Day | Temp |
Monday | 77 |
Tuesday | 76 |
Wednesday | 74 |
Thursday | 78 |
Friday | 78 |
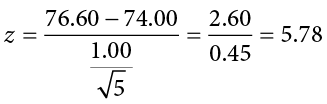
This value falls so far into the tail that it cannot even be plotted on the distribution ( Figure 7.7 )! Because the result is significant, you also calculate an effect size:
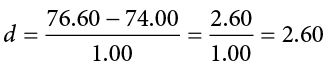
The effect size you calculate is definitely large, meaning someone has some explaining to do!
Figure 7.7. Obtained z statistic. (“ Obtained z5.77 ” by Judy Schmitt is licensed under CC BY-NC-SA 4.0 .)
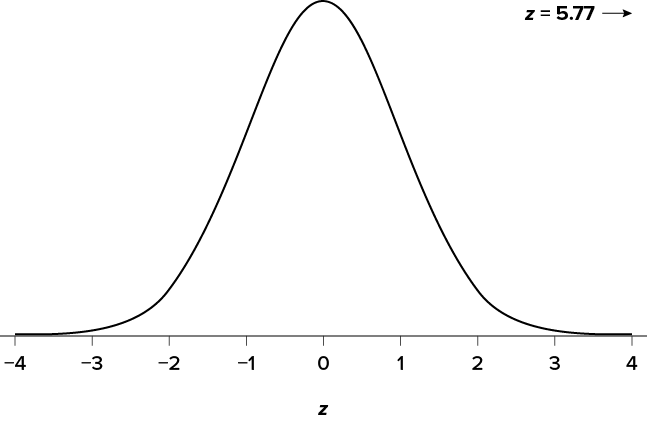
You compare your obtained z statistic, z = 5.77, to the critical value, z * = 1.645, and find that z > z *. Therefore you reject the null hypothesis, concluding:
Reject H 0 . Based on 5 observations, the average temperature ( M = 76.6 degrees) is statistically significantly higher than it is supposed to be, and the effect size was large, z = 5.77, p < .05, d = 2.60.
Example C Different Significance Level
Finally, let’s take a look at an example phrased in generic terms, rather than in the context of a specific research question, to see the individual pieces one more time. This time, however, we will use a stricter significance level, a = .01, to test the hypothesis.
We will use 60 as an arbitrary null hypothesis value:

We will assume a two-tailed test:
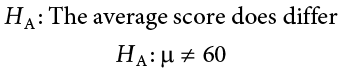
We have seen the critical values for z tests at a = .05 levels of significance several times. To find the values for a = .01, we will go to the Standard Normal Distribution Table and find the z score cutting off .005 (.01 divided by 2 for a two-tailed test) of the area in the tail, which is z * = ±2.575. Notice that this cutoff is much higher than it was for a = .05. This is because we need much less of the area in the tail, so we need to go very far out to find the cutoff. As a result, this will require a much larger effect or much larger sample size in order to reject the null hypothesis.
We can now calculate our test statistic. We will use s = 10 as our known population standard deviation and the following data to calculate our sample mean:
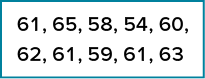
The average of these scores is M = 60.40. From this we calculate our z statistic as:
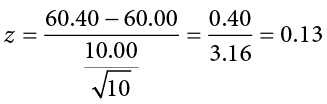
The Cohen’s d effect size calculation is:

Our obtained z statistic, z = 0.13, is very small. It is much less than our critical value of 2.575. Thus, this time, we fail to reject the null hypothesis. Our conclusion would look something like:
Fail to reject H 0 . Based on the sample of 10 scores, we cannot conclude that there is an effect causing the mean ( M = 60.40) to be statistically significantly different from 60.00, z = 0.13, p > .01, d = 0.04, and the effect size supports this interpretation.
Other Considerations in Hypothesis Testing
There are several other considerations we need to keep in mind when performing hypothesis testing.
Errors in Hypothesis Testing
In the Physicians’ Reactions case study, the probability value associated with the significance test is .0057. Therefore, the null hypothesis was rejected, and it was concluded that physicians intend to spend less time with obese patients. Despite the low probability value, it is possible that the null hypothesis of no true difference between obese and average-weight patients is true and that the large difference between sample means occurred by chance. If this is the case, then the conclusion that physicians intend to spend less time with obese patients is in error. This type of error is called a Type I error. More generally, a Type I error occurs when a significance test results in the rejection of a true null hypothesis.
The second type of error that can be made in significance testing is failing to reject a false null hypothesis. This kind of error is called a Type II error . Unlike a Type I error, a Type II error is not really an error. When a statistical test is not significant, it means that the data do not provide strong evidence that the null hypothesis is false. Lack of significance does not support the conclusion that the null hypothesis is true. Therefore, a researcher should not make the mistake of incorrectly concluding that the null hypothesis is true when a statistical test was not significant. Instead, the researcher should consider the test inconclusive. Contrast this with a Type I error in which the researcher erroneously concludes that the null hypothesis is false when, in fact, it is true.
