2007 ) found that shuffling math problems that involved calculating the volume of different shapes resulted in better test performance 1 week later than when students answered multiple problems about the same type of shape in a row. This pattern of results has also been replicated with younger students, for example 7 th grade students learning to solve graph and slope problems (Rohrer, Dedrick, & Stershic, 2015 ). The proposed explanation for the benefit of interleaving is that switching between different problem types allows students to acquire the ability to choose the right method for solving different types of problems rather than learning only the method itself, and not when to apply it.
Do the benefits of interleaving extend beyond problem solving? The answer appears to be yes. Interleaving can be helpful in other situations that require discrimination, such as inductive learning. Kornell and Bjork ( 2008 ) examined the effects of interleaving in a task that might be pertinent to a student of the history of art: the ability to match paintings to their respective painters. Students who studied different painters’ paintings interleaved at study were more successful on a later identification test than were participants who studied the paintings blocked by painter. Birnbaum, Kornell, Bjork, and Bjork ( 2013 ) proposed the discriminative-contrast hypothesis to explain that interleaving enhances learning by allowing the comparison between exemplars of different categories. They found support for this hypothesis in a set of experiments with bird categorization: participants benefited from interleaving and also from spacing, but not when the spacing interrupted side-by-side comparisons of birds from different categories.
Another type of interleaving involves the interleaving of study and test opportunities. This type of interleaving has been applied, once again, to problem solving, whereby students alternate between attempting a problem and viewing a worked example (Trafton & Reiser, 1993 ); this pattern appears to be superior to answering a string of problems in a row, at least with respect to the amount of time it takes to achieve mastery of a procedure (Corbett, Reed, Hoffmann, MacLaren, & Wagner, 2010 ). The benefits of interleaving study and test opportunities – rather than blocking study followed by attempting to answer problems or questions – might arise due to a process known as “test-potentiated learning”. That is, a study opportunity that immediately follows a retrieval attempt may be more fruitful than when that same studying was not preceded by retrieval (Arnold & McDermott, 2013 ).
For problem-based subjects, the interleaving technique is straightforward: simply mix questions on homework and quizzes with previous materials (which takes care of spacing as well); for languages, mix vocabulary themes rather than blocking by theme (Thomson & Mehring, 2016 ). But interleaving as an educational strategy ought to be presented to teachers with some caveats. Research has focused on interleaving material that is somewhat related (e.g., solving different mathematical equations, Rohrer et al., 2015 ), whereas students sometimes ask whether they should interleave material from different subjects – a practice that has not received empirical support (Hausman & Kornell, 2014 ). When advising students how to study independently, teachers should thus proceed with caution. Since it is easy for younger students to confuse this type of unhelpful interleaving with the more helpful interleaving of related information, it may be best for teachers of younger grades to create opportunities for interleaving in homework and quiz assignments rather than putting the onus on the students themselves to make use of the technique. Technology can be very helpful here, with apps such as Quizlet, Memrise, Anki, Synap, Quiz Champ, and many others (see also “Learning Scientists”, 2017 ) that not only allow instructor-created quizzes to be taken by students, but also provide built-in interleaving algorithms so that the burden does not fall on the teacher or the student to carefully plan which items are interleaved when.
An important point to consider is that in educational practice, the distinction between spacing and interleaving can be difficult to delineate. The gap between the scientific and classroom definitions of interleaving is demonstrated by teachers’ own writings about this technique. When they write about interleaving, teachers often extend the term to connote a curriculum that involves returning to topics multiple times throughout the year (e.g., Kirby, 2014 ; see “Learning Scientists” ( 2016a ) for a collection of similar blog posts by several other teachers). The “interleaving” of topics throughout the curriculum produces an effect that is more akin to what cognitive psychologists call “spacing” (see Fig. 2 b for a visual representation of the difference between interleaving and spacing). However, cognitive psychologists have not examined the effects of structuring the curriculum in this way, and open questions remain: does repeatedly circling back to previous topics throughout the semester interrupt the learning of new information? What are some effective techniques for interleaving old and new information within one class? And how does one determine the balance between old and new information?
Retrieval practice
While tests are most often used in educational settings for assessment, a lesser-known benefit of tests is that they actually improve memory of the tested information. If we think of our memories as libraries of information, then it may seem surprising that retrieval (which happens when we take a test) improves memory; however, we know from a century of research that retrieving knowledge actually strengthens it (see Karpicke, Lehman, & Aue, 2014 ). Testing was shown to strengthen memory as early as 100 years ago (Gates, 1917 ), and there has been a surge of research in the last decade on the mnemonic benefits of testing, or retrieval practice . Most of the research on the effectiveness of retrieval practice has been done with college students (see Roediger & Karpicke, 2006 ; Roediger, Putnam, & Smith, 2011 ), but retrieval-based learning has been shown to be effective at producing learning for a wide range of ages, including preschoolers (Fritz, Morris, Nolan, & Singleton, 2007 ), elementary-aged children (e.g., Karpicke, Blunt, & Smith, 2016 ; Karpicke, Blunt, Smith, & Karpicke, 2014 ; Lipko-Speed, Dunlosky, & Rawson, 2014 ; Marsh, Fazio, & Goswick, 2012 ; Ritchie, Della Sala, & McIntosh, 2013 ), middle-school students (e.g., McDaniel, Thomas, Agarwal, McDermott, & Roediger, 2013 ; McDermott, Agarwal, D’Antonio, Roediger, & McDaniel, 2014 ), and high-school students (e.g., McDermott et al., 2014 ). In addition, the effectiveness of retrieval-based learning has been extended beyond simple testing to other activities in which retrieval practice can be integrated, such as concept mapping (Blunt & Karpicke, 2014 ; Karpicke, Blunt, et al., 2014 ; Ritchie et al., 2013 ).
A debate is currently ongoing as to the effectiveness of retrieval practice for more complex materials (Karpicke & Aue, 2015 ; Roelle & Berthold, 2017 ; Van Gog & Sweller, 2015 ). Practicing retrieval has been shown to improve the application of knowledge to new situations (e.g., Butler, 2010 ; Dirkx, Kester, & Kirschner, 2014 ); McDaniel et al., 2013 ; Smith, Blunt, Whiffen, & Karpicke, 2016 ); but see Tran, Rohrer, and Pashler ( 2015 ) and Wooldridge, Bugg, McDaniel, and Liu ( 2014 ), for retrieval practice studies that showed limited or no increased transfer compared to restudy. Retrieval practice effects on higher-order learning may be more sensitive than fact learning to encoding factors, such as the way material is presented during study (Eglington & Kang, 2016 ). In addition, retrieval practice may be more beneficial for higher-order learning if it includes more scaffolding (Fiechter & Benjamin, 2017 ; but see Smith, Blunt, et al., 2016 ) and targeted practice with application questions (Son & Rivas, 2016 ).
How does retrieval practice help memory? Figure 3 illustrates both the direct and indirect benefits of retrieval practice identified by the literature. The act of retrieval itself is thought to strengthen memory (Karpicke, Blunt, et al., 2014 ; Roediger & Karpicke, 2006 ; Smith, Roediger, & Karpicke, 2013 ). For example, Smith et al. ( 2013 ) showed that if students brought information to mind without actually producing it (covert retrieval), they remembered the information just as well as if they overtly produced the retrieved information (overt retrieval). Importantly, both overt and covert retrieval practice improved memory over control groups without retrieval practice, even when feedback was not provided. The fact that bringing information to mind in the absence of feedback or restudy opportunities improves memory leads researchers to conclude that it is the act of retrieval – thinking back to bring information to mind – that improves memory of that information.
The benefit of retrieval practice depends to a certain extent on successful retrieval (see Karpicke, Lehman, et al., 2014 ). For example, in Experiment 4 of Smith et al. ( 2013 ), students successfully retrieved 72% of the information during retrieval practice. Of course, retrieving 72% of the information was compared to a restudy control group, during which students were re-exposed to 100% of the information, creating a bias in favor of the restudy condition. Yet retrieval led to superior memory later compared to the restudy control. However, if retrieval success is extremely low, then it is unlikely to improve memory (e.g., Karpicke, Blunt, et al., 2014 ), particularly in the absence of feedback. On the other hand, if retrieval-based learning situations are constructed in such a way that ensures high levels of success, the act of bringing the information to mind may be undermined, thus making it less beneficial. For example, if a student reads a sentence and then immediately covers the sentence and recites it out loud, they are likely not retrieving the information but rather just keeping the information in their working memory long enough to recite it again (see Smith, Blunt, et al., 2016 for a discussion of this point). Thus, it is important to balance success of retrieval with overall difficulty in retrieving the information (Smith & Karpicke, 2014 ; Weinstein, Nunes, & Karpicke, 2016 ). If initial retrieval success is low, then feedback can help improve the overall benefit of practicing retrieval (Kang, McDermott, & Roediger, 2007 ; Smith & Karpicke, 2014 ). Kornell, Klein, and Rawson ( 2015 ), however, found that it was the retrieval attempt and not the correct production of information that produced the retrieval practice benefit – as long as the correct answer was provided after an unsuccessful attempt, the benefit was the same as for a successful retrieval attempt in this set of studies. From a practical perspective, it would be helpful for teachers to know when retrieval attempts in the absence of success are helpful, and when they are not. There may also be additional reasons beyond retrieval benefits that would push teachers towards retrieval practice activities that produce some success amongst students; for example, teachers may hesitate to give students retrieval practice exercises that are too difficult, as this may negatively affect self-efficacy and confidence.
In addition to the fact that bringing information to mind directly improves memory for that information, engaging in retrieval practice can produce indirect benefits as well (see Roediger et al., 2011 ). For example, research by Weinstein, Gilmore, Szpunar, and McDermott ( 2014 ) demonstrated that when students expected to be tested, the increased test expectancy led to better-quality encoding of new information. Frequent testing can also serve to decrease mind-wandering – that is, thoughts that are unrelated to the material that students are supposed to be studying (Szpunar, Khan, & Schacter, 2013 ).
Practicing retrieval is a powerful way to improve meaningful learning of information, and it is relatively easy to implement in the classroom. For example, requiring students to practice retrieval can be as simple as asking students to put their class materials away and try to write out everything they know about a topic. Retrieval-based learning strategies are also flexible. Instructors can give students practice tests (e.g., short-answer or multiple-choice, see Smith & Karpicke, 2014 ), provide open-ended prompts for the students to recall information (e.g., Smith, Blunt, et al., 2016 ) or ask their students to create concept maps from memory (e.g., Blunt & Karpicke, 2014 ). In one study, Weinstein et al. ( 2016 ) looked at the effectiveness of inserting simple short-answer questions into online learning modules to see whether they improved student performance. Weinstein and colleagues also manipulated the placement of the questions. For some students, the questions were interspersed throughout the module, and for other students the questions were all presented at the end of the module. Initial success on the short-answer questions was higher when the questions were interspersed throughout the module. However, on a later test of learning from that module, the original placement of the questions in the module did not matter for performance. As with spaced practice, where the optimal gap between study sessions is contingent on the retention interval, the optimum difficulty and level of success during retrieval practice may also depend on the retention interval. Both groups of students who answered questions performed better on the delayed test compared to a control group without question opportunities during the module. Thus, the important thing is for instructors to provide opportunities for retrieval practice during learning. Based on previous research, any activity that promotes the successful retrieval of information should improve learning.
Retrieval practice has received a lot of attention in teacher blogs (see “Learning Scientists” ( 2016b ) for a collection). A common theme seems to be an emphasis on low-stakes (Young, 2016 ) and even no-stakes (Cox, 2015 ) testing, the goal of which is to increase learning rather than assess performance. In fact, one well-known charter school in the UK has an official homework policy grounded in retrieval practice: students are to test themselves on subject knowledge for 30 minutes every day in lieu of standard homework (Michaela Community School, 2014 ). The utility of homework, particularly for younger children, is often a hotly debated topic outside of academia (e.g., Shumaker, 2016 ; but see Jones ( 2016 ) for an opposing viewpoint and Cooper ( 1989 ) for the original research the blog posts were based on). Whereas some research shows clear links between homework and academic achievement (Valle et al., 2016 ), other researchers have questioned the effectiveness of homework (Dettmers, Trautwein, & Lüdtke, 2009 ). Perhaps amending homework to involve retrieval practice might make it more effective; this remains an open empirical question.
One final consideration is that of test anxiety. While retrieval practice can be very powerful at improving memory, some research shows that pressure during retrieval can undermine some of the learning benefit. For example, Hinze and Rapp ( 2014 ) manipulated pressure during quizzing to create high-pressure and low-pressure conditions. On the quizzes themselves, students performed equally well. However, those in the high-pressure condition did not perform as well on a criterion test later compared to the low-pressure group. Thus, test anxiety may reduce the learning benefit of retrieval practice. Eliminating all high-pressure tests is probably not possible, but instructors can provide a number of low-stakes retrieval opportunities for students to help increase learning. The use of low-stakes testing can serve to decrease test anxiety (Khanna, 2015 ), and has recently been shown to negate the detrimental impact of stress on learning (Smith, Floerke, & Thomas, 2016 ). This is a particularly important line of inquiry to pursue for future research, because many teachers who are not familiar with the effectiveness of retrieval practice may be put off by the implied pressure of “testing”, which evokes the much maligned high-stakes standardized tests (e.g., McHugh, 2013 ).
Elaboration
Elaboration involves connecting new information to pre-existing knowledge. Anderson ( 1983 , p.285) made the following claim about elaboration: “One of the most potent manipulations that can be performed in terms of increasing a subject’s memory for material is to have the subject elaborate on the to-be-remembered material.” Postman ( 1976 , p. 28) defined elaboration most parsimoniously as “additions to nominal input”, and Hirshman ( 2001 , p. 4369) provided an elaboration on this definition (pun intended!), defining elaboration as “A conscious, intentional process that associates to-be-remembered information with other information in memory.” However, in practice, elaboration could mean many different things. The common thread in all the definitions is that elaboration involves adding features to an existing memory.
One possible instantiation of elaboration is thinking about information on a deeper level. The levels (or “depth”) of processing framework, proposed by Craik and Lockhart ( 1972 ), predicts that information will be remembered better if it is processed more deeply in terms of meaning, rather than shallowly in terms of form. The leves of processing framework has, however, received a number of criticisms (Craik, 2002 ). One major problem with this framework is that it is difficult to measure “depth”. And if we are not able to actually measure depth, then the argument can become circular: is it that something was remembered better because it was studied more deeply, or do we conclude that it must have been studied more deeply because it is remembered better? (See Lockhart & Craik, 1990 , for further discussion of this issue).
Another mechanism by which elaboration can confer a benefit to learning is via improvement in organization (Bellezza, Cheesman, & Reddy, 1977 ; Mandler, 1979 ). By this view, elaboration involves making information more integrated and organized with existing knowledge structures. By connecting and integrating the to-be-learned information with other concepts in memory, students can increase the extent to which the ideas are organized in their minds, and this increased organization presumably facilitates the reconstruction of the past at the time of retrieval.
Elaboration is such a broad term and can include so many different techniques that it is hard to claim that elaboration will always help learning. There is, however, a specific technique under the umbrella of elaboration for which there is relatively strong evidence in terms of effectiveness (Dunlosky et al., 2013 ; Pashler et al., 2007 ). This technique is called elaborative interrogation, and involves students questioning the materials that they are studying (Pressley, McDaniel, Turnure, Wood, & Ahmad, 1987 ). More specifically, students using this technique would ask “how” and “why” questions about the concepts they are studying (see Fig. 4 for an example on the physics of flight). Then, crucially, students would try to answer these questions – either from their materials or, eventually, from memory (McDaniel & Donnelly, 1996 ). The process of figuring out the answer to the questions – with some amount of uncertainty (Overoye & Storm, 2015 ) – can help learning. When using this technique, however, it is important that students check their answers with their materials or with the teacher; when the content generated through elaborative interrogation is poor, it can actually hurt learning (Clinton, Alibali, & Nathan, 2016 ).
Students can also be encouraged to self-explain concepts to themselves while learning (Chi, De Leeuw, Chiu, & LaVancher, 1994 ). This might involve students simply saying out loud what steps they need to perform to solve an equation. Aleven and Koedinger ( 2002 ) conducted two classroom studies in which students were either prompted by a “cognitive tutor” to provide self-explanations during a problem-solving task or not, and found that the self-explanations led to improved performance. According to the authors, this approach could scale well to real classrooms. If possible and relevant, students could even perform actions alongside their self-explanations (Cohen, 1981 ; see also the enactment effect, Hainselin, Picard, Manolli, Vankerkore-Candas, & Bourdin, 2017 ). Instructors can scaffold students in these types of activities by providing self-explanation prompts throughout to-be-learned material (O’Neil et al., 2014 ). Ultimately, the greatest potential benefit of accurate self-explanation or elaboration is that the student will be able to transfer their knowledge to a new situation (Rittle-Johnson, 2006 ).
The technical term “elaborative interrogation” has not made it into the vernacular of educational bloggers (a search on https://educationechochamberuncut.wordpress.com , which consolidates over 3,000 UK-based teacher blogs, yielded zero results for that term). However, a few teachers have blogged about elaboration more generally (e.g., Hobbiss, 2016 ) and deep questioning specifically (e.g., Class Teaching, 2013 ), just without using the specific terminology. This strategy in particular may benefit from a more open dialog between researchers and teachers to facilitate the use of elaborative interrogation in the classroom and to address possible barriers to implementation. In terms of advancing the scientific understanding of elaborative interrogation in a classroom setting, it would be informative to conduct a larger-scale intervention to see whether having students elaborate during reading actually helps their understanding. It would also be useful to know whether the students really need to generate their own elaborative interrogation (“how” and “why”) questions, versus answering questions provided by others. How long should students persist to find the answers? When is the right time to have students engage in this task, given the levels of expertise required to do it well (Clinton et al., 2016 )? Without knowing the answers to these questions, it may be too early for us to instruct teachers to use this technique in their classes. Finally, elaborative interrogation takes a long time. Is this time efficiently spent? Or, would it be better to have the students try to answer a few questions, pool their information as a class, and then move to practicing retrieval of the information?
Concrete examples
Providing supporting information can improve the learning of key ideas and concepts. Specifically, using concrete examples to supplement content that is more conceptual in nature can make the ideas easier to understand and remember. Concrete examples can provide several advantages to the learning process: (a) they can concisely convey information, (b) they can provide students with more concrete information that is easier to remember, and (c) they can take advantage of the superior memorability of pictures relative to words (see “Dual Coding”).
Words that are more concrete are both recognized and recalled better than abstract words (Gorman, 1961 ; e.g., “button” and “bound,” respectively). Furthermore, it has been demonstrated that information that is more concrete and imageable enhances the learning of associations, even with abstract content (Caplan & Madan, 2016 ; Madan, Glaholt, & Caplan, 2010 ; Paivio, 1971 ). Following from this, providing concrete examples during instruction should improve retention of related abstract concepts, rather than the concrete examples alone being remembered better. Concrete examples can be useful both during instruction and during practice problems. Having students actively explain how two examples are similar and encouraging them to extract the underlying structure on their own can also help with transfer. In a laboratory study, Berry ( 1983 ) demonstrated that students performed well when given concrete practice problems, regardless of the use of verbalization (akin to elaborative interrogation), but that verbalization helped students transfer understanding from concrete to abstract problems. One particularly important area of future research is determining how students can best make the link between concrete examples and abstract ideas.
Since abstract concepts are harder to grasp than concrete information (Paivio, Walsh, & Bons, 1994 ), it follows that teachers ought to illustrate abstract ideas with concrete examples. However, care must be taken when selecting the examples. LeFevre and Dixon ( 1986 ) provided students with both concrete examples and abstract instructions and found that when these were inconsistent, students followed the concrete examples rather than the abstract instructions, potentially constraining the application of the abstract concept being taught. Lew, Fukawa-Connelly, Mejí-Ramos, and Weber ( 2016 ) used an interview approach to examine why students may have difficulty understanding a lecture. Responses indicated that some issues were related to understanding the overarching topic rather than the component parts, and to the use of informal colloquialisms that did not clearly follow from the material being taught. Both of these issues could have potentially been addressed through the inclusion of a greater number of relevant concrete examples.
One concern with using concrete examples is that students might only remember the examples – especially if they are particularly memorable, such as fun or gimmicky examples – and will not be able to transfer their understanding from one example to another, or more broadly to the abstract concept. However, there does not seem to be any evidence that fun relevant examples actually hurt learning by harming memory for important information. Instead, fun examples and jokes tend to be more memorable, but this boost in memory for the joke does not seem to come at a cost to memory for the underlying concept (Baldassari & Kelley, 2012 ). However, two important caveats need to be highlighted. First, to the extent that the more memorable content is not relevant to the concepts of interest, learning of the target information can be compromised (Harp & Mayer, 1998 ). Thus, care must be taken to ensure that all examples and gimmicks are, in fact, related to the core concepts that the students need to acquire, and do not contain irrelevant perceptual features (Kaminski & Sloutsky, 2013 ).
The second issue is that novices often notice and remember the surface details of an example rather than the underlying structure. Experts, on the other hand, can extract the underlying structure from examples that have divergent surface features (Chi, Feltovich, & Glaser, 1981 ; see Fig. 5 for an example from physics). Gick and Holyoak ( 1983 ) tried to get students to apply a rule from one problem to another problem that appeared different on the surface, but was structurally similar. They found that providing multiple examples helped with this transfer process compared to only using one example – especially when the examples provided had different surface details. More work is also needed to determine how many examples are sufficient for generalization to occur (and this, of course, will vary with contextual factors and individual differences). Further research on the continuum between concrete/specific examples and more abstract concepts would also be informative. That is, if an example is not concrete enough, it may be too difficult to understand. On the other hand, if the example is too concrete, that could be detrimental to generalization to the more abstract concept (although a diverse set of very concrete examples may be able to help with this). In fact, in a controversial article, Kaminski, Sloutsky, and Heckler ( 2008 ) claimed that abstract examples were more effective than concrete examples. Later rebuttals of this paper contested whether the abstract versus concrete distinction was clearly defined in the original study (see Reed, 2008 , for a collection of letters on the subject). This ideal point along the concrete-abstract continuum might also interact with development.
Finding teacher blog posts on concrete examples proved to be more difficult than for the other strategies in this review. One optimistic possibility is that teachers frequently use concrete examples in their teaching, and thus do not think of this as a specific contribution from cognitive psychology; the one blog post we were able to find that discussed concrete examples suggests that this might be the case (Boulton, 2016 ). The idea of “linking abstract concepts with concrete examples” is also covered in 25% of teacher-training textbooks used in the US, according to the report by Pomerance et al. ( 2016 ); this is the second most frequently covered of the six strategies, after “posing probing questions” (i.e., elaborative interrogation). A useful direction for future research would be to establish how teachers are using concrete examples in their practice, and whether we can make any suggestions for improvement based on research into the science of learning. For example, if two examples are better than one (Bauernschmidt, 2017 ), are additional examples also needed, or are there diminishing returns from providing more examples? And, how can teachers best ensure that concrete examples are consistent with prior knowledge (Reed, 2008 )?
Dual coding
Both the memory literature and folk psychology support the notion of visual examples being beneficial—the adage of “a picture is worth a thousand words” (traced back to an advertising slogan from the 1920s; Meider, 1990 ). Indeed, it is well-understood that more information can be conveyed through a simple illustration than through several paragraphs of text (e.g., Barker & Manji, 1989 ; Mayer & Gallini, 1990 ). Illustrations can be particularly helpful when the described concept involves several parts or steps and is intended for individuals with low prior knowledge (Eitel & Scheiter, 2015 ; Mayer & Gallini, 1990 ). Figure 6 provides a concrete example of this, illustrating how information can flow through neurons and synapses.
In addition to being able to convey information more succinctly, pictures are also more memorable than words (Paivio & Csapo, 1969 , 1973 ). In the memory literature, this is referred to as the picture superiority effect , and dual coding theory was developed in part to explain this effect. Dual coding follows from the notion of text being accompanied by complementary visual information to enhance learning. Paivio ( 1971 , 1986 ) proposed dual coding theory as a mechanistic account for the integration of multiple information “codes” to process information. In this theory, a code corresponds to a modal or otherwise distinct representation of a concept—e.g., “mental images for ‘book’ have visual, tactual, and other perceptual qualities similar to those evoked by the referent objects on which the images are based” (Clark & Paivio, 1991 , p. 152). Aylwin ( 1990 ) provides a clear example of how the word “dog” can evoke verbal, visual, and enactive representations (see Fig. 7 for a similar example for the word “SPOON”, based on Aylwin, 1990 (Fig. 2 ) and Madan & Singhal, 2012a (Fig. 3 )). Codes can also correspond to emotional properties (Clark & Paivio, 1991 ; Paivio, 2013 ). Clark and Paivio ( 1991 ) provide a thorough review of dual coding theory and its relation to education, while Paivio ( 2007 ) provides a comprehensive treatise on dual coding theory. Broadly, dual coding theory suggests that providing multiple representations of the same information enhances learning and memory, and that information that more readily evokes additional representations (through automatic imagery processes) receives a similar benefit.
Paivio and Csapo ( 1973 ) suggest that verbal and imaginal codes have independent and additive effects on memory recall. Using visuals to improve learning and memory has been particularly applied to vocabulary learning (Danan, 1992 ; Sadoski, 2005 ), but has also shown success in other domains such as in health care (Hartland, Biddle, & Fallacaro, 2008 ). To take advantage of dual coding, verbal information should be accompanied by a visual representation when possible. However, while the studies discussed all indicate that the use of multiple representations of information is favorable, it is important to acknowledge that each representation also increases cognitive load and can lead to over-saturation (Mayer & Moreno, 2003 ).
Given that pictures are generally remembered better than words, it is important to ensure that the pictures students are provided with are helpful and relevant to the content they are expected to learn. McNeill, Uttal, Jarvin, and Sternberg ( 2009 ) found that providing visual examples decreased conceptual errors. However, McNeill et al. also found that when students were given visually rich examples, they performed more poorly than students who were not given any visual example, suggesting that the visual details can at times become a distraction and hinder performance. Thus, it is important to consider that images used in teaching are clear and not ambiguous in their meaning (Schwartz, 2007 ).
Further broadening the scope of dual coding theory, Engelkamp and Zimmer ( 1984 ) suggest that motor movements, such as “turning the handle,” can provide an additional motor code that can improve memory, linking studies of motor actions (enactment) with dual coding theory (Clark & Paivio, 1991 ; Engelkamp & Cohen, 1991 ; Madan & Singhal, 2012c ). Indeed, enactment effects appear to primarily occur during learning, rather than during retrieval (Peterson & Mulligan, 2010 ). Along similar lines, Wammes, Meade, and Fernandes ( 2016 ) demonstrated that generating drawings can provide memory benefits beyond what could otherwise be explained by visual imagery, picture superiority, and other memory enhancing effects. Providing convergent evidence, even when overt motor actions are not critical in themselves, words representing functional objects have been shown to enhance later memory (Madan & Singhal, 2012b ; Montefinese, Ambrosini, Fairfield, & Mammarella, 2013 ). This indicates that motoric processes can improve memory similarly to visual imagery, similar to memory differences for concrete vs. abstract words. Further research suggests that automatic motor simulation for functional objects is likely responsible for this memory benefit (Madan, Chen, & Singhal, 2016 ).
When teachers combine visuals and words in their educational practice, however, they may not always be taking advantage of dual coding – at least, not in the optimal manner. For example, a recent discussion on Twitter centered around one teacher’s decision to have 7 th Grade students replace certain words in their science laboratory report with a picture of that word (e.g., the instructions read “using a syringe …” and a picture of a syringe replaced the word; Turner, 2016a ). Other teachers argued that this was not dual coding (Beaven, 2016 ; Williams, 2016 ), because there were no longer two different representations of the information. The first teacher maintained that dual coding was preserved, because this laboratory report with pictures was to be used alongside the original, fully verbal report (Turner, 2016b ). This particular implementation – having students replace individual words with pictures – has not been examined in the cognitive literature, presumably because no benefit would be expected. In any case, we need to be clearer about implementations for dual coding, and more research is needed to clarify how teachers can make use of the benefits conferred by multiple representations and picture superiority.
Critically, dual coding theory is distinct from the notion of “learning styles,” which describe the idea that individuals benefit from instruction that matches their modality preference. While this idea is pervasive and individuals often subjectively feel that they have a preference, evidence indicates that the learning styles theory is not supported by empirical findings (e.g., Kavale, Hirshoren, & Forness, 1998 ; Pashler, McDaniel, Rohrer, & Bjork, 2008 ; Rohrer & Pashler, 2012 ). That is, there is no evidence that instructing students in their preferred learning style leads to an overall improvement in learning (the “meshing” hypothesis). Moreover, learning styles have come to be described as a myth or urban legend within psychology (Coffield, Moseley, Hall, & Ecclestone, 2004 ; Hattie & Yates, 2014 ; Kirschner & van Merriënboer, 2013 ; Kirschner, 2017 ); skepticism about learning styles is a common stance amongst evidence-informed teachers (e.g., Saunders, 2016 ). Providing evidence against the notion of learning styles, Kraemer, Rosenberg, and Thompson-Schill ( 2009 ) found that individuals who scored as “verbalizers” and “visualizers” did not perform any better on experimental trials matching their preference. Instead, it has recently been shown that learning through one’s preferred learning style is associated with elevated subjective judgements of learning, but not objective performance (Knoll, Otani, Skeel, & Van Horn, 2017 ). In contrast to learning styles, dual coding is based on providing additional, complementary forms of information to enhance learning, rather than tailoring instruction to individuals’ preferences.
Genuine educational environments present many opportunities for combining the strategies outlined above. Spacing can be particularly potent for learning if it is combined with retrieval practice. The additive benefits of retrieval practice and spacing can be gained by engaging in retrieval practice multiple times (also known as distributed practice; see Cepeda et al., 2006 ). Interleaving naturally entails spacing if students interleave old and new material. Concrete examples can be both verbal and visual, making use of dual coding. In addition, the strategies of elaboration, concrete examples, and dual coding all work best when used as part of retrieval practice. For example, in the concept-mapping studies mentioned above (Blunt & Karpicke, 2014 ; Karpicke, Blunt, et al., 2014 ), creating concept maps while looking at course materials (e.g., a textbook) was not as effective for later memory as creating concept maps from memory. When practicing elaborative interrogation, students can start off answering the “how” and “why” questions they pose for themselves using class materials, and work their way up to answering them from memory. And when interleaving different problem types, students should be practicing answering them rather than just looking over worked examples.
But while these ideas for strategy combinations have empirical bases, it has not yet been established whether the benefits of the strategies to learning are additive, super-additive, or, in some cases, incompatible. Thus, future research needs to (a) better formalize the definition of each strategy (particularly critical for elaboration and dual coding), (b) identify best practices for implementation in the classroom, (c) delineate the boundary conditions of each strategy, and (d) strategically investigate interactions between the six strategies we outlined in this manuscript.
Aleven, V. A., & Koedinger, K. R. (2002). An effective metacognitive strategy: learning by doing and explaining with a computer-based cognitive tutor. Cognitive Science, 26 , 147–179.
Article Google Scholar
Anderson, J. R. (1983). A spreading activation theory of memory. Journal of Verbal Learning and Verbal Behavior, 22 , 261–295.
Arnold, K. M., & McDermott, K. B. (2013). Test-potentiated learning: distinguishing between direct and indirect effects of tests. Journal of Experimental Psychology: Learning, Memory, and Cognition, 39 , 940–945.
PubMed Google Scholar
Aylwin, S. (1990). Imagery and affect: big questions, little answers. In P. J. Thompson, D. E. Marks, & J. T. E. Richardson (Eds.), Imagery: Current developments . New York: International Library of Psychology.
Google Scholar
Baldassari, M. J., & Kelley, M. (2012). Make’em laugh? The mnemonic effect of humor in a speech. Psi Chi Journal of Psychological Research, 17 , 2–9.
Barker, P. G., & Manji, K. A. (1989). Pictorial dialogue methods. International Journal of Man-Machine Studies, 31 , 323–347.
Bauernschmidt, A. (2017). GUEST POST: two examples are better than one. [Blog post]. The Learning Scientists Blog . Retrieved from http://www.learningscientists.org/blog/2017/5/30-1 . Accessed 25 Dec 2017.
Beaven, T. (2016). @doctorwhy @FurtherEdagogy @doc_kristy Right, I thought the whole point of dual coding was to use TWO codes: pics + words of the SAME info? [Tweet]. Retrieved from https://twitter.com/TitaBeaven/status/807504041341308929 . Accessed 25 Dec 2017.
Bellezza, F. S., Cheesman, F. L., & Reddy, B. G. (1977). Organization and semantic elaboration in free recall. Journal of Experimental Psychology: Human Learning and Memory, 3 , 539–550.
Benney, D. (2016). (Trying to apply) spacing in a content heavy subject [Blog post]. Retrieved from https://mrbenney.wordpress.com/2016/10/16/trying-to-apply-spacing-in-science/ . Accessed 25 Dec 2017.
Berry, D. C. (1983). Metacognitive experience and transfer of logical reasoning. Quarterly Journal of Experimental Psychology, 35A , 39–49.
Birnbaum, M. S., Kornell, N., Bjork, E. L., & Bjork, R. A. (2013). Why interleaving enhances inductive learning: the roles of discrimination and retrieval. Memory & Cognition, 41 , 392–402.
Bjork, R. A. (1999). Assessing our own competence: heuristics and illusions. In D. Gopher & A. Koriat (Eds.), Attention and peformance XVII. Cognitive regulation of performance: Interaction of theory and application (pp. 435–459). Cambridge, MA: MIT Press.
Bjork, R. A. (1994). Memory and metamemory considerations in the training of human beings. In J. Metcalfe & A. Shimamura (Eds.), Metacognition: Knowing about knowing (pp. 185–205). Cambridge, MA: MIT Press.
Bjork, R. A., & Bjork, E. L. (1992). A new theory of disuse and an old theory of stimulus fluctuation. From learning processes to cognitive processes: Essays in honor of William K. Estes, 2 , 35–67.
Bjork, E. L., & Bjork, R. A. (2011). Making things hard on yourself, but in a good way: creating desirable difficulties to enhance learning. Psychology and the real world: Essays illustrating fundamental contributions to society , 56–64.
Blunt, J. R., & Karpicke, J. D. (2014). Learning with retrieval-based concept mapping. Journal of Educational Psychology, 106 , 849–858.
Boulton, K. (2016). What does cognitive overload look like in the humanities? [Blog post]. Retrieved from https://educationechochamberuncut.wordpress.com/2016/03/05/what-does-cognitive-overload-look-like-in-the-humanities-kris-boulton-2/ . Accessed 25 Dec 2017.
Brown, P. C., Roediger, H. L., & McDaniel, M. A. (2014). Make it stick . Cambridge, MA: Harvard University Press.
Book Google Scholar
Butler, A. C. (2010). Repeated testing produces superior transfer of learning relative to repeated studying. Journal of Experimental Psychology: Learning, Memory, and Cognition, 36 , 1118–1133.
Caplan, J. B., & Madan, C. R. (2016). Word-imageability enhances association-memory by recruiting hippocampal activity. Journal of Cognitive Neuroscience, 28 , 1522–1538.
Article PubMed Google Scholar
Cepeda, N. J., Pashler, H., Vul, E., Wixted, J. T., & Rohrer, D. (2006). Distributed practice in verbal recall tasks: a review and quantitative synthesis. Psychological Bulletin, 132 , 354–380.
Cepeda, N. J., Vul, E., Rohrer, D., Wixted, J. T., & Pashler, H. (2008). Spacing effects in learning a temporal ridgeline of optimal retention. Psychological Science, 19 , 1095–1102.
Chi, M. T., De Leeuw, N., Chiu, M. H., & LaVancher, C. (1994). Eliciting self-explanations improves understanding. Cognitive Science, 18 , 439–477.
Chi, M. T., Feltovich, P. J., & Glaser, R. (1981). Categorization and representation of physics problems by experts and novices. Cognitive Science, 5 , 121–152.
CIFE. (2012). No January A level and other changes. Retrieved from http://www.cife.org.uk/cife-general-news/no-january-a-level-and-other-changes/ . Accessed 25 Dec 2017.
Clark, D. (2016). One book on learning that every teacher, lecturer & trainer should read (7 reasons) [Blog post]. Retrieved from http://donaldclarkplanb.blogspot.com/2016/03/one-book-on-learning-that-every-teacher.html . Accessed 25 Dec 2017.
Clark, J. M., & Paivio, A. (1991). Dual coding theory and education. Educational Psychology Review, 3 , 149–210.
Class Teaching. (2013). Deep questioning [Blog post]. Retrieved from https://classteaching.wordpress.com/2013/07/12/deep-questioning/ . Accessed 25 Dec 2017.
Clinton, V., Alibali, M. W., & Nathan, M. J. (2016). Learning about posterior probability: do diagrams and elaborative interrogation help? The Journal of Experimental Education, 84 , 579–599.
Coffield, F., Moseley, D., Hall, E., & Ecclestone, K. (2004). Learning styles and pedagogy in post-16 learning: a systematic and critical review . London: Learning & Skills Research Centre.
Cohen, R. L. (1981). On the generality of some memory laws. Scandinavian Journal of Psychology, 22 , 267–281.
Cooper, H. (1989). Synthesis of research on homework. Educational Leadership, 47 , 85–91.
Corbett, A. T., Reed, S. K., Hoffmann, R., MacLaren, B., & Wagner, A. (2010). Interleaving worked examples and cognitive tutor support for algebraic modeling of problem situations. In Proceedings of the Thirty-Second Annual Meeting of the Cognitive Science Society (pp. 2882–2887).
Cox, D. (2015). No stakes testing – not telling students their results [Blog post]. Retrieved from https://missdcoxblog.wordpress.com/2015/06/06/no-stakes-testing-not-telling-students-their-results/ . Accessed 25 Dec 2017.
Cox, D. (2016a). Ditch revision. Teach it well [Blog post]. Retrieved from https://missdcoxblog.wordpress.com/2016/01/09/ditch-revision-teach-it-well/ . Accessed 25 Dec 2017.
Cox, D. (2016b). ‘They need to remember this in three years time’: spacing & interleaving for the new GCSEs [Blog post]. Retrieved from https://missdcoxblog.wordpress.com/2016/03/25/they-need-to-remember-this-in-three-years-time-spacing-interleaving-for-the-new-gcses/ . Accessed 25 Dec 2017.
Craik, F. I. (2002). Levels of processing: past, present… future? Memory, 10 , 305–318.
Craik, F. I., & Lockhart, R. S. (1972). Levels of processing: a framework for memory research. Journal of Verbal Learning and Verbal Behavior, 11 , 671–684.
Danan, M. (1992). Reversed subtitling and dual coding theory: new directions for foreign language instruction. Language Learning, 42 , 497–527.
Dettmers, S., Trautwein, U., & Lüdtke, O. (2009). The relationship between homework time and achievement is not universal: evidence from multilevel analyses in 40 countries. School Effectiveness and School Improvement, 20 , 375–405.
Dirkx, K. J., Kester, L., & Kirschner, P. A. (2014). The testing effect for learning principles and procedures from texts. The Journal of Educational Research, 107 , 357–364.
Dunlosky, J. (2013). Strengthening the student toolbox: study strategies to boost learning. American Educator, 37 (3), 12–21.
Dunlosky, J., Rawson, K. A., Marsh, E. J., Nathan, M. J., & Willingham, D. T. (2013). Improving students’ learning with effective learning techniques: promising directions from cognitive and educational psychology. Psychological Science in the Public Interest, 14 , 4–58.
Ebbinghaus, H. (1913). Memory (HA Ruger & CE Bussenius, Trans.). New York: Columbia University, Teachers College. (Original work published 1885) . Retrieved from http://psychclassics.yorku.ca/Ebbinghaus/memory8.htm . Accessed 25 Dec 2017.
Eglington, L. G., & Kang, S. H. (2016). Retrieval practice benefits deductive inference. Educational Psychology Review , 1–14.
Eitel, A., & Scheiter, K. (2015). Picture or text first? Explaining sequential effects when learning with pictures and text. Educational Psychology Review, 27 , 153–180.
Engelkamp, J., & Cohen, R. L. (1991). Current issues in memory of action events. Psychological Research, 53 , 175–182.
Engelkamp, J., & Zimmer, H. D. (1984). Motor programme information as a separable memory unit. Psychological Research, 46 , 283–299.
Fawcett, D. (2013). Can I be that little better at……using cognitive science/psychology/neurology to plan learning? [Blog post]. Retrieved from http://reflectionsofmyteaching.blogspot.com/2013/09/can-i-be-that-little-better-atusing.html . Accessed 25 Dec 2017.
Fiechter, J. L., & Benjamin, A. S. (2017). Diminishing-cues retrieval practice: a memory-enhancing technique that works when regular testing doesn’t. Psychonomic Bulletin & Review , 1–9.
Firth, J. (2016). Spacing in teaching practice [Blog post]. Retrieved from http://www.learningscientists.org/blog/2016/4/12-1 . Accessed 25 Dec 2017.
Fordham, M. [mfordhamhistory]. (2016). Is there a meaningful distinction in psychology between ‘thinking’ & ‘critical thinking’? [Tweet]. Retrieved from https://twitter.com/mfordhamhistory/status/809525713623781377 . Accessed 25 Dec 2017.
Fritz, C. O., Morris, P. E., Nolan, D., & Singleton, J. (2007). Expanding retrieval practice: an effective aid to preschool children’s learning. The Quarterly Journal of Experimental Psychology, 60 , 991–1004.
Gates, A. I. (1917). Recitation as a factory in memorizing. Archives of Psychology, 6.
Gick, M. L., & Holyoak, K. J. (1983). Schema induction and analogical transfer. Cognitive Psychology, 15 , 1–38.
Gorman, A. M. (1961). Recognition memory for nouns as a function of abstractedness and frequency. Journal of Experimental Psychology, 61 , 23–39.
Hainselin, M., Picard, L., Manolli, P., Vankerkore-Candas, S., & Bourdin, B. (2017). Hey teacher, don’t leave them kids alone: action is better for memory than reading. Frontiers in Psychology , 8 .
Harp, S. F., & Mayer, R. E. (1998). How seductive details do their damage. Journal of Educational Psychology, 90 , 414–434.
Hartland, W., Biddle, C., & Fallacaro, M. (2008). Audiovisual facilitation of clinical knowledge: A paradigm for dispersed student education based on Paivio’s dual coding theory. AANA Journal, 76 , 194–198.
Hattie, J., & Yates, G. (2014). Visible learning and the science of how we learn . New York: Routledge.
Hausman, H., & Kornell, N. (2014). Mixing topics while studying does not enhance learning. Journal of Applied Research in Memory and Cognition, 3 , 153–160.
Hinze, S. R., & Rapp, D. N. (2014). Retrieval (sometimes) enhances learning: performance pressure reduces the benefits of retrieval practice. Applied Cognitive Psychology, 28 , 597–606.
Hirshman, E. (2001). Elaboration in memory. In N. J. Smelser & P. B. Baltes (Eds.), International encyclopedia of the social & behavioral sciences (pp. 4369–4374). Oxford: Pergamon.
Chapter Google Scholar
Hobbiss, M. (2016). Make it meaningful! Elaboration [Blog post]. Retrieved from https://hobbolog.wordpress.com/2016/06/09/make-it-meaningful-elaboration/ . Accessed 25 Dec 2017.
Jones, F. (2016). Homework – is it really that useless? [Blog post]. Retrieved from http://www.learningscientists.org/blog/2016/4/5-1 . Accessed 25 Dec 2017.
Kaminski, J. A., & Sloutsky, V. M. (2013). Extraneous perceptual information interferes with children’s acquisition of mathematical knowledge. Journal of Educational Psychology, 105 (2), 351–363.
Kaminski, J. A., Sloutsky, V. M., & Heckler, A. F. (2008). The advantage of abstract examples in learning math. Science, 320 , 454–455.
Kang, S. H. (2016). Spaced repetition promotes efficient and effective learning policy implications for instruction. Policy Insights from the Behavioral and Brain Sciences, 3 , 12–19.
Kang, S. H. K., McDermott, K. B., & Roediger, H. L. (2007). Test format and corrective feedback modify the effects of testing on long-term retention. European Journal of Cognitive Psychology, 19 , 528–558.
Karpicke, J. D., & Aue, W. R. (2015). The testing effect is alive and well with complex materials. Educational Psychology Review, 27 , 317–326.
Karpicke, J. D., Blunt, J. R., Smith, M. A., & Karpicke, S. S. (2014). Retrieval-based learning: The need for guided retrieval in elementary school children. Journal of Applied Research in Memory and Cognition, 3 , 198–206.
Karpicke, J. D., Lehman, M., & Aue, W. R. (2014). Retrieval-based learning: an episodic context account. In B. H. Ross (Ed.), Psychology of Learning and Motivation (Vol. 61, pp. 237–284). San Diego, CA: Elsevier Academic Press.
Karpicke, J. D., Blunt, J. R., & Smith, M. A. (2016). Retrieval-based learning: positive effects of retrieval practice in elementary school children. Frontiers in Psychology, 7 .
Kavale, K. A., Hirshoren, A., & Forness, S. R. (1998). Meta-analytic validation of the Dunn and Dunn model of learning-style preferences: a critique of what was Dunn. Learning Disabilities Research & Practice, 13 , 75–80.
Khanna, M. M. (2015). Ungraded pop quizzes: test-enhanced learning without all the anxiety. Teaching of Psychology, 42 , 174–178.
Kirby, J. (2014). One scientific insight for curriculum design [Blog post]. Retrieved from https://pragmaticreform.wordpress.com/2014/05/05/scientificcurriculumdesign/ . Accessed 25 Dec 2017.
Kirschner, P. A. (2017). Stop propagating the learning styles myth. Computers & Education, 106 , 166–171.
Kirschner, P. A., & van Merriënboer, J. J. G. (2013). Do learners really know best? Urban legends in education. Educational Psychologist, 48 , 169–183.
Knoll, A. R., Otani, H., Skeel, R. L., & Van Horn, K. R. (2017). Learning style, judgments of learning, and learning of verbal and visual information. British Journal of Psychology, 108 , 544-563.
Kornell, N., & Bjork, R. A. (2008). Learning concepts and categories is spacing the “enemy of induction”? Psychological Science, 19 , 585–592.
Kornell, N., & Finn, B. (2016). Self-regulated learning: an overview of theory and data. In J. Dunlosky & S. Tauber (Eds.), The Oxford Handbook of Metamemory (pp. 325–340). New York: Oxford University Press.
Kornell, N., Klein, P. J., & Rawson, K. A. (2015). Retrieval attempts enhance learning, but retrieval success (versus failure) does not matter. Journal of Experimental Psychology: Learning, Memory, and Cognition, 41 , 283–294.
Kraemer, D. J. M., Rosenberg, L. M., & Thompson-Schill, S. L. (2009). The neural correlates of visual and verbal cognitive styles. Journal of Neuroscience, 29 , 3792–3798.
Article PubMed PubMed Central Google Scholar
Kraft, N. (2015). Spaced practice and repercussions for teaching. Retrieved from http://nathankraft.blogspot.com/2015/08/spaced-practice-and-repercussions-for.html . Accessed 25 Dec 2017.
Learning Scientists. (2016a). Weekly Digest #3: How teachers implement interleaving in their curriculum [Blog post]. Retrieved from http://www.learningscientists.org/blog/2016/3/28/weekly-digest-3 . Accessed 25 Dec 2017.
Learning Scientists. (2016b). Weekly Digest #13: how teachers implement retrieval in their classrooms [Blog post]. Retrieved from http://www.learningscientists.org/blog/2016/6/5/weekly-digest-13 . Accessed 25 Dec 2017.
Learning Scientists. (2016c). Weekly Digest #40: teachers’ implementation of principles from “Make It Stick” [Blog post]. Retrieved from http://www.learningscientists.org/blog/2016/12/18-1 . Accessed 25 Dec 2017.
Learning Scientists. (2017). Weekly Digest #54: is there an app for that? Studying 2.0 [Blog post]. Retrieved from http://www.learningscientists.org/blog/2017/4/9/weekly-digest-54 . Accessed 25 Dec 2017.
LeFevre, J.-A., & Dixon, P. (1986). Do written instructions need examples? Cognition and Instruction, 3 , 1–30.
Lew, K., Fukawa-Connelly, T., Mejí-Ramos, J. P., & Weber, K. (2016). Lectures in advanced mathematics: Why students might not understand what the mathematics professor is trying to convey. Journal of Research in Mathematics Education, 47 , 162–198.
Lindsey, R. V., Shroyer, J. D., Pashler, H., & Mozer, M. C. (2014). Improving students’ long-term knowledge retention through personalized review. Psychological Science, 25 , 639–647.
Lipko-Speed, A., Dunlosky, J., & Rawson, K. A. (2014). Does testing with feedback help grade-school children learn key concepts in science? Journal of Applied Research in Memory and Cognition, 3 , 171–176.
Lockhart, R. S., & Craik, F. I. (1990). Levels of processing: a retrospective commentary on a framework for memory research. Canadian Journal of Psychology, 44 , 87–112.
Lovell, O. (2017). How do we know what to put on the quiz? [Blog Post]. Retrieved from http://www.ollielovell.com/olliesclassroom/know-put-quiz/ . Accessed 25 Dec 2017.
Luehmann, A. L. (2008). Using blogging in support of teacher professional identity development: a case study. The Journal of the Learning Sciences, 17 , 287–337.
Madan, C. R., Glaholt, M. G., & Caplan, J. B. (2010). The influence of item properties on association-memory. Journal of Memory and Language, 63 , 46–63.
Madan, C. R., & Singhal, A. (2012a). Motor imagery and higher-level cognition: four hurdles before research can sprint forward. Cognitive Processing, 13 , 211–229.
Madan, C. R., & Singhal, A. (2012b). Encoding the world around us: motor-related processing influences verbal memory. Consciousness and Cognition, 21 , 1563–1570.
Madan, C. R., & Singhal, A. (2012c). Using actions to enhance memory: effects of enactment, gestures, and exercise on human memory. Frontiers in Psychology, 3 .
Madan, C. R., Chen, Y. Y., & Singhal, A. (2016). ERPs differentially reflect automatic and deliberate processing of the functional manipulability of objects. Frontiers in Human Neuroscience, 10 .
Mandler, G. (1979). Organization and repetition: organizational principles with special reference to rote learning. In L. G. Nilsson (Ed.), Perspectives on Memory Research (pp. 293–327). New York: Academic Press.
Marsh, E. J., Fazio, L. K., & Goswick, A. E. (2012). Memorial consequences of testing school-aged children. Memory, 20 , 899–906.
Mayer, R. E., & Gallini, J. K. (1990). When is an illustration worth ten thousand words? Journal of Educational Psychology, 82 , 715–726.
Mayer, R. E., & Moreno, R. (2003). Nine ways to reduce cognitive load in multimedia learning. Educational Psychologist, 38 , 43–52.
McDaniel, M. A., & Donnelly, C. M. (1996). Learning with analogy and elaborative interrogation. Journal of Educational Psychology, 88 , 508–519.
McDaniel, M. A., Thomas, R. C., Agarwal, P. K., McDermott, K. B., & Roediger, H. L. (2013). Quizzing in middle-school science: successful transfer performance on classroom exams. Applied Cognitive Psychology, 27 , 360–372.
McDermott, K. B., Agarwal, P. K., D’Antonio, L., Roediger, H. L., & McDaniel, M. A. (2014). Both multiple-choice and short-answer quizzes enhance later exam performance in middle and high school classes. Journal of Experimental Psychology: Applied, 20 , 3–21.
McHugh, A. (2013). High-stakes tests: bad for students, teachers, and education in general [Blog post]. Retrieved from https://teacherbiz.wordpress.com/2013/07/01/high-stakes-tests-bad-for-students-teachers-and-education-in-general/ . Accessed 25 Dec 2017.