A Type II error can only occur if the null hypothesis is false. If the null hypothesis is false, then the probability of a Type II error is called b (“beta”). The probability of correctly rejecting a false null hypothesis equals 1 − b and is called statistical power . Power is simply our ability to correctly detect an effect that exists. It is influenced by the size of the effect (larger effects are easier to detect), the significance level we set (making it easier to reject the null makes it easier to detect an effect, but increases the likelihood of a Type I error), and the sample size used (larger samples make it easier to reject the null).
Misconceptions in Hypothesis Testing
Misconceptions about significance testing are common. This section lists three important ones.
- Misconception: The probability value ( p value) is the probability that the null hypothesis is false. Proper interpretation: The probability value ( p value) is the probability of a result as extreme or more extreme given that the null hypothesis is true. It is the probability of the data given the null hypothesis. It is not the probability that the null hypothesis is false.
- Misconception: A low probability value indicates a large effect. Proper interpretation: A low probability value indicates that the sample outcome (or an outcome more extreme) would be very unlikely if the null hypothesis were true. A low probability value can occur with small effect sizes, particularly if the sample size is large.
- Misconception: A non-significant outcome means that the null hypothesis is probably true. Proper interpretation: A non-significant outcome means that the data do not conclusively demonstrate that the null hypothesis is false.
- In your own words, explain what the null hypothesis is.
- What are Type I and Type II errors?
- Why do we phrase null and alternative hypotheses with population parameters and not sample means?
- Why do we state our hypotheses and decision criteria before we collect our data?
- Why do you calculate an effect size?
- z = 1.99, two-tailed test at a = .05
- z = 0.34, z * = 1.645
- p = .03, a = .05
- p = .015, a = .01
Answers to Odd-Numbered Exercises
Your answer should include mention of the baseline assumption of no difference between the sample and the population.
Alpha is the significance level. It is the criterion we use when deciding to reject or fail to reject the null hypothesis, corresponding to a given proportion of the area under the normal distribution and a probability of finding extreme scores assuming the null hypothesis is true.
We always calculate an effect size to see if our research is practically meaningful or important. NHST (null hypothesis significance testing) is influenced by sample size but effect size is not; therefore, they provide complimentary information.

“ Null Hypothesis ” by Randall Munroe/xkcd.com is licensed under CC BY-NC 2.5 .)
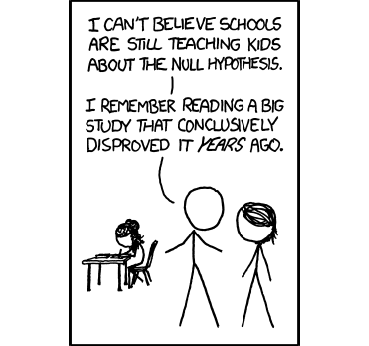
Introduction to Statistics in the Psychological Sciences Copyright © 2021 by Linda R. Cote Ph.D.; Rupa G. Gordon Ph.D.; Chrislyn E. Randell Ph.D.; Judy Schmitt; and Helena Marvin is licensed under a Creative Commons Attribution-NonCommercial-ShareAlike 4.0 International License , except where otherwise noted.
Share This Book
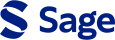
- Request new password
- Create a new account
A Step-by-Step Introduction to Statistics for Business
Student resources, chapter 7: hypothesis testing.
Answers for all ‘Test Yourself’ questions from the book to check your performance and widen your overall understanding of the contents.
- Bad; we can’t test for “the same”
- Bad; this is not specific – better than when?
- One-tailed, “more”
- Two-tailed, “different”
- One-tailed, “increase”
- No, .02 > .01
- No, .06 > .05
- Yes, .06 < .10
- Retain the null. The difference is not statistically significant. There is insufficient evidence to conclude that women have more fun than men at our store.
- Reject the null and accept the alternative. The difference is statistically significant. Employee retention is different between branches.
- Reject the null. The difference is statistically significant. Sales increased after sales training.
- H 1 : μ ≠ 10
- H 1 : μ < 0
- H 1 : μ > 15
- H 0 : μ ≤ -10
- H 0 : μ = 60
- H 0 : μ ≥ 100
- You are more likely to reject the null is α = .10 because the region of rejection is larger (10% of the sampling distribution described by the null instead of 5%).
- ξ = .02, p > .05
Information
Initiatives
You are accessing a machine-readable page. In order to be human-readable, please install an RSS reader.
All articles published by MDPI are made immediately available worldwide under an open access license. No special permission is required to reuse all or part of the article published by MDPI, including figures and tables. For articles published under an open access Creative Common CC BY license, any part of the article may be reused without permission provided that the original article is clearly cited. For more information, please refer to https://www.mdpi.com/openaccess .