McNeill, N. M., Uttal, D. H., Jarvin, L., & Sternberg, R. J. (2009). Should you show me the money? Concrete objects both hurt and help performance on mathematics problems. Learning and Instruction, 19 , 171–184.
Meider, W. (1990). “A picture is worth a thousand words”: from advertising slogan to American proverb. Southern Folklore, 47 , 207–225.
Michaela Community School. (2014). Homework. Retrieved from http://mcsbrent.co.uk/homework-2/ . Accessed 25 Dec 2017.
Montefinese, M., Ambrosini, E., Fairfield, B., & Mammarella, N. (2013). The “subjective” pupil old/new effect: is the truth plain to see? International Journal of Psychophysiology, 89 , 48–56.
O’Neil, H. F., Chung, G. K., Kerr, D., Vendlinski, T. P., Buschang, R. E., & Mayer, R. E. (2014). Adding self-explanation prompts to an educational computer game. Computers In Human Behavior, 30 , 23–28.
Overoye, A. L., & Storm, B. C. (2015). Harnessing the power of uncertainty to enhance learning. Translational Issues in Psychological Science, 1 , 140–148.
Paivio, A. (1971). Imagery and verbal processes . New York: Holt, Rinehart and Winston.
Paivio, A. (1986). Mental representations: a dual coding approach . New York: Oxford University Press.
Paivio, A. (2007). Mind and its evolution: a dual coding theoretical approach . Mahwah: Erlbaum.
Paivio, A. (2013). Dual coding theory, word abstractness, and emotion: a critical review of Kousta et al. (2011). Journal of Experimental Psychology: General, 142 , 282–287.
Paivio, A., & Csapo, K. (1969). Concrete image and verbal memory codes. Journal of Experimental Psychology, 80 , 279–285.
Paivio, A., & Csapo, K. (1973). Picture superiority in free recall: imagery or dual coding? Cognitive Psychology, 5 , 176–206.
Paivio, A., Walsh, M., & Bons, T. (1994). Concreteness effects on memory: when and why? Journal of Experimental Psychology: Learning, Memory, and Cognition, 20 , 1196–1204.
Pashler, H., McDaniel, M., Rohrer, D., & Bjork, R. (2008). Learning styles: concepts and evidence. Psychological Science in the Public Interest, 9 , 105–119.
Pashler, H., Bain, P. M., Bottge, B. A., Graesser, A., Koedinger, K., McDaniel, M., & Metcalfe, J. (2007). Organizing instruction and study to improve student learning. IES practice guide. NCER 2007–2004. National Center for Education Research .
Patel, R., Liu, R., & Koedinger, K. (2016). When to block versus interleave practice? Evidence against teaching fraction addition before fraction multiplication. In Proceedings of the 38th Annual Meeting of the Cognitive Science Society, Philadelphia, PA .
Penfound, B. (2017). Journey to interleaved practice #2 [Blog Post]. Retrieved from https://fullstackcalculus.com/2017/02/03/journey-to-interleaved-practice-2/ . Accessed 25 Dec 2017.
Penfound, B. [BryanPenfound]. (2016). Does blocked practice/learning lessen cognitive load? Does interleaved practice/learning provide productive struggle? [Tweet]. Retrieved from https://twitter.com/BryanPenfound/status/808759362244087808 . Accessed 25 Dec 2017.
Peterson, D. J., & Mulligan, N. W. (2010). Enactment and retrieval. Memory & Cognition, 38 , 233–243.
Picciotto, H. (2009). Lagging homework [Blog post]. Retrieved from http://blog.mathedpage.org/2013/06/lagging-homework.html . Accessed 25 Dec 2017.
Pomerance, L., Greenberg, J., & Walsh, K. (2016). Learning about learning: what every teacher needs to know. Retrieved from http://www.nctq.org/dmsView/Learning_About_Learning_Report . Accessed 25 Dec 2017.
Postman, L. (1976). Methodology of human learning. In W. K. Estes (Ed.), Handbook of learning and cognitive processes (Vol. 3). Hillsdale: Erlbaum.
Pressley, M., McDaniel, M. A., Turnure, J. E., Wood, E., & Ahmad, M. (1987). Generation and precision of elaboration: effects on intentional and incidental learning. Journal of Experimental Psychology: Learning, Memory, and Cognition, 13 , 291–300.
Reed, S. K. (2008). Concrete examples must jibe with experience. Science, 322 , 1632–1633.
researchED. (2013). How it all began. Retrieved from http://www.researched.org.uk/about/our-story/ . Accessed 25 Dec 2017.
Ritchie, S. J., Della Sala, S., & McIntosh, R. D. (2013). Retrieval practice, with or without mind mapping, boosts fact learning in primary school children. PLoS One, 8 (11), e78976.
Rittle-Johnson, B. (2006). Promoting transfer: effects of self-explanation and direct instruction. Child Development, 77 , 1–15.
Roediger, H. L. (1985). Remembering Ebbinghaus. [Retrospective review of the book On Memory , by H. Ebbinghaus]. Contemporary Psychology, 30 , 519–523.
Roediger, H. L. (2013). Applying cognitive psychology to education translational educational science. Psychological Science in the Public Interest, 14 , 1–3.
Roediger, H. L., & Karpicke, J. D. (2006). The power of testing memory: basic research and implications for educational practice. Perspectives on Psychological Science, 1 , 181–210.
Roediger, H. L., Putnam, A. L., & Smith, M. A. (2011). Ten benefits of testing and their applications to educational practice. In J. Mester & B. Ross (Eds.), The psychology of learning and motivation: cognition in education (pp. 1–36). Oxford: Elsevier.
Roediger, H. L., Finn, B., & Weinstein, Y. (2012). Applications of cognitive science to education. In Della Sala, S., & Anderson, M. (Eds.), Neuroscience in education: the good, the bad, and the ugly . Oxford, UK: Oxford University Press.
Roelle, J., & Berthold, K. (2017). Effects of incorporating retrieval into learning tasks: the complexity of the tasks matters. Learning and Instruction, 49 , 142–156.
Rohrer, D. (2012). Interleaving helps students distinguish among similar concepts. Educational Psychology Review, 24(3), 355–367.
Rohrer, D., Dedrick, R. F., & Stershic, S. (2015). Interleaved practice improves mathematics learning. Journal of Educational Psychology, 107 , 900–908.
Rohrer, D., & Pashler, H. (2012). Learning styles: Where’s the evidence? Medical Education, 46 , 34–35.
Rohrer, D., & Taylor, K. (2007). The shuffling of mathematics problems improves learning. Instructional Science, 35 , 481–498.
Rose, N. (2014). Improving the effectiveness of homework [Blog post]. Retrieved from https://evidenceintopractice.wordpress.com/2014/03/20/improving-the-effectiveness-of-homework/ . Accessed 25 Dec 2017.
Sadoski, M. (2005). A dual coding view of vocabulary learning. Reading & Writing Quarterly, 21 , 221–238.
Saunders, K. (2016). It really is time we stopped talking about learning styles [Blog post]. Retrieved from http://martingsaunders.com/2016/10/it-really-is-time-we-stopped-talking-about-learning-styles/ . Accessed 25 Dec 2017.
Schwartz, D. (2007). If a picture is worth a thousand words, why are you reading this essay? Social Psychology Quarterly, 70 , 319–321.
Shumaker, H. (2016). Homework is wrecking our kids: the research is clear, let’s ban elementary homework. Salon. Retrieved from http://www.salon.com/2016/03/05/homework_is_wrecking_our_kids_the_research_is_clear_lets_ban_elementary_homework . Accessed 25 Dec 2017.
Smith, A. M., Floerke, V. A., & Thomas, A. K. (2016). Retrieval practice protects memory against acute stress. Science, 354 , 1046–1048.
Smith, M. A., Blunt, J. R., Whiffen, J. W., & Karpicke, J. D. (2016). Does providing prompts during retrieval practice improve learning? Applied Cognitive Psychology, 30 , 784–802.
Smith, M. A., & Karpicke, J. D. (2014). Retrieval practice with short-answer, multiple-choice, and hybrid formats. Memory, 22 , 784–802.
Smith, M. A., Roediger, H. L., & Karpicke, J. D. (2013). Covert retrieval practice benefits retention as much as overt retrieval practice. Journal of Experimental Psychology: Learning, Memory, and Cognition, 39 , 1712–1725.
Son, J. Y., & Rivas, M. J. (2016). Designing clicker questions to stimulate transfer. Scholarship of Teaching and Learning in Psychology, 2 , 193–207.
Szpunar, K. K., Khan, N. Y., & Schacter, D. L. (2013). Interpolated memory tests reduce mind wandering and improve learning of online lectures. Proceedings of the National Academy of Sciences, 110 , 6313–6317.
Thomson, R., & Mehring, J. (2016). Better vocabulary study strategies for long-term learning. Kwansei Gakuin University Humanities Review, 20 , 133–141.
Trafton, J. G., & Reiser, B. J. (1993). Studying examples and solving problems: contributions to skill acquisition . Technical report, Naval HCI Research Lab, Washington, DC, USA.
Tran, R., Rohrer, D., & Pashler, H. (2015). Retrieval practice: the lack of transfer to deductive inferences. Psychonomic Bulletin & Review, 22 , 135–140.
Turner, K. [doc_kristy]. (2016a). My dual coding (in red) and some y8 work @AceThatTest they really enjoyed practising the technique [Tweet]. Retrieved from https://twitter.com/doc_kristy/status/807220355395977216 . Accessed 25 Dec 2017.
Turner, K. [doc_kristy]. (2016b). @FurtherEdagogy @doctorwhy their work is revision work, they already have the words on a different page, to compliment not replace [Tweet]. Retrieved from https://twitter.com/doc_kristy/status/807360265100599301 . Accessed 25 Dec 2017.
Valle, A., Regueiro, B., Núñez, J. C., Rodríguez, S., Piñeiro, I., & Rosário, P. (2016). Academic goals, student homework engagement, and academic achievement in elementary school. Frontiers in Psychology, 7 .
Van Gog, T., & Sweller, J. (2015). Not new, but nearly forgotten: the testing effect decreases or even disappears as the complexity of learning materials increases. Educational Psychology Review, 27 , 247–264.
Wammes, J. D., Meade, M. E., & Fernandes, M. A. (2016). The drawing effect: evidence for reliable and robust memory benefits in free recall. Quarterly Journal of Experimental Psychology, 69 , 1752–1776.
Weinstein, Y., Gilmore, A. W., Szpunar, K. K., & McDermott, K. B. (2014). The role of test expectancy in the build-up of proactive interference in long-term memory. Journal of Experimental Psychology: Learning, Memory, and Cognition, 40 , 1039–1048.
Weinstein, Y., Nunes, L. D., & Karpicke, J. D. (2016). On the placement of practice questions during study. Journal of Experimental Psychology: Applied, 22 , 72–84.
Weinstein, Y., & Weinstein-Jones, F. (2017). Topic and quiz spacing spreadsheet: a planning tool for teachers [Blog Post]. Retrieved from http://www.learningscientists.org/blog/2017/5/11-1 . Accessed 25 Dec 2017.
Weinstein-Jones, F., & Weinstein, Y. (2017). Topic spacing spreadsheet for teachers [Excel macro]. Zenodo. http://doi.org/10.5281/zenodo.573764 . Accessed 25 Dec 2017.
Williams, D. [FurtherEdagogy]. (2016). @doctorwhy @doc_kristy word accompanying the visual? I’m unclear how removing words benefit? Would a flow chart better suit a scientific exp? [Tweet]. Retrieved from https://twitter.com/FurtherEdagogy/status/807356800509104128 . Accessed 25 Dec 2017.
Wood, B. (2017). And now for something a little bit different….[Blog post]. Retrieved from https://justateacherstandinginfrontofaclass.wordpress.com/2017/04/20/and-now-for-something-a-little-bit-different/ . Accessed 25 Dec 2017.
Wooldridge, C. L., Bugg, J. M., McDaniel, M. A., & Liu, Y. (2014). The testing effect with authentic educational materials: a cautionary note. Journal of Applied Research in Memory and Cognition, 3 , 214–221.
Young, C. (2016). Mini-tests. Retrieved from https://colleenyoung.wordpress.com/revision-activities/mini-tests/ . Accessed 25 Dec 2017.
Download references
Acknowledgements
Not applicable.
YW and MAS were partially supported by a grant from The IDEA Center.
Availability of data and materials
Author information, authors and affiliations.
Department of Psychology, University of Massachusetts Lowell, Lowell, MA, USA
Yana Weinstein
Department of Psychology, Boston College, Chestnut Hill, MA, USA
Christopher R. Madan
School of Psychology, University of Nottingham, Nottingham, UK
Department of Psychology, Rhode Island College, Providence, RI, USA
Megan A. Sumeracki
You can also search for this author in PubMed Google Scholar
Contributions
YW took the lead on writing the “Spaced practice”, “Interleaving”, and “Elaboration” sections. CRM took the lead on writing the “Concrete examples” and “Dual coding” sections. MAS took the lead on writing the “Retrieval practice” section. All authors edited each others’ sections. All authors were involved in the conception and writing of the manuscript. All authors gave approval of the final version.
Corresponding author
Correspondence to Yana Weinstein .
Ethics declarations
Ethics approval and consent to participate, consent for publication, competing interests.
YW and MAS run a blog, “The Learning Scientists Blog”, which is cited in the tutorial review. The blog does not make money. Free resources on the strategies described in this tutorial review are provided on the blog. Occasionally, YW and MAS are invited by schools/school districts to present research findings from cognitive psychology applied to education.
Publisher’s Note
Springer Nature remains neutral with regard to jurisdictional claims in published maps and institutional affiliations.
Rights and permissions
Open Access This article is distributed under the terms of the Creative Commons Attribution 4.0 International License ( http://creativecommons.org/licenses/by/4.0/ ), which permits unrestricted use, distribution, and reproduction in any medium, provided you give appropriate credit to the original author(s) and the source, provide a link to the Creative Commons license, and indicate if changes were made.
Reprints and permissions
About this article
Cite this article.
Weinstein, Y., Madan, C.R. & Sumeracki, M.A. Teaching the science of learning. Cogn. Research 3 , 2 (2018). https://doi.org/10.1186/s41235-017-0087-y
Download citation
Received : 20 December 2016
Accepted : 02 December 2017
Published : 24 January 2018
DOI : https://doi.org/10.1186/s41235-017-0087-y
Share this article
Anyone you share the following link with will be able to read this content:
Sorry, a shareable link is not currently available for this article.
Provided by the Springer Nature SharedIt content-sharing initiative
- Share full article
Advertisement
Supported by
Student Opinion
Is Online Learning Effective?
A new report found that the heavy dependence on technology during the pandemic caused “staggering” education inequality. What was your experience?

By Natalie Proulx
During the coronavirus pandemic, many schools moved classes online. Was your school one of them? If so, what was it like to attend school online? Did you enjoy it? Did it work for you?
In “ Dependence on Tech Caused ‘Staggering’ Education Inequality, U.N. Agency Says ,” Natasha Singer writes:
In early 2020, as the coronavirus spread, schools around the world abruptly halted in-person education. To many governments and parents, moving classes online seemed the obvious stopgap solution. In the United States, school districts scrambled to secure digital devices for students. Almost overnight, videoconferencing software like Zoom became the main platform teachers used to deliver real-time instruction to students at home. Now a report from UNESCO , the United Nations’ educational and cultural organization, says that overreliance on remote learning technology during the pandemic led to “staggering” education inequality around the world. It was, according to a 655-page report that UNESCO released on Wednesday, a worldwide “ed-tech tragedy.” The report, from UNESCO’s Future of Education division, is likely to add fuel to the debate over how governments and local school districts handled pandemic restrictions, and whether it would have been better for some countries to reopen schools for in-person instruction sooner. The UNESCO researchers argued in the report that “unprecedented” dependence on technology — intended to ensure that children could continue their schooling — worsened disparities and learning loss for hundreds of millions of students around the world, including in Kenya, Brazil, Britain and the United States. The promotion of remote online learning as the primary solution for pandemic schooling also hindered public discussion of more equitable, lower-tech alternatives, such as regularly providing schoolwork packets for every student, delivering school lessons by radio or television — and reopening schools sooner for in-person classes, the researchers said. “Available evidence strongly indicates that the bright spots of the ed-tech experiences during the pandemic, while important and deserving of attention, were vastly eclipsed by failure,” the UNESCO report said. The UNESCO researchers recommended that education officials prioritize in-person instruction with teachers, not online platforms, as the primary driver of student learning. And they encouraged schools to ensure that emerging technologies like A.I. chatbots concretely benefited students before introducing them for educational use. Education and industry experts welcomed the report, saying more research on the effects of pandemic learning was needed. “The report’s conclusion — that societies must be vigilant about the ways digital tools are reshaping education — is incredibly important,” said Paul Lekas, the head of global public policy for the Software & Information Industry Association, a group whose members include Amazon, Apple and Google. “There are lots of lessons that can be learned from how digital education occurred during the pandemic and ways in which to lessen the digital divide. ” Jean-Claude Brizard, the chief executive of Digital Promise, a nonprofit education group that has received funding from Google, HP and Verizon, acknowledged that “technology is not a cure-all.” But he also said that while school systems were largely unprepared for the pandemic, online education tools helped foster “more individualized, enhanced learning experiences as schools shifted to virtual classrooms.” Education International, an umbrella organization for about 380 teachers’ unions and 32 million teachers worldwide, said the UNESCO report underlined the importance of in-person, face-to-face teaching. “The report tells us definitively what we already know to be true, a place called school matters,” said Haldis Holst, the group’s deputy general secretary. “Education is not transactional nor is it simply content delivery. It is relational. It is social. It is human at its core.”
Students, read the entire article and then tell us:
We are having trouble retrieving the article content.
Please enable JavaScript in your browser settings.
Thank you for your patience while we verify access. If you are in Reader mode please exit and log into your Times account, or subscribe for all of The Times.
Thank you for your patience while we verify access.
Already a subscriber? Log in .
Want all of The Times? Subscribe .
Effective Learning Essays
Contribution of technology in higher education., popular essay topics.
- American Dream
- Artificial Intelligence
- Black Lives Matter
- Bullying Essay
- Career Goals Essay
- Causes of the Civil War
- Child Abusing
- Civil Rights Movement
- Community Service
- Cultural Identity
- Cyber Bullying
- Death Penalty
- Depression Essay
- Domestic Violence
- Freedom of Speech
- Global Warming
- Gun Control
- Human Trafficking
- I Believe Essay
- Immigration
- Importance of Education
- Israel and Palestine Conflict
- Leadership Essay
- Legalizing Marijuanas
- Mental Health
- National Honor Society
- Police Brutality
- Pollution Essay
- Racism Essay
- Romeo and Juliet
- Same Sex Marriages
- Social Media
- The Great Gatsby
- The Yellow Wallpaper
- Time Management
- To Kill a Mockingbird
- Violent Video Games
- What Makes You Unique
- Why I Want to Be a Nurse
- Send us an e-mail
- Bipolar Disorder
- Therapy Center
- When To See a Therapist
- Types of Therapy
- Best Online Therapy
- Best Couples Therapy
- Managing Stress
- Sleep and Dreaming
- Understanding Emotions
- Self-Improvement
- Healthy Relationships
- Student Resources
- Personality Types
- Sweepstakes
- Guided Meditations
- Verywell Mind Insights
- 2024 Verywell Mind 25
- Mental Health in the Classroom
- Editorial Process
- Meet Our Review Board
- Crisis Support
How to Learn More Effectively
10 Learning Techniques to Try
Knowing the most effective strategies for how to learn can help you maximize your efforts when trying to acquire new ideas, concepts, and skills. If you are like many people, your time is limited, so it is important to get the most educational value out of the time you have. Speed of learning is not the only important factor, however.
It is also important to be able to accurately remember the information that you learn, recall it at a later time, and use it effectively in a wide variety of situations. How can you teach yourself to learn? As you approach a new subject, incorporate some of the following tactics:
- Find ways to boost your memory
- Always keep learning new things
- Use a variety of learning techniques
- Try teaching it to someone else
- Connect new information to things you already know
- Look for opportunities to have hands-on experiences
- Remember that mistakes are part of the process
- Study a little bit every day
- Test yourself
- Focus on one thing at a time
Knowing how to learn well doesn't happen overnight, but putting a few of these learning techniques into daily practice can help you get more out of your study time .
Improve Your Memory
There are a number of different strategies that can boost memory . Basic tips such as improving your focus, avoiding cram sessions, and structuring your study time are good places to start, but there are even more lessons from psychology that can dramatically improve your learning efficiency.
If you're wondering how to learn better by improving your memory, these strategies can help:
- Getting regular physical exercise , which is linked to improvements in memory and brain health
- Spending time socializing with other people
- Getting enough sleep
- Eliminating distractions so you can focus on what you are learning
- Organizing the information you are studying to make it easier to remember
- Using elaborative rehearsal when studying; when you learn something new, spend a few moments describing it to yourself in your own words
- Using visual aids like photographs, graphs, and charts
- Reading the information you are studying out loud
For example, you might use general learning techniques like setting aside quiet time to study, rehearsing, and reading information aloud. You might combine this with strategies that can foster better memory, such as exercising and socializing.
If you're pressed for time, consider combining study strategies. Listen to a podcast while taking a walk or join a group where you can practice your new skills with others.
Keep Learning New Things
Prasit photo / Getty Images
One surefire way to become a more effective learner is to simply keep learning. Research has found that the brain is capable of producing new brain cells , a process known as neurogenesis. However, many of these cells will eventually die unless a person engages in some type of effortful learning.
By learning new things, these cells are kept alive and incorporated into brain circuits.
If you want to learn a new language, for instance, it is important to keep practicing the language to maintain the gains you have achieved. This "use-it-or-lose-it" phenomenon involves a brain process known as "pruning."
In pruning, certain pathways in the brain are maintained while others are eliminated. If you want the new information you just learned to stay put, keep practicing and rehearsing it.
Learn in Multiple Ways
Another good "how to learn" strategy is to focus on learning in more than one way. For example, instead of just listening to a podcast, which involves auditory learning, find a way to rehearse the information both verbally and visually.
This might involve describing what you learned to a friend, taking notes , or drawing a mind map. By learning in more than one way, you’re further cementing the knowledge in your mind.
For example, if you are trying to pick up a new language, try varying learning techniques such as listening to language examples, reading written language, practicing with a friend, and writing down your own notes.
One helpful tip is to try writing your notes on paper rather than typing on a laptop, tablet, or computer. Research has found that longhand notes can help cement information in memory more effectively than digital note-taking.
Varying your learning techniques and giving yourself the opportunity to learn in different ways and in different contexts can help make you a more efficient learner.
Teach What You Are Learning
Educators have long noted that one of the best ways to learn something is to teach it to someone else. Remember your seventh-grade presentation on Costa Rica? By teaching to the rest of the class, your teacher hoped you would gain even more from the assignment.
You can apply the same principle today by sharing newly learned skills and knowledge with others. Start by translating the information into your own words. This process alone helps solidify new knowledge in your brain. Next, find some way to share what you’ve learned.
Some ideas include writing a blog post, creating a podcast, or participating in a group discussion.
Build on Previous Learning
Tara Moore\ / Getty Images
Another great way to become a more effective learner is to use relational learning. This involves relating new information to things that you already know.
For example, if you are learning a new language, you might associate the new vocabulary and grammar you are learning with what you already know about your native language or other languages you may already speak.
Gain Practical Experience
LWA / Dann Tardif / Getty Images
For many students, learning typically involves reading textbooks, attending lectures, or doing research in the library or online. While seeing information and then writing it down is important, actually putting new knowledge and skills into practice can be one of the best ways to improve learning.
If it is a sport or athletic skill, perform the activity on a regular basis. If you are learning a new language, practice speaking with another person and surround yourself with language-immersion experiences. Watch foreign-language films and strike up conversations with native speakers to practice your budding skills.
If you are trying to acquire a new skill or ability, focus on gaining practical experience.
Don't Be Afraid to Make Mistakes
Research suggests that making mistakes when learning can improve learning outcomes. According to one study, trial-and-error learning where the mistakes were close to the actual answer was actually a helpful part of the learning process.
Another study found that mistakes followed by corrective feedback can be beneficial to learning. So if you make a mistake when learning something new, spend some time correcting the mistake and examining how you arrived at the incorrect answer.
This strategy can help foster critical thinking skills and make you more adaptable in learning situations that require being able to change your mind.
Research suggests that making mistakes when learning can actually help improve outcomes, especially if you correct your mistake and take the time to understand why it happened.
Use Distributed Practice
David Schaffer / Getty Images
Another strategy for how to learn better is known as distributed practice. Instead of trying to cram all of your learning into a few long study sessions, try a brief, focused session, and then take a break.
So if you were learning a new language, you might devote a period of time to an intensive session of studying. After a break, you would then come back and rehearse your previous learning while also extending it to new learning.
This process of returning for brief sessions over a long period of time is one of the best ways to learn efficiently and effectively.
What is the best way to learn?
Research suggests that this type of distributed learning is one of the most effective learning techniques. Focus on spending a little time studying each topic every day.
While it may seem that spending more time studying is one of the best ways to maximize learning, research has demonstrated that taking tests actually helps you better remember what you've learned—even if the topic wasn't covered on the test.
This phenomenon, known as the testing effect, suggests that spending time retrieving information from memory improves the long-term memory of that information. This retrieval practice makes it more likely that you will be able to remember that information again in the future.
Stop Multitasking
For many years, it was thought that people who multitask had an edge over those who did not. However, research now suggests that multitasking can actually make learning less effective.
Multitasking can involve trying to do more than one thing at the same time. But it can also involve quickly switching back and forth between tasks or trying to rapidly perform tasks one after the other.
According to research, doing this not only makes people less productive when they work but also impairs attention and reduces comprehension. Multitasking when you are studying makes it harder to focus on the information and reduces how much you understand it.
Research has also found that media multitasking, or dividing attention between different media sources, can also have a detrimental impact on learning and academic performance.
To avoid the pitfalls of multitasking, focus your attention on the task at hand and continue working for a predetermined amount of time.
If you want to know how to learn, it is important to explore learning techniques that have been shown to be effective. Strategies such as boosting your memory and learning in multiple ways can be helpful. Regularly learning new things, using distributed practice, and testing yourself often can also be helpful ways to become a more efficient learner.
This process can take time, and it always takes practice and determination to establish new habits . Start by focusing on just a few of these tips to see if you can get more out of your next study session.
Perhaps most importantly, work on developing the mindset that you are capable of improving your knowledge and skills. Research suggests that believing in your own capacity for growth is one of the best ways to take advantage of the learning opportunities you pursue.
Chaire A, Becke A, Düzel E. Effects of physical exercise on working memory and attention-related neural oscillations . Front Neurosci . 2020;14:239. doi:10.3389/fnins.2020.00239
Mazza S, Gerbier E, Gustin M-P, et al. Relearn faster and retain longer: Along with practice, sleep makes perfect . Psychol Sci. 2016;27(10):1321-1330. doi:10.1177/0956797616659930
University of North Carolina at Chapel Hill. Memorization strategies .
Forrin ND, Macleod CM. This time it's personal: the memory benefit of hearing oneself . Memory. 2018;26(4):574-579. doi:10.1080/09658211.2017.1383434
Cunnington R. Neuroplasticity: How the brain changes with learning . IBE - UNESCO.
Mueller PA, Oppenheimer DM. The pen Is mightier than the keyboard: Advantages of longhand over laptop note taking . Psychol Sci . 2014. 2014;25(6):1159-1168. doi:10.1177/0956797614524581
Cyr AA, Anderson ND. Learning from your mistakes: does it matter if you’re out in left foot, I mean field? Memory . 2018;26(9):1281-1290. doi:10.1080/09658211.2018.1464189
Metcalfe J. Learning from errors . Ann Rev Psychol . 2017;68(1):465-489. doi:10.1146/annurev-psych-010416-044022
Kang SHK. Spaced repetition promotes efficient and effective learning: Policy implications for instruction . Policy Insights Behav Brain Sci . 2016;3(1):12-19. doi:10.1177/2372732215624708
Pastotter B, Bauml KHT. Retrieval practice enhances new learning: the forward effect of testing . Front Psychol . 2014;5:286. doi:10.3389/fpsyg.2014.00286
Jeong S-H, Hwang Y. Media multitasking effects on cognitive vs. attitudinal outcomes: A meta-analysis . Hum Commun Res . 2016;42(4):599-618. doi:10.1111/hcre.12089
May K, Elder A. Efficient, helpful, or distracting? A literature review of media multitasking in relation to academic performance . Int J Educ Technol High Educ. 2018;15(1):13. doi:10.1186/s41239-018-0096-z
Sarrasin JB, Nenciovici L, Foisy LMB, Allaire-Duquette G, Riopel M, Masson S. Effects of teaching the concept of neuroplasticity to induce a growth mindset on motivation, achievement, and brain activity: A meta-analysis . Trends Neurosci Educ . 2018;12:22-31. doi:10.1016/j.tine.2018.07.003
By Kendra Cherry, MSEd Kendra Cherry, MS, is a psychosocial rehabilitation specialist, psychology educator, and author of the "Everything Psychology Book."
Essay Papers Writing Online
Tips and tricks for crafting engaging and effective essays.
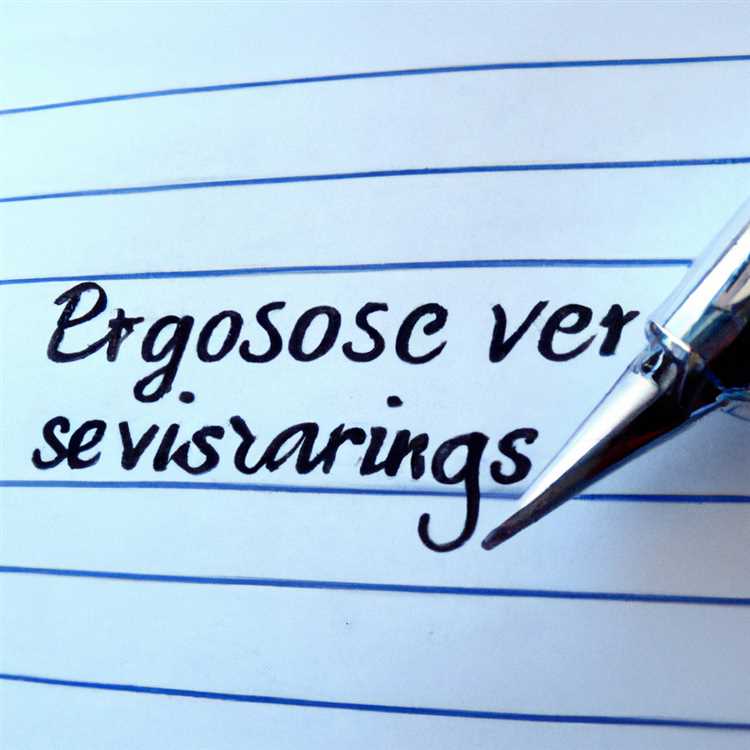
Writing essays can be a challenging task, but with the right approach and strategies, you can create compelling and impactful pieces that captivate your audience. Whether you’re a student working on an academic paper or a professional honing your writing skills, these tips will help you craft essays that stand out.
Effective essays are not just about conveying information; they are about persuading, engaging, and inspiring readers. To achieve this, it’s essential to pay attention to various elements of the essay-writing process, from brainstorming ideas to polishing your final draft. By following these tips, you can elevate your writing and produce essays that leave a lasting impression.
Understanding the Essay Prompt
Before you start writing your essay, it is crucial to thoroughly understand the essay prompt or question provided by your instructor. The essay prompt serves as a roadmap for your essay and outlines the specific requirements or expectations.
Here are a few key things to consider when analyzing the essay prompt:
- Read the prompt carefully and identify the main topic or question being asked.
- Pay attention to any specific instructions or guidelines provided, such as word count, formatting requirements, or sources to be used.
- Identify key terms or phrases in the prompt that can help you determine the focus of your essay.
By understanding the essay prompt thoroughly, you can ensure that your essay addresses the topic effectively and meets the requirements set forth by your instructor.
Researching Your Topic Thoroughly
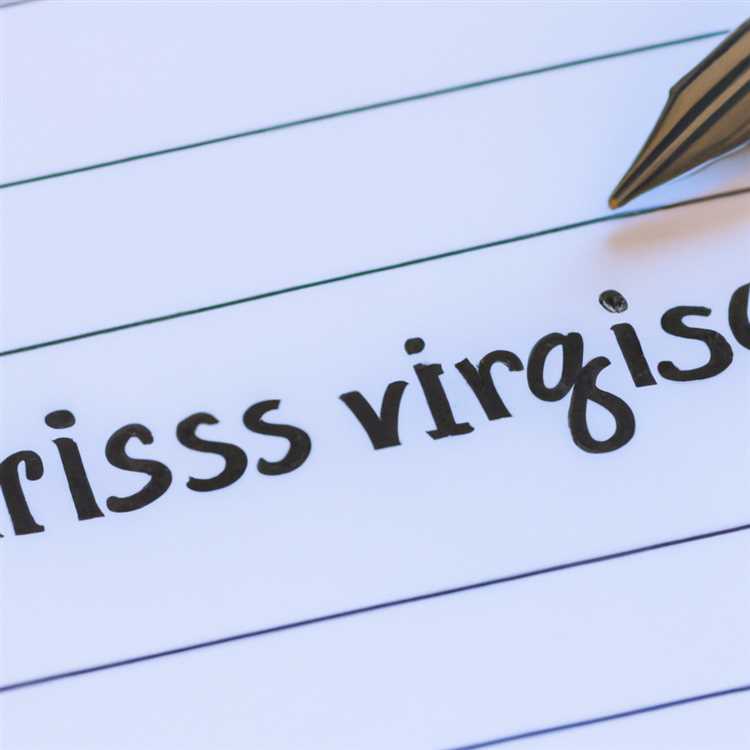
One of the key elements of writing an effective essay is conducting thorough research on your chosen topic. Research helps you gather the necessary information, facts, and examples to support your arguments and make your essay more convincing.
Here are some tips for researching your topic thoroughly:
Don’t rely on a single source for your research. Use a variety of sources such as books, academic journals, reliable websites, and primary sources to gather different perspectives and valuable information. | |
While conducting research, make sure to take detailed notes of important information, quotes, and references. This will help you keep track of your sources and easily refer back to them when writing your essay. | |
Before using any information in your essay, evaluate the credibility of the sources. Make sure they are reliable, up-to-date, and authoritative to strengthen the validity of your arguments. | |
Organize your research materials in a systematic way to make it easier to access and refer to them while writing. Create an outline or a research plan to structure your essay effectively. |
By following these tips and conducting thorough research on your topic, you will be able to write a well-informed and persuasive essay that effectively communicates your ideas and arguments.
Creating a Strong Thesis Statement
A thesis statement is a crucial element of any well-crafted essay. It serves as the main point or idea that you will be discussing and supporting throughout your paper. A strong thesis statement should be clear, specific, and arguable.
To create a strong thesis statement, follow these tips:
- Be specific: Your thesis statement should clearly state the main idea of your essay. Avoid vague or general statements.
- Be concise: Keep your thesis statement concise and to the point. Avoid unnecessary details or lengthy explanations.
- Be argumentative: Your thesis statement should present an argument or perspective that can be debated or discussed in your essay.
- Be relevant: Make sure your thesis statement is relevant to the topic of your essay and reflects the main point you want to make.
- Revise as needed: Don’t be afraid to revise your thesis statement as you work on your essay. It may change as you develop your ideas.
Remember, a strong thesis statement sets the tone for your entire essay and provides a roadmap for your readers to follow. Put time and effort into crafting a clear and compelling thesis statement to ensure your essay is effective and persuasive.
Developing a Clear Essay Structure
One of the key elements of writing an effective essay is developing a clear and logical structure. A well-structured essay helps the reader follow your argument and enhances the overall readability of your work. Here are some tips to help you develop a clear essay structure:
1. Start with a strong introduction: Begin your essay with an engaging introduction that introduces the topic and clearly states your thesis or main argument.
2. Organize your ideas: Before you start writing, outline the main points you want to cover in your essay. This will help you organize your thoughts and ensure a logical flow of ideas.
3. Use topic sentences: Begin each paragraph with a topic sentence that introduces the main idea of the paragraph. This helps the reader understand the purpose of each paragraph.
4. Provide evidence and analysis: Support your arguments with evidence and analysis to back up your main points. Make sure your evidence is relevant and directly supports your thesis.
5. Transition between paragraphs: Use transitional words and phrases to create flow between paragraphs and help the reader move smoothly from one idea to the next.
6. Conclude effectively: End your essay with a strong conclusion that summarizes your main points and reinforces your thesis. Avoid introducing new ideas in the conclusion.
By following these tips, you can develop a clear essay structure that will help you effectively communicate your ideas and engage your reader from start to finish.
Using Relevant Examples and Evidence
When writing an essay, it’s crucial to support your arguments and assertions with relevant examples and evidence. This not only adds credibility to your writing but also helps your readers better understand your points. Here are some tips on how to effectively use examples and evidence in your essays:
- Choose examples that are specific and relevant to the topic you’re discussing. Avoid using generic examples that may not directly support your argument.
- Provide concrete evidence to back up your claims. This could include statistics, research findings, or quotes from reliable sources.
- Interpret the examples and evidence you provide, explaining how they support your thesis or main argument. Don’t assume that the connection is obvious to your readers.
- Use a variety of examples to make your points more persuasive. Mixing personal anecdotes with scholarly evidence can make your essay more engaging and convincing.
- Cite your sources properly to give credit to the original authors and avoid plagiarism. Follow the citation style required by your instructor or the publication you’re submitting to.
By integrating relevant examples and evidence into your essays, you can craft a more convincing and well-rounded piece of writing that resonates with your audience.
Editing and Proofreading Your Essay Carefully
Once you have finished writing your essay, the next crucial step is to edit and proofread it carefully. Editing and proofreading are essential parts of the writing process that help ensure your essay is polished and error-free. Here are some tips to help you effectively edit and proofread your essay:
1. Take a Break: Before you start editing, take a short break from your essay. This will help you approach the editing process with a fresh perspective.
2. Read Aloud: Reading your essay aloud can help you catch any awkward phrasing or grammatical errors that you may have missed while writing. It also helps you check the flow of your essay.
3. Check for Consistency: Make sure that your essay has a consistent style, tone, and voice throughout. Check for inconsistencies in formatting, punctuation, and language usage.
4. Remove Unnecessary Words: Look for any unnecessary words or phrases in your essay and remove them to make your writing more concise and clear.
5. Proofread for Errors: Carefully proofread your essay for spelling, grammar, and punctuation errors. Pay attention to commonly misused words and homophones.
6. Get Feedback: It’s always a good idea to get feedback from someone else. Ask a friend, classmate, or teacher to review your essay and provide constructive feedback.
By following these tips and taking the time to edit and proofread your essay carefully, you can improve the overall quality of your writing and make sure your ideas are effectively communicated to your readers.
Related Post
How to master the art of writing expository essays and captivate your audience, convenient and reliable source to purchase college essays online, step-by-step guide to crafting a powerful literary analysis essay, unlock success with a comprehensive business research paper example guide, unlock your writing potential with writers college – transform your passion into profession, “unlocking the secrets of academic success – navigating the world of research papers in college”, master the art of sociological expression – elevate your writing skills in sociology.
Information
- Author Services
Initiatives
You are accessing a machine-readable page. In order to be human-readable, please install an RSS reader.
All articles published by MDPI are made immediately available worldwide under an open access license. No special permission is required to reuse all or part of the article published by MDPI, including figures and tables. For articles published under an open access Creative Common CC BY license, any part of the article may be reused without permission provided that the original article is clearly cited. For more information, please refer to https://www.mdpi.com/openaccess .
Feature papers represent the most advanced research with significant potential for high impact in the field. A Feature Paper should be a substantial original Article that involves several techniques or approaches, provides an outlook for future research directions and describes possible research applications.
Feature papers are submitted upon individual invitation or recommendation by the scientific editors and must receive positive feedback from the reviewers.
Editor’s Choice articles are based on recommendations by the scientific editors of MDPI journals from around the world. Editors select a small number of articles recently published in the journal that they believe will be particularly interesting to readers, or important in the respective research area. The aim is to provide a snapshot of some of the most exciting work published in the various research areas of the journal.
Original Submission Date Received: .
- Active Journals
- Find a Journal
- Proceedings Series
- For Authors
- For Reviewers
- For Editors
- For Librarians
- For Publishers
- For Societies
- For Conference Organizers
- Open Access Policy
- Institutional Open Access Program
- Special Issues Guidelines
- Editorial Process
- Research and Publication Ethics
- Article Processing Charges
- Testimonials
- Preprints.org
- SciProfiles
- Encyclopedia
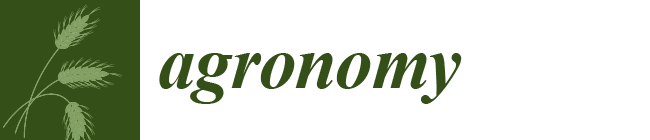
Article Menu
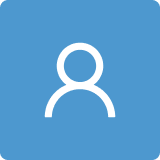
- Subscribe SciFeed
- Recommended Articles
- Google Scholar
- on Google Scholar
- Table of Contents
Find support for a specific problem in the support section of our website.
Please let us know what you think of our products and services.
Visit our dedicated information section to learn more about MDPI.
JSmol Viewer
Integration of remote sensing and machine learning for precision agriculture: a comprehensive perspective on applications.
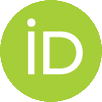
1. Introduction
2. remote sensing technology and the machine learning method, 2.1. remote sensing data in precision agriculture, 2.2. overview of the use of ml algorithms in precision agriculture, 3. integrated application of remote sensing technology and the machine learning method, 3.1. agricultural monitoring and identification, 3.2. stress detection of diseases and insect pests, 3.3. management and analysis of soil and land, 3.4. prediction and decision making regarding crop yield, 4. discussion, 4.1. current challenges, 4.1.1. acquisition and processing of multi-source rs data, 4.1.2. interpretability and generalization of the model, 4.2. prospects for the future, 4.2.1. trend of intelligence and automation, 4.2.2. data sharing and multidisciplinary interaction, 5. conclusions, author contributions, data availability statement, conflicts of interest.
- Tran, T.-N.-D.; Lakshmi, V. Enhancing human resilience against climate change: Assessment of hydroclimatic extremes and sea level rise impacts on the Eastern Shore of Virginia, United States. Sci. Total Environ. 2024 , 947 , 174289. [ Google Scholar ] [ CrossRef ] [ PubMed ]
- Tran, T.-N.-D.; Nguyen, B.Q.; Grodzka-Lukaszewska, M.; Sinicyn, G.; Lakshmi, V. The role of reservoirs under the impacts of climate change on the Srepok River basin, Central Highlands of Vietnam. Front. Environ. 2023 , 11 , 1304845. [ Google Scholar ] [ CrossRef ]
- Tran, T.-N.-D.; Tapas, M.R.; Do, S.K.; Etheridge, R.; Lakshmi, V. Investigating the impacts of climate change on hydroclimatic extremes in the Tar-Pamlico River basin, North Carolina. J. Environ. Manag. 2024 , 363 , 121375. [ Google Scholar ] [ CrossRef ]
- Tran, T.N.D.; Do, S.K.; Nguyen, B.Q.; Tran, V.N.; Grodzka-Łukaszewska, M.; Sinicyn, G.; Lakshmi, V. Investigating the Future Flood and Drought Shifts in the Transboundary Srepok River Basin Using CMIP6 Projections. IEEE J. Sel. Top. Appl. Earth Obs. Remote Sens. 2024 , 17 , 7516–7529. [ Google Scholar ] [ CrossRef ]
- Matton, N.; Canto, G.S.; Waldner, F.; Valero, S.; Morin, D.; Inglada, J.; Arias, M.; Bontemps, S.; Koetz, B.; Defourny, P. An Automated Method for Annual Cropland Mapping along the Season for Various Globally-Distributed Agrosystems Using High Spatial and Temporal Resolution Time Series. Remote Sens. 2015 , 7 , 13208–13232. [ Google Scholar ] [ CrossRef ]
- Alavi, M.; Albaji, M.; Golabi, M.; Ali Naseri, A.; Homayouni, S. Estimation of sugarcane evapotranspiration from remote sensing and limited meteorological variables using machine learning models. J. Hydrol. 2024 , 629 , 130605. [ Google Scholar ] [ CrossRef ]
- Sadiq, M.A.; Sarkar, S.K.; Raisa, S.S. Meteorological drought assessment in northern Bangladesh: A machine learning-based approach considering remote sensing indices. Ecol. Indic. 2023 , 157 , 111233. [ Google Scholar ] [ CrossRef ]
- Bellvert, J.; Mata, M.; Vallverdú, X.; Paris, C.; Marsal, J. Optimizing precision irrigation of a vineyard to improve water use efficiency and profitability by using a decision-oriented vine water consumption model. Precis. Agric. 2021 , 22 , 319–341. [ Google Scholar ] [ CrossRef ]
- Yomo, M.; Yalo, E.N.; Gnazou, M.D.-T.; Silliman, S.; Larbi, I.; Mourad, K.A. Forecasting land use and land cover dynamics using combined remote sensing, machine learning algorithm and local perception in the Agoènyivé Plateau, Togo. Remote Sens. Appl. Soc. Environ. 2023 , 30 , 100928. [ Google Scholar ] [ CrossRef ]
- Kumar, M.; Bhattacharya, B.K.; Pandya, M.R.; Handique, B.K. Machine learning based plot level rice lodging assessment using multi-spectral UAV remote sensing. Comput. Electron. Agric. 2024 , 219 , 108754. [ Google Scholar ] [ CrossRef ]
- Kganyago, M.; Adjorlolo, C.; Mhangara, P.; Tsoeleng, L. Optical remote sensing of crop biophysical and biochemical parameters: An overview of advances in sensor technologies and machine learning algorithms for precision agriculture. Comput. Electron. Agric. 2024 , 218 , 108730. [ Google Scholar ] [ CrossRef ]
- Petrović, B.; Bumbálek, R.; Zoubek, T.; Kuneš, R.; Smutný, L.; Bartoš, P. Application of precision agriculture technologies in Central Europe-review. J. Agric. Food Res. 2024 , 15 , 101048. [ Google Scholar ] [ CrossRef ]
- Mana, A.A.; Allouhi, A.; Hamrani, A.; Rehman, S.; el Jamaoui, I.; Jayachandran, K. Sustainable AI-based production agriculture: Exploring AI applications and implications in agricultural practices. Smart Agric. Technol. 2024 , 7 , 100416. [ Google Scholar ] [ CrossRef ]
- Brewster, C.; Roussaki, I.; Kalatzis, N.; Doolin, K.; Ellis, K. IoT in Agriculture: Designing a Europe-Wide Large-Scale Pilot. IEEE Commun. Mag. 2017 , 55 , 26–33. [ Google Scholar ] [ CrossRef ]
- Shuai, L.; Li, Z.; Chen, Z.; Luo, D.; Mu, J. A research review on deep learning combined with hyperspectral Imaging in multiscale agricultural sensing. Comput. Electron. Agric. 2024 , 217 , 108577. [ Google Scholar ] [ CrossRef ]
- Diaz-Gonzalez, F.A.; Vuelvas, J.; Correa, C.A.; Vallejo, V.E.; Patino, D. Machine learning and remote sensing techniques applied to estimate soil indicators. Review Ecol. Indic. 2022 , 135 , 108517. [ Google Scholar ] [ CrossRef ]
- El-Omairi, M.A.; El Garouani, A. A review on advancements in lithological mapping utilizing machine learning algorithms and remote sensing data. Heliyon 2023 , 9 , e20168. [ Google Scholar ] [ CrossRef ]
- Kasampalis, D.A.; Alexandridis, T.K.; Deva, C.; Challinor, A.; Moshou, D.; Zalidis, G. Contribution of Remote Sensing on Crop Models: A Review. J. Imaging 2018 , 4 , 52. [ Google Scholar ] [ CrossRef ]
- Tran, T.-N.-D.; Nguyen, B.Q.; Zhang, R.; Aryal, A.; Grodzka-Lukaszewska, M.; Sinicyn, G.; Lakshmi, V. Quantification of Gridded Precipitation Products for the Streamflow Simulation on the Mekong River Basin Using Rainfall Assessment Framework: A Case Study for the Srepok River Subbasin, Central Highland Vietnam. Remote Sens. 2023 , 15 , 1030. [ Google Scholar ] [ CrossRef ]
- Tran, T.-N.-D.; Le, M.-H.; Zhang, R.; Nguyen, B.Q.; Bolten, J.D.; Lakshmi, V. Robustness of gridded precipitation products for vietnam basins using the comprehensive assessment framework of rainfall. Atmos. Res. 2023 , 293 , 106923. [ Google Scholar ] [ CrossRef ]
- Tran, T.-N.-D.; Nguyen, Q.B.; Vo, N.D.; Marshall, R.; Gourbesville, P. Assessment of Terrain Scenario Impacts on Hydrological Simulation with SWAT Model. Application to Lai Giang Catchment, Vietnam. In Advances in Hydroinformatics ; Springer: Singapore, 2022; pp. 1205–1222. [ Google Scholar ]
- Aryal, A.; Tran, T.-N.-D.; Kumar, B.; Lakshmi, V. Evaluation of Satellite-Derived Precipitation Products for Streamflow Simulation of a Mountainous Himalayan Watershed: A Study of Myagdi Khola in Kali Gandaki Basin, Nepal. Remote Sens. 2023 , 15 , 4762. [ Google Scholar ] [ CrossRef ]
- Mani, P.K.; Mandal, A.; Biswas, S.; Sarkar, B.; Mitran, T.; Meena, R.S. Remote Sensing and Geographic Information System: In A Tool for Precision Farming ; Mitran, T., Meena, R.S., Chakraborty, A., Eds.; Geospatial Technologies for Crops and Soils; Springer: Singapore, 2021; pp. 49–111. [ Google Scholar ]
- Carneiro, F.M.; Filho, A.L.d.B.; Ferreira, F.M.; Junior, G.d.F.S.; Brandão, Z.N.; da Silva, R.P.; Shiratsuchi, L.S. Soil and satellite remote sensing variables importance using machine learning to predict cotton yield. Smart Agric. Technol. 2023 , 5 , 100292. [ Google Scholar ] [ CrossRef ]