Feature papers represent the most advanced research with significant potential for high impact in the field. A Feature Paper should be a substantial original Article that involves several techniques or approaches, provides an outlook for future research directions and describes possible research applications.
Feature papers are submitted upon individual invitation or recommendation by the scientific editors and must receive positive feedback from the reviewers.
Editor’s Choice articles are based on recommendations by the scientific editors of MDPI journals from around the world. Editors select a small number of articles recently published in the journal that they believe will be particularly interesting to readers, or important in the respective research area. The aim is to provide a snapshot of some of the most exciting work published in the various research areas of the journal.
Original Submission Date Received: .
- Active Journals
- Find a Journal
- Proceedings Series
- For Authors
- For Reviewers
- For Editors
- For Librarians
- For Publishers
- For Societies
- For Conference Organizers
- Open Access Policy
- Institutional Open Access Program
- Special Issues Guidelines
- Editorial Process
- Research and Publication Ethics
- Article Processing Charges
- Testimonials
- Preprints.org
- SciProfiles
- Encyclopedia
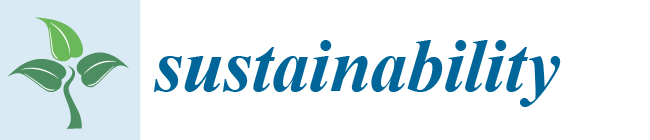
Article Menu
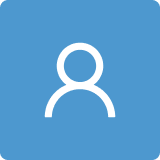
- Subscribe SciFeed
- Recommended Articles
- Google Scholar
- on Google Scholar
- Table of Contents
Find support for a specific problem in the support section of our website.
Please let us know what you think of our products and services.
Visit our dedicated information section to learn more about MDPI.
JSmol Viewer
Filling discrepancies between consumer perception and actual piped water quality to promote the potable use of the municipal water supply in indonesia.

1. Introduction
2. materials and methods, 2.1. study areas, 2.2. questionnaire survey, 2.3. water quality analyses, 2.4. cost analysis, 2.5. public drinking water tap, 2.6. data analysis, 3.1. perception of water quality, 3.2. water quality, 3.3. cost comparison of different water sources for drinking, 3.4. public drinking stations, 4. discussion, 5. conclusions, author contributions, institutional review board statement, informed consent statement, data availability statement, acknowledgments, conflicts of interest.
Click here to enlarge figure
- Greene, J. Bottled Water in Mexico: The Rise of a New Access to Water Paradigm. Wiley Interdiscip. Rev. Water 2018 , 5 , e1286. [ Google Scholar ] [ CrossRef ]
- Cohen, A.; Ray, I. The Global Risks of Increasing Reliance on Bottled Water. In Nature Sustainability ; Nature Publishing Group: Berlin, Germany, 2018; pp. 327–329. [ Google Scholar ] [ CrossRef ]
- Bouhlel, Z.; Köpke, J.; Mina, M.; Smakhtin, V. Global Bottled Water Industry: A Review of Impacts and Trends ; University Institute for Water, Environment and Health: Hamilton, ON, Canada, 2023. [ Google Scholar ]
- Foster, T.; Priadi, C.; Kotra, K.K.; Odagiri, M.; Rand, E.C.; Willetts, J. Self-Supplied Drinking Water in Low- and Middle-Income Countries in the Asia-Pacific. NPJ Clean Water 2021 , 4 , 37. [ Google Scholar ] [ CrossRef ]
- Tosun, J.; Scherer, U.; Schaub, S.; Horn, H. Making Europe Go from Bottles to the Tap: Political and Societal Attempts to Induce Behavioral Change. Wiley Interdiscip. Rev. Water 2020 , 7 , e1435. [ Google Scholar ] [ CrossRef ]
- Brei, V.A. How Is a Bottled Water Market Created? Wiley Interdiscip. Rev. Water 2018 , 5 , e1220. [ Google Scholar ] [ CrossRef ]
- Geerts, R.; Vandermoere, F.; Van Winckel, T.; Halet, D.; Joos, P.; Van Den Steen, K.; Van Meenen, E.; Blust, R.; Borregán-Ochando, E.; Vlaeminck, S.E. Bottle or Tap? Toward an Integrated Approach to Water Type Consumption. Water Res. 2020 , 173 , 115578. [ Google Scholar ] [ CrossRef ]