- Morlin Carneiro, F.; Angeli Furlani, C.E.; Zerbato, C.; Candida de Menezes, P.; da Silva Gírio, L.A.; Freire de Oliveira, M. Comparison between vegetation indices for detecting spatial and temporal variabilities in soybean crop using canopy sensors. Precis. Agric. 2020 , 21 , 979–1007. [ Google Scholar ] [ CrossRef ]
- Ai, B.; Wen, Z.; Jiang, Y.C.; Gao, S.; Lv, G.N. Sea surface temperature inversion model for infrared remote sensing images based on deep neural network. Infrared Phys. Technol. 2019 , 99 , 231–239. [ Google Scholar ] [ CrossRef ]
- Zhang, W.H.; Sun, L.; Lian, L.S.; Yang, Y.K. MODIS Aerosol Optical Depth Inversion Over Urban Areas Supported by BRDF/Albedo Products. J. Indian Soc. Remote Sens. 2020 , 48 , 1345–1354. [ Google Scholar ] [ CrossRef ]
- Aires, F.; Pellet, V. Estimating Retrieval Errors from Neural Network Inversion Schemes—Application to the Retrieval of Temperature Profiles From IASI. IEEE Trans. Geosci. Remote Sens. 2021 , 59 , 6386–6396. [ Google Scholar ] [ CrossRef ]
- Liu, B.; Liu, L.; Tian, L.; Cao, W.; Zhu, Y.; Asseng, S. Post-heading heat stress and yield impact in winter wheat of China. Glob. Change Biol. 2014 , 20 , 372–381. [ Google Scholar ] [ CrossRef ] [ PubMed ]
- Akter, N.; Rafiqul Islam, M. Heat stress effects and management in wheat. A review. Agron. Sustain. Dev. 2017 , 37 , 37. [ Google Scholar ] [ CrossRef ]
- Wójtowicz, M.; Wójtowicz, A.; Piekarczyk, J. Application of remote sensing methods in agriculture. Commun. Biometry Crop Sci. 2016 , 11 , 31–50. [ Google Scholar ]
- Skendžić, S.; Zovko, M.; Lešić, V.; Pajač Živković, I.; Lemić, D. Detection and Evaluation of Environmental Stress in Winter Wheat Using Remote and Proximal Sensing Methods and Vegetation Indices—A review. Diversity 2023 , 15 , 481. [ Google Scholar ] [ CrossRef ]
- Kumar, A.S.; Reddy, A.M.; Srinivas, L.; Reddy, P.M. Assessment of Surface Water Quality in Hyderabad Lakes by Using Multivariate Statistical Techniques, Hyderabad-India. Environ. Pollut. 2015 , 4 , 4. [ Google Scholar ] [ CrossRef ]
- Odermatt, D.; Danne, O.; Philipson, P.; Brockmann, C. Diversity II water quality parameters from ENVISAT (2002–2012): A new global information source for lakes. Earth Syst. Sci. Data. 2018 , 10 , 1527–1549. [ Google Scholar ] [ CrossRef ]
- Shang, P.; Shen, F. Atmospheric Correction of Satellite GF-1/WFV Imagery and Quantitative Estimation of Suspended Particulate Matter in the Yangtze Estuary. Sensors 2016 , 16 , 1997. [ Google Scholar ] [ CrossRef ] [ PubMed ]
- Colomina, I.; Molina, P. Unmanned aerial systems for photogrammetry and remote sensing: A review. ISPRS J. Photogramm. Remote Sens. 2014 , 92 , 79–97. [ Google Scholar ] [ CrossRef ]
- Lee, C.-J.; Yang, M.-D.; Tseng, H.-H.; Hsu, Y.-C.; Sung, Y.; Chen, W.-L. Single-plant broccoli growth monitoring using deep learning with UAV imagery. Comput. Electron. Agric. 2023 , 207 , 107739. [ Google Scholar ] [ CrossRef ]
- Marques, T.; Carreira, S.; Miragaia, R.; Ramos, J.; Pereira, A. Applying deep learning to real-time UAV-based forest monitoring: Leveraging multi-sensor imagery for improved results. Expert Syst. Appl. 2024 , 245 , 123107. [ Google Scholar ] [ CrossRef ]
- Bah, M.D.; Hafiane, A.; Canals, R. Weeds detection in UAV imagery using SLIC and the hough transform. In Proceedings of the 7th International Conference on Image Processing Theory, Tools and Applications, Montreal, QC, Canada, 28 November–1 December 2017; pp. 1–6. [ Google Scholar ]
- Yang, M.-D.; Huang, K.-S.; Kuo, Y.-H.; Tsai, H.P.; Lin, L.-M. Spatial and Spectral Hybrid Image Classification for Rice Lodging Assessment through UAV Imagery. Remote Sens. 2017 , 9 , 583. [ Google Scholar ] [ CrossRef ]
- Yang, Q.; She, B.; Huang, L.S.; Yang, Y.Y.; Zhang, G.; Zhang, M.; Hong, Q.; Zhang, D.Y. Extraction of soybean planting area based on feature fusion technology of multi-source low altitude unmanned aerial vehicle images. Ecol. Inform. 2022 , 70 , 101715. [ Google Scholar ] [ CrossRef ]
- Maimaitijiang, M.; Sagan, V.; Sidike, P.; Hartling, S.; Esposito, F.; Fritschi, F.B. Soybean yield prediction from UAV using multimodal data fusion and deep learning. Remote Sens. Environ. 2020 , 237 , 111599. [ Google Scholar ] [ CrossRef ]
- Peng, J.B.; Wang, D.L.; Zhu, W.X.; Yang, T.; Liu, Z.; Rezaei, E.E.; Li, J.; Sun, Z.G.; Xin, X.P. Combination of UAV and deep learning to estimate wheat yield at ripening stage: The potential of phenotypic features. Int. J. Appl. Earth Obs. Geoinf. 2023 , 124 , 103494. [ Google Scholar ] [ CrossRef ]
- Khan, A.; Vibhute, A.D.; Mali, S.; Patil, C.H. A systematic review on hyperspectral imaging technology with a machine and deep learning methodology for agricultural applications. Ecol. Inform. 2022 , 69 , 101678. [ Google Scholar ] [ CrossRef ]
- Han, W.; Zhang, X.; Wang, Y.; Wang, L.; Huang, X.; Li, J.; Wang, S.; Chen, W.; Li, X.; Feng, R.; et al. A survey of machine learning and deep learning in remote sensing of geological environment: Challenges, advances, and opportunities. ISPRS J. Photogramm. Remote Sens. 2023 , 202 , 87–113. [ Google Scholar ] [ CrossRef ]
- Coulibaly, S.; Kamsu-Foguem, B.; Kamissoko, D.; Traore, D. Deep learning for precision agriculture: A bibliometric analysis. Intelligent Syst. Appl. 2022 , 16 , 200102. [ Google Scholar ] [ CrossRef ]
- Liakos, K.G.; Busato, P.; Moshou, D.; Pearson, S.; Bochtis, D. Machine Learning in Agriculture: A review. Sensors 2018 , 18 , 2674. [ Google Scholar ] [ CrossRef ]
- Sarkar, C.; Gupta, D.; Gupta, U.; Hazarika, B.B. Leaf disease detection using machine learning and deep learning: Review and challenges. Appl. Soft Comput. 2023 , 145 , 110534. [ Google Scholar ] [ CrossRef ]
- Miao, Z.H.; Yu, X.Y.; Li, N.; Zhang, Z.; He, C.X.; Li, Z.; Deng, C.Y.; Sun, T. Efficient tomato harvesting robot based on image processing and deep learning. Precis. Agric. 2023 , 24 , 254–287. [ Google Scholar ] [ CrossRef ]
- Fu, Y.; Yang, G.; Pu, R.; Li, Z.; Li, H.; Xu, X.; Song, X.; Yang, X.; Zhao, C. An overview of crop nitrogen status assessment using hyperspectral remote sensing: Current status and perspectives. Eur. J. Agron. 2021 , 124 , 126241. [ Google Scholar ] [ CrossRef ]
- Casagli, N.; Cigna, F.; Bianchini, S.; Hölbling, D.; Füreder, P.; Righini, G.; Del Conte, S.; Friedl, B.; Schneiderbauer, S.; Iasio, C.; et al. Landslide mapping and monitoring by using radar and optical remote sensing: Examples from the EC-FP7 project SAFER. Remote Sens. Appl. Soc. Environ. 2016 , 4 , 92–108. [ Google Scholar ] [ CrossRef ]
- Knoll, F.J.; Czymmek, V.; Poczihoski, S.; Holtorf, T.; Hussmann, S. Improving efficiency of organic farming by using a deep learning classification approach. Comput. Electron. Agric. 2018 , 153 , 347–356. [ Google Scholar ] [ CrossRef ]
- Ouma, Y.O. Advancements in medium and high resolution Earth observation for land-surface imaging: Evolutions, future trends and contributions to sustainable development. Adv. Space Res. 2016 , 57 , 110–126. [ Google Scholar ] [ CrossRef ]
- Sofia, G. Combining geomorphometry, feature extraction techniques and Earth-surface processes research: The way forward. Geomorphology 2020 , 355 , 107055. [ Google Scholar ] [ CrossRef ]
- Saha, A.; Chandra Pal, S. Application of machine learning and emerging remote sensing techniques in hydrology: A state-of-the-art review and current research trends. J. Hydrol. 2024 , 632 , 130907. [ Google Scholar ] [ CrossRef ]
- Rodi, N.S.N.; Malek, M.A.; Ismail, A.R. Monthly Rainfall Prediction Model of Peninsular Malaysia Using Clonal Selection Algorithm. Int. J. Eng. Technol. 2018 , 7 , 182–185. [ Google Scholar ] [ CrossRef ]
- Latif, S.D.; Alyaa Binti Hazrin, N.; Hoon Koo, C.; Lin Ng, J.; Chaplot, B.; Feng Huang, Y.; El-Shafie, A.; Najah Ahmed, A. Assessing rainfall prediction models: Exploring the advantages of machine learning and remote sensing approaches. Alex. Eng. J. 2023 , 82 , 16–25. [ Google Scholar ] [ CrossRef ]
- Khanal, S.; Fulton, J.; Shearer, S. An overview of current and potential applications of thermal remote sensing in precision agriculture. Comput. Electron. Agric. 2017 , 139 , 22–32. [ Google Scholar ] [ CrossRef ]
- Ahmed, Z.; Shew, A.; Nalley, L.; Popp, M.; Green, V.S.; Brye, K. An examination of thematic research, development, and trends in remote sensing applied to conservation agriculture. Int. Soil Water Conserv. Res. 2024 , 12 , 77–95. [ Google Scholar ] [ CrossRef ]
- Jafarbiglu, H.; Pourreza, A. A comprehensive review of remote sensing platforms, sensors, and applications in nut crops. Comput. Electron. Agric. 2022 , 197 , 106844. [ Google Scholar ] [ CrossRef ]
- Degerickx, J.; Roberts, D.A.; McFadden, J.P.; Hermy, M.; Somers, B. Urban tree health assessment using airborne hyperspectral and LiDAR imagery. Int. J. Appl. Earth Obs. Geoinf. 2018 , 73 , 26–38. [ Google Scholar ] [ CrossRef ]
- Duan, M.; Wang, Z.; Sun, L.; Liu, Y.; Yang, P. Monitoring apple flowering date at 10 m spatial resolution based on crop reference curves. Comput. Electron. Agric. 2024 , 225 , 109260. [ Google Scholar ] [ CrossRef ]
- Meng, R.; Gao, R.; Zhao, F.; Huang, C.; Sun, R.; Lv, Z.; Huang, Z. Landsat-based monitoring of southern pine beetle infestation severity and severity change in a temperate mixed forest. Remote Sens. Environ. 2022 , 269 , 112847. [ Google Scholar ] [ CrossRef ]
- Wu, B.; Liang, A.; Zhang, H.; Zhu, T.; Zou, Z.; Yang, D.; Tang, W.; Li, J.; Su, J. Application of conventional UAV-based high-throughput object detection to the early diagnosis of pine wilt disease by deep learning. For. Ecol. Manag. 2021 , 486 , 118986. [ Google Scholar ] [ CrossRef ]
- Zhu, X.; Wang, R.; Shi, W.; Yu, Q.; Li, X.; Chen, X. Automatic Detection and Classification of Dead Nematode-Infested Pine Wood in Stages Based on YOLO v4 and GoogLeNet. Forests 2023 , 14 , 601. [ Google Scholar ] [ CrossRef ]
- Luo, Y.; Huang, H.; Roques, A. Early Monitoring of Forest Wood-Boring Pests with Remote Sensing. Annu. Rev. Entomol. 2023 , 68 , 277–298. [ Google Scholar ] [ CrossRef ] [ PubMed ]
- Ren, S.; Chen, H.; Hou, J.; Zhao, P.; Dong Qg Feng, H. Based on historical weather data to predict summer field-scale maize yield: Assimilation of remote sensing data to WOFOST model by ensemble Kalman filter algorithm. Comput. Electron. Agric. 2024 , 219 , 108822. [ Google Scholar ] [ CrossRef ]
- Guerrero, N.M.; Aparicio, J.; Valero-Carreras, D. Combining Data Envelopment Analysis and Machine Learning. Mathematics 2022 , 10 , 909. [ Google Scholar ] [ CrossRef ]
- Sharma, A.; Jain, A.; Gupta, P.; Chowdary, V. Machine Learning Applications for Precision Agriculture: A Comprehensive Review. IEEE Access 2021 , 9 , 4843–4873. [ Google Scholar ] [ CrossRef ]
- Behmann, J.; Mahlein, A.K.; Rumpf, T.; Römer, C.; Plümer, L. A review of advanced machine learning methods for the detection of biotic stress in precision crop protection. Precis. Agric. 2015 , 16 , 239–260. [ Google Scholar ] [ CrossRef ]
- Helm, J.M.; Swiergosz, A.M.; Haeberle, H.S.; Karnuta, J.M.; Schaffer, J.L.; Krebs, V.E.; Spitzer, A.I.; Ramkumar, P.N. Machine Learning and Artificial Intelligence: Definitions, Applications, and Future Directions. Curr. Rev. Musculoskelet. Med. 2020 , 13 , 69–76. [ Google Scholar ] [ CrossRef ]
- Gao, Z.; Luo, Z.; Zhang, W.; Lv, Z.; Xu, Y. Deep Learning Application in Plant Stress Imaging: A Review. AgriEngineering 2020 , 2 , 430–446. [ Google Scholar ] [ CrossRef ]
- Benos, L.; Tagarakis, A.C.; Dolias, G.; Berruto, R.; Kateris, D.; Bochtis, D. Machine Learning in Agriculture: A Comprehensive Updated Review. Sensors 2021 , 21 , 3758. [ Google Scholar ] [ CrossRef ]
- Choi, R.Y.; Coyner, A.S.; Kalpathy-Cramer, J.; Chiang, M.F.; Campbell, J.P. Introduction to Machine Learning, Neural Networks, and Deep Learning. Transl. Vis. Sci. Technol. 2020 , 9 , 14. [ Google Scholar ] [ CrossRef ] [ PubMed ]
- Simeone, O. A Very Brief Introduction to Machine Learning with Applications to Communication Systems. IEEE Trans. Cogn. Commun. Netw. 2018 , 4 , 648–664. [ Google Scholar ] [ CrossRef ]
- Albarakati, H.M.; Khan, M.A.; Hamza, A.; Khan, F.; Kraiem, N.; Jamel, L.; Almuqren, L.; Alroobaea, R. A Novel Deep Learning Architecture for Agriculture Land Cover and Land Use Classification from Remote Sensing Images Based on Network-Level Fusion of Self-Attention Architecture. IEEE J. Sel. Top. Appl. Earth Obs. Remote Sens. 2024 , 17 , 6338–6353. [ Google Scholar ] [ CrossRef ]
- Finley, A.O.; Andersen, H.E.; Babcock, C.; Cook, B.D.; Morton, D.C.; Banerjee, S. Models to Support Forest Inventory and Small Area Estimation Using Sparsely Sampled LiDAR: A Case Study Involving G-LiHT LiDAR in Tanana, Alaska. J. Agric. Biol. Environ. Stat. 2024 , 28 . [ Google Scholar ] [ CrossRef ]
- Shafik, W.; Tufail, A.; Namoun, A.; De Silva, L.C.; Apong, R. A Systematic Literature Review on Plant Disease Detection: Motivations, Classification Techniques, Datasets, Challenges, and Future Trends. IEEE Access 2023 , 11 , 59174–59203. [ Google Scholar ] [ CrossRef ]
- El Akhal, H.; Ben Yahya, A.; Moussa, N.; El Alaouil, A.E. A novel approach for image-based olive leaf diseases classification using a deep hybrid model. Ecol. Inform. 2023 , 77 , 102276. [ Google Scholar ] [ CrossRef ]
- Abbas, F.; Afzaal, H.; Farooque, A.A.; Tang, S. Crop Yield Prediction through Proximal Sensing and Machine Learning Algorithms. Agronomy 2020 , 10 , 1046. [ Google Scholar ] [ CrossRef ]
- Fu, Z.P.; Jiang, J.; Gao, Y.; Krienke, B.; Wang, M.; Zhong, K.T.; Cao, Q.; Tian, Y.C.; Zhu, Y.; Cao, W.X.; et al. Wheat Growth Monitoring and Yield Estimation based on Multi-Rotor Unmanned Aerial Vehicle. Remote Sens. 2020 , 12 , 508. [ Google Scholar ] [ CrossRef ]
- Guo, H.L.; Zhang, R.R.; Dai, W.H.; Zhou, X.W.; Zhang, D.J.; Yang, Y.H.; Cui, J. Mapping Soil Organic Matter Content Based on Feature Band Selection with ZY1-02D Hyperspectral Satellite Data in the Agricultural Region. Agronomy 2022 , 12 , 2111. [ Google Scholar ] [ CrossRef ]
- Erler, A.; Riebe, D.; Beitz, T.; Löhmannsröben, H.G.; Gebbers, R. Soil Nutrient Detection for Precision Agriculture Using Handheld Laser-Induced Breakdown Spectroscopy (LIBS) and Multivariate Regression Methods (PLSR, Lasso and GPR). Sensors 2020 , 20 , 418. [ Google Scholar ] [ CrossRef ]
- Yoon, H.I.; Lee, H.; Yang, J.S.; Choi, J.H.; Jung, D.H.; Park, Y.J.; Park, J.E.; Kim, S.M.; Park, S.H. Predicting Models for Plant Metabolites Based on PLSR, AdaBoost, XGBoost, and LightGBM Algorithms Using Hyperspectral Imaging Brassica juncea . Agriculture 2023 , 13 , 1477. [ Google Scholar ] [ CrossRef ]
- Bakhshipour, A. Cascading Feature Filtering and Boosting Algorithm for Plant Type Classification Based on Image Features. IEEE Access 2021 , 9 , 82021–82030. [ Google Scholar ] [ CrossRef ]
- Luo, L.L.; Chang, Q.R.; Wang, Q.; Huang, Y. Identification and Severity Monitoring of Maize Dwarf Mosaic Virus Infection Based on Hyperspectral Measurements. Remote Sens. 2021 , 13 , 4560. [ Google Scholar ] [ CrossRef ]
- Shinde, S.; Patidar, H. Hyperspectral Image Classification for Vegetation Detection Using Lightweight Cascaded Deep Convolutional Neural Network. J. Indian Soc. Remote Sens. 2023 , 51 , 2159–2166. [ Google Scholar ] [ CrossRef ]
- Barbedo, J.G.A.; Koenigkan, L.V.; Santos, P.M.; Ribeiro, A.R.B. Counting Cattle in UAV Images—Dealing with Clustered Animals and Animal/Background Contrast Changes. Sensors 2020 , 20 , 2126. [ Google Scholar ] [ CrossRef ]
- Han, T.; Hu, X.M.; Zhang, J.; Xue, W.H.; Che, Y.F.; Deng, X.Q.; Zhou, L.H. Rebuilding high-quality near-surface ozone data based on the combination of WRF-Chem model with a machine learning method to better estimate its impact on crop yields in the Beijing-Tianjin-Hebei region from 2014 to 2019. Environ. Pollut. 2023 , 336 , 122334. [ Google Scholar ] [ CrossRef ]
- Gauci, A.; Abela, J.; Austad, M.; Cassar, L.F.; Zarb Adami, K. A Machine Learning approach for automatic land cover mapping from DSLR images over the Maltese Islands. Environ. Model. Softw. 2018 , 99 , 1–10. [ Google Scholar ] [ CrossRef ]
- Idol, T.; Haack, B.; Mahabir, R. Radar speckle reduction and derived texture measures for land cover/use classification: A case study. Geocarto Int. 2017 , 32 , 18–29. [ Google Scholar ] [ CrossRef ]
- Li, L.; Dong, Y.Y.; Xiao, Y.X.; Liu, L.Y.; Zhao, X.; Huang, W.J. Combining Disease Mechanism and Machine Learning to Predict Wheat Fusarium Head Blight. Remote Sens. 2022 , 14 , 2732. [ Google Scholar ] [ CrossRef ]
- Bebie, M.; Cavalaris, C.; Kyparissis, A. Assessing Durum Wheat Yield through Sentinel-2 Imagery: A Machine Learning Approach. Remote Sens. 2022 , 14 , 3880. [ Google Scholar ] [ CrossRef ]
- Zhou, Y.N.; Luo, J.C.; Feng, L.; Yang, Y.P.; Chen, Y.H.; Wu, W. Long-short-term-memory-based crop classification using high-resolution optical images and multi-temporal SAR data. GISci. Remote Sens. 2019 , 56 , 1170–1191. [ Google Scholar ] [ CrossRef ]
- Jimenez, A.F.; Ortiz, B.V.; Bondesan, L.; Morata, G.; Damianidis, D. Long Short-Term Memory Neural Network for irrigation management: A case study from Southern Alabama, USA. Precis. Agric. 2021 , 22 , 475–492. [ Google Scholar ] [ CrossRef ]
- Chen, C.; Bao, Y.X.; Zhu, F.; Yang, R.M. Remote sensing monitoring of rice growth under Cnaphalocrocis medinalis (Guenée) damage by integrating satellite and UAV remote sensing data. Int. J. Remote Sens. 2024 , 45 , 772–790. [ Google Scholar ] [ CrossRef ]
- Dumdumaya, C.E.; Cabrera, J.S. Determination of future land use changes using remote sensing imagery and artificial neural network algorithm: A case study of Davao City, Philippines. Artif. Intell. Geosci. 2023 , 4 , 111–118. [ Google Scholar ] [ CrossRef ]
- Bao Pham, Q.; Ajim Ali, S.; Parvin, F.; Van On, V.; Mohd Sidek, L.; Đurin, B.; Cetl, V.; Šamanović, S.; Nguyet Minh, N. Multi-spectral remote sensing and GIS-based analysis for decadal land use land cover changes and future prediction using random forest tree and artificial neural network. Adv. Space Res. 2024 , 10 , 29900–29926. [ Google Scholar ] [ CrossRef ]
- Zhang, J.; Zhang, Y.; Zhou, T.; Sun, Y.; Yang, Z.; Zheng, S. Research on the identification of land types and tree species in the Engebei ecological demonstration area based on GF-1 remote sensing. Ecol. Inform. 2023 , 77 , 102242. [ Google Scholar ] [ CrossRef ]
- Belgiu, M.; Drăguţ, L. Random forest in remote sensing: A review of applications and future directions. ISPRS Journal of Photogrammetry and Remote Sens. 2016 , 114 , 24–31. [ Google Scholar ] [ CrossRef ]
- Whyte, A.; Ferentinos, K.P.; Petropoulos, G.P. A new synergistic approach for monitoring wetlands using Sentinels -1 and 2 data with object-based machine learning algorithms. Environ. Model. Softw. 2018 , 104 , 40–54. [ Google Scholar ] [ CrossRef ]
- Ali, M.Z.; Qazi, W.; Aslam, N. A comparative study of ALOS-2 PALSAR and landsat-8 imagery for land cover classification using maximum likelihood classifier. Egypt J. Remote Sens. Space Sci. 2018 , 21 , S29–S35. [ Google Scholar ] [ CrossRef ]
- Ghayour, L.; Neshat, A.; Paryani, S.; Shahabi, H.; Shirzadi, A.; Chen, W.; Al-Ansari, N.; Geertsema, M.; Pourmehdi Amiri, M.; Gholamnia, M.; et al. Performance Evaluation of Sentinel-2 and Landsat 8 OLI Data for Land Cover/Use Classification Using a Comparison between Machine Learning Algorithms. Remote Sens. 2021 , 13 , 1349. [ Google Scholar ] [ CrossRef ]
- Nguyen, T.T.; Ngo, H.H.; Guo, W.S.; Chang, S.W.; Nguyen, D.D.; Nguyen, C.T.; Zhang, J.; Liang, S.; Bui, X.T.; Hoang, N.B. A low-cost approach for soil moisture prediction using multi-sensor data and machine learning algorithm. Sci. Total Environ. 2022 , 833 , 12–155066. [ Google Scholar ] [ CrossRef ] [ PubMed ]
- Liu, Y.; Sun, Q.; Huang, J.; Feng, H.K.; Wang, J.J.; Yang, G.J. Estimation of Potato Above Ground Biomass Based on UAV Multispectral Images. Spectrosc. Spectr. Anal. 2021 , 41 , 2549–2555. [ Google Scholar ]
- Li, Z.P.; Zhou, X.G.; Cheng, Q.; Fei, S.P.; Chen, Z. A Machine-Learning Model Based on the Fusion of Spectral and Textural Features from UAV Multi-Sensors to Analyse the Total Nitrogen Content in Winter Wheat. Remote Sens. 2023 , 15 , 2152. [ Google Scholar ] [ CrossRef ]
- Pejak, B.; Lugonja, P.; Antic, A.; Panic, M.; Pandzic, M.; Alexakis, E.; Mavrepis, P.; Zhou, N.A.; Marko, O.; Crnojevic, V. Soya Yield Prediction on a Within-Field Scale Using Machine Learning Models Trained on Sentinel-2 and Soil Data. Remote Sens. 2022 , 14 , 2256. [ Google Scholar ] [ CrossRef ]
- Ye, Y.; Huang, Q.Q.; Rong, Y.; Yu, X.H.; Liang, W.J.; Chen, Y.X.; Xiong, S.W. Field detection of small pests through stochastic gradient descent with genetic algorithm. Comput. Electron. Agric. 2023 , 206 , 107694. [ Google Scholar ] [ CrossRef ]
- Zualkernan, I.; Abuhani, D.A.; Hussain, M.H.; Khan, J.; El Mohandes, M. Machine Learning for Precision Agriculture Using Imagery from Unmanned Aerial Vehicles (UAVs): A Survey. Drones 2023 , 7 , 382. [ Google Scholar ] [ CrossRef ]
- Khan, S.; Tufail, M.; Khan, M.T.; Khan, Z.A.; Iqbal, J.; Alam, M. A novel semi-supervised framework for UAV based crop/weed classification. PLoS ONE 2021 , 16 , e0251008. [ Google Scholar ] [ CrossRef ]
- Mujkic, E.; Philipsen, M.P.; Moeslund, T.B.; Christiansen, M.P.; Ravn, O. Anomaly Detection for Agricultural Vehicles Using Autoencoders. Sensors 2022 , 22 , 3608. [ Google Scholar ] [ CrossRef ]
- Chen, X.; Zhang, C.; Yan, K.; Wei, Z.; Cheng, N. Risk Assessment of Agricultural Soil Heavy Metal Pollution Under the Hybrid Intelligent Evaluation Model. IEEE Access 2023 , 11 , 106847–106858. [ Google Scholar ] [ CrossRef ]
- Alvarenga, T.C.; De Lima, R.R.; Simao, S.D.; Brandao Junior, L.C.; Bueno Filho, J.S.D.S.; Alvarenga, R.R.; Rodrigues, P.B.; Leite, D.F. Ensemble of hybrid Bayesian networks for predicting the AMEn of broiler feedstuffs. Comput. Electron. Agric. 2022 , 198 , 107067. [ Google Scholar ] [ CrossRef ]
- Lu, Q.K.; Xie, Y.P.; Wei, L.F.; Wei, Z.Y.; Tian, S.; Liu, H.; Cao, L. Extended Attribute Profiles for Precise Crop Classification in UAV-Borne Hyperspectral Imagery. IEEE Geosci. Remote Sens. Lett. 2024 , 21 , 2500805. [ Google Scholar ] [ CrossRef ]
- Maeda, N.; Tonooka, H. Early Stage Forest Fire Detection from Himawari-8 AHI Images Using a Modified MOD14 Algorithm Combined with Machine Learning. Sensors 2023 , 23 , 210. [ Google Scholar ] [ CrossRef ]
- Furuya, D.E.G.; Ma, L.F.; Pinheiro, M.M.F.; Gomes, F.D.G.; Gonçalvez, W.N.; Marcato, J.; Rodrigues, D.D.; Blassioli-Moraes, M.C.; Michereff, M.F.F.; Borges, M.; et al. Prediction of insect-herbivory-damage and insect-type attack in maize plants using hyperspectral data. Int. J. Appl. Earth Obs. Geoinf. 2021 , 105 , 102608. [ Google Scholar ] [ CrossRef ]
- Javadi, S.H.; Guerrero, A.; Mouazen, A.M. Clustering and Smoothing Pipeline for Management Zone Delineation Using Proximal and Remote Sensing. Sensors 2022 , 22 , 645. [ Google Scholar ] [ CrossRef ]
- Devarajan, G.G.; Nagarajan, S.M.; Ramana, T.V.; Vignesh, T.; Ghosh, U.; Alnumay, W. DDNSAS: Deep reinforcement learning based deep Q-learning network for smart agriculture system. Sust. Comput. 2023 , 39 , 100890. [ Google Scholar ] [ CrossRef ]
- Din, A.; Ismail, M.Y.; Shah, B.B.; Babar, M.; Ali, F.; Baig, S.U. A deep reinforcement learning-based multi-agent area coverage control for smart agriculture. Comput. Electr. Eng. 2022 , 101 , 108089. [ Google Scholar ] [ CrossRef ]
- García, R.; Aguilar, J.; Toro, M.; Pinto, A.; Rodríguez, P. A systematic literature review on the use of machine learning in precision livestock farming. Comput. Electron. Agric. 2020 , 179 , 105826. [ Google Scholar ] [ CrossRef ]
- Shahab, H.; Iqbal, M.; Sohaib, A.; Ullah Khan, F.; Waqas, M. IoT-based agriculture management techniques for sustainable farming: A comprehensive review. Comput. Electron. Agric. 2024 , 220 , 108851. [ Google Scholar ] [ CrossRef ]
- Rehman, T.U.; Mahmud, M.S.; Chang, Y.K.; Jin, J.; Shin, J. Current and future applications of statistical machine learning algorithms for agricultural machine vision systems. Comput. Electron. Agric. 2019 , 156 , 585–605. [ Google Scholar ] [ CrossRef ]
- Sladojevic, S.; Arsenovic, M.; Anderla, A.; Culibrk, D.; Stefanovic, D. Deep Neural Networks Based Recognition of Plant Diseases by Leaf Image Classification. Comput. Intell. Neurosci. 2016 , 2016 , 3289801. [ Google Scholar ] [ CrossRef ] [ PubMed ]
- Li, J.; Qiao, Y.; Liu, S.; Zhang, J.; Yang, Z.; Wang, M. An improved YOLOv5-based vegetable disease detection method. Comput. Electron. Agric. 2022 , 202 , 107345. [ Google Scholar ] [ CrossRef ]
- Ashwinkumar, S.; Rajagopal, S.; Manimaran, V.; Jegajothi, B. Automated plant leaf disease detection and classification using optimal MobileNet based convolutional neural networks. Mater. Today Proc. 2022 , 51 , 480–487. [ Google Scholar ] [ CrossRef ]
- Yu, Y. Research Progress of Crop Disease Image Recognition Based on Wireless Network Communication and Deep Learning. Wirel. Commun. Mob. Comput. 2021 , 2021 , 7577349. [ Google Scholar ] [ CrossRef ]
- Ang, Y.H.; Shafri, H.Z.M.; Lee, Y.P.; Abidin, H.; Bakar, S.A.; Hashim, S.J.; Che’Ya, N.N.; Hassan, M.R.; San Lim, H.; Abdullah, R. A novel ensemble machine learning and time series approach for oil palm yield prediction using Landsat time series imagery based on NDVI. Geocarto Int. 2022 , 37 , 9865–9896. [ Google Scholar ] [ CrossRef ]
- Aydin, Y.; Isikdag, U.; Bekdas, G.; Nigdeli, S.M.; Geem, Z.W. Use of Machine Learning Techniques in Soil Classification. Sustainability 2023 , 15 , 2374. [ Google Scholar ] [ CrossRef ]
- Osco, L.P.; Nogueira, K.; Marques Ramos, A.P.; Faita Pinheiro, M.M.; Furuya, D.E.G.; Gonçalves, W.N.; de Castro Jorge, L.A.; Marcato Junior, J.; dos Santos, J.A. Semantic segmentation of citrus-orchard using deep neural networks and multispectral UAV-based imagery. Precis. Agric. 2021 , 22 , 1171–1188. [ Google Scholar ] [ CrossRef ]
- Kellenberger, B.; Marcos, D.; Tuia, D. Detecting mammals in UAV images: Best practices to address a substantially imbalanced dataset with deep learning. Remote Sens. Environ. 2018 , 216 , 139–153. [ Google Scholar ] [ CrossRef ]
- Kamath, R.; Balachandra, M.; Vardhan, A.; Maheshwari, U. Classification of paddy crop and weeds using semantic segmentation. Cogent Eng. 2022 , 9 , 2018791. [ Google Scholar ] [ CrossRef ]
- Jin, X.; Sun, Y.; Che, J.; Bagavathiannan, M.; Yu, J.; Chen, Y. A novel deep learning-based method for detection of weeds in vegetables. Pest Manag. Sci. 2022 , 78 , 1861–1869. [ Google Scholar ] [ CrossRef ]
- Xun, L.; Zhang, J.; Cao, D.; Wang, J.; Zhang, S.; Yao, F. Mapping cotton cultivated area combining remote sensing with a fused representation-based classification algorithm. Comput. Electron. Agric. 2021 , 181 , 105940. [ Google Scholar ] [ CrossRef ]
- Zhao, H.; Huang, Y.; Wang, X.; Li, X.; Lei, T. The performance of SPEI integrated remote sensing data for monitoring agricultural drought in the North China Plain. Field Crops Res. 2023 , 302 , 109041. [ Google Scholar ] [ CrossRef ]
- Lyu, X.; Li, X.; Dang, D.; Dou, H.; Xuan, X.; Liu, S.; Li, M.; Gong, J. A new method for grassland degradation monitoring by vegetation species composition using hyperspectral remote sensing. Ecol. Indic. 2020 , 114 , 106310. [ Google Scholar ] [ CrossRef ]
- Xiao, D.; Niu, H.; Guo, F.; Zhao, S.; Fan, L. Monitoring irrigation dynamics in paddy fields using spatiotemporal fusion of Sentinel-2 and MODIS. Agric. Water Manag. 2022 , 263 , 107409. [ Google Scholar ] [ CrossRef ]
- Zhang, G.; Xiao, X.; Dong, J.; Kou, W.; Jin, C.; Qin, Y.; Zhou, Y.; Wang, J.; Menarguez, M.A.; Biradar, C. Mapping paddy rice planting areas through time series analysis of MODIS land surface temperature and vegetation index data. ISPRS J. Photogramm. Remote Sens. 2015 , 106 , 157–171. [ Google Scholar ] [ CrossRef ]
- Liu, J.-R.; Liu, Q.; Khoury, J.; Li, Y.-J.; Han, X.-H.; Li, J.; Ibla, J.C. Hypoxic preconditioning decreases nuclear factor κB activity via Disrupted in Schizophrenia-1. Int. J. Biochem. Cell Biol. 2016 , 70 , 140–148. [ Google Scholar ] [ CrossRef ]
- Guo, Y.; Ren, H. Remote sensing monitoring of maize and paddy rice planting area using GF-6 WFV red edge features. Comput. Electron. Agric. 2023 , 207 , 107714. [ Google Scholar ] [ CrossRef ]
- DeVries, B.; Verbesselt, J.; Kooistra, L.; Herold, M. Robust monitoring of small-scale forest disturbances in a tropical montane forest using Landsat time series. Remote Sens. Environ. 2015 , 161 , 107–121. [ Google Scholar ] [ CrossRef ]
- Jevsenak, J.; Arnic, D.; Krajnc, L.; Skudnik, M. Machine Learning Forest Simulator (MLFS): R package for data-driven assessment of the future state of forests. Ecol. Inform. 2023 , 75 , 102115. [ Google Scholar ] [ CrossRef ]
- Bagheri Bodaghabadi, M.; Martínez-Casasnovas, J.A.; Esfandiarpour Borujeni, I.; Salehi, M.H.; Mohammadi, J.; Toomanian, N. Database extension for digital soil mapping using artificial neural networks. Arab. J. Geosci. 2016 , 9 , 701. [ Google Scholar ] [ CrossRef ]
- Dornik, A.; Drăguț, L.; Urdea, P. Classification of Soil Types Using Geographic Object-Based Image Analysis and Random Forests. Pedosphere 2018 , 28 , 913–925. [ Google Scholar ] [ CrossRef ]
- Lu, H.; Liu, C.; Li, N.; Fu, X.; Li, L. Optimal segmentation scale selection and evaluation of cultivated land objects based on high-resolution remote sensing images with spectral and texture features. Environ. Sci. Pollut. Res. 2021 , 28 , 27067–27083. [ Google Scholar ] [ CrossRef ]
- Rai, N.; Flores, P. Leveraging transfer learning in ArcGIS Pro to detect “doubles” in a sunflower field. In ASABE Annual International Virtual Meeting ; ASABE: St. Joseph, MI, USA, 2021; p. 1. [ Google Scholar ]
- Butte, S.; Vakanski, A.; Duellman, K.; Wang, H.; Mirkouei, A. Potato crop stress identification in aerial images using deep learning-based object detection. Agron. J. 2021 , 113 , 3991–4002. [ Google Scholar ] [ CrossRef ]
- Rong, J.; Zhou, H.; Zhang, F.; Yuan, T.; Wang, P. Tomato cluster detection and counting using improved YOLOv5 based on RGB-D fusion. Comput. Electron. Agric. 2023 , 207 , 107741. [ Google Scholar ] [ CrossRef ]
- Guo, Q.; Potter, K.M.; Ren, H.; Zhang, P. Impacts of Exotic Pests on Forest Ecosystems: An Update. Forests 2023 , 14 , 605. [ Google Scholar ] [ CrossRef ]
- Li, W.; Zheng, T.; Yang, Z.; Li, M.; Sun, C.; Yang, X. Classification and detection of insects from field images using deep learning for smart pest management: A systematic review. Ecol. Inform. 2021 , 66 , 101460. [ Google Scholar ] [ CrossRef ]
- Sun, Y.; Liu, X.; Yuan, M.; Ren, L.; Wang, J.; Chen, Z. Automatic in-trap pest detection using deep learning for pheromone-based Dendroctonus valens monitoring. Biosyst. Eng. 2018 , 176 , 140–150. [ Google Scholar ] [ CrossRef ]
- Partel, V.; Nunes, L.; Stansly, P.; Ampatzidis, Y. Automated vision-based system for monitoring Asian citrus psyllid in orchards utilizing artificial intelligence. Comput. Electron. Agric. 2019 , 162 , 328–336. [ Google Scholar ] [ CrossRef ]
- Mahanta, D.K.; Bhoi, T.K.; Komal, J.; Samal, I.; Mastinu, A. Spatial, spectral and temporal insights: Harnessing high-resolution satellite remote sensing and artificial intelligence for early monitoring of wood boring pests in forests. Plant Stress. 2024 , 11 , 100381. [ Google Scholar ] [ CrossRef ]
- Bhatnagar, S.; Mahanta, D.K.; Vyas, V.; Samal, I.; Komal, J.; Bhoi, T.K. Storage Pest Management with Nanopesticides Incorporating Silicon Nanoparticles: A Novel Approach for Sustainable Crop Preservation and Food Security. Silicon 2024 , 16 , 471–483. [ Google Scholar ] [ CrossRef ]
- Barchenkov, A.; Rubtsov, A.; Safronova, I.; Astapenko, S.; Tabakova, K.; Bogdanova, K.; Anuev, E.; Arzac, A. Features of Scots Pine Mortality Due to Incursion of Pine Bark Beetles in Symbiosis with Ophiostomatoid Fungi in the Forest-Steppe of Central Siberia. Forests 2023 , 14 , 1301. [ Google Scholar ] [ CrossRef ]
- Ballesteros, R.; Ortega, J.F.; Hernández, D.; Moreno, M.A. Applications of georeferenced high-resolution images obtained with unmanned aerial vehicles. Part II: Application to maize and onion crops of a semi-arid region in Spain. Precis. Agric. 2014 , 15 , 593–614. [ Google Scholar ] [ CrossRef ]
- Gopalakrishnan, R.; Subhash, C.; Kalpana, K. Predictive zoning of rice stem borer damage in southern India through spatial interpolation of weather-based models. J. Environ. Biol. 2014 , 35 , 923–928. [ Google Scholar ]
- Nurfaiz Abd Kharim, M.; Wayayok, A.; Fikri Abdullah, A.; Rashid Mohamed Shariff, A.; Mohd Husin, E.; Razif Mahadi, M. Predictive zoning of pest and disease infestations in rice field based on UAV aerial imagery. Egypt. J. Remote Sens. Space Sci. 2022 , 25 , 831–840. [ Google Scholar ] [ CrossRef ]
- Shi, Y.; Huang, W.; Luo, J.; Huang, L.; Zhou, X. Detection and discrimination of pests and diseases in winter wheat based on spectral indices and kernel discriminant analysis. Comput. Electron. Agric. 2017 , 141 , 171–180. [ Google Scholar ] [ CrossRef ]
- Yuan, L.; Zhang, H.; Zhang, Y.; Xing, C.; Bao, Z. Feasibility assessment of multi-spectral satellite sensors in monitoring and discriminating wheat diseases and insects. Optik 2017 , 131 , 598–608. [ Google Scholar ] [ CrossRef ]
- Ebrahimi, M.A.; Khoshtaghaza, M.H.; Minaei, S.; Jamshidi, B. Vision-based pest detection based on SVM classification method. Comput. Electron. Agric. 2017 , 137 , 52–58. [ Google Scholar ] [ CrossRef ]
- Kumar, D.; Kukreja, V. An Instance Segmentation Approach for Wheat Yellow Rust Disease Recognition. In Proceedings of the International Conference on Decision Aid Sciences and Application (DASA), Sakheer, Bahrain, 7–8 December 2021; pp. 926–931. [ Google Scholar ]
- Amarathunga, D.C.; Grundy, J.; Parry, H.; Dorin, A. Methods of insect image capture and classification: A Systematic literature review. Smart Agric. Technol. 2021 , 1 , 100023. [ Google Scholar ] [ CrossRef ]
- Tetila, E.C.; Machado, B.B.; Menezes, G.V.; Belete, N.A.d.S.; Astolfi, G.; Pistori, H. A Deep-Learning Approach for Automatic Counting of Soybean Insect Pests. IEEE Geosci. Remote Sens. Lett. 2020 , 17 , 1837–1841. [ Google Scholar ] [ CrossRef ]
- Abade, A.; Porto, L.F.; Ferreira, P.A.; de Barros Vidal, F. NemaNet: A convolutional neural network model for identification of soybean nematodes. Biosyst. Eng. 2022 , 213 , 39–62. [ Google Scholar ] [ CrossRef ]
- Kamilaris, A.; Prenafeta-Boldú, F.X. Deep learning in agriculture: A survey. Comput. Electron. Agric. 2018 , 147 , 70–90. [ Google Scholar ] [ CrossRef ]
- Li, R.; Wang, R.; Zhang, J.; Xie, C.; Liu, L.; Wang, F.; Chen, H.; Chen, T.; Hu, H.; Jia, X.; et al. An Effective Data Augmentation Strategy for CNN-Based Pest Localization and Recognition in the Field. IEEE Access 2019 , 7 , 160274–160283. [ Google Scholar ] [ CrossRef ]
- Vélez, S.; Ariza-Sentís, M.; Valente, J. Mapping the spatial variability of Botrytis bunch rot risk in vineyards using UAV multispectral imagery. Eur. J. Agron. 2023 , 142 , 126691. [ Google Scholar ] [ CrossRef ]
- Gomez Selvaraj, M.; Vergara, A.; Montenegro, F.; Alonso Ruiz, H.; Safari, N.; Raymaekers, D.; Ocimati, W.; Ntamwira, J.; Tits, L.; Omondi, A.B.; et al. Detection of banana plants and their major diseases through aerial images and machine learning methods: A case study in DR Congo and Republic of Benin. ISPRS J. Photogramm. Remote Sens. 2020 , 169 , 110–124. [ Google Scholar ] [ CrossRef ]
- Alshammari, H.H.; Alzahrani, A. Employing a hybrid lion-firefly algorithm for recognition and classification of olive leaf disease in Saudi Arabia. Alexandria. Eng. J. 2023 , 84 , 215–226. [ Google Scholar ] [ CrossRef ]
- Zhang, T.; Xu, Z.; Su, J.; Yang, Z.; Liu, C.; Chen, W.-H.; Li, J. Ir-UNet: Irregular Segmentation U-Shape Network for Wheat Yellow Rust Detection by UAV Multispectral Imagery. Remote Sens. 2021 , 13 , 3892. [ Google Scholar ] [ CrossRef ]
- Jin, X.; Jie, L.; Wang, S.; Qi, H.J.; Li, S.W. Classifying Wheat Hyperspectral Pixels of Healthy Heads and Fusarium Head Blight Disease Using a Deep Neural Network in the Wild Field. Remote Sens. 2018 , 10 , 395. [ Google Scholar ] [ CrossRef ]
- Zhang, Y.; Lv, C. TinySegformer: A lightweight visual segmentation model for real-time agricultural pest detection. Comput. Electron. Agric. 2024 , 218 , 108740. [ Google Scholar ] [ CrossRef ]
- Lu, S.; Ye, S.-j. Using an image segmentation and support vector machine method for identifying two locust species and instars. J. Integr. Agric. 2020 , 19 , 1301–1313. [ Google Scholar ] [ CrossRef ]
- Barbedo, J.G.A.; Tibola, C.S.; Fernandes, J.M.C. Detecting Fusarium head blight in wheat kernels using hyperspectral imaging. Biosyst. Eng. 2015 , 131 , 65–76. [ Google Scholar ] [ CrossRef ]
- Mumtaz, R.; Maqsood, M.H.; Haq Iu Shafi, U.; Mahmood, Z.; Mumtaz, M. Integrated digital image processing techniques and deep learning approaches for wheat stripe rust disease detection and grading. Decis. Anal. J. 2023 , 8 , 100305. [ Google Scholar ] [ CrossRef ]
- Bao, W.; Zhu, Z.; Hu, G.; Zhou, X.; Zhang, D.; Yang, X. UAV remote sensing detection of tea leaf blight based on DDMA-YOLO. Comput. Electron. Agric. 2023 , 205 , 107637. [ Google Scholar ] [ CrossRef ]
- Li, D.; Song, Z.; Quan, C.; Xu, X.; Liu, C. Recent advances in image fusion technology in agriculture. Comput. Electron. Agric. 2021 , 191 , 106491. [ Google Scholar ] [ CrossRef ]
- Ali, M.A.; Sharma, A.K.; Dhanaraj, R.K. Heterogeneous features and deep learning networks fusion-based pest detection, prevention and controlling system using IoT and pest sound analytics in a vast agriculture system. Comput. Electr. Eng. 2024 , 116 , 109146. [ Google Scholar ] [ CrossRef ]
- Lin, Q.; Huang, H.; Wang, J.; Chen, L.; Du, H.; Zhou, G. Early detection of pine shoot beetle attack using vertical profile of plant traits through UAV-based hyperspectral, thermal, and lidar data fusion. Int. J. Appl. Earth Obs. Geoinf. 2023 , 125 , 103549. [ Google Scholar ] [ CrossRef ]
- Dalagnol, R.; Phillips, O.L.; Gloor, E.; Galvão, L.S.; Wagner, F.H.; Locks, C.J.; Aragão, L.E.O.C. Quantifying Canopy Tree Loss and Gap Recovery in Tropical Forests under Low-Intensity Logging Using VHR Satellite Imagery and Airborne LiDAR. Remote Sens. 2019 , 11 , 817. [ Google Scholar ] [ CrossRef ]
- Pantazi, X.E.; Moshou, D.; Bochtis, D. Chapter 5-Tutorial II: Disease detection with fusion techniques. In Intelligent Data Mining and Fusion Systems in Agriculture ; Pantazi, X.E., Moshou, D., Bochtis, D., Eds.; Academic Press: Cambridge, MA, USA, 2020; pp. 199–221. [ Google Scholar ]
- Kaya, Y.; Gürsoy, E. A novel multi-head CNN design to identify plant diseases using the fusion of RGB images. Ecol. Inform. 2023 , 75 , 101998. [ Google Scholar ] [ CrossRef ]
- Ma, R.; Zhang, N.; Zhang, X.; Bai, T.; Yuan, X.; Bao, H.; He, D.; Sun, W.; He, Y. Cotton Verticillium wilt monitoring based on UAV multispectral-visible multi-source feature fusion. Comput. Electron. Agric. 2024 , 217 , 108628. [ Google Scholar ] [ CrossRef ]
- De Cesaro Júnior, T.; Rieder, R.; Di Domênico, J.R.; Lau, D. InsectCV: A system for insect detection in the lab from trap images. Ecol. Inform. 2022 , 67 , 101516. [ Google Scholar ] [ CrossRef ]
- Ishengoma, F.S.; Rai, I.A.; Ngoga, S.R. Hybrid convolution neural network model for a quicker detection of infested maize plants with fall armyworms using UAV-based images. Ecol. Inform. 2022 , 67 , 101502. [ Google Scholar ] [ CrossRef ]
- Waheed, A.; Goyal, M.; Gupta, D.; Khanna, A.; Hassanien, A.E.; Pandey, H.M. An optimized dense convolutional neural network model for disease recognition and classification in corn leaf. Comput. Electron. Agric. 2020 , 175 , 105456. [ Google Scholar ] [ CrossRef ]
- Sunil, C.K.; Jaidhar, C.D.; Patil, N. Tomato plant disease classification using Multilevel Feature Fusion with adaptive channel spatial and pixel attention mechanism. Expert Syst. Appl. 2023 , 228 , 120381. [ Google Scholar ] [ CrossRef ]
- Dong, S.; Teng, Y.; Jiao, L.; Du, J.; Liu, K.; Wang, R. ESA-Net: An efficient scale-aware network for small crop pest detection. Expert Syst. Appl. 2024 , 236 , 121308. [ Google Scholar ] [ CrossRef ]
- Amarathunga, D.C.; Ratnayake, M.N.; Grundy, J.; Dorin, A. Fine-grained image classification of microscopic insect pest species: Western Flower thrips and Plague thrips. Comput. Electron. Agric. 2022 , 203 , 107462. [ Google Scholar ] [ CrossRef ]
- Ye, W.; Lao, J.; Liu, Y.; Chang, C.-C.; Zhang, Z.; Li, H.; Zhou, H. Pine pest detection using remote sensing satellite images combined with a multi-scale attention-UNet model. Ecol. Inform. 2022 , 72 , 101906. [ Google Scholar ] [ CrossRef ]
- Kaliraj, S.; Adhikari, K.; Dharumarajan, S.; Lalitha, M.; Kumar, N. Chapter 3-Remote sensing and geographic information system applications. In Mapping and Assessment of Soil Resources ; Dharumarajan, S., Kaliraj, S., Adhikari, K., Lalitha, M., Kumar, N., Eds.; Remote Sensing of Soils Elsevier: Amsterdam, The Netherlands, 2024; pp. 25–41. [ Google Scholar ]
- Yang, H.; Zhang, X.; Xu, M.; Shao, S.; Wang, X.; Liu, W.; Wu, D.; Ma, Y.; Bao, Y.; Zhang, X.; et al. Hyper-temporal remote sensing data in bare soil period and terrain attributes for digital soil mapping in the Black soil regions of China. Catena 2020 , 184 , 104259. [ Google Scholar ] [ CrossRef ]
- Das, B.; Rathore, P.; Roy, D.; Chakraborty, D.; Bhattacharya, B.K.; Mandal, D.; Jatav, R.; Sethi, D.; Mukherjee, J.; Sehgal, V.K.; et al. Ensemble surface soil moisture estimates at farm-scale combining satellite-based optical-thermal-microwave remote sensing observations. Agric. For. Meteorol. 2023 , 339 , 109567. [ Google Scholar ] [ CrossRef ]
- Dash, P.K. Chapter 22—Remote sensing as a potential tool for advancing digital soil mapping. In Remote Sensing of Soils ; Dharumarajan, S., Kaliraj, S., Adhikari, K., Lalitha, M., Kumar, N., Eds.; Elsevier: Amsterdam, The Netherlands, 2024; pp. 357–370. [ Google Scholar ]
- Das, S.; Ghimire, D. Chapter 25—Soil organic carbon: Measurement and monitoring using remote sensing data. In Remote Sensing of Soils ; Dharumarajan, S., Kaliraj, S., Adhikari, K., Lalitha, M., Kumar, N., Eds.; Elsevier: Amsterdam, The Netherlands, 2024; pp. 395–409. [ Google Scholar ]
- Hareesh, S.B. Chapter 7—The latest applications of remote sensing technologies for soil management in precision agriculture practices. In Remote Sensing in Precision Agriculture ; Lamine, S., Srivastava, P.K., Kayad, A., Muñoz-Arriola, F., Pandey, P.C., Eds.; Academic Press: Cambridge, MA, USA, 2024; pp. 105–135. [ Google Scholar ]
- Peña-Arancibia, J.L.; Mainuddin, M.; Kirby, J.M.; Chiew, F.H.S.; McVicar, T.R.; Vaze, J. Assessing irrigated agriculture’s surface water and groundwater consumption by combining satellite remote sensing and hydrologic modelling. Sci. Total Environ. 2016 , 542 , 372–382. [ Google Scholar ] [ CrossRef ] [ PubMed ]
- Li, Q.; Hao, H.; Zhao, Y.; Geng, Q.; Liu, G.; Zhang, Y.; Yu, F. GANs-LSTM Model for Soil Temperature Estimation From Meteorological: A New Approach. IEEE Access 2020 , 8 , 59427–59443. [ Google Scholar ] [ CrossRef ]
- Li, Q.; Li, Z.; Shangguan, W.; Wang, X.; Li, L.; Yu, F. Improving soil moisture prediction using a novel encoder-decoder model with residual learning. Comput. Electron. Agric. 2022 , 195 , 106816. [ Google Scholar ] [ CrossRef ]
- Mohanty, B.P.; Cosh, M.H.; Lakshmi, V.; Montzka, C. Soil Moisture Remote Sensing: State-of-the-Science. Vadose Zone J. 2017 , 16 , 1–9. [ Google Scholar ] [ CrossRef ]
- Maynard, J.J.; Levi, M.R. Hyper-temporal remote sensing for digital soil mapping: Characterizing soil-vegetation response to climatic variability. Geoderma 2017 , 285 , 94–109. [ Google Scholar ] [ CrossRef ]
- Duan, M.; Song, X.; Li, Z.; Zhang, X.; Ding, X.; Cui, D. Identifying soil groups and selecting a high-accuracy classification method based on multi-textural features with optimal window sizes using remote sensing images. Ecol. Inform. 2024 , 81 , 102563. [ Google Scholar ] [ CrossRef ]
- Zhou, Q.B.; Yu, Q.Y.; Liu, J.; Wu, W.B.; Tang, H.J. Perspective of Chinese GF-1 high-resolution satellite data in agricultural remote sensing monitoring. J. Integr. Agric. 2017 , 16 , 242–251. [ Google Scholar ] [ CrossRef ]