- Jaffee, D. Unequal Trust: Bottled Water Consumption, Distrust in Tap Water, and Economic and Racial Inequality in the United States. Wiley Interdiscip. Rev. Water 2024 , 11 , e1700. [ Google Scholar ] [ CrossRef ]
- Alfonso, S.M.; Kazama, S.; Takizawa, S. Inequalities in Access to and Consumption of Safely Managed Water Due to Socio-Economic Factors: Evidence from Quezon City, Philippines. Curr. Res. Environ. Sustain. 2021 , 4 , 100117. [ Google Scholar ] [ CrossRef ]
- Ochoo, B.; Valcour, J.; Sarkar, A. Association between Perceptions of Public Drinking Water Quality and Actual Drinking Water Quality: A Community-Based Exploratory Study in Newfoundland (Canada). Environ. Res. 2017 , 159 , 435–443. [ Google Scholar ] [ CrossRef ] [ PubMed ]
- Dianty, F.R.; Martono, D.N.; Priadi, C.R. Public Perception of Drinking Water Quality in Bekasi City, Indonesia. J. Penelit. Pendidik. IPA 2022 , 8 , 2551–2555. [ Google Scholar ] [ CrossRef ]
- de França Doria, M. Factors Influencing Public Perception of Drinking Water Quality. Water Policy 2009 , 12 , 1–19. [ Google Scholar ] [ CrossRef ]
- Hopland, A.O.; Kvamsdal, S.F. Tap Water Quality: In the Eye of the Beholder. J. Water Health 2022 , 20 , 1436–1444. [ Google Scholar ] [ CrossRef ]
- Delpla, I.; Legay, C.; Proulx, F.; Rodriguez, M.J. Perception of Tap Water Quality: Assessment of the Factors Modifying the Links between Satisfaction and Water Consumption Behavior. Sci. Total Environ. 2020 , 722 , 137786. [ Google Scholar ] [ CrossRef ] [ PubMed ]
- Lestari, P.; Trihadiningrum, Y. The Impact of Improper Solid Waste Management to Plastic Pollution in Indonesian Coast and Marine Environment. Mar. Pollut. Bull. 2019 , 149 , 110505. [ Google Scholar ] [ CrossRef ] [ PubMed ]
- Jaffee, D.; Newman, S. A Bottle Half Empty: Bottled Water, Commodification, and Contestation. Organ. Environ. 2013 , 26 , 318–335. [ Google Scholar ] [ CrossRef ]
- Puspita, T.; Dharmayanti, I.; Tjandrarini, D.H.; Zahra, Z.; Anwar, A.; Irianto, J.; Rachmat, B.; Yunianto, A. Packaged Drinking Water in Indonesia: The Determinants of Household in the Selection and Management Process. J. Water Sanit. Hyg. Dev. 2023 , 13 , 508–519. [ Google Scholar ] [ CrossRef ]
- Horowitz, N.; Frago, J.; Mu, D. Life Cycle Assessment of Bottled Water: A Case Study of Green2O Products. Waste Manag. 2018 , 76 , 734–743. [ Google Scholar ] [ CrossRef ] [ PubMed ]
- Gleick, P.H.; Cooley, H.S. Energy Implications of Bottled Water. Environ. Res. Lett. 2009 , 4 , 014009. [ Google Scholar ] [ CrossRef ]
- Garfí, M.; Cadena, E.; Sanchez-Ramos, D.; Ferrer, I. Life Cycle Assessment of Drinking Water: Comparing Conventional Water Treatment, Reverse Osmosis and Mineral Water in Glass and Plastic Bottles. J. Clean. Prod. 2016 , 137 , 997–1003. [ Google Scholar ] [ CrossRef ]
- Garcia-Suarez, T.; Kulak, M.; King, H.; Chatterton, J.; Gupta, A.; Saksena, S. Life Cycle Assessment of Three Safe Drinking-Water Options in India: Boiledwater, Bottledwater, Andwater Purified with a Domestic Reverse-Osmosis Device. Sustainability 2019 , 11 , 6233. [ Google Scholar ] [ CrossRef ]
- Circular, G.A. Accelerating the Circular Economy for Post-Consumer PET Bottles in Southeast Asia ; EcoKnights: Singapore, 2019. [ Google Scholar ]
- Pinter, E.; Welle, F.; Mayrhofer, E.; Pechhacker, A.; Motloch, L.; Lahme, V.; Grant, A.; Tacker, M. Circularity Study on Pet Bottle-to-Bottle Recycling. Sustainability 2021 , 13 , 7370. [ Google Scholar ] [ CrossRef ]
- Smith, R.L.; Takkellapati, S.; Riegerix, R.C. Recycling of Plastics in the United States: Plastic Material Flows and Polyethylene Terephthalate (PET) Recycling Processes. ACS Sustain. Chem. Eng. 2022 , 10 , 2084–2096. [ Google Scholar ] [ CrossRef ]
- Andrady, A.L. The Plastic in Microplastics: A Review. Mar. Pollut. Bull. 2017 , 119 , 12–22. [ Google Scholar ] [ CrossRef ]
- Umoafia, N.; Joseph, A.; Edet, U.; Nwaokorie, F.; Henshaw, O.; Edet, B.; Asanga, E.; Mbim, E.; Chikwado, C.; Obeten, H. Deterioration of the Quality of Packaged Potable Water (Bottled Water) Exposed to Sunlight for a Prolonged Period: An Implication for Public Health. Food Chem. Toxicol. 2023 , 175 , 113728. [ Google Scholar ] [ CrossRef ]
- Akhbarizadeh, R.; Dobaradaran, S.; Schmidt, T.C.; Nabipour, I.; Spitz, J. Worldwide Bottled Water Occurrence of Emerging Contaminants: A Review of the Recent Scientific Literature. J. Hazard. Mater. 2020 , 392 , 122271. [ Google Scholar ] [ CrossRef ]
- Schymanski, D.; Goldbeck, C.; Humpf, H.U.; Fürst, P. Analysis of Microplastics in Water by Micro-Raman Spectroscopy: Release of Plastic Particles from Different Packaging into Mineral Water. Water Res. 2018 , 129 , 154–162. [ Google Scholar ] [ CrossRef ]
- Oßmann, B.E.; Sarau, G.; Holtmannspötter, H.; Pischetsrieder, M.; Christiansen, S.H.; Dicke, W. Small-Sized Microplastics and Pigmented Particles in Bottled Mineral Water. Water Res. 2018 , 141 , 307–316. [ Google Scholar ] [ CrossRef ] [ PubMed ]
- Kooy, M.; Walter, C.T. Towards a Situated Urban Political Ecology Analysis of Packaged Drinking Water Supply. Water 2019 , 11 , 225. [ Google Scholar ] [ CrossRef ]
- Ko, S.H.; Sakai, H. Perceptions of Water Quality, and Current and Future Water Consumption of Residents in the Central Business District of Yangon City Myanmar. Water Supply 2022 , 22 , 1094–1106. [ Google Scholar ] [ CrossRef ]
- Ikhsan, A.N.; Thohira, M.C.; Daniel, D. Analysis of Packaged Drinking Water Use in Indonesia in the Last Decades: Trends, Socio-Economic Determinants, and Safety Aspect. Water Policy 2022 , 24 , 1287–1305. [ Google Scholar ] [ CrossRef ]
- Prasetiawan, T.; Nastiti, A.; Muntalif, B.S. ‘Bad’ Piped Water and Other Perceptual Drivers of Bottled Water Consumption in Indonesia. Wiley Interdiscip. Rev. Water 2017 , 4 , e1219. [ Google Scholar ] [ CrossRef ]
- Indonesia Statistics Agency. Percentage Distribution of Households by Province and Source of Drinking Water, 2021 ; Indonesia Statistics Agency: Jakarta, Republic of Indonesia, 2021. Available online: https://www.bps.go.id/id/statistics-table/3/YzBaMlduSlFVbTVrUnpWeU9YRTJka0pVTTFkU1FUMDkjMw==/distribusi-persentase-rumah-tangga-menurut-provinsi-dan-sumber-air-minum.html?year=2021 (accessed on 2 April 2024).
- Ministry of Public Works and Housing (Indonesia). PDAM Capacity and Services. Ministry of Public Works and Housing, Indonesia. Available online: https://data.pu.go.id/dataset/kapasitas-dan-layanan-pdam (accessed on 29 April 2024).
- Jambeck, J.R.; Geyer, R.; Wilcox, C.; Siegler, T.R.; Perryman, M.; Andrady, A.; Narayan, R.; Law, K.L. Plastic Waste Inputs from Land into the Ocean. Mar. Pollut. 2015 , 347 , 768–771. [ Google Scholar ] [ CrossRef ] [ PubMed ]
- Bogor City Statistics Agency. Bogor City Rainfall (Curah Hujan Kota Bogor—In Bahasa Indonesia). Available online: https://bogorkota.bps.go.id/indicator/151/153/1/jumlah-curah-hujan.html (accessed on 28 June 2024).
- Malang City Statistics Agency. Malang City Rainfall (Curah Hujan Kota Malang—In Bahasa Indonesia). Available online: https://malangkota.bps.go.id/indicator/151/508/1/jumlah-curah-hujan-di-kota-malang.html (accessed on 28 June 2024).
- Semarang City Statistics Agency. Semarang City Rainfall (Curah Hujan Kota Semarang—In Bahasa Indonesia). Available online: https://semarangkota.bps.go.id/indicator/151/79/1/curah-hujan-kota-semarang.html (accessed on 28 June 2024).
- Bogor City Statistics Agency. Bogor City Population Based on Age and Gender Groups 2014–2021 (Penduduk Kota Bogor Berdasarkan Kelompok Umur dan Jenis Kelamin, 2014–2021—In Bahasa Indonesia). Available online: https://bogorkota.bps.go.id/indicator/12/31/1/penduduk-kota-bogor-berdasarkan-kelompok-umur-dan-jenis-kelamin.html (accessed on 13 May 2024).
- Malang City Statistics Agency. Malang City Population Density 2019–2021 (Kepadatan Penduduk Kota Malang—In Bahasa Indonesia). Available online: https://malangkota.bps.go.id/indicator/12/304/1/kepadatan-penduduk-menurut-kecamatan.html (accessed on 13 May 2024).