- Musasa, T.; Dube, T.; Marambanyika, T. Landsat satellite programme potential for soil erosion assessment and monitoring in arid environments: A review of applications and challenges. Int. Soil Water Conserv. Res. 2023 , 12 , 267–278. [ Google Scholar ] [ CrossRef ]
- Wang, J.; Zhang, Y.; Song, P.; Tian, J. Estimating sub-daily resolution soil moisture using Fengyun satellite data and machine learning. J. Hydrol. 2024 , 632 , 130814. [ Google Scholar ] [ CrossRef ]
- Kolassa, J.; Reichle, R.H.; Liu, Q.; Alemohammad, S.H.; Gentine, P.; Aida, K.; Asanuma, J.; Bircher, S.; Caldwell, T.; Colliander, A.; et al. Estimating surface soil moisture from SMAP observations using a Neural Network technique. Remote Sens. Environ. 2018 , 204 , 43–59. [ Google Scholar ] [ CrossRef ]
- Wang La Zhou, X.; Zhu, X.; Dong, Z.; Guo, W. Estimation of biomass in wheat using random forest regression algorithm and remote sensing data. Crop J. 2016 , 4 , 212–219. [ Google Scholar ] [ CrossRef ]
- Yang, H.; Xiong, L.; Liu, D.; Cheng, L.; Chen, J. High spatial resolution simulation of profile soil moisture by assimilating multi-source remote-sensed information into a distributed hydrological model. J. Hydrol. 2021 , 597 , 126311. [ Google Scholar ] [ CrossRef ]
- Mammadov, E.; Nowosad, J.; Glaesser, C. Estimation and mapping of surface soil properties in the Caucasus Mountains, Azerbaijan using high-resolution remote sensing data. Geoderma Reg. 2021 , 26 , e00411. [ Google Scholar ] [ CrossRef ]
- Straffelini, E.; Pijl, A.; Otto, S.; Marchesini, E.; Pitacco, A.; Tarolli, P. A high-resolution physical modelling approach to assess runoff and soil erosion in vineyards under different soil managements. Soil Tillage Res. 2022 , 222 , 105418. [ Google Scholar ] [ CrossRef ]
- Koley, S.; Jeganathan, C. Estimation and evaluation of high spatial resolution surface soil moisture using multi-sensor multi-resolution approach. Geoderma 2020 , 378 , 114618. [ Google Scholar ] [ CrossRef ]
- Bertalan, L.; Holb, I.; Pataki, A.; Négyesi, G.; Szabó, G.; Kupásné Szalóki, A.; Szabo, S. UAV-based multispectral and thermal cameras to predict soil water content–A machine learning approach. Comput. Electron. Agric. 2022 , 200 , 107262. [ Google Scholar ] [ CrossRef ]
- Menzies Pluer, E.G.; Robinson, D.T.; Meinen, B.U.; Macrae, M.L. Pairing soil sampling with very-high resolution UAV imagery: An examination of drivers of soil and nutrient movement and agricultural productivity in southern Ontario. Geoderma 2020 , 379 , 114630. [ Google Scholar ] [ CrossRef ]
- Cheng, M.; Jiao, X.; Liu, Y.; Shao, M.; Yu, X.; Bai, Y.; Wang, Z.; Wang, S.; Tuohuti, N.; Liu, S.; et al. Estimation of soil moisture content under high maize canopy coverage from UAV multimodal data and machine learning. Agric. Water Manag. 2022 , 264 , 107530. [ Google Scholar ] [ CrossRef ]
- Huuskonen, J.; Oksanen, T. Soil sampling with drones and augmented reality in precision agriculture. Comput. Electron. Agric. 2018 , 154 , 25–35. [ Google Scholar ] [ CrossRef ]
- Shokati, H.; Mashal, M.; Noroozi, A.; Mirzaei, S.; Mohammadi-Doqozloo, Z. Assessing soil moisture levels using visible UAV imagery and machine learning models. Remote Sens. Appl. Soc. Environ. 2023 , 32 , 101076. [ Google Scholar ] [ CrossRef ]
- Wang, Z.; Zhang, X.; Zhang, F.; Chan, N.W.; Kung, H.-t.; Liu, S.; Deng, L. Estimation of soil salt content using machine learning techniques based on remote-sensing fractional derivatives, a case study in the Ebinur Lake Wetland National Nature Reserve, Northwest China. Ecol. Indic. 2020 , 119 , 106869. [ Google Scholar ] [ CrossRef ]
- Ma, S.; He, B.; Ge, X.; Luo, X. Spatial prediction of soil salinity based on the Google Earth Engine platform with multitemporal synthetic remote sensing images. Ecol. Inform. 2023 , 75 , 102111. [ Google Scholar ] [ CrossRef ]
- Du, R.; Chen, J.; Xiang, Y.; Xiang, R.; Yang, X.; Wang, T.; He, Y.; Wu, Y.; Yin, H.; Zhang, Z.; et al. Timely monitoring of soil water-salt dynamics within cropland by hybrid spectral unmixing and machine learning models. Int. Soil Water Conserv. Res. 2023 , 12 , 726–740. [ Google Scholar ] [ CrossRef ]
- Golestani, M.; Mosleh Ghahfarokhi, Z.; Esfandiarpour-Boroujeni, I.; Shirani, H. Evaluating the spatiotemporal variations of soil salinity in Sirjan Playa, Iran using Sentinel-2A and Landsat-8 OLI imagery. Catena 2023 , 231 , 107375. [ Google Scholar ] [ CrossRef ]
- Sothe, C.; Gonsamo, A.; Arabian, J.; Snider, J. Large scale mapping of soil organic carbon concentration with 3D machine learning and satellite observations. Geoderma 2022 , 405 , 115402. [ Google Scholar ] [ CrossRef ]
- Rahman, A.; Abdullah, H.M.; Tanzir, M.T.; Hossain, M.J.; Khan, B.M.; Miah, M.G.; Islam, I. Performance of different machine learning algorithms on satellite image classification in rural and urban setup. Remote Sens. Appl. Soc. Environ. 2020 , 20 , 100410. [ Google Scholar ] [ CrossRef ]
- Huang, H.; Wang, J.; Liu, C.; Liang, L.; Li, C.; Gong, P. The migration of training samples towards dynamic global land cover mapping. ISPRS J. Photogramm. Remote Sens. 2020 , 161 , 27–36. [ Google Scholar ] [ CrossRef ]
- Zafar, Z.; Zubair, M.; Zha, Y.; Fahd, S.; Ahmad Nadeem, A. Performance assessment of machine learning algorithms for mapping of land use/land cover using remote sensing data. Egypt. J. Remote Sens. Space Sci. 2024 , 27 , 216–226. [ Google Scholar ] [ CrossRef ]
- Elhadi, M.I.A.; Mutanga, O.; Odindi, J.; Abdel-Rahman, E.M. Land-use/cover classification in a heterogeneous coastal landscape using RapidEye imagery: Evaluating the performance of random forest and support vector machines classifiers. Int. J. Remote Sens. 2014 , 35 , 3440–3458. [ Google Scholar ]
- Gómez, C.; White, J.C.; Wulder, M.A. Optical remotely sensed time series data for land cover classification: A review. ISPRS J. Photogramm. Remote Sens. 2016 , 116 , 55–72. [ Google Scholar ] [ CrossRef ]
- Matlhodi, B.; Kenabatho, P.K.; Parida, B.P.; Maphanyane, J.G. Evaluating Land Use and Land Cover Change in the Gaborone Dam Catchment, Botswana, from 1984–2015 Using GIS and Remote Sensing. Sustainability 2019 , 11 , 5174. [ Google Scholar ] [ CrossRef ]
- Liu, J.; Yang, K.; Tariq, A.; Lu, L.; Soufan, W.; El Sabagh, A. Interaction of climate, topography and soil properties with cropland and cropping pattern using remote sensing data and machine learning methods. Egypt. J. Remote Sens. Space Sci. 2023 , 26 , 415–426. [ Google Scholar ] [ CrossRef ]
- Yuh, Y.G.; Tracz, W.; Matthews, H.D.; Turner, S.E. Application of machine learning approaches for land cover monitoring in northern Cameroon. Ecol. Inform. 2023 , 74 , 101955. [ Google Scholar ] [ CrossRef ]
- Khatami, R.; Mountrakis, G.; Stehman, S.V. A meta-analysis of remote sensing research on supervised pixel-based land-cover image classification processes: General guidelines for practitioners and future research. Remote Sens. Environ. 2016 , 177 , 89–100. [ Google Scholar ] [ CrossRef ]
- Nitze, I.; Barrett, B.; Cawkwell, F. Temporal optimisation of image acquisition for land cover classification with Random Forest and MODIS time-series. Int. J. Appl. Earth Obs. Geoinf. 2015 , 34 , 136–146. [ Google Scholar ] [ CrossRef ]
- Zhang, S.; Liu, L.Y. The potential of the MERIS Terrestrial Chlorophyll Index for crop yield prediction. Remote Sens. Lett. 2014 , 5 , 733–742. [ Google Scholar ] [ CrossRef ]
- Teodoro, A. Applicability of data mining algorithms in the identification of beach features/patterns on high-resolution satellite data. J. Appl. Remote Sens. 2015 , 9 , 095095. [ Google Scholar ] [ CrossRef ]
- Sinha, S.; Sharma, L.K.; Nathawat, M.S. Improved Land-use/Land-cover classification of semi-arid deciduous forest landscape using thermal remote sensing. Egypt. J. Remote Sens. Space Sci. 2015 , 18 , 217–233. [ Google Scholar ] [ CrossRef ]
- Mei, A.; Manzo, C.; Fontinovo, G.; Bassani, C.; Allegrini, A.; Petracchini, F. Assessment of land cover changes in Lampedusa Island (Italy) using Landsat TM and OLI data. J. Afr. Earth Sci. 2016 , 122 , 15–24. [ Google Scholar ] [ CrossRef ]
- Silva, L.P.E.; Xavier, A.P.C.; da Silva, R.M.; Santos, C.A.G. Modeling land cover change based on an artificial neural network for a semiarid river basin in northeastern Brazil. Glob. Ecol. Conserv. 2020 , 21 , e00811. [ Google Scholar ] [ CrossRef ]
- Zhang, H.K.; Roy, D.P.; Luo, D. Demonstration of large area land cover classification with a one dimensional convolutional neural network applied to single pixel temporal metric percentiles. Remote Sens. Environ. 2023 , 295 , 113653. [ Google Scholar ] [ CrossRef ]
- Zhang, C.; Yue, P.; Tapete, D.; Shangguan, B.; Wang, M.; Wu, Z. A multi-level context-guided classification method with object-based convolutional neural network for land cover classification using very high resolution remote sensing images. Int. J. Appl. Earth Obs. Geoinf. 2020 , 88 , 102086. [ Google Scholar ] [ CrossRef ]
- Loukika, K.N.; Keesara, V.R.; Sridhar, V. Analysis of Land Use and Land Cover Using Machine Learning Algorithms on Google Earth Engine for Munneru River Basin, India. Sustainability 2021 , 13 , 13758. [ Google Scholar ] [ CrossRef ]
- Prasad, P.; Loveson, V.J.; Chandra, P.; Kotha, M. Evaluation and comparison of the earth observing sensors in land cover/land use studies using machine learning algorithms. Ecol. Inform. 2022 , 68 , 101522. [ Google Scholar ] [ CrossRef ]
- Zhou, X.; Zheng, H.B.; Xu, X.Q.; He, J.Y.; Ge, X.K.; Yao, X.; Cheng, T.; Zhu, Y.; Cao, W.X.; Tian, Y.C. Predicting grain yield in rice using multi-temporal vegetation indices from UAV-based multispectral and digital imagery. ISPRS J. Photogramm. Remote Sens. 2017 , 130 , 246–255. [ Google Scholar ] [ CrossRef ]
- Wang, L.; Tian, Y.; Yao, X.; Zhu, Y.; Cao, W. Predicting grain yield and protein content in wheat by fusing multi-sensor and multi-temporal remote-sensing images. Field Crops Res. 2014 , 164 , 178–188. [ Google Scholar ] [ CrossRef ]
- Furukawa, F.; Maruyama, K.; Saito, Y.K.; Kaneko, M. Corn Height Estimation Using UAV for Yield Prediction and Crop Monitoring. In Unmanned Aerial Vehicle: Applications in Agriculture and Environment ; Avtar, R., Watanabe, T., Eds.; Springer International Publishing: Cham, Switzerland, 2020; pp. 51–69. [ Google Scholar ]
- Johnson, D.M. An assessment of pre- and within-season remotely sensed variables for forecasting corn and soybean yields in the United States. Remote Sens. Environ. 2014 , 141 , 116–128. [ Google Scholar ] [ CrossRef ]
- Shao, M.; Nie, C.; Zhang, A.; Shi, L.; Zha, Y.; Xu, H.; Yang, H.; Yu, X.; Bai, Y.; Liu, S.; et al. Quantifying effect of maize tassels on LAI estimation based on multispectral imagery and machine learning methods. Comput. Electron. Agric. 2023 , 211 , 108029. [ Google Scholar ] [ CrossRef ]
- Yang, C.; Lee, W.S.; Gader, P. Hyperspectral band selection for detecting different blueberry fruit maturity stages. Comput. Electron. Agric. 2014 , 109 , 23–31. [ Google Scholar ] [ CrossRef ]
- Peña, M.A.; Brenning, A. Assessing fruit-tree crop classification from Landsat-8 time series for the Maipo Valley, Chile. Remote Sens. Environ. 2015 , 171 , 234–244. [ Google Scholar ] [ CrossRef ]
- Liang, L.; Di, L.; Zhang, L.; Deng, M.; Qin, Z.; Zhao, S.; Lin, H. Estimation of crop LAI using hyperspectral vegetation indices and a hybrid inversion method. Remote Sens. Environ. 2015 , 165 , 123–134. [ Google Scholar ] [ CrossRef ]
- Yang, Z.; Shao, Y.; Li, K.; Liu, Q.; Liu, L.; Brisco, B. An improved scheme for rice phenology estimation based on time-series multispectral HJ-1A/B and polarimetric RADARSAT-2 data. Remote Sens. Environ. 2017 , 195 , 184–201. [ Google Scholar ] [ CrossRef ]
- Azadbakht, M.; Ashourloo, D.; Aghighi, H.; Homayouni, S.; Shahrabi, H.S.; Matkan, A.; Radiom, S. Alfalfa yield estimation based on time series of Landsat 8 and PROBA-V images: An investigation of machine learning techniques and spectral-temporal features. Remote Sens. Appl. Soc. Environ. 2022 , 25 , 100657. [ Google Scholar ] [ CrossRef ]
- Görgens, E.B.; Montaghi, A.; Rodriguez, L.C.E. A performance comparison of machine learning methods to estimate the fast-growing forest plantation yield based on laser scanning metrics. Comput. Electron. Agric. 2015 , 116 , 221–227. [ Google Scholar ] [ CrossRef ]
- Guo, Z.; Chamberlin, J.; You, L. Smallholder maize yield estimation using satellite data and machine learning in Ethiopia. Crop Environ. 2023 , 2 , 165–174. [ Google Scholar ] [ CrossRef ]
- Van Ewijk, K.Y.; Randin, C.F.; Treitz, P.M.; Scott, N.A. Predicting fine-scale tree species abundance patterns using biotic variables derived from LiDAR and high spatial resolution imagery. Remote Sens. Environ. 2014 , 150 , 120–131. [ Google Scholar ] [ CrossRef ]
- Khanal, S.; Klopfenstein, A.; Kc, K.; Ramarao, V.; Fulton, J.; Douridas, N.; Shearer, S.A. Assessing the impact of agricultural field traffic on corn grain yield using remote sensing and machine learning. Soil Tillage Res. 2021 , 208 , 104880. [ Google Scholar ] [ CrossRef ]
- Habibi, L.N.; Matsui, T.; Tanaka, T.S.T. Critical evaluation of the effects of a cross-validation strategy and machine learning optimization on the prediction accuracy and transferability of a soybean yield prediction model using UAV-based remote sensing. J. Agric. Food Res. 2024 , 16 , 101096. [ Google Scholar ] [ CrossRef ]
- Zhang, S.; Qi, X.; Gao, M.; Dai, C.; Yin, G.; Ma, D.; Feng, W.; Guo, T.; He, L. Estimation of wheat protein content and wet gluten content based on fusion of hyperspectral and RGB sensors using machine learning algorithms. Food Chem. 2024 , 448 , 139103. [ Google Scholar ] [ CrossRef ]
- Guo, Y.; Xiao, Y.; Hao, F.; Zhang, X.; Chen, J.; de Beurs, K.; He, Y.; Fu, Y.H. Comparison of different machine learning algorithms for predicting maize grain yield using UAV-based hyperspectral images. Int. J. Appl. Earth Obs. Geoinf. 2023 , 124 , 103528. [ Google Scholar ] [ CrossRef ]
- Qu, H.; Zheng, C.; Ji, H.; Barai, K.; Zhang, Y.-J. A fast and efficient approach to estimate wild blueberry yield using machine learning with drone photography: Flight altitude, sampling method and model effects. Comput. Electron. Agric. 2024 , 216 , 108543. [ Google Scholar ] [ CrossRef ]
- Yu, N.; Li, L.; Schmitz, N.; Tian, L.F.; Greenberg, J.A.; Diers, B.W. Development of methods to improve soybean yield estimation and predict plant maturity with an unmanned aerial vehicle based platform. Remote Sens. Environ. 2016 , 187 , 91–101. [ Google Scholar ] [ CrossRef ]
- Maimaitijiang, M.; Ghulam, A.; Sidike, P.; Hartling, S.; Maimaitiyiming, M.; Peterson, K.; Shavers, E.; Fishman, J.; Peterson, J.; Kadam, S.; et al. Unmanned Aerial System (UAS)-based phenotyping of soybean using multi-sensor data fusion and extreme learning machine. ISPRS J. Photogramm. Remote Sens. 2017 , 134 , 43–58. [ Google Scholar ] [ CrossRef ]
- Xu, W.; Chen, P.; Zhan, Y.; Chen, S.; Zhang, L.; Lan, Y. Cotton yield estimation model based on machine learning using time series UAV remote sensing data. Int. J. Appl. Earth Obs. Geoinf. 2021 , 104 , 102511. [ Google Scholar ] [ CrossRef ]
- Liu, S.; Jin, X.; Bai, Y.; Wu, W.; Cui, N.; Cheng, M.; Liu, Y.; Meng, L.; Jia, X.; Nie, C.; et al. UAV multispectral images for accurate estimation of the maize LAI considering the effect of soil background. Int. J. Appl. Earth Obs. Geoinf. 2023 , 121 , 103383. [ Google Scholar ] [ CrossRef ]
- Kern, A.; Barcza, Z.; Marjanović, H.; Árendás, T.; Fodor, N.; Bónis, P.; Bognár, P.; Lichtenberger, J. Statistical modelling of crop yield in Central Europe using climate data and remote sensing vegetation indices. Agric. For. Meteorol. 2018 , 260 , 300–320. [ Google Scholar ] [ CrossRef ]
- Bai, H.; Xiao, D.; Tang, J.; Liu, D.L. Evaluation of wheat yield in North China Plain under extreme climate by coupling crop model with machine learning. Comput. Electron. Agric. 2024 , 217 , 108651. [ Google Scholar ] [ CrossRef ]
- Khanal, S.; Fulton, J.; Klopfenstein, A.; Douridas, N.; Shearer, S. Integration of high resolution remotely sensed data and machine learning techniques for spatial prediction of soil properties and corn yield. Comput. Electron. Agric. 2018 , 153 , 213–225. [ Google Scholar ] [ CrossRef ]
- Jagdeep, S.; Gobinder, S.; Gupta, N. Balancing phosphorus fertilization for sustainable maize yield and soil test phosphorus management: A long-term study using machine learning. Field Crops Res. 2023 , 304 , 109169. [ Google Scholar ] [ CrossRef ]
- Fry, J.; Guber, A.K.; Ladoni, M.; Munoz, J.D.; Kravchenko, A.N. The effect of up-scaling soil properties and model parameters on predictive accuracy of DSSAT crop simulation model under variable weather conditions. Geoderma 2017 , 287 , 105–115. [ Google Scholar ] [ CrossRef ]
- Zain, M.; Si, Z.; Li, S.; Gao, Y.; Mehmood, F.; Rahman, S.-U.; Mounkaila Hamani, A.K.; Duan, A. The Coupled Effects of Irrigation Scheduling and Nitrogen Fertilization Mode on Growth, Yield and Water Use Efficiency in Drip-Irrigated Winter Wheat. Sustainability 2021 , 13 , 2742. [ Google Scholar ] [ CrossRef ]
- Wang, Y.; Shi, W.; Wen, T. Prediction of winter wheat yield and dry matter in North China Plain using machine learning algorithms for optimal water and nitrogen application. Agric. Water Manag. 2023 , 277 , 108140. [ Google Scholar ] [ CrossRef ]
- Kaur Dhaliwal, J.; Panday, D.; Saha, D.; Lee, J.; Jagadamma, S.; Schaeffer, S.; Mengistu, A. Predicting and interpreting cotton yield and its determinants under long-term conservation management practices using machine learning. Comput. Electron. Agric. 2022 , 199 , 107107. [ Google Scholar ] [ CrossRef ]
- Elavarasan, D.; Vincent, D.R.; Sharma, V.; Zomaya, A.Y.; Srinivasan, K. Forecasting yield by integrating agrarian factors and machine learning models: A survey. Comput. Electron. Agric. 2018 , 155 , 257–282. [ Google Scholar ] [ CrossRef ]
- Singh, B.; Jana, A.K. Forecast of agri-residues generation from rice, wheat and oilseed crops in India using machine learning techniques: Exploring strategies for sustainable smart management. Environ. Res. 2024 , 245 , 117993. [ Google Scholar ] [ CrossRef ]
- Zhou, H.K.; Yang, J.H.; Lou, W.D.; Sheng, L.; Li, D.; Hu, H. Improving grain yield prediction through fusion of multi-temporal spectral features and agronomic trait parameters derived from UAV imagery. Front. Plant Sci. 2023 , 14 , 1217448. [ Google Scholar ] [ CrossRef ]
- Habyarimana, E.; Piccard, I.; Catellani, M.; De Franceschi, P.; Dall’Agata, M. Towards Predictive Modeling of Sorghum Biomass Yields Using Fraction of Absorbed Photosynthetically Active Radiation Derived from Sentinel-2 Satellite Imagery and Supervised Machine Learning Techniques. Agronomy 2019 , 9 , 203. [ Google Scholar ] [ CrossRef ]
- Kowalik, W.; Dabrowska-Zielinska, K.; Meroni, M.; Raczka, T.U.; de Wit, A. Yield estimation using SPOT-VEGETATION products: A case study of wheat in European countries. Int. J. Appl. Earth Obs. Geoinf. 2014 , 32 , 228–239. [ Google Scholar ] [ CrossRef ]
- Castaldi, F.; Casa, R.; Pelosi, F.; Yang, H. Influence of acquisition time and resolution on wheat yield estimation at the field scale from canopy biophysical variables retrieved from SPOT satellite data. Int. J. Remote Sens. 2015 , 36 , 2438–2459. [ Google Scholar ] [ CrossRef ]
- Naghdyzadegan Jahromi, M.; Zand-Parsa, S.; Razzaghi, F.; Jamshidi, S.; Didari, S.; Doosthosseini, A.; Pourghasemi, H.R. Developing machine learning models for wheat yield prediction using ground-based data, satellite-based actual evapotranspiration and vegetation indices. Eur. J. Agron. 2023 , 146 , 126820. [ Google Scholar ] [ CrossRef ]
- Jurečka, F.; Fischer, M.; Hlavinka, P.; Balek, J.; Semerádová, D.; Bláhová, M.; Anderson, M.C.; Hain, C.; Žalud, Z.; Trnka, M. Potential of water balance and remote sensing-based evapotranspiration models to predict yields of spring barley and winter wheat in the Czech Republic. Agric. Water Manag. 2021 , 256 , 107064. [ Google Scholar ] [ CrossRef ]
- Yang, C.; Lei, H. Evaluation of data assimilation strategies on improving the performance of crop modeling based on a novel evapotranspiration assimilation framework. Agric. For. Meteorol. 2024 , 346 , 109882. [ Google Scholar ] [ CrossRef ]
- Gilardelli, C.; Stella, T.; Confalonieri, R.; Ranghetti, L.; Campos-Taberner, M.; García-Haro, F.J.; Boschetti, M. Downscaling rice yield simulation at sub-field scale using remotely sensed LAI data. Eur. J. Agron. 2019 , 103 , 108–116. [ Google Scholar ] [ CrossRef ]
- Gaso, D.V.; de Wit, A.; Berger, A.G.; Kooistra, L. Predicting within-field soybean yield variability by coupling Sentinel-2 leaf area index with a crop growth model. Agric. For. Meteorol. 2021 , 308 , 108553. [ Google Scholar ] [ CrossRef ]
- Liu, C.; Liu, Y.; Lu, Y.H.; Liao, Y.L.; Nie, J.; Yuan, X.L.; Chen, F. Use of a leaf chlorophyll content index to improve the prediction of above-ground biomass and productivity. PeerJ 2019 , 6 . [ Google Scholar ] [ CrossRef ] [ PubMed ]
- Singh, V.; Kunal Singh, M.; Singh, B. Spectral indices measured with proximal sensing using canopy reflectance sensor, chlorophyll meter and leaf color chart for in-season grain yield prediction of basmati rice. Pedosphere 2022 , 32 , 812–822. [ Google Scholar ] [ CrossRef ]
- Zhang, J.; Feng, L.; Yao, F. Improved maize cultivated area estimation over a large scale combining MODIS–EVI time series data and crop phenological information. ISPRS J. Photogramm. Remote Sens. 2014 , 94 , 102–113. [ Google Scholar ] [ CrossRef ]
- De la Casa, A.; Ovando, G.; Bressanini, L.; Martínez, J.; Díaz, G.; Miranda, C. Soybean crop coverage estimation from NDVI images with different spatial resolution to evaluate yield variability in a plot. ISPRS J. Photogramm. Remote Sens. 2018 , 146 , 531–547. [ Google Scholar ] [ CrossRef ]
- Kitano, B.T.; Mendes, C.C.T.; Geus, A.R.; Oliveira, H.C.; Souza, J.R. Corn Plant Counting Using Deep Learning and UAV Images. IEEE Geosci. Remote Sens. Lett. 2019 , 1–5. [ Google Scholar ] [ CrossRef ]
- Jhajharia, K.; Mathur, P. Prediction of crop yield using satellite vegetation indices combined with machine learning approaches. Adv. Space Res. 2023 , 72 , 3998–4007. [ Google Scholar ] [ CrossRef ]