- Semarang City Government. Semarang City Population (Jumlah Penduduk Kota Semarang—In Bahasa Indonesia). Available online: https://data.semarangkota.go.id/elemendata/cari?cari=penduduk&tahunAwal=2021&tahunAkhir=2021 (accessed on 13 May 2024).
- Bogor City Government. Bogor City Water Utility Company Targets 97% Service Coverage in 2019 (PDAM Tirta Pakuan Targetkan Cakupan Layanan 97 Persen di 2019—In Bahasa Indonesia). Available online: https://kotabogor.go.id/index.php/show_post/detail/11460 (accessed on 13 May 2024).
- Malang City Water Utility Company (PDAM Tugu Tirta Kota Malang. Water Utility Statistics (Statistik PDAM Kota Malang—In Bahasa Indonesia). Available online: https://perumdatugutirta.co.id/info/statistik (accessed on 13 May 2024).
- Semarang City Water Utility Company (PDAM Tirta Moedal Kota Semarang). Production Infrastructure (Infrastruktur Produksi—In Bahasa Indonesia). Available online: https://pdamkotasmg.co.id/page/instalasi_pengolahan_air (accessed on 13 May 2024).
- Nastiti, A.; Sudradjat, A.; Geerling, G.W.; Smits, A.J.M.; Roosmini, D.; Muntalif, B.S. The Effect of Physical Accessibility and Service Level of Water Supply on Economic Accessibility: A Case Study of Bandung City, Indonesia. Water Int. 2017 , 42 , 831–851. [ Google Scholar ] [ CrossRef ]
- Khanal, S.; Kazama, S.; Benyapa, S.; Takizawa, S. Performance Assessment of Household Water Treatment and Safe Storage in Kathmandu Valley, Nepal. Water 2023 , 15 , 2305. [ Google Scholar ] [ CrossRef ]
- Devesa, R.; Dietrich, A.M. Guidance for Optimizing Drinking Water Taste by Adjusting Mineralization as Measured by Total Dissolved Solids (TDS). Desalination 2018 , 439 , 147–154. [ Google Scholar ] [ CrossRef ]
- Shuai, Y.; Zhang, K.; Zhu, H.; Lou, J.; Zhang, T. Toward the Upgrading Quality of Drinking Water from Flavor Evaluation: Taste, Feeling, and Retronasal Odor Issues. ACS ES T Eng. 2023 , 3 , 308–321. [ Google Scholar ] [ CrossRef ]
- Fankhauser, S.; Tepic, S. Can Poor Consumers Pay for Energy and Water? An Affordability Analysis for Transition Countries. Energy Policy 2007 , 35 , 1038–1049. [ Google Scholar ] [ CrossRef ]
- Prouty, C.; Zhang, Q. How Do People’s Perceptions of Water Quality Influence the Life Cycle Environmental Impacts of Drinking Water in Uganda? Resour. Conserv. Recycl. 2016 , 109 , 24–33. [ Google Scholar ] [ CrossRef ]
- Etale, A.; Jobin, M.; Siegrist, M. Tap versus Bottled Water Consumption: The Influence of Social Norms, Affect and Image on Consumer Choice. Appetite 2018 , 121 , 138–146. [ Google Scholar ] [ CrossRef ]
- Nastiti, A.; Muntalif, B.S.; Roosmini, D.; Sudradjat, A.; Meijerink, S.V.; Smits, A.J.M. Coping with Poor Water Supply in Peri-Urban Bandung, Indonesia: Towards a Framework for Understanding Risks and Aversion Behaviours. Environ. Urban 2017 , 29 , 69–88. [ Google Scholar ] [ CrossRef ]
- Espinosa-García, A.C.; Díaz-Ávalos, C.; González-Villarreal, F.J.; Val-Segura, R.; Malvaez-Orozco, V.; Mazari-Hiriart, M. Drinking Water Quality in a Mexico City University Community: Perception and Preferences. Ecohealth 2015 , 12 , 88–97. [ Google Scholar ] [ CrossRef ]
- Piriou, P.; Mackey, E.D.; Suffet, I.H.; Bruchet, A. Chlorinous Flavor Perception in Drinking Water. Water Sci. Technol. 2004 , 49 , 321–328. [ Google Scholar ] [ CrossRef ] [ PubMed ]
- Mackey, E.D.; Baribeau, H.; Crozes, G.F.; Suffet, I.H.; Piriou, P. Public Thresholds for Chlorinous Flavors in U.S. Tap Water. Water Sci. Technol. 2004 , 49 , 335–340. [ Google Scholar ] [ CrossRef ] [ PubMed ]
- Wening Purwandari, T.; Kazama, S.; Takizawa, S. Water Consumption Analysis of Small Islands Supplied with Desalinated Water in Indonesia. J. Jpn. Soc. Civ. Eng. Ser. G (Environ. Res.) 2021 , 77 , 129–140. [ Google Scholar ] [ CrossRef ] [ PubMed ]