- Shammi, S.A.; Meng, Q. Use time series NDVI and EVI to develop dynamic crop growth metrics for yield modeling. Ecol. Indic. 2021 , 121 , 107124. [ Google Scholar ] [ CrossRef ]
- Zhao, Y.; Vergopolan, N.; Baylis, K.; Blekking, J.; Caylor, K.; Evans, T.; Giroux, S.; Sheffield, J.; Estes, L. Comparing empirical and survey-based yield forecasts in a dryland agro-ecosystem. Agric. For. Meteorol. 2018 , 262 , 147–156. [ Google Scholar ] [ CrossRef ]
- Zhang, H.; Wang, L.; Tian, T.; Yin, J. A Review of Unmanned Aerial Vehicle Low-Altitude Remote Sensing (UAV-LARS) Use in Agricultural Monitoring in China. Remote Sens. 2021 , 13 , 1221. [ Google Scholar ] [ CrossRef ]
- Zhang, Y.X.; Walker, J.P.; Pauwels, V.R.N.; Sadeh, Y. Assimilation of Wheat and Soil States into the APSIM-Wheat Crop Model: A Case Study. Remote Sens. 2022 , 14 , 65. [ Google Scholar ] [ CrossRef ]
- Kheir, A.M.S.; Mkuhlani, S.; Mugo, J.W.; Elnashar, A.; Nangia, V.; Devare, M.; Govind, A. Integrating APSIM model with machine learning to predict wheat yield spatial distribution. Agron. J. 2023 , 115 , 3188–3196. [ Google Scholar ] [ CrossRef ]
- Bai, T.; Zhang, N.; Mercatoris, B.; Chen, Y. Improving Jujube Fruit Tree Yield Estimation at the Field Scale by Assimilating a Single Landsat Remotely-Sensed LAI into the WOFOST Model. Remote Sens. 2019 , 11 , 1119. [ Google Scholar ] [ CrossRef ]
- Tie-cheng, B.; Wang, T.; Zhang, N.N.; Chen, Y.Q.; Mercatoris, B. Growth simulation and yield prediction for perennial jujube fruit tree by integrating age into the WOFOST model. J. Integr. Agric. 2020 , 19 , 721–734. [ Google Scholar ] [ CrossRef ]
- Shi, Y.; Wang, Z.; Hou, C.; Zhang, P. Yield estimation of Lycium barbarum L. based on the WOFOST model. Ecol. Model. 2022 , 473 , 110146. [ Google Scholar ] [ CrossRef ]
- Bellakanji, A.C.; Zribi, M.; Lili-Chabaane, Z.; Mougenot, B. Forecasting of Cereal Yields in a Semi-arid Area Using the Simple Algorithm for Yield Estimation (SAFY) Agro-Meteorological Model Combined with Optical SPOT/HRV Images. Sensors 2018 , 18 , 2138. [ Google Scholar ] [ CrossRef ]
- Huang, J.; Sedano, F.; Huang, Y.; Ma, H.; Li, X.; Liang, S.; Tian, L.; Zhang, X.; Fan, J.; Wu, W. Assimilating a synthetic Kalman filter leaf area index series into the WOFOST model to improve regional winter wheat yield estimation. Agric. For. Meteorol. 2016 , 216 , 188–202. [ Google Scholar ] [ CrossRef ]
- Fattori Junior, I.M.; dos Santos Vianna, M.; Marin, F.R. Assimilating leaf area index data into a sugarcane process-based crop model for improving yield estimation. Eur. J. Agron. 2022 , 136 , 126501. [ Google Scholar ] [ CrossRef ]
- Hu, S.; Shi, L.; Huang, K.; Zha, Y.; Hu, X.; Ye, H.; Yang, Q. Improvement of sugarcane crop simulation by SWAP-WOFOST model via data assimilation. Field Crops Res. 2019 , 232 , 49–61. [ Google Scholar ] [ CrossRef ]
- Tang, Y.; Zhou, R.; He, P.; Yu, M.; Zheng, H.; Yao, X.; Cheng, T.; Zhu, Y.; Cao, W.; Tian, Y. Estimating wheat grain yield by assimilating phenology and LAI with the WheatGrow model based on theoretical uncertainty of remotely sensed observation. Agric. For. Meteorol. 2023 , 339 , 109574. [ Google Scholar ] [ CrossRef ]
- Li, Z.; Ding, L.; Shen, B.; Chen, J.; Xu, D.; Wang, X.; Fang, W.; Pulatov, A.; Kussainova, M.; Amarjargal, A.; et al. Quantifying key vegetation parameters from Sentinel-3 and MODIS over the eastern Eurasian steppe with a Bayesian geostatistical model. Sci. Total Environ. 2024 , 909 , 168594. [ Google Scholar ] [ CrossRef ]
- Xue, H.; Xu, X.; Zhu, Q.; Meng, Y.; Long, H.; Li, H.; Song, X.; Yang, G.; Yang, M.; Li, Y.; et al. Rice yield and quality estimation coupling hierarchical linear model with remote sensing. Comput. Electron. Agric. 2024 , 218 , 108731. [ Google Scholar ] [ CrossRef ]
- Pandey, D.K.; Mishra, R. Towards sustainable agriculture: Harnessing AI for global food security. Artif. Intell. Agric. 2024 , 12 , 72–84. [ Google Scholar ] [ CrossRef ]
- Liu, Q.; Wang, C.; Jiang, J.; Wu, J.; Wang, X.; Cao, Q.; Tian, Y.; Zhu, Y.; Cao, W.; Liu, X. Multi-source data fusion improved the potential of proximal fluorescence sensors in predicting nitrogen nutrition status across winter wheat growth stages. Comput. Electron. Agric. 2024 , 219 , 108786. [ Google Scholar ] [ CrossRef ]
- Zhao, M.; Meng, Q.; Wang, L.; Zhang, L.; Hu, X.; Shi, W. Towards robust classification of multi-view remote sensing images with partial data availability. Remote Sens. Environ. 2024 , 306 , 114112. [ Google Scholar ] [ CrossRef ]
- Baltodano, A.; Agramont, A.; Lekarkar, K.; Spyrakos, E.; Reusen, I.; van Griensven, A. Exploring global remote sensing products for water quality assessment: Lake Nicaragua case study. Remote Sens. Appl. Soc. Environ. 2024 , 36 , 101331. [ Google Scholar ] [ CrossRef ]
- Zhang, H.K.; Qiu, S.; Suh, J.W.; Luo, D.; Zhu, Z. Machine Learning and Deep Learning in Remote Sensing Data Analysis. In Reference Module in Earth Systems and Environmental Sciences ; Elsevier: Amsterdam, The Netherlands, 2024. [ Google Scholar ]
- Feng, H.; Li, Q.; Wang, W.; Bashir, A.K.; Singh, A.K.; Xu, J.; Fang, K. Security of target recognition for UAV forestry remote sensing based on multi-source data fusion transformer framework. Inf. Fusion 2024 , 112 , 102555. [ Google Scholar ] [ CrossRef ]
- Joshi, P.; Sandhu, K.S.; Singh Dhillon, G.; Chen, J.; Bohara, K. Detection and monitoring wheat diseases using unmanned aerial vehicles (UAVs). Comput. Electron. Agric. 2024 , 224 , 109158. [ Google Scholar ] [ CrossRef ]
- Wu, Z.; Luo, J.; Rao, K.; Lin, H.; Song, X. Estimation of wheat kernel moisture content based on hyperspectral reflectance and satellite multispectral imagery. Int. J. Appl. Earth Obs. Geoinf. 2024 , 126 , 103597. [ Google Scholar ] [ CrossRef ]
- Qin, P.; Huang, H.; Tang, H.; Wang, J.; Liu, C. MUSTFN: A spatiotemporal fusion method for multi-scale and multi-sensor remote sensing images based on a convolutional neural network. Int. J. Appl. Earth Obs. Geoinf. 2022 , 115 , 103113. [ Google Scholar ] [ CrossRef ]
- Marin, D.B.; Ferraz, G.A.e.S.; Santana, L.S.; Barbosa, B.D.S.; Barata, R.A.P.; Osco, L.P.; Ramos, A.P.M.; Guimarães, P.H.S. Detecting coffee leaf rust with UAV-based vegetation indices and decision tree machine learning models. Comput. Electron. Agric. 2021 , 190 , 106476. [ Google Scholar ] [ CrossRef ]
- López-Pérez, E.; Sanchis-Ibor, C.; Jiménez-Bello, M.Á.; Pulido-Velazquez, M. Mapping of irrigated vineyard areas through the use of machine learning techniques and remote sensing. Agric. Water Manag. 2024 , 302 , 108988. [ Google Scholar ] [ CrossRef ]
- Hao, S.; Ryu, D.; Western, A.W.; Perry, E.; Bogena, H.; Franssen, H.J.H. Global sensitivity analysis of APSIM-wheat yield predictions to model parameters and inputs. Ecol. Model. 2024 , 487 , 110551. [ Google Scholar ] [ CrossRef ]
- Fawakherji, M.; Suriani, V.; Nardi, D.; Bloisi, D.D. Shape and style GAN-based multispectral data augmentation for crop/weed segmentation in precision farming. Crop Prot. 2024 , 184 , 106848. [ Google Scholar ] [ CrossRef ]
- Dos Santos, E.P.; Moreira, M.C.; Fernandes-Filho, E.I.; Demattê, J.A.M.; Santos, U.J.d.; da Silva, D.D.; Cruz, R.R.P.; Moura-Bueno, J.M.; Santos, I.C.; Sampaio, E.V.d.S.B. Improving the generalization error and transparency of regression models to estimate soil organic carbon using soil reflectance data. Ecol. Inform. 2023 , 77 , 102240. [ Google Scholar ] [ CrossRef ]
- Goodridge, W.; Bernard, M.; Jordan, R.; Rampersad, R. Intelligent diagnosis of diseases in plants using a hybrid Multi-Criteria decision making technique. Comput. Electron. Agric. 2017 , 133 , 80–87. [ Google Scholar ] [ CrossRef ]
- Kumar, V.; Sharma, K.V.; Kedam, N.; Patel, A.; Kate, T.R.; Rathnayake, U. A comprehensive review on smart and sustainable agriculture using IoT technologies. Smart Agric. Technol. 2024 , 8 , 100487. [ Google Scholar ] [ CrossRef ]
- Zhou, J.; Gu, X.; Gong, H.; Yang, X.; Sun, Q.; Guo, L.; Pan, Y. Intelligent classification of maize straw types from UAV remote sensing images using DenseNet201 deep transfer learning algorithm. Ecol. Indic. 2024 , 166 , 112331. [ Google Scholar ] [ CrossRef ]
- Prasanna Lakshmi, G.S.; Asha, P.N.; Sandhya, G.; Vivek Sharma, S.; Shilpashree, S.; Subramanya, S.G. An intelligent IOT sensor coupled precision irrigation model for agriculture. Meas. Sens. 2023 , 25 , 100608. [ Google Scholar ] [ CrossRef ]
- Bissadu, K.D.; Sonko, S.; Hossain, G. Society 5.0 enabled agriculture: Drivers, enabling technologies, architectures, opportunities, and challenges. Inf. Process. Agric. 2024 . [ Google Scholar ] [ CrossRef ]
- Et-taibi, B.; Abid, M.R.; Boufounas, E.-M.; Morchid, A.; Bourhnane, S.; Abu Hamed, T.; Benhaddou, D. Enhancing water management in smart agriculture: A cloud and IoT-Based smart irrigation system. Results Eng. 2024 , 22 , 102283. [ Google Scholar ] [ CrossRef ]
- Rostami, K.; Salehi, L. Rural cooperatives social responsibility in promoting Sustainability-oriented Activities in the agricultural sector: Nexus of community, enterprise, and government. Sustain. Futures 2024 , 7 , 100150. [ Google Scholar ] [ CrossRef ]
- Pingali, P.; Plavšić, M. Hunger and environmental goals for Asia: Synergies and trade-offs among the SDGs. Environ. Chall. 2022 , 7 , 100491. [ Google Scholar ] [ CrossRef ]
Click here to enlarge figure
Model Name | Application of Precision Agriculture | Reference | |
---|---|---|---|
Supervised Learning | Naive Bayes | Classification of different crop diseases, soil types, etc.; prediction of the yield of wheat, corn, and other crops. | [ , ] |
Logistic Regression | Assessment of the risk level of pest occurrence; prediction of the yield of wheat, corn, and other crops. | [ , ] | |
Linear Regression | Optimization of the amount of fertilizer application to improve the prediction accuracy of wheat, corn, and other crops yield. | [ , ] | |
Lasso Regression | Detection of the extent to which crops are attacked by diseases and insect pests. | [ , ] | |
AdaBoosT Algorithm | Classification and identification of different crop species and detection of crop diseases and insect pests. | [ , ] | |
Linear Discriminant Analysis | Classification of soil types, identification of crop varieties, and determination of the effects of different soil fertilities on crop growth. | [ , ] | |
Recurrent Neural Network | Analysis of crop growth time series data and prediction of time series changes in crop diseases and insect pests. | [ , ] | |
Decision Tree | Selection of pest management strategies; identification of crop pest types. | [ , ] | |
Nearest Neighbor Algorithm | Identification of different crop varieties; evaluation of soil fertility grades. | [ , ] | |
XGBoost Algorithm | Prediction of yield of wheat, corn, and other crops based on climate, soil conditions, and other variables. | [ , ] | |
Long Short-Term Memory Network | Forecasting the long-term trend of crop yield based on climate variables, such as precipitation and temperature, and prediction of the outbreak of crop diseases and insect pests by time series. | [ , ] | |
Support Vector Regression | Crop growth monitoring and modeling, using remote sensing reflectance data to predict crop leaf area index, yield, etc. | [ , ] | |
Artificial Neural Network | Identification of crop diseases and insect pests; crop growth monitoring and modeling; prediction of crop leaf area index, yield, etc. | [ , ] | |
Convolutional Neural Algorithm | Identification of crop leaf diseases and detection of disease invasion degree of crop leaves; prediction of crop leaf area index, yield, etc. | [ , ] | |
Random Forest | Identification of crop diseases and insect pests; crop growth monitoring and modeling; prediction of crop leaf area index, yield, etc. | [ , ] | |
Support Vector Machine | Identification of crop diseases and insect pests; crop growth monitoring and modeling; prediction of crop leaf area index, yield, etc. | [ , ] | |
CatBoosT Algorithm | Identification of crop leaf diseases and detection of disease invasion degree of crop leaves. | [ , ] | |
Ridge Regression | Prediction of soil nutrients and key nutrient content based on soil sample data. | [ , ] | |
Random Gradient Descent | Optimization of model parameters to improve the accuracy of agricultural prediction and decision-making models; application to complex agricultural system modeling and prediction. | [ , ] | |
Semi supervised learning | Generative Semi-Supervised Learning | Assessment of soil quality; prediction of soil fertility, acidity, alkalinity, etc.; prediction and control of diseases and insect pests. | [ , ] |
Autoencoders | Identification and classification of diseases and insect pests; assessment of the risk level of pest occurrence. | [ ] | |
Unsupervised | Co-Training | Identification, classification, and risk assessment of diseases and insect pests; soil type classification. | [ ] |
Learning | Probabilistic Graphical Model | Identification of crop diseases and insect pests; crop growth monitoring and modeling; prediction of crop leaf area index, yield, etc. | [ ] |
Independent Component Analysis | Identification, classification, and risk assessment of diseases and insect pests; soil type classification. | [ ] | |
Anomaly Detection Algorithm | Detection of crop wilt, soil moisture, and pH anomaly. | [ ] | |
Self-Organizing Maps | Classification of crops and rapid identification of soil types. | [ ] | |
K-Means Clustering | Accurate identification of crops. | [ ] | |
Principal Component Analysis | Accurate classification of crops based on their growth characteristics (such as color, texture, size, etc.). | [ ] | |
Reinforcement | Deep Q-Network | Retrieval of key growth information, such as vegetation index, to effectively monitor crop growth and development. | [ ] |
Policy Gradient Methods | Optimization of crop irrigation and fertilization strategies. | [ ] | |
Q-learning | Optimization of agricultural decision making and environmental interaction. | [ ] |
The statements, opinions and data contained in all publications are solely those of the individual author(s) and contributor(s) and not of MDPI and/or the editor(s). MDPI and/or the editor(s) disclaim responsibility for any injury to people or property resulting from any ideas, methods, instructions or products referred to in the content. |
Share and Cite
Wang, J.; Wang, Y.; Li, G.; Qi, Z. Integration of Remote Sensing and Machine Learning for Precision Agriculture: A Comprehensive Perspective on Applications. Agronomy 2024 , 14 , 1975. https://doi.org/10.3390/agronomy14091975
Wang J, Wang Y, Li G, Qi Z. Integration of Remote Sensing and Machine Learning for Precision Agriculture: A Comprehensive Perspective on Applications. Agronomy . 2024; 14(9):1975. https://doi.org/10.3390/agronomy14091975
Wang, Jun, Yanlong Wang, Guang Li, and Zhengyuan Qi. 2024. "Integration of Remote Sensing and Machine Learning for Precision Agriculture: A Comprehensive Perspective on Applications" Agronomy 14, no. 9: 1975. https://doi.org/10.3390/agronomy14091975
Article Metrics
Article access statistics, further information, mdpi initiatives, follow mdpi.
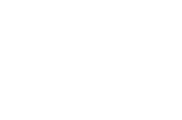
Subscribe to receive issue release notifications and newsletters from MDPI journals
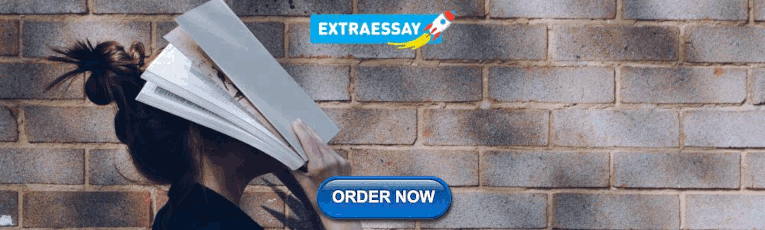
IMAGES
VIDEO
COMMENTS
Introduction. Learning is a continuous process that involves the transformation of information and experience into abilities and knowledge. Learning, according to me, is a two way process that involves the learner and the educator leading to knowledge acquisition as well as capability. It informs my educational sector by making sure that both ...
So, almost certainly, online classes sometimes benefit students. In comparisons of online and in-person classes, however, online classes aren't as effective as in-person classes for most ...
Learning Is An Effective Learner Essay. Learning is, for me, a continuous journey that is always changing. It creates personal and memorable experiences that allows me to come away a better person than I was the day before. Learning has enabled me to discover myself as an individual and recognise my strengths and weaknesses which I continue to ...
F eatures highlighted by this definition include: • an active process in which the learner relates new. experience to existing meaning, and may. accommodate and assimilate new ideas. • past ...
Learning is essential for personal growth and development. It equips us with the knowledge and skills necessary to navigate life effectively. Through learning, we acquire the ability to think critically, solve problems, and make informed decisions. It fosters creativity and innovation, enabling us to generate new ideas and solutions.
Designing effective learning environments includes considering the goals for learning and goals for students. This comparison highlights the fact that there are various means for approaching goals of learning, and furthermore, that goals for students change over time. As goals and objectives have changed, so has the research base on effective ...
Improving Students' Learning With Effective ...
It's creating better learning. And then as a learner, you are more motivated to replace these ineffective techniques with more effective techniques. Harvard Extension: You talk about tips for learners, how to make it stick. And there are several methods or tips that you share: elaboration, generation, reflection, calibration, among others.
3. Neither the amount of time spent on an app nor the number of sessions in an app correlates with effectiveness. A recent study found that the "dosage" of the app, such as the number of sessions, time spent per session, and duration of the study, did not predict effectiveness of the app.Thus, learning outcomes did not change if a student spent more or less time in an application.
Stanford University's first essay prompt asks you to respond to the following: "The Stanford community is deeply curious and driven to learn in and out of the classroom. Reflect on an idea or experience that makes you genuinely excited about learning. (100-250 words)". For this short answer question, your response is limited to a maximum ...
Jonassen's (1994) description of 8 pedagogical practices that differentiate constructivist learning from other learning environments is a succinct and practical summary of the constructivist perspective: 1. Constructivist learning environments provide multiple representations of reality. 2.
Introduction. The learning environment (LE) comprises the psychological, social, cultural, and physical setting in which learning occurs and in which experiences and expectations are co-created among its participants (Rusticus et al., 2020; Shochet et al., 2013).These individuals, who are primarily students, faculty and staff, engage in this environment and the learning process as they ...
Reflective Essay on Learning and Teaching. Kerwin Anthony Livingstone, PhD. Applied Linguist/Language Scientist/Education Specialist, Guyana. ABSTRACT: Learning and teaching are inextricably ...
Conclusion. In conclusion, good study habits are essential for academic success. Various study habits such as time management, active learning, note-taking, repetition and practice, mnemonics, visualization, test-taking strategies, reviewing material, time management during exams, time management during online learning, active learning during online learning, and self-motivation during online ...
This process of self-reflection has allowed me to gain a deeper understanding of myself, my values, and my goals. It has also empowered me to challenge my own assumptions, embrace personal growth, and develop a greater sense of self-awareness. Another important lesson I have learned through my learning experience is the importance of resilience ...
The science of learning has made a considerable contribution to our understanding of effective teaching and learning strategies. However, few instructors outside of the field are privy to this research. In this tutorial review, we focus on six specific cognitive strategies that have received robust support from decades of research: spaced practice, interleaving, retrieval practice, elaboration ...
Is Online Learning Effective?
It has significantly improved the entire learning process and learning experience and improved the accessibility of learners to learning material, thus psychologically preparing students for the ... Read More. Pages: 6 Words: 1567. View Sample. Essay writing services. for smart students. Thousands of students use our. services for writing their ...
How to Learn More Effectively: 10 Learning Techniques
How to Study Effectively: 12 Secrets For Success
2. Organize your ideas: Before you start writing, outline the main points you want to cover in your essay. This will help you organize your thoughts and ensure a logical flow of ideas. 3. Use topic sentences: Begin each paragraph with a topic sentence that introduces the main idea of the paragraph.
With support from the principals, these expert teachers were also able to promote effective and rigorous teacher professional learning, for example, by supporting teacher involvement in action learning projects in the classroom; in external professional development programs; and by fostering professional discussions on language development and ...
Due to current global population growth, resource shortages, and climate change, traditional agricultural models face major challenges. Precision agriculture (PA), as a way to realize the accurate management and decision support of agricultural production processes using modern information technology, is becoming an effective method of solving these challenges. In particular, the combination ...
Context: Software Quality Assurance (SQA) is a fundamental part of software engineering to ensure stakeholders that software products work as expected after release in operation. Machine Learning (ML) has proven to be able to boost SQA activities and contribute to the development of quality software systems. In this context, Causal Reasoning is gaining increasing interest as a methodology to ...