- Prayoga, R.; Nastiti, A.; Schindler, S.; Kusumah, S.W.D.; Sutadian, A.D.; Sundana, E.J.; Simatupang, E.; Wibowo, A.; Budiwantoro, B.; Sedighi, M. Perceptions of Drinking Water Service of the ‘off-Grid’ Community in Cimahi, Indonesia. Water 2021 , 13 , 1398. [ Google Scholar ] [ CrossRef ]
- Javidi, A.; Pierce, G.U.S. Households’ Perception of Drinking Water as Unsafe and Its Consequences: Examining Alternative Choices to the Tap. Water Resour. Res. 2018 , 54 , 6100–6113. [ Google Scholar ] [ CrossRef ]
| Bogor City | Malang City | Semarang City |
---|
Total area (km ) | 118.15 | 101.1 | 373.78 |
Annual rainfall (mm) | 3400 [ ] | 2400 [ ] | 2500 [ ] |
Total population (persons) | 1,052,359 [ ] | 864,173 [ ] | 1,694,743 [ ] |
Population density (persons/km ) | 8782 [ ] | 7677 [ ] | 4534 [ ] |
Service coverage (%) | 91 [ ] | 100 [ ] | 60 [ ] |
Water production (m /d) | 230,794 [ ] | 151,042 [ ] | 284,497 [ ] |
Production per capita (m /d. person) | 0.22 | 0.17 | 0.17 |
Water supply source (m /d) | Rivers 200,844 Springs 29,950 [ ] | Springs 118,914 Deep wells 32,128 [ ] | Rivers 213,415 Springs 26,542 Deep wells 44,540 [ ] |
Cost | PDAM Water | Packaged Water | Refill Station Water |
---|
Boiling | LPG stove IDR 29,200 (USD 1.9) | Electric kettle IDR 6800 (USD 0.4) | Not applicable | Not applicable |
Water | 16,000–30,000 (USD 1.0–2.0) | 16,000–30,000 (USD 1.0–2.0) | IDR 170,000–500,000 (USD 10.9–32.3) | IDR 60,000–200,000 USD (3.9–12.9) |
Total | IDR 45,200–59,200 (USD 2.9–3.9) | IDR 22,800–36,800 (USD 1.4–2.4) | IDR 170,000–500,000 (USD 10.9–32.3.0) | IDR 60,000–200,000 USD (3.9–12.9) |
Factors | Count |
---|
Clear color/appearance | 22 |
Collective experience | 4 |
No smell | 21 |
Taste good | 12 |
Trust in institution | 4 |
Total | 63 |
| The statements, opinions and data contained in all publications are solely those of the individual author(s) and contributor(s) and not of MDPI and/or the editor(s). MDPI and/or the editor(s) disclaim responsibility for any injury to people or property resulting from any ideas, methods, instructions or products referred to in the content. |
Share and Cite
Zikrina, M.N.; Kazama, S.; Sawangjang, B.; Takizawa, S. Filling Discrepancies between Consumer Perception and Actual Piped Water Quality to Promote the Potable Use of the Municipal Water Supply in Indonesia. Sustainability 2024 , 16 , 7082. https://doi.org/10.3390/su16167082
Zikrina MN, Kazama S, Sawangjang B, Takizawa S. Filling Discrepancies between Consumer Perception and Actual Piped Water Quality to Promote the Potable Use of the Municipal Water Supply in Indonesia. Sustainability . 2024; 16(16):7082. https://doi.org/10.3390/su16167082
Zikrina, Masayu Nadiya, Shinobu Kazama, Benyapa Sawangjang, and Satoshi Takizawa. 2024. "Filling Discrepancies between Consumer Perception and Actual Piped Water Quality to Promote the Potable Use of the Municipal Water Supply in Indonesia" Sustainability 16, no. 16: 7082. https://doi.org/10.3390/su16167082
Article Metrics
Article access statistics, further information, mdpi initiatives, follow mdpi.
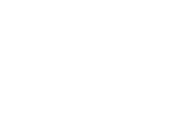
Subscribe to receive issue release notifications and newsletters from MDPI journals
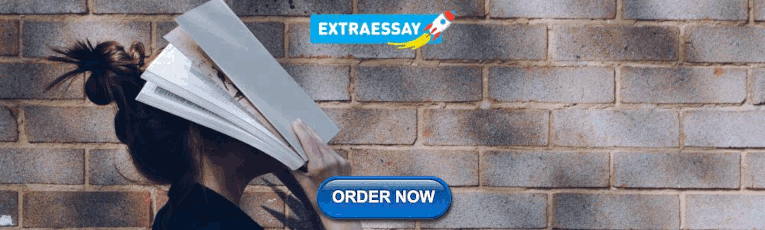
IMAGES
COMMENTS
ANSWER: (a) B. null and alternative. (b) B. They are complements. Explanation: (a) The null hypothesis H0 is a statistical hypothesis that contains a statement of equality, such as ≤ , = , or ≥. The alternative hypothesis Ha is the complement of the null hypothesis. It is a statement that must be true if H0 is false and it contains a statement of strict inequality, such as > , ≠ , or <.
If the null hypothesis is true, then a P-value (or probability value) of a hypothesis test is the probability of obtaining a sample statistic with a value as extreme or more extreme than the one determined from the sample data. There are three types of hypothesis tests: 1. left -tailed test. 2.right tailed test.
A hypothesis is a statement that something is true. A hypothesis test involves two (competing) hypotheses: The null hypothesis, denoted H 0 H 0, is the hypothesis to be tested. This is the "default" assumption. The alternative hypothesis, denoted H A H A is the alternative to the null.
Section 7.1 - Introduction to Hypothesis Testing. How do you want to study today? Flashcards. Review terms and definitions. Learn. Focus your studying with a path ... amelia-e-m. Terms in this set (20) hypothesis test. a process that uses sample statistics to test a claim about the value of a population parameter. sample statistic. a numerical ...
As stated earlier in the chapter, the critical values for a two-tailed z test at a = .05 are z * = ±1.96. This will be the criteria we use to test our hypothesis. We can now draw out our distribution, as shown in Figure 7.4, so we can visualize the rejection region and make sure it makes sense. Figure 7.4.
Question: Section 7.1 : Introduction to HypothesisTesting1. State the claim mathematically. Thenwrite the null and alternative hypothesis. Determine whether thehypothesis test is left-tailed, right-tailed, ortwo-tailed. a. A research hospital claims that more than3.7% of the population suffers from high blood pressure. Use p, therepresent the ...
Introduction to Hypothesis Testing. Section 7.1 Objectives. • State a null hypothesis and an alternative hypothesis. • Identify type I and type II errors and interpret the. level of significance. • Determine whether to use a one-tailed or two-tailed. statistical test and find a p-value. • Make and interpret a decision based on the ...
Figure 7.1.1 7.1. 1 You can use a hypothesis test to decide if a dog breeder's claim that every Dalmatian has 35 spots is statistically sound. (Credit: Robert Neff) The scientific method, briefly, states that only by following a careful and specific process can some assertion be included in the accepted body of knowledge.
This chapter lays out the basic logic and process of hypothesis testing. We will perform z tests, which use the z score formula from Chapter 6 and data from a sample mean to make an inference about a population.. Logic and Purpose of Hypothesis Testing. A hypothesis is a prediction that is tested in a research study. The statistician R. A. Fisher explained the concept of hypothesis testing ...
Jan 7, 2024. This chapter lays out the basic logic and process of hypothesis testing. We will perform z-tests, which use the z-score formula from chapter 6 and data from a sample mean to make an inference about a ….
7.1 Introduction to Hypothesis Testing 7.1.1 Null and Alternative Hypotheses A statistical hypothesis test is a procedure for deciding between two competing claims, or hypotheses, about the values of the parameters of one or more populations, such as their means. A hypothesis test
8.2 FOUR STEPS TO HYPOTHESIS TESTING The goal of hypothesis testing is to determine the likelihood that a population parameter, such as the mean, is likely to be true. In this section, we describe the four steps of hypothesis testing that were briefly introduced in Section 8.1: Step 1: State the hypotheses. Step 2: Set the criteria for a decision.
left-tailed test (p. 354) Type of hypothesis test that occurs if the alternative hypothesis H∨a contains the less-than inequality symbol (<). (Since I don't have Quizlet+, I can't insert the image of the actual normal curve; ergo, I pasted (some of) the information.)H₀: μ ≥ kH∨a: μ < k(P is the area to the left of the standardized ...
rtion(p) or population mean (μ).A hypothesis test is a standard procedure for testing a. Inc. 17Hypotheses come in pairsThere are always at least two. In the admissions example, the two hypotheses are essentially: H 0: That the acceptance rate is 0.70 (null) e)Null and Alternative HypothesesThe null hypothesis, or H0, is the startin.
The difference is statistically significant. Sales increased after sales training. You are more likely to reject the null is α = .10 because the region of rejection is larger (10% of the sampling distribution described by the null instead of 5%). ξ = .02, p > .05. Answers for all 'Test Yourself' questions from the book to check your ...
Hypothesis. This page titled 8.1.1: Introduction to Hypothesis Testing Part 1 is shared under a CC BY 4.0 license and was authored, remixed, and/or curated by OpenStax via source content that was edited to the style and standards of the LibreTexts platform. The actual test begins by considering two hypotheses.
A statistician will make a decision about these claims. This process is called " hypothesis testing ". A hypothesis test involves collecting data from a sample and evaluating the data. Then, the statistician makes a decision as to whether or not there is sufficient evidence, based upon analyses of the data, to reject the null hypothesis.
A statistical analysis was performed using R (v. 4.3) to compare the water quality data and the respondents' answers. The Kruskal-Wallis test was used for an analysis of the differences among more than two sample groups. The Chi-squared test was used to examine associations between categorical data.